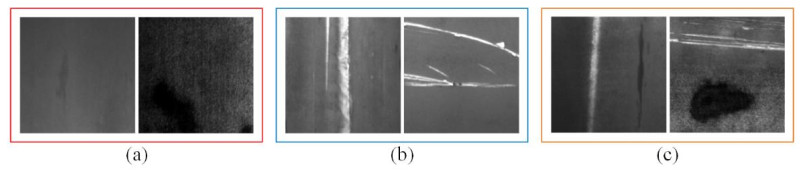
Citation: Benjamin Hersh, Amin Mirkouei, John Sessions, Behnaz Rezaie, Yaqi You. A review and future directions on enhancing sustainability benefits across food-energy-water systems: the potential role of biochar-derived products[J]. AIMS Environmental Science, 2019, 6(5): 379-416. doi: 10.3934/environsci.2019.5.379
[1] | Yong Tian, Tian Zhang, Qingchao Zhang, Yong Li, Zhaodong Wang . Feature fusion–based preprocessing for steel plate surface defect recognition. Mathematical Biosciences and Engineering, 2020, 17(5): 5672-5685. doi: 10.3934/mbe.2020305 |
[2] | Fang Luo, Yuan Cui, Xu Wang, Zhiliang Zhang, Yong Liao . Adaptive rotation attention network for accurate defect detection on magnetic tile surface. Mathematical Biosciences and Engineering, 2023, 20(9): 17554-17568. doi: 10.3934/mbe.2023779 |
[3] | Xian Fu, Xiao Yang, Ningning Zhang, RuoGu Zhang, Zhuzhu Zhang, Aoqun Jin, Ruiwen Ye, Huiling Zhang . Bearing surface defect detection based on improved convolutional neural network. Mathematical Biosciences and Engineering, 2023, 20(7): 12341-12359. doi: 10.3934/mbe.2023549 |
[4] | Lili Wang, Chunhe Song, Guangxi Wan, Shijie Cui . A surface defect detection method for steel pipe based on improved YOLO. Mathematical Biosciences and Engineering, 2024, 21(2): 3016-3036. doi: 10.3934/mbe.2024134 |
[5] | Yinghong Xie, Biao Yin, Xiaowei Han, Yan Hao . Improved YOLOv7-based steel surface defect detection algorithm. Mathematical Biosciences and Engineering, 2024, 21(1): 346-368. doi: 10.3934/mbe.2024016 |
[6] | Liyong Ma, Wei Xie, Haibin Huang . Convolutional neural network based obstacle detection for unmanned surface vehicle. Mathematical Biosciences and Engineering, 2020, 17(1): 845-861. doi: 10.3934/mbe.2020045 |
[7] | Jiaming Ding, Peigang Jiao, Kangning Li, Weibo Du . Road surface crack detection based on improved YOLOv5s. Mathematical Biosciences and Engineering, 2024, 21(3): 4269-4285. doi: 10.3934/mbe.2024188 |
[8] | Zhangjie Wu, Minming Gu . A novel attention-guided ECA-CNN architecture for sEMG-based gait classification. Mathematical Biosciences and Engineering, 2023, 20(4): 7140-7153. doi: 10.3934/mbe.2023308 |
[9] | Zhongliang Zhang, Yao Fu, Huiling Huang, Feng Rao, Jun Han . Lightweight network study of leather defect segmentation with Kronecker product multipath decoding. Mathematical Biosciences and Engineering, 2022, 19(12): 13782-13798. doi: 10.3934/mbe.2022642 |
[10] | Yalong Yang, Zhen Niu, Liangliang Su, Wenjing Xu, Yuanhang Wang . Multi-scale feature fusion for pavement crack detection based on Transformer. Mathematical Biosciences and Engineering, 2023, 20(8): 14920-14937. doi: 10.3934/mbe.2023668 |
Strip steel is widely used in industrial production, including automobile, electromechanical, aerospace, ship and so on. Fundamentally speaking, there are inherent problems in the quality of strip steel, which will not only affect the beauty and comfort of products, but also these areas are usually the starting point of physical damage or chemical corrosion, which also has an adverse impact on the quality and service performance. The main defects of strip steel surface products are poor appearance, quality and use safety. Therefore, effective, rapid and accurate detection of surface defects is the primary problem in the iron and steel industry. At present, the whole manufacturing industry pays more and more attention to the surface defect detection technology, so as to find and effectively control the product quality in time, infer the causes of defects according to the detection results, and improve the production process, so as to reduce or eliminate the occurrence of defects.
The early detection methods of surface defects are mainly based on the manual inspection techniques, which have low efficiency and high cost. Recently, automatic defect inspection (ADI) technology methods based on machine learning have developed rapidly. ADI method not only has higher detection efficiency and accuracy, but also significantly reduces the human and financial resources. Despite this, it is still a very challenging task for ADI to identify the intrinsic and diverse defects in steel. The varieties of strip steel surface defects are shown in Figure 1. The surface defects of strip steel mainly have the following three characteristics, which make it difficult for surface detect detection.
1) Low contrast quality. Surface defect images are usually captured by CDD cameras. However, the environment for the image acquisition of surface defects are affected by light and dust, which resulting in low contrast between background and defects, as shown in Figure 1(a). This case increases the difficulty in defect detection.
2) Different textural and geometric structures. Generally, the defect images collected from different materials exhibit diverse textures. The Figure 1(b) depicts differences in texture features in same type of defects in different materials, where the boundary of defects is fuzzy and irregular. These factors also increase the difficulty in surface defect detection.
3) Diversity of defects. Surface defects always include many categories like inclusion, patches and scratches in which, some features are obvious while others are ambiguous. Further, the defects of the same category invariably show significant differences in appearance, while some defects of different categories have great similarities in appearance, as shown in Figure 1(c). These factors further improve the difficulty in detection process.
To address the above challenges, local binary pattern was applied for surface defects detection [1,2]. Djukic et al. [3] distinguished real defects from random noise pixels by dynamic threshold processing. An entity sparsity pursuit approach was also proposed for surface defects inspection [4]. Neogi et al. [5] suggested a global adaptive percentile thresholding of gradient images, which segment the defect regions and retain the characteristics of the defect without considering the size of the defect. In [6], a Gabor filter combination is proposed to detect the tiny holes on steel slabs. Li et al. [7] proposed an unsupervised approach based on a small number of flawless samples to detect and locate defects in random colour texture. On the other hand, Cohen et al. [8] connected the Markov with Gaussian distribution, and proposed Gaussian Markov Random Field to model the texture image of a non-defective fabric texture. However, all these methods are designed to identify defect detection by designing some artificial features, which lack generality.
Recently, especially CNN based methods are outstanding in the field of machine vision. These methods can automatically extract target features, find the internal feature relationship and law in the sample through iterative optimization, adaptively learn image features and complete object detection tasks, and solve the shortcomings of low efficiency and low detection accuracy of manual design features. A semi-supervised approach based on CNN was used to classify the strip steel surface defect [9]. Since the industrial defect images are difficult to collect, Natarajan et al. [10] adopted transfer learning to extract multi-level features and then input these features into SVM classifiers to avoid the over fitting caused by the small samples. However, the accuracy of these methods needs further improvement.
In this work, a pixel-wise detection framework based on CNN for strip steel surface defect detection is proposed to obtain multi-scale context information from high-level features by different sizes of convolution kernels. A cross integration is adopted to realize the effective utilization of these context information and to decode the information, which realizes the feature information complementation. The output of the framework is accurate pixel-wise classification and location. The main contributions of this study are:
● A pixel-wise detection framework based on CNN for strip steel surface defect detection is introduced. The output of the detection framework is the pixel-wise binary saliency maps of defect regions, which can effectively evaluate the quality of strip steel products.
● A contextual weighting module is proposed, which uses convolutional kernels with different size to obtain multi-scale context feature information from the convolution layers to achieve overall perception of the defect.
● In the decoder module, the cross integration is used to integrate the context information and previous decoded information into the current decoding block to realize feature information complementation.
● The proposed method is tested on the NEU-strip steel surface defect dataset, and the experimental results prove the effectiveness of the proposed method.
In this section, two kinds of detection methods for surface defect will be introduced, including: i) traditional approaches; ii) deep learning-based approaches.
The traditional methods for surface defect detection mainly include three categories: the statistical-based approaches, the filter-based approaches and the model-based approaches.
These methods use random phenomenon to analysis the distribution of random variables from the perspective of statistics, so as to realize the description of the image texture. Neogi et al. [5] proposed a global adaptive percentile thresholding of gradient images, which segment the defect regions and retain the characteristics of the defect without considering the size of the defect. Win et al. [11] proposed two thresholding methods namely, contrast-adjusted Otsu's method and contrast-adjusted median-based Otsu's method for automated defect detection system. Ricci et al. [12] used canny operator to detect the defect edges. Hu et al. [13] used Fourier shape descriptors for description of outline features in steel surface defects. Zhao et al. [14] proposed a two-level labelling technique based on super pixels. This method clustered pixels into super pixels and then the super pixels into sub-regions. Wang et al. [15] extracted and fused features of co-occurrence matrix and the histogram of oriented gradient to describe the local and the global texture characteristics, respectively. Chu et al. [16] proposed a smoothed local binary patterns by applying weight on the local neighbourhood. Fekri-Ershad et al. [17] applied a new noise-resistant and multi-resolution version of the LBP to extract jointly the colour and texture features jointly. Song et al. [1] proposed an adjacent evaluation completed local binary patterns against noise for defect inspection. Zhang et al. [18] used gray level co-occurrence matrix (GLCM) and HU invariant moments for feature extraction, and then applied adaptive genetic algorithm for feature selection.
The principle of this method was to transform the original image in frequency domain, and then use the corresponding filter to consider the image and to remove the features with low noise and correlation, so that the algorithm can extract more valuable information. Ai et al. [19] adopted kernel locality preserving projections and curvelet transform extract feature for the surface longitudinal cracks detection of the slabs. In [6,20], a Gabor filter combination is proposed to detect the tiny holed on steel slabs. Other method [On the other hand, Choi et al. [21] adopted two Gabor filters to detect the seam cracks on the steel plates, which have high detection performance and can effectively reduce noise. Wu et al. [22] used modular maximum of inter scale correlation of wavelet coefficient to determine the positions of the defects, and then used the prior knowledge about the characteristics of the surface defect defects for their classification. Öztürk et al. [23] proposed novel BiasFeed cellular neural network model for glass defect inspection. Li et al. [24] proposed a second-order derivative and morphology operations, the row-by-row adaptive thresholding, and 2-D wavelet transform to process the images showing different defects of the castings. Liu et al. [25] applied a non-subsampled shearlet transform and the kernel locality preserving projection to the surface defect detection. Akdemir et al. [26] adopted wavelet transforms to glass surface defects detection.
These methods are based on the construction model of the image, and uses the statistics of model parameters as texture features. Different textures are expressed as different values of model parameters under some assumptions. In [7], an unsupervised approach based on a small number of flawless samples was used to detect and locate the defects in random color texture. Cohen et al. [27] connected Markov with Gaussian distribution, and proposed Gaussian Markov Random Field to model the texture image of a non-defective fabric texture. Song et al. [28] proposed a saliency propagation algorithm based on multiple constraints and improved texture features (MCITF) for surface defect detection.
Recently, deep learning based on CNN approaches have achieved outperformed in the field of machine vision tasks. Many scholars have solved the problem of industrial defect detection by deep learning. In [9], a semi-supervised approach based on CNN was used to classify the strip steel surface defect. Since the industrial defect images are difficult to collect, Natarajan et al. [10] adopted transfer learning to extract the multi-level features and then input these features into SVM classifiers to avoid the over fitting caused by small samples. Masci et al. [29] proposed a Multi-scale pyramidal pooling network for generic steel defect classification. He et al. [30] proposed a multi-group convolutional neural network (MG-CNN) to inspect the defects of the steel surface. In [31], an end-to-end detection framework was proposed, which integrated multi-level features to complete the detection of the strip steel surface defect. The output of the network located the defect areas through some dense bounding boxes and gave the category name to these defects. Kou et al. [32] developed an end-to-end defect detection model based on YOLO-V3 for the surface defect detection on strip steel. In [33], a pre-trained deep learning network is used to extract multi-scale features from raw image patches to achieve image classification and defect segmentation. In [34], a multi-scale feature-clustering-based fully convolutional was proposed for the texture surface defect detection. Neven et al. [35] proposed a multi-branch U-Net for steel surface defect type and severity segmentation. Zhou et al. [36] proposed edge-aware multi-level interactive network for salient object detection of strip steel surface defects. Song et al. [37] adopted encoder-decoder residual network for salient object detection of strip steel surface defects. Dong et al. [38] proposed a pyramid feature fusion and global context attention network for automated surface defect segmentation. Although these methods achieved outstanding performance in the defects detection, they still need to be improved especially, in the feature extraction and utilization. Unlike previous studies, this paper proposes a pixel-wise detection framework based on CNN for strip steel surface defect detection.
The surface defect inception is formulated in this work as a pixel-wise segmentation task. Given a defect image, the proposed framework outputs a binary map, the defect area is represented by "1", while the non-defect area is represented by "0". The architecture of the framework mainly includes three parts: an encoder, the contextual weighting module and a decoder as shown in Figure 2.
Given a defect image, the framework first extracts the multi-level features from fine, shallow layers (enc1) to coarse, deep layers (enc5) by a pre-trained VGG-16 [39] network which is called an encoder module. The encoder module is composed of convolution layers and max pooling layers. In order to retain the spatial information of each pixel, the fully connection layers of VGG-16 network is removed. Subsequently, a contextual weighting module is adopted to obtain multi-scale contextual information from the high-level features to keep the shape and size in variance of the final features. In the encoder, the features extracted from enc3, enc4 and enc5 are considered as high-level features. In the decoder, the output of each con-textual weighting network is fused to the input of the same decoder in a feedback fashion. The final output of the decoder is a defect binary saliency map.
The encoder is used to extract multi-level features of the defect images, which is built on the pre-trained VGG-16 network. The encoder module mainly consists of 5 convolution layers and 4 max pooling layers. The details of the encoder module, i.e. blocks encx where, x = 1, …, 5 are listed in Table 1. In the encoder, the convolutional layer performs sliding on the input local areas through a series of convolutional kernels to obtain the features of the input image, followed by ReLU and BN. Let T={(Xn,Zn),n=1,...,N} represents training data, where Xn={xni,i=1,⋅⋅⋅,|Xn|} denotes the input image and Zn={zni,i=1,⋅⋅⋅,|Zn|} is the corresponding ground truth for Xn. The convolution of Xn is as follows:
C=σ(Wxni+b) | (1) |
Stage | Template | ||
enc1 | conv 3 × 3, stride = 1, D = 64, BN + ReLU | max_pooling 2 × 2, stride = 2 | ×2 |
enc2 | conv 3 × 3, stride = 1, D = 128, BN + ReLU | max_pooling 2 × 2, stride = 2 | ×2 |
enc3 | conv 3 × 3, stride = 1, D = 256, BN + ReLU | max_pooling 2 × 2, stride = 2 | ×2 |
enc4 | conv 3 × 3, stride = 1, D = 512, BN + ReLU | max_pooling 2 × 2, stride = 2 | ×2 |
enc5 | conv 3 × 3, stride = 1, D = 512, BN + ReLU | max_pooling 2 × 2, stride = 2 | ×2 |
where, W denotes weights, b refers bias, and σ represents the ReLU activation. By sliding the convolution kernels to obtain the feature sets. The pooling layers adopt 2 × 2 pool filter to down-scale the input feature maps, which is to change the spatial dimension and reduce the amount of calculation. The output of pooling layer is given below:
ˆC=pool(C) | (2) |
where pool denotes the max pooling with 2×2 pool filter and stride 2. The encoder finally generates five resolution feature maps F = {f1, f2, …, f5}, and f1 denotes the enc1 features and so on.
The fusion of convolutional features obtained from different stages is a common mechanism in most detection methods, because these features not only contain low-level visual information, but also include high-level abstract information. The earlier methods [40,41] combine these features directly from bottom to top. However, this simple combination may induce some bad features in the images to be integrated into the final prediction. To address this issue, a contextual weighting module, inspired by [42], is proposed in Figure 3. The CWM applies different convolution kernels to extract multi-scale contextual information from high-level features, which provides entire description for interpretation of the whole scene especially, multi-scale and multi shape objects. In the CWM, the features f3, f4 and f5 are used as high-level features. CWM used four stacked convolutional kernels (1×1, 3×3, 5×5, 7×7) to obtain multi-scale contextual information from the high-level features, and each kernel generates a feature map with the size of high-level features. For high-level feature f3, the output multi-scale contextual information can be denoted by F3:
M13=BN(σ(W1×1f3+b)) | (3) |
M33=BN(σ(W3×3f3+b)) | (4) |
M53=BN(σ(W5×5f3+b)) | (5) |
M73=BN(σ(W7×7f3+b)) | (6) |
Where BN denotes Batch Normalization, σ is nonlinear activation function ReLU. Wi×i denote the i × i convolutional kernel. The size of each generated features Mi3 (i = 1, 3, 5, 7) is the same as that of f3, and the number of channels is 32.
Then these feature maps are fused by concatenation. After that, 1×1 convolutional kernels are used to resize the channel of concatenated features to reduce the computation of the contextual weighting. The output saliency map G3 is formulated as:
G3=BN(σ(W1×1CAT(M13+M33+M53+M73)+b)) | (7) |
Where BN denotes Batch Normalization, σ is nonlinear activation function ReLU, CAT denotes concatenation. W1×1 is 1×1 convolutional kernel with 128 channels. The number of channels of G3 is 128.
For high-level feature f4 and f5, the model generates G4 and G5 in the same way as G3.
In this section, a novel decoder module is proposed, which includes 4 blocks (dec2, dec3, dec4, dec5), as shown in Figure 2. The dec3 and dec4 are fusion decoders, which are composed of the former one or two decoders and the output from contextual weighting module connected with enc3 and enc4, respectively. To enable effective fusion of these features, which must ensure that they have the same dimensionality. Firstly, a series of 3×3×D convolution kernels are applied to reduce channel dimension of these fused feature maps, where D is 32. Then a bilinear interpolation is applied to upsample low-resolution features to the target spatial resolution of the features that will be fused. Subsequently, these feature maps are fused by element-wise concatenation, as shown in Figure 4. The output decx is defined as follows:
decx={up×2(decx+1)x=2CAT(up×4(ϕ(ch(decx+2;θ))),up×2(ϕ(ch(decx+1;θ))),ϕ(ch(Gx;θ)))x=3CAT(up×2(ϕ(ch(decx+1;θ))),ϕ(ch(Gx;θ)))x=4 | (8) |
The final prediction Yp is formulated as:
Yp=up×2(W1×1∗dec2+b) | (9) |
where, CAT refers concatenation, up denotes upsample, ϕ represents the ReLU activation and ch is 3×3 convolution.
Loss function is the most basic and key factor in machine learning, which is used to measure the quality of model prediction. In this paper, three losses are applied to optimize the model. The final loss is defined as:
L=lBCE+lIoU+lSSIM | (10) |
where lBCE, lIoU and lSSIM represent the BCE loss, IoU loss and SSIM loss, respectively.
The BCE [43] loss is applied to compute the similarity between the prediction and ground truth, which is defined as:
lBCE=−∑TlogP−∑(1−T)log(1−P) | (11) |
where T ∈[0, 1] denotes the ground truth, and P∈[0, 1] is the predicted probability.
The IOU [44] loss is used to measure the repeatability between the prediction and the ground truth, which is defined as:
lIoU=1−∑TP∑[P+T−PT] | (12) |
where T ∈[0, 1] denotes the ground truth, and P∈[0, 1] is the predicted probability.
The SSIM [45] are originally applied to measure the structural similarity of two images. Let p={pi=1,⋅⋅⋅,N2} and t={ti=1,⋅⋅⋅,N2} represent the pixel values of two corresponding patches (size: N × N) cropped from the prediction P and ground truth T, respectively. The SSIM is computed as:
lIoU=1−(2vpvt+C1)(2σpt+C2)(v2p+v2t+C1)(σ2p+σ2t+C2) | (13) |
where vp and vt are the mean of p and t, respectively. σ2p and σ2t are the variance of p and t, respectively. σpt is their covariance. C1 and C2 are small constants that are applied to avoid dividing by zero.
This section mainly consists of six experimental parts: the details of implementation, the dataset and the evaluation metrics, the performance of the proposed method and other previous methods, followed by the ablation study and analysis of failure cases.
The proposed method is implemented based on TensorFlow [46] framework. The weights of new convolution layers in the framework are initialized with standard deviation 0.01 and biases are initialized to 0. The weights of backbone network are initialized using pre-trained ImageNet [47] network. The momentum and weight decay are set to 0.9 and 0.0005, respectively. The initial learning rate is set to 5e-5, which decreased by 10 after 10 epochs. The framework is trained for 300 epochs in total.
In the experiment of this study, three kinds of surface defect of strip steel [1] are selected, including Scratches, Patches, and Inclusion, as shown in Figure 5. All categories of defects are considered as detection targets. In the dataset, the training set includes 3630 defect samples, and the test set includes 792 defect samples. All the samples are resized to 256×256 during in the process of training network.
To evaluate the proposed framework, four metrics are used along with other previous state-of-the-art approaches, namely precision-recall (PR) curves, F-measure score and mean absolute error (MAE). The PR curve demonstrates the average recall and precision and of saliency maps at different thresholds, formulated as follows:
Recall=TPTP+FN | (14) |
Precision=TPTP+FP | (15) |
where FN, FP and TP indicate correctly the number judge of false negative pixels, false positive and true positive, respectively. F-measure, refers Fβ and is computed by weighted harmonic mean of recall and precision under nonnegative weight β, which defined as:
Fβ=(1+β2)Precision×Recallβ2×Precision+Recall | (16) |
the β2 = 0.3 is used in other methods.
MAE [48] is used to calculate the mean absolute error between the ground truth and the prediction. First, the prediction and the ground truth are binarized. Then, the MAE score is computed by:
MAE=1W×HW∑x=1H∑y=1|P(x,y)−S(x,y)| | (17) |
where P and S refer the prediction and the ground truth, respectively, while H and W are the height and width of images, respectively.
In this subsection, the proposed method is compared with 10 previous state-of-the-art methods, including BSCA [49], FT [50], MIL [51], RC [52], SMD [53], FCN [40], UNet [41], DN [54], DHSNet [55] and DSS [56], all the compared are pixel-wised method. For the sake of comparison, the same evaluation metrics and code are used to evaluate the output prediction maps.
Qualitative comparison results are shown in Figure 5, the proposed method can accurately detect defects and highlight them evenly in various challenging cases, i) low contrast quality between the defect region and background (e.g., row 1 and 3); ii) different textural and geometric structures (e.g., row 4 and 5); iii) diversity of defects (e.g., row 6, 7 and 8). For low contrast quality: some methods are missing or detecting a rough defect area which cannot express the defects vividly. For different textural and geometric structures, the defect region detected by methods are with little noise and not obvious. For diversity of defects, some methods cannot detect all categories of defects. The detection effect of deep learning-based methods is better than that of the traditional methods. However, for some minor defects, FCN, UNet, DN, DSS and DHSNet are either missing or incomplete detection areas. Instead, the proposed approach not only can distinguish the defect area and background effectively under low contrast, but also locate and detect the defects in different positions, scales and shapes accurately.
The advantages of the proposed method are shown in Figure 6. The method achieves outstanding performance among all the compared methods on strip steel surface defect dataset in terms of all evaluation metrics. It further improves the P-R curve and F-measure, and reduces MAE significantly. As listed in Table 2, the proposed method outperforms the competitive methods in Fβ and MAE. Compared with the traditional methods, Fβ is improved 36.12%, and decreased by 12.08% in MAE. Compared with deep learning method, the Fβ is improved by 0.38%, and decreased by 0.02% in MAE. The comparison of the above qualitative and quantitative analysis further proves the effectiveness of this method.
Method | BSCA[49] | FT[50] | MIL[51] | RC[52] | SMD[53] | FCN[40] | UNet[41] | DN[54] | DHSNet[55] | DSS[56] | My |
MAE↓ | 0.2462 | 0157. 7 | 0.1764 | 0.1228 | 0.1913 | 0.0604 | 0.0643 | 0.0481 | 0.0399 | 0.0371 | 0.0369 |
Fβ | 0.3404 | 0.4462 | 0.4873 | 0.3719 | 0.4665 | 0.6788 | 0.6783 | 0.7272 | 0.8447 | 0.8051 | 0.8485 |
In this work, the ablation study on the proposed CWN to verify the effectiveness of CWN. First, the CWN is removed, and directly combined the feature maps output from the encoder with the decoded feature maps through dense short connections, and output the optimal model after training the overall network. The ablation study further add the CWN module into the proposed method, and output the trained model after the same training. Finally, the two trained models are tested separately and output saliency prediction maps. As listed in Table 3, the contextual weighting module get declines by 0.21% in MAE and improve the performance by 0.48% in Fβ. These results prove the effectiveness of contextual weighting module to in the framework. In addition, the ablation study on the loss function to verify the effectiveness of the loss function. As listed in Table 3, the loss function get declines by 1.21% in MAE and improve the performance by 14.60% in Fβ.
Method | MAE | Fβ |
CMN−+ lBCE | 0.0511 | 0.6977 |
CMN++lBCE | 0.0490 | 0.7025 |
CMN++L | 0.0369 | 0.8485 |
The results of this study show that the proposed method is outstanding over the previous state-of-the-art methods on the strip steel surface defect dataset. However, some defect images still pose challenges to these methods. The images (c) and (d) of Figure 7 show that the detection of some defect images are lack of integrity. The images (a), (b), (e) and (f) show that some defects are missed. Figure 7 shows the reasons leading to failure detection are attributed to some defects are too small to be detected; some defects show low contrast, so it is difficult to judge whether they really are defects, and in some cases, the characteristics of some defect areas apparently change. In the future, I plan to focus on solving these problems.
In this paper, a pixel-wise inspection framework based on CNN for the surface defect inspection of strip steel is proposed. Firstly, the encoder of the framework is built on the pre-trained VGG-16 network, which is used to extract multi-level features. Next, the contextual weighting module uses convolutional kernels with different size to obtain multi-scale context feature information from the convolution layers, which achieve overall perception of defect. Finally, in the decoder module, the cross integration is used to integrate the context information and previously decoded information into the current decoding block, which realizes the feature information complementation. The experiments of this study demonstrate that the proposed method is outstanding over the previous state-of-the-art methods in detection of strip steel defect dataset. To sum up, the proposed method can detect defects accurately, which makes the network strong robust and effective in defect detection. In the future, I will further optimize the algorithm model.
This work is supported by the National Key R & D Program of China with No.2018YFB1800402.
The authors declared that they have no conflicts of interest in this work.
[1] | DOE (2016) Strategic Plan for a Thriving and Sustainable Bioeconomy. 56. |
[2] |
Lin L, Xu F, Ge X, et al. (2018) Improving the sustainability of organic waste management practices in the food-energy-water nexus: A comparative review of anaerobic digestion and composting. Renew Sustain Energy Rev 89: 151-167. doi: 10.1016/j.rser.2018.03.025
![]() |
[3] | Daher B, Mohtar R (2012) Water, energy, and food: The Ultimate Nexus, Encyclopedia of Agricultural, Food, and Biological Engineering, Second Edition. |
[4] |
Albrecht TR, Crootof A, Scott CA (2018) The Water-Energy-Food Nexus: A systematic review of methods for nexus assessment. Environ Res Lett 13: 043002. doi: 10.1088/1748-9326/aaa9c6
![]() |
[5] |
Popp J, Lakner Z, Harangi-Rákos M, et al. (2014) The effect of bioenergy expansion: Food, energy, and environment. Renew Sustain Energy Rev 32: 559-578. doi: 10.1016/j.rser.2014.01.056
![]() |
[6] | Gomo FF, Macleod C, Rowan J, et al. (2018) Supporting better decisions across the nexus of water, energy and food through earth observation data: \hack\break case of the Zambezi basin. Proc Int Assoc Hydrol Sci 376: 15-23. |
[7] | Belmonte BA, Benjamin MFD, Tan RR (2017) Biochar systems in the water-energy-food nexus: the emerging role of process systems engineering. Biotechnol Bioprocess Eng Process Syst Eng 18: 32-37. |
[8] | Hansen S, Mirkouei A (2019) Bio-Oil Upgrading Via Micro-Emulsification And Ultrasound Treatment: Examples For Analysis And Discussion, ASME 2019 International Design Engineering Technical Conferences & Computers and Information in Engineering Conference. |
[9] | Hansen S, Mirkouei A, Diaz LA (2020) A Comprehensive State-of-Technology Review for Upgrading Bio-oil to Renewable or Blended Hydrocarbon Fuels. Renew Sustain Energy Rev in press. |
[10] |
Roy P, Dias G (2017) Prospects for pyrolysis technologies in the bioenergy sector: A review. Renew Sustain Energy Rev 77: 59-69. doi: 10.1016/j.rser.2017.03.136
![]() |
[11] | Mirkouei A, Haapala KR (2015) A network model to optimize upstream and midstream biomass-to-bioenergy supply chain costs, ASME 2015 International Manufacturing Science and Engineering Conference (MSEC), MSEC2015-9355. |
[12] | Mirkouei A (2016) Techno-Economic Optimization and Environmental Impact Analysis for a Mixed-Mode Upstream and Midstream Forest Biomass to Bio-Products Supply Chain. |
[13] |
Cha JS, Park SH, Jung S-C, et al. (2016) Production and utilization of biochar: A review. J Ind Eng Chem 40: 1-15. doi: 10.1016/j.jiec.2016.06.002
![]() |
[14] |
Krajačić G, Vujanović M, Duić N, et al. (2018) Integrated approach for sustainable development of energy, water and environment systems. Energy Convers Manag 159: 398-412. doi: 10.1016/j.enconman.2017.12.016
![]() |
[15] | Hugh McLaughlin (2016) An Overview of the current Biochar and Activated Carbon Markets : Biofuels Digest, 2016. Available from: https://www.biofuelsdigest.com/bdigest/2016/10/11/an-overview-of-the-current-biochar-and-activated-carbon-markets/. |
[16] | Mirkouei A (2019) Cyber-Physical Real-time Monitoring and Control for Biomass-based Energy Production, Emerging Frontiers in Industrial and Systems Engineering: Growing Research and Practice, Taylor & Francis. |
[17] | Hansen S, Mirkouei A (2018) Past Infrastructures and Future Machine Intelligence (MI) for Biofuel Production: A Review and MI-Based Framework. ASME 2018 Int Des Eng Tech Conf Comput Inf Eng Conf V004T05A022. |
[18] | Mirkouei A, Kardel K (2017) Enhance Sustainability Benefits Through Scaling-up Bioenergy Production from Terrestrial and Algae Feedstocks, Proceedings of the 2017 ASME IDETC/CIE: 22nd Design for Manufacturing and the Life Cycle Conference. |
[19] | Mirkouei A, Haapala KR, Murthy GS, et al. (2017) Evolutionary Optimization of Bioenergy Supply Chain Cost with Uncertain Forest Biomass Quality and Availability, Proceedings of the 2016 Industrial and Systems Engineering Research Conference H. Yang, Z. Kong, and MD Sarder, eds., May 21-24, Anaheim, California, USA. |
[20] | Mirkouei A, Haapala K (2014) Integration of machine learning and mathematical programming methods into the biomass feedstock supplier selection process, Proc. 24th Int. Conf. Flex. Autom. Intell. Manuf. FAIM May, 20-23. |
[21] |
Biggs EM, Bruce E, Boruff B, et al. (2015) Sustainable development and the water-energy-food nexus: A perspective on livelihoods. Environ Sci Policy 54: 389-397. doi: 10.1016/j.envsci.2015.08.002
![]() |
[22] | Wallington K, Cai X (2017) The Food-Energy-Water Nexus: A Framework to Address Sustainable Development in the Tropics. Trop Conserv Sci 10: 1940082917720665. |
[23] | GVR (2017) Biochar Market Size, 2017. Available from: https://www.marketwatch.com/press-release/biochar-market-size-worth-31461-million-by-2025-cagr-132-grand-view-research-inc-2018-05-30. |
[24] |
Jeffery S, Verheijen FGA, van der Velde M, et al. (2011) A quantitative review of the effects of biochar application to soils on crop productivity using meta-analysis. Agric Ecosyst Environ 144: 175-187. doi: 10.1016/j.agee.2011.08.015
![]() |
[25] |
Atkinson CJ, Fitzgerald JD, Hipps NA (2010) Potential mechanisms for achieving agricultural benefits from biochar application to temperate soils: a review. Plant Soil 337: 1-18. doi: 10.1007/s11104-010-0464-5
![]() |
[26] |
Ahmad M, Rajapaksha AU, Lim JE, et al. (2014) Biochar as a sorbent for contaminant management in soil and water: A review. Chemosphere 99: 19-33. doi: 10.1016/j.chemosphere.2013.10.071
![]() |
[27] |
Liu W-J, Jiang H, Yu H-Q (2015) Development of Biochar-Based Functional Materials: Toward a Sustainable Platform Carbon Material. Chem Rev 115: 12251-12285. doi: 10.1021/acs.chemrev.5b00195
![]() |
[28] |
Das O, Sarmah AK, Bhattacharyya D (2015) A sustainable and resilient approach through biochar addition in wood polymer composites. Sci Total Environ 512-513: 326-336. doi: 10.1016/j.scitotenv.2015.01.063
![]() |
[29] |
Carpenter AW, de Lannoy C-F, Wiesner MR (2015) Cellulose Nanomaterials in Water Treatment Technologies. Environ Sci Technol 49: 5277-5287. doi: 10.1021/es506351r
![]() |
[30] |
Mousa E, Wang C, Riesbeck J, et al. (2016) Biomass applications in iron and steel industry: An overview of challenges and opportunities. Renew Sustain Energy Rev 65: 1247-1266. doi: 10.1016/j.rser.2016.07.061
![]() |
[31] | Nemati M, Simard F, Fortin J-P, et al. (2015) Potential Use of Biochar in Growing Media. Soil Sci Soc Am J 14. |
[32] | Berry M, Seppalaomem O (2014) OSUAA_Biochar_MarketStudy_6_6_14.pdf. |
[33] | Delaney M (2015) Northwest Biochar commercialization strategy paper, U.S. Forest Service and the Oregon Department of Forestry. |
[34] | Ferroukhi R, Nagpal D, Alvaro Lopez-Peña, et al. (2015) Renewable Energy in the Water, Energy and Food Nexus, IREA. |
[35] | Jha P, Biswas AK, Lakaria BL, et al. (2010) Biochar in agriculture - prospects and related implications. Curr Sci 99: 1218-1225. |
[36] | Jeffery S, Bezemer TM, Cornelissen G, et al. (2013) The way forward in biochar research: targeting trade-offs between the potential wins. GCB Bioenergy 7: 1-13. |
[37] |
Gwenzi W, Musarurwa T, Nyamugafata P, et al. (2014) Adsorption of Zn2+ and Ni2+ in a binary aqueous solution by biosorbents derived from sawdust and water hyacinth (Eichhornia crassipes). Water Sci Technol 70: 1419. doi: 10.2166/wst.2014.391
![]() |
[38] |
Yoo G, Kim H, Chen J, et al. (2014) Effects of Biochar Addition on Nitrogen Leaching and Soil Structure following Fertilizer Application to Rice Paddy Soil. Soil Sci Soc Am J 78: 852-860. doi: 10.2136/sssaj2013.05.0160
![]() |
[39] |
Bucheli TD, Bachmann HJ, Blum F, et al. (2014) On the heterogeneity of biochar and consequences for its representative sampling. J Anal Appl Pyrolysis 107: 25-30. doi: 10.1016/j.jaap.2014.01.020
![]() |
[40] | Gerlach A, Schmidt HP (2014) The use of biochar in cattle farming. Biochar J 2014. |
[41] |
Xu R, Ferrante L, Hall K, et al. (2011) Thermal self-sustainability of biochar production by pyrolysis. J Anal Appl Pyrolysis 91: 55-66. doi: 10.1016/j.jaap.2011.01.001
![]() |
[42] |
Han J, Elgowainy A, Dunn JB, et al. (2013) Life cycle analysis of fuel production from fast pyrolysis of biomass. Bioresour Technol 133: 421-428. doi: 10.1016/j.biortech.2013.01.141
![]() |
[43] |
Agegnehu G, Srivastava AK, Bird MI (2017) The role of biochar and biochar-compost in improving soil quality and crop performance: A review. Appl Soil Ecol 119: 156-170. doi: 10.1016/j.apsoil.2017.06.008
![]() |
[44] | Biederman LA, Harpole WS (2012) Biochar and its effects on plant productivity and nutrient cycling: a meta-analysis. GCB Bioenergy 5: 202-214. |
[45] |
Agegnehu G, Nelson PN, Bird MI (2016) Crop yield, plant nutrient uptake and soil physicochemical properties under organic soil amendments and nitrogen fertilization on Nitisols. Soil Tillage Res 160: 1-13. doi: 10.1016/j.still.2016.02.003
![]() |
[46] |
Aldridge CA, Baker BH, Omer AR (2019) Investigation of short-term effects of winter cover crops on compaction and total soil carbon in a long-term no-till agricultural system. J Soil Water Conserv 74: 77-84. doi: 10.2489/jswc.74.1.77
![]() |
[47] |
Boateng AA, Mullen CA, Goldberg NM, et al. (2010) Sustainable production of bioenergy and biochar from the straw of high-biomass soybean lines via fast pyrolysis. Environ Prog Sustain Energy 29: 175-183. doi: 10.1002/ep.10446
![]() |
[48] |
Ali I (2014) Water Treatment by Adsorption Columns: Evaluation at Ground Level. Sep Purif Rev 43: 175-205. doi: 10.1080/15422119.2012.748671
![]() |
[49] |
Barber ST, Yin J, Draper K, et al. (2018) Closing nutrient cycles with biochar- from filtration to fertilizer. J Clean Prod 197: 1597-1606. doi: 10.1016/j.jclepro.2018.06.136
![]() |
[50] |
Shackley S, Hammond J, Gaunt J, et al. (2011) The feasibility and costs of biochar deployment in the UK. Carbon Manag 2: 335-356. doi: 10.4155/cmt.11.22
![]() |
[51] |
Sessions J, Smith D, Trippe KM, et al. (2019) Can biochar link forest restoration with commercial agriculture? Biomass Bioenergy 123: 175-185. doi: 10.1016/j.biombioe.2019.02.015
![]() |
[52] |
Rosas JG, Gómez N, Cara J, et al. (2015) Assessment of sustainable biochar production for carbon abatement from vineyard residues. J Anal Appl Pyrolysis 113: 239-247. doi: 10.1016/j.jaap.2015.01.011
![]() |
[53] | Shackley S, Carter S, Knowles T, et al. (2012) Sustainable gasification-biochar systems? A case-study of rice-husk gasification in Cambodia, Part I: Context, chemical properties, environmental and health and safety issues. Energy Policy 42: 49-58. |
[54] |
Qin K, Jensen PA, Lin W, et al. (2012) Biomass gasification behavior in an entrained flow reactor: gas product distribution and soot formation. Energy Fuels 26: 5992-6002. doi: 10.1021/ef300960x
![]() |
[55] |
Gwenzi W, Chaukura N, Noubactep C, et al. (2017) Biochar-based water treatment systems as a potential low-cost and sustainable technology for clean water provision. J Environ Manage 197: 732-749. doi: 10.1016/j.jenvman.2017.03.087
![]() |
[56] |
Zygourakis K (2017) Biochar soil amendments for increased crop yields: How to design a "designer" biochar. AIChE J 63: 5425-5437. doi: 10.1002/aic.15870
![]() |
[57] |
Suter GW (2013) Review papers are important and worth writing. Environ Toxicol Chem 32: 1929-1930. doi: 10.1002/etc.2316
![]() |
[58] |
Mirkouei A, Haapala KR, Sessions J, et al. (2017) A review and future directions in techno-economic modeling and optimization of upstream forest biomass to bio-oil supply chains. Renew Sustain Energy Rev 67: 15-35. doi: 10.1016/j.rser.2016.08.053
![]() |
[59] | Taticchi P, Garengo P, Nudurupati SS, et al. (2014) A review of decision-support tools and performance measurement and sustainable supply chain management. Int J Prod Res 1-22. |
[60] | WEF (2011) Water Security: the water-food-energy-climate nexus, Island Press. |
[61] | Franz M, Schlitz N, Schumacher KP (2017) Globalization and the water-energy-food nexus - Using the global production networks approach to analyze society-environment relations. Environ Sci Policy. |
[62] | Endo A, Tsurita I, Burnett K, et al. (2017) A review of the current state of research on the water, energy, and food nexus. Water Energy Food Nexus Asia-Pac Reg 11: 20-30. |
[63] | Venghaus S, Hake J-F (2018) Nexus thinking in current EU policies - The interdependencies among food, energy and water resources. Environ Sci Policy. |
[64] |
Bazilian M, Rogner H, Howells M, et al. (2011) Considering the energy, water and food nexus: Towards an integrated modelling approach. Energy Policy 39: 7896-7906. doi: 10.1016/j.enpol.2011.09.039
![]() |
[65] |
Leck H, Conway D, Bradshaw M, et al. (2015) Tracing the Water-Energy-Food Nexus: Description, Theory and Practice. Geogr Compass 9: 445-460. doi: 10.1111/gec3.12222
![]() |
[66] |
Al-Saidi M, Elagib NA (2017) Towards understanding the integrative approach of the water, energy and food nexus. Sci Total Environ 574: 1131-1139. doi: 10.1016/j.scitotenv.2016.09.046
![]() |
[67] | Bates B, Kundzewicz ZW, Wu S, et al. (Eds.) (2008) Climate Change and Water. Technical Paper of the Intergovernmental Panel on Climate Change, Geneva, IPCC Secretariat. |
[68] | Alexandratos N, Bruinsma J (2012) World agriculture towards 2030/2050: the 2012 revision. 160. |
[69] | Mateo-Sagasta J, Burke J (2008) Agriculture and water quality interactions: a global overview. |
[70] | Ongley ED (1996) Control of water pollution from agriculture; Chapter 1: Introduction to agricultural water pollution. |
[71] |
Wiedmann T (2018) Eutrophication's neglected drivers. Nat Sustain 1: 273-274. doi: 10.1038/s41893-018-0084-2
![]() |
[72] | Yue Y, Guo WN, Lin QM, et al. (2016) Improving salt leaching in a simulated saline soil column by three biochars derived from rice straw (Oryza sativa L.), sunflower straw (Helianthus annuus), and cow manure. J Soil Water Conserv 71: 467-475. |
[73] |
Bell JM, Schwartz R, McInnes KJ, et al. (2018) Deficit irrigation effects on yield and yield components of grain sorghum. Agric Water Manag 203: 289-296. doi: 10.1016/j.agwat.2018.03.002
![]() |
[74] | Wagner K (2012) Status and trends of irrigated agriculture in Texas. Spec Rep Tex Water Resour Inst Tex AM Univ Coll Stn Tex Tex Water Resour Inst. |
[75] |
Bordovsky JP, Mustian JT, Cranmer AM, et al. (2011) Cotton-grain sorghum rotation under extreme deficit irrigation conditions. Appl Eng Agric 27: 359-371. doi: 10.13031/2013.37079
![]() |
[76] |
Grafton RQ, Williams J, Perry CJ, et al. (2018) The paradox of irrigation efficiency. Science 361: 748-750. doi: 10.1126/science.aat9314
![]() |
[77] | Vadez V, Kholová J, Yadav RS, et al. (2013) Small temporal differences in water uptake among varieties of pearl millet (Pennisetum glaucum (L.) R. Br.) are critical for grain yield under terminal drought. Plant Soil 371: 447-462. |
[78] |
Compton M, Willis S, Rezaie B, et al. (2018) Food processing industry energy and water consumption in the Pacific northwest. Innov Food Sci Emerg Technol 47: 371-383. doi: 10.1016/j.ifset.2018.04.001
![]() |
[79] | Ralph E. H. Sims (2011) Energy-Smart Food for People and Climate. UN Food and Agriculture Organisation. |
[80] | FAO (2018) Food and Agriculture Organization of the United Nations, 2018.Available from: http://www.fao.org/home/en/. |
[81] |
Reganold JP, Wachter JM (2016) Organic agriculture in the twenty-first century. Nat Plants 2: 15221. doi: 10.1038/nplants.2015.221
![]() |
[82] |
Dong X, Vuran MC, Irmak S (2013) Autonomous precision agriculture through integration of wireless underground sensor networks with center pivot irrigation systems. Ad Hoc Netw 11: 1975-1987. doi: 10.1016/j.adhoc.2012.06.012
![]() |
[83] | Helu M, Hedberg T, Barnard Feeney A (2017) Reference architecture to integrate heterogeneous manufacturing systems for the digital thread. CIRP J Manuf Sci Technol. |
[84] | Vogl GW, Weiss BA, Helu M (2016) A review of diagnostic and prognostic capabilities and best practices for manufacturing. J Intell Manuf 1-17. |
[85] |
Wolfe ML, Ting KC, Scott N, et al. (2016) Engineering solutions for food-energy-water systems: it is more than engineering. J Environ Stud Sci 6: 172-182. doi: 10.1007/s13412-016-0363-z
![]() |
[86] |
Lehmann J, Gaunt J, Rondon M (2006) Bio-char Sequestration in Terrestrial Ecosystems - A Review. Mitig Adapt Strateg Glob Change 11: 403-427. doi: 10.1007/s11027-005-9006-5
![]() |
[87] | Napolitano G, Isaac J, Bizzarri G, et al. (2010) Bioenergy and Food Security. Food and Agriculture Organization of the United Nations. |
[88] | Khan Z, Linares P, García-González J (2017) Integrating water and energy models for policy driven applications. A review of contemporary work and recommendations for future developments. Renew Sustain Energy Rev 67: 1123-1138. |
[89] | Pate R, Hightower M, Cameron C, et al. (2007) Overview of Energy-Water Interdependencies and the emerging energy demands on Water Resources. |
[90] | Scott CA, Pierce SA, Pasqualetti MJ, et al. (2011) Policy and institutional dimensions of the water-energy nexus. Sustain Biofuels 39: 6622-6630. |
[91] |
Hamiche AM, Stambouli AB, Flazi S (2016) A review of the water-energy nexus. Renew Sustain Energy Rev 65: 319-331. doi: 10.1016/j.rser.2016.07.020
![]() |
[92] | Erik Mielke, Laura Diaz Anadon, Vankatesh Narayanamurti (2010) Water Consumption of Energy Resource Extraction, Processing, and Conversion, A review of the literature for estimates of water intensity of energy-resource extraction, processing to fuels,and conversion to electricity, Belfer Center for Science and International Affairs, Harvard University. |
[93] |
Bartos MD, Chester MV (2014) The Conservation Nexus: Valuing Interdependent Water and Energy Savings in Arizona. Environ Sci Technol 48: 2139-2149. doi: 10.1021/es4033343
![]() |
[94] |
Wu M, Mintz M, Wang M, et al. (2009) Water Consumption in the Production of Ethanol and Petroleum Gasoline. Environ Manage 44: 981. doi: 10.1007/s00267-009-9370-0
![]() |
[95] | Ernst KM, Preston BL (2017) Adaptation opportunities and constraints in coupled systems: Evidence from the U.S. energy-water nexus. Environ Sci Policy 70: 38-45. |
[96] |
Liu J, Hull V, Godfray HCJ, et al. (2018) Nexus approaches to global sustainable development. Nat Sustain 1: 466. doi: 10.1038/s41893-018-0135-8
![]() |
[97] |
Jägerskog A, Lindström A, Björklund G, et al. (2012) Regional Options for Addressing the Water, Energy and Food Nexus in Central Asia and the Aral Sea Basin AU - Granit, Jakob. Int J Water Resour Dev 28: 419-432. doi: 10.1080/07900627.2012.684307
![]() |
[98] |
Villarroel Walker R, Beck MB, Hall JW (2012) Water - and nutrient and energy - systems in urbanizing watersheds. Front Environ Sci Eng 6: 596-611. doi: 10.1007/s11783-012-0445-4
![]() |
[99] | Siddiqi A, Anadon LD (2011) The water-energy nexus in Middle East and North Africa. Crossroads Pathw Renew Nucl Energy Policy North Afr 39: 4529-4540. |
[100] | Ringler C, Bhaduri A, Lawford R (2013) The nexus across water, energy, land and food (WELF): potential for improved resource use efficiency? Aquat Mar Syst 5: 617-624. |
[101] | Lawford R, Bogardi J, Marx S, et al. (2013) Basin perspectives on the Water-Energy-Food Security Nexus. Aquat Mar Syst 5: 607-616. |
[102] |
Rasul G (2014) Food, water, and energy security in South Asia: A nexus perspective from the Hindu Kush Himalayan region☆. Environ Sci Policy 39: 35-48. doi: 10.1016/j.envsci.2014.01.010
![]() |
[103] | Stein C, Barron J, Nigussie L, et al. (2014) Advancing the water-energy-food nexus: social networks and institutional interplay in the Blue Nile, International Water Management Institute (IWMI). CGIAR Research Program. |
[104] |
Villarroel R, Beck MB, Hall JW, et al. (2014) The energy-water-food nexus: Strategic analysis of technologies for transforming the urban metabolism. J Environ Manage 141: 104-115. doi: 10.1016/j.jenvman.2014.01.054
![]() |
[105] |
Biggs EM, Bruce E, Boruff B, et al. (2015) Sustainable development and the water-energy-food nexus: A perspective on livelihoods. Environ Sci Policy 54: 389-397. doi: 10.1016/j.envsci.2015.08.002
![]() |
[106] |
Conway D, van Garderen EA, Deryng D, et al. (2015) Climate and southern Africa's water-energy-food nexus. Nat Clim Change 5: 837. doi: 10.1038/nclimate2735
![]() |
[107] | Daher BT, Mohtar RH (2015) Water-energy-food (WEF) Nexus Tool 2.0: guiding integrative resource planning and decision-making. Water Int 40: 748-771. |
[108] |
Jeswani HK, Burkinshaw R, Azapagic A (2015) Environmental sustainability issues in the food-energy-water nexus: Breakfast cereals and snacks. Sustain Prod Consum 2: 17-28. doi: 10.1016/j.spc.2015.08.001
![]() |
[109] |
Kraucunas I, Clarke L, Dirks J, et al. (2015) Investigating the nexus of climate, energy, water, and land at decision-relevant scales: the Platform for Regional Integrated Modeling and Analysis (PRIMA). Clim Change 129: 573-588. doi: 10.1007/s10584-014-1064-9
![]() |
[110] |
Mukuve FM, Fenner RA (2015) Scale variability of water, land, and energy resource interactions and their influence on the food system in Uganda. Sustain Prod Consum 2: 79-95. doi: 10.1016/j.spc.2015.07.009
![]() |
[111] |
Ozturk I (2015) Sustainability in the food-energy-water nexus: Evidence from BRICS (Brazil, the Russian Federation, India, China, and South Africa) countries. Energy 93: 999-1010. doi: 10.1016/j.energy.2015.09.104
![]() |
[112] |
Endo A, Burnett K, Orencio P, et al. (2015) Methods of the water-energy-food nexus. Water 7: 5806-5830. doi: 10.3390/w7105806
![]() |
[113] | Middleton C, Allouche J, Gyawali D, et al. (2015) The Rise and Implications of the Water-Energy-Food Nexus in Southeast Asia through an Environmental Justice Lens. |
[114] | Villamayor-Tomas S, Grundmann P, Epstein G, et al. (2015) The Water-Energy-Food Security Nexus through the Lenses of the Value Chain and the Institutional Analysis and Development Frameworks. 8: 21. |
[115] | Keskinen M, Someth P, Salmivaara A, et al. (2015) Water-Energy-Food Nexus in a Transboundary River Basin: The Case of Tonle Sap Lake, Mekong River Basin. |
[116] | Garcia DJ, You F (2016) The water-energy-food nexus and process systems engineering: A new focus. 12th Int Symp Process Syst Eng 25th Eur Symp Comput Aided Process Eng PSE-2015ESCAPE-25 31 May - 4 June 2015 Cph Den 91: 49-67. |
[117] |
Rasul G (2016) Managing the food, water, and energy nexus for achieving the Sustainable Development Goals in South Asia. Environ Dev 18: 14-25. doi: 10.1016/j.envdev.2015.12.001
![]() |
[118] |
De Laurentiis V, Hunt D, Rogers C (2016) Overcoming food security challenges within an energy/water/food nexus (EWFN) approach. Sustainability 8: 95. doi: 10.3390/su8010095
![]() |
[119] |
Cairns R, Krzywoszynska A (2016) Anatomy of a buzzword: The emergence of 'the water-energy-food nexus' in UK natural resource debates. Environ Sci Policy 64: 164-170. doi: 10.1016/j.envsci.2016.07.007
![]() |
[120] |
Yang YE, Wi S, Ray PA, et al. (2016) The future nexus of the Brahmaputra River Basin: climate, water, energy and food trajectories. Glob Environ Change 37: 16-30. doi: 10.1016/j.gloenvcha.2016.01.002
![]() |
[121] |
de Strasser L, Lipponen A, Howells M, et al. (2016) A methodology to assess the water energy food ecosystems nexus in transboundary river basins. Water 8: 59. doi: 10.3390/w8020059
![]() |
[122] |
Fasel M, Bréthaut C, Rouholahnejad E, et al. (2016) Blue water scarcity in the Black Sea catchment: Identifying key actors in the water-ecosystem-energy-food nexus. Environ Sci Policy 66: 140-150. doi: 10.1016/j.envsci.2016.09.004
![]() |
[123] |
Perrone D, Hornberger G (2016) Frontiers of the food-energy-water trilemma: Sri Lanka as a microcosm of tradeoffs. Environ Res Lett 11: 014005. doi: 10.1088/1748-9326/11/1/014005
![]() |
[124] |
Mortensen JG, González-Pinzón R, Dahm CN, et al. (2016) Advancing the Food-Energy-Water Nexus: Closing Nutrient Loops in Arid River Corridors. Environ Sci Technol 50: 8485-8496. doi: 10.1021/acs.est.6b01351
![]() |
[125] |
Wichelns D (2017) The water-energy-food nexus: Is the increasing attention warranted, from either a research or policy perspective? Environ Sci Policy 69: 113-123. doi: 10.1016/j.envsci.2016.12.018
![]() |
[126] | Endo A, Tsurita I, Burnett K, et al. (2017) A review of the current state of research on the water, energy, and food nexus. Water Energy Food Nexus Asia-Pac Reg 11: 20-30. |
[127] |
Howarth C, Monasterolo I (2017) Opportunities for knowledge co-production across the energy-food-water nexus: Making interdisciplinary approaches work for better climate decision making. Environ Sci Policy 75: 103-110. doi: 10.1016/j.envsci.2017.05.019
![]() |
[128] | Flammini A, Puri M, Pluschke L, et al. (2014) Walking the nexus talk: assessing the water-energy-food nexus in the context of the sustainable energy for all initiative, Rome, Climate, Energy and Tenure Division (NRC), Food and Agriculture Organization of the United Nations. |
[129] |
Pahl-Wostl C (2019) Governance of the water-energy-food security nexus: A multi-level coordination challenge. Environ Sci Policy 92: 356-367. doi: 10.1016/j.envsci.2017.07.017
![]() |
[130] |
El Gafy I, Grigg N, Reagan W (2017) Dynamic Behaviour of the Water-Food-Energy Nexus: Focus on Crop Production and Consumption. Irrig Drain 66: 19-33. doi: 10.1002/ird.2060
![]() |
[131] | Wicaksono A, Jeong G, Kang D (2017) Water, energy, and food nexus: Review of global implementation and simulation model development. |
[132] | Dhaubanjar S, Davidsen C, Bauer-Gottwein P (2017) Multi-Objective Optimization for Analysis of Changing Trade-Offs in the Nepalese Water-Energy-Food Nexus with Hydropower Development. Water 9. |
[133] |
Hussien WA, Memon FA, Savic DA (2017) An integrated model to evaluate water-energy-food nexus at a household scale. Environ Model Softw 93: 366-380. doi: 10.1016/j.envsoft.2017.03.034
![]() |
[134] |
Johnson OW, Karlberg L (2017) Co-exploring the Water-Energy-Food Nexus: Facilitating Dialogue through Participatory Scenario Building. Front Environ Sci 5: 24. doi: 10.3389/fenvs.2017.00024
![]() |
[135] |
White DJ, Hubacek K, Feng K, et al. (2018) The Water-Energy-Food Nexus in East Asia: A tele-connected value chain analysis using inter-regional input-output analysis. Appl Energy 210: 550-567. doi: 10.1016/j.apenergy.2017.05.159
![]() |
[136] | Albrecht TR, Crootof A, Scott CA (2018) The water-energy-food nexus: A comprehensive review of nexus-specific methods. Environ Res Lett. |
[137] | Siddiqi A, Anadon LD (2011) The water-energy nexus in Middle East and North Africa. Crossroads Pathw Renew Nucl Energy Policy North Afr 39: 4529-4540. |
[138] |
Villarroel Walker R, Beck MB, Hall JW (2012) Water - and nutrient and energy - systems in urbanizing watersheds. Front Environ Sci Eng 6: 596-611. doi: 10.1007/s11783-012-0445-4
![]() |
[139] | Ringler C, Bhaduri A, Lawford R (2013) The nexus across water, energy, land and food (WELF): potential for improved resource use efficiency? Aquat Mar Syst 5: 617-624. |
[140] |
Rasul G (2016) Managing the food, water, and energy nexus for achieving the Sustainable Development Goals in South Asia. Environ Dev 18: 14-25. doi: 10.1016/j.envdev.2015.12.001
![]() |
[141] |
Wichelns D (2017) The water-energy-food nexus: Is the increasing attention warranted, from either a research or policy perspective? Environ Sci Policy 69: 113-123. doi: 10.1016/j.envsci.2016.12.018
![]() |
[142] |
White DJ, Hubacek K, Feng K, et al. (2018) The Water-Energy-Food Nexus in East Asia: A tele-connected value chain analysis using inter-regional input-output analysis. Appl Energy 210: 550-567. doi: 10.1016/j.apenergy.2017.05.159
![]() |
[143] |
Qambrani NA, Rahman MdM, Won S, et al. (2017) Biochar properties and eco-friendly applications for climate change mitigation, waste management, and wastewater treatment: A review. Renew Sustain Energy Rev 79: 255-273. doi: 10.1016/j.rser.2017.05.057
![]() |
[144] |
Bruun EW, Müller-Stöver D, Ambus P, et al. (2011) Application of biochar to soil and N2O emissions: potential effects of blending fast-pyrolysis biochar with anaerobically digested slurry. Eur J Soil Sci 62: 581-589. doi: 10.1111/j.1365-2389.2011.01377.x
![]() |
[145] |
Laird DA (2008) The Charcoal Vision: A Win-Win-Win Scenario for Simultaneously Producing Bioenergy, Permanently Sequestering Carbon, while Improving Soil and Water Quality. Agron J 100: 178-181. doi: 10.2134/agronj2007.0161
![]() |
[146] |
Kuppusamy S, Thavamani P, Megharaj M, et al. (2016) Agronomic and remedial benefits and risks of applying biochar to soil: Current knowledge and future research directions. Environ Int 87: 1-12. doi: 10.1016/j.envint.2015.10.018
![]() |
[147] | Vanholme B, Desmet T, Ronsse F, et al. (2013) Towards a carbon-negative sustainable bio-based economy. Front Plant Sci 4: 174. |
[148] |
Tan Z, Lin CSK, Ji X, et al. (2017) Returning biochar to fields: A review. Appl Soil Ecol 116: 1-11. doi: 10.1016/j.apsoil.2017.03.017
![]() |
[149] |
Beesley L, Moreno-Jiménez E, Gomez-Eyles JL, et al. (2011) A review of biochars' potential role in the remediation, revegetation and restoration of contaminated soils. Environ Pollut 159: 3269-3282. doi: 10.1016/j.envpol.2011.07.023
![]() |
[150] | Lehmann J, Joseph S (2009) Biochar for Environmental Management: An Introduction. 12. |
[151] |
Mohan D, Pittman Charles U, Steele PH (2006) Pyrolysis of Wood/Biomass for Bio-oil: A Critical Review. Energy Fuels 20: 848-889. doi: 10.1021/ef0502397
![]() |
[152] |
Kong S-H, Loh S-K, Bachmann RT, et al. (2014) Biochar from oil palm biomass: A review of its potential and challenges. Renew Sustain Energy Rev 39: 729-739. doi: 10.1016/j.rser.2014.07.107
![]() |
[153] |
Qian K, Kumar A, Zhang H, et al. (2015) Recent advances in utilization of biochar. Renew Sustain Energy Rev 42: 1055-1064. doi: 10.1016/j.rser.2014.10.074
![]() |
[154] |
Shea EC (2014) Adaptive management: The cornerstone of climate-smart agriculture. J Soil Water Conserv 69: 198A-199A. doi: 10.2489/jswc.69.6.198A
![]() |
[155] | Tenenbaum DJ (2009) Biochar: Carbon Mitigation from the Ground Up. Environ Health Perspect 117: A70-A73. |
[156] | Liang B, Lehmann J, Solomon D, et al. (2006) Black Carbon Increases Cation Exchange Capacity in Soils. Soil Sci Soc Am J 70. |
[157] | Jeffery S, Bezemer TM, Cornelissen G, et al. (2013) The way forward in biochar research: targeting trade-offs between the potential wins. GCB Bioenergy 7: 1-13. |
[158] |
Taha SM, Amer ME, Elmarsafy AE, et al. (2014) Adsorption of 15 different pesticides on untreated and phosphoric acid treated biochar and charcoal from water. J Environ Chem Eng 2: 2013-2025. doi: 10.1016/j.jece.2014.09.001
![]() |
[159] |
Taha SM, Amer ME, Elmarsafy AE, et al. (2014) Adsorption of 15 different pesticides on untreated and phosphoric acid treated biochar and charcoal from water. J Environ Chem Eng 2: 2013-2025. doi: 10.1016/j.jece.2014.09.001
![]() |
[160] |
Liu J, Schulz H, Brandl S, et al. (2012) Short-term effect of biochar and compost on soil fertility and water status of a Dystric Cambisol in NE Germany under field conditions. J Plant Nutr Soil Sci 175: 698-707. doi: 10.1002/jpln.201100172
![]() |
[161] |
Van Zwieten L, Kimber S, Morris S, et al. (2010) Effects of biochar from slow pyrolysis of papermill waste on agronomic performance and soil fertility. Plant Soil 327: 235-246. doi: 10.1007/s11104-009-0050-x
![]() |
[162] | Kookana RS, Sarmah AK, Van Zwieten L, et al. (2011) Chapter three - Biochar Application to Soil: Agronomic and Environmental Benefits and Unintended Consequences, In: Sparks DL (Ed.), Advances in Agronomy, Academic Press, 103-143. |
[163] |
Major J, Rondon M, Molina D, et al. (2010) Maize yield and nutrition during 4 years after biochar application to a Colombian savanna oxisol. Plant Soil 333: 117-128. doi: 10.1007/s11104-010-0327-0
![]() |
[164] |
Mohammadi A, Cowie AL, Mai TLA, et al. (2017) Climate-change and health effects of using rice husk for biochar-compost: Comparing three pyrolysis systems. J Clean Prod 162: 260-272. doi: 10.1016/j.jclepro.2017.06.026
![]() |
[165] |
Mohammadi A, Cowie A, Mai TLA, et al. (2016) Biochar use for climate-change mitigation in rice cropping systems. J Clean Prod 116: 61-70. doi: 10.1016/j.jclepro.2015.12.083
![]() |
[166] |
Mohammadi A, Cowie A, Mai TLA, et al. (2016) Quantifying the greenhouse gas reduction benefits of utilising straw biochar and enriched biochar. Energy Procedia 97: 254-261. doi: 10.1016/j.egypro.2016.10.069
![]() |
[167] |
Agegnehu G, Bass AM, Nelson PN, et al. (2016) Benefits of biochar, compost and biochar-compost for soil quality, maize yield and greenhouse gas emissions in a tropical agricultural soil. Sci Total Environ 543: 295-306. doi: 10.1016/j.scitotenv.2015.11.054
![]() |
[168] |
Schimmelpfennig S, Glaser B (2012) One Step Forward toward Characterization: Some Important Material Properties to Distinguish Biochars. J Environ Qual 41: 1001-1013. doi: 10.2134/jeq2011.0146
![]() |
[169] | Kloss S, Zehetner F, Wimmer B, et al. (2013) Biochar application to temperate soils: Effects on soil fertility and crop growth under greenhouse conditions. J Plant Nutr Soil Sci 177: 3-15. |
[170] |
Zhou H, Zhang D, Wang P, et al. (2017) Changes in microbial biomass and the metabolic quotient with biochar addition to agricultural soils: A Meta-analysis. Agric Ecosyst Environ 239: 80-89. doi: 10.1016/j.agee.2017.01.006
![]() |
[171] |
Burns RG, DeForest JL, Marxsen J, et al. (2013) Soil enzymes in a changing environment: current knowledge and future directions. Soil Biol Biochem 58: 216-234. doi: 10.1016/j.soilbio.2012.11.009
![]() |
[172] |
Lehmann J, Rillig MC, Thies J, et al. (2011) Biochar effects on soil biota - A review. Soil Biol Biochem 43: 1812-1836. doi: 10.1016/j.soilbio.2011.04.022
![]() |
[173] |
Caroline A, Debode J, Vandecasteele B, et al. (2016) Biological, physicochemical and plant health responses in lettuce and strawberry in soil or peat amended with biochar. Appl Soil Ecol 107: 1-12. doi: 10.1016/j.apsoil.2016.05.001
![]() |
[174] |
Kolton M, Graber ER, Tsehansky L, et al. (2017) Biochar-stimulated plant performance is strongly linked to microbial diversity and metabolic potential in the rhizosphere. New Phytol 213: 1393-1404. doi: 10.1111/nph.14253
![]() |
[175] |
Graber ER, Frenkel O, Jaiswal AK, et al. (2014) How may biochar influence severity of diseases caused by soilborne pathogens? Carbon Manag 5: 169-183. doi: 10.1080/17583004.2014.913360
![]() |
[176] |
Jaiswal AK, Frenkel O, Elad Y, et al. (2015) Non-monotonic influence of biochar dose on bean seedling growth and susceptibility to Rhizoctonia solani: the "Shifted R max-Effect". Plant Soil 395: 125-140. doi: 10.1007/s11104-014-2331-2
![]() |
[177] |
Zhu X, Chen B, Zhu L, et al. (2017) Effects and mechanisms of biochar-microbe interactions in soil improvement and pollution remediation: a review. Environ Pollut 227: 98-115. doi: 10.1016/j.envpol.2017.04.032
![]() |
[178] |
Lal R, Smith P, Jungkunst HF, et al. (2018) The carbon sequestration potential of terrestrial ecosystems. J Soil Water Conserv 73: 145A-152A. doi: 10.2489/jswc.73.6.145A
![]() |
[179] |
Karhu K, Mattila T, Bergström I, et al. (2011) Biochar addition to agricultural soil increased CH4 uptake and water holding capacity - Results from a short-term pilot field study. Agric Ecosyst Environ 140: 309-313. doi: 10.1016/j.agee.2010.12.005
![]() |
[180] |
Bu XL, Su J, Xue JH, et al. (2019) Effect of rice husk biochar addition on nutrient leaching and microbial properties of Calcaric Cambisols. J Soil Water Conserv 74: 172-179. doi: 10.2489/jswc.74.2.172
![]() |
[181] |
Uchimiya M, Lima IM, Klasson KT, et al. (2010) Contaminant immobilization and nutrient release by biochar soil amendment: Roles of natural organic matter. Chemosphere 80: 935-940. doi: 10.1016/j.chemosphere.2010.05.020
![]() |
[182] |
Xu S, Adhikari D, Huang R, et al. (2016) Biochar-facilitated microbial reduction of hematite. Environ Sci Technol 50: 2389-2395. doi: 10.1021/acs.est.5b05517
![]() |
[183] |
Downie A, Munroe P, Cowie A, et al. (2012) Biochar as a Geoengineering Climate Solution: Hazard Identification and Risk Management. Crit Rev Environ Sci Technol 42: 225-250. doi: 10.1080/10643389.2010.507980
![]() |
[184] |
Campbell JL, Sessions J, Smith D, et al. (2018) Potential carbon storage in biochar made from logging residue: Basic principles and Southern Oregon case studies. PLOS ONE 13: e0203475. doi: 10.1371/journal.pone.0203475
![]() |
[185] |
Creamer AE, Gao B, Zhang M (2014) Carbon dioxide capture using biochar produced from sugarcane bagasse and hickory wood. Chem Eng J 249: 174-179. doi: 10.1016/j.cej.2014.03.105
![]() |
[186] |
Hasler K, Bröring S, Omta SWF, et al. (2015) Life cycle assessment (LCA) of different fertilizer product types. Eur J Agron 69: 41-51. doi: 10.1016/j.eja.2015.06.001
![]() |
[187] |
Duku MH, Gu S, Hagan EB (2011) Biochar production potential in Ghana-A review. Renew Sustain Energy Rev 15: 3539-3551. doi: 10.1016/j.rser.2011.05.010
![]() |
[188] |
Lee JW, Hawkins B, Day DM, et al. (2010) Sustainability: the capacity of smokeless biomass pyrolysis for energy production, global carbon capture and sequestration. Energy Environ Sci 3: 1695-1705. doi: 10.1039/c004561f
![]() |
[189] |
Yang Q, Han F, Chen Y, et al. (2016) Greenhouse gas emissions of a biomass-based pyrolysis plant in China. Renew Sustain Energy Rev 53: 1580-1590. doi: 10.1016/j.rser.2015.09.049
![]() |
[190] | Mirkouei A, Haapala KR, Sessions J, et al. (2016) Reducing greenhouse gas emissions for sustainable bio-oil production using a mixed supply chain, ASME 2016 International Design Engineering Technical Conferences and Computers and Information in Engineering Conference, American Society of Mechanical Engineers, V004T05A031. |
[191] | Dougherty B (2016) Biochar as a cover for dairy manure lagoons: reducing odor and gas emissions while capturing nutrients, Oregon State University. |
[192] | Stetina K (2017) Control of Fecal Malodor by Adsorption onto Biochar. |
[193] | Tsutomu I, Asada T, Kuniaki K, et al. (2004) Comparison of Removal Efficiencies for Ammonia and Amine Gases between Woody Charcoal and Activated Carbon. J Health Sci - J Health SCI 50: 148-153. |
[194] |
Parham J, Deng S, Raun W, et al. (2002) Long-term cattle manure application in soil. Biol Fertil Soils 35: 328-337. doi: 10.1007/s00374-002-0476-2
![]() |
[195] | Gasser JKR (1987) The future of animal manures as fertilizer or waste, In: Van Der Meer HG, Unwin RJ, Van Dijk TA, et al. (Eds.), Animal Manure on Grassland and Fodder Crops. Fertilizer or Waste? Proceedings of an International Symposium of the European Grassland Federation, Wageningen, The Netherlands, 31 August-3 September 1987, Dordrecht, Springer Netherlands, 259-278. |
[196] |
Kleinman PJ, Sharpley AN, Wolf AM, et al. (2002) Measuring water-extractable phosphorus in manure as an indicator of phosphorus in runoff. Soil Sci Soc Am J 66: 2009-2015. doi: 10.2136/sssaj2002.2009
![]() |
[197] | Sharpley A, Moyer B (2000) Phosphorus forms in manure and compost and their release during simulated rainfall. J Environ Qual 29: 1462-1469. |
[198] |
Heuer H, Schmitt H, Smalla K (2011) Antibiotic resistance gene spread due to manure application on agricultural fields. Curr Opin Microbiol 14: 236-243. doi: 10.1016/j.mib.2011.04.009
![]() |
[199] | Spencer JL, Guan J (2004) Public Health Implications Related to Spread of Pathogens in Manure From Livestock and Poultry Operations, In: Spencer JFT, Ragout de Spencer AL (Eds.), Public Health Microbiology: Methods and Protocols, Totowa, NJ, Humana Press, 503-515. |
[200] | You Y, Silbergeld EK (2014) Learning from agriculture: understanding low-dose antimicrobials as drivers of resistome expansion. Front Microbiol 5: 284. |
[201] |
Casey JA, Curriero FC, Cosgrove SE, et al. (2013) High-density livestock operations, crop field application of manure, and risk of community-associated methicillin-resistant Staphylococcus aureus infection in Pennsylvania. JAMA Intern Med 173: 1980-1990. doi: 10.1001/jamainternmed.2013.10408
![]() |
[202] |
Manyi-Loh CE, Mamphweli SN, Meyer EL, et al. (2016) An Overview of the Control of Bacterial Pathogens in Cattle Manure. Int J Environ Res Public Health 13: 843. doi: 10.3390/ijerph13090843
![]() |
[203] |
Nicholson FA, Groves SJ, Chambers BJ (2005) Pathogen survival during livestock manure storage and following land application. Bioresour Technol 96: 135-143. doi: 10.1016/j.biortech.2004.02.030
![]() |
[204] | Qiu M, Sun K, Jin J, et al. (2014) Properties of the plant- and manure-derived biochars and their sorption of dibutyl phthalate and phenanthrene. Sci Rep 4: 5295. |
[205] |
Batista EMCC, Shultz J, Matos TTS, et al. (2018) Effect of surface and porosity of biochar on water holding capacity aiming indirectly at preservation of the Amazon biome. Sci Rep 8: 10677. doi: 10.1038/s41598-018-28794-z
![]() |
[206] |
Brown TR, Thilakaratne R, Brown RC, et al. (2013) Techno-economic analysis of biomass to transportation fuels and electricity via fast pyrolysis and hydroprocessing. Fuel 106: 463-469. doi: 10.1016/j.fuel.2012.11.029
![]() |
[207] |
Huang Y-F, Syu F-S, Chiueh P-T, et al. (2013) Life cycle assessment of biochar cofiring with coal. Bioresour Technol 131: 166-171. doi: 10.1016/j.biortech.2012.12.123
![]() |
[208] | Bergman PCA, Boersma AR, Zwart RWR, et al. (2005) Torrefaction for biomass co-firing in existing coal-fired power stations, Energy research Centre of the Netherlands. |
[209] | Abdullah H, Wu H (2009) Biochar as a Fuel: 1. Properties and Grindability of Biochars Produced from the Pyrolysis of Mallee Wood under Slow-Heating Conditions. Energy Fuels 23: 4174-4181. |
[210] |
Yang X, Wang H, Strong P, et al. (2017) Thermal properties of biochars derived from waste biomass generated by agricultural and forestry sectors. Energies 10: 469. doi: 10.3390/en10040469
![]() |
[211] | Engineering ToolBox (2003) Classification of Coal, 2003.Available from: https://www.engineeringtoolbox.com/classification-coal-d_164.html. |
[212] |
Vikrant K, Kim K-H, Ok YS, et al. (2018) Engineered/designer biochar for the removal of phosphate in water and wastewater. Sci Total Environ 616-617: 1242-1260. doi: 10.1016/j.scitotenv.2017.10.193
![]() |
[213] |
Tan X, Liu Y, Gu Y, et al. (2016) Biochar-based nano-composites for the decontamination of wastewater: A review. Bioresour Technol 212: 318-333. doi: 10.1016/j.biortech.2016.04.093
![]() |
[214] | Sizmur T, Fresno T, Akgül G, et al. (2017) Biochar modification to enhance sorption of inorganics from water. Spec Issue Biochar Prod Charact Appl - Soil Appl 246: 34-47. |
[215] |
Husk BR, Sanchez JS, Anderson BC, et al. (2018) Removal of phosphorus from agricultural subsurface drainage water with woodchip and mixed-media bioreactors. J Soil Water Conserv 73: 265-275. doi: 10.2489/jswc.73.3.265
![]() |
[216] |
Inyang MI, Gao B, Yao Y, et al. (2016) A review of biochar as a low-cost adsorbent for aqueous heavy metal removal. Crit Rev Environ Sci Technol 46: 406-433. doi: 10.1080/10643389.2015.1096880
![]() |
[217] |
Jing X-R, Wang Y-Y, Liu W-J, et al. (2014) Enhanced adsorption performance of tetracycline in aqueous solutions by methanol-modified biochar. Chem Eng J 248: 168-174. doi: 10.1016/j.cej.2014.03.006
![]() |
[218] |
Regmi P, Garcia Moscoso JL, Kumar S, et al. (2012) Removal of copper and cadmium from aqueous solution using switchgrass biochar produced via hydrothermal carbonization process. J Environ Manage 109: 61-69. doi: 10.1016/j.jenvman.2012.04.047
![]() |
[219] |
Ahmed MB, Zhou JL, Ngo HH, et al. (2016) Progress in the preparation and application of modified biochar for improved contaminant removal from water and wastewater. Bioresour Technol 214: 836-851. doi: 10.1016/j.biortech.2016.05.057
![]() |
[220] |
DeBoe G, Bock E, Stephenson K, et al. (2017) Nutrient biofilters in the Virginia Coastal Plain: Nitrogen removal, cost, and potential adoption pathways. J Soil Water Conserv 72: 139-149. doi: 10.2489/jswc.72.2.139
![]() |
[221] |
Cao X, Ma L, Gao B, et al. (2009) Dairy-Manure Derived Biochar Effectively Sorbs Lead and Atrazine. Environ Sci Technol 43: 3285-3291. doi: 10.1021/es803092k
![]() |
[222] |
Xu X, Cheng K, Wu H, et al. (2019) Greenhouse gas mitigation potential in crop production with biochar soil amendment-a carbon footprint assessment for cross-site field experiments from China. GCB Bioenergy 11: 592-605. doi: 10.1111/gcbb.12561
![]() |
[223] | Wijitkosum S, Jiwnok P, UNISEARCH C (2019) Effect of biochar on Chinese kale and carbon storage in an agricultural area on a high rise building. |
[224] |
Shaheen A, Turaib Ali Bukhari S (2018) Potential of sawdust and corn cobs derived biochar to improve soil aggregate stability, water retention, and crop yield of degraded sandy loam soil. J Plant Nutr 41: 2673-2682. doi: 10.1080/01904167.2018.1509092
![]() |
[225] |
Sarma B, Farooq M, Gogoi N, et al. (2018) Soil organic carbon dynamics in wheat-Green gram crop rotation amended with vermicompost and biochar in combination with inorganic fertilizers: A comparative study. J Clean Prod 201: 471-480. doi: 10.1016/j.jclepro.2018.08.004
![]() |
[226] | Hersh B, Mirkouei A (2019) Life Cycle Assessment of Pyrolysis-Derived Biochar from Organic Wastes and Advanced Feedstocks, Proceedings of the ASME 2019 International Design Engineering Technical Conferencesand Computers and Information in Engineering Conference, IDETC2019-97896. |
[227] |
Uusitalo V, Leino M (2019) Neutralizing global warming impacts of crop production using biochar from side flows and buffer zones: A case study of oat production in the boreal climate zone. J Clean Prod 227: 48-57. doi: 10.1016/j.jclepro.2019.04.175
![]() |
[228] |
Thers H, Djomo SN, Elsgaard L, et al. (2019) Biochar potentially mitigates greenhouse gas emissions from cultivation of oilseed rape for biodiesel. Sci Total Environ 671: 180-188. doi: 10.1016/j.scitotenv.2019.03.257
![]() |
[229] |
Alotaibi KD, Schoenau JJ (2019) Addition of Biochar to a Sandy Desert Soil: Effect on Crop Growth, Water Retention and Selected Properties. Agronomy 9: 327. doi: 10.3390/agronomy9060327
![]() |
[230] |
Wang D, Li C, Parikh SJ, et al. (2019) Impact of biochar on water retention of two agricultural soils-A multi-scale analysis. Geoderma 340: 185-191. doi: 10.1016/j.geoderma.2019.01.012
![]() |
[231] | Ding Y, Liu Y, Liu S, et al. (2017) Potential Benefits from Biochar Application for Agricultural Use: A Review. Pedosphere. |
[232] |
Wang L, Li L, Cheng K, et al. (2018) An assessment of emergy, energy, and cost-benefits of grain production over 6 years following a biochar amendment in a rice paddy from China. Environ Sci Pollut Res 25: 9683-9696. doi: 10.1007/s11356-018-1245-6
![]() |
[233] |
Shahzad K, Abid M, Sintim HY (2018) Wheat productivity and economic implications of biochar and inorganic nitrogen application. Agron J 110: 2259-2267. doi: 10.2134/agronj2018.01.0055
![]() |
[234] |
Jalal F, Arif M, Ahmad I, et al. (2018) Increasing Farm Productivity and Soil Fertility on Sustainable Basis Through "Summer Gap" Utilization with Biochar and Legumes. Gesunde Pflanz 70: 45-53. doi: 10.1007/s10343-017-0412-x
![]() |
[235] |
Mohammadi A, Cowie AL, Cacho O, et al. (2017) Biochar addition in rice farming systems: Economic and energy benefits. Energy 140: 415-425. doi: 10.1016/j.energy.2017.08.116
![]() |
[236] |
El-Naggar A, Lee SS, Rinklebe J, et al. (2019) Biochar application to low fertility soils: A review of current status, and future prospects. Geoderma 337: 536-554. doi: 10.1016/j.geoderma.2018.09.034
![]() |
[237] |
Al-Wabel MI, Hussain Q, Usman AR, et al. (2018) Impact of biochar properties on soil conditions and agricultural sustainability: a review. Land Degrad Dev 29: 2124-2161. doi: 10.1002/ldr.2829
![]() |
[238] |
Robb S, Dargusch P (2018) A financial analysis and life-cycle carbon emissions assessment of oil palm waste biochar exports from Indonesia for use in Australian broad-acre agriculture. Carbon Manag 9: 105-114. doi: 10.1080/17583004.2018.1435958
![]() |
[239] |
Dai L, Li H, Tan F, et al. (2016) Biochar: a potential route for recycling of phosphorus in agricultural residues. Gcb Bioenergy 8: 852-858. doi: 10.1111/gcbb.12365
![]() |
[240] | Munoz C, Gongora S, Zagal E (2016) Use of biochar as a soil amendment: a brief review. Chil J Agric Anim Sci Ex Agro-Cienc 32: 37-47. |
[241] | Li W (2018) Investigating the development of the bioproducts production platform from a techno-economic and environmental perspective. |
[242] |
Ji C, Cheng K, Nayak D, et al. (2018) Environmental and economic assessment of crop residue competitive utilization for biochar, briquette fuel and combined heat and power generation. J Clean Prod 192: 916-923. doi: 10.1016/j.jclepro.2018.05.026
![]() |
[243] |
Aller DM, Archontoulis SV, Zhang W, et al. (2018) Long term biochar effects on corn yield, soil quality and profitability in the US Midwest. Field Crops Res 227: 30-40. doi: 10.1016/j.fcr.2018.07.012
![]() |
[244] |
Woolf D, Amonette JE, Street-Perrott FA, et al. (2010) Sustainable biochar to mitigate global climate change. Nat Commun 1: 56. doi: 10.1038/ncomms1053
![]() |
[245] | Mohan D, Sarswat A, Ok YS, et al. (2014) Organic and inorganic contaminants removal from water with biochar, a renewable, low cost and sustainable adsorbent - A critical review. Spec Issue Biosorption 160: 191-202. |
[246] |
Woolf D, Amonette JE, Street-Perrott FA, et al. (2010) Sustainable biochar to mitigate global climate change. Nat Commun 1: 56. doi: 10.1038/ncomms1053
![]() |
[247] | Mohan D, Sarswat A, Ok YS, et al. (2014) Organic and inorganic contaminants removal from water with biochar, a renewable, low cost and sustainable adsorbent - A critical review. Spec Issue Biosorption 160: 191-202. |
[248] |
Beesley L, Moreno-Jiménez E, Gomez-Eyles JL, et al. (2011) A review of biochars' potential role in the remediation, revegetation and restoration of contaminated soils. Environ Pollut 159: 3269-3282. doi: 10.1016/j.envpol.2011.07.023
![]() |
[249] |
Van Zwieten L, Kimber S, Morris S, et al. (2010) Effects of biochar from slow pyrolysis of papermill waste on agronomic performance and soil fertility. Plant Soil 327: 235-246. doi: 10.1007/s11104-009-0050-x
![]() |
[250] |
Laird DA, Fleming P, Davis DD, et al. (2010) Impact of biochar amendments on the quality of a typical Midwestern agricultural soil. Geoderma 158: 443-449. doi: 10.1016/j.geoderma.2010.05.013
![]() |
[251] |
Steinbeiss S, Gleixner G, Antonietti M (2009) Effect of biochar amendment on soil carbon balance and soil microbial activity. Soil Biol Biochem 41: 1301-1310. doi: 10.1016/j.soilbio.2009.03.016
![]() |
[252] |
Laird DA, Brown RC, Amonette JE, et al. (2009) Review of the pyrolysis platform for coproducing bio-oil and biochar. Biofuels Bioprod Biorefining 3: 547-562. doi: 10.1002/bbb.169
![]() |
[253] | Kookana RS, Sarmah AK, Van Zwieten L, et al. (2011) Chapter three - Biochar Application to Soil: Agronomic and Environmental Benefits and Unintended Consequences, In: Sparks DL (Ed.), Advances in Agronomy, Academic Press, 103-143. |
[254] |
Regmi P, Garcia Moscoso JL, Kumar S, et al. (2012) Removal of copper and cadmium from aqueous solution using switchgrass biochar produced via hydrothermal carbonization process. J Environ Manage 109: 61-69. doi: 10.1016/j.jenvman.2012.04.047
![]() |
[255] |
Méndez A, Gómez A, Paz-Ferreiro J, et al. (2012) Effects of sewage sludge biochar on plant metal availability after application to a Mediterranean soil. Chemosphere 89: 1354-1359. doi: 10.1016/j.chemosphere.2012.05.092
![]() |
[256] |
Laird DA, Fleming P, Davis DD, et al. (2010) Impact of biochar amendments on the quality of a typical Midwestern agricultural soil. Geoderma 158: 443-449. doi: 10.1016/j.geoderma.2010.05.013
![]() |
[257] |
Steinbeiss S, Gleixner G, Antonietti M (2009) Effect of biochar amendment on soil carbon balance and soil microbial activity. Soil Biol Biochem 41: 1301-1310. doi: 10.1016/j.soilbio.2009.03.016
![]() |
[258] |
Laird DA, Brown RC, Amonette JE, et al. (2009) Review of the pyrolysis platform for coproducing bio-oil and biochar. Biofuels Bioprod Biorefining 3: 547-562. doi: 10.1002/bbb.169
![]() |
[259] |
Méndez A, Gómez A, Paz-Ferreiro J, et al. (2012) Effects of sewage sludge biochar on plant metal availability after application to a Mediterranean soil. Chemosphere 89: 1354-1359. doi: 10.1016/j.chemosphere.2012.05.092
![]() |
[260] | Hansen S, Mirkouei A (2019) Prototyping of a Laboratory-scale Cyclone Separator for Biofuel Production from Biomass Feedstocks Using a Fused Deposition Modeling Printer, The Minerals, Metals & Materials Society (TMS) Conference Proceedings. |
[261] | Hansen S, Mirkouei A, Xian M (2019) Cyber-Physical Control and Optimization for Biofuel 4.0.Proc 2019 IISE Annu Conf H.E. Romeijn, A Schaefer, R. Thomas, eds. |
[262] |
Tripathi M, Sahu JN, Ganesan P (2016) Effect of process parameters on production of biochar from biomass waste through pyrolysis: A review. Renew Sustain Energy Rev 55: 467-481. doi: 10.1016/j.rser.2015.10.122
![]() |
[263] |
Bis Z, Koby\lecki R, Ścis\lowska M, et al. (2018) Biochar-Potential tool to combat climate change and drought. Ecohydrol Hydrobiol 18: 441-453. doi: 10.1016/j.ecohyd.2018.11.005
![]() |
1. | Wei Chen, Bin Zou, GongXian Yang, QinBing Zheng, Ting Lei, Chuanzhen Huang, JiKai Liu, Lei Li, A real-time detection system for multiscale surface defects of 3D printed ceramic parts based on deep learning, 2024, 50, 02728842, 13101, 10.1016/j.ceramint.2024.01.220 | |
2. | Wenyue Sun, Jindong Zhang, Yitong Liu, Adversarial-based refinement dual-branch network for semi-supervised salient object detection of strip steel surface defects, 2024, 0178-2789, 10.1007/s00371-024-03442-y | |
3. | Zefeng Li, Zeyu Zhang, Jixin Ma, Lele Tian, 2023, Improved YOLOV5 based industrial surface defect detection method, 979-8-3503-4271-0, 367, 10.1109/CIPAE60493.2023.00077 | |
4. | Emine Aşar, Atilla Özgür, 2024, Chapter 3, 978-3-031-57467-2, 37, 10.1007/978-3-031-57468-9_3 | |
5. | Fang Luo, Yuan Cui, Xu Wang, Zhiliang Zhang, Yong Liao, Adaptive rotation attention network for accurate defect detection on magnetic tile surface, 2023, 20, 1551-0018, 17554, 10.3934/mbe.2023779 | |
6. | Rong Wang, Zhenzhong Zhang, Guozhao Wei, Haijun Zhang, Xiaoliang Liang, Intelligent recognition method for material removal mode during high-quality ground surface of RB-SiC ceramics based on YOLOv8-Slim-Neck-Ca model, 2025, 1058-9759, 1, 10.1080/10589759.2025.2453945 | |
7. | Chenjie Li, Mian Zhou, Weiwei Pan, 2024, Visual inspection of coatings in hydrogen fuel cells, 9798400707308, 298, 10.1145/3707292.3707380 |
Stage | Template | ||
enc1 | conv 3 × 3, stride = 1, D = 64, BN + ReLU | max_pooling 2 × 2, stride = 2 | ×2 |
enc2 | conv 3 × 3, stride = 1, D = 128, BN + ReLU | max_pooling 2 × 2, stride = 2 | ×2 |
enc3 | conv 3 × 3, stride = 1, D = 256, BN + ReLU | max_pooling 2 × 2, stride = 2 | ×2 |
enc4 | conv 3 × 3, stride = 1, D = 512, BN + ReLU | max_pooling 2 × 2, stride = 2 | ×2 |
enc5 | conv 3 × 3, stride = 1, D = 512, BN + ReLU | max_pooling 2 × 2, stride = 2 | ×2 |
Method | BSCA[49] | FT[50] | MIL[51] | RC[52] | SMD[53] | FCN[40] | UNet[41] | DN[54] | DHSNet[55] | DSS[56] | My |
MAE↓ | 0.2462 | 0157. 7 | 0.1764 | 0.1228 | 0.1913 | 0.0604 | 0.0643 | 0.0481 | 0.0399 | 0.0371 | 0.0369 |
Fβ | 0.3404 | 0.4462 | 0.4873 | 0.3719 | 0.4665 | 0.6788 | 0.6783 | 0.7272 | 0.8447 | 0.8051 | 0.8485 |
Method | MAE | Fβ |
CMN−+ lBCE | 0.0511 | 0.6977 |
CMN++lBCE | 0.0490 | 0.7025 |
CMN++L | 0.0369 | 0.8485 |
Stage | Template | ||
enc1 | conv 3 × 3, stride = 1, D = 64, BN + ReLU | max_pooling 2 × 2, stride = 2 | ×2 |
enc2 | conv 3 × 3, stride = 1, D = 128, BN + ReLU | max_pooling 2 × 2, stride = 2 | ×2 |
enc3 | conv 3 × 3, stride = 1, D = 256, BN + ReLU | max_pooling 2 × 2, stride = 2 | ×2 |
enc4 | conv 3 × 3, stride = 1, D = 512, BN + ReLU | max_pooling 2 × 2, stride = 2 | ×2 |
enc5 | conv 3 × 3, stride = 1, D = 512, BN + ReLU | max_pooling 2 × 2, stride = 2 | ×2 |
Method | BSCA[49] | FT[50] | MIL[51] | RC[52] | SMD[53] | FCN[40] | UNet[41] | DN[54] | DHSNet[55] | DSS[56] | My |
MAE↓ | 0.2462 | 0157. 7 | 0.1764 | 0.1228 | 0.1913 | 0.0604 | 0.0643 | 0.0481 | 0.0399 | 0.0371 | 0.0369 |
Fβ | 0.3404 | 0.4462 | 0.4873 | 0.3719 | 0.4665 | 0.6788 | 0.6783 | 0.7272 | 0.8447 | 0.8051 | 0.8485 |
Method | MAE | Fβ |
CMN−+ lBCE | 0.0511 | 0.6977 |
CMN++lBCE | 0.0490 | 0.7025 |
CMN++L | 0.0369 | 0.8485 |