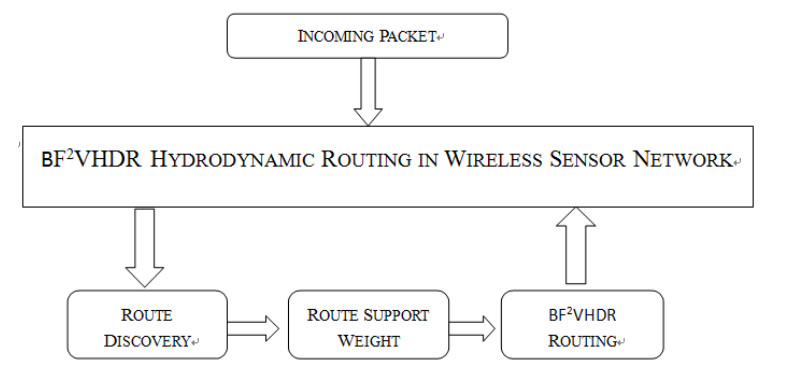
Citation: Suresh Y, Kalaivani T, Senthilkumar J, Mohanraj V. BF2 VHDR based dynamic routing with hydrodynamics for QoS development in WSN[J]. Mathematical Biosciences and Engineering, 2020, 17(1): 930-947. doi: 10.3934/mbe.2020050
[1] | Nawaz Ali Zardari, Razali Ngah, Omar Hayat, Ali Hassan Sodhro . Adaptive mobility-aware and reliable routing protocols for healthcare vehicular network. Mathematical Biosciences and Engineering, 2022, 19(7): 7156-7177. doi: 10.3934/mbe.2022338 |
[2] | B. Kiruthika, Shyamala Bharathi P . Intelligent dynamic trust secure attacker detection routing for WSN-IoT networks. Mathematical Biosciences and Engineering, 2023, 20(2): 4243-4257. doi: 10.3934/mbe.2023198 |
[3] | Tahir Rasheed, Faran Nabeel, Muhammad Bilal, Yuping Zhao, Muhammad Adeel, Hafiz. M. N. Iqbal . Aqueous monitoring of toxic mercury through a rhodamine-based fluorescent sensor. Mathematical Biosciences and Engineering, 2019, 16(4): 1861-1873. doi: 10.3934/mbe.2019090 |
[4] | Yong Zhu, Zhipeng Jiang, Xiaohui Mo, Bo Zhang, Abdullah Al-Dhelaan, Fahad Al-Dhelaan . A study on the design methodology of TAC3 for edge computing. Mathematical Biosciences and Engineering, 2020, 17(5): 4406-4421. doi: 10.3934/mbe.2020243 |
[5] | Tamás Tekeli, Attila Dénes, Gergely Röst . Adaptive group testing in a compartmental model of COVID-19*. Mathematical Biosciences and Engineering, 2022, 19(11): 11018-11033. doi: 10.3934/mbe.2022513 |
[6] | Honglei Wang, Wenliang Zeng, Xiaoling Huang, Zhaoyang Liu, Yanjing Sun, Lin Zhang . MTTLm6A: A multi-task transfer learning approach for base-resolution mRNA m6A site prediction based on an improved transformer. Mathematical Biosciences and Engineering, 2024, 21(1): 272-299. doi: 10.3934/mbe.2024013 |
[7] | Yejun Hu, Liangcai Dong, Lei Xu . Multi-AGV dispatching and routing problem based on a three-stage decomposition method. Mathematical Biosciences and Engineering, 2020, 17(5): 5150-5172. doi: 10.3934/mbe.2020279 |
[8] | Hao Yuan, Qiang Chen, Hongbing Li, Die Zeng, Tianwen Wu, Yuning Wang, Wei Zhang . Improved beluga whale optimization algorithm based cluster routing in wireless sensor networks. Mathematical Biosciences and Engineering, 2024, 21(3): 4587-4625. doi: 10.3934/mbe.2024202 |
[9] | Xiao Chu, Weiqing Wang, Zhaoyun Sun, Feichao Bao, Liang Feng . An N6-methyladenosine and target genes-based study on subtypes and prognosis of lung adenocarcinoma. Mathematical Biosciences and Engineering, 2022, 19(1): 253-270. doi: 10.3934/mbe.2022013 |
[10] | Fang Zhang, Wenzhe Cui, Yanfei Dai, Yulin Zhao . Bifurcations of an SIRS epidemic model with a general saturated incidence rate. Mathematical Biosciences and Engineering, 2022, 19(11): 10710-10730. doi: 10.3934/mbe.2022501 |
The hydrodynamics is about fluid mechanics which present the flow of fluids in any channel. The fluid has different properties which change according to the friction and volume. When a pipeline has different junction or obstacles, the flow of fluid gets disturbed and its velocity has been decreased. The velocity of the fluid is depending on the number of obstacles and size of the channel considered. They intended to perform any data collection or to provide any service. The nodes of WSN perform data transmission in a cooperative manner. They have bounded energy which gets lost at each data transmission and reception.
The packets transmitted through any route in WSN faces many challenges. The presence of number of sensor node increases the latency which in turn reduces the throughput performance. When the same routes or the hops are used by several nodes, the traffic gets increased and turns the latency to higher and throughput to lower.
The active routing is the procedure of choosing an efficient route to transmit a packet towards any destination. As the status of node keep changing at all the time, it is necessary to choose an optimal route towards destination. As the physical characteristics of the sensor nodes gets changed dynamically, the dynamic routing algorithm has to consider the traffic, flow of packets, the nodes energy, and hops before choosing a route for data transmission.
The most algorithms consider only the flow and friction to choose the route towards the destination. This highly affects the performance of the wireless sensor network. Towards this issue, a BF3-VHDR (BackForward-Flow-Friction-Velocity HDR) routing is presented in this paper. The algorithm considers the flow frequency and velocity in both the directions of the route to choose a route. Because, there will be condition that the packets being reroute due to higher traffic or route failure. Also there will be better route being used by an intermediate route to reach the destination. By considering all this, the proposed algorithm is presented to improve the network performance. The detailed approach is presented in the next section.
There are number of routing approaches has been discussed in literature. This section discusses set of methods towards the problem of routing and QoS development of WSN.
PACO (Probabilistic Ant Colony Optimization) [1] routing algorithm minimizes the number of hops. Further, the algorithm defines the energy distance and discusses a routing algorithm to minimize the distance. The algorithm overrides the performance of ant colony optimization based routing and improves the performance of routing. Mohsen Kariman-Khorasani et al. discuss a joint routing method which employs the duty cycle scheduling algorithm [2]. Also, the algorithm presents an energy balance technique for the wireless sensor networks. The author further introduces a flow sharing algorithm for the maximization of network lifetime.
H. R. Karkvandi et al. maintains various information of resource nodes using which the method computes the lifetime value for each of the nodes [3]. The method uses transmission range to compute their confidence level. Also, the authors introduce a lifetime criterion approach to improve the network lifetime. P. Edith et al. introduce a lifetime maximization technique for the wireless sensor networks [4]. The method computes the task cycles and uses the mean square error function. Finally, an optimal multi path routing is described to improve the network lifetime.
Veeranji Reddy et al. consider the transmission power assignment strategy in Wireless Sensor Networks to increase lifespan [5]. Gribaudo et al. consider that a WSN inquiry to prove that the investigation is based on hydrodynamics [6]. In the model, the sensor nodes are taken as a liquid component and are reported in soft and non-interactive space. It is characterized by the concept of near-knuckle thickness which is indirectly the size of the nodes intended to be given in the unit by the creators. They are expected to be fixed on the nodes in the networks. Nodes are spread with multiple nodes through multi-hop data. Limited time network regulated state. However, regardless of its various problems, such as the noses 'use of vulnerability and the ends of the' fight for just routine routing wireless channels.
Erkmen et al. exhibited a novel hydrodynamic reenactment strategy to take care of the organization issue of versatile sensor networks in an obscure situation with respect to every node in the network as liquid component [7]. Concerning this, the liquid drove nodes to "stream" with liquid, in order to make the network sending with a normal powerful inclusion rate.
Zhao Wei et al. discuss the author considers the packets as fluids in field. Also, the sink nodes are considered as the sink flow where the packets are flowing to their potential to reach the sink nodes [8]. Alexandros Schillings et al. discuss a theory of games where the players are as nodes of wireless sensor networks. In the theory the algorithm looks to maximize the profits of players [9].
Khawam et al. discuss a fluid model to improve the network lifetime and accounts the network interference, radio propagation to estimate the network lifetime [10]. Also, the algorithm counts the network density as a key factor to improve the network lifetime. Chiasserini et al. discuss the development of large scale of WSN [11]. The authors present fluid scheme which represent the network by continuous fluid flow. The algorithm estimates the network traffic by computing the fluid flow.
H. Kim and C. Jennifer [12] develop the method approximates the behaviors in the simulation model. The algorithm adapts various measures to improve the lifetime of the wireless sensor networks. Aysal et al. describe a decentralized model to maximize the network lifetime [13,14]. The algorithm uses the newton’s law in estimating the maximum likelihood of the node. Also, the algorithm uses a polynomial algorithm to select the region o f interest to schedule the nodes.
In [15], Mohammed Zaki Hasan et al. proposes a bio-motivated Particle Multi-Swarm Optimization (PMSO) Routing calculation, k is a period of time, and a selective selection is common in common ways that management fails when parameters are fulfilled. Many swarm techniques have the power to determine the best bearings for selecting routing of the same time business in all situations in the network.
Psychometric data drives sensor networks. G. Singh et al. discuss that wireless sensor networks speak of which a world view from the sense of data is using sensitive data, which speaks, and intelligence components are used to convey data end-fulfillment quality tank [16]. The specific edges are known as Local Cognition Node (LCNs), learning information as a rational component in thinking networking.
Blame tolerant calculations for availability rebuilding in wireless sensor networks. Y. Zeng et al. presents F2CRA (Full 2 Line Current Rehabilitation Algorithm) for a full 2-line rehabilitation, which performs routing based on delay tolerant [17].
Molecule swarm improvement with versatile time-fluctuating topology network [18], W. H. Lim et al. proposes another PSO variation called PSO with versatile time-changing topology availability (PSO-ATVTC) that utilizes an ATVTC module and another learning system. The proposed ATVTC module explicitly expects to adjust the calculation's investigation/abuse seeks by shifting the molecule's topology availability with time as indicated by its looking execution. The proposed learning structure comprises of another speed refresh system and another area seek administrator to enhance the calculation's execution. Similarly in [19] H. Bagci et al. present a distributed fault-tolerant topology control algorithm for heterogeneous wireless sensor networks.
Vitality mindful multipath routing plan dependent on molecule swarm streamlining in versatile specially appointed networks [20]. Y. H. Robinson et al. proposed a vitality mindful multipath routing plan dependent on molecule swarm enhancement that utilizes ceaseless time repetitive neural network (CTRNN) to take care of improvement issues. CTRNN finds the ideal circle free ways to comprehend connect disjoint ways in a MANET. The CTRNN is utilized as an ideal way choice strategy that creates a lot of ideal ways among source and goal.
Energy-Efficient Algorithm for Reliable Routing of Wireless Sensor Networks [21], Habib Mostafaei et al. proposed a distributed learning automata (DLA) based multi constrained routing. The method is focused to leverage and maintain the QoS performance even with the presence of small set of nodes. The method considered delay and link reliability in path selection.
From the above review, it has been identified that the algorithms suffer to achieve higher performance in all the QoS parameters. The PACO consider the distance which affects the throughput and PDF of the network [1]. Similarly, Mohsen et al. consider the energy parameter and lifetime of the network [2]. This makes the most nodes to be in sleep mode in most time. Also, this affects the throughput and packet delivery of the network. The methods focused on identifying the trusted route and this reduces the lifetime of the network [3,4] but the throughput ratio does not considered in [5]. The approaches [8,9,10,11,12] have considered the network lifetime and suffer with higher latency. In general, the methods reviewed have the problem of poor efficiency in routing and produces inefficient results. The PACO algorithm works towards the minimization of number of hops with the ant colony optimization routing. But suffer to produce higher throughput performance as there will be huge traffic or friction would be present in the shortest route. The VGTR (Virtual Game Theory Routing) algorithm considers only the flow factor to identify the route for data transmission which increases the latency and affects the throughput performance. The DLA algorithm considers only link quality and latency but does not considered the traffic parameters. Similarly, the self-deployment algorithm considers the flow factor as the key to perform routing which affects the throughput performance and increases the latency as well. All the above discussed methods have the problem of poor efficiency in routing and produce inefficient results.
The proposed hydrodynamic routing algorithm consider number of features of hydrodynamic namely back flow, forward flow, traffic, velocity to perform route selection. The method monitors the network at each time and estimates the velocity and friction measures. Using the measures estimated, the method computes the packet transmission strength to select a single route among number of available routes. The detailed approach work is showed in Figure 1.
Figure 1 demonstration the construction of the proposed algorithm and shows the functional stages. The proposed BF2VHDR algorithm performs route discovery to identify the list of routes available towards the destination. For each route being identified, the route support weight has been measured according to friction, flow, and velocity factors. According to the value of route support weight, a single route has been selected by the packet forwarding algorithm.
The route discovery is the procedure of recognizing the list of routes available in the network considered. Consider, there exist N amount of nodes present in the simulation where each has been located in a geographic position Gp. However, they are located within the coverage of others. By using this, the number of routes available between any source S and destination D can be identified. To perform this task, the method generates a route request HDR-RR and broadcast into the network. The broadcast is utilized to override the neighbor discovery process initially. The nodes in a sleep state would not receive in the next cycle but initially it will be obtained. Upon receiving this, the neighbor sensor forwards them to its neighbors. When a node identifies the presence of the destination in its neighbor list, it generates the route reply HDR-RREP. Generated reply will be reversed in the same route the RR is received. The source node collects all the routes and extracts the friction, flow, back-flow and its energy details with the reply. From the reply received, the source extracts various features and updates the node table.
![]() |
![]() |
From the route reply message, the method extracts various features required to perform route selection.
In this stage, the method compute different measures on the route identified. First, the number of packets being sent on the route has been identified and the number of bytes being sent also identified. Using the node table entries, the technique recognizes the list of hops and friction in each hop and back flow and forward flow on the hop are identified. Then the node energy on each hop also identified. Using all the features identified, the method compute the route factor, friction factor, forward flow factor, backward flow factor, and velocity factor are estimated. Using the estimated measures, the route support weight has been estimated.
Route Support Weight Estimation Algorithm:
Input: Route R, Node Table NT
Output: RSW
Start
Read Route R given.
Read Node Table NT.
Identify the list of hops present in the route R.
Hop List Hl = ∑Hops∈R
For each hop Hi from Hl
Identify forward flow in Hi as FH.
FH=size (NT) i=1NT(i).Hop==Hi & & NT(i).Hop→Forward | (1) |
Identify back flow in Hi as BFH
BFH =size (NT) i = 1NT(i).Hop==Hi & & NT(i).Hop←Back | (2) |
Identify the energy of Hi as EH
EH =size (NT) i = 1NT(i).Hop==Hi & & NT(i).Hop(.Energy) | (3) |
End
Compute total number of packets sent in R as Tps
Tps=∑size(Hl)i = 1TPS+BFH+FH | (4) |
Compute route factor RF=TPSsize(Hl)× β ×(∑size(Hl)i = 1EH μ ) | (5) |
Here β-represent the initial energy of the nodes where μ-represent the energy required to transmit or receive a packet.
Compute Friction Factor FF =size(Hl)i=1FF+FH+BFHTPS | (6) |
Compute Forward Flow Factor FoFF =size(Hl)i=1FoFF+FHTPS | (7) |
Compute Backward Flow Factor BFF = size(Hl)i=1BFF+BFHTPS | (8) |
Compute Velocity Factor VF=RFFF×k | (9) |
Here k- minimum latency value.
Compute Route Support Weight RSW =FoFFFF×BFFFF×FFVF×RF | (10) |
The route support weight estimation algorithm has computed the different factors of flow, friction, velocity. Using the factors estimated, the method computes the route support weight.
The routing is the major issue in the wireless sensor networks. The routing is performed based on the details of various factors of the sensor nodes present in the network. In this approach, the list of routes available in the network is identified. Then for each route the method identifies the list of hops present. For each hop, the method maintains the node details in the Node Table NT, which contains the information regarding the flow, friction, back flow ad energy. Using all these information, the method estimates the route support weight, which has been used to select a single optimal route for data transmission.
![]() |
The dynamic routing procedure calculates the direction support weight for the routes available. Among them a single one is selected for data transmission which has higher RSW value.
The proposed method has been realized and estimated for its routine in various factors. The detail of implementation and evaluation has been given in Table 1.
Parameter | Value |
Tool Used | Advanced Java |
Number of nodes | 50 |
Transmission Range | 100 |
Simulation Area | 1000/1000 meters |
Simulation Time | 5 minutes |
The Table 1 presents the reproduction details being used and lists the set of parameters of simulation. To evaluate the performance, the simulation has been carried out with the presence of large number of nodes and data rate has been varied for different simulations. In each duty cycle, the algorithm estimates the route support weight for each route identified. According to the weight being estimated, an optimal route has been selected for data transmission.
Figure 2 shows the sample network topology considered for the evaluation of proposed algorithm.
The Table 2 shows the details of traffic present in different intermediate nodes of the network considered. Also, it shows the value of forward flow, backward flow, and energy of the nodes. Also, it is considered that, the nodes have the initial energy as 100 joules and current energy represents the energy value the nodes have right now.
Node ID | Current Energy in joules | Traffic bytes/sec | Forward Flow | Backward Flow | |
1 | 67 | 0 | 32 | 0 | |
2 | 72 | 3 | 12 | 0 | |
3 | 68 | 5 | 14 | 0 | |
4 | 59 | 7 | 15 | 2 | |
5 | 85 | 6 | 11 | 1 | |
6 | 64 | 3 | 16 | 1 | |
7 | 76 | 4 | 21 | 3 | |
8 | 79 | 8 | 14 | 2 | |
9 | 83 | 9 | 16 | 5 | |
10 | 79 | 8 | 17 | 1 | |
21 | 78 | 7 | 18 | 3 | |
11 | 69 | 8 | 15 | 2 | |
12 | 59 | 9 | 16 | 4 | |
13 | 72 | 11 | 12 | 3 | |
14 | 82 | 9 | 13 | 7 | |
15 | 86 | 11 | 11 | 8 | |
16 | 89 | 12 | 13 | 4 | |
17 | 93 | 11 | 18 | 3 | |
18 | 94 | 13 | 19 | 7 | |
19 | 95 | 16 | 21 | 6 | |
20 | 97 | 17 | 11 | 1 |
Using the details of traffic value and the traffic constant value is of 1.273, the velocity, hydro dynamic weight and cumulative hydrodynamic weight. For example, for the route “1-2-8-9-15-12-20”, we can compute different measures as follows:
Compute Total number of packets sent in R as Tps = ∑size(Hl)i=1TPS+BFH+FH
Tps =(32+0)+(0+0)+(12+0)+(14+2)+(16+5)+(11+8)+(16+4)+(11+1)=130 |
Compute route factor RF = TPSsize(Hl)×β×(∑size(Hl)i=1EHμ)
RF=(100/(7∗100))∗(67+72+79+83+86+59+97)/0.5=54.212 |
Consider the minimum joules for a data transmission is 0.5 joules and the value of μ=0.5joules.
Compute Friction Factor FF.
FF=32+0130+12+0130+14+2130+16+5130+11+8130+16+4130+11+1130=0.99 |
Compute Forward Flow Factor FoFF.
FoFF =32130+12130+14130+16130+11130+16130+11130=0.83 |
Compute Backward Flow Factor BFF
BFF=0130+0130+2130+5130+8130+4130+1130=0.98 |
Compute Velocity Factor VF = 154.210.99×0.8 = 125.3
Here k- minimum latency value = 0.88 milliseconds.
Compute Route Support Weight RSW = 0.830.99×0.980.99×0.99125.3×154.2 = 0, 89
Similarly, the route support weight can be measured for different routes. Based on the values of route support weight, the optimal route can be selected.
The performance of the proposed method has been measured by computing different parameters namely throughput performance, packet delivery ratio and latency. The throughput performance is computed using the equation (11)
ThroughputPerformance=NumberofbytesdeliveredpersecondTotalNumberofbytessentpersecond | (11) |
The Figure 3 shows the comparison of throughput performance achieved by various approaches in varying number of nodes. Also the Figure 3 claims that the proposed method has produced higher throughput than other methods. The PACO algorithm works towards the minimization of number of hops with the ant colony optimization routing. However, the PACO would select a shortest route but there will be always higher traffic in the shortest route which leads to packet drop. Also, the PACO algorithm does not consider the other factors like flow, friction, velocity and so on. Similarly, the VGTR algorithm focused to improve the throughput performance with the consideration of channel allocation, which produces low throughput performance. The DLA algorithm considers only the reliability of links in achieving higher throughput performance but misses the traffic conditions which affect the throughput performance. Also, the Self Deployment algorithm considers only the value of flow to perform routing. However, all the PACO, VGTR, Self-Deployment and DLA algorithms produces low throughput performance. But the enforcement of proposed BF2VHDR algorithm selects the routes based on the above mentioned parameters which support the improvement of throughput performance. By considering the friction which represents the traffic, flow and backflow which represent the amount of data pass through and velocity, the proposed algorithm performs route selection. This helps to achieve higher throughput compare to other methods.
The packet delivery ratio represents the efficiency in packet delivery by the protocol discussed. The packet delivery ratio is computed using the equation (12)
PacketDeliveryRatio=NumberofpacketsdeliveredsuccessfullyTotalNumberofPacketsSent | (12) |
The Figure 4 demonstrates the examination of packet transport part created by various strategies and it indicates obviously that the proposed technique has produces higher parcel conveyance portion than different techniques. The PACO algorithm works towards the minimization of number of hops with the ant colony optimization routing. However, the PACO would select a shortest route but there will be always higher traffic in the shortest route which leads to packet drop. Similarly, the VGTR algorithm works based on tokens which produce less packet delivery ratio. Also, the Self Deployment algorithm considers only the value of flow to perform routing. The DLA algorithm considers only the link quality which reduces the packet delivery ratio. However, all the PACO, VGTR, Self-Deployment and DLA algorithms produces less packet delivery ratio. However, the adaption of BF2VHDR algorithm increases the packet delivery ratio as it selects the transmission route according to back flow, flow, friction, and velocity. Because of the proposed algorithm, considers the friction, flow, forward flow an backward flow and velocity features of available routes in route selection stage, the algorithm could deliver the data packets in least time. This helps to improve the packet delivery fraction of the network.
Similarly the latency ratio is measured by computing the time duration between the packet sent time and the packet delivery time. The efficiency of the protocol will be higher when the latency ratio is less.
The Figure 5 shows the comparison of latency ratio produced by different methods and it shows clearly that the method has produces less latency than other methods. The PACO algorithm works towards the minimization of number of hops with the ant colony optimization routing. However, the PACO would select a shortest route but there will be always higher traffic in the shortest route which leads to packet drop. So that, the packet has to be retransmitted this increases the latency of transmission. Similarly, the VGTR algorithm uses only the channel availability to perform routing which leads to higher latency. Also, the Self Deployment algorithm considers only the value of flow to perform routing. The self-deployment method selects the route with least flow for transmission, which leads to higher latency. The DLA algorithm considers the link quality in path selection which introduces the selection of higher hop counted route which increases the latency. However, all the PACO, VGTR, Self-Deployment and DLA algorithms produces low throughput performance. However, the adaption of BF2VHDR algorithm reduces the latency value as it considers back flow, flow, friction, and velocity in the selection of route. However the route may be longer, the latency of the packet has been reduced. The Figure 5, shows the proposed algorithm, performs route selection based on six factors namely friction, flow, forward flow, backward flow, energy and velocity. By considering these factors, the algorithm is capable of identifying the traffic free route and transmits through the identified route. This helps to reduce the latency of the data packets.
The Table 3 shows the near outcomes on different nature of management parameters created by various techniques and it demonstrates plainly that the proposed strategy has produces proficient outcomes on every one of the variables of nature of administration of wireless sensor networks. The proposed algorithm outperform efficiently in each factors considered and produces efficient results than other methods considered.
S.No | Protocol | Throughput % with number of nodes | Average Delay(ms) with number of nodes | PDF % with number of nodes | ||||||
70 | 100 | 200 | 70 | 100 | 200 | 70 | 100 | 200 | ||
1. | PACO | 72 | 76 | 81 | 72 | 68 | 61 | 74 | 77 | 81 |
2. | VGTR | 79 | 81 | 85 | 43 | 39 | 32 | 76 | 79 | 83 |
3. | Self-Deployment | 83 | 86 | 89 | 32 | 29 | 23 | 81 | 84 | 87 |
4. | DLA | 86 | 88 | 91 | 29 | 26 | 21 | 84 | 87 | 89 |
5. | F2VHDR | 96.5 | 97.8 | 98.9 | 17 | 14 | 11 | 97 | 98.5 | 99.6 |
6 | BF2VHDR | 97.2 | 98.3 | 99.4 | 15 | 12 | 8 | 97.6 | 99.1 | 99.8 |
The Figure 6 shows the comparison of throughput performance achieved by various approaches in different simulation time. Also the Figure 6 claims that the proposed method has produced higher throughput than other methods. The PACO algorithm works towards the minimization of number of hops with the ant colony optimization routing. However, the PACO would select a shortest route but there will be always higher traffic in the shortest route which leads to packet drop. Also, the PACO algorithm does not consider the other factors like flow, friction, velocity and so on. Similarly, the VGTR algorithm focused to improve the throughput performance with the consideration of channel allocation, which produces low throughput performance. The DLA algorithm considers only the reliability of links in achieving higher throughput performance but misses the traffic conditions which affect the throughput performance. Also, the Self Deployment algorithm considers only the value of flow to perform routing. However, all the PACO, VGTR, Self-Deployment and DLA algorithms produces low throughput performance, because the methods does not considered the dynamic conditions as the set of sensor nodes would be down after some time which affect the throughput performance. But the enforcement of proposed BF2VHDR algorithm selects the routes based on the above mentioned parameters which support the improvement of throughput performance. By considering the friction which represents the traffic, flow and backflow which represent the amount of data pass through and velocity, the proposed algorithm performs route selection. This helps to achieve higher throughput compare to other methods.
The Figure 7 demonstrates the examination of packet transport part created by various strategies and it indicates obviously that the proposed technique has produces higher parcel conveyance portion than different techniques. The PACO algorithm works towards the minimization of number of hops with the ant colony optimization routing. However, the PACO would select a shortest route but there will be always higher traffic in the shortest route which leads to packet drop. Similarly, the VGTR algorithm works based on tokens which produce less packet delivery ratio. Also, the Self Deployment algorithm considers only the value of flow to perform routing. The DLA algorithm considers only the link quality which reduces the packet delivery ratio. However, all the PACO, VGTR, Self-Deployment and DLA algorithms produces less packet delivery ratio. The methods produces poor packet delivery ratio in different simulation time because they does not consider all the parameters which reduces the packet delivery ratio. However, the adaption of BF2VHDR algorithm increases the packet delivery ratio as it selects the transmission route according to back flow, flow, friction, and velocity. Because of the proposed algorithm, considers the friction, flow, forward flow an backward flow and velocity features of available routes in route selection stage, the algorithm could deliver the data packets in least time. This helps to improve the packet delivery fraction of the network.
Similarly the latency ratio is measured by computing the time duration between the packet sent time and the packet delivery time. The efficiency of the protocol will be higher when the latency ratio is less.
The Figure 8 shows the comparison of latency ratio produced by different methods at different simulation time and it shows clearly that the method has produces less latency than other methods. The PACO algorithm works towards the minimization of number of hops with the ant colony optimization routing. However, the PACO would select a shortest route but there will be always higher traffic in the shortest route which leads to packet drop. So that, the packet has to be retransmitted this increases the latency of transmission. Similarly, the VGTR algorithm uses only the channel availability to perform routing which leads to higher latency. Also, the Self Deployment algorithm considers only the value of flow to perform routing. The self-deployment method selects the route with least flow for transmission, which leads to higher latency. The DLA algorithm considers the link quality in path selection which introduces the selection of higher hop counted route which increases the latency. However, all the PACO, VGTR, Self Deployment and DLA algorithms produces low throughput performance. However, the adaption of BF2VHDR algorithm reduces the latency value as it considers back flow, flow, friction, and velocity in the selection of route. However the route may be longer, the latency of the packet has been reduced. The Figure 8, shows the proposed algorithm, performs route selection based on six factors namely friction, flow, forward flow, backward flow, energy and velocity. By considering these factors, the algorithm is capable of identifying the traffic free route and transmits through the identified route. This helps to reduce the latency of the data packets.
The Table 4 shows the near outcomes on different nature of management parameters created by various techniques and it demonstrates plainly that the proposed strategy has produces proficient outcomes on every one of the variables of nature of administration of wireless sensor networks. The evaluation result is obtained at different simulation time. The proposed algorithm outperform efficiently in each factors considered and produces efficient results than other methods considered.
S.No | Protocol | Throughput % with number of nodes | Average Delay(ms) with number of nodes | PDF % with number of nodes | ||||||
1 Min. | 3 Min. | 5 Min. | 1 Min. | 3 Min. | 5 Min. | 1 Min. | 3 Min. | 5 Min. | ||
1. | PACO | 72 | 70 | 69 | 72 | 68 | 61 | 74 | 70 | 67 |
2. | VGTR | 79 | 77 | 73 | 43 | 39 | 32 | 76 | 72 | 70 |
3. | Self-Deployment | 83 | 80 | 77 | 32 | 29 | 23 | 81 | 78 | 75 |
4. | DLA | 86 | 84 | 82 | 29 | 26 | 21 | 84 | 81 | 78 |
5. | F2VHDR | 96.5 | 97.8 | 98.9 | 17 | 14 | 11 | 97 | 98.5 | 99.6 |
6 | BF2VHDR | 97.2 | 98.3 | 99.4 | 15 | 12 | 8 | 97.6 | 99.1 | 99.8 |
In this paper, we proposed a novel BF2VHDR-based hydraulic based routing program to improve the performance of wireless sensor networks. First the method identifies the list of paths available to reach the destination. For each route, the flow rate, forward flow factor, calculates backward flow factor, speed, and energy factors has been identified. Using this, the method calculates the weight of the weight supported. We will send the packet through a single path selection and the selected route, based on the calculated path weight support weight. All the factors of the quality of service in the wireless sensor networks were made efficiently. By considering the back flow, flow, velocity, friction and other factors, the method achieves higher performance in hydro dynamic routing in WSN. The protocol increases the throughput performance up to 98.3% and reduces the 11 seconds latency.
The authors declare no competing interests.
[1] | Y. H. Su, J. X. Li, Z. Q. Qin, et al., Maximizing the network lifetime by using PACO routing algorithm in wireless sensor networks, in Advances in Wireless Sensor Networks CWSN 2013, 418 (2013),155-165. |
[2] | M. Kariman-Khorasani, M. Ali Pourmina and A. Salahi, Energy balance based lifetime maximization in wireless sensor networks employing joint routing and asynchronous duty cycle scheduling techniques, Wireless Pers. Commun., 83 (2015),1057-1083. |
[3] | H. R. Karkvandi, E. Pecht and O. Yadid, Effective lifetime-aware routing in wireless sensor networks, IEEE Sensors J., 11 (2011), 3359-3367. |
[4] | P. E. Linda, S. Dinesh and P. Edreena, Network lifetime maximization for estimation in multihop wireless sensor networks, Int. J. Adv. Res. Comput. Sci. Software Eng., 4 (2014). |
[5] | N. V. Reddy and L. Jeba, Lifetime maximization in wireless sensor network, Int. J. Eng. Trends Technol., 9 (2014), 218-222. |
[6] | M. Gribaudo, C. F. Chiasserini, R. Gaeta, et al., A spatial fluid-based framework toanalyze large-scale wireless sensor networks, in Proceedings of the International Conference on Dependable Systems and Networks, (2005), 694-703. |
[7] | M. R. Pac, A. M. Erkmen and I. Erkmen, Towards fluent sensor networks: A scalable and robust self-deployment approach, in Proceedings of the 1st NASA/ESA Conference on Adaptive Hardware and Systems (AHS '06), (2006), 365-372. |
[8] | W. Zhao, X. Y. Yan, F. Shao, et al., Collaborative routing protocol based on hydrodynamics for wireless sensor networks, Int. J. Distrib. Sensor Networks, 1 (2015). |
[9] | A. Schillings and K. Yang, VGTR: A collaborative, energy and information aware routing algorithm for wireless sensor networks through the use of game theory, Geo. Sensor Networks, 5659 (2015), 51-62. |
[10] | K. Khawam, A. E. Samhat, M. Ibrahim, et al., Fluid model for wireless adhoc networks, in Proceedings of the 18th IEEE International Symposium on Personal, Indoor and Mobile Radio Communications, (2007),1-5, |
[11] | C. F. Chiasserini, R. Gaeta, M. Garetto, et al., Fluid models for large-scale wireless sensor networks, Perform. Evalu., 64 (2007), 715-736. |
[12] | H. Kim and C. Jennifer, A fast simulation framework for IEEE 802.11-operated wireless LANs, ACM SIGMETRICS Perform. Evalu. Rev., 32 (2004), 143-154. |
[13] | T. C. Aysal and K. E. Barner, Constrained decentralized estimation over noisy channels for sensor networks, IEEE Trans. Signal Process, 56 (2008), 1398-1410. |
[14] | T. C. Aysal and K. E. Barner, Blind decentralized estimation for bandwidth constrained wireless sensor networks, IEEE Trans. Wireless Commun., 7 (2008), 1466-1471. |
[15] | M. Z. Hasan and F. Al-Turjman, Optimizing multipath routing with guaranteed fault tolerance in Internet of Things, IEEE Sensors J., 17(2017), 6463-6473. |
[16] | G. Singh and F. Al-Turjman, A data delivery framework for cognitive information-centric sensor networks in smart outdoor monitoring, Comput. Commun. J., 74 (2015), 38-51. |
[17] | Y. Zeng, L. Xu and Z. Chen, Fault-tolerant algorithms for connectivity restoration in wireless sensor networks, Sensors, 16 (2016). |
[18] | W. H. Lim and N. A. M. Isa, Particle swarm optimization with adaptive time-varying topology connectivity, Appl. Soft Comput., 24 (2014), 623-642. |
[19] | H. Bagci, I. Korpeoglu and A. Yazici, A distributed fault-tolerant topology control algorithm for heterogeneous wireless sensor networks, IEEE Trans. Parall. Distrib. Syst., 26(2015), 914-923. |
[20] | Y. H. Robinson and M. Rajaram, Energy-aware multipath routing scheme based on particle swarm optimization in mobile ad hoc networks, Sci. World J., (2015), Article ID 284276. |
[21] | H. Mostafaei, Energy efficient algorithm for reliable routing of wireless sensor networks, IEEE Transact. Indust. Elect, 66(2019), 5567-5575. |
Parameter | Value |
Tool Used | Advanced Java |
Number of nodes | 50 |
Transmission Range | 100 |
Simulation Area | 1000/1000 meters |
Simulation Time | 5 minutes |
Node ID | Current Energy in joules | Traffic bytes/sec | Forward Flow | Backward Flow | |
1 | 67 | 0 | 32 | 0 | |
2 | 72 | 3 | 12 | 0 | |
3 | 68 | 5 | 14 | 0 | |
4 | 59 | 7 | 15 | 2 | |
5 | 85 | 6 | 11 | 1 | |
6 | 64 | 3 | 16 | 1 | |
7 | 76 | 4 | 21 | 3 | |
8 | 79 | 8 | 14 | 2 | |
9 | 83 | 9 | 16 | 5 | |
10 | 79 | 8 | 17 | 1 | |
21 | 78 | 7 | 18 | 3 | |
11 | 69 | 8 | 15 | 2 | |
12 | 59 | 9 | 16 | 4 | |
13 | 72 | 11 | 12 | 3 | |
14 | 82 | 9 | 13 | 7 | |
15 | 86 | 11 | 11 | 8 | |
16 | 89 | 12 | 13 | 4 | |
17 | 93 | 11 | 18 | 3 | |
18 | 94 | 13 | 19 | 7 | |
19 | 95 | 16 | 21 | 6 | |
20 | 97 | 17 | 11 | 1 |
S.No | Protocol | Throughput % with number of nodes | Average Delay(ms) with number of nodes | PDF % with number of nodes | ||||||
70 | 100 | 200 | 70 | 100 | 200 | 70 | 100 | 200 | ||
1. | PACO | 72 | 76 | 81 | 72 | 68 | 61 | 74 | 77 | 81 |
2. | VGTR | 79 | 81 | 85 | 43 | 39 | 32 | 76 | 79 | 83 |
3. | Self-Deployment | 83 | 86 | 89 | 32 | 29 | 23 | 81 | 84 | 87 |
4. | DLA | 86 | 88 | 91 | 29 | 26 | 21 | 84 | 87 | 89 |
5. | F2VHDR | 96.5 | 97.8 | 98.9 | 17 | 14 | 11 | 97 | 98.5 | 99.6 |
6 | BF2VHDR | 97.2 | 98.3 | 99.4 | 15 | 12 | 8 | 97.6 | 99.1 | 99.8 |
S.No | Protocol | Throughput % with number of nodes | Average Delay(ms) with number of nodes | PDF % with number of nodes | ||||||
1 Min. | 3 Min. | 5 Min. | 1 Min. | 3 Min. | 5 Min. | 1 Min. | 3 Min. | 5 Min. | ||
1. | PACO | 72 | 70 | 69 | 72 | 68 | 61 | 74 | 70 | 67 |
2. | VGTR | 79 | 77 | 73 | 43 | 39 | 32 | 76 | 72 | 70 |
3. | Self-Deployment | 83 | 80 | 77 | 32 | 29 | 23 | 81 | 78 | 75 |
4. | DLA | 86 | 84 | 82 | 29 | 26 | 21 | 84 | 81 | 78 |
5. | F2VHDR | 96.5 | 97.8 | 98.9 | 17 | 14 | 11 | 97 | 98.5 | 99.6 |
6 | BF2VHDR | 97.2 | 98.3 | 99.4 | 15 | 12 | 8 | 97.6 | 99.1 | 99.8 |
Parameter | Value |
Tool Used | Advanced Java |
Number of nodes | 50 |
Transmission Range | 100 |
Simulation Area | 1000/1000 meters |
Simulation Time | 5 minutes |
Node ID | Current Energy in joules | Traffic bytes/sec | Forward Flow | Backward Flow | |
1 | 67 | 0 | 32 | 0 | |
2 | 72 | 3 | 12 | 0 | |
3 | 68 | 5 | 14 | 0 | |
4 | 59 | 7 | 15 | 2 | |
5 | 85 | 6 | 11 | 1 | |
6 | 64 | 3 | 16 | 1 | |
7 | 76 | 4 | 21 | 3 | |
8 | 79 | 8 | 14 | 2 | |
9 | 83 | 9 | 16 | 5 | |
10 | 79 | 8 | 17 | 1 | |
21 | 78 | 7 | 18 | 3 | |
11 | 69 | 8 | 15 | 2 | |
12 | 59 | 9 | 16 | 4 | |
13 | 72 | 11 | 12 | 3 | |
14 | 82 | 9 | 13 | 7 | |
15 | 86 | 11 | 11 | 8 | |
16 | 89 | 12 | 13 | 4 | |
17 | 93 | 11 | 18 | 3 | |
18 | 94 | 13 | 19 | 7 | |
19 | 95 | 16 | 21 | 6 | |
20 | 97 | 17 | 11 | 1 |
S.No | Protocol | Throughput % with number of nodes | Average Delay(ms) with number of nodes | PDF % with number of nodes | ||||||
70 | 100 | 200 | 70 | 100 | 200 | 70 | 100 | 200 | ||
1. | PACO | 72 | 76 | 81 | 72 | 68 | 61 | 74 | 77 | 81 |
2. | VGTR | 79 | 81 | 85 | 43 | 39 | 32 | 76 | 79 | 83 |
3. | Self-Deployment | 83 | 86 | 89 | 32 | 29 | 23 | 81 | 84 | 87 |
4. | DLA | 86 | 88 | 91 | 29 | 26 | 21 | 84 | 87 | 89 |
5. | F2VHDR | 96.5 | 97.8 | 98.9 | 17 | 14 | 11 | 97 | 98.5 | 99.6 |
6 | BF2VHDR | 97.2 | 98.3 | 99.4 | 15 | 12 | 8 | 97.6 | 99.1 | 99.8 |
S.No | Protocol | Throughput % with number of nodes | Average Delay(ms) with number of nodes | PDF % with number of nodes | ||||||
1 Min. | 3 Min. | 5 Min. | 1 Min. | 3 Min. | 5 Min. | 1 Min. | 3 Min. | 5 Min. | ||
1. | PACO | 72 | 70 | 69 | 72 | 68 | 61 | 74 | 70 | 67 |
2. | VGTR | 79 | 77 | 73 | 43 | 39 | 32 | 76 | 72 | 70 |
3. | Self-Deployment | 83 | 80 | 77 | 32 | 29 | 23 | 81 | 78 | 75 |
4. | DLA | 86 | 84 | 82 | 29 | 26 | 21 | 84 | 81 | 78 |
5. | F2VHDR | 96.5 | 97.8 | 98.9 | 17 | 14 | 11 | 97 | 98.5 | 99.6 |
6 | BF2VHDR | 97.2 | 98.3 | 99.4 | 15 | 12 | 8 | 97.6 | 99.1 | 99.8 |