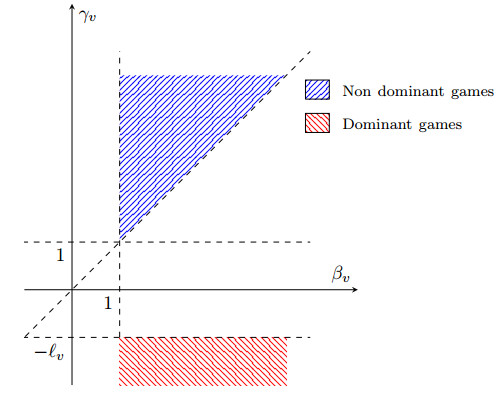
Citation: Dario Madeo, Chiara Mocenni, Jean Carlo Moraes, Jorge P. Zubelli. The role of self-loops and link removal in evolutionary games on networks[J]. Mathematical Biosciences and Engineering, 2019, 16(5): 5287-5306. doi: 10.3934/mbe.2019264
[1] | Bo Lan, Lei Zhuang, Qin Zhou . An evolutionary game analysis of digital currency innovation and regulatory coordination. Mathematical Biosciences and Engineering, 2023, 20(5): 9018-9040. doi: 10.3934/mbe.2023396 |
[2] | Yuanyuan Huang, Yiping Hao, Min Wang, Wen Zhou, Zhijun Wu . Optimality and stability of symmetric evolutionary games with applications in genetic selection. Mathematical Biosciences and Engineering, 2015, 12(3): 503-523. doi: 10.3934/mbe.2015.12.503 |
[3] | Huanhuan Guo, Biao Gao . Game theory analysis of self-awareness and politeness. Mathematical Biosciences and Engineering, 2022, 19(10): 10493-10532. doi: 10.3934/mbe.2022491 |
[4] | A. Swierniak, M. Krzeslak, D. Borys, M. Kimmel . The role of interventions in the cancer evolution–an evolutionary games approach. Mathematical Biosciences and Engineering, 2019, 16(1): 265-291. doi: 10.3934/mbe.2019014 |
[5] | Jialing Li, Guiju Zhu, Xinya Hu, Ruqian Fei, Dan Yu, Dong Wang . Study on the evolutionary strategy of upward patient transfer in the loose medical consortia. Mathematical Biosciences and Engineering, 2023, 20(9): 16846-16865. doi: 10.3934/mbe.2023751 |
[6] | Huan Zhao, Xi Chen . Study on knowledge cooperation of interdisciplinary research team based on evolutionary game theory. Mathematical Biosciences and Engineering, 2023, 20(5): 8782-8799. doi: 10.3934/mbe.2023386 |
[7] | Zheng Liu, Lingling Lang, Lingling Li, Yuanjun Zhao, Lihua Shi . Evolutionary game analysis on the recycling strategy of household medical device enterprises under government dynamic rewards and punishments. Mathematical Biosciences and Engineering, 2021, 18(5): 6434-6451. doi: 10.3934/mbe.2021320 |
[8] | Sharon M. Cameron, Ariel Cintrón-Arias . Prisoner's Dilemma on real social networks: Revisited. Mathematical Biosciences and Engineering, 2013, 10(5&6): 1381-1398. doi: 10.3934/mbe.2013.10.1381 |
[9] | Zhiyan Xing, Yanlong Yang, Zuopeng Hu . Nash equilibrium realization of population games based on social learning processes. Mathematical Biosciences and Engineering, 2023, 20(9): 17116-17137. doi: 10.3934/mbe.2023763 |
[10] | Andrzej Swierniak, Michal Krzeslak . Application of evolutionary games to modeling carcinogenesis. Mathematical Biosciences and Engineering, 2013, 10(3): 873-911. doi: 10.3934/mbe.2013.10.873 |
Many physical systems of interest can be described by evolutionary games on graphs within the more general framework of dynamical systems on complex networks [2]. For example, opinion dynamics under social network influence [3], spread of contagious diseases subject to competition and selection [4,5,6], crime dynamics [7], bacterial networks [8], multi-agent decision-making dynamics [9,10], and the emergence of cooperation in networked populations [11,12,13,14,15,16,17,18]. Among the different interaction mechanisms, the simplest ones can be modeled as two-strategy games [19,20,21]. Differently from the standard approaches presented in [11,14,16,17,18], where the interactions among players are described as discrete time stochastic processes, the evolutionary games equation on networks (EGN) proposed in [1], is a system of ordinary differential equations. More specifically, each of them takes the form of a replicator equation [19], ruling the dynamics of any single player in a population of N individuals arranged on a network, while other standard approaches [12,13,15] consider the average dynamics of the whole population. Recently, model reduction and symmetries have been investigated by using the concept of lumpability of graphs [22].
Similarly to the standard case, the replicator equation on networks possesses different kinds of steady states: mixed steady states that belong to the interior of the simplex (all players play all strategies with the probability in (0, 1)), pure steady states for which all entries belong to the border of the simplex (all players play all strategies with probability 0 or 1), and pure/mixed steady states (where some players play a pure strategy and others play a mixed one. Mixed steady states, hereafter called internal steady states, are particularly important in the EGN because they represent situations where a player assumes hybrid decisions corresponding to partially agree to all available strategies. This includes the case for which some of the strategies are strongly preferred to the others, for example the probability to choose a given strategy can be very close to 1, although different. As a consequence, the probability to choose all the other strategies will be very close to 0 since the sum of all strategy components for each player equals 1. Internal steady states are the most reasonable states for which a group of individuals can be able to agree on a compromised decision.
Moreover, the importance of the internal states mentioned above lies on the fact that they represent situations where different subpopulations may coexist. Thus, studying the attractiveness of these states is connected to the possibility that subetaoups of the players eventually coexist in an asymptotically stable manner [1,19,20]. On the contrary, oscillations making the dynamics more interesting will be produced only if we have unstable internal steady states [23].
In this paper, we study the feasibility of internal steady states in the EGN proposed in [1], by considering different situations, such as, for example, the presence of self-connections in the network. This is particularly relevant for social applications, since self-loops describe well how a player is able to interact also with himself, thus modeling positive or negative feedback on player decisions. We find necessary conditions for the feasibility of internal steady states of EGN. We distinguish the cases of dominant, coordination and anti-coordination payoff matrices of the underlying games. Moreover, we prove sufficient conditions for the feasibility of internal steady states when the graph is complete. Existence and feasibility of internal steady states are relevant for solving control and consensus problems. Controlling dynamical systems over networks in order to drive a population of agents towards a specific steady state has been widely studied [24,25,26,27], while the presence of adaptive networks has been tackled in [28,29].
In [30], the authors developed a new notion: equilibrium interdependence of agents. This investigates how the changes in the parameters impact the equilibrium of the system and how they influence the strategy of other players. In the same direction, we propose a similar approach, where after analyzing the effect of changing some model parameters, we also investigate the effect of modifying the connectivity of players. Therefore, in this work, we prove results concerning how the dynamics of the whole system is influenced by varying the network connectivity of a single node. The problem under investigation connects with the diffusion centrality issue [31], whereby the role of the central individuals in a social network is analyzed by observing indirect information flow.
Finally, the effect of link removal from central players is studied theoretically for graphs with no self-edges, while numerical results are proposed to investigate the case of graphs with self-edges. Including self-edges in the model proposed in [1] is very promising to study the stability of internal steady states [32]. Indeed, it has been shown that stability of certain internal steady states is not possible for graph with no self-edges. Thus, the stability strongly depends on the strength of self-connections as well as on graph topology.
The paper is organized as follows. In Section 2 we illustrate the basics of evolutionary games on networks and in particular on two-strategy game for graphs including self-loops. Section 3 states the necessary conditions for the existence of internal steady states. Some numerical results are provided to analyze the existence of mixed steady states for a generic and heterogeneous scenario. Then, in Section 4 we present sufficient conditions in the case of complete graphs. In Section 5 we tackle the problem of link removal from a player by providing theoretical results for the case of no self-loops, and numerical experiments for the case with self-loops. Finally, in Section 6 we state some conclusions and suggest future developments.
We start by considering the evolutionary game equation on a network (EGN) introduced in [1]:
˙xv,s=xv,s(pGv,s−ϕGv), | (2.1) |
where s belongs to a set of strategies S={1,2,…,M}, v belongs to a set of vertices V={1,2,…,N} and xv,s is the probability that vertex v chooses strategy s, pGv,s is a Von-Neumann Morgenstern utility payoff of player v using pure strategy s, and ϕGv is the average payoff over the set of strategies available to vertex v.
In this paper we consider a generalization of the model where pGv,s and ϕGv are both defined by means of player-to-player payoff matrices Bv,u∈RM×M, such that Bv,u is the payoff matrix used by player v against player u. As a consequence, the model presented in [1] coincides with the special case, whereby Bv,u=Bv,∀u,v∈V. As usual, pGv,s and ϕGv depend on the graph G, which in turn is defined by means of an N×N adjacency matrix A=(av,u)∈RN×N≥0 with (v,u)∈V2. More precisely,
pGv,s=N∑u=1av,ue⊤sBv,uxu, |
and
ϕGv=N∑u=1av,ux⊤vBv,uxu, |
where es is the s-th canonical-basis vector of RM and xv=[xv,1xv,2…xv,M]T is the distribution of pure strategies of player v.
Moreover, we consider graphs with self-loops, i.e., av,v≥0. In this regard, it is straightforward to consider also self-games described by payoff matrices Bv,v, where a player v plays against itself. For convenience, we define the degree of a node as deg(v)=∑Nu=1av,u. Since we are only concerned with graphs without isolated vertices, then ∑Nu=1,u≠vav,u=deg(v)−av,v>0. Hence, δv=av,v/deg(v), which is the relative self-connectivity (i.e., how strong is the self-connection with respect to the sum of all connection weights) is such that 0≤δv<1.
Since for every v the constraint xv,1+xv,2+…+xv,M=1 holds, we have that for M strategies and N vertices the EGN leads to a system with N(M−1) ordinary differential equations. Furthermore, in this article we analyze the EGN equation for two-strategy games (M=2). Therefore, for convenience, we drop the second index s of xv,s, introducing the variable yv=xv,1, whereas xv,2=1−yv. Thus, in our case the EGN becomes a system of N ODEs:
˙yv=yv(pGv−ϕGv). | (2.2) |
Equation (2.2) can be rewritten in a more convenient way as follows: let bv,us,r be the payoff of player v against u when they use strategies s and r, respectively. Then, the payoff function for vertex v against u can be defined by means of the payoff matrix:
Bv,u=(b1,1v,ub1,2v,ub2,1v,ub2,2v,u) . | (2.3) |
We denote by σrv,u=(−1)r+1(b1,rv,u−b2,rv,u) the payoff difference of player v when u uses strategy r. The sign of parameters σ is associated with the game characteristics:
● if σ1v,u>0 and σ2v,u>0, then v is playing a coordination game against u. A prototypical example of this kind of games is the Stag Hunt game.
● if σ1v,u<0 and σ2v,u<0, then v is playing an anti-coordination game against u. A prototypical example of this kind of games is the Chicken game.
● if σ1v,u<0 and σ2v,u>0, or σ1v,u>0 and σ2v,u<0, then v is playing a dominant game against u. A prototypical example of this kind of games for which σ1v,u<0 and σ2v,u>0 is the well-known Prisoner's dilemma game.
According to [19], Bv,u can be equivalently rewritten as a diagonal matrix, namely
Bv,u=diag(σ1v,u,σ2v,u), | (2.4) |
and Eq (2.2) reads as
˙yv=yv(1−yv)fv(y) , | (2.5) |
where y=(y1,…,yN)⊤, fv(y)=∑Nu=1av,ufv,u(yu) and fv,u(yu)=yuTr(Bv,u)−σ2v,u, where Tr(Bv,u) is the trace of matrix Bv,u. Steady states of (2.5) are very important solutions because they influence significantly the asymptotic dynamics of the system. Moreover, they can be related to the Nash equilibria of the game described by the payoff matrix of Eq (2.4). Nash equilibria represent a cornerstone of game theory, since they correspond to strategies such that no player has any incentive to change his or her own strategy. The relationship between steady states and Nash equilibria will be clarified later in the paper.
A solution y∗ of the EGN is a steady state if, and only if, for all v, yv=0, or yv=1, or fv(y∗)=0. But,
fv(y∗)=0⇔N∑u=1av,uTr(Bv,u)yu=N∑u=1av,uσ2v,u,∀v∈V, |
or equivalently
[(Σ1+Σ2)∘A]y∗=[Σ2∘A]1, | (2.6) |
where Σ1 and Σ2 are matrices with Σ1v,u=σ1v,u, Σ2v,u=σ2v,u, A is the adjacency matrix, 1 is the N dimensional vector with one in every entry, and ∘ denotes the Hadamard product defined as P∘Q=R where R={ri,j}:={pi,jqi,j}, provided that matrices P and Q have the same dimension.
Our goal is to study how the connectivity of the graph and the presence of self-loops impact the existence of an internal steady state. From [1] we know that if all players have the same payoff matrix and there are no self-loops, then the topology does not matter. We will start by looking at theoretical results on the existence of mixed equilibrium for more general cases than those studied in [1].
If y∗ satisfies Eq (2.6), then it is a steady state for the ODE system (2.5). Whenever [(Σ1+Σ2)∘A] is invertible, then we have a unique solution:
y∗=[(Σ1+Σ2)∘A]−1[Σ2∘A]1. | (3.1) |
A steady state y∗ satisfying (2.6) is feasible if, and only if, y∗v∈[0,1],∀v∈V. A feasible steady state y∗ is pure if y∗v∈{0,1}N,∀v∈V. A feasible steady state y∗ is said to be internal or mixed if, and only if, y∗v∈(0,1),∀v∈V. Finally, a feasible steady state is non pure if its components belong to both sets (0,1) and {0,1}. We can relate steady states of EGN to Nash equilibria of the underlying game described by the payoff matrices (2.3). Indeed, in two-strategy games, if y∗ is a feasible internal steady state, then it is also a Nash equilibrium of the underlying static game [19].
However, it is not enough to guarantee the solvability of Eq (2.6) in order for the mixed steady states to exist, because we also need that the solutions of the linear equations (2.6) to belong to the interior of the hypercube ΔS={y∈RN:0≤yi≤1, ∀i∈V}. We start by giving necessary conditions on the values of the σs in order for the mixed steady states to be feasible.
Suppose that for every game, the payoff matrix that player v uses when it plays with his neighbors is equal for every opponent. In other words, ∀v∈V,Bv,u=Bv=diag(σv,1,σv,2),∀u≠v. In contradistinction, the self-game of player v is represented by the matrix Bv,v=B↺v=diag(σ↺v,1,σ↺v,2). Now let us define βv and γv as follows:
{T↺v=βvTvσ↺v,2=γvσv,2, |
where Tv=Tr(Bv) and T↺v=Tr(B↺v). Note that, if Tv=0 for some v, then either player v has a dominant strategy or it is indifferent to any strategy. In the case he has a dominant strategy, no internal equilibria can be obtained. In the case the player is indifferent, then he will always play the same strategy that he used at the beginning of the game; therefore, in order to look for an internal equilibrium, we could consider the network game without this player. We define dv=σv,2Tv and d↺v=σ↺v,2T↺v=γvσv,2βvTv=γvβvdv. The following theoretical result holds.
Proposition 3.1. Suppose that the adjacency matrix A is non-negative (A∈RN×N≥0) and that each node has at least one neighbor. Moreover, suppose that Tv≠0. If y∗ is a mixed steady state, then for any v∈V:
{dv(1+δv(γv−1))−y∗vδv(βv−1)∈(0,1)ifav,v≠0dv∈(0,1)ifav,v=0. |
Proof. Let [Ay∗]v and [A1]v be the v-th component of the vector Ay∗ and A1, respectively.
Since each node has at least one neighbor, then deg(v)>0,∀v∈V. Therefore,
[Ay∗]v=∑Nu=1av,u⋅y∗u≥min(y∗)⋅∑Nu=1av,u==min(y∗)⋅deg(v)>0, ∀v∈V. |
Furthermore,
[Ay∗]v=∑Nu=1av,u⋅y∗u<∑Nu=1av,u⋅1==deg(v),∀v∈V . |
This implies that 0<[Ay∗]v<deg(v), and hence
[Ay∗]vdeg(v)∈(0,1)∀v∈V. | (3.2) |
From Eq (2.6), we know that
∑Nu=1av,uTr(Bv,u)y∗u=∑Nu=1av,uσv,2⇒Tv[Ay∗]v+av,v(T↺v−Tv)y∗v=σv,2deg(v)+av,v(σ↺v,2−σv,2)⇒Tv([Ay∗]v+av,v(βv−1)y∗v)=σv,2(deg(v)+av,v(γv−1)), | (3.3) |
∀v∈V. If av,v=0 then
[Ay∗]vdeg(v)=dv∈(0,1),∀v∈V. |
If av,v≠0, then dividing both sides of Eq (3.3) by deg(v)Tv, we have:
[Ay∗]vdeg(v)+δv(βv−1)y∗v=dv(1+δv(γv−1))⇒[Ay∗]vdeg(v)=dv(1+δv(γv−1))−δv(βv−1)y∗v∈(0,1).∀v∈V. |
This result represents a necessary condition for having an internal steady state y∗. The condition depends on the game played, represented by the parameter dv, and on the self-loop, ruled by the parameters δv, βv and γv. If we know the components of y∗, no such condition is needed. However, the proposition allows us to prove the interesting corollary below. This result will give us a truly necessary condition for having an internal steady state in the cases where we have coordination and anti-coordination payoff matrices. It is also interesting to note that, if δv=0 (i.e., no self-loops), then we have that dv∈(0,1), recovering the results of [1] for the uniform payoff case. In this sense, the results in the previous theorem are an extension of the results presented in [1].
Corollary 3.1. Suppose that y∗ is a mixed steady state. Let ℓv=deg(v)−av,v be the v-th element of the Laplacian matrix of the graph, i.e., L=diag(deg(v)v∈V)−A. For each v, then:
(a) if δv=0, then Bv represents a coordination game if Tv>0 or an anti-coordination game if Tv<0;
(b) if δv>0 and 1<βv<γv, then Bv represents a coordination game if Tv>0 or an anti-coordination game if Tv<0;
(c) if δv>0, βv>1 and γv<−ℓv, then the pure strategy y∗v=1 is dominant for the game represented by Bv if Tv>0, or the pure strategy y∗v=0 is dominant for the game represented by Bv if Tv<0.
Proof.
● Proof of (a)
If δv=0 then av,v=0, by the previous proposition we have that dv∈(0,1), then if Tv>0, then Bv represents a coordination game, while for Tv<0 we have an anti-coordination game.
For the cases with δv>0, firstly, notice that
1−1δv=av,v−deg(v)av,v. |
Moreover, since δv>0⇒av,v=1, then:
1−1δv=av,v−deg(v)=−ℓv. |
Secondly, if βv>1, then δv(βv−1)>0. Furthermore:
0<dv(1+δv(γv−1))−y∗vδv(βv−1)<dv(1+δv(γv−1)), |
and
1>dv(1+δv(γv−1))−y∗vδv(βv−1)>dv(1+δv(γv−1))−δv(βv−1), |
yielding that
0<dv(1+δv(γv−1))<1+δv(βv−1). |
● Proof of (b)
If 1<βv<γv, then 1+δv(γv−1)>0 and
0<dv(1+δv(γv−1))<1+δv(βv−1)⇒dv∈(0,1+δv(βv−1)1+δv(γv−1)). |
Since γv>βv then 1+δv(γv−1)>1+δv(βv−1), which implies dv∈(0,1) and the conclusion follows.
● Proof of (c)
βv>1 and γv<−ℓv, then 1+δv(βv−1)1+δv(γv−1)<0 and
0<dv(1+δv(γv−1))<1+δv(βv−1)⇒dv∈(1+δv(βv−1)1+δv(γv−1),0). |
It turns out that dv is a negative number and hence σv,1 and σv,2 have different signs. In particular, if Tv>0, then σv,2<0 and 0<|σv,2|<σv,1, while Tv<0 implies that σv,1<0 and 0<|σv,1|<σv,2. In the former case, Bv represents a game with the pure strategy y=1 dominant; instead for the latter case the pure strategy y=0 is dominant.
The results of Corollary 3.1 are depicted in Figure 1 for a generic player v. We report the regions were an internal steady state is feasible. Blue and red colors indicates the areas where it is necessary a non-dominant game (bistable or coexistent) and a dominant game, respectively.
Proposition 1 and Corollary 3.1 relate the existence of the internal steady state with the connectivity of each player v, i.e., δv, the strength of its self-games, i.e., βv and γv, and the game itself. However, these results only provide necessary conditions for the existence of internal mixed steady states.
While sufficient conditions that work for particular graph structures will be proven in the next sections, here we show a numerical example using an Erdös-Rényi graph sample with N=150 nodes and average degree 10. We assume that av,v=1 for all v∈V, i.e., each player has a self-loop. For this numerical example, we divide the nodes into six groups of 25 elements each. For each group, we choose the parameters σv,1 and σv,2 in the set {(1,1),(0.9,1),(1,0.9),(−1,−1),(−0.9,−1),(−1,−0.9)}. In this way, half of the nodes have a coordination game payoff matrix, while the other half play anti-coordination games. We also assume that βv=β and γv=γ for all the players. Then, for each couple of values β and γ in the set [−30,30]×[−30,30], we evaluated the solution y∗ of Eq (3.1). In Figure 2 we report in blue the couples (β,γ) for which Eq (3.1) has no solution, or if the solution cannot be classified as an internal steady state (i.e., y∗v∉(0,1)∀v). Instead, if the solution y∗ is internal, then the couple (β,γ) is depicted in light green. Finally, in dark green we report the non internal steady states that anyway satisfy the condition of Proposition 1. We remark that light and dark green areas refer to the points (β,γ) which satisfy the condition of Proposition 1 for all the players.
In this section we report some theoretical results on the feasibility of mixed steady states for complete graphs. In order to study how connectivity plays a role on the existence of steady states, we start our study with the most connected graph: the complete one. Our result shows that the presence of many connections in the graph implies that the payoff matrices of the players should be very similar in order for the system to be able to have an internal steady state.
Consider a complete undirected and unweighted graph of N nodes with self-edges. Then, the adjacency matrix A is equal to 1N×N. Moreover, we consider that the self-game strengths are given by βv=β and γv=γ for all players. In this case, we get that (Σ1+Σ2)∘A=diag(Tv)Aβ and Σ2∘A=diag(σv,2)Aγ where Aβ=1N×N+(β−1)IN×N and Aγ=1N×N+(γ−1)IN×N.
Lemma 4.1. Let N∈N+. If β≠1 and β≠1−N, then Aβ is invertible and its inverse is given by A−1β=[qv,u] where
{qv,v=β+(N−2)(β−1)(β+N−1)qv,u=−1(β−1)(β+N−1),v≠u. |
The proof of this lemma is a direct consequence of the remark that Aβ is a circulant matrix.
To ease the discussion of the upcoming results, we introduce the average of a vector over a set of indices Ψ, ⟨x⟩Ψ=1|Ψ|∑v∈Ψxv, where |Ψ| is the cardinality of the set.
Theorem 4.2. Let A, with N≥3 vertices, be the adjacency matrix of a complete graph with self-edges. If Tv≠0 and βv=β∉{1,1−N} for all v∈V, then there is at most one non-pure steady-state y∗ for the system of ODEs in Eq (2.5) and ⟨y∗⟩V=γ+N−1β+N−1⟨d⟩V. Moreover, y∗ is an internal steady-state if, and only if, for all v∈V:
If sign(γ+N−1)=sign(β−1), then:
N⟨d⟩Vβ+N−1<dv<N⟨d⟩Vβ+N−1+β−1γ+N−1. | (4.1) |
If sign(γ+N−1)≠sign(β−1), then:
N⟨d⟩Vβ+N−1+β−1γ+N−1<dv<N⟨d⟩Vβ+N−1. | (4.2) |
Remark 4.3. For the case N=2, it is easy to check that y∗ is feasible if, and only if, 0<dv<1 for v=1,2.
Proof. For β∉{1,1−N} and σv,1+σv,2≠0 we have that (Σ1+Σ2)∘A is invertible. Then, Eq (3.1) becomes:
y∗=A−1βDAγ1, | (4.3) |
where D=diag(Tv)−1diag(σv,2)=diag(dv) is a diagonal matrix.
In this case, the components of the steady state in Eq (4.3) are defined as follows:
y∗v=(β+N−2)(γ+N−1)(β−1)(β+N−1)dv−(γ+N−1)(β−1)(β+N−1)∑Nu=1u≠vdu⇒y∗v=(β+N−1)(γ+N−1)(β−1)(β+N−1)dv−(γ+N−1)(β−1)(β+N−1)∑Nu=1du⇒y∗v=γ+N−1β−1(dv−N⟨d⟩Vβ+N−1), |
while the average of all the components of y∗ is:
⟨y∗⟩=γ+N−1β−1(⟨d⟩V−N⟨d⟩Vβ+N−1)=γ+N−1β+N−1⟨d⟩V. |
Since each component y∗v is in the set (0,1), then:
0<γ+N−1β−1(dv−N⟨d⟩Vβ+N−1)<1. |
If sign(γ+N−1)=sign(β−1), then:
N⟨d⟩Vβ+N−1<dv<N⟨d⟩Vβ+N−1+β−1γ+N−1. |
On the other hand, if sign(γ+N−1)≠sign(β−1), then:
N⟨d⟩Vβ+N−1+β−1γ+N−1<dv<N⟨d⟩Vβ+N−1. |
Corollary 4.4. Under the assumptions of Theorem 4.2, if dv=d for all v∈V, then y∗ is internal to the simplex if, and only if,
γ+N−1β+N−1d∈(0,1). |
The proof of the corollary is straightforward by plugging dv=⟨d⟩V in (4.1) or (4.2).
In the following theorems we discuss the feasibility of internal steady states for complete graphs with no self-edges. In this case Theorem 4.2 simplifies as follows.
Theorem 4.5. Let A, with N≥3 vertices, be the adjacency matrix of a complete graph with no self-edges. If Tv≠0,∀v∈V, then there is at most one non-pure steady-state y∗ for the system of ODEs in Eq (2.5) and ⟨y∗⟩V=⟨d⟩V. Moreover, y∗ is an internal steady-state if, and only if,
N⟨d⟩V−1N−1≤dv≤N⟨d⟩VN−1,∀v∈V. | (4.4) |
Corollary 4.6. Under the assumptions of Theorem 4.2, if y∗ is an internal steady-state then:
|dv−⟨d⟩V|<1N−1,∀v∈V. | (4.5) |
Proof. From (4.4), we have that:
⟨d⟩V−1N−1<dv−⟨d⟩V<⟨d⟩VN−1,∀v∈V. |
Since y∗ is an internal steady state and Tv≠0, then by Theorem (4.2), dv∈(0,1), since βv=γv=0. Therefore,
−1N−1<dv−⟨d⟩V<1N−1,∀v∈V, |
which is the statement of the corollary.
The Corollary 4.6 provides a necessary condition for the system (2.5) to have an internal steady state. If for any vertex v, the distance of dv to the average ⟨d⟩V is greater than or equal to 1N−1, then the system can only have pure steady states. We can also see that if N is large, then we may only have internal steady states whose components dvs are very close, i.e., in a complete graph, the system can only have internal steady states if the payoff's ratio of every player dv, does not get more than 1N−1 distant from the average of all payoff's ratios. For a large system, this will require similar payoffs for all players.
It is worthwhile to note that the results presented in Sections 3 and 4 highlight a strong dependence of the internal steady state on the connectivity of the network and on self-loops. The numerical experiments reported in Figure 3 show that the steady states reached by a population playing different game typologies without (first row) and with (second row) self-loops, may change significantly according to the network connectivity. The detail of the dynamics of a specific node is also reported (third row). In subplot (A), individuals play anti-coordination games, with σv,1=σv,2=−1 for all v. For the case with self-loops, we set βv=4 and γv=6 for all v. In subplot (B), individuals play coordination games, with σv,1=σv,2=1 for all v. For the case with self-loops, we set βv=−5 and γv=−6 for all v. In subplot (C), individuals play coordination games, with σv,1=−1 and σv,2=1.2 for all v. For the case with self-loops, we set βv=−2.5 and γv=−3.5 for all v. Initial condition used for all experiments is x1(0)=x2(0)=x3(0)=x4(0)=x5(0)=0.3.
As a conclusive remark, while in [30] it has been shown that, generally, equilibrium agents are indifferent to small changes in games with two strategies, in our framework we show that modifications in the network structure, as well as the presence of self-loops, may produce significant changes in the dynamics of the system. This connects to Section 5, where the effect of link removal has been analyzed from both theoretical and numerical points of view.
We now consider the following scenario: take a fully connected graph, choose one specific node and start deleting successively different links from this node. The general question under consideration is: what is the effect in the dynamics of such procedure? In general terms, such circle of ideas has attracted the attention of other researchers. For instance, in telecommunications and computer networks this corresponds to the so-called "bond percolation" process (see Section 16.1 of [33]). In Chapter 16 of [33], a comprehensive review of percolation and network resilience can be found. In contradistinction with such approach, we focus on one single node and analyze the resilience with respect to link removal in a deterministic fashion.
First, we study the effect of link removal from central player starting from a complete network. In particular, we report some theoretical results for the case of graphs with no self-edges (βv=γv=0,∀v∈V). The case of networks including self-edges is then investigated by means of numerical simulations, showing that removing links can change dramatically the asymptotic behavior of the system, sometimes destroying the internal steady states.
Theorem 5.1. Let A be the adjacency matrix of a complete graph with N>3 vertices and no self-edges. Assume that the connection between vertices v0 and u0 is removed and Γ=V∖{v0,u0}. Moreover, assume that σv,1+σv,2≠0 for all v. If an internal steady state y∗ exists, then following conditions hold
i)−2+⟨d⟩Γ(N−1)N−3<dv0=du0<⟨d⟩Γ(N−1)N−3,ii)−1+dv0N−1+⟨d⟩Γ<dv<dv0N−1+⟨d⟩Γ,∀v∈Γ. |
Proof. Without loss of generality, let us assume v0=1, u0=2. Writing Eq (2.6) as a system of linear equations, we get
{y3+y4+…+yN=d1(N−2)y3+y4+…+yN=d2(N−2)y1+y2+y4…+yN=d3(N−1)⋮y1+y2+y3⋯+yN−1=dN(N−1). |
If d1≠d2 then the first two equations would be incompatible, therefore d1=d2. In this case, the system has infinite solutions with y1 and y2 as free variables. Let z=y1+y2 and assume d1=d2, then the system with N equations can be reduced to a system with N−1 equations
{y3+y4+…+yN=d1(N−2)z+y4+…+yN=d3(N−1)⋮z+y3+…+yN−1=dN(N−1). |
This system has only one solution given by:
z∗=−(N−3)d1+(N−1)⟨d⟩Γ,y∗v=d1+(N−1)⟨d⟩Γ−(N−1)dv,∀v∈Γ. |
If the solution is in the simplex, then for all v∈Γ, it is true that 0<z∗<2 and 0<y∗v<1. This implies that ⅰ) and ⅱ) must hold.
Suppose now that we start from an almost complete graph and iteratively remove additional links from the same vertex v0. Let Λ={v1,v2,…,vM−1} be the set of vertices that have been disconnected from vertex v0 and Γ=V∖(Λ∪{v0}) the set of vertex that is still connected to v0.
Theorem 5.2. Let A, with N>3 vertices, be the adjacency matrix of a complete graph where K−1 vertices have been disconnected from vertex v0. Let Λ be the set of disconnected vertices and Γ the remaining set of connected vertices. Moreover, let us assume that σv,1+σv,2≠0 for all v∈V. Then, there exists an internal steady state, if, and only if, all the following conditions hold:
i) (K−1)⟨d⟩Λ−(N−1)(K−2)(N−2)⟨d⟩Γ<dv0<(K−1)⟨d⟩Λ−(N−1)(K−2)(N−2)⟨d⟩Γ+K−2N−2,
ii) −1−N−KK−2dv0+(N−2)(K−1)K−2⟨d⟩Λ<(N−2)dv<−N−KK−2dv0+(N−2)(K−1)K−2⟨d⟩Λ,∀v∈Λ,
iii) −1+dv0+(N−1)⟨d⟩Γ<(N−1)dv<dv0+(N−1)⟨d⟩Γ,∀v∈Γ.
Proof. Let us assume without loss of generality that Λ={2,3,⋯,K}. Then, A=(av,u)N×N where
av,u={0,if{v=u or v=1,1≤u≤K or 1≤v≤K,u=11,otherwise. . |
The matrix A is invertible for all N>2 and its inverse is given by the block matrix
A−1N=[R1R2R3R⊤2R4R5R⊤3R⊤5R6], |
where
R1=N−2(K−2)(N−K)R2=−1K−211×(K−1)R3=1N−K11×(N−K)R4=1K−21(K−1)×(K−1)−I(K−1)R5=0(K−1)×(N−K)R6=1N−K1(N−K)×(N−K)−I(N−K). |
In the above formulas, Ii is the i-dimensional identity matrix, and 0i×j and 1i×j are the i×j matrices of all 0 and 1 entries, respectively.
By Eq (3.1), the steady state can be expressed as
y∗1=d1N−2K−2−(N−2)(K−1)(K−2)⟨d⟩Λ+(N−1)⟨d⟩Γy∗v=−(N−K)d1−(N−2)(K−2)dv+(N−2)(K−1)⟨d⟩Λ(K−2),v∈Λy∗u=d1−du+(N−1)⟨d⟩Γ,u∈Γ. |
The result thus follows from the fact the y∗v∈(0,1), for all v∈V.
Remark 5.3 If dv=d∈(0,1),∀v∈V, then we can show that inequalities i), ii) and iii) hold. Then, from Theorem 5.2, we have that the internal steady state exists. This is in agreement with the conclusions obtained by Theorem 1 in [1].
In the following, we report some numerical results on the effect of link removal in networks with self-edges. In order to study the link removal from networks including self-edges we develop a numerical experiment employing random graphs with 60 nodes. Starting from the complete graph, we use 3 different removal strategies to produce different graphs whose average degree is ¯k∈{60,59,58,…,6,5}, thus obtaining a sequence of 56 graphs with different degrees.
A comment concerning the different strategies for edge removal is in order. In the so-called random removal strategy, we start from a complete graph, and then we randomly remove 60 links, in order to obtain a new graph with an average degree of 59. In general, starting from a graph with average degree ¯k, we remove 60 links in order to obtain a graph with average degree ¯k−1. The random regular removal strategy is similar to the random removal approach, but we remove exactly one link for each node, in order to obtain at each step a random regular graph (i.e., all nodes have the same degree). Finally, the Erdös-Rényi removal strategy consists of starting from an Erdös-Rényi graph sample with average degree ¯k, then removing a certain amount of links in order to obtain an Erdös-Rényi graph sample with average degree ¯k. An existing link remains with probability ¯k−1¯k, otherwise, it is removed. In this way we are able to build Erdös-Rényi graph samples using a removal process. For each node we fix βv=γv=−30. For this numerical example, we divide the nodes into six groups of 10 elements each. For each group, we choose the parameters σv,1 and σv,2 in the set {(1,1),(0.9,1),(1,0.9),(−1,−1),(−0.9,−1),(−1,−0.9)}. In this way, half on the nodes have a coordination game payoff matrix, while the other half play anti-coordination games. The three random removal strategies have been repeated 1000 times, thus obtaining 3⋅56⋅1000=168000 random graphs. For each of them, a random initial condition has been created (i.e., xv(0) is a uniformly distributed random number in the set (0,1)). Thereafter, we let the solutions of the ODE (2.5) evolve towards a steady state. An example of the reached steady states is depicted in the subplots (A), (B) and (C) of Figure (4), where a colored point represents the value of the v-th component of the steady state for a given graph whose average degree is reported in the abscissa. We notice that, for sparser graphs, the behavior of the bistable nodes (i.e., the first 30 nodes), becomes more regular, that is, it is easier for the whole population to reach the similar steady state of consensus as long as the neighbor's size decreases. For supporting this claim, in subplots (D), (E) and (F), we report with lines the average of the whole population steady states as a function of the average degree of the network for each link removal strategy. The lighter dots correspond to the steady states reached by members of the population over 1000 numerical experiments. The dots allow us to show that the variance of steady state decreases as the number of removed links increases. A similar numerical experiment has been performed for a population of players involved in a dominant game. More specifically, we assume that a prisoner's dilemma game, where strategies 1 and 2 stand for cooperation and defection, respectively, is simulated by means of the EGN Eq (2.5). For this numerical example, we divide the nodes into six groups of 10 elements each. For each group, we choose the parameters σv,1 and σv,2 in the set {(−1,1.1), (−1,0.9), (−1.1,1), (−1.1,0.9), (−0.9,1), (−0.9,1.1)}. For each node we fix βv=γv=−30. All the couples (σv,1,σv,2) represent games where strategy 2 is dominant, coherently with a prisoner's dilemma game. An example of the reached steady states is depicted in the subplots (A), (B) and (C) of Figure (5). The lines in subplots (D), (E) and (F) represent the average of the whole population steady states as a function of the average degree of the network for each link removal strategy. The lighter dots correspond to the steady states reached by members of the population over 1000 numerical experiments. Interestingly, this experiment shows that link removal policies, as well as the presence of self-loops, allow the system to converge toward strategy 1 for low connectivity, even though the original game is strategy 2 dominant. Moreover, this transition from a population of individuals all converging to strategy 2 to a population of individuals all converging to strategy 1 is sharper if the removal policy is random regular. This result may be useful for further studies investigating the interplay between cooperation and defection in a population of decision makers playing the prisoner's dilemma game. Indeed, Figure (5) shows that including the self-loop as well as removing links can drive individuals to reach some level of cooperation. As a final remark, we note that in [30] the authors conclude that changing the preference of one player usually will not lead a change in the system, which in fact depends on switching the number of strategies' players. We can see a similar effect for the link removal in Figure 5.
In this paper, we study the relationship between network topology and self-loops in the Evolutionary Game Equation on Graphs. The mathematical form of this equation allows us to analyze separately the dynamics of any single individual, starting from his initial condition, which refers to his status in the beginning of the observation, to transient evolution of his strategy, until the asymptotic regime.
In this framework, we state some necessary results for the existence and feasibility of internal steady states. Necessary and sufficient conditions for the case of complete graphs have also been provided. In this scenario, we proved that we can only have a mixed steady state if all payoff matrices are almost similar in terms of the parameters dv (i.e., these parameters must be sufficiently close to their average). In this respect, we also show that the larger the number of nodes of the network, the stronger the conditions for the mixed steady state to be feasible.
The investigation of the internal steady states conducted in this study is an attempt to solve a well-known problem related to games on networks, such as the cooperation in the prisoner's dilemma game from a different point of view. Specifically, the existence of mixed steady states is an alternative way of conceiving cooperation, moving from a discrete (a player cooperates or defects) to a continuous setup (a player can exhibit an intermediate level of cooperation). Then, we exploited the influence of varying the connectivity of the network by removing iteratively the edges of a single node. This link removal process has been studied starting from a complete network without and with self-loops. The former is developed through theoretical results, whereas the latter through numerical simulations. We showed that the effect of link removal may also favor the presence of mixed steady states, thus fostering the onset of a partial level of cooperation. The present research shows that self-loops and link removal have a deep impact on the steady states of each individual of a networked finite population, and consequently, on the whole dynamics. These two ingredients may be fruitfully used for control purposes: for instance, we showed that it is possible to control the dynamics of a population playing a dominant game like the prisoner's dilemma, driving individuals towards a more profitable strategy. Moreover, our approach shows that, although the individuals are involved in coordination and/or anti-coordination games, which naturally splits the population strategy, the presence of self-loops and the link removal may help to reduce the difference of these strategies, thus driving the population towards a consensus steady state.
A natural continuation of this research, which is presently under investigation by the authors, concerns the modification of the present model to account for the weak-selection limit, by preserving all the main peculiarities of the EGN equation. Similar investigations are under way concerning the effect of different network topologies, such as small world or scale-free networks, on the feasibility of the steady states of the EGN equation.
The research of Chiara Mocenni, Jean Carlo Moraes and Jorge P. Zubelli was supported by grant 313773/2013-0 of the Science without Borders Program of CNPq/Brazil. Jorge P. Zubelli was also supported by the CNPq under grant 307873 and by FAPERJ through the program Cientistas do Nosso Estado.
The authors do not have any conflict of interest.
[1] | D. Madeo and C. Mocenni, Game Interactions and dynamics on networked populations, IEEE T. Automat. Contr., 60 (2015), 1801–1810. |
[2] | A. Barrat, M. Barthelemy and A. Vespignani, Dynamical Processes on Complex Networks. Cambridge University Press, UK, 2008. |
[3] | G. Ehrhardt, M. Marsili and F. Vega-Redondo, Diffusion and growth in an evolving network, Int. J. Game Theory, 334 (2006), 383–397. |
[4] | V. Colizza, A. Barrat, M. Barthélemy, et al., The role of the airline transportation network in the prediction and predictability of global epidemics, P. Natl. Acad. Sci. USA, 103 (2006), 2015–2020. |
[5] | V. Colizza and A. Vespignani, Epidemic modeling in metapopulation systems with heterogeneous coupling pattern: Theory and simulations, J. Theor. Biol., 251 (2008), 450–467. |
[6] | S. Tully, M. G. Cojocaru and C. T. Bauch, Multiplayer games and HIV transmission via casual encounters, Math. Biosci. Eng., 14 (2017), 359–376. |
[7] | M. D'Orsogna and M. Perc, Statistical physics of crime: A review, Phys. Life Rev., 12 (2015), 1–21. |
[8] | D. Madeo, L. R. Comolli and C. Mocenni, Emergence of microbial networks as response to hostile environments, Front. Microbiol., 5 (2014), 407. |
[9] | N. Quijano, C. Ocampo-Martinez, J. Barreiro-Gomez, et al., The role of population games and evolutionary dynamics in distributed control systems: The advantages of evolutionary game theory, IEEE Contr. Sys. Mag., 37 (2017), 70–97. |
[10] | R. Gray, A. Franci, V. Srivastava, et al., Multi-agent decision-making dynamics inspired by honeybees, IEEE T. Contr. Netw. Sys., 5 (2018), 793–806. |
[11] | F. C. Santos, J. M. Pacheco and T. Lenaerts, Evolutionary dynamics of social dilemmas in structured heterogeneous populations, P. Natl. Acad. Sci. USA, 103 (2006), 3490–3494. |
[12] | H. Ohtsuki and M. A. Nowak, The replicator equation on graphs, J. Theor. Biol., 243 (2006), 86–97. |
[13] | T. Konno, A condition for cooperation in a game on complex networks, J. Theor. Biol., 269 (2011), 224–233. |
[14] | J. Gómez-Gardenes, I. Reinares, A. Arenas, et al., Evolution of cooperation in multiplex networks, Sci. Rep., 2 (2012), 620. |
[15] | S. M. Cameron and A. Cintrón-Arias, Prisoner's Dilemma on real social networks: Revisited, Math. Biosci. Eng., 10 (2013), 1381–1398. |
[16] | D. G. Rand, M. A. Nowak, J. H. Fowler, et al., Static network structure can stabilize human cooperation, P. Natl. Acad. Sci. USA, 11 (2014), 17093–17098. |
[17] | B. Allen, G. Lippner, Y. Chen, et al., Evolutionary dynamics on any population structure, Nature, 544 (2017), 227. |
[18] | B. Fotouhi, N. Momeni, B. Allen, et al., Evolution of Cooperation on Stochastic Block Models, preprint, arXiv:1807.03093. |
[19] | J. Weibull, Evolutionary Game Theory, MIT Press, Cambridge, MA, 1995. |
[20] | J. Hofbauer and K. Sigmund, Evolutionary game dynamics, B. Am. Math. Soc, 40 (2003) 479–519. |
[21] | M. A. Nowak, Evolutionary Dynamics: Exploring the Equations of Life, Belknap Press of Harvard University Press, Harvard, MA, 2006. |
[22] | G. Iacobelli, D. Madeo and C. Mocenni, Lumping evolutionary game dynamics on networks, J. Theor. Biol., 407 (2016), 328–338. |
[23] | D. Pais, C. H. Caicedo-Nùñez and N. E. Leonard, Hopf bifurcations and limit cycles in evolutionary network dynamics, SIAM J. Appl. Dyn. Syst., 11 (2012), 1754–1884. |
[24] | W. Ren and R. Beard, Consensus seeking in multiagent systems under dynamically changing interaction topologies, IEEE T. Automat. Contr., 50 (2005), 655–661. |
[25] | R. Olfati-Saber, A. Fax and R. Murray, Consensus and cooperation in networked multi-agent systems, P. IEEE, 95 (2007), 215–233. |
[26] | B. Kozma and A. Barrat, Consensus formation on adaptive networks, Phys. Rev. E, 77 (2008), 016102. |
[27] | G. Punzo, G. F. Young, M Macdonald, et al., Using network dynamical influence to drive consensus, Sci. Rep., 6 (2016), 26318. |
[28] | A. Traulsen, F. C. Santos and J. M. Pacheco, Evolutionary Games in Self-Organizing Populations, in Adaptive networks: Theory, Models and Applications (eds. T. Gross and H. Sayama), Springer Berlin Heidelberg, Germany, (2009), 253–267. |
[29] | S. Boccaletti, V. Latora, Y. Moreno, et al., Complex networks: Structure and dynamics, Phys. Rep., 424 (2006), 175–308. |
[30] | Y. Bramoullé and R. Kranton, Games Played on Networks, in The Oxford Handbook of the Economics of Networks (eds. Y. Bramoullé, A. Galeotti and B. Rogers), Oxford University Press. Available from: http://www.oxfordhandbooks.com/view/10.1093/oxfordhb/9780199948277.001. 0001/oxfordhb-9780199948277. |
[31] | A. Banerjee, A. G. Chandrasekhar, E. Duflo, et al., Gossip: Identifying Central Individuals in a Social Network, preprint, arXiv:1406.2293v3. |
[32] | D. Madeo and C. Mocenni, Self-regulation promotes cooperation in social networks, preprint, arXiv:1807.07848. |
[33] | M. Newman, Network: An introduction, Oxford University Press, 2010. |
1. | Dario Madeo, Somnath Mazumdar, Chiara Mocenni, Roberto Zingone, Evolutionary game for task mapping in resource constrained heterogeneous environments, 2020, 108, 0167739X, 762, 10.1016/j.future.2020.03.026 | |
2. | D. Madeo, C. Mocenni, Evolutionary Game Theoretic Insights on the SIRS Model of the COVID-19 Pandemic, 2021, 54, 24058963, 1, 10.1016/j.ifacol.2021.11.016 | |
3. | Dario Madeo, Sergio Salvatore, Terri Mannarini, Chiara Mocenni, Modeling pluralism and self-regulation explains the emergence of cooperation in networked societies, 2021, 11, 2045-2322, 10.1038/s41598-021-98524-5 |