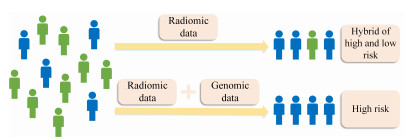
Citation: Kanishka Jha, Ravinder Kataria, Jagesvar Verma, Swastik Pradhan. Potential biodegradable matrices and fiber treatment for green composites: A review[J]. AIMS Materials Science, 2019, 6(1): 119-138. doi: 10.3934/matersci.2019.1.119
[1] | Jingren Niu, Qing Tan, Xiufen Zou, Suoqin Jin . Accurate prediction of glioma grades from radiomics using a multi-filter and multi-objective-based method. Mathematical Biosciences and Engineering, 2023, 20(2): 2890-2907. doi: 10.3934/mbe.2023136 |
[2] | Hakan Özcan, Bülent Gürsel Emiroğlu, Hakan Sabuncuoğlu, Selçuk Özdoğan, Ahmet Soyer, Tahsin Saygı . A comparative study for glioma classification using deep convolutional neural networks. Mathematical Biosciences and Engineering, 2021, 18(2): 1550-1572. doi: 10.3934/mbe.2021080 |
[3] | Sonam Saluja, Munesh Chandra Trivedi, Shiv S. Sarangdevot . Advancing glioma diagnosis: Integrating custom U-Net and VGG-16 for improved grading in MR imaging. Mathematical Biosciences and Engineering, 2024, 21(3): 4328-4350. doi: 10.3934/mbe.2024191 |
[4] | Yutao Wang, Qian Shao, Shuying Luo, Randi Fu . Development of a nomograph integrating radiomics and deep features based on MRI to predict the prognosis of high grade Gliomas. Mathematical Biosciences and Engineering, 2021, 18(6): 8084-8095. doi: 10.3934/mbe.2021401 |
[5] | Sonam Saluja, Munesh Chandra Trivedi, Ashim Saha . Deep CNNs for glioma grading on conventional MRIs: Performance analysis, challenges, and future directions. Mathematical Biosciences and Engineering, 2024, 21(4): 5250-5282. doi: 10.3934/mbe.2024232 |
[6] | Xiaowei Zhang, Jiayu Tan, Xinyu Zhang, Kritika Pandey, Yuqing Zhong, Guitao Wu, Kejun He . Aggrephagy-related gene signature correlates with survival and tumor-associated macrophages in glioma: Insights from single-cell and bulk RNA sequencing. Mathematical Biosciences and Engineering, 2024, 21(2): 2407-2431. doi: 10.3934/mbe.2024106 |
[7] | Hongwei Sun, Qian Gao, Guiming Zhu, Chunlei Han, Haosen Yan, Tong Wang . Identification of influential observations in high-dimensional survival data through robust penalized Cox regression based on trimming. Mathematical Biosciences and Engineering, 2023, 20(3): 5352-5378. doi: 10.3934/mbe.2023248 |
[8] | Moxuan Zhang, Quan Zhang, Jilin Bai, Zhiming Zhao, Jian Zhang . Transcriptome analysis revealed CENPF associated with glioma prognosis. Mathematical Biosciences and Engineering, 2021, 18(3): 2077-2096. doi: 10.3934/mbe.2021107 |
[9] | Yuan Yang, Lingshan Zhou, Xi Gou, Guozhi Wu, Ya Zheng, Min Liu, Zhaofeng Chen, Yuping Wang, Rui Ji, Qinghong Guo, Yongning Zhou . Comprehensive analysis to identify DNA damage response-related lncRNA pairs as a prognostic and therapeutic biomarker in gastric cancer. Mathematical Biosciences and Engineering, 2022, 19(1): 595-611. doi: 10.3934/mbe.2022026 |
[10] | Wei Niu, Lianping Jiang . A seven-gene prognostic model related to immune checkpoint PD-1 revealing overall survival in patients with lung adenocarcinoma. Mathematical Biosciences and Engineering, 2021, 18(5): 6136-6154. doi: 10.3934/mbe.2021307 |
Low-grade glioma (LGG) is a uniformly fatal tumor, and the survival from this tumor is approximately 7 years [1]. Because of the heterogeneity in LGG patients, different LGG subtypes increase the difficulty of optimizing management of adult low-grade gliomas [2,3]. Magnetic Resonance Imaging (MRI) is an imaging technique that can capture tumors of the brain clearly [4]. Clinicians often use MRI images to diagnose the agammaessiveness of the tumor. Therefore, the analysis of MRI data and feature extraction are becoming more challenging. To address these issues, many studies have used MRI data to extract prognostic factors for LGG patients. In a study by Pignatti et al. [5], the authors established a score system that can be used to determine the prognostic score. In adult patients with LGG, the age of the patients, the astrocytoma histology, the largest diameter of the tumor, the tumor crossing the midline and the presence of a neurologic deficit before surgery are all important prognostic factors for survival. These factors can be used to identify low-risk and high-risk patients. In a study by Chen et al. [6], the authors developed a computer-assisted algorithm for tumor segmentation and characterization using both kinetic information and morphological features of 3-D DCE-MRI. They differentiated benign and malignant lesions by analyzing 3-D morphological features including shape features and texture features of the segmented tumor. In a study by Agravat et al. [7], the authors implemented the DeepMedic CNN architecture for tumor segmentation and the extracted features are fed to a random forest classifier to obtain 59% overall survival accuracy. In another study by Shboul et al. [8], 40 features were extracted from the predicted brain tumor mask and fed to a random forest regression to predict the overall survival of a glioma patient, with an accuracy of 67% on the training dataset and 57.9% on the testing dataset. In an attempt at prediction of survival [9], the authors extracted 26 image-derived geometrical features and used SVM to predict the risk of death and classify glioma patients into three groups, with an accuracy of 56.8%. In another attempt [10], hundreds of intensity and texture features were extracted from MR images of glioblastoma multiforme, and principal component analysis (PCA) was used to reduce dimensionality. Then, these features were fed to an artificial neural network (ANN). A result with accuracy of 65.1% was obtained based on two classes: short-overall survivor and long-overall survivor. In another study [11], Chato et al. attempted the use of support vector machines (SVMs), k-nearest neighbors (KNNs), linear discriminants, tree, ensembles and logistic regression to classify survivors into two or three classes. The features from segmentations are used to train the linear discriminant for prediction of survival. The texture features resulted in the accuracy of 46%, and histogram features achieved an accuracy of 68.5% for the test dataset.
The above methods predicted survival by using only image information or clinical information. However, the tumor heterogeneity possibly comes from strong phenotypic differences, and it is difficult to predict prognosis accurately by using only medical imaging analysis (see Figure 1), thus motivating the need for integrating another kind of data. Along with the rapid development of deep-sequencing technology, the output of sequencing has made huge progress not only in equality but also in speed [12]. If radiomic data and genomic data can be integrated, this integration will build a bridge between micro and macro and increase the accuracy of the precision diagnosis and treatment of the brain tumor [13]. Grossmann et al. [14] found that prognostic biomarkers performed better in lung cancer when radiomic, genetic, and clinical information was combined. The C-index was 0.73, while the result is only 0.66 when lacking genetic information. Xia et al. [15] created a radiogenomic strategy that can obtain significant associations between imaging features and gene expression patterns in hepatocellular carcinoma. However, similar work is lacking in LGG. Therefore, in this study we integrated two different types of data, i.e., radiomic features of MRI and gene signatures, to develop a new integrated survival prediction measure for LGG.
The framework of this study is shown in Figure 2. First, we used gene expression data to construct a gene regulatory network and identify network modules and then used imaging data to extract significant radiomic biomarkers that are associated with the survival of the patient (Parts (a) and (b)), respectively. Then, we calculated the correlation between gene modules and image features to obtain a small number of gene signatures that are connected with these image features (Part (c)). Furthermore, we established a Lasso (least absolute shrinkage and selection operator) model to predict the image features with only gene expression values (Parts (d) and (e)). Based on gene expression data, we used support vector machines (SVMs) to identify the gene signatures (Parts (f) and (g)). We combined the predicted image features and the gene signatures to establish an integrated measure that can predict survival of the LGG patient (Parts (h) and (i)). The results show that the integrated measure performed better on survival prediction than any other single index.
Computer-aided and manually corrected segmentation labels for the preoperative multi-institutional scans of 65 LGG patients and 724 radiomic features along with the corresponding skull-stripped and coregistered multimodal (i.e., T1, T1-Gd, T2, T2-FLAIR) MRI data were collected from the Cancer Imaging Archive (TCIA) [16,17,18]. The corresponding RNA-seq data and Disease Free Survival (DSS) data for these 65 patients were also obtained from The Cancer Genome Atlas (TCGA) database. These data were used in this study as the training dataset.
The gene expression data and the corresponding DSS data of 455 LGG patients were downloaded from TCGA and used in this study as the validation dataset.
A gene coexpression network was constructed using gene expression data in the training dataset. We deleted genes that express in less than 20% of the patients or have no expression values. Then, we retained genes that have the highest 25% variance. A pairwise correlation matrix was calculated, and then we adjusted the matrix by raising it to the power of five using the R package WGCNA [19,20]. The minimum module size was set to 50, and the minimum height for merging modules was set to 0.25.
We identified significant image features that are associated with patient DSS by training a multivariate Cox regression model [21] on the training dataset. Image features were filtered with the standard that p value must be less than 0.01. Then, these image features were treated as image biomarkers and survival prediction indexes. For each image feature, we divided patients on the validation dataset into two groups—high-risk group and low-risk group—by taking the median value of the feature as the threshold and plotted the Kaplan-Meier curves. The concordance index (C-index) [22] and the log-rank test were also used to assess the prognostic prediction performance.
The basic formula of the multivariate Cox regression model is described as follows:
h(t,X)=h0(t)⋅exp(β1X1+β2X2+...+βmXm) | (1) |
h(t,X) represents the hazard function and h0(t) is the baseline hazard function. The factor X1, X2, ..., Xm correspond to the image features here and β1, β2, ..., βm are the corresponding regression coefficients.
We calculated Pearson correlation coefficients and their statistical significance to obtain the correlations between gene modules and selected image features. Because there are many genes in each module, the principal component analysis (PCA) was used to reduce the dimension of gene expression data of 65 patients in the training dataset. Then, image features were filtered. Features that showed significant correlation (p value less than 0.05) with at least one gene module were retained, and others were removed. Then, gene modules associated with the same image feature were integrated. The enrichment analysis was performed to identify the significantly enriched molecular pathways on these modules.
We established a radiogenomic map by identifying gene signatures associated with the prognostic imaging features. Lasso (least absolute shrinkage and selection operator) is a regression analysis method that performs both variable selection and regularization [23,24]. This method can enhance the prediction accuracy and interpretability of the statistical model it produces.
Q(β)=‖y−Xβ‖2+λ‖β‖1 | (2) |
Among the above formulas, X is the variable and y is the label. β is the coefficient that we want to optimize. Q(β) is the objective function that we want to minimize. Compared with the method of least squares, the objective function in the Lasso model has a regularization term λ‖β‖1. With this L1 norm regularization term, Lasso can control the number of variables used and improve the generalization ability of the model. For each image feature remaining in the gene module analysis, Lasso was trained to select gene signatures from related gene modules and make a prediction on image features with MRI data and gene expression data in the training dataset. We determined the regularization coefficient λ by minimizing the MSE (mean squared error) of the model.
In this step, we obtained a survival prediction index using only gene signatures, without the information of image features. SVMs (support vector machines) are supervised learning models that can be used for classification and regression problems [25,26,27]. For a classification problem, the optimal hyperplane is searched to separate data into two classes with the max margin. For new data, the trained hyperplane is used to predict the label or the probability of each class. Sometimes, data may not be separated completely, and a soft margin [25] can be used by adding a penalty parameter C and slack variables ξi to obtain the minimum error. The SVM optimization problem is
minω,C12‖ω‖2+CN∑i=1ξi | (3) |
subject to
yif(xi)≥1−ξi, and ξi≥0 | (4) |
The vector ω is the vector orthogonal to the hyperplane. xi, yi are an observation pair of data points, and f(xi) is the label of xi predicted by the SVM. SVM-RFE (support vector machine-recursive feature elimination) [28] is a powerful feature selection algorithm based on SVM that can avoid overfitting when the number of features is high. In each iteration, features are scored and sorted through model training and the least important feature is removed. Remaining features are used for a next training, and the above step is repeated. The score for sorting of the ith feature is defined as
ci=ω2i | (5) |
ωi is the ith dimension of the hyperplane orthogonal vector ω in SVM. Finally, the optimal number of features that have the minimum error is determined.
We use SVM-FRE to select gene signatures and train a classification SVM model with expression data of these selected gene signatures and DSS data in the training dataset. The patient labels are set to 0 or 1 based on their prognostic situation—survival or death. Then, the predicted probability is treated as a survival prediction index. Survival curve and C-index are used to access the prediction performance.
Further, we consider a combination of selected image biomarkers and the index calculated by SVM with gene signatures. To ensure improvement of the new agammaegated index, we transform the calculation of optimal combination coefficients of all features into an optimization problem. Specifically, suppose that N image features are considered to be associated with DSS independently—which are recorded as f1, f2, ..., fN and the gene index value from SVM is recorded as g. The integrated measure we want to determine is recorded as f. The optimization problem needing to be solved can be described as follows.
maxfCf | (6) |
subject to
f=N∑i=1αi⋅fi+β⋅g | (7) |
N∑i=1αi+β=1 | (8) |
where Cf is the C-index of integrated measure f on the training dataset. Our goal is to search optimal parameters α1, α2, ..., αN and β in Eq (7) to maximize the Cf in (6).
The Particle Swarm Optimization (PSO) algorithm [29] is used to solve the optimization problem (6) in this study. PSO is an evolutionary computation algorithm inspired by bird activities that can solve any optimization problem. Initial population with some random particle is created first. For each particle, the position represents a solution, and the corresponding fitness means a value of target function. The object of PSO is to find the optimal particle that has the minimized fitness by updating the velocity and position of particle as the following formula:
vi=ω⋅vi+c1⋅r1⋅(pbesti−xi)+c2⋅r2 | (9) |
xi=xi+vi | (10) |
xi, vi is the position and velocity of the ith particle. pbesti is the best position of the ith particle in history and gbest is the best position of all particles currently. r1, r2 are random numbers between 0 and 1. ω is the inertia weight, and c1, c2 are the acceleration constants.
We take a log-rank test on 724 image features using DSS data of 65 patients in TCIA and filter these features with a standard that the p value is less than 0.01. Then, 21 features remain. Features with high similarity to each other are removed: we calculate the Pearson correlation coefficient between features and remove the one that has the bigger log-rank p value if the Pearson correlation coefficient between two image features is greater than 0.8. After this step, 6 features are removed, and 15 features remain. Based on the above univariable analysis, we first implement the proportional hazard test [21]. Each image feature meets the proportional hazard assumption (detailed information is shown in Additional file 1: Table S1). Then, we train a multivariate Cox regression model on these remaining image features with gene expression data and DSS data in the training dataset. The result is shown in Table 1, and eight features marked with ∗ are considered to be independently correlated with DSS (p<0.05).
Image features | exp(coef) | exp(coef) lower 95% | exp(coef) upper 95% | Wald test | p value |
TEXTURE_GLSZM_ET_T1Gd_SZLGE* | 0 | 0 | 0 | −3.12 | 0.00178 |
HISTO_ED_T2_Bin8* | 0.7 | 0.55 | 0.88 | −3.02 | 0.00254 |
TEXTURE_GLOBAL_ET_T1Gd_Skewness* | 3.03E+05 | 47.29 | 1.94E+09 | 2.82 | 0.00477 |
TEXTURE_GLRLM_NET_FLAIR_LRHGE* | 1 | 0.99 | 1 | −2.66 | 0.0078 |
HISTO_NET_T1_Bin4* | 0.89 | 0.8 | 0.98 | −2.42 | 0.01559 |
HISTO_ET_T1Gd_Bin10* | 1.19 | 1.03 | 1.38 | 2.35 | 0.01877 |
TEXTURE_GLSZM_NET_T1Gd_ZSV* | 0 | 0 | 0 | −2.34 | 0.01906 |
TEXTURE_GLRLM_NET_T1Gd_GLV* | 2.00E+42 | 2230.45 | 1.79E+81 | 2.13 | 0.0333 |
HISTO_ET_T1_Bin10 | 0.81 | 0.63 | 1.05 | −1.59 | 0.11219 |
TEXTURE_GLCM_ET_T2_SumAverage | 0 | 0 | 9.95E+83 | −1.57 | 0.11569 |
TEXTURE_GLRLM_NET_T1_LGRE | 0 | 0 | 2.73E+38 | −1.49 | 0.13526 |
TEXTURE_GLRLM_ED_T1_RLV | inf | 0 | inf | 1.4 | 0.16185 |
HISTO_ED_T2_Bin4 | 0.96 | 0.88 | 1.05 | −0.91 | 0.36241 |
TEXTURE_GLCM_ED_FLAIR_Energy | inf | 0 | inf | 0.78 | 0.43387 |
TEXTURE_GLSZM_NET_T1_LZLGE | 0.99 | 0.96 | 1.03 | −0.39 | 0.69976 |
A gene coexpression network is constructed using gene expression data of 65 patients in the training dataset. We delete genes that express in less than 20% of the patients or have no expression values (n = 1875). Then, we retain genes that have the highest 25% variance (n = 4663). A pairwise correlation matrix is calculated, and then we adjust the matrix by raising it to the power of five using the R package WGCNA [19,20]. The minimum module size is set to 50 and the minimum height for merging modules is set to 0.25. Then, we get 12 gene modules. Detailed information on the modules is shown in Additional file 2: Table S2.
The Pearson correlation coefficient and their statistical significance were calculated between the 12 gene modules and the 8 image features. The result is shown in Figure 3. Four image features that show significant correlation (p<0.05) with at least one gene module were obtained. HISTO_ED_T2_Bin8 is the 8-bin histogram feature of the peritumoral edema in T2-weighted precontrast, TEXTURE_GLSZM_NET_T1Gd_ZSV is the zone size variance of gray level size zone matrix (GLSZM) of the nonenhancing part of the tumor core in T1-weighted postcontrast, TEXTURE_GLRLM_NET_FLAIR_LRHGE is the long run high gray level emphasis of gray level run length matrix (GLRLM) of the nonenhancing part of the tumor core in T2 Fluid-Attenuated Inversion Recovery, and TEXTURE_GLRLM_NET_T1Gd_GLV is the gray level variance of GLRLM of the nonenhancing part of the tumor core in T1-weighted postcontrast. Then, their corresponding gene modules were integrated. The statistical results are shown in Table 2 and the detailed list of genes is shown in Additional file 3: Table S3.
Image features | Associated gene modules | Number of associated genes |
HISTO_ED_T2_Bin8 | module2, module4, module5, module7 | 2794 |
TEXTURE_GLSZM_NET_T1Gd_ZSV | module6 | 506 |
TEXTURE_GLRLM_NET_FLAIR_LRHGE | module6 | 506 |
TEXTURE_GLRLM_NET_T1Gd_GLV | module2, module4, module6, module8, module10 | 1421 |
A further KEGG enrichment analysis was performed on integrated gene modules using the Metascape website [30], which is shown in Figure 4. The complete list of biological annotations is shown in Additional file 4: Table S4. Among these, the neuroactive ligand-receptor interaction pathway is mostly enriched in all integrated gene modules with the minimum p value of 1.259×10−41, which is reported to be associated with glioma [31,32].
Then, the Lasso method described in section 2.5 was used to select gene signatures from the related gene modules and establish a map from genes to image features. We determined the regularization coefficient λ by minimizing the MSE (mean squared error) of the model. The process is shown in Figure 6. The optimal coefficient λ and the corresponding RMSE (root mean squared error) of 65 patients are shown in Table 3. The number of selected gene signatures is also shown. The detailed list of gene signatures is shown in Additional file 5: Table S5.
Image feature | Number of genes in associated modules | Optimal λ | RMSE | Number of genes selected by Lasso |
HISTO_ED_T2_Bin8 | 2794 | 1.6627 | 6.0847 | 12 |
TEXTURE_GLSZM_NET_T1Gd_ZSV | 506 | 7.81E-06 | 2.0195E-5 | 3 |
TEXTURE_GLRLM_NET_FLAIR_LRHGE | 506 | 163.9677 | 528.16 | 6 |
TEXTURE_GLRLM_NET_T1Gd_GLV | 1421 | 3.01E-03 | 0.0120 | 18 |
We made a prediction on the 4 image features using Lasso with gene expression data of 455 patients in TCGA as the validation dataset. We then took the value of each image feature as a survival prediction index. We calculated the C-index and plotted the Kaplan-Meier curves on the validation dataset. The result is shown in Figure 7. The C-index of these four survival prediction indexes are 0.6945, 0.7321, 0.7926, and 0.7985. These results indicate that these four image features perform well in survival prediction.
From the selected 4663 genes with high variance, we fed gene expression data and DSS data of 65 patients in TCIA to SVM-FRE and obtained 43 gene signatures (shown in Additional file 6: Table S6). Then, we trained a classification SVM model with these selected genes. The variables were gene expression data of 65 patients, and the labels were set to 0 or 1 based on the patient prognostic situation—survival or death. Penalty parameter C was set to 2 and 5-fold cross-validation was used to evaluate the error in the recursive feature elimination process. We trained the SVM model and took the predicted probability of survival as a survival prediction index. C-index and survival curve are shown in Figure 8. The C-index is 0.7627.
We took a linear combination of four significant image features and the index calculated by SVM with gene signatures. A better integrated measure was obtained that represents patient survival situation. Set N=4 in formula (7). Four normalized image feature values were recorded as f1, f2, f3, and f4, and the index value from SVM was recorded as g. The integrated measure is recorded as f. Then, we get
f=4∑i=1αi⋅fi+β⋅g | (11) |
We used PSO algorithm to calculate the optimal coefficient to maximize the C-index of 65 patients in the training dataset, with parameters ω, C1 and C2 of 0.8, 0.5 and 0.5. The initial population size was set to 20, 25, 30, 35 and 40, and the corresponding iteration number was set to 30 to ensure the convergence of PSO. We repeated numerical experiments 10 times and recorded the average result for different parameters. Detailed results of each experiment are shown in Additional file 7: Table S7. For each population size, we then brought the coefficients into formula (11) and obtained integrated measure f with different forms. C-index was calculated using gene expression data on the validation dataset. The validation result is shown in Table 4.
Populations sizes | 20 | 25 | 30 | 35 | 40 |
α1 | 0.2926 | 0.3187 | 0.2792 | 0.3303 | 0.276 |
α2 | 0.0663 | 0.0394 | 0.0739 | 0.0505 | 0.068 |
α3 | 0.2171 | 0.2329 | 0.2076 | 0.2214 | 0.2102 |
α4 | 0.0091 | 0.019 | 0.0107 | 0.0298 | 0.0159 |
β | 0.4149 | 0.39 | 0.4288 | 0.368 | 0.4298 |
C-index | 0.8065 | 0.807 | 0.8061 | 0.807 | 0.8057 |
From Table 4, we observe that β is more or less than 0.4 with different parameters. Therefore, the proportion of gene signatures in integration is approximately 40%. α1 is approximately 0.3, α2 is approximately 0.06 and α3 is approximately 0.24. α4 is nearly 0, indicating that the gray level variance of GLRLM of the nonenhancing part of the tumor core in T1-weighted postcontrast can be removed in the integration. We then set parameters α1, α2, α3, α4 and β to 0.3, 0.06, 0.24, 0 and 0.4. We brought these coefficients into formula (11) and calculated the integrated measure f on the validation dataset. The Kaplan-Meier curve is shown in Figure 9. The C-index of the four independent image features, gene signatures and integrated measures are shown in Table 5.
Image features | f1 | f2 | f3 | f4 | g | f |
C-index | 0.6945 | 0.7321 | 0.7926 | 0.7985 | 0.7627 | 0.8071 |
The C-index of the integrated measure f is 0.8071 and is higher than any other measure based on image signatures or gene signature. This result indicates that the integrated measure can improve the prediction accuracy. The integrated measure is recorded as follows.
f=0.3f1+0.06f2+0.24f3+0.4g | (12) |
Furthermore, we use the time dependent Receiver Operating Characteristic (ROC) [33] to further assess the predictive power and compare different prediction models. Time-dependent ROC analysis showed that the integrated measure improved our ability to predict prognosis [AUC, 0.79; and 95% confidence intervals (CI), 0.71 to 0.87] (see Figure 10), when compared with other measures based on image signatures or gene signatures.
Patients are defined into two groups—high-risk group and low-risk group, based on their prognosis—DSS value in this study, by taking the median value of DSS of 65 patients in the training dataset as a threshold. Then, classification is conducted on 455 patients in the validation dataset by taking a threshold of the median value of the integrated measure in the training dataset. The accuracy is 72.1%, which is higher than the accuracy of the published studies [7,8,9,10,11].
The primary goal of phenotyping and classifying a human tumor is to capture tumor heterogeneity and realize personalized precision diagnosis and therapy. In clinical practice, the massive and multiple types of big medical data are available with the rapid development of biomedical engineering and computer application technology. However, one of the biggest challenges in clinical applications is how to integrate these different types of data to extract accuracy information.
In this study, we attempted to integrate both MRI data and gene expression data to propose a new feature measure that could be used to identify subsets of LGG patients at low and high risk for progression to DSS. Based on gene expression data, we first used the WGCNA method to construct the network and identify twelve network modules. With MRI data, eight image biomarkers were obtained by using the Cox regression model. Furthermore, through correlation analysis between gene modules and image features, four radiomic biomarkers were identified. Because MRI data are not available in our test dataset, the Lasso method was applied to build a map from gene expression data to these image features. In addition, we also independently used gene expression data to predict image biomarkers through the SVM method. Finally, an integrated measure (IM) for combining image and gene signatures was obtained through the PSO algorithm. We validated IM with gene expression data and DSS data on 455 patients in the validation dataset. The C-index of IM is 0.8071 and its Area Under Curve (AUC) of the ROC curve is 0.79, higher than any other single measure. The accuracy of classification of patients is 72.1%, which is higher than the accuracy of the published work using only radiomic data [7,8,9,10,11]. The results demonstrate that the proposed IM enhances the prediction accuracy for lower grade gliomas.
In summary, the accuracy of DSS prediction of LGG patients is successfully improved by integrating radiomic features in Macro with the gene expression data in Micro. The proposed method in this study can also be extended to analyze different data sources of other tumors.
This work was supported by the National Key Research and Development Program of China (No. 2018YFC1314600), the Key Program of the National Natural Science Foundation of China (No. 11831015) and the Chinese National Natural Science Foundation (No. 61672388).
All authors declare no conflicts of interest in this paper.
[1] | Bogoeva-Gaceva G, Dimeski D, Srebrenkoska V (2013) Biocomposites based on poly(lactic acid) and kenaf fibers: Effect of micro-fibrillated cellulose. Maced J Chem Chem En 32: 331–335. |
[2] | Dundar T, Ayrilmis N, Büyüksari U (2010) Utilization of waste pine cone in manufacture of wood/plastic composite. Second International Conference on Sustainable Construction Materials and Technologies, Ancona, Italy. |
[3] | Kabir MM, Wang H, Aravinthan T, et al. (2011) Effects of natural fibre surface on composite properties: A review. Proceedings of the 1st international postgraduate conference on engineering, designing and developing the built environment for sustainable wellbeing (eddBE2011), Queensland University of Technology, 94–99. |
[4] |
Mittal G, Dhand V, Rhee KY, et al. (2015) A review on carbon nanotubes and graphene as fillers in reinforced polymer nanocomposites. J Ind Eng Chem 21: 11–25. doi: 10.1016/j.jiec.2014.03.022
![]() |
[5] |
Reddy MM, Vivekanandhan S, Misra M, et al. (2013) Biobased plastics and bionanocomposites: Current status and future opportunities. Prog Polym Sci 38: 1653–1689. doi: 10.1016/j.progpolymsci.2013.05.006
![]() |
[6] |
Rahman A, Ali I, Al Zahrani SM, et al. (2011) A review of the applications of nanocarbon polymer composites. Nano 6: 185–203. doi: 10.1142/S179329201100255X
![]() |
[7] |
Ogunsona EO, Misra M, Mohanty AK (2017) Impact of interfacial adhesion on the microstructure and property variations of biocarbons reinforced nylon 6 biocomposites. Compos Part A-Appl S 98: 32–44. doi: 10.1016/j.compositesa.2017.03.011
![]() |
[8] |
Bledzki AK, Gassan J (1999) Composites reinforced with cellulose based fibres. Prog Polym Sci 24: 221–274. doi: 10.1016/S0079-6700(98)00018-5
![]() |
[9] | Cyras VP, Iannace S, Kenny JM, et al. (2001) Relationship between processing and properties of biodegradable composites based on PCL/starch matrix and sisal fibers. Polym Composite 22: 104–110. |
[10] |
Chiellini E, Cinelli P, Chiellini F, et al. (2004) Environmentally degradable bio-based polymeric blends and composites. Macromol Biosci 4: 218–231. doi: 10.1002/mabi.200300126
![]() |
[11] | Lee SG, Choi SS, Park WH, et al. (2003) Characterization of surface modified flax fibers and their biocomposites with PHB. Macromolecular symposia, Weinheim: WILEY-VCH Verlag, 197: 89–100. |
[12] |
Barari B, Omrani E, Moghadam AD, et al. (2016) Mechanical, physical and tribological characterization of nano-cellulose fibers reinforced bio-epoxy composites: an attempt to fabricate and scale the 'Green' composite. Carbohyd Polym 147: 282–293. doi: 10.1016/j.carbpol.2016.03.097
![]() |
[13] | Omrani E, Menezes PL, Rohatgi PK (2016) State of the art on tribological behavior of polymer matrix composites reinforced with natural fibers in the green materials world. JESTECH 19: 717–736. |
[14] |
Varma IK, Krishnan SRA, Krishnamoorthy S (1989) Composites of glass/modified jute fabric and unsaturated polyester resin. Composites 20: 383–388. doi: 10.1016/0010-4361(89)90664-2
![]() |
[15] |
Valadez-Gonzalez A, Cervantes-Uc JM, Olayo R, et al. (1999) Effect of fiber surface treatment on the fiber–matrix bond strength of natural fiber reinforced composites. Compos Part B-Eng 30: 309–320. doi: 10.1016/S1359-8368(98)00054-7
![]() |
[16] | Mohanty AK, Misra MA, Hinrichsen G (2000) Biofibres, biodegradable polymers and biocomposites: An overview. Macromol Mater Eng 276: 1–24. |
[17] | Wallenberger FT, Weston N (2003) Natural fibers, plastics and composites, Springer Science & Business Media. |
[18] | Cicala G, Cristaldi G, Recca G, et al. (2010) Composites based on natural fibre fabrics. In: Woven fabric engineering, InTech. |
[19] |
Abdelmouleh M, Boufi S, Belgacem MN, et al. (2007) Short natural-fibre reinforced polyethylene and natural rubber composites: effect of silane coupling agents and fibres loading. Compos Sci Technol 67: 1627–1639. doi: 10.1016/j.compscitech.2006.07.003
![]() |
[20] | Joseph PV (2001) Studies on short sisal fibre reinforced isotactic polypropylene composites. |
[21] | Taj S, Munawar MA, Khan S (2007) Natural fiber-reinforced polymer composites. Proc Pakistan Acad Sci 44: 129–144. |
[22] | Sutton A, Black D, Walker P (2011) Natural Fiber Insulation: An Introduction to Low Impact Building Material, IHS BRE Press. |
[23] |
Dhakal HN, Zhang ZY, Richardson MOW (2007) Effect of water absorption on the mechanical properties of hemp fibre reinforced unsaturated polyester composites. Compos Sci Technol 67: 1674–1683. doi: 10.1016/j.compscitech.2006.06.019
![]() |
[24] |
Razak NIA, Ibrahim NA, Zainuddin N, et al. (2014) The influence of chemical surface modification of kenaf fiber using hydrogen peroxide on the mechanical properties of biodegradable kenaf fiber/poly(lactic acid) composites. Molecules 19: 2957–2968. doi: 10.3390/molecules19032957
![]() |
[25] |
Misra S, Misra M, Tripathy SS, et al. (2002) The influence of chemical surface modification on the performance of sisal-polyester biocomposites. Polym Composite 23: 164–170. doi: 10.1002/pc.10422
![]() |
[26] |
Krishnaiah P, Ratnam CT, Manickam S (2017) Enhancements in crystallinity, thermal stability, tensile modulus and strength of sisal fibres and their PP composites induced by the synergistic effects of alkali and high intensity ultrasound (HIU) treatments. Ultrason Sonochem 34: 729–742. doi: 10.1016/j.ultsonch.2016.07.008
![]() |
[27] |
Asim M, Jawaid M, Abdan K, et al. (2016) Effect of alkali and silane treatments on mechanical and fibre-matrix bond strength of kenaf and pineapple leaf fibres. J Bionic Eng 13: 426–435. doi: 10.1016/S1672-6529(16)60315-3
![]() |
[28] | Mathew L, Joseph KU, Rani J (2004) Isora fibres and their composites with natural rubber. Prog Rubber Plast Re 20: 337. |
[29] |
Mohanty AK, Misra M, Drzal LT (2001) Surface modifications of natural fibers and performance of the resulting biocomposites: an overview. Compos Interface 8: 313–343. doi: 10.1163/156855401753255422
![]() |
[30] | Fengel D, Wegener G (1983) Wood: chemistry, ultrastructure, reactions, Walter de Gruyter. |
[31] |
Behera AK, Avancha S, Basak RK, et al. (2012) Fabrication and characterizations of biodegradable jute reinforced soy based green composites. Carbohyd Polym 88: 329–335. doi: 10.1016/j.carbpol.2011.12.023
![]() |
[32] |
Aziz SH, Ansell MP (2004) The effect of alkalization and fibre alignment on the mechanical and thermal properties of kenaf and hemp bast fibre composites: Part 1-polyester resin matrix. Compos Sci Technol 64: 1219–1230. doi: 10.1016/j.compscitech.2003.10.001
![]() |
[33] |
Xie Y, Hill CA, Xiao Z, et al. (2010) Silane coupling agents used for natural fiber/polymer composites: A review. Compos Part A-Appl S 41: 806–819. doi: 10.1016/j.compositesa.2010.03.005
![]() |
[34] |
Devi LU, Bhagawan SS, Thomas S (1997) Mechanical properties of pineapple leaf fiber-reinforced polyester composites. J Appl Polym Sci 64: 1739–1748. doi: 10.1002/(SICI)1097-4628(19970531)64:9<1739::AID-APP10>3.0.CO;2-T
![]() |
[35] |
George J, Sreekala MS, Thomas S (2001) A review on interface modification and characterization of natural fiber reinforced plastic composites. Polym Eng Sci 41: 1471–1485. doi: 10.1002/pen.10846
![]() |
[36] |
Wang B, Panigrahi S, Tabil L, et al. (2007) Pre-treatment of flax fibers for use in rotationally molded biocomposites. J Reinf Plast Comp 26: 447–463. doi: 10.1177/0731684406072526
![]() |
[37] |
Valadez-Gonzalez A, Cervantes-Uc JM, Olayo R, et al. (1999) Chemical modification of henequen fibers with an organosilane coupling agent. Compos Part B-Eng 30: 321–331. doi: 10.1016/S1359-8368(98)00055-9
![]() |
[38] |
Rong MZ, Zhang MQ, Liu Y, et al. (2001) The effect of fiber treatment on the mechanical properties of unidirectional sisal-reinforced epoxy composites. Compos Sci Technol 61: 1437–1447. doi: 10.1016/S0266-3538(01)00046-X
![]() |
[39] | Tserki V, Zafeiropoulos NE, Simon F, et al. (2005) A study of the effect of acetylation and propionylation surface treatments on natural fibres. Compos Part A-Appl S 36: 1110–1118. |
[40] |
Hill CA, Khalil HA, Hale MD (1998) A study of the potential of acetylation to improve the properties of plant fibres. Ind Crop Prod 8: 53–63. doi: 10.1016/S0926-6690(97)10012-7
![]() |
[41] |
Bledzki AK, Mamun AA, Lucka-Gabor M, et al. (2008) The effects of acetylation on properties of flax fibre and its polypropylene composites. Express Polym Lett 2: 413–422. doi: 10.3144/expresspolymlett.2008.50
![]() |
[42] |
Paul S, Nanda P, Gupta R (2003) PhCOCl-Py/basic alumina as a versatile reagent for benzoylation in solvent-free conditions. Molecules 8: 374–380. doi: 10.3390/80400374
![]() |
[43] |
Nair KM, Thomas S, Groeninckx G (2001) Thermal and dynamic mechanical analysis of polystyrene composites reinforced with short sisal fibres. Compos Sci Technol 61: 2519–2529. doi: 10.1016/S0266-3538(01)00170-1
![]() |
[44] | Li X, Tabil LG, Panigrahi S (2007) Chemical treatments of natural fiber for use in natural fiber-reinforced composites: a review. J Polym Environ 15: 25–33. |
[45] |
Joseph K, Thomas S, Pavithran C (1996) Effect of chemical treatment on the tensile properties of short sisal fiber reinforced polyethylene composites. Polymer 37: 5139–5149. doi: 10.1016/0032-3861(96)00144-9
![]() |
[46] | Rao CHC, Madhusudan S, Raghavendra G, et al. (2012) Investigation in to wear behavior of coir fiber reinforced epoxy composites with the Taguchi method. Int J Eng Res Appl 2: 2248–9622. |
[47] |
Paul A, Joseph K, Thomas S (1997) Effect of surface treatments on the electrical properties of low-density polyethylene composites reinforced with short sisal fibers. Compos Sci Technol 57: 67–79. doi: 10.1016/S0266-3538(96)00109-1
![]() |
[48] |
Khan MA, Hassan MM, Drzal LT (2005) Effect of 2-hydroxyethyl methacrylate (HEMA) on the mechanical and thermal properties of jute-polycarbonate composite. Compos Part A-Appl S 36: 71–81. doi: 10.1016/S1359-835X(04)00178-2
![]() |
[49] | Aji IS, Sapuan SM, Zainudin ES, et al. (2009) Kenaf fibres as reinforcement for polymeric composites: a review. Int J Mech Mater Eng 4: 239–248. |
[50] |
Wang B, Panigrahi S, Tabil L, et al. (2007) Pre-treatment of flax fibers for use in rotationally molded biocomposites. J Reinf Plast Comp 26: 447–463. doi: 10.1177/0731684406072526
![]() |
[51] | Rivera-Armenta JL, Flores-Hernández CG, Del Angel-Aldana RZ, et al. (2012) Evaluation of graft copolymerization of acrylic monomers onto natural polymers by means infrared spectroscopy, In: Infrared Spectroscopy-Materials Science, Engineering and Technology, InTech. |
[52] | Singha AS, Rana AK (2012) A comparative study on functionalization of cellulosic biofiber by graft copolymerization of acrylic acid in air and under microwave radiation. BioResources 7: 2019–2037. |
[53] | Wang B, Panigrahi S, Crerar W, et al. (2003) Application of pre-treated flax fibers in composites. CSAE/SCGR Paper No. 03-367. |
[54] |
George J, Sreekala MS, Thomas S (2001) A review on interface modification and characterization of natural fiber reinforced plastic composites. Polym Eng Sci 41: 1471–1485. doi: 10.1002/pen.10846
![]() |
[55] |
Kalia S, Kaith BS, Kaur I (2009) Pretreatments of natural fibers and their application as reinforcing material in polymer composites-a review. Polym Eng Sci 49: 1253–1272. doi: 10.1002/pen.21328
![]() |
[56] | Wallenberger FT, Weston N (2004) Natural fibers plastics and composites, Springer Science & Business Media. |
[57] |
Rahman MM, Mallik AK, Khan MA (2007) Influences of various surface pre-treatments on the mechanical and degradable properties of photo grafted oil palm fibres. J Appl Polym Sci 105: 3077–3086. doi: 10.1002/app.26481
![]() |
[58] |
Paul SA, Joseph K, Mathew GG, et al. (2010) Influence of polarity parameters on the mechanical properties of composites from polypropylene fiber and short banana fiber. Compos Part A-Appl S 41: 1380–1387. doi: 10.1016/j.compositesa.2010.04.015
![]() |
[59] | Kiattipanich N, Kreua-Ongarjnukool N, Pongpayoon T, et al. (2007) Properties of polypropylene composites reinforced with stearic acid treated sugarcane fiber. J Polym Eng 27: 411–428. |
[60] |
Kalaprasad G, Francis B, Thomas S, et al. (2004) Effect of fibre length and chemical modifications on the tensile properties of intimately mixed short sisal/glass hybrid fibre reinforced low density polyethylene composites. Polym Int 53: 1624–1638. doi: 10.1002/pi.1453
![]() |
[61] |
Torres FG, Cubillas ML (2005) Study of the interfacial properties of natural fibre reinforced polyethylene. Polym Test 24: 694–698. doi: 10.1016/j.polymertesting.2005.05.004
![]() |
[62] | Pickering KL, Li Y, Farrell RL, et al. (2007) Interfacial modification of hemp fiber reinforced composites using fungal and alkali treatment. J Biobased Mater Bio 1: 109–117. |
[63] | Jafari MA, Nikkhah A, Sadeghi AA, et al. (2007) The effect of Pleurotus spp. fungi on chemical composition and in vitro digestibility of rice straw. Pak J Biol Sci 10: 2460–2464. |
[64] | Kolybaba M, Tabil LG, Panigrahi S, et al. (2006) Biodegradable polymers: past, present, and future. ASABE/CSBE North Central Intersectional Meeting, American Society of Agricultural and Biological Engineers. |
[65] | Mohanty AK, Misra MA, Hinrichsen G (2000) Biofibres, biodegradable polymers and biocomposites: An overview. Macromol Mater Eng 276: 1–24. |
[66] |
Riedel U, Nickel J (1999) Natural fibre-reinforced biopolymers as construction materials-new discoveries. Die Angewandte Makromolekulare Chemie 272: 34–40. doi: 10.1002/(SICI)1522-9505(19991201)272:1<34::AID-APMC34>3.0.CO;2-H
![]() |
[67] | Mohanty AK, Wibowo A, Misra M, et al. (2004) Effect of process engineering on the performance of natural fiber reinforced cellulose acetate biocomposites. Compos Part A-Appl S 35: 363–370. |
[68] | Ghanbarzadeh B, Almasi H (2013) Biodegradable Polymer, In: Chamy R, Rosenkranz F, Biodegradation-Life of Science, InTech. |
[69] |
Albertsson AC, Karlsson S (1995) Degradable polymers for the future. Acta Polym 46: 114-123. doi: 10.1002/actp.1995.010460203
![]() |
[70] |
Chandra R, Rustgi R (1998) Biodegradable polymers. Prog Polym Sci 23: 1273–1335. doi: 10.1016/S0079-6700(97)00039-7
![]() |
[71] |
Liu D, Tian H, Zhang L, et al. (2008) Structure and properties of blend films prepared from castor oil-based polyurethane/soy protein derivative. Ind Eng Chem Res 47: 9330–9336. doi: 10.1021/ie8009632
![]() |
[72] | Kumar R, Liu D, Zhang L (2008) Advances in proteinous biomaterials. J Biobased Mater Bio 2: 1–24. |
[73] |
Cao X, Chen Y, Chang PR, et al. (2008) Green composites reinforced with hemp nanocrystals in plasticized starch. J Appl Polym Sci 109: 3804–3810. doi: 10.1002/app.28418
![]() |
[74] |
Vazquez A, Dominguez VA, Kenny JM (1999) Bagasse fiber-polypropylene based composites. J Thermoplast Compos 12: 477–497. doi: 10.1177/089270579901200604
![]() |
[75] |
Chen X, Guo Q, Mi Y (1998) Bamboo fiber-reinforced polypropylene composites: A study of the mechanical properties. J Appl Polym Sci 69: 1891–1899. doi: 10.1002/(SICI)1097-4628(19980906)69:10<1891::AID-APP1>3.0.CO;2-9
![]() |
[76] |
Thakore IM, Desai S, Sarawade BD, et al. (2001) Studies on biodegradability, morphology and thermo-mechanical properties of LDPE/modified starch blends. Eur Polym J 37: 151–160. doi: 10.1016/S0014-3057(00)00086-0
![]() |
[77] |
Zhang JF, Sun X (2004) Mechanical and thermal properties of poly(lactic acid)/starch blends with dioctyl maleate. J Appl Polym Sci 94: 1697–1704. doi: 10.1002/app.21078
![]() |
[78] | Wattanakornsiri A, Pachana K, Kaewpirom S, et al. (2011) Green composites of thermoplastic corn starch and recycled paper cellulose fibers. Songklanakarin J Sci Technol 33: 461–467. |
[79] | Avérous L (2008) Polylactic acid: synthesis, properties and applications, In: Belgacem MN, Gandini A, Monomers, polymers and composites from renewable resources, Elsevier Ltd., 433–450. |
[80] | Auras R, Harte B, Selke S (2005) Polylactides. A new era of biodegradable polymers for packaging application. In Ann Tech Conf–ANTEC Conf Proc, 8: 320–324. |
[81] |
Oksman K, Skrifvars M, Selin JF (2003) Natural fibres as reinforcement in polylactic acid (PLA) composites. Compos Sci Technol 63: 1317–1324. doi: 10.1016/S0266-3538(03)00103-9
![]() |
[82] |
Fuqua MA, Huo S, Ulven CA (2012) Natural fiber reinforced composites. Polym Rev 52: 259–320. doi: 10.1080/15583724.2012.705409
![]() |
[83] |
Simoes CL, Viana JC, Cunha AM (2009) Mechanical properties of poly(ɛ-caprolactone) and poly(lactic acid) blends. J Appl Polym Sci 112: 345–352. doi: 10.1002/app.29425
![]() |
[84] |
Jain S, Reddy MM, Mohanty AK, et al. (2010) A new biodegradable flexible composite sheet from poly(lactic acid)/poly(ɛ-caprolactone) blends and Micro-Talc. Macromol Mater Eng 295: 750–762. doi: 10.1002/mame.201000063
![]() |
[85] | Tuil R, Fowler P, Lawther M, et al. (2000) Properties of biobased packaging materials. In: Production of Biobased Packaging Materials for the Food Industry, Center for Skov, Landskab og Planlægning/Københavns Universitet. |
[86] |
Lehermeir HJ, Dorgan JR, Way JD (2001) Gas permeation properties of poly(lactic acid). J Membrane Sci 190: 243–251. doi: 10.1016/S0376-7388(01)00446-X
![]() |
[87] | Rasal RM, Hirt DE (2009) Toughness decrease of PLA-PHBHHx blend films upon surface-confined photopolymerization. J Biomed Mater Res A 88: 1079–1086. |
[88] |
Hiljanen-Vainio M, Varpomaa P, Seppälä J, et al. (1996) Modification of poly(L-lactides) by blending: mechanical and hydrolytic behavior. Macromol Chem Phys 197: 1503–1523. doi: 10.1002/macp.1996.021970427
![]() |
[89] |
Sinclair RG (1996) The case for polylactic acid as a commodity packaging plastic. J Macromol Sci A 33: 585–597. doi: 10.1080/10601329608010880
![]() |
[90] |
Martin O, Averous L (2001) Poly(lactic acid): plasticization and properties of biodegradable multiphase systems. Polymer 42: 6209–6219. doi: 10.1016/S0032-3861(01)00086-6
![]() |
[91] |
Ljungberg N, Wesslen B (2002) The effects of plasticizers on the dynamic mechanical and thermal properties of poly(lactic acid). J Appl Polym Sci 86: 1227–1234. doi: 10.1002/app.11077
![]() |
[92] | Pongtanayut K, Thongpin C, Santawitee O (2013) The effect of rubber on morphology, thermal properties and mechanical properties of PLA/NR and PLA/ENR blends. Energy Procedia 34: 888–897. |
[93] | Senawi R, Alauddin SM, Saleh RM, et al. (2013) Polylactic acid/empty fruit bunch fiber biocomposite: Influence of alkaline and silane treatment on the mechanical properties. Int J Biosci Biochem Bioin 3: 59. |
[94] |
Huda MS, Drzal LT, Misra M, et al. (2006) Wood-fiber-reinforced poly(lactic acid) composites: evaluation of the physico-mechanical and morphological properties. J Appl Polym Sci 102: 4856–4869. doi: 10.1002/app.24829
![]() |
[95] |
Oksman K, Skrifvars M, Selin JF (2003) Natural fibres as reinforcement in polylactic acid (PLA) composites. Compos Sci Technol 63: 1317–1324. doi: 10.1016/S0266-3538(03)00103-9
![]() |
[96] |
Tang G, Zhang R, Wang X, et al. (2013) Enhancement of flame retardant performance of bio-based polylactic acid composites with the incorporation of aluminum hypophosphite and expanded graphite. J Macromol Sci A 50: 255–269. doi: 10.1080/10601325.2013.742835
![]() |
[97] | Huang SJ, Edelman PG (1995) An overview of biodegradable polymers and biodegradation of polymers, In: Scott G, Gilead D, Degradable Polymers, Dordrecht: Springer. |
[98] |
Wu CS (2003) Physical properties and biodegradability of maleated-polycaprolactone/starch composite. Polym Degrad Stabil 80: 127–134. doi: 10.1016/S0141-3910(02)00393-2
![]() |
[99] |
Nair LS, Laurencin CT (2007) Biodegradable polymers as biomaterials. Prog Polym Sci 32: 762–798. doi: 10.1016/j.progpolymsci.2007.05.017
![]() |
[100] |
Honma T, Zhao L, Asakawa N, et al. (2006) Poly(ɛ-caprolactone)/chitin and poly(ɛ-caprolactone)/chitosan blend films with compositional gradients: fabrication and their biodegradability. Macromol Biosci 6: 241–249. doi: 10.1002/mabi.200500216
![]() |
[101] |
Wu CS (2005) A comparison of the structure, thermal properties, and biodegradability of polycaprolactone/chitosan and acrylic acid grafted polycaprolactone/chitosan. Polymer 46: 147–155. doi: 10.1016/j.polymer.2004.11.013
![]() |
[102] |
Wu KJ, Wu CS, Chang JS (2007) Biodegradability and mechanical properties of polycaprolactone composites encapsulating phosphate-solubilizing bacterium Bacillus sp. PG01. Process Biochem 42: 669–675. doi: 10.1016/j.procbio.2006.12.009
![]() |
[103] | Jha K, Chamoli S, Tyagi YK, et al. (2018) Characterization of biodegradable composites and application of preference selection index for deciding optimum phase combination. Mater Today Proc 5: 3353–3360. |
[104] |
Steinbüchel A, Valentin HE (1995) Diversity of bacterial polyhydroxyalkanoic acids. FEMS Microbiol Lett 128: 219–228. doi: 10.1111/j.1574-6968.1995.tb07528.x
![]() |
[105] |
Reis KC, Pereira J, Smith AC, et al. (2008) Characterization of polyhydroxybutyrate-hydroxyvalerate (PHB-HV)/maize starch blend films. J Food Eng 89: 361–369. doi: 10.1016/j.jfoodeng.2008.04.022
![]() |
[106] |
Barham PJ, Organ SJ (1994) Mechanical properties of polyhydroxybutyrate-hydroxybutyrate-hydroxyvalerate copolymer blends. J Mater Sci 29: 1676–1679. doi: 10.1007/BF00368945
![]() |
[107] | Mohammadi M, Ghaffari-Moghaddam M (2014) Recovery and Extraction of Polyhydroxyalkanoates (PHAs), In: Polyhydroxyalkanoate (PHA) based Blends, Composites and Nanocomposites, 30: 47. |
[108] |
Macedo JS, Costa MF, Tavares MIB, et al. (2010) Preparation and characterization of composites based on polyhydroxybutyrate and waste powder from coconut fibers processing. Polym Eng Sci 50: 1466–1475. doi: 10.1002/pen.21669
![]() |
[109] |
Fragassa C, de Camargo FV, Pavlovic A, et al. (2018) Experimental evaluation of static and dynamic properties of low styrene emission vinylester laminates reinforced by natural fibres. Polym Test 69: 437–449. doi: 10.1016/j.polymertesting.2018.05.050
![]() |
[110] |
Fragassa C, Pavlovic A, Živković I (2018) The accelerated aging effect of salt water on lignocellulosic fibre reinforced composites. Tribol Ind 40: 1–9. doi: 10.24874/ti.2018.40.01.01
![]() |
[111] | Fragassa C, Pavlovic A, Santulli C (2018) Mechanical and impact characterisation of flax and basalt fibre vinylester composites and their hybrids. Compos Part B-Eng 137: 247–259. |
[112] | Mundera F (2003) Advanced Technology for Processing of NFP for Industrial Applications. 7th International Conference on Wood Plastic Composites, Madison, WI, May 19–20. |
[113] |
Shakoor A, Muhammad R, Thomas NL, et al. (2013) Mechanical and thermal characterisation of poly(l-lactide) composites reinforced with hemp fibers. J Phys Conf Ser 451: 012010. doi: 10.1088/1742-6596/451/1/012010
![]() |
[114] |
Dey K, Ganguly S, Khan RA, et al. (2013) Surface treatment of areca-nut fiber using silane and gamma irradiation: fabrication of polycaprolactone based composite. J Compos Biodegrad Polym 1: 1–7. doi: 10.12974/2311-8717.2013.01.01.1
![]() |
[115] | Khandanlou R, Ahmad MB, Shameli K, et al. (2014) Mechanical and thermal stability properties of modified rice straw fiber blend with polycaprolactone composite. J Nanomater 2014: 93. |
[116] | Sandeep Laxmeshwar S, Viveka S, Madhu Kumar DJ, et al. (2012) Preparation and properties of composite films from modified cellulose fiber-reinforced with PLA. Pharma Chemica 4: 159–168. |
[117] |
Wu CS (2010) Preparation and characterizations of polycaprolactone/green coconut fiber composites. J Appl Polym Sci 115: 948–956. doi: 10.1002/app.30955
![]() |
[118] | Phua YJ, Chow WS, Mohd Ishak ZA (2013) Mechanical properties and structure development in poly(butylene succinate)/organo-montmorillonite nanocomposites under uniaxial cold rolling. Express Polym Lett 5: 93–103. |
[119] |
Barkoula NM, Garkhail SK, Peijs T (2010) Biodegradable composites based on flax/polyhydroxybutyrate and its copolymer with hydroxyvalerate. Ind Crop Prod 31: 34–42. doi: 10.1016/j.indcrop.2009.08.005
![]() |
[120] | Jha K, Tyagi YK, Yadav AS (2018) Mechanical and thermal behaviour of biodegradable composites based on polycaprolactone with pine cone particle. Sādhanā 43: 135. |
1. | Farinaz Forouzannia, Vahid Shahrezaei, Mohammad Kohandel, The impact of random microenvironmental fluctuations on tumor control probability, 2021, 509, 00225193, 110494, 10.1016/j.jtbi.2020.110494 | |
2. | Anuraag Bukkuri, Kenneth J. Pienta, Robert H. Austin, Emma U. Hammarlund, Sarah R. Amend, Joel S. Brown, A mathematical investigation of polyaneuploid cancer cell memory and cross-resistance in state-structured cancer populations, 2023, 13, 2045-2322, 10.1038/s41598-023-42368-8 | |
3. | Anuraag Bukkuri, Modeling stress-induced responses: plasticity in continuous state space and gradual clonal evolution, 2024, 143, 1431-7613, 63, 10.1007/s12064-023-00410-3 |
Image features | exp(coef) | exp(coef) lower 95% | exp(coef) upper 95% | Wald test | p value |
TEXTURE_GLSZM_ET_T1Gd_SZLGE* | 0 | 0 | 0 | −3.12 | 0.00178 |
HISTO_ED_T2_Bin8* | 0.7 | 0.55 | 0.88 | −3.02 | 0.00254 |
TEXTURE_GLOBAL_ET_T1Gd_Skewness* | 3.03E+05 | 47.29 | 1.94E+09 | 2.82 | 0.00477 |
TEXTURE_GLRLM_NET_FLAIR_LRHGE* | 1 | 0.99 | 1 | −2.66 | 0.0078 |
HISTO_NET_T1_Bin4* | 0.89 | 0.8 | 0.98 | −2.42 | 0.01559 |
HISTO_ET_T1Gd_Bin10* | 1.19 | 1.03 | 1.38 | 2.35 | 0.01877 |
TEXTURE_GLSZM_NET_T1Gd_ZSV* | 0 | 0 | 0 | −2.34 | 0.01906 |
TEXTURE_GLRLM_NET_T1Gd_GLV* | 2.00E+42 | 2230.45 | 1.79E+81 | 2.13 | 0.0333 |
HISTO_ET_T1_Bin10 | 0.81 | 0.63 | 1.05 | −1.59 | 0.11219 |
TEXTURE_GLCM_ET_T2_SumAverage | 0 | 0 | 9.95E+83 | −1.57 | 0.11569 |
TEXTURE_GLRLM_NET_T1_LGRE | 0 | 0 | 2.73E+38 | −1.49 | 0.13526 |
TEXTURE_GLRLM_ED_T1_RLV | inf | 0 | inf | 1.4 | 0.16185 |
HISTO_ED_T2_Bin4 | 0.96 | 0.88 | 1.05 | −0.91 | 0.36241 |
TEXTURE_GLCM_ED_FLAIR_Energy | inf | 0 | inf | 0.78 | 0.43387 |
TEXTURE_GLSZM_NET_T1_LZLGE | 0.99 | 0.96 | 1.03 | −0.39 | 0.69976 |
Image features | Associated gene modules | Number of associated genes |
HISTO_ED_T2_Bin8 | module2, module4, module5, module7 | 2794 |
TEXTURE_GLSZM_NET_T1Gd_ZSV | module6 | 506 |
TEXTURE_GLRLM_NET_FLAIR_LRHGE | module6 | 506 |
TEXTURE_GLRLM_NET_T1Gd_GLV | module2, module4, module6, module8, module10 | 1421 |
Image feature | Number of genes in associated modules | Optimal λ | RMSE | Number of genes selected by Lasso |
HISTO_ED_T2_Bin8 | 2794 | 1.6627 | 6.0847 | 12 |
TEXTURE_GLSZM_NET_T1Gd_ZSV | 506 | 7.81E-06 | 2.0195E-5 | 3 |
TEXTURE_GLRLM_NET_FLAIR_LRHGE | 506 | 163.9677 | 528.16 | 6 |
TEXTURE_GLRLM_NET_T1Gd_GLV | 1421 | 3.01E-03 | 0.0120 | 18 |
Populations sizes | 20 | 25 | 30 | 35 | 40 |
α1 | 0.2926 | 0.3187 | 0.2792 | 0.3303 | 0.276 |
α2 | 0.0663 | 0.0394 | 0.0739 | 0.0505 | 0.068 |
α3 | 0.2171 | 0.2329 | 0.2076 | 0.2214 | 0.2102 |
α4 | 0.0091 | 0.019 | 0.0107 | 0.0298 | 0.0159 |
β | 0.4149 | 0.39 | 0.4288 | 0.368 | 0.4298 |
C-index | 0.8065 | 0.807 | 0.8061 | 0.807 | 0.8057 |
Image features | f1 | f2 | f3 | f4 | g | f |
C-index | 0.6945 | 0.7321 | 0.7926 | 0.7985 | 0.7627 | 0.8071 |
Image features | exp(coef) | exp(coef) lower 95% | exp(coef) upper 95% | Wald test | p value |
TEXTURE_GLSZM_ET_T1Gd_SZLGE* | 0 | 0 | 0 | −3.12 | 0.00178 |
HISTO_ED_T2_Bin8* | 0.7 | 0.55 | 0.88 | −3.02 | 0.00254 |
TEXTURE_GLOBAL_ET_T1Gd_Skewness* | 3.03E+05 | 47.29 | 1.94E+09 | 2.82 | 0.00477 |
TEXTURE_GLRLM_NET_FLAIR_LRHGE* | 1 | 0.99 | 1 | −2.66 | 0.0078 |
HISTO_NET_T1_Bin4* | 0.89 | 0.8 | 0.98 | −2.42 | 0.01559 |
HISTO_ET_T1Gd_Bin10* | 1.19 | 1.03 | 1.38 | 2.35 | 0.01877 |
TEXTURE_GLSZM_NET_T1Gd_ZSV* | 0 | 0 | 0 | −2.34 | 0.01906 |
TEXTURE_GLRLM_NET_T1Gd_GLV* | 2.00E+42 | 2230.45 | 1.79E+81 | 2.13 | 0.0333 |
HISTO_ET_T1_Bin10 | 0.81 | 0.63 | 1.05 | −1.59 | 0.11219 |
TEXTURE_GLCM_ET_T2_SumAverage | 0 | 0 | 9.95E+83 | −1.57 | 0.11569 |
TEXTURE_GLRLM_NET_T1_LGRE | 0 | 0 | 2.73E+38 | −1.49 | 0.13526 |
TEXTURE_GLRLM_ED_T1_RLV | inf | 0 | inf | 1.4 | 0.16185 |
HISTO_ED_T2_Bin4 | 0.96 | 0.88 | 1.05 | −0.91 | 0.36241 |
TEXTURE_GLCM_ED_FLAIR_Energy | inf | 0 | inf | 0.78 | 0.43387 |
TEXTURE_GLSZM_NET_T1_LZLGE | 0.99 | 0.96 | 1.03 | −0.39 | 0.69976 |
Image features | Associated gene modules | Number of associated genes |
HISTO_ED_T2_Bin8 | module2, module4, module5, module7 | 2794 |
TEXTURE_GLSZM_NET_T1Gd_ZSV | module6 | 506 |
TEXTURE_GLRLM_NET_FLAIR_LRHGE | module6 | 506 |
TEXTURE_GLRLM_NET_T1Gd_GLV | module2, module4, module6, module8, module10 | 1421 |
Image feature | Number of genes in associated modules | Optimal λ | RMSE | Number of genes selected by Lasso |
HISTO_ED_T2_Bin8 | 2794 | 1.6627 | 6.0847 | 12 |
TEXTURE_GLSZM_NET_T1Gd_ZSV | 506 | 7.81E-06 | 2.0195E-5 | 3 |
TEXTURE_GLRLM_NET_FLAIR_LRHGE | 506 | 163.9677 | 528.16 | 6 |
TEXTURE_GLRLM_NET_T1Gd_GLV | 1421 | 3.01E-03 | 0.0120 | 18 |
Populations sizes | 20 | 25 | 30 | 35 | 40 |
α1 | 0.2926 | 0.3187 | 0.2792 | 0.3303 | 0.276 |
α2 | 0.0663 | 0.0394 | 0.0739 | 0.0505 | 0.068 |
α3 | 0.2171 | 0.2329 | 0.2076 | 0.2214 | 0.2102 |
α4 | 0.0091 | 0.019 | 0.0107 | 0.0298 | 0.0159 |
β | 0.4149 | 0.39 | 0.4288 | 0.368 | 0.4298 |
C-index | 0.8065 | 0.807 | 0.8061 | 0.807 | 0.8057 |
Image features | f1 | f2 | f3 | f4 | g | f |
C-index | 0.6945 | 0.7321 | 0.7926 | 0.7985 | 0.7627 | 0.8071 |