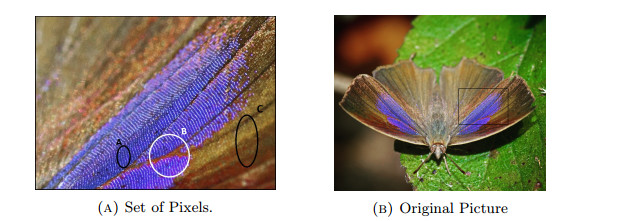
In this paper, we introduce the concept of rough semi-uniform spaces as a supercategory of rough pseudometric spaces and approximation spaces. A completion of approximation spaces has been constructed using rough semi-uniform spaces. Applications of rough semi-uniform spaces in the construction of proximities of digital images is also discussed.
Citation: Surabhi Tiwari, Pankaj Kumar Singh. Rough semi-uniform spaces and its image proximities[J]. Electronic Research Archive, 2020, 28(2): 1095-1106. doi: 10.3934/era.2020060
[1] | Surabhi Tiwari, Pankaj Kumar Singh . Rough semi-uniform spaces and its image proximities. Electronic Research Archive, 2020, 28(2): 1095-1106. doi: 10.3934/era.2020060 |
[2] | Duraisamy Balraj, Muthaiah Marudai, Zoran D. Mitrovic, Ozgur Ege, Veeraraghavan Piramanantham . Existence of best proximity points satisfying two constraint inequalities. Electronic Research Archive, 2020, 28(1): 549-557. doi: 10.3934/era.2020028 |
[3] | Francisco Javier García-Pacheco, Ramazan Kama . $ f $-Statistical convergence on topological modules. Electronic Research Archive, 2022, 30(6): 2183-2195. doi: 10.3934/era.2022110 |
[4] | Li-Bin Liu, Ying Liang, Jian Zhang, Xiaobing Bao . A robust adaptive grid method for singularly perturbed Burger-Huxley equations. Electronic Research Archive, 2020, 28(4): 1439-1457. doi: 10.3934/era.2020076 |
[5] | Weiran Ding, Jiansong Li, Yumeng Jia, Wanru Yang . Tractability of $ L_2 $-approximation and integration over weighted Korobov spaces of increasing smoothness in the worst case setting. Electronic Research Archive, 2025, 33(2): 1160-1184. doi: 10.3934/era.2025052 |
[6] | Huixia Liu, Zhihong Qin . Deep quantization network with visual-semantic alignment for zero-shot image retrieval. Electronic Research Archive, 2023, 31(7): 4232-4247. doi: 10.3934/era.2023215 |
[7] | Han-Cheng Dan, Yongcheng Long, Hui Yao, Songlin Li, Yanhao Liu, Quanfeng Zhou . Investigation on the fractal characteristic of asphalt pavement texture roughness incorporating 3D reconstruction technology. Electronic Research Archive, 2023, 31(4): 2337-2357. doi: 10.3934/era.2023119 |
[8] | Haibo Cui, Junpei Gao, Lei Yao . Asymptotic behavior of the one-dimensional compressible micropolar fluid model. Electronic Research Archive, 2021, 29(2): 2063-2075. doi: 10.3934/era.2020105 |
[9] | Xintao Li, Rongrui Lin, Lianbing She . Periodic measures for a neural field lattice model with state dependent superlinear noise. Electronic Research Archive, 2024, 32(6): 4011-4024. doi: 10.3934/era.2024180 |
[10] | Wenbin Zheng, Jinjin Li, Shujiao Liao . Decision self-information based on parameterized fuzzy β neighborhood and its application in three-way multi-attribute group decision-making. Electronic Research Archive, 2022, 30(12): 4553-4573. doi: 10.3934/era.2022231 |
In this paper, we introduce the concept of rough semi-uniform spaces as a supercategory of rough pseudometric spaces and approximation spaces. A completion of approximation spaces has been constructed using rough semi-uniform spaces. Applications of rough semi-uniform spaces in the construction of proximities of digital images is also discussed.
Various topological notions have been involved in the conceptual frameworks of data analysis theories and successfully applied in solving many problems and tasks in science and engineering, e.g., image processing, forgery detection, determining the age of fossils, pattern recognition, image classification, etc. Rough set theory is the quintessence of data processing methodology, which is infused with topological concepts (see [13]). Another great exemplar is given by near set theory, which has shifted from standard topologies towards uniformities. The near set theory was introduced by Peters and had applications in various fields [8,9,11]. Peters et al. used the concept of descriptive proximity to study digital images. In [10], Peters et al. used rough sets and nearness-like structures to describe and compare visual objects. Pessoa et al. [7] used the concept of rough sets theory for image classification. In this paper, we use semi-uniform spaces in the framework of rough sets to study nearness of digital images.
Zdzisław Pawlak used an equivalence relation on a non-empty set to introduce approximation spaces during the early 1980s for the classification of objects employing attributes of information systems [6]. The rough set theory is an extension of the set theory mainly used for the study of intelligent systems characterized by insufficient and incomplete information. There is a close similitude between rough set theory and general topology. Topology is a rich source of constructs that can be helpful to enrich the original modal of approximation spaces [14,15]. The approximation operators (upper approximation and lower approximation) are topological operators (closure and interior, respectively). Conjoint study of topology and rough set theory has been widely applied to many real-world problems and in the development of the new mathematical structures [13,14,15].
Biswas firstly gave the idea of rough distance [14]. In [14,15], we defined a proximity relation over an approximation space by using the concept of a rough metric and named it a rough proximity relation. In [15], we studied the compactification of rough proximity spaces. It is well known that every proximity
In practice, an information system describes a finite sample
In this paper, we introduce the notion of rough semi-uniform spaces as a supercategory of rough pseudometric spaces and construct its completion by using the concept of generalized Cauchy sequences [5]. The cardinal motive of this paper is the construction of a semi-uniform structure on an approximation space to study the completion of an approximation space. The category of rough semi-uniform spaces is shown to be a supercategory of the categories of metric spaces, ultrametric spaces and rough metric spaces with their respective mappings. Therefore, the study in this paper presents a unified study of all these spaces. Finally, the application of rough semi-uniform spaces in the study of proximities of digital images is also investigated. Image proximities are used in the classification of digital images that have applications in image analysis, pattern recognition, fluid dynamics and many more [2,3,4,9,17,18].
In this paper, we deal with the concept of rough sets defined by Yao [20], which are a generalized form of Pawlak's rough sets. Throughout this paper, let
Let
¯R(A)={x:R(x)∩A≠∅},R_(A)={x:R(x)⊆A},A⊆U. |
A set
Remark 2.1. Relation between
Throughout the paper, we restrict ourselves to the tolerance relation
Definition 2.1. [9]. Let
1.
2. If
3. If
The pair
Closure operation was proposed by Riesz as a primitive topological notion [14].
Definition 2.2. [14]. Let
1.
2.
3.
Further,
In general way of representation, topology deals with structures "a point is near to a set", while proximity structures [5] are finer than topology and are based on the concept "one set is near to another set". The following definition axiomatize a proximity space.
Definition 2.3. [5]. Let
1.
2.
3.
4.
A binary relation
5.
and
where means that the set
The pair
If a basic proximity
AδB and {b}δC, for each b∈B⇒AδC; |
then
Let
clδ(A)={x∈U:{x}δA}. |
Every proximity
Definition 2.4. [5]. Let
1.
2.
3.
4. If
A semi-uniform space is a struct
Sometimes the axiom '
Remark 2.2. There is a natural transformation from a uniform space to a proximity space, i.e., every uniform space gives rise to a proximity space.
In this section, we define a rough semi-uniform space as a supercategory of approximation spaces, rough pseudometric spaces and pseudometric spaces. Some results on rough semi-uniform spaces are proved. Examples are constructed to well support the theory of rough semi-uniform spaces. Finally, we construct the completion of a rough semi-uniform space. The upper approximation of a set
Definition 3.1. [14] Let
The Čech rough closure operator returns sets divorced from
Definition 3.2. Let
Let
● Let
● Let
● The semi-uniform spaces can be embedded into rough semi-uniform spaces with their respective mappings (consider the relation
Let
Now, we will show that the category of rough semi-uniform spaces is a super category of the category of rough pseudometric spaces. In the process, let us first define rough pseudometric spaces. Biswas defined a rough metric on Pawlak's approximation spaces [6] and discussed the properties of rough metric spaces (see [15]). Equivalently, a rough pseudometric on a Yao's approximation space can be defined as follows:
Definition 3.3. [14] Let
1.
2.
3.
The pair
If
Proposition 3.1. Let
Thus, the category of rough pseudometric spaces and hence the category of pseudometric spaces can be embedded into the category of rough semi-uniform spaces, with their respective mappings. We now present some examples of rough semi-uniform spaces.
Example 3.1. Let
Remark 3.1. From Example 3.1, it is clear that the category of approximation spaces can be embedded into the category of rough semi-uniform spaces with their respective mappings.
Example 3.2. Let
Example 3.3. Let
Let
In Example 3.3, we can easily verify that the closure operator
Example 3.4. Let
Keeping in mind the importance of complete (well-behaved) spaces (discussed in the Introduction section), we will construct the completion of a rough semi-uniform space. Since the category of approximation spaces, rough pseudometric spaces, pseudometric spaces are embedded into the category of rough semi-uniform spaces, therefore, the theory developed in this section includes the study of all these aforementioned topological structures. In the process, we first define rough filters and rough Cauchy filters and use these concepts to construct the completion of a rough semi-uniform space.
Definition 3.4. A collection
1.
2.
Definition 3.5. Let
Definition 3.6. Let
Theorem 3.7. Let
ˆU=U∪{ζR:ζR is a non−convergent rough Cauchy filter on (U,UR)}. |
Let
xR′y iff xRy for all,x,y∈U and ζRR′ηR iff ζR=ηR for all,ζR,ηR∈ˆU−U. |
Let
Proof. Clearly
Remark 3.2. Let
The idea of perceptual nearness was introduced by Peters et al. when they used the concept of probe functions to classify digital images [11]. Later, probe functions were used to define descriptive nearness [2] between granules of a digital image. Probe functions work on feature vectors to perceptually differentiate digital objects. Descriptive nearness measures the perceptual nearness of objects, which are digital images that can be spatially far but may have similar feature vectors. Tolerance spaces and a perceptual approach in image analysis can be found in [8]. A set is a near perceptual set if and only if it is never empty, and it contains pairs of perceived objects that have descriptions that are within some tolerance of each other. From the beginning, the near set approach to perception has had direct links to rough sets in its approach to the perception of objects and the classification of objects. This is evident in the early work on the nearness of objects and the extension of the approximation space model (see [2,3,4,8,9,17,18]). In [10], Peters et al. proved that two sets could be descriptively near if their upper approximations intersect. This motivated us to define Čech rough proximity spaces [14] for the purpose of studying image proximities. In this section, we gave a natural transformation of a rough semi-uniform space to a Čech rough proximity space. We used this transformation to give the perceptual applications of rough semi-uniform structures.
Example 4.2 gives the classification of digital images using a rough semi-uniformity. In Example 4.3, we use a rough semi-uniformity to distinguish the velocities of the fluid particles. First of all, let us define a Čech rough proximity space.
Definition 4.1. [14] Let
P1.
P2.
P3.
P4.
The relation
Proposition 4.1.
Then
There is a natural transformation of a rough semi-uniform space to a Čech rough proximity space which can be seen in the following theorem.
Theorem 4.2. Let
AδRB iff (A∗×B∗)∩P≠∅, for all P∈UR, |
where
Proof. Properties P1, P3 and P4 are obvious. For P2, if
Example 4.1. Let
Now, we present the illustration of rough semi-uniform spaces in finding the proximities of digital images. The illustration of image proximities in a butterfly and color thresholding in the context of nearness-like structures have been extensively used in [8,9].
Example 4.2. Consider the image of a butterfly in Figure
d:U×U→R as:d(p,q):=max{∣pr−qr∣,∣pg−qg∣,∣pb−qb∣}. |
Define a tolerance relation
p1Rp2 iff d(p1,p2)≤5. |
Thus the neighborhood
Uε:={(R(x),R(y)):d(x,y)<5}, for every real number ε>0. |
Then
AδURB iff (A∗×B∗)∩Uε≠∅, for all Uε∈UR. |
Here
Let we can distinguish different kinds of objects in a picture on a digital platform.
In the next example, we discuss the application of a rough semi-uniformity to distinguish the velocity of a fluid at different time and space.
Example 4.3. Let us consider a two dimensional fluid flow past a cylinder. The contours in Figure
f(p,q)=max{∣pr−qr∣,∣pg−qg∣,∣pb−qb∣}. |
Using this function, define a tolerance relation
p1Rp2 iff f(p1,p2)≤7. |
Thus the neighborhood
R(x)PR(y) iff R(x)∩R(y)≠∅. |
Define
Let
We have discussed here the concept of semi-rough uniformity over an approximation space, which provides us topology over the universe
Furthermore, completion is an essential requirement for the study of various topological problems, such as finding fixed points of a map. Therefore, completing a rough semi-uniform space opens the way of finding rough fixed points of a rough contraction mapping. This forms the content of our future research [12].
[1] |
Completion of semi-uniform spaces. Appl. Categ. Structures (2007) 15: 483-491. ![]() |
[2] |
Descriptive proximities. Properties and interplay between classical proximities and overlap. Math. Comput. Sci. (2018) 12: 91-106. ![]() |
[3] |
Rough-set-based color channel selection. IEEE Geoscience and Remote Sensing Letters (2017) 14: 52-56. ![]() |
[4] |
Signature-based perceptual nearness: Application of near sets to image retrieval. Math. Comput. Sci. (2013) 7: 71-85. ![]() |
[5] |
S. A. Naimpally and B. Warrack, Proximity Spaces, Reprint of the 1970 original [MR0278261].
Cambridge Tracts in Mathematics, 59. Cambridge University Press, Cambridge, 2008. doi: 10.1017/CBO9780511569364
![]() |
[6] |
Rough sets. Internat. J. Comput. Inform. Sci. (1982) 11: 341-356. ![]() |
[7] |
A. S. Aguiar Pessoa, S. Stephany and L. M. Garcia Fonseca, Feature selection and image classification using rough sets theory, 2011 IEEE International Geoscience and Remote Sensing Symposium, (2011), 2904–2907. doi: 10.1109/IGARSS.2011.6049822
![]() |
[8] |
J. F. Peters, Computational Proximity. Excursions in the Topology of Digital Images, Intelligent Systems Reference Library, 102. Springer, [Cham], 2016. doi: 10.1007/978-3-319-30262-1
![]() |
[9] |
J. F. Peters, Topology of Digital Images: Visual Pattern Discovery in Proximity Spaces, Intell. Syst. Ref. Libr., Springer, 2014. doi: 10.1007/978-3-642-53845-2
![]() |
[10] |
Nearness of visual objects. Application of rough sets in proximity spaces. Fund. Inform. (2013) 128: 159-176. ![]() |
[11] |
Foundation of near sets. Inform. Sci. (2009) 179: 3091-3109. ![]() |
[12] | P. K. Singh and S. Tiwari, A fixed point theorem in rough semi-linear uniform spaces, Submitted. |
[13] |
P. K. Singh and S. Tiwari, Topological structures in rough set theory: A survey, Hacet. J. Math. Stat., (2020), 1–25. doi: 10.15672/hujms.662711
![]() |
[14] | Čech rough proximity spaces. Mat. Vesnik (2020) 72: 6-16. |
[15] |
An approach of proximity in rough set theory. Fund. Inform. (2019) 166: 251-271. ![]() |
[16] | M. Vlach, Algebraic and topological aspects of rough set theory, Fourth International Workshop on Computational Intelligence and Application-IEEE, SMC, (2008), 23–30. |
[17] |
Granular computing: Topological and categorical aspects of near and rough set approaches to granulation of knowledge. Lecture Notes in Comput. Sci. (2013) 7736: 34-52. ![]() |
[18] |
Perception and classification. A note on near sets and rough sets. Fund. Inform. (2010) 101: 143-155. ![]() |
[19] | Some mathematical structures of generalized rough sets in infinite universes of discourse. Lecture Notes in Comput. Sci. (2011) 6499: 175-206. |
[20] |
Relational interpretations of neighborhood operators and rough set approximation operators. Inform. Sci. (1998) 111: 239-259. ![]() |
1. | Amani A. Rawshdeh, Heyam H. Al-jarrah, Surabhi Tiwari, Abdalla A. Tallafha, Soft semi-linear uniform spaces and their perceptual application, 2022, 10641246, 1, 10.3233/JIFS-220587 | |
2. | T. M. G. Ahsanullah, Rough uniformity of topological rough groups and L-fuzzy approximation groups, 2022, 43, 10641246, 1129, 10.3233/JIFS-212634 | |
3. | Pankaj Kumar Singh, Surabhi Tiwari, A fixed point theorem in rough semi-linear uniform spaces, 2021, 851, 03043975, 111, 10.1016/j.tcs.2020.11.011 | |
4. | Surabhi Tiwari, Pankaj Kumar Singh, Smirnov type compactification of rough pseudo metric spaces using proximity approach, 2021, 32, 1012-9405, 77, 10.1007/s13370-020-00811-3 | |
5. | S. Atmaca, I. Zorlutuna, Continuity on r-near topological spaces, 2021, 41, 10641246, 3629, 10.3233/JIFS-211017 | |
6. | Nandini Upadhyay, Ashutosh Pandey, 2023, 2755, 0094-243X, 040039, 10.1063/5.0140789 | |
7. | Virendra Kumar, Surabhi Tiwari, A survey on topological structures on fuzzy rough sets, 2024, 35, 1012-9405, 10.1007/s13370-024-01181-w | |
8. | Satya Narayan Shukla, Surabhi Tiwari, H-partial uniform spaces and their application in the compression of digital images, 2024, 25, 1989-4147, 441, 10.4995/agt.2024.20973 | |
9. | Virendra Kumar, Surabhi Tiwari, L-Fuzzy rough point-wise proximity spaces, 2025, 26, 1989-4147, 255, 10.4995/agt.2025.21682 |