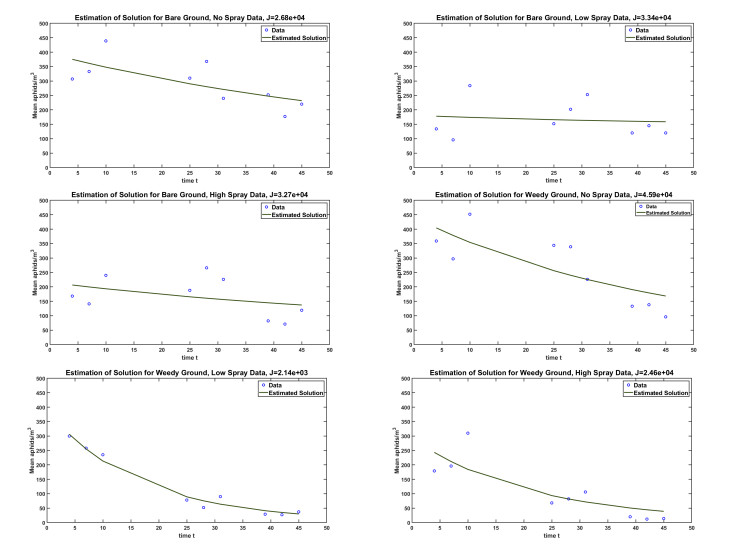
Citation: H. T. Banks, John E. Banks, Jared Catenacci, Michele Joyner, John Stark. Correctly modeling plant-insect-herbivore-pesticide interactions as aggregate data[J]. Mathematical Biosciences and Engineering, 2020, 17(2): 1743-1756. doi: 10.3934/mbe.2020091
[1] | Ya Li, Z. Feng . Dynamics of a plant-herbivore model with toxin-induced functional response. Mathematical Biosciences and Engineering, 2010, 7(1): 149-169. doi: 10.3934/mbe.2010.7.149 |
[2] | Xinru Zhou, Xinmiao Rong, Meng Fan, Josué-Antonio Nescolarde-Selvaa . Stoichiometric modeling of aboveground-belowground interaction of herbaceous plant. Mathematical Biosciences and Engineering, 2019, 16(1): 25-55. doi: 10.3934/mbe.2019002 |
[3] | Alhadi E. Alamir, Gomah E. Nenaah, Mohamed A. Hafiz . Mathematical probit and logistic mortality models of the Khapra beetle fumigated with plant essential oils. Mathematical Biosciences and Engineering, 2015, 12(4): 687-697. doi: 10.3934/mbe.2015.12.687 |
[4] | Jeong-Mi Yoon, Volodymyr Hrynkiv, Lisa Morano, Anh Tuan Nguyen, Sara Wilder, Forrest Mitchell . Mathematical modeling of Glassy-winged sharpshooter population. Mathematical Biosciences and Engineering, 2014, 11(3): 667-677. doi: 10.3934/mbe.2014.11.667 |
[5] | Yang Kuang, Jef Huisman, James J. Elser . Stoichiometric Plant-Herbivore Models and Their Interpretation. Mathematical Biosciences and Engineering, 2004, 1(2): 215-222. doi: 10.3934/mbe.2004.1.215 |
[6] | Guangyu Sui, Meng Fan, Irakli Loladze, Yang Kuang . The dynamics of a stoichiometric plant-herbivore model and its discrete analog. Mathematical Biosciences and Engineering, 2007, 4(1): 29-46. doi: 10.3934/mbe.2007.4.29 |
[7] | Zhilan Feng, Wenzhang Huang, Donald L. DeAngelis . Spatially heterogeneous invasion of toxic plant mediated by herbivory. Mathematical Biosciences and Engineering, 2013, 10(5&6): 1519-1538. doi: 10.3934/mbe.2013.10.1519 |
[8] | Yang Li, Jia Li . Stage-structured discrete-time models for interacting wild and sterile mosquitoes with beverton-holt survivability. Mathematical Biosciences and Engineering, 2019, 16(2): 572-602. doi: 10.3934/mbe.2019028 |
[9] | Sunmi Lee, Chang Yong Han, Minseok Kim, Yun Kang . Optimal control of a discrete-time plant–herbivore/pest model with bistability in fluctuating environments. Mathematical Biosciences and Engineering, 2022, 19(5): 5075-5103. doi: 10.3934/mbe.2022237 |
[10] | Luca Galbusera, Sara Pasquali, Gianni Gilioli . Stability and optimal control for some classes of tritrophic systems. Mathematical Biosciences and Engineering, 2014, 11(2): 257-283. doi: 10.3934/mbe.2014.11.257 |
In the summer of 1999, Banks and Stark [2] conducted a full-factorial design field experiment to explore the potentially combined effects of vegetation diversity and selective pesticide disturbance on aphid populations in a broccoli agroecosystem. They applied three concentration levels of the biorational pesticide imidacloprid to broccoli patches surrounded by either bare ground or weedy vegetation. These efforts by necessity resulted in what is now referred to as agammaegate data [3,Chapter 14], [4,Chapter 5] wherein possibly (almost certainly) different subpopulations are counted in a longitudinal manner over the life of the experiment.
In earlier efforts [1], authors sought to fit several autonomous ordinary differential equation (ODE) models to each of the six data sets (two margin types each with three pesticide spray levels),
1. Bare margin, no spray;
2. Bare margin, low spray (15 g ai/ha);
3. Bare margin, high spray (30 g ai/ha);
4. Weedy margin, no spray;
5. Weedy margin, low spray (15 g ai/ha); and
6. Weedy margin, high spray (30 g ai/ha),
in an attempt to understand the influence of natural enemies or other margin-based factors on pest suppression separately from and in interaction with that of the insecticide. In these early attempts, the authors ignored the agammaegate aspects of the data and used ordinary least squares techniques to fit the models. In addition, motivated by both varying environmental factors and changes in dynamics due to pesticide application, the authors also investigated the same set of models with piecewise constant and piecewise linear time-varying coefficients in the corresponding non-autonomous ODEs. Parameterizing these time-varying coefficients introduced additional degrees of freedom, but in general the non-autonomous systems provided substantially better fits to the data. Specifically, chi-squared tests revealed that increasing degrees of freedom to move from constant to time-varying coefficients yielded statistically significant improvement in model fit. Details of the data sets, the methods used in data collection, and the model fit are given in [1,5,6,2] and summarized in a readily available technical report [7].
An obvious goal of that earlier paper was to contribute to the literature on plant–insect herbivore–pesticide interactions which would help raise questions for future investigations supported by experiments. Although this is still a secondary goal of this paper, our main objective in this paper is to discuss the similarities and differences between previous efforts in which data was simply treated as longitudinal data (i.e., the true agammaegate nature of the data was ignored) to current, more accurate efforts of treating the data correctly as agammaegate data. Therefore, in this paper, we correctly acknowledge the agammaegate nature of the data and apply the more recently developed methodology for treating such data sets in the context of the Prohorov Metric Framework (PMF) [3,4,8,9,10,11,12,13,14]. Hence, we present a combined mathematical/statistical modelling methodology that has broad applicability to other problems in biology as well as engineering and general sciences in which agammaegate data has been collected and must be treated accordingly. We compare the results of this methodology to the standard, although erroneous, treatment of agammaegate data as simply longitudinal data.
While the authors of [1] considered 7 different ordinary differential equation models of population dynamics, we focus on only one of these models to compare results when treating the data as truly agammaegate data versus ignoring the agammaegate nature of the data. This model was called Model 3 in the earlier reference [1]. The model involves a single state variable N(t), which denotes the aphid population density: (mean aphids)/m3. The model has the general form
dN(t)dt=B(N(t),t)N(t)−D(N(t),t)N(t), (MM) |
where dNdt (equivalently ˙N) denotes the time derivative of the state variable N(t); B, a (potentially time- and/or state-dependent and/or random variable) birth rate; and D, a (potentially time- and/or state-dependent and/or random variable) death rate.
The model used in our current study (omitting the implicit time dependence of N and ˙N) assumes mortality due to pesticides and/or predation is the driving force behind aphid population dynamics and is the standard exponential model for population dynamics, allowing for simultaneous exponential birth and death. Here B>0 and D>0 in (MM) above for the deterministic time dependent coefficient models. In the case of random differential equation (RDE) models we take B−D=A for a random variable and we have an RDE model
˙N=BN−DN=AN. | (2.1) |
Note that we let A=B−D denote a combined birth/death rate in this model. Because aphids reproduce by parthenogenesis (i.e., asexually) in the field, exponential growth is a reasonable assumption for their population dynamics, especially when densities are below the carrying capacity. Densities during the field study considered here were consistently below carrying capacity densities observed in previous years.
For more information on models of the form (MM), see Boyce and DiPrima [15]. Note that in each case, a solution to the ordinary differential equation is uniquely determined by imposing a single initial condition, denoted N0=N(t0), where t0=0 is the initial time considered in our studies.
In [1], authors used an ordinary least squares cost functional to measure the fit to the data when assuming a constant parameter A=a in Model (2.1) and then piecewise constant and piecewise linear coefficients a(t). In all six data sets, except the weedy margin, low spray data set, the piecewise constant coefficient provided a statistically better fit with a confidence level of over 0.77 (see [7] Figures 2–7, Tables 1–6). Furthermore, authors in [1] found little perceptible difference in statistical significance between adding piecewise constant versus piecewise linear coefficients. Although the time-varying estimated rates were within an order of magnitude of the values derived in an (in vitro) laboratory study (unpublished) by Stark, the authors in [1] failed to recognize the agammaegate nature of the data to which they were fitting the model.
Data Set | Cost Function - Ignore Aggregate Nature | Cost Function - Incorporate Aggregate Nature |
Bare Ground, No Spray | 1.57e+04 | 1.04e+04 |
Bare Ground, Low Spray | 2.51e+04 | 1.60e+04 |
Bare Ground, High Spray | 1.34e+04 | 1.11e+04 |
Weedy Ground, No Spray | 1.19e+04 | 1.16e+04 |
Weedy Ground, Low Spray | 1.83e+03 | 1.87e+03 |
Weedy Ground, High Spray | 1.57e+04 | 4.84e+03 |
The modeling problem which should have been considered given the nature of the data, and that which is considered in this paper, is referred to as an individual dynamics / agammaegate data problem or a Type I problem in [4] in which we have a mathematical model for individual dynamics but only have agammaegate data, not individual data. In this case, we have data from randomly selected plants in multiple plots which were averaged to reduce inter-block variability (agammaegate data). Therefore, we should not be interested in a single parameter value a for our model (or piecewise constant parameters); instead, the underlying goal should be to determine a probability measure which describes the distribution of the parameter across all members of the population.
In [1], the authors estimated the parameter a or a(t) in a deterministic, least squares setting. In other words, it was assumed the experimental data {ˆzi}, i=1,...,n corresponds to individual observations of the state variable, i.e., zi, i=1,...,n are realizations of a random variable Zi satisfying the statistical model
Zi=N(ti;a0)+Ei,i=1,...,n |
(when assuming constant variance) [1,4]. Here N(t;a0) is the solution to (2.1) with some "truth" parameter a0 and error Ei. However, in the collection of the data in this study, the experimental observations were collected from multiple plants and averaged across different plots. Therefore, we do not have longitudinal measurements of an independent state variable but expected values across the population which we will refer to as {ˆui}, i=1,...,n. If the parameters do not vary to a large extent across the population, then these "mean" value approximations determined in [1] may suffice. However, in many cases, these "mean" value approximations, in which the agammaegate nature of the data is ignored, may lead to inaccurate parameter estimates and an inaccurate description of the dynamics within the population. Therefore, in this paper, we want to adequately consider the agammaegate nature of the data.
To incorporate the potential population-dependent variability in the model parameter, we now assume the parameter A in Eq (2.1) is a random variable with probability distribution P which belongs to a probability space P(Q) that may be infinite dimensional. As in [16], we define the set P(Q) of all probability distributions on the admissible parameter space Q and seek to minimize the objective function
J(P)=n∑i=1|u(ti;P)−ˆui|2=n∑i=1((u(ti;P))2−2u(ti;P)ˆui+(ˆui)2) | (3.1) |
over P∈P(Q) where the expected value is defined by
u(t;P)=E[N(t)|P]=∫QN(t;a)dP(a). | (3.2) |
If it is possible to predict the expected form of the probability distribution P a priori, then we could use the parametric form of the distribution in (3.2) and estimate the parameters describing the distribution by minimizing (3.1) using a least-squares methodology (see [17]). However, in general, it is typically not plausible to predict the expected form of the distribution a priori. In this case, P(Q) may be chosen as the space of all probability distributions. Thus we need non-parametric approaches that do not require any assumptions with respect to the form of the probability distribution. In [19], two approaches were compared in which P(Q) was approximated by finite dimensional sets PM(Q), yielding computationally feasible schemes. Using the Prohorov metric [18,23] and the theoretical framework in [16], if Q is compact, then we can define a family of finite dimensional approximation problems in which we are guaranteed convergence (in the Prohorov metric) of a minimizer of
J(PM)=n∑i=1|u(ti;PM)−ˆui|2=n∑i=1((u(ti;PM))2−2u(ti;PM)ˆui+(ˆui)2) | (3.3) |
over the set PM to a minimizer for the original problem, i.e., the minimization of (3.1).
One approach is to assume a discrete collection of admissible parameter values QM={qMk}Mk=0 and approximate P(Q) with
PM(Q)={P∈P(Q)|P′=∑kpMkδqMk,∑kpMk=1} | (3.4) |
where δqMk is the delta function with an atom at qMk. In this case, the population density is approximated by
u(t;{pMk})=M∑k=0N(t;aMk)pMk |
where N(t;aMk) is the subpopulation density from (2.1) with birth/death rate aMk. This is the delta function approximation method, denoted DEL(M), where M is the number of nodes or elements used in this approximation.
An alternative approach, and the one used in this paper, is to assume P is a continuous probability distribution on the parameter and use piecewise linear splines to approximate the distribution. In this methodology, we still assume a discrete collection of admissible parameter values QM={qMk}Mk=0 but now approximate P(Q) with
PM(Q)={P∈P(Q)|P′=∑kbMklMk(q),∑kbMk∫QlMk(q)dq=1} | (3.5) |
where the piecewise linear splines are represented by lMk. Using this approximation method, the population density from (3.2) is approximated by
u(t;{bMk})=M∑k=0bMk∫QN(t;a)lMk(a)da |
where pMk(a)=bMklMk(a) is the probability density for individuals in subpopulation k. This spline based method is denoted by SPL(M, N) where M is the number of basis elements used to approximate the birth/death rate probability distribution and N is the number of quadrature nodes used to approximate the integral.
In both approaches, the least squares problem in (3.3) reduces to a constrained quadratic programming problem [21,22] in which we minimize
F(p)=pTHp+2pTf+c | (3.6) |
over PM(Q), where p is the vector containing pMk, 0≤k≤M or bMk, 0≤k≤M when using DEL(M) or SPL(M, N), respectively. We let H be the matrix with entries given by
Hkm=∑iN(ti;aMk)N(ti;aMm); |
f is the vector with entries
fk=−∑iˆuiN(ti;aMk) |
and
c=∑i(ˆui)2. |
When using DEL(M), the constraint
M∑k=1pMk=1 |
is imposed; whereas when using SPL(M, N), we include the constraint
M∑k=1bMk∫QlMk(q)dq=1. |
In [20], Banks and Davis provided a computational framework for the quantification of uncertainty associated with estimating probability distributions or densities for a Type 1, individual dynamics/agammaegate data problem. In their trials, they computed confidence bands for both the cumulative distribution and probability density functions for the estimated probability distribution for a growth rate parameter in the Sinko-Streifer model. They were able to show that as the number of nodes, M, increases, the confidence bands appear to converge nicely until M becomes too large, i.e., the problem becomes over-parameterized. They showed that the condition number for the matrix H in the quadratic programming problem (3.6) increases as M increases and causes the inverse problem to become ill-posed.
Prior to estimating a probability distribution for the growth/death rate A in (2.1) using the experimental data, we performed trials using simulated data with a known probability distribution, assuming a small number of data points (as given in this study). Even for small values of M, the condition number was fairly large, providing poor fits to the true density. To improve the fit to the simulated data, we first incorporated regularization with a small regularization parameter and then determined that M = 15 nodes provided a good fit to both the cumulative distribution and probability density functions for the known probability distribution as well as a good fit to the solution.
In this section, we use the techniques outlined in Section 3 to estimate a probability distribution for random variable parameter A in Eq (2.1), accounting for the fact that we have agammaegate data. We do this in two ways; we first assumed that the distribution did not change with respect to time. This is comparable to the case in which a was assumed to be constant in [1]; however, we do not estimate a single parameter value, but assume potential population-dependent variability in the model parameter since we have agammaegate data and estimate a probability distribution for A. Therefore, in our initial efforts, we assume there is variability across the population, but there is no change in variability with respect to time. Hence, we estimate one probability distribution for A for the entire time frame for each of the six data sets using the initial population N(0) estimated in [1] and equally spaced nodes in the constrained quadratic programming problem given in Eq (3.6). The model fit to the data can be found in Figure 1 for all six data sets with the respective probability distributions and cumulative distribution functions given in Figure 2. Notice that in most cases, the fit is fairly poor and although the distributions behave similar to a gaussian distribution, the interval of definition for this distribution is small. We refer the reader to [7] for full results and comparisons; however, in almost all cases, the results when considering the agammaegate nature of the data with no time dependence (this paper) is almost identical to when the agammaegate nature of the data is ignored [1].
We note that the estimated probability density function for A varies depending on the choice of QM, i.e., it changes depending on the nodes which are chosen in the interval as well as the interval over which the nodes are chosen. This makes sense as one could miss crucial variability in the probability density function if nodes are not chosen for values in which there is a noticeable change. The choice of QM and the placement of the nodes is a future research question to be addressed. We note that in our initial trials, we chose QM as indicated by the standard error estimates for a given in [1]. However, using this large interval with only M = 15 nodes, the estimates indicated point distributions at values close to the estimated constant values estimated in [1]. This could explain the similarity in model fits and cost estimates between the two methodologies. Therefore, in this case, when ignoring time-dependence, the "mean" value approximation may suffice.
In our next efforts, we assumed time-dependence variability. In this situation, we split the time interval into three subintervals over which we estimated subinterval population distributions. In other words, we assumed on each subinterval, there was potentially a different probability distribution for Ai, i=1, 2, 3. We considered three subintervals based on the previous efforts in [1] and due to the collection of the data between sprays. This scenario is comparable to the piecewise constant estimates considered in [1]. We assumed the solution was a piecewise continuous function in which the value of the solution at the end of one subinterval was the initial value of the solution at the start of the next subinterval. We want to emphasize the fact that these solutions are not unique. In future research efforts, one might like to investigate how one "best" chooses the interval endpoints as well as the choice of QM on each subinterval. In current efforts, the model fits can vary depending on these factors. The continuous piecewise model solution fits are given in Figure 3. These can be compared to the case when the agammaegate nature of the data was ignored. We refer the reader to [7] for a full comparison, but we highlight some main differences here. In almost all conditions (almost all data sets), when the agammaegate data was not ignored and treated correctly, the cost function values were smaller and produced better fits to the data; see Table 1. The one case in which the cost function was slightly higher was in the weedy ground, low spray case in which the data on all three intervals appears to behave very similarly; see graph in bottom left row of Figure 3.
We refer the reader to [7] for the estimated distributions for all the data sets. However, we want to highlight two cases in this paper, the bare ground, low spray case and the weedy ground, high spray case in which the differences in cost functions were more remarkable. In the bare ground, low spray case, the result when correctly considering the agammaegate nature of the data resulted in a decrease in the cost function by a factor of about 1.6 while in the weedy ground, high spray case, there was a decrease in the cost function by a factor of about 3.2. The estimated piecewise distributions for these are given in Figures 4 and 5, respectively. Notice that the distributions on each segment are slightly different in both shape and spread of values. These are not nested models; therefore, we cannot statistically compare the model fits as outline in [1]. Nonetheless, the differences in model fits illustrates the potential errors which may occur when one naively treats agammaegate longitudinal data as individual longitudinal data.
One may on initial observation, perceive strong similarities in our findings above whether one treats the data as individual longitudinal data or as the agammaegate data which it is. However, in the 3 subinterval approach shown above, the results were different with quite different cost functions. Moreover, in other examples not given in this paper, we found vastly different or non-converging estimates when treating the data correctly as agammaegate data. Our results in this paper underscore the potential pitfalls inherent in using field census data in modeling population dynamics in applied ecology. Many sampling schemes in agroecosystems as well as natural systems rely on regular population counts. These data are often regarded as individual longitudinal data, which is reflected in assumptions underlying the statistical analyses. In many of these cases, however, because of reproduction, mortality, and redistribution of individuals, repeated population sampling results in counts of different individuals each time period. This necessitates treating the data as agammaegate individual data. In the current illustration, the difference in data interpretation differs little between these two approaches; we offer here a strong cautionary tale nonetheless because in many cases the two approaches may lead to markedly different conclusions.
This research was supported in part by the U.S. Air Force Office of Scientific Research under grant AFOSR FA9550-18-1-0457.
The authors declare there is no conflicts of interest.
[1] | B. M. Adams, H. T. Banks, J. E. Banks, J. D. Stark, Population dynamics models in plant-insectherbivore-pesticide interactions, Math. Biosci., 196 (2005), 39-64. |
[2] | J. E. Banks, J. D. Stark, Aphid response to vegetation diversity and insecticide disturbance, Agric. Ecosyst. Environ., 103 (2004), 595-599. |
[3] | H. T. Banks, A Functional Analysis Framework for Modeling, Estimation and Control in Science and Engineering, Taylor and Frances Publishing, CRC Press, Boca Raton, FL, 2012. Secs 1.3, 1.4, and 14.4. |
[4] | H. T. Banks, S. Hu, W. C. Thompson, Modeling and Inverse Problems in the Presence of Uncertainty, Taylor and Frances Publishing, CRC Press, Boca Raton, FL, 2014. Chapter 5. |
[5] | J. E. Banks, The scale of landscape fragmentation affects herbivore response to vegetation heterogeneity, Oecologia, 117 (1998), 239-246. |
[6] | J. E. Banks, Effects of weedy field margins on Myzus persicae (Hemiptera: Aphididae) in a broccoli agroecosystem, Pan-Pac. Entomol., 76 (2000), 95-101. |
[7] | H. T.Banks, J. E. Banks, J. Catenacci, M. L. Joyner, J. D. Stark, Comparison of dynamic models for plant-insect herbivore-pesticide interactions CRSC-TR19-11, Center for Research in Scientific Computation, N. C. State University, Raleigh, NC, July, 2019. |
[8] | H. T. Banks, J. E. Banks, N. Murad, J. A Rosenheim, K. Tillman, Modelling pesticide treatment effects on Lygus hesperus in cotton fields, Proceedings, 27 th IFIP TC7 Conference 2015 on System Modelling and Optimization, L. Bociu et al (Eds.) CSMO 2015 IFIP AICT 494, p.1-12, 2017, Springer. |
[9] | H. T. Banks, J. Catenacci, Aggregate data and the Prohorov Metric Framework: Efficient gradient computation, CRSC-TR15-13, Center for Research in Scientific Computation, N. C. State University, Raleigh, NC, November, 2015; Appl. Math. Lett., 56 (2016), 1-9. |
[10] | H. T. Banks, Jared Catenacci and Shuhua Hu, Asymptotic properties of probability measure estimators in a nonparametric model, CRSC TR14-05, N. C. State University, Raleigh, NC, May, 2014; SIAM/ASA J. Uncertain., 3 (2015), 417-433. |
[11] | H. T. Banks, K. B. Flores, I. G. Rosen, E. M. Rutter, M. Sirlanci, W. C. Thompson, The Prohorov Metric Framework and aggregate data inverse problems for random PDEs, Commun. Appl. Anal., 22 (2018), 415-446. |
[12] | H. T. Banks, W. C. Thompson, Least squares estimation of probability measures in the Prohorov Metric Framework, CRSC-TR12-21, N. C. State University, Raleigh, NC, November, 2012. |
[13] | H. T. Banks, W. C. Thompson, Existence and consistency of a nonparametric estimator of probability measures in the Prohorov metric framework, Int. J. Pure Appl. Math., 103 (2015), 819-843. |
[14] | H. T. Banks, W. C. Thompson, Random delay differential equations and inverse problems for aggregate data problems, Eurasian J. Math. Computer Appl., 6 (2018), 4-16. |
[15] | W. E. Boyce, R. C. DiPrima, Elementary Differential Equations and Boundary Value Problems, John Wiley and Sons,Inc., 1997, 6th edition, New York. |
[16] | H. T. Banks, K. L. Bihari, Modeling and estimating uncertainty in parameter estimation, Inverse Probl., 17 (2001), 95-111. |
[17] | L. K. Potter, Physiologically based pharmacokinetic models for the systemic transport of trichloroethylene, Ph. D. thesis, North Carolina State University, 2001, www.lib.ncsu.edu. |
[18] | H. T. Banks, D. M. Bortz, G. A. Pinter, L. K. Potter, Modeling and imaging techniques with potential for application in bioterrorism, Chapter 6 in Bioterrorism: Mathematical Modeling Applications in Homeland Security, (H.T. Banks and C. Castillo-Chavez, eds.), Frontiers in Applied Math, FR28, SIAM, 2003, Philadelphia, PA, 129-154. |
[19] | H. T. Banks, J. L. Davis, A comparison of approximation methods for the estimation of probability distributions on parameters, Appl. Numer. Math., 57 (2007), 753-777. |
[20] | H. T. Banks, J. L. Davis, Quantifying uncertainty in the estimation of probability distributions, Math. Biosci. Eng., 5, (2008), 647-667. |
[21] | H. T. Banks, B. G. Fitzpatrick, Estimation of growth rate distributions in size-structured population models, Q. Appl. Math., 49 (1991), 215-235. |
[22] | H. T. Banks, B. G. Fitzpatrick, L. K. Potter, Y. Zhang, Estimation of probability distributions for individual parameters using aggregate population data, In Stochastic Analysis, Control, Optimization and Applications, (W. McEneaney, G. Yin and Q. Zhang, eds.), Birkhauser, 1989, Boston. |
[23] | P. Billingsley, Convergence of Probability Measures, Wiley, New York,1968. |
Data Set | Cost Function - Ignore Aggregate Nature | Cost Function - Incorporate Aggregate Nature |
Bare Ground, No Spray | 1.57e+04 | 1.04e+04 |
Bare Ground, Low Spray | 2.51e+04 | 1.60e+04 |
Bare Ground, High Spray | 1.34e+04 | 1.11e+04 |
Weedy Ground, No Spray | 1.19e+04 | 1.16e+04 |
Weedy Ground, Low Spray | 1.83e+03 | 1.87e+03 |
Weedy Ground, High Spray | 1.57e+04 | 4.84e+03 |
Data Set | Cost Function - Ignore Aggregate Nature | Cost Function - Incorporate Aggregate Nature |
Bare Ground, No Spray | 1.57e+04 | 1.04e+04 |
Bare Ground, Low Spray | 2.51e+04 | 1.60e+04 |
Bare Ground, High Spray | 1.34e+04 | 1.11e+04 |
Weedy Ground, No Spray | 1.19e+04 | 1.16e+04 |
Weedy Ground, Low Spray | 1.83e+03 | 1.87e+03 |
Weedy Ground, High Spray | 1.57e+04 | 4.84e+03 |