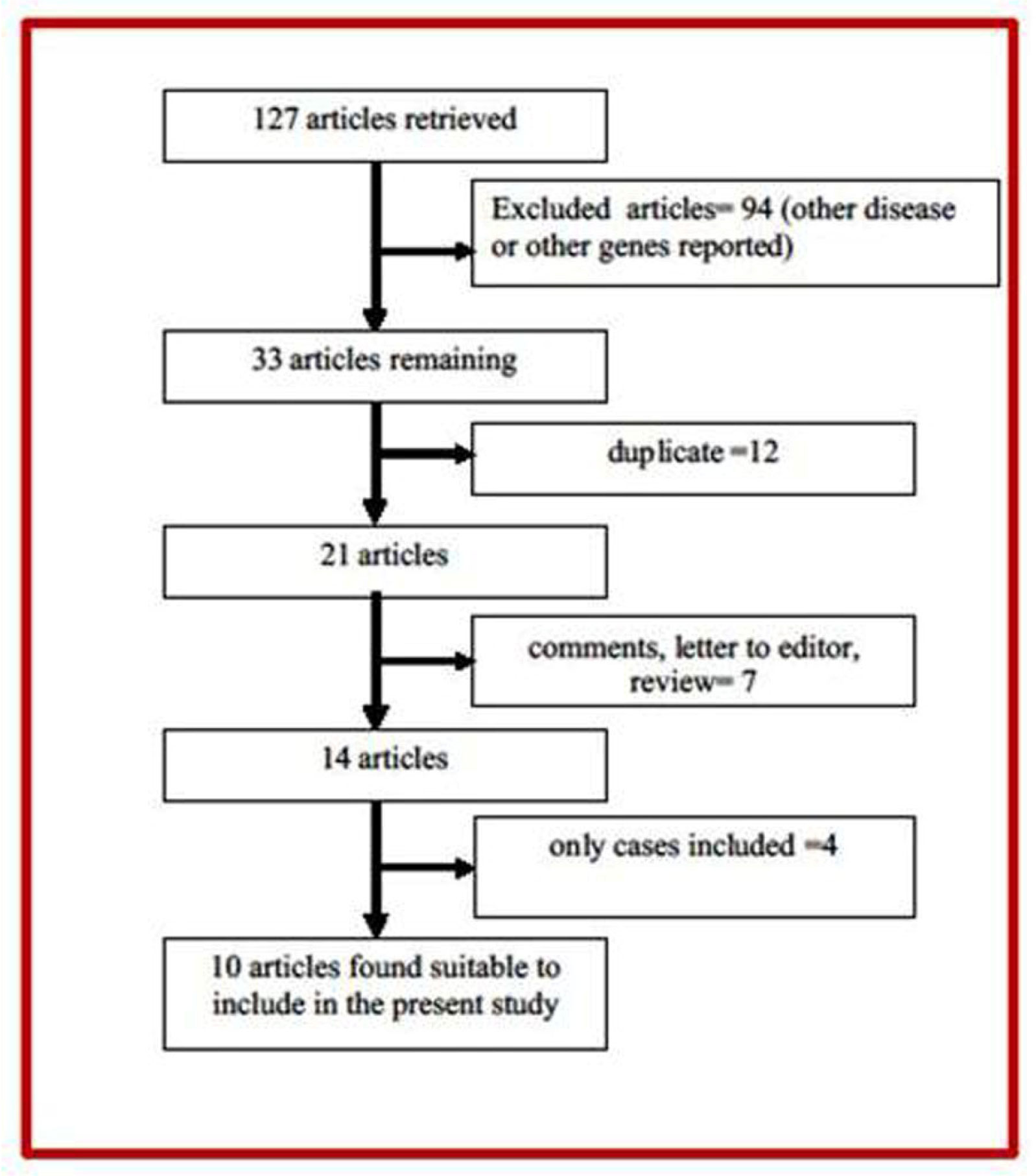
This study examined the misalignment between cognitive development and mathematics education in senior secondary schools. Using an ex post facto research design and a descriptive survey approach, data were collected from the Senior Secondary Mathematics Achievement Test (SSMAT), which showed a strong reliability coefficient (r = 0.89). The analysis, which included descriptive statistics and independent sample t-tests, revealed that gender does not have a significant impact on student's performance in SSS2 mathematics across different content areas (number and numeration, algebra, geometry, and everyday statistics) and cognitive dimensions (recall, conceptual understanding, and reasoning). The results highlight the need for a comprehensive approach to mathematics education that balances content coverage and the development of cognitive skills. Addressing this misalignment can improve students' ability to creatively apply mathematical concepts, think critically, and effectively communicate their mathematical ideas, ultimately enhancing overall academic achievement. Our study identifies clear gaps in current educational assessment methods, emphasizing the importance of focusing on content and cognitive dimensions. The results will assist in developing targeted instructional strategies that align with cognitive demands, improve teaching effectiveness, and inform educational policy to enhance student outcomes in mathematics.
Citation: Joshua Oluwatoyin Adeleke, Hameed Adedeji Balogun, Musa Adekunle Ayanwale. Assessment of content and cognitive dimensions of learners' mathematics performance[J]. STEM Education, 2025, 5(3): 383-400. doi: 10.3934/steme.2025019
[1] | Qianqian Zhang, Mingye Mu, Heyuan Ji, Qiushi Wang, Xingyu Wang . An adaptive type-2 fuzzy sliding mode tracking controller for a robotic manipulator. Electronic Research Archive, 2023, 31(7): 3791-3813. doi: 10.3934/era.2023193 |
[2] | Lingling Zhang . Vibration analysis and multi-state feedback control of maglev vehicle-guideway coupling system. Electronic Research Archive, 2022, 30(10): 3887-3901. doi: 10.3934/era.2022198 |
[3] | Duhui Chang, Yan Geng . Distributed data-driven iterative learning control for multi-agent systems with unknown input-output coupled parameters. Electronic Research Archive, 2025, 33(2): 867-889. doi: 10.3934/era.2025039 |
[4] | Zhizhou Zhang, Yueliang Pan, Weilong Zhao, Jinchu Zhang, Zheng Zi, Yuan Xie, Hehong Zhang . Frequency analysis of a discrete-time fast nonlinear tracking differentiator algorithm based on isochronic region method. Electronic Research Archive, 2024, 32(9): 5157-5175. doi: 10.3934/era.2024238 |
[5] | Jian Gong, Yuan Zhao, Jinde Cao, Wei Huang . Platoon-based collision-free control for connected and automated vehicles at non-signalized intersections. Electronic Research Archive, 2023, 31(4): 2149-2174. doi: 10.3934/era.2023111 |
[6] | Chao Ma, Hang Gao, Wei Wu . Adaptive learning nonsynchronous control of nonlinear hidden Markov jump systems with limited mode information. Electronic Research Archive, 2023, 31(11): 6746-6762. doi: 10.3934/era.2023340 |
[7] | Tongtong Yu, Zhizhou Zhang, Yang Li, Weilong Zhao, Jinchu Zhang . Improved active disturbance rejection controller for rotor system of magnetic levitation turbomachinery. Electronic Research Archive, 2023, 31(3): 1570-1586. doi: 10.3934/era.2023080 |
[8] | Xingting Geng, Jianwen Feng, Yi Zhao, Na Li, Jingyi Wang . Fixed-time synchronization of nonlinear coupled memristive neural networks with time delays via sliding-mode control. Electronic Research Archive, 2023, 31(6): 3291-3308. doi: 10.3934/era.2023166 |
[9] | Ning Li, Yuequn Gao . Modified fractional order social media addiction modeling and sliding mode control considering a professionally operating population. Electronic Research Archive, 2024, 32(6): 4043-4073. doi: 10.3934/era.2024182 |
[10] | Chao Yang, Juntao Wu, Zhengyang Qiao . An improved fixed-time stabilization problem of delayed coupled memristor-based neural networks with pinning control and indefinite derivative approach. Electronic Research Archive, 2023, 31(5): 2428-2446. doi: 10.3934/era.2023123 |
This study examined the misalignment between cognitive development and mathematics education in senior secondary schools. Using an ex post facto research design and a descriptive survey approach, data were collected from the Senior Secondary Mathematics Achievement Test (SSMAT), which showed a strong reliability coefficient (r = 0.89). The analysis, which included descriptive statistics and independent sample t-tests, revealed that gender does not have a significant impact on student's performance in SSS2 mathematics across different content areas (number and numeration, algebra, geometry, and everyday statistics) and cognitive dimensions (recall, conceptual understanding, and reasoning). The results highlight the need for a comprehensive approach to mathematics education that balances content coverage and the development of cognitive skills. Addressing this misalignment can improve students' ability to creatively apply mathematical concepts, think critically, and effectively communicate their mathematical ideas, ultimately enhancing overall academic achievement. Our study identifies clear gaps in current educational assessment methods, emphasizing the importance of focusing on content and cognitive dimensions. The results will assist in developing targeted instructional strategies that align with cognitive demands, improve teaching effectiveness, and inform educational policy to enhance student outcomes in mathematics.
Alcohol dependence (AD) or Alcoholism, also regarded as alcohol use disorder (AUD), is a complex and relapsing neuropsychiatric disorder [1],[2]. World Health Organization (WHO) reported that approximately 140 million individuals addicted to alcohol globally, resulting in to 2.5 million death each year [3]. AD is regarded as a “reward deficiency syndrome” that intemperately affects public health [4],[5]. It has been found to be influenced by both genetic and environmental factors [6],[7]. Exact patho-physiological and molecular mechanism of AD is not known yet. However, molecular genetic studies support that multiple genes determine an individual's predisposition to AD [8]. Heritability of AD likely plays an important role in its development and is determined to be moderate to high [9],[10]. It was reported frequently that alcohol consumption increased homocysteine (Hcy) concentration i.e hyperhomocysteinaemia [11]. However, inconsistent results of the combined effect of both positive and negative association have been reported between alcohol intake and Hcy [12]. Hyperhomocystenemia is already reported as risk factor for several diseases or disorders including neural tube defects, Alzheimer disease, schizophrenia, pregnancy complications, cardiovascular diseases, noninsulin dependent diabetes and end-stage renal disease as evidenced from several studies [13].
Homocysteine is a sulfur containing amino acid, several genetic and environmental risk factor are reported for higher plasma concentration of homocysteine [14]. Homocysteine (Hcy) is synthesized in methionine and folate cycle by demethylation of methionine. 5,10-methylenetetrahydrofolate reductase (MTHFR) enzyme of folate cycle plays an important role in homocysteine metabolism. MTHFR gene is present on chromosome 1p36.3. Numerous single nucleotide polymorphisms (SNP) are known in MTHFR gene like C677T and A1298C etc [15],[16]. The most clinically important and studies polymorphism is C677T (rs1801133), in which cytosine (C) is substituted with thymine (T) at 677 nucleotide position and consequently alanine is replaced by valine in MTHFR enzyme (Ala 222 Val) [17],[18]. The variant MTHFR enzyme is thermolabile with reduced activity (~70%) and it increased the plasma homocysteine concentrations [15] (Frosst et al., 1995). Globally, frequency of mutant T allele varies greatly [19]–[23]. Yadav et al. [23] have conducted a comprehensive C667T polymorphism study and reported the highest frequency in European populations ranging from 24.1% to 64.3% and, lowest frequency from African population. Several studies revealed association of MTHFR gene C677T polymorphism with AD. However, findings showed inconsistent results [24]–[26]. To derive a more precise estimation of the relationship, authors have performed a meta-analysis.
Present meta-analysis is carried out according to MOOSE (Meta-analysis of observational studies in epidemiology) guidelines.
Articles were retrieved through Pubmed, Google scholar, Springer Link, and Science Direct databases up to February 28, 2020, using following key words: “Methylenetetrahydrofolate reductase” or “MTHFR” or “C677T” or “rs1801133” or “polymorphism” and “Alcohol dependence” or “Alcoholism” or “AD” or “Addiction”.
Inclusion criteria were following: (1) MTHFR C677T polymorphism and alcohol dependence association was investigated in the study, (2) MTHFR C677T genotype/allele numbers in alcohol dependence cases and controls were given in the study, (3) sufficient information for calculating the odds ratio (OR) with 95% confidence interval (CI) and (4) Articles published in English language were only considered. Major reasons for studies exclusion were as follows: (1) no alcohol dependence cases analyzed, (2) the C677T polymorphism details information missing, and (3) duplicate article.
Name of first author, country name, number of cases and controls, number of genotypes in cases and controls and journal name with full reference from each article were extracted.
All analysis were done according to the method of Rai et al. [27]. Odds ratio (ORs) with 95% confidence intervals (CIs) were calculated using fixed effect and random effect models [28],[29]. A five genetic models viz. allele contrast, co-dominant, homozygote, dominant and recessive models were calculated. Heterogeneity was investigated and quantified by I2 statistic [30]. Chi-squared analysis was used to determine whether the genotype distribution of control group was in Hardy–Weinberg equilibrium or not. Subetaoup analysis was conducted by ethnicity. In included articles, case samples were not categorized on the basis of gender, so the subetaoup analysis based on gender did not performed in present meta-analysis. Publication bias was investigated by Egger's regression intercept test [31]. P value <0.05 was considered statistically significant. All calculations were done by softwares MIX version 1.7 [32] and MetaAnalyst [33] program.
Selection of studies is given in fow diagram (Figure 1). Following the exclusion criteria, 10 individual case-control studies with a total of 1676 cases and 1594 controls were included into this meta-analysis [24]–[26],[34]–[40]. One author [38] reported their data in to two categories, we included both set of data as different studies. Hence, total number of included studies in present meta-analysis is eleven (Table 1).
Study | Ethnicity | Control | Case | Case Genotype |
Control Genotype |
HWE p-value of controls | ||||
CC | CT | TT | CC | CT | TT | |||||
Bonsch, 2006 | Caucasian | 115 | 134 | 64 | 56 | 14 | 60 | 41 | 14 | 0.10 |
Lutz, 2006 | Caucasian | 102 | 221 | 95 | 94 | 32 | 53 | 41 | 8 | 0.98 |
Lutz, 2007 | Caucasian | 101 | 142 | 65 | 58 | 19 | 53 | 40 | 8 | 0.90 |
Saffroy, 2008 | Caucasian | 93 | 242 | 108 | 113 | 21 | 35 | 41 | 17 | 0.41 |
Benyamina, 2009 | Caucasian | 93 | 120 | 56 | 53 | 11 | 35 | 41 | 17 | 0.41 |
Fabris, 2009 | Caucasian | 236 | 63 | 17 | 35 | 11 | 69 | 113 | 54 | 0.55 |
Shin, 2010 | Asian | 232 | 68 | 11 | 39 | 18 | 42 | 129 | 61 | 0.07 |
Supic, 2010, Heavy Alcoholic | Caucasian | 57 | 32 | 13 | 9 | 10 | 27 | 24 | 6 | 0.84 |
Supic, 2010, Non heavy Alcoholic | Caucasian | 105 | 64 | 37 | 23 | 4 | 53 | 42 | 10 | 0.69 |
Singh, 2014 | Asian | 313 | 139 | 91 | 44 | 4 | 228 | 78 | 7 | 0.91 |
Singh, 2015 | Asian | 147 | 451 | 312 | 125 | 14 | 107 | 35 | 5 | 0.32 |
Overall, eleven studies provided 1676/1594 cases/controls for MTHFR C677T polymorphism. The prevalence of C and T alleles in AD cases was 71.22% and 28.79% respectively. The percentage frequency of TT genotype among cases and controls was 9.43% and 12.98%, respectively whereas prevalence of CT heterozygote among AD cases was 38.72% and 39.21% in controls. The prevalence of CC homozygote among AD cases and controls was 51.85% and 47.80%, respectively. Genotypes were in Hardy-Weinberg equilibrium in all controls. In control group the percentage of C and T allele frequencies was 67.41% and 32.59% respectively (Figure 2).
No significant association was observed between the MTHFR C677T polymorphism and the susceptibility to AD in all the genetic models using random effect model (for T vs. C (allele contrast): OR = 1.04, 95% CI = 0.88–1.24; CT vs. CC (co-dominant): OR = 1.02, 95% CI = 0.62–1.68; for TT+CT vs. CC (dominant): OR = 1.10, 95% CI = 0.94–1.29; for TT vs. CC (homozygote): OR = 1.01, 95% CI = 0.66–1.51; for TT vs. CT + CC (recessive): OR = 0.97, 95% CI = 0.66–1.40) (Table 2; Figures 3).
A true heterogeneity existed between studies for allele contrast (Pheterogeneity = 0.02, Q = 20.64, I2 = 51.56%, t2 = 0.04, z = 0.69), co-dominant genotype (Pheterogeneity < 0.0001, Q = 86.64, I2 = 88.46%, t2 = 0.61, z = 4.29), homozygote genotype (Pheterogeneity = 0.02, Q = 20.93, I2 = 52.24%, t2 = 0.24, z = 0.1), and recessive genotype (Pheterogeneity = 0.02, Q = 21.00, I2 = 52.40%, t2 = 0.20, z = 0.47) comparisons. The “I2” value of more than 50% shows high level of true heterogeneity.
Genetic Models | Fixed effect OR (95% CI), p | Random effect OR (95% CI), p | Heterogeneity p-value (Q test) | I2 (%) | Publication Bias (p of Egger's test) |
Allele Contrast (T vs. C) | 1.04 (0.92–1.17), 0.48 | 1.04 (0.88–1.24), 0.60 | 0.02 | 51.56 | 0.62 |
Dominant (TT+CT vs. CC) | 1.41 (1.20–1.65), <0.0001 | 1.02 (0.62–1.68), 0.92 | <0.0001 | 88.46 | 0.06 |
Homozygote (TT vs. CC) | 0.98 (0.75–1.29), 0.92 | 1.01 (0.66–1.51), 0.97 | 0.02 | 52.24 | 0.26 |
Co-dominant (CT vs. CC) | 1.10 (0.94–1.29), 0.21 | 1.10 (0.94–1.29), 0.21 | 0.43 | 0 | 0.48 |
Recessive (CC+CT vs. TT) | 0.94 (0.73–1.20), 0.63 | 0.97 (0.66–1.40), 0.86 | 0.02 | 52.4 | 0.28 |
Out of 11 studies included in the present meta-analysis, 3 studies were carried out in Asian countries, and 8 studies were carried out on Caucasian (Table 1). The subetaoup analysis by ethnicity did not reveal any significant association between MTHFR C677T polymorphism and AD in Asian population (T vs. C: OR = 1.16; 95% CI = 0.93–1.44; p = 0.17; I2 = 3.1%; Pheterogeneity = 0.65; TT vs. CC: OR = 1.16; 95% CI = 0.62–2.02; p = 0.69; I2 = 3.1%; Pheterogeneity = 0.89; and TT+CT vs. CC: OR = 1.26; 95% CI = 0.96–1.67; p = 0.09; I2 = 3.1%; Pheterogeneity = 0.81); and Caucasian population (T vs. C: OR = 0.99; 95% CI = 0.86–1.14; p = 0.93; I2 = 61.75%; Pheterogeneity = 0.01; TT vs. CC: OR= 0.95; 95% CI = 0.70–1.29; p = 0.75; I2 = 65.63%; Pheterogeneity = 0.004; and TT+CT vs. CC: OR = 1.03; 95% CI = 0.85–1.25; p = 0.75; I2 = 13.93%; Pheterogeneity = 0.32) (Figures 4 and 5).
Symmetrical shape of Funnel plots' revealed absence of publication bias. P values of Egger's test were more than 0.05, also provided statistical evidence for the funnel plots' symmetry (p = 0.62 for T vs. C; p = 0.48 for TT vs. CC; p = 0.26 for CT vs. CC; p = 0.48 for TT+AC vs. CC; p = 0.28 for TT vs. CT+CC) (Table 2; Figure 6).
In vivo and in vitro studies has demonstrated that homocysteine has neurotoxic effects especially on dopamine neurons of reward pathway [11]. In addition, hyperhomocysteinemia is also reported in AD [11],[41]. In MTHFR gene several polymorphisms are reported but according to deficit hypothesis of addiction, only C677T polymorphism-dependent alteration of the reward system possibly leads to alcohol addiction. Further, homovanillic acid (HVA) is a potential indicator of central dopaminergic neuronal activity [42] and experimentally, it was demonstrated that higher concentration of homocysteine lowers the level of HVA in rat striatum region [43]. On the basis of 11 studies providing data on MTHFR C677T genotype and AD risk in two ethnic populations, including over 3,205 subjects, our meta-analysis provides an evidence that TT and CT genotypes or T allele are not associated with AD risk. Hence the present meta-analysis indicated that C677T is not a risk factor of AD.
Meta-analysis is a statistical tool to combine the information of independent case-control studies with similar target [44]. Several meta-analysis are published, which evaluated effects of folate pathway genes polymorphisms in susceptibility of diseases/disorders- cleft lip and palate [45], down syndrome [46]–[48], male infertility [49], bipolar disorder [50], schizophrenia [51],[52], depression [53], obsessive compulsive disorder [54], hyperurecemia [55], epilepsy [56], Alzheimers disease [57], esophageal cancer [58], and ovary cancer [59].
Several limitations that should be acknowledged like (i) calculated crude Odds ratio, (ii) included the less number of available studies (10 studies) and the limited sample size of each included study, (iii) observed higher between study heterogeneity, (iv) considered only one gene polymorphism and (v) not considered other confounding factors like diet, gender etc. In addition to limitations, current meta-analysis has several strength also such as—higher study power and larger sample size in comparison to individual case control studies, and absence of publication bias etc.
In conclusion, pooled analysis of data from 11 separate articles indicates that the MTHFR 677TT genotype is not a risk factor for AD. The results of present meta-analysis should be interpreted with certain cautions due to presence of higher heterogeneity and small number of included studies. Future large-scale, population-based association studies from different regions of the world are required to investigate potential gene-gene and gene-environment interactions involving the MTHFR C677T polymorphism in determining AD risk.
[1] | Ayanwale, M.A., Efficacy of item response theory in the validation and score ranking of dichotomous and polytomous response mathematics achievement tests in Osun State, Nigeria. Nigeria. doi, 2019. |
[2] | National Council of Teachers of Mathematics, Principles to Actions: Ensuring Mathematical Success for All. Reston, VA: NCTM, 2020. |
[3] |
Ayanwale, M.A., Calibration of polytomous response mathematics achievement test using generalized partial credit model of item response theory. EDUCATUM Journal of Science, Mathematics and Technology, 2021, 8(1): 57‒69. https://doi.org/10.37134/ejsmt.vol8.1.7.2021 doi: 10.37134/ejsmt.vol8.1.7.2021
![]() |
[4] | Borko, H., Jacobs, J. and Koellner, K., Challenges and promises of using video to study and support teacher learning in mathematics. ZDM Mathematics Education, 2019, 51(5): 779‒792. |
[5] |
Akinboboye, J.T. and Ayanwale, M.A., Bloom taxonomy usage and psychometric analysis of classroom teacher made test. African Multidisciplinary Journal of Development, 2021, 10(1): 10‒21. https://doi.org/10.4038/kjms.v3i2.25 doi: 10.4038/kjms.v3i2.25
![]() |
[6] |
Peng, P. and Kievit, R.A., The development of academic achievement and cognitive abilities: A bidirectional perspective. Child Development Perspectives, 2021, 14(1): 15–20. https://doi.org/10.1111/cdep.12352 doi: 10.1111/cdep.12352
![]() |
[7] | Bergsten, C., Critical factors and prognostic validity in mathematics assessment. ICTM2, at Crete, 2002. |
[8] |
Brown, R.G., Does the introduction of the graphics calculator into systemwide examinations lead to change in the types of mathematical skills tested? Educational Studies in Mathematics, 2010, 73(2): 181‒203. https://doi.org/10.1007/s10649-009-9220-2 doi: 10.1007/s10649-009-9220-2
![]() |
[9] |
Tan, K., Assessment for learning in Singapore: unpacking its meanings and identifying some areas for improvement. Educational Research for Policy and Practice, 2011, 10(2): 91‒103. https://doi.org/10.1007/s10671-010-9096-z doi: 10.1007/s10671-010-9096-z
![]() |
[10] |
Li, Q. and Ma, X., A meta-analysis of the effects of computer technology on school students' mathematics learning. Educational Psychology Review, 2010, 22(3): 215–243. https://doi.org/10.1007/s10648-010-9125-8 doi: 10.1007/s10648-010-9125-8
![]() |
[11] | Hiebert, J. and Grouws, D.A., The Effects of Classroom Mathematics Teaching on Students' Learning. In F. Lester (Ed.), Second Handbook of Research on Mathematics Teaching and Learning, 2007,371‒404. Charlotte, NC: Information Age. |
[12] | National Council of Teachers of Mathematics, (Principles and standards for school mathematics. Reston, VA: National Council of Teachers of Mathematics, 2000. |
[13] | National Research Council, Adding it up: Helping children learn mathematics. J. Kilpatrick, J. Swafford, and B. Findell (Eds.). Mathematics Learning Study Committee, Center for Education, Division of Behavioral and Social Sciences and Education. Washington, DC: National Academy Press, 2001. Retrieved from: http://www.nap.edu/catalog/9822.html. |
[14] |
Gunawan, W. and Hadi, S., The effect of a Realistic Mathematics Education (RME) approach and reasoning ability on students' conceptual and procedural understanding. Contemporary Educational Researches Journal, 2024, 14(2): 90‒102. https://doi.org/10.18844/cerj.v14i2.9318 doi: 10.18844/cerj.v14i2.9318
![]() |
[15] |
Ding, M., Hassler, R. and Li, X., Cognitive instructional principles in elementary mathematics classrooms: A case of teaching inverse relations. International Journal of Mathematical Education in Science and Technology, 2021, 52(8): 1195–1224. https://doi.org/10.1080/0020739X.2020.1749319 doi: 10.1080/0020739X.2020.1749319
![]() |
[16] |
Layne, T., Yli-Piipari, S. and Knox, T., Physical activity break program to improve elementary students' executive function and mathematics performance. Education 3-13, 2021, 49(5): 583–591. https://doi.org/10.1080/03004279.2020.1746820 doi: 10.1080/03004279.2020.1746820
![]() |
[17] |
Anderson, K., Gong, X., Hong, K. and Zhang, X., The impacts of transition to middle school on student cognitive, non-cognitive and perceptual developments: Evidence from China. Education Economics, 2020, 28(4): 384–402. https://doi.org/10.1080/09645292.2020.1749234 doi: 10.1080/09645292.2020.1749234
![]() |
[18] |
Shen, Z., Tan, S. and Siau, K., Use of mental models and cognitive maps to understand students' learning challenges. Journal of Education for Business, 2019, 94(5): 281–289. https://doi.org/10.1080/08832323.2018.1527748 doi: 10.1080/08832323.2018.1527748
![]() |
[19] |
Trinidad, J.E., Understanding student-centred learning in higher education: Students' and teachers' perceptions, challenges, and cognitive gaps. Journal of Further and Higher Education, 2020, 44(8): 1013–1023. https://doi.org/10.1080/0309877X.2019.1636214 doi: 10.1080/0309877X.2019.1636214
![]() |
[20] | Makar, K., Ben-Zvi, D. and Bakker, A., (Eds.) International handbook of statistical reasoning, Springer, 2020. |
[21] |
Arigbabu, A.A. and Mji, A., Is gender a factor in mathematics performance among Nigerian pre-service teachers? Sex Roles, 2004, 51(11): 749–753. doi: 10.1007/s11199-004-0724-z. doi: 10.1007/s11199-004-0724-z
![]() |
Study | Ethnicity | Control | Case | Case Genotype |
Control Genotype |
HWE p-value of controls | ||||
CC | CT | TT | CC | CT | TT | |||||
Bonsch, 2006 | Caucasian | 115 | 134 | 64 | 56 | 14 | 60 | 41 | 14 | 0.10 |
Lutz, 2006 | Caucasian | 102 | 221 | 95 | 94 | 32 | 53 | 41 | 8 | 0.98 |
Lutz, 2007 | Caucasian | 101 | 142 | 65 | 58 | 19 | 53 | 40 | 8 | 0.90 |
Saffroy, 2008 | Caucasian | 93 | 242 | 108 | 113 | 21 | 35 | 41 | 17 | 0.41 |
Benyamina, 2009 | Caucasian | 93 | 120 | 56 | 53 | 11 | 35 | 41 | 17 | 0.41 |
Fabris, 2009 | Caucasian | 236 | 63 | 17 | 35 | 11 | 69 | 113 | 54 | 0.55 |
Shin, 2010 | Asian | 232 | 68 | 11 | 39 | 18 | 42 | 129 | 61 | 0.07 |
Supic, 2010, Heavy Alcoholic | Caucasian | 57 | 32 | 13 | 9 | 10 | 27 | 24 | 6 | 0.84 |
Supic, 2010, Non heavy Alcoholic | Caucasian | 105 | 64 | 37 | 23 | 4 | 53 | 42 | 10 | 0.69 |
Singh, 2014 | Asian | 313 | 139 | 91 | 44 | 4 | 228 | 78 | 7 | 0.91 |
Singh, 2015 | Asian | 147 | 451 | 312 | 125 | 14 | 107 | 35 | 5 | 0.32 |
Genetic Models | Fixed effect OR (95% CI), p | Random effect OR (95% CI), p | Heterogeneity p-value (Q test) | I2 (%) | Publication Bias (p of Egger's test) |
Allele Contrast (T vs. C) | 1.04 (0.92–1.17), 0.48 | 1.04 (0.88–1.24), 0.60 | 0.02 | 51.56 | 0.62 |
Dominant (TT+CT vs. CC) | 1.41 (1.20–1.65), <0.0001 | 1.02 (0.62–1.68), 0.92 | <0.0001 | 88.46 | 0.06 |
Homozygote (TT vs. CC) | 0.98 (0.75–1.29), 0.92 | 1.01 (0.66–1.51), 0.97 | 0.02 | 52.24 | 0.26 |
Co-dominant (CT vs. CC) | 1.10 (0.94–1.29), 0.21 | 1.10 (0.94–1.29), 0.21 | 0.43 | 0 | 0.48 |
Recessive (CC+CT vs. TT) | 0.94 (0.73–1.20), 0.63 | 0.97 (0.66–1.40), 0.86 | 0.02 | 52.4 | 0.28 |
Study | Ethnicity | Control | Case | Case Genotype |
Control Genotype |
HWE p-value of controls | ||||
CC | CT | TT | CC | CT | TT | |||||
Bonsch, 2006 | Caucasian | 115 | 134 | 64 | 56 | 14 | 60 | 41 | 14 | 0.10 |
Lutz, 2006 | Caucasian | 102 | 221 | 95 | 94 | 32 | 53 | 41 | 8 | 0.98 |
Lutz, 2007 | Caucasian | 101 | 142 | 65 | 58 | 19 | 53 | 40 | 8 | 0.90 |
Saffroy, 2008 | Caucasian | 93 | 242 | 108 | 113 | 21 | 35 | 41 | 17 | 0.41 |
Benyamina, 2009 | Caucasian | 93 | 120 | 56 | 53 | 11 | 35 | 41 | 17 | 0.41 |
Fabris, 2009 | Caucasian | 236 | 63 | 17 | 35 | 11 | 69 | 113 | 54 | 0.55 |
Shin, 2010 | Asian | 232 | 68 | 11 | 39 | 18 | 42 | 129 | 61 | 0.07 |
Supic, 2010, Heavy Alcoholic | Caucasian | 57 | 32 | 13 | 9 | 10 | 27 | 24 | 6 | 0.84 |
Supic, 2010, Non heavy Alcoholic | Caucasian | 105 | 64 | 37 | 23 | 4 | 53 | 42 | 10 | 0.69 |
Singh, 2014 | Asian | 313 | 139 | 91 | 44 | 4 | 228 | 78 | 7 | 0.91 |
Singh, 2015 | Asian | 147 | 451 | 312 | 125 | 14 | 107 | 35 | 5 | 0.32 |
Genetic Models | Fixed effect OR (95% CI), p | Random effect OR (95% CI), p | Heterogeneity p-value (Q test) | I2 (%) | Publication Bias (p of Egger's test) |
Allele Contrast (T vs. C) | 1.04 (0.92–1.17), 0.48 | 1.04 (0.88–1.24), 0.60 | 0.02 | 51.56 | 0.62 |
Dominant (TT+CT vs. CC) | 1.41 (1.20–1.65), <0.0001 | 1.02 (0.62–1.68), 0.92 | <0.0001 | 88.46 | 0.06 |
Homozygote (TT vs. CC) | 0.98 (0.75–1.29), 0.92 | 1.01 (0.66–1.51), 0.97 | 0.02 | 52.24 | 0.26 |
Co-dominant (CT vs. CC) | 1.10 (0.94–1.29), 0.21 | 1.10 (0.94–1.29), 0.21 | 0.43 | 0 | 0.48 |
Recessive (CC+CT vs. TT) | 0.94 (0.73–1.20), 0.63 | 0.97 (0.66–1.40), 0.86 | 0.02 | 52.4 | 0.28 |