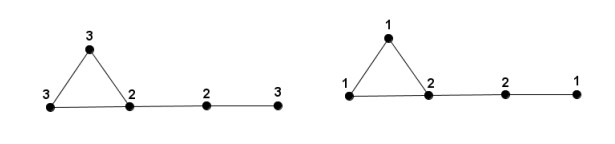
The improper circulation of blood flow inside the retinal vessel is the primary source of most of the optical disorders including partial vision loss and blindness. Accurate blood vessel segmentation of the retinal image is utilized for biometric identification, computer-assisted laser surgical procedure, automatic screening, and diagnosis of ophthalmologic diseases like Diabetic retinopathy, Age-related macular degeneration, Hypertensive retinopathy, and so on. Proper identification of retinal blood vessels at its early stage assists medical experts to take expedient treatment procedures which could mitigate potential vision loss. This paper presents an efficient retinal blood vessel segmentation approach where a 4-D feature vector is constructed by the outcome of Bendlet transform, which can capture directional information much more efficiently than the traditional wavelets. Afterward, a bunch of ensemble classifiers is applied to find out the best possible result of whether a pixel falls inside a vessel or non-vessel segment. The detailed and comprehensive experiments operated on two benchmark and publicly available retinal color image databases (DRIVE and STARE) prove the effectiveness of the proposed approach where the average accuracy for vessel segmentation accomplished approximately 95%. Furthermore, in comparison with other promising works on the aforementioned databases demonstrates the enhanced performance and robustness of the proposed method.
Citation: Rafsanjany Kushol, Md. Hasanul Kabir, M. Abdullah-Al-Wadud, Md Saiful Islam. Retinal blood vessel segmentation from fundus image using an efficient multiscale directional representation technique Bendlets[J]. Mathematical Biosciences and Engineering, 2020, 17(6): 7751-7771. doi: 10.3934/mbe.2020394
[1] | Hua Zhong, Zijian Feng, Zifan Wang, Yougui Wang . Revisiting the monetary transmission mechanism via banking from the perspective of credit creation. National Accounting Review, 2024, 6(1): 116-147. doi: 10.3934/NAR.2024006 |
[2] | Guillermo Peña . VAT experience as an influence on banking crises. National Accounting Review, 2020, 2(1): 53-65. doi: 10.3934/NAR.2020003 |
[3] | Joana Cobbinah, Tan Zhongming, Albert Henry Ntarmah . Banking competition and stability: evidence from West Africa. National Accounting Review, 2020, 2(3): 263-284. doi: 10.3934/NAR.2020015 |
[4] | Van Hoa Tran, Quang Thao Pham . Growth and poverty reduction in Vietnam: A strategic policy modelling study. National Accounting Review, 2024, 6(3): 449-464. doi: 10.3934/NAR.2024020 |
[5] | Shengyin Ouyang, Ziqing Du, Meiling Cai . Statistical measurement of the impact of monetary policy on price levels. National Accounting Review, 2020, 2(2): 188-203. doi: 10.3934/NAR.2020011 |
[6] | Miguel Á. Martínez-García, Ángeles Cámara . How the 2008 financial crisis affected the Spanish economy due to household income. National Accounting Review, 2024, 6(4): 465-479. doi: 10.3934/NAR.2024021 |
[7] | Giorgio Colacchio, Anna Serena Vergori . GDP growth rate, tourism expansion and labor market dynamics: Applied research focused on the Italian economy. National Accounting Review, 2022, 4(3): 310-328. doi: 10.3934/NAR.2022018 |
[8] | Jawad Saleemi . COVID-19 and liquidity risk, exploring the relationship dynamics between liquidity cost and stock market returns. National Accounting Review, 2021, 3(2): 218-236. doi: 10.3934/NAR.2021011 |
[9] | Mahinur Begum Mimi, Md. Ahasan Ul Haque, Md. Golam Kibria . Does human capital investment influence unemployment rate in Bangladesh: a fresh analysis. National Accounting Review, 2022, 4(3): 273-286. doi: 10.3934/NAR.2022016 |
[10] | Serhii Shvets . Public investment as a growth driver for a commodity-exporting economy: Sizing up the fiscal-monetary involvement. National Accounting Review, 2024, 6(1): 95-115. doi: 10.3934/NAR.2024005 |
The improper circulation of blood flow inside the retinal vessel is the primary source of most of the optical disorders including partial vision loss and blindness. Accurate blood vessel segmentation of the retinal image is utilized for biometric identification, computer-assisted laser surgical procedure, automatic screening, and diagnosis of ophthalmologic diseases like Diabetic retinopathy, Age-related macular degeneration, Hypertensive retinopathy, and so on. Proper identification of retinal blood vessels at its early stage assists medical experts to take expedient treatment procedures which could mitigate potential vision loss. This paper presents an efficient retinal blood vessel segmentation approach where a 4-D feature vector is constructed by the outcome of Bendlet transform, which can capture directional information much more efficiently than the traditional wavelets. Afterward, a bunch of ensemble classifiers is applied to find out the best possible result of whether a pixel falls inside a vessel or non-vessel segment. The detailed and comprehensive experiments operated on two benchmark and publicly available retinal color image databases (DRIVE and STARE) prove the effectiveness of the proposed approach where the average accuracy for vessel segmentation accomplished approximately 95%. Furthermore, in comparison with other promising works on the aforementioned databases demonstrates the enhanced performance and robustness of the proposed method.
A topological index is a mathematical measure that can be computed from chemical structures and can be depicted as simple graphs. They play an important role in the study of QSPR and QSAR. In theoretical chemistry, molecular structure descriptors (also called topological indices) are used for modeling physico-chemical, pharmacological, toxicological, biological and other properties of chemical compounds. Chemoinformatics is an active area of research where quantitative pattern behavior and structure-property relations detect biological processes and other properties [].
The chemical graph theory is a branch of graph theory that is concerned with analyses of all consequences of connectivity in a chemical graph. A structural formula of a chemical compound can be represented by a molecular graph which is used to characterize a molecule by depicting the atoms as the vertices of the graph and the molecular bonds as the edges. In chemistry, molecular topological indices, also known as topological indices, are utilized. Wiener [2] was the first to demonstrate that the Wiener index is strongly connected to the boiling points of alkanes. Feng et al. [3] provided a necessary criterion for a graph to be 'l-connected' in 2017 using the well-known Wiener index and Harary index. Su et al. [4] discussed the sufficient condition used with the forgotten topological index.
To predict physico-chemical properties and bioactivity of chemical compounds in QSPR/QSAR investigation, topological indices are employed. Zagreb indices [5,6] are among the most effective for identifying physical attributes and chemical processes of chemical compounds. Gutman and Trinajstic [7] considered the first Zagreb index M1(G) and the second Zagreb index M2(G) for the first time in 1972. Ghobadi and Ghorbaninejad [8] developed accurate formulae for the Zagreb and Hyper-Zagreb indices of some molecular graphs in 2018. They defined the Forgotten topological index or super F-index, a novel distance-based Zagreb index. Estrada et al. [9] developed the Atom-Bond Connectivity (ABC) index, while Vukicevic and Furtula [10] introduced the geometric-arithmetic index. Gutman [11] proposed a novel graph-based topological index, the Sombor index. The index was initially used in chemistry, but it quickly piqued the curiosity of mathematicians [12]. Randic index is one of the oldest, most often used and most successful topological indices in QSPR and QSAR. Bollabas and Erdos [13] later proposed the generalized Randic index. The Harmonic index is a version of the Randic index, which was initially presented by Fajtlowicz [14].
The physico-chemical properties and biological activities of a compound are crucial in the development of pharmaceutical drugs. Many researchers are working on the QSPR analysis of various chemical compounds [15,16], since it is an economically efficient approach to test a compound instead of testing the compounds in a wet lab. Gupta, Singh and Madan [17] proposed a unique graph invariant for predicting biological and physical features using the eccentric distance sum index (EDS). This topological index has a wide range of possible applications in the structure activity/property interactions of molecules, and it has a high discriminating power for both biological activities and physical characteristics. Hua, Xu and Shu [18] obtained a strong lower bound on the EDS index of n-vertex cacti. Yu, Feng and Ili'c [19] investigated the lower and upper bounds of the EDS index in terms of other graph invariants such as the Wiener index, the degree distance index, the eccentric connectivity index and so on.
Chemical qualities, physical properties, pharmacological properties and biological properties of medications are all important in medical research for drug creation. Topological indices can be used to identify these features [20]. Recently, researchers have started researching topological indices and COVID-19 medications. In addition, the following studies are notable in the investigation of medications repurposed against SARS-CoV-2 [21]. Kirmani et al. [22] established QSPR models with linear regression between physico-chemical properties of potential antiviral drugs and some topological indices for various antiviral drugs used in the treatment of COVID-19 patients. Shirkol et al. [23] investigated the predictive power of degree-distance and distance-based topological indices through QSPR analysis. Similarly, Lučić et al. [24] performed QSPR analysis using novel distance-related indices.
Deutsch and Klavžar [25] defined the M-Polynomial in 2015. Using the edge partition technique, M− Polynomial is employed to perform degree based computations. It is crucial in calculating the exact expressions of several degree-based topological indices. The Neighborhood M− polynomial (NM− Polynomial) was introduced by Mondal et al. [26]. It performs the same functions as the M− polynomial for neighborhood degree sum-based indices. It is used to make computations related to neighborhood degree sum-based indices easier. These polynomials are determined by the sum of neighboring vertex degrees. The RNM− polynomial is used to evaluate several forms of reduced neighborhood indices [27].
In this paper, we have considered some antiviral drugs that are used for the treatment of COVID−19 patients, namely, Arbidol, Chloroquine, Hydroxy-Chloroquine, Lopinavir, Remdesivir, Ritonavir, Thalidomide and Theaflavin. We have introduced a new type of vertex degree called Diameter Eccentricity Based vertex degree and employed it to introduce a new polynomial called Dε-polynomial. We use the newly introduced polynomial to derive our proposed topological indices. We further check the applicability of our indices by deriving the Dε-polynomials for the eight afore-mentioned COVID-19 drugs. We perform Quantitative Structure-Property Relationship (QSPR) analysis by arriving at the best fit curvilinear and multilinear regression models based on our topological descriptors for 8 physico-chemical properties of the COVID-19 drugs. We also perform Quantitative Structure-Activity Relationship (QSAR) analysis and identify the best fit multilinear regression model to predict the IC50 values of the eight COVID-19 drugs.
Let G be a simple, finite and connected graph with vertex set as V(G) and edge set as E(G). Let dp denote the degree of a vertex p in G and εp the eccentricity of the vertex p. Let Δ(G) denote the maximum degree and δ(G) denote minimum degree among the vertices of G, respectively.
Definition 1.1. [28] The eccentricity index for a graph G is defined as
ε(G)=∑pq∈E[εp+εq] | (1.1) |
where εp denotes the eccentricity of the vertex p.
Definition 1.2. [29] The Ediz eccentric connectivity index EEc(G) of G is defined as
EEc(G)=∑v∈V(G)S(v)ε(v) | (1.2) |
where S(v) is the sum of degrees of all vertices adjacent to vertex v and ε(v) is the eccentricity of the vertex v.
The Revan vertex degree of p in G is defined as rp=Δ(G)+δ(G)−dp. Based on the Revan vertex degree, Revan indices were introduced by Kulli [30,31]. He has studied oxide networks, honeycomb networks, drugs and antibiotic structures with the help of Revan and Banhatti indices [32]. Inspired by this definition, we have introduced and defined a new type of vertex degree called Diameter Eccentricity Based vertex degree of p in G as
dεp = D(G)+1−εp |
where D(G) denotes the diameter of the graph G.
Figure 1 shows the eccentricity and diameter eccentricity based vertex degrees (dε−values) of the Tadpole Graph T(3,2).
Eccentricity of a vertex is a measure of the centrality of a vertex. Diameter, on the other hand, is a global entity with respect to the graph, that can be considered as a measure of the spread of the graph. Our diameter eccentricity based vertex degree captures both these measures and determines the importance of a vertex with respect to the whole graph..
Based on this new vertex degree definition, we define diameter eccentricity based and hyper diameter eccentricity based indices for any connected graph G by introducing a new polynomial called Dε−polynomial as
Dε(G)=∑i≤j(number of edges pq such that dεp=i,dεq=j)αiβj=∑i≤jrρ(i,j)αiβj=f(α,β) | (1.3) |
where rρ(i,j) denotes the number of edges in the edge partition and α,β are variables. Our Dε− Polynomial is a function of α and β. It is dependent on the diameter eccentricity based vertex degrees of the end vertices of the edges of the graph. The M− polynomial, NM− polynomial and RNM− polynomial can be used to derive degree based, neighborhood degree based and reverse neighborhood degree based indices. Our polynomial is novel and the first of its kind related to eccentricity based indices.
In Table 1, we introduce our new indices as functions of dεp and dεq
Indices | f(dεp,dεq) |
First DRε Index (DR1ε) | dεp+dεq |
Second DRε Index (DR2ε) | dεp∗dεq |
First Hyper DRε Index (HDR1ε) | (dεp+dεq)2 |
Second Hyper DRε Index (HDR2ε) | (dεp∗dεq)2 |
Our diameter eccentricity based indices, that is DR1ε and DR2ε and the Hyper Diameter Eccentricity Based indices, that is HDR1ε and HDR2ε indices can be derived using our Dε-polynomial.
Table 2 shows the formulae for deriving our indices using the Dε-polynomial In Table 2,
∂αf(α,β)=α∂(f(α,β))∂α,∂βf(α,β)=β∂(f(α,β))∂β |
Indices | Derivation from Dε(G) |
DR1ε | (∂α+∂β)(Dε(G))|α=1,β=1 |
DR2ε | (∂α∗∂β)(Dε(G))|α=1,β=1 |
HDR1ε | (∂α+∂β)2(Dε(G))|α=1,β=1 |
HDR2ε | (∂α∗∂β)2(Dε(G))|α=1,β=1 |
The chemical structures (molecular graphs) of the drugs which we have considered in this paper are given in Figures 2 to 5.
We use the method of edge partitions and the newly introduced Dε-polynomial to compute our proposed indices. Edge partitioning is the process of grouping (partitioning) edges based on the appropriate vertex degrees of the end vertices of the edges of a graph. By "appropriate", we refer to the type of vertex degrees used to compute the topological indices. For example, in order to partition the edges for computing our proposed indices, we make use of the diameter eccentricity based vertex degrees of the end vertices of the edges.
In Tables 3 to 10, we present the distance eccentricity based edge partitions for the eight COVID-19 drugs. Here, rρ(p,q) is the number of edges in the edge partition (dεp,dεq) in a graph G. From Table 3 we observe that the edges of Arbidol can be partitioned into 8 partitions based on the distance eccentricity based vertex degrees of the end vertices of the edges of the molecular graph of Arbidol. From Table 4 we observe that the edges of Chloroquine can be partitioned into 7 partitions based on the distance eccentricity based vertex degrees of the end vertices of the edges of the molecular graph of Chloroquine. From Table 5 we observe that the edges of Hydroxy-Chloroquine can be partitioned into 7 partitions based on the distance eccentricity based vertex degrees of the end vertices of the edges of the molecular graph of Hydroxy-Chloroquine. From Table 6 we observe that the edges of Lopinavir can be partitioned into 9 partitions based on the distance eccentricity based vertex degrees of the end vertices of the edges of the molecular graph of Lopinavir. From Table 7 we observe that the edges of Remdesivir can be partitioned into 11 partitions based on the distance eccentricity based vertex degrees of the end vertices of the edges of the molecular graph of Remdesivir. From Table 8 we observe that the edges of Ritonavir can be partitioned into 13 partitions based on the distance eccentricity based vertex degrees of the end vertices of the edges of the molecular graph of Ritonavir. From Table 9 we observe that the edges of Thalidomide can be partitioned into 7 partitions based on the distance eccentricity based vertex degrees of the end vertices of the edges of the molecular graph of Thalidomide. From Table 10 we observe that the edges of Theaflavin can be partitioned into 10 partitions based on the distance eccentricity based vertex degrees of the end vertices of the edges of the molecular graph of Theaflavin.
(dεp,dεq): | (1,2) | (2,3) | (3,3) | (3,4) | (4,5) | (5,5) | (5,6) | (6,7) |
rρ(p,q): | 4 | 6 | 1 | 6 | 5 | 1 | 5 | 3 |
(dεp,dεq): | (1,2) | (2,3) | (3,4) | (4,5) | (5,6) | (6,7) | (7,7) |
rρ(p,q): | 3 | 4 | 5 | 4 | 3 | 3 | 1 |
(dεp,dεq): | (1,2) | (2,3) | (3,4) | (4,5) | (5,6) | (6,7) | (7,8) |
rρ(p,q): | 2 | 4 | 6 | 4 | 3 | 2 | 3 |
(dεp,dεq): | (1,2) | (2,3) | (3,4) | (4,5) | (5,6) | (6,7) | (7,8) | (8,9) | (9,10) |
rρ(p,q): | 5 | 6 | 8 | 7 | 7 | 6 | 4 | 3 | 3 |
(dεp,dεq): | (1,2) | (2,3) | (3,3) | (3,4) | (4,5) | (5,6) | (6,6) | (6,7) | (7,8) | (8,9) | (9,10) |
rρ(p,q): | 5 | 4 | 1 | 4 | 5 | 7 | 1 | 7 | 4 | 4 | 2 |
(dεp,dεq): | (1,1) | (1,2) | (2,3) | (3,3) | (3,4) | (4,5) | (5,6) | (6,7) | (7,8) | (8,9) | (9,10) | (10,11) | (11,12) |
rρ(p,q): | 1 | 4 | 3 | 1 | 5 | 5 | 5 | 6 | 6 | 7 | 4 | 4 | 2 |
(dεp,dεq): | (1,1) | (1,2) | (2,3) | (3,3) | (3,4) | (4,5) | (5,5) |
rρ(p,q): | 1 | 3 | 4 | 1 | 7 | 4 | 1 |
(dεp,dεq): | (1,2) | (2,3) | (3,4) | (4,5) | (5,6) | (6,6) | (6,7) | (7,7) | (7,8) | (8,8) |
rρ(p,q): | 8 | 4 | 6 | 7 | 8 | 1 | 7 | 1 | 3 | 1 |
In this section, we compute Dε-polynomial of the molecular graphs of the mentioned COVID-19 drugs and obtain our proposed topological indices values.
Theorem 3.1. Let A be the molecular graph of Arbidol. Then, we have,
Dε(A)=4α1β2+6α2β3+α3β3+6α3β4+5α4β5+α5β5+5α5β6+3α6β7. |
Proof. Let A be the molecular graph of Arbidol represented in Figure 2. It has 29 vertices and 31 edges. Consider
Dε(A)=∑i≤j(rρ(i,j))αiβj=rρ(1,2)α1β2+rρ(2,3)α2β3+rρ(3,3)α3β3+rρ(3,4)α3β4+rρ(4,5)α4β5+rρ(5,5)α5β5+rρ(5,6)α5β6+rρ(6,7)α6β7. |
Using the edge partitions given in Table 3, it is clear that rρ(1,2)=4, rρ(2,3)=6, rρ(3,3)=1, rρ(3,4)=6, rρ(4,5)=5, rρ(5,5)=1, rρ(5,6)=5 and rρ(6,7)=3.
Applying these values in Dε(A), we get the Dε-polynomial for Arbidol as follows:
Dε(A)=4α1β2+6α2β3+α3β3+6α3β4+5α4β5+α5β5+5α5β6+3α6β7. |
Proposition 3.2. Let A be the molecular graph of Arbidol. Then, we have,
1) DR1ε(A)=239.
2) DR2ε(A)=546.
3) HDR1ε(A)=2133.
4) HDR2ε(A)=13594.
Proof. Using the derivation formula given in Table 2 and the Dε-polynomial for Arbidol from Theorem 3.1, we have
DR1ε(A)=(∂α+∂β)(Dε(A))|α=β=1=(∂αDε(A)+∂βDε(A))|α=β=1=(∂α(f(α,β))+∂β(f(α,β)))|α=β=1=((α∂(4α1β2+6α2β3+α3β3+6α3β4+5α4β5+α5β5+5α5β6+3α6β7)∂α)+(β∂(4α1β2+6α2β3+α3β3+6α3β4+5α4β5+α5β5+5α5β6+3α6β7)∂β))|α=β=1=(12α1β2+30α2β3+6α3β3+42α3β4+10α4β5+α5β5+55α5β6+39α6β7)|α=β=1=239. |
Similarly, the other three indices can be obtained as follows:
DR2ε(A)=(∂α∗∂β)(Dε(A))|α=β=1=(∂α∗∂β)(f(α,β))|α=β=1=(8α1β2+36α2β3+9α3β3+72α3β4+120α4β5+25α5β5+150α5β6+126α6β7)|α=β=1=546. |
HDR1ε(A)=(∂α+∂β)2(Dε(A))|α=β=1=(∂α+∂β)2(f(α,β))|α=β=1=(36α1β2+150α2β3+36α3β3+294α3β4+405α4β5+100α5β5+605α5β6+507α6β7)|α=β=1=2133. |
HDR2ε(A)=(∂α∗∂β)2(Dε(A))|α=β=1=(∂α∗∂β)2(f(α,β))|α=β=1=(16α1β2+216α2β3+81α3β3+864α3β4+2000α4β5+625α5β5+4500α5β6+5292α6β7)|α=β=1=13594. |
Theorem 3.3. Let C be the molecular graph of Chloroquine. Then, we have,
Dε(C)=3α1β2+4α2β3+5α3β4+4α4β5+3α5β6+3α6β7+α7β7. |
Proof. Let C be the molecular graph of Chloroquine represented in Figure 2. It has 21 vertices and 23 edges. Consider
Dε(C)=∑i≤j(rρ(i,j))αiβj=rρ(1,2)α1β2+rρ(2,3)α2β3+rρ(3,4)α3β4+rρ(4,5)α4β5+rρ(5,6)α5β6+rρ(6,7)α6β7+rρ(7,7)α7β7. |
Using the edge partitions given in Table 4, it is clear that rρ(1,2)=3, rρ(2,3)=4, rρ(3,4)=5, rρ(4,5)=4, rρ(5,6)=3, rρ(6,7)=3 and rρ(7,7)=1.
Applying the values in Dε(C), we get the Dε-polynomial for Chloroquine as follows:
Dε(C)=3α1β2+4α2β3+5α3β4+4α4β5+3α5β6+3α6β7+α7β7. |
Proposition 3.4. Let C be the molecular graph of Chloroquine. Then, we have,
1) DR1ε(C)=186.
2) DR2ε(C)=435.
3) HDR1ε(C)=1762.
4) HDR2ε(C)=12869.
Proof is similar to that of proposition 3.2.
Theorem 3.5. Let HC be the molecular graph of Hydroxy-Chloroquine. Then, we have,
Dε(HC)=2α1β2+4α2β3+6α3β4+4α4β5+3α5β6+2α6β7+3α7β8. |
Proof. Let HC be the molecular graph of Hydroxy-Chloroquine represented in Figure 3. It has 22 vertices and 24 edges. Consider
Dε(HC)=∑i≤j(rρ(i,j))αiβj=rρ(1,2)α1β2+rρ(2,3)α2β3+rρ(3,4)α3β4+rρ(4,5)α4β5+rρ(5,6)α5β6+rρ(6,7)α6β7+rρ(7,8)α7β8. |
Using the edge partitions given in Table 5, it is clear that rρ(1,2)=2, rρ(2,3)=4, rρ(3,4)=6, rρ(4,5)=4, rρ(5,6)=3, rρ(6,7)=2 and rρ(7,8)=3.
Applying the values in Dε(HC), we get the Dε-polynomial for Hydroxy-Chloroquine as follows:
Dε(HC)=2α1β2+4α2β3+6α3β4+4α4β5+3α5β6+2α6β7+3α7β8. |
Proposition 3.6. Let HC be the molecular graph of Hydroxy-Chloroquine. Then, we have,
1) DR1ε(HC)=208.
2) DR2ε(HC)=522.
3) HDR1ε(HC)=2092.
4) HDR2ε(HC)=18252.
Proof is similar to that of proposition 3.2.
Theorem 3.7. Let L be the molecular graph of Lopinavir. Then, we have,
Dε(L)=5α1β2+6α2β3+8α3β4+7α4β5+7α5β6+6α6β7+4α7β8. |
Proof. Let L be the molecular graph of Lopinavir represented in Figure 3. It has 46 vertices and 49 edges. Consider
Dε(L)=∑i≤j(rρ(i,j))αiβj=rρ(1,2)α1β2+rρ(2,3)α2β3+rρ(3,4)α3β4+rρ(4,5)α4β5+rρ(5,6)α5β6+rρ(6,7)α6β7+rρ(7,8)α7β8. |
Using the edge partitions given in Table 6, it is clear that rρ(1,2)=5, rρ(2,3)=6, rρ(3,4)=8, rρ(4,5)=7, rρ(5,6)=7, rρ(6,7)=6 and rρ(7,8)=4.
Applying the values in Dε(L), we get the Dε-polynomial for Lopinavir as follows:
Dε(L)=5α1β2+6α2β3+8α3β4+7α4β5+7α5β6+6α6β7+4α7β8. |
Proposition 3.8. Let L be the molecular graph of Lopinavir. Then, we have,
1) DR1ε(L)=487.
2) DR2ε(L)=1454.
3) HDR1ε(L)=5865.
4) HDR2ε(L)=73468.
Proof is similar to that of proposition 3.2.
Theorem 3.9. Let R be the molecular graph of Remdesivir. Then, we have,
Dε(R)=5α1β2+4α2β3+1α3β3+4α3β4+5α4β5+7α5β6+1α6β6+7α6β7+4α7β8+4α8β9+2α9β10. |
Proof. Let R be the molecular graph of Remdesivir represented in Figure 4. It has 41 vertices and 44 edges. Consider
Dε(R)=∑i≤j(rρ(i,j))αiβj=rρ(1,2)α1β2+rρ(2,3)α2β3+rρ(3,3)α3β3+rρ(3,4)α3β4+rρ(4,5)α4β5+rρ(5,6)α5β6+rρ(6,6)α6β6+rρ(6,7)α6β7+rρ(7,8)α7β8+rρ(8,9)α8β9+rρ(9,19)α9β10. |
Using the edge partitions given in Table 7, it is clear that rρ(1,2)=5, rρ(2,3)=4, rρ(3,3)=1, rρ(3,4)=4, rρ(4,5)=5, rρ(5,6)=7, rρ(6,6)=1, rρ(6,7)=7, rρ(7,8)=4, rρ(8,9)=4 and rρ(9,10)=2.
Applying the values in Dε(R), we get the Dε-polynomial for Remdesivir as follows:
Dε(R)=5α1β2+4α2β3+1α3β3+4α3β4+5α4β5+7α5β6+1α6β6+7α6β7+4α7β8+4α8β9+2α9β10. |
Proposition 3.10. Let R be the molecular graph of Remdesivir. Then, we have,
1) DR1ε(R)=460.
2) DR2ε(R)=1423.
3) HDR1ε(R)=5734.
4) HDR2ε(R)=72245.
Proof is similar to that of proposition 3.2.
Theorem 3.11. Let Ri be the molecular graph of Ritonavir. Then, we have,
Dε(Ri)=1α1β1+4α1β2+3α2β3+1α3β3+5α3β4+5α4β5+5α5β6+6α6β7+6α7β8+7α8β9+4α9β10+4α10β11+2α11β12. |
Proof. Let Ri be the molecular graph of Ritonavir represented in Figure 4. It has 50 vertices and 53 edges. Consider
Dε(Ri)=∑i≤j(rρ(i,j))αiβj=rρ(1,1)α1β1+rρ(1,2)α1β2+rρ(2,3)α2β3+rρ(3,3)α3β3+rρ(3,4)α3β4+rρ(4,5)α4β5+rρ(5,6)α5β6+rρ(6,7)α6β7+rρ(7,8)α7β8+rρ(8,9)α8β9+rρ(9,10)α9β10+rρ(10,11)α10β11+rρ(11,12)α11β12. |
Using the edge partitions given in Table 8, it is clear that rρ(1,1)=1, rρ(1,2)=4, rρ(2,3)=3, rρ(3,3)=1, rρ(3,4)=5, rρ(4,5)=5, rρ(5,6)=5, rρ(6,7)=6, rρ(7,8)=6, rρ(8,9)=7, rρ(9,10)=4, rρ(10,11)=4, rρ11,12)=2.
Applying the values in Dε(Ri), we get the Dε-polynomial for Ritonavir as follows:
Dε(Ri)=1α1β1+4α1β2+3α2β3+1α3β3+5α3β4+5α4β5+5α5β6+6α6β7+6α7β8+7α8β9+4α9β10+4α10β11+2α11β12. |
Proposition 3.12. Let Ri be the molecular graph of Ritonavir. Then, we have,
1) DR1ε(Ri)=629.
2) DR2ε(Ri)=2502.
3) HDR1ε(Ri)=10059.
4) HDR2ε(Ri)=188762.
Proof is similar to that of proposition 3.2.
Theorem 3.13. Let T be the molecular graph of Thalidomide. Then, we have,
Dε(T)=1α1β1+3α1β2+4α2β3+1α3β3+7α3β4+4α4β5+1α5β5. |
Proof. Let T be the molecular graph of Thalidomide represented in Figure 5. It has 19 vertices and 21 edges. Consider
Dε(T)=∑i≤j(rρ(i,j))αiβj=rρ(1,1)α1β1+rρ(1,2)α1β2+rρ(2,3)α2β3+rρ(3,3)α3β3+rρ(3,4)α3β4+rρ(4,5)α4β5+rρ(5,5)α5β5. |
Using the edge partitions given in Table 9, it is clear that rρ(1,1)=1, rρ(1,2)=3, rρ(2,3)=4, rρ(3,3)=1, rρ(3,4)=7, rρ(4,5)=4 and rρ(5,5)=1.
Applying the values in Dε(T), we get the Dε-polynomial for Thalidomide as follows:
Dε(T)=1α1β1+3α1β2+4α2β3+1α3β3+7α3β4+4α4β5+1α5β5. |
Proposition 3.14. Let T be the molecular graph of Thalidomide. Then, we have,
1) DR1ε(T)=132.
2) DR2ε(T)=229.
3) HDR1ε(T)=934.
4) HDR2ε(T)=3471.
Proof is similar to that of proposition 3.2.
Theorem 3.15. Let Th be the molecular graph of Theaflavin. Then, we have,
Dε(Th)=8α1β2+4α2β3+6α3β4+7α4β5+8α5β6+1α6β6+7α6β7+1α7β7+3α7β8+1α8β8. |
Proof. Let Th be the molecular graph of Theaflavin represented in Figure 5. It has 41 vertices and 46 edges. Consider
Dε(Th)=∑i≤j(rρ(i,j))αiβj=rρ(1,2)α1β2+rρ(2,3)α2β3+rρ(3,4)α3β4+rρ(4,5)α4β5+rρ(5,6)α5β6+rρ(6,6)α6β6+rρ(6,7)α6β7+rρ(7,7)α7β7+rρ(7,8)α7β8+rρ(8,8)α8β8. |
Using the edge partitions given in Table 10, it is clear that rρ(1,2)=8, rρ(2,3)=4, rρ(3,4)=6, rρ(4,5)=7, rρ(5,6)=8, rρ(6,6)=1, rρ(6,7)=7, rρ(7,7)=1, rρ(7,8)=3 and rρ(8,8)=1.
Applying the values in Dε(Th), we get the Dε-polynomial for Theaflavin as follows:
Dε(Th)=8α1β2+4α2β3+6α3β4+7α4β5+8α5β6+1α6β6+7α6β7+1α7β7+3α7β8+1α8β8. |
Proposition 3.16. Let Th be the molecular graph of Theaflavin. Then, we have,
1) DR1ε(Th)=415.
2) DR2ε(Th)=1103.
3) HDR1ε(Th)=3944.
4) HDR2ε(Th)=40589.
Proof is similar to that of proposition 3.2.
In this section, we analyze the topological indices given in Table 1 for the following physico-chemical properties of the 8 COVID-19 antiviral drugs: Boiling point (BP), Enthalpy (E), Flash point (FP), Molar refraction (MR), Polar Surface Area (PSA), Polarizability (P), Surface Tension (T), Molar Volume (MV).
Experimental values of physico-chemical properties of the antiviral drugs presented in Table 11 were obtained from Kirmani et al. [22]. In Table 12 we have presented the values of the proposed indices calculated in Section 3.
Drugs | BP | E | FP | MR | PSA | P | T | MV |
Arbidol | 591.8 | 91.5 | 311.7 | 121.9 | 80 | 48.3 | 45.3 | 347.3 |
Chloroquine | 460.6 | 72.1 | 232.3 | 97.4 | 28 | 38.6 | 44 | 287.9 |
Hydroxy-Chloroquine | 516.7 | 83 | 266.3 | 99 | 48 | 39.2 | 49.8 | 285.4 |
Lopinavir | 924.2 | 140.8 | 512.7 | 179.2 | 120 | 71 | 49.5 | 540.5 |
Remdesivir | - | - | - | 149.5 | 213 | 59.3 | 62.3 | 409 |
Ritonavir | 947 | 144.4 | 526.6 | 198.9 | 202 | 78.9 | 53.7 | 581.7 |
Thalidomide | 487.8 | 79.4 | 248.8 | 65.2 | 87 | 25.9 | 71.6 | 161 |
Theaflavin | 1003.9 | 153.5 | 336.5 | 137.3 | 218 | 54.4 | 138.6 | 301 |
Drugs | DR1ε | DR2ε | HDR1ε | HDR2ε |
Arbidol | 239 | 546 | 2133 | 13594 |
Chloroquine | 186 | 435 | 1762 | 12869 |
Hydroxy-Chloroquine | 208 | 522 | 2092 | 18252 |
Lopinavir | 487 | 1454 | 5865 | 73468 |
Remdesivir | 460 | 1423 | 5734 | 72245 |
Ritonavir | 629 | 2502 | 10059 | 188762 |
Thalidomide | 132 | 229 | 934 | 3471 |
Theaflavin | 415 | 1103 | 3944 | 40589 |
We performed curvilinear regression analysis for our proposed indices aganist the physico-chemical properties.
The general form of the curvilinear regression model is
P=[n∑i=1~αi(TI)i]+˜γ | (4.1) |
where P is the physico-chemical property (dependent variable), ˜γ is the regression model constant, and ~αi are the coefficients corresponding to topological descriptors (TI), i=1,2,…,n.
We performed a comparative analysis of the linear, quadratic, cubic and fourth order curvilinear regression models. The models for which R2≥0.8 (as per International Academy of Mathematical Chemistry Guidelines) were considered for further analysis. We observed the following:
● From Table 13, it can be seen that the R2 values for the following properties: boiling point (BP), enthalpy (E), flash point (FP), molar refraction (MR), polarizability (P) and molar volume (MV), are higher for the cubic models and they can be used to predict these properties. However, polar surface area (PSA) and surface tension (T) could not be predicted using the cubic models.
Property | Linear | R2 | RMSE | Quadratic | R2 | RMSE | Cubic | R2 | RMSE |
BP | DR1ε | 0.8273 | 110.1231 | DR1ε | 0.9112 | 88.2792 | DR1ε | 0.9494 | 76.9536 |
E | DR1ε | 0.8170 | 16.5276 | DR1ε | 0.8965 | 13.8998 | DR1ε | 0.9413 | 12.0807 |
FP | DR1ε | 0.8851 | 45.5738 | HDR2ε | 0.9200 | 42.5140 | HDR2ε | 0.9625 | 33.5907 |
MR | DR1ε | 0.9356 | 12.2073 | DR1ε | 0.9377 | 13.1566 | DR1ε | 0.9551 | 12.4885 |
P | DR1ε | 0.9363 | 4.8138 | DR1ε | 0.9382 | 5.1930 | DR1ε | 0.9554 | 4.9334 |
MV | HDR1ε | 0.8187 | 64.5810 | HDR1ε | 0.8423 | 65.9762 | HDR1ε | 0.8431 | 73.5649 |
● All the eight physico-chemical properties could be predicted using fourth order regression models.
In this subsection, we present the fourth order regression models for the eight physico-chemical properties. Based on the R2 and RMSE values we obtained the following best fit fourth order regression models for the physico-chemical properties.
BP=2e−07(DR1ε)4−0.0003(DR1ε)3+0.1557(DR1ε)2−30.235(DR1ε)+2399.2 | (4.2) |
E=3e−08(DR1ε)4−4E−05(DR1ε)3+0.0211(DR1ε)2−4.1382(DR1ε)+342.92 | (4.3) |
FP=−6e−13(HDR1ε)4+9E−09(HDR1ε)3−4E−05(HDR1ε)2+0.0913(HDR1ε)+186.46 | (4.4) |
MR=−3e−08(DR1ε)4+4E−05(DR1ε)3−0.0212(DR1ε)2+4.7925(DR1ε)−284.37 | (4.5) |
PSA=1e−07(DR1ε)4−0.0002(DR1ε)3+0.1117(DR1ε)2−22.298(DR1ε)+1544.4 | (4.6) |
P=−1e−08(DR1ε)4+2E−05(DR1ε)3−0.0084(DR1ε)2+1.8909(DR1ε)−112.02 | (4.7) |
T=2e−17(HDR2ε)4−7E−12(HDR2ε)3+5E−07(HDR2ε)2−0.0099(HDR2ε)+100.2 | (4.8) |
MV=−2e−07(DR1ε)4+0.0003(DR1ε)3−0.1535(DR1ε)2+32.073(DR1ε)−2037.6 | (4.9) |
From Table 13, it is clear that the mentioned indices can be used to predict all the physico-chemical properties of the COVID-19 drugs. From our analysis of the proposed indices, we observe that
● DR1ε index can be used to predict the Boiling Point (BP), Enthalpy of Vaporization (E), Molar Refraction (MR), Polar Surface Area (PSA), Polarizability (P) and Molar Volume (MV) with the corresponding R2 values as 0.9995, 0.9978, 0.9776, 0.9583, 0.9777 and 0.9634, respectively.
● HDR1ε index can be used to predict the Flash Point (FP) with the corresponding R2 value as 0.9704.
● HDR2ε index can be used to predict the Surface Tension (T) with the corresponding R2 value as 0.9996.
Figure 6 to Figure 8 show the plots of the fourth order regression equations for Boiling Point (BP), Enthalpy of Vaporization (E), Molar Refraction (MR), Polar Surface Area (PSA), Polarizability (P) and Molar Volume (MV) with respect to DR1ε index. Figure 9 shows the plots for Flash Point (FP) and Surface Tension (T) with respect to HDR1ε and HDR2ε indices, respectively.
In this section, we compare the predictive capability of our proposed indices against the ediz eccentric connectivity index. Table 15 shows the comparison of fourth order regression models of ediz eccentric connectivity index (EEcI) aganist the fourth order regression models based on our proposed indices.
Property | Equation No. | Best Predictors | R2 | RMSE |
BP | (4.2) | DR1ε | 0.9995 | 9.7565 |
E | (4.3) | DR1ε | 0.9978 | 2.8616 |
FP | (4.4) | HDR1ε | 0.9704 | 36.6018 |
MR | (4.5) | DR1ε | 0.9776 | 10.1867 |
PSA | (4.6) | DR1ε | 0.9583 | 23.9260 |
P | (4.7) | DR1ε | 0.9777 | 4.0273 |
T | (4.8) | HDR2ε | 0.9996 | 1.0035 |
MV | (4.9) | DR1ε | 0.9634 | 41.0211 |
Property | Ediz | R2 | RMSE | Proposed Indices | R2 | RMSE |
BP | EEcI | 0.5516 | 280.5630 | DR1ε | 0.9995 | 9.7565 |
E | EEcI | 0.5696 | 40.0779 | DR1ε | 0.9978 | 2.8616 |
FP | EEcI | 0.3588 | 170.2130 | HDR1ε | 0.9704 | 36.6018 |
MR | EEcI | 0.2212 | 60.0279 | DR1ε | 0.9776 | 10.1867 |
PSA | EEcI | 0.8423 | 46.5065 | DR1ε | 0.9583 | 23.9260 |
P | EEcI | 0.2227 | 23.7770 | DR1ε | 0.9777 | 4.0273 |
T | EEcI | 0.9607 | 9.5006 | HDR2ε | 0.9996 | 1.0035 |
MV | EEcI | 0.1849 | 193.6289 | DR1ε | 0.9634 | 41.0211 |
From Table 15, based on R2 and RMSE values it can be observed that the fourth order regression models based on our proposed indices provide the best fits for the eight physico-chemical properties.
In this section we present the Multilinear regression analysis performed for the eight physico-chemical properties aganist our proposed indices. The general form of Multilinear regression is
P=~α1(TI)1++~α2(TI)2+...++~αn(TI)n+˜γ | (5.1) |
where P is the physico-chemical property (dependent variable), ˜γ is the regression model constant and ~αi are the regression coefficients for the topological descriptors.
In this subsection we present the Multilinear regression models and the analysis for the eight physico-chemical properties.
BP=0.0165(DR1ε)−0.5221(DR2ε)−0.0187(HDR1ε)+5.3124(HDR2ε)+213.299 | (5.2) |
E=0,0029(DR1ε)−0.0869(DR2ε)−0.0036(HDR1ε)+0.8214(HDR2ε)+41.6226 | (5.3) |
FP=−0.0005(DR1ε)+0.1072(DR2ε)−0.0122(HDR1ε)−0.5611(HDR2ε)+196.499 | (5.4) |
MR=−0.0050(DR1ε)+0,0144(DR2ε)+0.0087(HDR1ε)−0.21(HDR2ε)+11.05149 | (5.5) |
PSA=0.0095(DR1ε)−0.2453(DR2ε)−0.0133(HDR1ε)+1.9034(HDR2ε)+16.6273 | (5.6) |
P=−0.0020(DR1ε)+0.0412(DR2ε)+0.0034(HDR1ε)−0.0828(HDR2ε)+4.4940 | (5.7) |
T=0.0080(DR1ε)−0.2286(DR2ε)−0.0089(HDR1ε)+1.6116(HDR2ε)+49.2483 | (5.8) |
MV=−0.0213(DR1ε)+0.5142(DR2ε0.0213)+0.0312(HDR1ε)2−2.2024(HDR2ε)+39.4661 | (5.9) |
From Table 16, it can be observed that except for Polar Surface Area with R2<0.8, all other properties are well predicted using the multilinear regression models.
Property | Equation No. | R2 | RMSE |
BP | (5.2) | 0.9975 | 20.8648 |
E | (5.3) | 0.9950 | 4.3123 |
FP | (5.4) | 0.9495 | 47.7738 |
MR | (5.5) | 0.9705 | 11.6830 |
PSA | (5.6) | 0.7812 | 54.7782 |
P | (5.7) | 0.9708 | 4.6056 |
T | (5.8) | 0.8872 | 16.1008 |
MV | (5.9) | 0.9137 | 63.0190 |
The most generally used and useful indicator of a drug's effectiveness is its half-maximal inhibitory concentration IC50. It is a quantitative metric that shows how much of a certain inhibitory substance (such as a medicine) is required in vitro to inhibit a specific biological process or biological component by 50 percent [33].
We fitted the Multilinear regression model given in Eq (5.10) to predict the IC50 values for the COVID-19 drugs.
IC50=0.0001(DR1ε)−0.0081(DR2ε)+0.0005(HDR1ε)+0.0966(HDR2ε)−4.2010 | (5.10) |
The predicted IC50 values for the seven COVID-19 drugs, for which the experimental values are available, are presented in Table 17. It can be seen that the proposed multilinear regression model can be used to predict IC50 values.
Drug Name | IC50 μM | Predicted IC50 using (5.10) | Absolute Residual |
Arbidol | 3.54 | 3.342368 | 0.197632 |
Chloroquine | 1.38 | 1.088041 | 0.291959 |
Hydroxy-Chloroquine | 0.72 | 0.993671 | 0.27367 |
Lopinavir | 5.25 | 3.880872 | 1.369128 |
Remdesivir | 0.987 | 2.187685 | 1.20069 |
Ritonavir | 8.63 | 8.627918 | 0.002082 |
Theaflavin | 8.44 | 8.826445 | 0.38645 |
In this article, we proposed and computed the Diameter Eccentricity Based and Hyper Diameter Eccentricity Based topological descriptors for eight COVID-19 drugs, namely, Arbidol, Chloroquine, Hydroxy-Chloroquine, Lopinavir, Remdesivir, Ritonavir, Thalidomide and Theaflavin through Dε-Polynomial. The indices were computed using our newly introduced Dε- polynomial.
From the QSPR analysis using the curvilinear regression models, we conclude that the best fit fourth order regression models based on our proposed index DR1ε predict Boiling Point, Enthalpy of Vaporization, Molar Refraction, Polar Surface Area, Polarizability and Molar Volume. The fourth order regression models based on DHR1ε and DHR2ε can be used to predict Flash Point and Surface Tension, respectively.
We computed the Ediz Eccentric Connectivity index for COVID-19 drugs. Based on R2 and RMSE values, it can be observed that the fourth order regression models based on our proposed indices provide good predictions for the eight physico-chemical properties of the 8 COVID-19 drugs compared to the fourth order regression models based on the Ediz Eccentric Connectivity index.
Our proposed multilinear regression models provide good predictions for the physico-chemical properties expect the Polar Surface Area.
Multilinear regression model is best suited for predicting the 50% inhibitory concentration (IC50) values with R2 value 0.947.
The proposed indices can be used in designing new drugs to combat COVID-19 and other diseases.
The authors would like to thank the editor and reviewers for their helpful remarks in improving this manuscript. In addition, the authors would like to express their gratitude to the management of Vellore Institute of Technology, Chennai, India, for their encouragement and assistance in carrying out this research. This research work is financially supported by Vellore Institute of Technology, Chennai, India.
The authors declare that they have not used artificial intelligence tools in the creation of this article.
The authors declare there is no conflict of interest.
[1] | A. M. Joussen, T. W. Gardner, B. Kirchhof, S. J. Ryan, Retinal vascular disease, Springer, 2007. |
[2] |
S. Chaudhuri, S. Chatterjee, N. Katz, M. Nelson, M. Goldbaum, Detection of blood vessels in retinal images using two-dimensional matched filters, IEEE Trans. Med. Imaging, 8 (1989), 263-269. doi: 10.1109/42.34715
![]() |
[3] | M. E. Martínez-Pérez, A. D. Hughes, A. V. Stanton, S. A. Thom, A. A. Bharath, K. H. Parker, Retinal blood vessel segmentation by means of scale-space analysis and region growing, in Inter-national Conference on Medical Image Computing and Computer-Assisted Intervention, Springer, (1999), 90-97. |
[4] |
A. M. Mendonca, A. Campilho, Segmentation of retinal blood vessels by combining the detection of centerlines and morphological reconstruction, IEEE Trans. Med. Imaging, 25 (2006), 1200-1213. doi: 10.1109/TMI.2006.879955
![]() |
[5] | N. Brancati, M. Frucci, D. Gragnaniello, D. Riccio, Retinal vessels segmentation based on a con-volutional neural network, in Iberoamerican Congress on Pattern Recognition, Springer, (2017), 119-126. |
[6] | E. J. Candes, D. L. Donoho, Curvelets: A surprisingly effective nonadaptive representationfor objects with edges, Technical report, Stanford Uni Ca Dept of Statistics, 2000. |
[7] |
M. N. Do, M. Vetterli, The contourlet transform: an efficient directional multiresolution image representation, IEEE Trans. Image Proc., 14 (2005), 2091-2106. doi: 10.1109/TIP.2005.859376
![]() |
[8] |
W.-Q. Lim, The discrete shearlet transform: a new directional transform and compactly supported shearlet frames., IEEE Trans. Image Proc., 19 (2010), 1166-1180. doi: 10.1109/TIP.2010.2041410
![]() |
[9] |
C. Lessig, P. Petersen, M. Schäfer, Bendlets: A second-order shearlet transform with bent elements, Appl. Comput. Harmonic Anal., 46 (2019), 384-399. doi: 10.1016/j.acha.2017.06.002
![]() |
[10] |
A. Hoover, V. Kouznetsova, M. Goldbaum, Locating blood vessels in retinal images by piecewise threshold probing of a matched filter response, IEEE Trans. Med. Imaging, 19 (2000), 203-210. doi: 10.1109/42.845178
![]() |
[11] |
B. Zhang, L. Zhang, L. Zhang, F. Karray, Retinal vessel extraction by matched filter with first-order derivative of gaussian, Comput. Biol. Med., 40 (2010), 438-445. doi: 10.1016/j.compbiomed.2010.02.008
![]() |
[12] | J. Odstrcilik, R. Kolar, A. Budai, J. Hornegger, J. Jan, J. Gazarek, et al., Retinal vessel segmentation by improved matched filtering: evaluation on a new high-resolution fundus image database, IET Image Proc., 7 (2013), 373-383. |
[13] |
T. Chakraborti, D. K. Jha, A. S. Chowdhury, X. Jiang, A self-adaptive matched filter for retinal blood vessel detection, Mach. Vision Appl., 26 (2015), 55-68. doi: 10.1007/s00138-014-0636-z
![]() |
[14] |
M. Vlachos, E. Dermatas, Multi-scale retinal vessel segmentation using line tracking, Comput. Med Imaging Graphics, 34 (2010), 213-227. doi: 10.1016/j.compmedimag.2009.09.006
![]() |
[15] |
U. T. Nguyen, A. Bhuiyan, L. A. Park, K. Ramamohanarao, An effective retinal blood vessel segmentation method using multi-scale line detection, Pattern Recognit., 46 (2013), 703-715. doi: 10.1016/j.patcog.2012.08.009
![]() |
[16] |
Y. Hou, Automatic segmentation of retinal blood vessels based on improved multiscale line detection, J. Comput. Sci. Eng.ng, 8 (2014), 119-128. doi: 10.5626/JCSE.2014.8.2.119
![]() |
[17] |
K. Yue, B. Zou, Z. Chen, Q. Liu, Improved multi-scale line detection method for retinal blood vessel segmentation, IET Image Proc., 12 (2018), 1450-1457. doi: 10.1049/iet-ipr.2017.1071
![]() |
[18] |
M. S. Miri, A. Mahloojifar, Retinal image analysis using curvelet transform and multistructure elements morphology by reconstruction, IEEE Trans. Biomed. Eng., 58 (2011), 1183-1192. doi: 10.1109/TBME.2010.2097599
![]() |
[19] | M. M. Fraz, S. A. Barman, P. Remagnino, A. Hoppe, A. Basit, B. Uyyanonvara, et al., An approach to localize the retinal blood vessels using bit planes and centerline detection, Comput. Methods Programs Biomed., 108 (2012), 600-616. |
[20] |
E. Imani, M. Javidi, H. R. Pourreza, Improvement of retinal blood vessel detection using morphological component analysis, Comput. Methods Programs Biomed., 118 (2015), 263-279. doi: 10.1016/j.cmpb.2015.01.004
![]() |
[21] | J. Gao, G. Chen, W. Lin, An effective retinal blood vessel segmentation by using automatic random walks based on centerline extraction, BioMed Res. Int., 2020 (2020). |
[22] |
E. Ricci, R. Perfetti, Retinal blood vessel segmentation using line operators and support vector classification, IEEE Trans. Med. Imaging, 26 (2007), 1357-1365. doi: 10.1109/TMI.2007.898551
![]() |
[23] |
D. Marín, A. Aquino, M. E. Gegúndez-Arias, J. M. Bravo, A new supervised method for blood vessel segmentation in retinal images by using gray-level and moment invariants-based features, IEEE Trans. Med. Imaging, 30 (2011), 146-158. doi: 10.1109/TMI.2010.2064333
![]() |
[24] | Z. Fan, Y. Rong, J. Lu, J. Mo, F. Li, X. Cai, et al., Automated blood vessel segmentation in fundus image based on integral channel features and random forests, 2016 12th World Congress on Intelligent Control and Automation (WCICA), IEEE, 2016. |
[25] |
J. V. Soares, J. J. Leandro, R. M. Cesar, H. F. Jelinek, M. J. Cree, Retinal vessel segmentation using the 2-d gabor wavelet and supervised classification, IEEE Trans. Med. Imaging, 25 (2006), 1214-1222. doi: 10.1109/TMI.2006.879967
![]() |
[26] | L. Xu, S. Luo, A novel method for blood vessel detection from retinal images, Biomed. Eng. online, 9 (2010), 1. |
[27] | M. M. Fraz, P. Remagnino, A. Hoppe, B. Uyyanonvara, A. R. Rudnicka, C. G. Owen, et al., An ensemble classification-based approach applied to retinal blood vessel segmentation, IEEE Trans. Biomed. Eng., 59 (2012), 2538-2548. |
[28] | F. Ghadiri, S. M. Zabihi, H. R. Pourreza, T. Banaee, A novel method for vessel detection using contourlet transform, 2012 National Conference on Communications (NCC), IEEE, 2012. |
[29] | P. Bankhead, C. N. Scholfield, J. G. McGeown, T. M. Curtis, Fast retinal vessel detection and measurement using wavelets and edge location refinement, PloS one, 7 (2012), e32435. |
[30] |
G. Azzopardi, N. Strisciuglio, M. Vento, N. Petkov, Trainable cosfire filters for vessel delineation with application to retinal images, Med. Image Anal., 19 (2015), 46-57. doi: 10.1016/j.media.2014.08.002
![]() |
[31] | F. Levet, M. A. Duval-Poo, E. De Vito, F. Odone, Retinal image analysis with shearlets, in STAG, (2016), 151-156. |
[32] |
B. Khomri, A. Christodoulidis, L. Djerou, M. C. Babahenini, F. Cheriet, Retinal blood vessel segmentation using the elite-guided multi-objective artificial bee colony algorithm, IET Image Proc., 12 (2018), 2163-2171. doi: 10.1049/iet-ipr.2018.5425
![]() |
[33] | M. Z. Alom, M. Hasan, C. Yakopcic, T. M. Taha, V. K. Asari, Recurrent residual convolutional neural network based on u-net (r2u-net) for medical image segmentation, Forthcoming, 2018. |
[34] | S. Y. Shin, S. Lee, I. D. Yun, K. M. Lee, Deep vessel segmentation by learning graphical connectivity, Med. Image Anal., 58 (2019), 101556. |
[35] |
M. Al-Rawi, M. Qutaishat, M. Arrar, An improved matched filter for blood vessel detection of digital retinal images, Comput. Biol. Med., 37 (2007), 262-267. doi: 10.1016/j.compbiomed.2006.03.003
![]() |
[36] | L. Li, M. Verma, Y. Nakashima, H. Nagahara, R. Kawasaki, Iternet: Retinal image segmentation utilizing structural redundancy in vessel networks, in The IEEE Winter Conference on Applications of Computer Vision, (2020), 3656-3665. |
[37] | R. Kushol, M. H. Kabir, M. S. Salekin, A. A. Rahman, Contrast enhancement by top-hat and bottom-hat transform with optimal structuring element: Application to retinal vessel segmentation, in International Conference Image Analysis and Recognition, Springer, (2017), 533-540. |
[38] | R. Kushol, M. N. Raihan, M. S. Salekin, A. A. Rahman, Contrast enhancement of medical x-ray image using morphological operators with optimal structuring element, Forthcoming, 2019. |
[39] | M. Niemeijer, J. Staal, B. van Ginneken, M. Loog, M. D. Abramoff, Comparative study of retinal vessel segmentation methods on a new publicly available database, Proceedings of SPIE. Belling-ham: Society of Photo-Optical Instrumentation Engineers Press, 2004. |
[40] | V. M. Saffarzadeh, A. Osareh, B. Shadgar, Vessel segmentation in retinal images using multi-scale line operator and k-means clustering, J. Med. Signals Sens., 4 (2014), 122. |
Indices | f(dεp,dεq) |
First DRε Index (DR1ε) | dεp+dεq |
Second DRε Index (DR2ε) | dεp∗dεq |
First Hyper DRε Index (HDR1ε) | (dεp+dεq)2 |
Second Hyper DRε Index (HDR2ε) | (dεp∗dεq)2 |
Indices | Derivation from Dε(G) |
DR1ε | (∂α+∂β)(Dε(G))|α=1,β=1 |
DR2ε | (∂α∗∂β)(Dε(G))|α=1,β=1 |
HDR1ε | (∂α+∂β)2(Dε(G))|α=1,β=1 |
HDR2ε | (∂α∗∂β)2(Dε(G))|α=1,β=1 |
(dεp,dεq): | (1,2) | (2,3) | (3,3) | (3,4) | (4,5) | (5,5) | (5,6) | (6,7) |
rρ(p,q): | 4 | 6 | 1 | 6 | 5 | 1 | 5 | 3 |
(dεp,dεq): | (1,2) | (2,3) | (3,4) | (4,5) | (5,6) | (6,7) | (7,7) |
rρ(p,q): | 3 | 4 | 5 | 4 | 3 | 3 | 1 |
(dεp,dεq): | (1,2) | (2,3) | (3,4) | (4,5) | (5,6) | (6,7) | (7,8) |
rρ(p,q): | 2 | 4 | 6 | 4 | 3 | 2 | 3 |
(dεp,dεq): | (1,2) | (2,3) | (3,4) | (4,5) | (5,6) | (6,7) | (7,8) | (8,9) | (9,10) |
rρ(p,q): | 5 | 6 | 8 | 7 | 7 | 6 | 4 | 3 | 3 |
(dεp,dεq): | (1,2) | (2,3) | (3,3) | (3,4) | (4,5) | (5,6) | (6,6) | (6,7) | (7,8) | (8,9) | (9,10) |
rρ(p,q): | 5 | 4 | 1 | 4 | 5 | 7 | 1 | 7 | 4 | 4 | 2 |
(dεp,dεq): | (1,1) | (1,2) | (2,3) | (3,3) | (3,4) | (4,5) | (5,6) | (6,7) | (7,8) | (8,9) | (9,10) | (10,11) | (11,12) |
rρ(p,q): | 1 | 4 | 3 | 1 | 5 | 5 | 5 | 6 | 6 | 7 | 4 | 4 | 2 |
(dεp,dεq): | (1,1) | (1,2) | (2,3) | (3,3) | (3,4) | (4,5) | (5,5) |
rρ(p,q): | 1 | 3 | 4 | 1 | 7 | 4 | 1 |
(dεp,dεq): | (1,2) | (2,3) | (3,4) | (4,5) | (5,6) | (6,6) | (6,7) | (7,7) | (7,8) | (8,8) |
rρ(p,q): | 8 | 4 | 6 | 7 | 8 | 1 | 7 | 1 | 3 | 1 |
Drugs | BP | E | FP | MR | PSA | P | T | MV |
Arbidol | 591.8 | 91.5 | 311.7 | 121.9 | 80 | 48.3 | 45.3 | 347.3 |
Chloroquine | 460.6 | 72.1 | 232.3 | 97.4 | 28 | 38.6 | 44 | 287.9 |
Hydroxy-Chloroquine | 516.7 | 83 | 266.3 | 99 | 48 | 39.2 | 49.8 | 285.4 |
Lopinavir | 924.2 | 140.8 | 512.7 | 179.2 | 120 | 71 | 49.5 | 540.5 |
Remdesivir | - | - | - | 149.5 | 213 | 59.3 | 62.3 | 409 |
Ritonavir | 947 | 144.4 | 526.6 | 198.9 | 202 | 78.9 | 53.7 | 581.7 |
Thalidomide | 487.8 | 79.4 | 248.8 | 65.2 | 87 | 25.9 | 71.6 | 161 |
Theaflavin | 1003.9 | 153.5 | 336.5 | 137.3 | 218 | 54.4 | 138.6 | 301 |
Drugs | DR1ε | DR2ε | HDR1ε | HDR2ε |
Arbidol | 239 | 546 | 2133 | 13594 |
Chloroquine | 186 | 435 | 1762 | 12869 |
Hydroxy-Chloroquine | 208 | 522 | 2092 | 18252 |
Lopinavir | 487 | 1454 | 5865 | 73468 |
Remdesivir | 460 | 1423 | 5734 | 72245 |
Ritonavir | 629 | 2502 | 10059 | 188762 |
Thalidomide | 132 | 229 | 934 | 3471 |
Theaflavin | 415 | 1103 | 3944 | 40589 |
Property | Linear | R2 | RMSE | Quadratic | R2 | RMSE | Cubic | R2 | RMSE |
BP | DR1ε | 0.8273 | 110.1231 | DR1ε | 0.9112 | 88.2792 | DR1ε | 0.9494 | 76.9536 |
E | DR1ε | 0.8170 | 16.5276 | DR1ε | 0.8965 | 13.8998 | DR1ε | 0.9413 | 12.0807 |
FP | DR1ε | 0.8851 | 45.5738 | HDR2ε | 0.9200 | 42.5140 | HDR2ε | 0.9625 | 33.5907 |
MR | DR1ε | 0.9356 | 12.2073 | DR1ε | 0.9377 | 13.1566 | DR1ε | 0.9551 | 12.4885 |
P | DR1ε | 0.9363 | 4.8138 | DR1ε | 0.9382 | 5.1930 | DR1ε | 0.9554 | 4.9334 |
MV | HDR1ε | 0.8187 | 64.5810 | HDR1ε | 0.8423 | 65.9762 | HDR1ε | 0.8431 | 73.5649 |
Property | Equation No. | Best Predictors | R2 | RMSE |
BP | (4.2) | DR1ε | 0.9995 | 9.7565 |
E | (4.3) | DR1ε | 0.9978 | 2.8616 |
FP | (4.4) | HDR1ε | 0.9704 | 36.6018 |
MR | (4.5) | DR1ε | 0.9776 | 10.1867 |
PSA | (4.6) | DR1ε | 0.9583 | 23.9260 |
P | (4.7) | DR1ε | 0.9777 | 4.0273 |
T | (4.8) | HDR2ε | 0.9996 | 1.0035 |
MV | (4.9) | DR1ε | 0.9634 | 41.0211 |
Property | Ediz | R2 | RMSE | Proposed Indices | R2 | RMSE |
BP | EEcI | 0.5516 | 280.5630 | DR1ε | 0.9995 | 9.7565 |
E | EEcI | 0.5696 | 40.0779 | DR1ε | 0.9978 | 2.8616 |
FP | EEcI | 0.3588 | 170.2130 | HDR1ε | 0.9704 | 36.6018 |
MR | EEcI | 0.2212 | 60.0279 | DR1ε | 0.9776 | 10.1867 |
PSA | EEcI | 0.8423 | 46.5065 | DR1ε | 0.9583 | 23.9260 |
P | EEcI | 0.2227 | 23.7770 | DR1ε | 0.9777 | 4.0273 |
T | EEcI | 0.9607 | 9.5006 | HDR2ε | 0.9996 | 1.0035 |
MV | EEcI | 0.1849 | 193.6289 | DR1ε | 0.9634 | 41.0211 |
Property | Equation No. | R2 | RMSE |
BP | (5.2) | 0.9975 | 20.8648 |
E | (5.3) | 0.9950 | 4.3123 |
FP | (5.4) | 0.9495 | 47.7738 |
MR | (5.5) | 0.9705 | 11.6830 |
PSA | (5.6) | 0.7812 | 54.7782 |
P | (5.7) | 0.9708 | 4.6056 |
T | (5.8) | 0.8872 | 16.1008 |
MV | (5.9) | 0.9137 | 63.0190 |
Drug Name | IC50 μM | Predicted IC50 using (5.10) | Absolute Residual |
Arbidol | 3.54 | 3.342368 | 0.197632 |
Chloroquine | 1.38 | 1.088041 | 0.291959 |
Hydroxy-Chloroquine | 0.72 | 0.993671 | 0.27367 |
Lopinavir | 5.25 | 3.880872 | 1.369128 |
Remdesivir | 0.987 | 2.187685 | 1.20069 |
Ritonavir | 8.63 | 8.627918 | 0.002082 |
Theaflavin | 8.44 | 8.826445 | 0.38645 |
Indices | f(dεp,dεq) |
First DRε Index (DR1ε) | dεp+dεq |
Second DRε Index (DR2ε) | dεp∗dεq |
First Hyper DRε Index (HDR1ε) | (dεp+dεq)2 |
Second Hyper DRε Index (HDR2ε) | (dεp∗dεq)2 |
Indices | Derivation from Dε(G) |
DR1ε | (∂α+∂β)(Dε(G))|α=1,β=1 |
DR2ε | (∂α∗∂β)(Dε(G))|α=1,β=1 |
HDR1ε | (∂α+∂β)2(Dε(G))|α=1,β=1 |
HDR2ε | (∂α∗∂β)2(Dε(G))|α=1,β=1 |
(dεp,dεq): | (1,2) | (2,3) | (3,3) | (3,4) | (4,5) | (5,5) | (5,6) | (6,7) |
rρ(p,q): | 4 | 6 | 1 | 6 | 5 | 1 | 5 | 3 |
(dεp,dεq): | (1,2) | (2,3) | (3,4) | (4,5) | (5,6) | (6,7) | (7,7) |
rρ(p,q): | 3 | 4 | 5 | 4 | 3 | 3 | 1 |
(dεp,dεq): | (1,2) | (2,3) | (3,4) | (4,5) | (5,6) | (6,7) | (7,8) |
rρ(p,q): | 2 | 4 | 6 | 4 | 3 | 2 | 3 |
(dεp,dεq): | (1,2) | (2,3) | (3,4) | (4,5) | (5,6) | (6,7) | (7,8) | (8,9) | (9,10) |
rρ(p,q): | 5 | 6 | 8 | 7 | 7 | 6 | 4 | 3 | 3 |
(dεp,dεq): | (1,2) | (2,3) | (3,3) | (3,4) | (4,5) | (5,6) | (6,6) | (6,7) | (7,8) | (8,9) | (9,10) |
rρ(p,q): | 5 | 4 | 1 | 4 | 5 | 7 | 1 | 7 | 4 | 4 | 2 |
(dεp,dεq): | (1,1) | (1,2) | (2,3) | (3,3) | (3,4) | (4,5) | (5,6) | (6,7) | (7,8) | (8,9) | (9,10) | (10,11) | (11,12) |
rρ(p,q): | 1 | 4 | 3 | 1 | 5 | 5 | 5 | 6 | 6 | 7 | 4 | 4 | 2 |
(dεp,dεq): | (1,1) | (1,2) | (2,3) | (3,3) | (3,4) | (4,5) | (5,5) |
rρ(p,q): | 1 | 3 | 4 | 1 | 7 | 4 | 1 |
(dεp,dεq): | (1,2) | (2,3) | (3,4) | (4,5) | (5,6) | (6,6) | (6,7) | (7,7) | (7,8) | (8,8) |
rρ(p,q): | 8 | 4 | 6 | 7 | 8 | 1 | 7 | 1 | 3 | 1 |
Drugs | BP | E | FP | MR | PSA | P | T | MV |
Arbidol | 591.8 | 91.5 | 311.7 | 121.9 | 80 | 48.3 | 45.3 | 347.3 |
Chloroquine | 460.6 | 72.1 | 232.3 | 97.4 | 28 | 38.6 | 44 | 287.9 |
Hydroxy-Chloroquine | 516.7 | 83 | 266.3 | 99 | 48 | 39.2 | 49.8 | 285.4 |
Lopinavir | 924.2 | 140.8 | 512.7 | 179.2 | 120 | 71 | 49.5 | 540.5 |
Remdesivir | - | - | - | 149.5 | 213 | 59.3 | 62.3 | 409 |
Ritonavir | 947 | 144.4 | 526.6 | 198.9 | 202 | 78.9 | 53.7 | 581.7 |
Thalidomide | 487.8 | 79.4 | 248.8 | 65.2 | 87 | 25.9 | 71.6 | 161 |
Theaflavin | 1003.9 | 153.5 | 336.5 | 137.3 | 218 | 54.4 | 138.6 | 301 |
Drugs | DR1ε | DR2ε | HDR1ε | HDR2ε |
Arbidol | 239 | 546 | 2133 | 13594 |
Chloroquine | 186 | 435 | 1762 | 12869 |
Hydroxy-Chloroquine | 208 | 522 | 2092 | 18252 |
Lopinavir | 487 | 1454 | 5865 | 73468 |
Remdesivir | 460 | 1423 | 5734 | 72245 |
Ritonavir | 629 | 2502 | 10059 | 188762 |
Thalidomide | 132 | 229 | 934 | 3471 |
Theaflavin | 415 | 1103 | 3944 | 40589 |
Property | Linear | R2 | RMSE | Quadratic | R2 | RMSE | Cubic | R2 | RMSE |
BP | DR1ε | 0.8273 | 110.1231 | DR1ε | 0.9112 | 88.2792 | DR1ε | 0.9494 | 76.9536 |
E | DR1ε | 0.8170 | 16.5276 | DR1ε | 0.8965 | 13.8998 | DR1ε | 0.9413 | 12.0807 |
FP | DR1ε | 0.8851 | 45.5738 | HDR2ε | 0.9200 | 42.5140 | HDR2ε | 0.9625 | 33.5907 |
MR | DR1ε | 0.9356 | 12.2073 | DR1ε | 0.9377 | 13.1566 | DR1ε | 0.9551 | 12.4885 |
P | DR1ε | 0.9363 | 4.8138 | DR1ε | 0.9382 | 5.1930 | DR1ε | 0.9554 | 4.9334 |
MV | HDR1ε | 0.8187 | 64.5810 | HDR1ε | 0.8423 | 65.9762 | HDR1ε | 0.8431 | 73.5649 |
Property | Equation No. | Best Predictors | R2 | RMSE |
BP | (4.2) | DR1ε | 0.9995 | 9.7565 |
E | (4.3) | DR1ε | 0.9978 | 2.8616 |
FP | (4.4) | HDR1ε | 0.9704 | 36.6018 |
MR | (4.5) | DR1ε | 0.9776 | 10.1867 |
PSA | (4.6) | DR1ε | 0.9583 | 23.9260 |
P | (4.7) | DR1ε | 0.9777 | 4.0273 |
T | (4.8) | HDR2ε | 0.9996 | 1.0035 |
MV | (4.9) | DR1ε | 0.9634 | 41.0211 |
Property | Ediz | R2 | RMSE | Proposed Indices | R2 | RMSE |
BP | EEcI | 0.5516 | 280.5630 | DR1ε | 0.9995 | 9.7565 |
E | EEcI | 0.5696 | 40.0779 | DR1ε | 0.9978 | 2.8616 |
FP | EEcI | 0.3588 | 170.2130 | HDR1ε | 0.9704 | 36.6018 |
MR | EEcI | 0.2212 | 60.0279 | DR1ε | 0.9776 | 10.1867 |
PSA | EEcI | 0.8423 | 46.5065 | DR1ε | 0.9583 | 23.9260 |
P | EEcI | 0.2227 | 23.7770 | DR1ε | 0.9777 | 4.0273 |
T | EEcI | 0.9607 | 9.5006 | HDR2ε | 0.9996 | 1.0035 |
MV | EEcI | 0.1849 | 193.6289 | DR1ε | 0.9634 | 41.0211 |
Property | Equation No. | R2 | RMSE |
BP | (5.2) | 0.9975 | 20.8648 |
E | (5.3) | 0.9950 | 4.3123 |
FP | (5.4) | 0.9495 | 47.7738 |
MR | (5.5) | 0.9705 | 11.6830 |
PSA | (5.6) | 0.7812 | 54.7782 |
P | (5.7) | 0.9708 | 4.6056 |
T | (5.8) | 0.8872 | 16.1008 |
MV | (5.9) | 0.9137 | 63.0190 |
Drug Name | IC50 μM | Predicted IC50 using (5.10) | Absolute Residual |
Arbidol | 3.54 | 3.342368 | 0.197632 |
Chloroquine | 1.38 | 1.088041 | 0.291959 |
Hydroxy-Chloroquine | 0.72 | 0.993671 | 0.27367 |
Lopinavir | 5.25 | 3.880872 | 1.369128 |
Remdesivir | 0.987 | 2.187685 | 1.20069 |
Ritonavir | 8.63 | 8.627918 | 0.002082 |
Theaflavin | 8.44 | 8.826445 | 0.38645 |