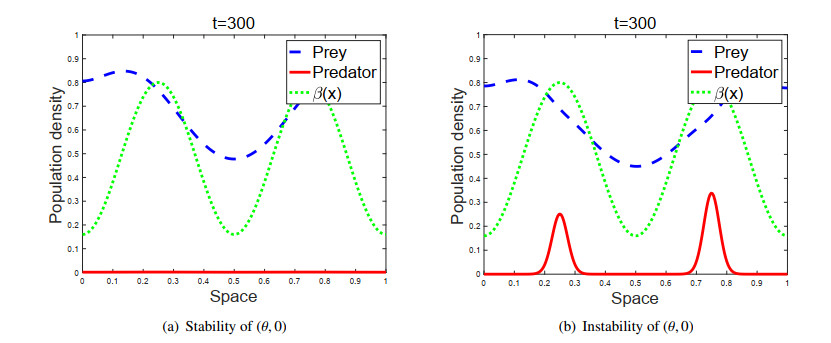
Integrating artificial intelligence (AI) into primary inclusive classrooms can significantly enhance teacher well-being by streamlining tasks, preventing burnout, fostering collaboration, and supporting diverse learning needs. However, for teachers to effectively use AI, they need to recognize its relevance, engage with its benefits, and promote its use to enhance their own well-being. The primary goal of this study is to examine the moderator effect of leadership support on the adoption of AI applications and tools and psychological well-being in primary inclusive classrooms in our hypothesized research model. To address this purpose, a quantitative methodology with structural equation modeling was utilized. Data was retrieved through an online questionnaire from 342 primary inclusive teachers, most being regular AI users. Our findings indicate that extensive use of AI tools is linked to engagement, relationships, and accomplishment but not to positive emotions or meaning. Surprisingly, school leadership negatively moderates factors outcomes, except for accomplishment, which remained effective. Leadership appeared less crucial in promoting AI adoption and enhancing teacher well-being. Leadership emerged as a less critical factor in promoting AI applications and tool adoption and teacher well-being. Professional programs for teachers should incorporate the impact of AI-based educational tools on psychological well-being, emphasizing the key role of leadership in AI adoption. Additionally, these programs should offer opportunities for sharing best practices to enhance the effective integration of AI in educational settings.
Citation: Samah Hatem Almaki, Nofouz Mafarja, Hamama Mubarak Saif Al Mansoori. Teacher well-being and use of artificial intelligence applications and tools: Moderation effects of leadership support in inclusive classroom[J]. STEM Education, 2025, 5(1): 109-129. doi: 10.3934/steme.2025006
[1] | Yuxuan Zhang, Xinmiao Rong, Jimin Zhang . A diffusive predator-prey system with prey refuge and predator cannibalism. Mathematical Biosciences and Engineering, 2019, 16(3): 1445-1470. doi: 10.3934/mbe.2019070 |
[2] | Yun Kang, Sourav Kumar Sasmal, Komi Messan . A two-patch prey-predator model with predator dispersal driven by the predation strength. Mathematical Biosciences and Engineering, 2017, 14(4): 843-880. doi: 10.3934/mbe.2017046 |
[3] | Yong Luo . Global existence and stability of the classical solution to a density-dependent prey-predator model with indirect prey-taxis. Mathematical Biosciences and Engineering, 2021, 18(5): 6672-6699. doi: 10.3934/mbe.2021331 |
[4] | Yongli Cai, Malay Banerjee, Yun Kang, Weiming Wang . Spatiotemporal complexity in a predator--prey model with weak Allee effects. Mathematical Biosciences and Engineering, 2014, 11(6): 1247-1274. doi: 10.3934/mbe.2014.11.1247 |
[5] | Eric M. Takyi, Charles Ohanian, Margaret Cathcart, Nihal Kumar . Dynamical analysis of a predator-prey system with prey vigilance and hunting cooperation in predators. Mathematical Biosciences and Engineering, 2024, 21(2): 2768-2786. doi: 10.3934/mbe.2024123 |
[6] | Jin Zhong, Yue Xia, Lijuan Chen, Fengde Chen . Dynamical analysis of a predator-prey system with fear-induced dispersal between patches. Mathematical Biosciences and Engineering, 2025, 22(5): 1159-1184. doi: 10.3934/mbe.2025042 |
[7] | Lazarus Kalvein Beay, Agus Suryanto, Isnani Darti, Trisilowati . Hopf bifurcation and stability analysis of the Rosenzweig-MacArthur predator-prey model with stage-structure in prey. Mathematical Biosciences and Engineering, 2020, 17(4): 4080-4097. doi: 10.3934/mbe.2020226 |
[8] | Yuanfu Shao . Bifurcations of a delayed predator-prey system with fear, refuge for prey and additional food for predator. Mathematical Biosciences and Engineering, 2023, 20(4): 7429-7452. doi: 10.3934/mbe.2023322 |
[9] | Swadesh Pal, Malay Banerjee, Vitaly Volpert . Spatio-temporal Bazykin’s model with space-time nonlocality. Mathematical Biosciences and Engineering, 2020, 17(5): 4801-4824. doi: 10.3934/mbe.2020262 |
[10] | Eric Ruggieri, Sebastian J. Schreiber . The Dynamics of the Schoener-Polis-Holt model of Intra-Guild Predation. Mathematical Biosciences and Engineering, 2005, 2(2): 279-288. doi: 10.3934/mbe.2005.2.279 |
Integrating artificial intelligence (AI) into primary inclusive classrooms can significantly enhance teacher well-being by streamlining tasks, preventing burnout, fostering collaboration, and supporting diverse learning needs. However, for teachers to effectively use AI, they need to recognize its relevance, engage with its benefits, and promote its use to enhance their own well-being. The primary goal of this study is to examine the moderator effect of leadership support on the adoption of AI applications and tools and psychological well-being in primary inclusive classrooms in our hypothesized research model. To address this purpose, a quantitative methodology with structural equation modeling was utilized. Data was retrieved through an online questionnaire from 342 primary inclusive teachers, most being regular AI users. Our findings indicate that extensive use of AI tools is linked to engagement, relationships, and accomplishment but not to positive emotions or meaning. Surprisingly, school leadership negatively moderates factors outcomes, except for accomplishment, which remained effective. Leadership appeared less crucial in promoting AI adoption and enhancing teacher well-being. Leadership emerged as a less critical factor in promoting AI applications and tool adoption and teacher well-being. Professional programs for teachers should incorporate the impact of AI-based educational tools on psychological well-being, emphasizing the key role of leadership in AI adoption. Additionally, these programs should offer opportunities for sharing best practices to enhance the effective integration of AI in educational settings.
For decades, many researchers have studied and established mathematical models describing interactions among species. Initially, most studies primarily focused on various types of interaction mechanisms and models using random dispersal of species. The importance of species dispersal, which is one of the main factors involved in species behavior, led to the establishment of more realistic models for species dispersal, for example, self-cross diffusion [1,2,3,4,5,6,7,8,9,10], advection-diffusion [11,12,13,14,15,16,17,18,19,20], and other types of non-uniform dispersal [21,22,23,24,25,26,27,28] models. For the predator–prey interaction, a popular way of describing the dispersal mechanism of predators is using prey-taxis [29,30,31,32,33,34]. There are some predator dispersal types such as the cross diffusion [2,3,4,5,6] and starvation-driven diffusion [22,25]. However, most models describing the dispersal mechanism of predators were dependent on prey's population density.
In this paper, we examine the predator–prey model describing a situation wherein the predator dispersal depends on other environmental factors rather than prey density. Here, we consider the following model with a directional dispersal of a predator in a spatially heterogeneous environment:
{ut=μΔu+u(m(x)−u)−α(x)uvu+d(x)vvt=∇⋅(η∇v−χv∇β)+v(−c+β(x)uu+d(x)v)inΩ×(0,∞),∂u∂→n=η∂v∂→n−χv∂β∂→n=0on∂Ω×(0,∞),u(x,0)=u0(x)≥0,v(x,0)=v0(x)≥0inΩ, | (P) |
where Ω is a bounded convex domain in Rn. The zero-flux condition is provided on the smooth boundary ∂Ω, where →n is the outward unit normal vector on the boundary. The functions u(x,t) and v(x,t) represent the population densities of the prey and predator, respectively. m(x) is the growth rate of the prey depending on the location x∈Ω, and μ and η are the diffusion rates of the prey and predator, respectively. α(x) is a positive function representing the capturing rate. β(x) is proportional to α(x), β=kα, where k>0 is a conversion efficiency of food into offspring. A positive function d(x) is a measure of the interference for the predators during hunting the prey. c and χ are positive constants representing the death rate of the predator and taxis sensitivity coefficient, respectively. The reaction term of the predator represents a case wherein the predator is fed only using the prey. To describe the predator–prey interaction, a ratio-dependent functional response is adopted. By considering the spatially heterogeneous environment, we use the predator-dependent form, such as the ratio-dependent form, for the system [35,36,37].
The model (P) represents a situation wherein the predator dispersal is unaffected by the prey density. The evolution of prey's behavior and traits has helped them against the threats of the predators. Consequently, these evolved traits and behaviors, for example, nocturnality [38,39], camouflage and masquerade [40,41], or living underground [42], make it difficult for the predators to chase the prey directly. This implies that the prey density does not affect the predator dispersal anymore. In response to defensive mechanisms of the prey, predators not only memorize the location where the prey was detected before but also learn the locations to hunt the prey in their habitat efficiently. Some studies have investigated the searching mechanism depending on such spatial memory [43,44,45,46]. In [43], the artificial experiments using birds was introduced, which provided some evidence of such animal learning to find better locations (see [43] and references therein). Thus, we assume that the prey has evolved to avoid detection from the predator, and the predator disperses using a spatial memory from experiences about the good locations to forage efficiently.
Such predator dispersal is mathematically described using the taxis term −∇⋅(χv∇β). An attractant function β(x) includes factors such as an encounter with prey and attack success rates. That is, β(x) indicates which area has high or low hunting efficiency for predators. Thus, the taxis term represents that the predator moves toward locations with high foraging efficiency using the spatial memory about β, which is a kind of mnemotaxis [44,45]. The reaction–diffusion system with the taxis term has a property that v is concentrated around the local maximums of β [15,16]. This implies that the predator gathers near the locations that have a high hunting efficiency. Thus, the predators' directional dispersal toward the location with better hunting efficiency can be described using such type of taxis term.
For the described dispersal mechanism of the predators, two situations can occur. The first situation wherein β is proportional to the resources of prey m (β=κm for some constant κ). This means that the predators move toward locations where the prey is abundant because the prey is distributed along with the distribution of resources. Mathematical and experimental works have been performed on a similar situation that the predators track the prey's resources instead of the prey density [47,48,49]. The second situation in which the distributions of β and m are different, i.e., β is not proportional to m. This situation can happen because some places are rich with prey but are easy to hide, making it difficult to hunt for predators, or because the predators memorized correctly, but the environment of prey's habitat has changed due to external factors. This situation seems to be disadvantageous to the predators. Thus, this paper aimed to show the effect of the directional movement of predators in both situations.
In our model, we let w=e−χβ(x)/ηv. Then, an alternative form of (P) is obtained as follows:
{ut=μΔu+u(m(x)−u)−α(x)uwu+d(x)weχβ(x)eχβ(x)/ηwt=ηΔw+χ∇β⋅∇w+w(−c+β(x)uu+d(x)weχβ(x)/η)inΩ×(0,∞),∂u∂→n=∂w∂→n=0on∂Ω×(0,∞),u(x,0)=u0(x)≥0,w(x,0)=w0(x)≥0inΩ, | (P') |
where w(x0)=e−χβ(x)/ηv0. Throughout this paper, we give assumptions on m, α and β:
m(x),α(x),β(x)andd(x)areinC2(ˉΩ),∫Ωm(x)dx>0 | (A) |
Under the assumption (A), the global existence and uniqueness of the classical solution to (P') follows from the Amann's results [50] for quasilinear parabolic equations. And (P') has one semi-trivial solution (θ,0), where θ is a unique positive steady state solution of
{μΔu+u(m(x)−u)=0inΩ,∂u∂→n=0on∂Ω. | (1.1) |
In this paper, we first investigate the local stability of the semi-trivial solution (θ,0), which is closely related to the survival of the predator. We also consider the elliptic system of (P'). We show that the elliptic system has a positive solution, called the coexistence steady-state, under the conditions for the instability of (θ,0). We also investigate the nonexistence of the positive solution.
The remaining part of this paper is organized as follows. In Section 2, we state the main theorems and give a biological explanation. The theorems address the stability of (θ,0) and the existence of a coexistence steady-state. Moreover, the effects of taxis sensitivity χ are observed. We provide the proofs of stability theorem using the principal eigenvalue analysis in Section 3. The existence of a coexistence steady-state is shown by the fixed-point index theory in Section 4. In Section 5, we present the numerical simulations for some cases. Section 6 summarizes the results obtained and concludes the paper.
First, we introduce the results for the stability of (θ,0). The instability of (θ,0) and the positivity of the prey density imply that the predator can invade the region. This implies that the predator can survive in the environment, even though this is rare. This allows us to examine whether the taxis is beneficial to the survival of the predator. As in [29], we can show the positivity of the prey density and lim inft→∞u(x,t)>0 if ∫Ωm(x)dx>∫Ωα(x)dx. Therefore, if we assume ∫Ωm(x)dx>∫Ωα(x)dx, the invasibility of the predator can be determined by the instability of (θ,0).
To study the stability of (θ,0), we consider the following linearized eigenvalue problem of (P') at (θ,0):
{μΔϕ+(m(x)−2θ)ϕ−α(x)eχβ(x)/ηψ=λϕinΩ,ηΔψ+χ∇β⋅∇ψ+(−c+β(x))ψ=λψinΩ,∂ϕ∂→n=∂ψ∂→n=0on∂Ω. | (2.1) |
We multiply eχβ/η by the second equation of Eq (2.1) and obtain
η∇⋅(eχβ/η∇ψ)+(−c+β(x))eχβ/ηψ=λeχβ/ηψ. | (2.2) |
Equation (2.2) is the weighted eigenvalue problem λeχβ/ηψ=Lψ, where Lψ=η∇⋅(eχβ/η∇ψ)+(−c+β(x))eχβ/ηψ. Since L is a self-adjoint, we have the following variational form of the principal eigenvalue:
λ1=supψ∈H1(Ω)∖{0},∫Ωeχβ/ηψ2=1∫Ω−ηeχβ/η|∇ψ|2+(−c+β(x))eχβ/ηψ2. |
We denote the principal eigenvalue of the operator L by λ1(L,χ). It is well-known that λ1(L,χ) and the local stability of (θ,0) have the following relation: If λ1(L,χ)>0, then (θ,0) is locally unstable; if λ1(L,χ)<0, then (θ,0) is locally stable (see [51]).
We define the ecological reproduction number R0 as
R0=supψ∈H1(Ω)∖{0}∫Ωβ(x)eχβ/ηψ2∫Ωηeχβ/η|∇ψ|2+ceχβ/ηψ2>0. | (2.3) |
Thus, we have the following lemma whose proof is analogous to the proof of Lemma 2, 3 in [52].
Lemma 2.1. R0>1 if and only if λ1(L,χ)>0.
Lemma 2.1 implies that (θ,0) is unstable if R0>1, and (θ,0) is locally asymptotically stable if R0<1. Then, R0 has a similar role as the basic reproduction number in epidemiology. This means that the survival of the predator is determined by the sign of R0−1.
Now, we introduce the results for the local stability of (θ,0) with an assumption on β, ∂β∂→n=0. For a function f, we denote the average of f in Ω by ¯f. We first present the stability result about the predator's death rate c when ∂β∂→n=0.
Theorem 2.2. Let η>0,χ≥0 be given. If ∂β∂→n=0, there exists ˜c∈(¯β−χ24η¯|∇β|2,maxˉΩβ) such that (θ,0) is unstable when c<˜c, and (θ,0) is locally asymptotically stable when c>˜c.
In Theorem 2.2, the sharp criteria for the local stability of (θ,0) about c is obtained, which represents invasibility of the predator. Given η>0 and χ≥0, there exists a threshold value ˜c such that λ1(L,χ)=0 for c=˜c and the sign of c−˜c determines the stability of (θ,0). From Theorem 2.2, (θ,0) is unstable for all η>0 when c<¯β and χ=0, but χ>0 gives a possibility (θ,0) to be stable if c∈(¯β−χ24η¯|∇β|,¯β). This means that the directional dispersal could be disadvantageous.
Next, we give the result for the local stability of (θ,0) about the death rate c and diffusion rate η of a predator.
Theorem 2.3. Suppose that ∂β∂→n=0. Let χ>0 and ξ>0 be given constants.
(i) If c>maxˉΩβ, then (θ,0) is locally asymptotically stable for all η>0.
(ii) If c<minˉΩβ, then (θ,0) is unstable for all η>0.
(iii) If c<¯β−14ξ¯|∇β|2, then (θ,0) is unstable when η>ξχ2.
(iv) Suppose that c∈(¯β−14ξ¯|∇β|2,maxˉΩβ). Then ˜η>0 exists such that if ˜η<ξχ2 then (θ,0) is locally asymptotically stable for η∈(˜η,ξχ2); and if ˜η>ξχ2 then (θ,0) is unstable for η∈(ξχ2,˜η).
Theorem 2.3 gives sufficient conditions of the stability of (θ,0) for the predators' diffusion rate η when c is in a proper region. A positively given ξ plays a role for obtaining the sufficient conditions for the stability. For example, we suppose that c and χ such that c<¯β−14ξ1¯|∇β|2 and c∈(¯β−14ξ2¯|∇β|2,maxˉΩβ) for some ξ1>ξ2. Then, we obtain from Theorem 2.3 that (θ,0) is unstable for η>ξ1χ2. Moreover, (θ,0) is unstable for η∈(ξ2χ2,˜η(ξ2)) if ~η(ξ2)>ξ2χ2, and (θ,0) is locally asymptotically stable for η∈(˜η,ξ2χ2) if ˜η<ξ2χ2.
Now, we investigate the effect of taxis χ for fixed c and η.
Theorem 2.4. Suppose that β has at least one isolated global maximum point and maxˉΩβ>c. For given η>0, there exists ˜χ>0 such that if χ≥˜χ then (θ,0) is unstable.
From Theorem 2.4, we can compare the stability of (θ,0) with or without the predator's directional movement. Without the taxis (χ=0), the predator cannot survive when they are rare for (c,η)∈(¯β,maxˉΩβ)×(η∗,∞) for some η∗, which will be immediately shown by lemma in Section 3. However, Theorem 2.4 shows that a large taxis χ>0 makes the predators invade a region. This implies that the strong taxis for predators gives a survival advantage to them compared with a random dispersal.
Next, we give the final result for the stability of (θ,0) when β(x)=κm(x) for x∈Ω. Consider the following equation Aχ2+Bχ+C=0, where
A=−κ24η∫Ω|∇m|2θ2dx,B=κ∫Ωθ∇θ⋅∇mdx,C=∫Ω−η|∇θ|2+(κm−c)θ2dx. | (2.4) |
If B is positive and B2−4AC>0, the equation has two real roots χ1<χ2 such that χ2 is positive.
Theorem 2.5. Suppose that m is positive, β=κm for some constant κ>0 and maxˉΩβ>c. If B2−4AC>0, (θ,0) is unstable when χ∈(max{χ1,0},χ2).
Remark that β(x)=κm(x) implies that the predator moves toward the favorable habitat of the prey. Because the prey has a property to be distributed according to m(x), such movement of the predator allows them to go to an environment where the prey population is sufficient. Moreover, as the assumption β(x)=κm(x) gives two effects, which are taxis toward the location with an abundance of prey and high hunting efficiency, the fitness of the predator increases. Therefore, a proper taxis sensitivity provides a survival advantage to the predator without the assumption on β in Theorem 2.4 for which predators cannot survive without taxis (Theorem 2.5).
Consider the steady-state of the system (P'):
{μΔu+u(m(x)−u)−α(x)uwu+d(x)weχβ(x)/ηeχβ(x)/η=0ηΔw+χ∇β⋅∇w+w(−c+β(x)uu+d(x)weχβ(x)/η)=0inΩ,∂u∂→n=∂w∂→n=0on∂Ω. | (2.5) |
In Eq (2.5), the ratio-dependent functional response is not defined at (u,w)=(0,0). Since
lim(u,w)→(0,0)uweχβ(x)/ηu+d(x)weχβ(x)/η=0, |
we can extend the domain of ud(x)weχβ(x)/ηu+d(x)weχβ(x)/η to {(u,w):u≥0,w≥0}, so that (0,0) becomes a trivial solution of Eq (2.5).
Let a positive solution of Eq (2.5) be a coexistence steady-state. Then, we have the following results for the existence of the coexistence steady-state of Eq (2.5), which is proven using the fixed-point index theory.
Theorem 2.6. Let η>0 and χ≥0 be given. If ∂β∂→n=0, there exists ˜c∈(¯β−χ24η¯|∇β|2,maxˉΩβ) such that Eq (2.5) has at least one coexistence steady state if and only if c<˜c.
Theorem 2.7. Suppose that ∂β∂→n=0. Let χ>0 and ξ>0 be given constants.
(i) If c<¯β−14ξ¯|∇β|2, then Eq (2.5) has at least one coexistence steady state when η>ξχ2.
(ii) If c<minˉΩβ, then Eq (2.5) has at least one coexistence steady state for all η>0.
(iii) Suppose that c∈(¯β−14ξ¯|∇β|2,maxˉΩβ). Then ˜η>0 exists such that if ˜η<ξχ2 then Eq (2.5) has at least one coexistence steady state for η∈(ξχ2,˜η).
Theorem 2.8. Suppose that β has at least one isolated global maximum point and maxˉΩβ>c. For given η>0, there exists ˜χ>0 such that if χ≥˜χ then Eq (2.5) has at least one coexistence steady state.
Theorem 2.9. Suppose that m is positive, β=κm for some constant κ>0 and maxˉΩβ>c. If B2−4AC>0 for given A,B and C as Eq (2.4), then Eq (2.5) has at least one coexistence steady state when χ∈(max{χ1,0},χ2).
It is noteworthy that the conditions for the existence of coexistence steady-state are closely related to the sign of λ1(L,χ), which will result in the proof of the above theorems. From the results in subsection 2.1, Eq (2.5) has more chance to have the coexistence steady-state when taxis sensitivity χ is properly chosen.
In this section, we prove Theorem 2.2–2.5. Recall that the principal eigenvalue of (2.2) is defined by
λ1(L,χ)=supψ∈H1(Ω)∖{0},∫Ωeχβ/ηψ2=1∫Ω−ηeχβ/η|∇ψ|2+(−c+β(x))eχβ/ηψ2. | (3.1) |
We assume that ∂β∂→n=0 and consider φ=eχβ/2ηψ. Since ψ∈H1(Ω) and β∈C2(ˉΩ), φ is also in H1(Ω). In addition, a mapping ψ↦eχβ/2ηψ is bijective. Thus, if we take ψ=e−χβ/2ηφ and take supremum over φ∈H1(Ω), we can rewrite the (3.1) as
λ1(L,χ)=supφ∈H1(Ω)∖{0},||φ||2=1∫Ω−η|∇φ|2+χφ∇φ⋅∇β+(−χ24η|∇β|2+β(x)−c)φ2. | (EV1) |
Then, by Green's identity and the assumption of β, we have
λ1(L,χ)=supφ∈H1(Ω)∖{0},||φ||2=1∫Ω−η|∇φ|2+(−χ2Δβ−χ24η|∇β|2+β(x)−c)φ2. | (EV2) |
It can be easily shown from (EV2) that λ1(L,χ) is the principal eigenvalue of
{ηΔφ+(−χ2Δβ−χ24η|∇β|2+β(x)−c)=λφinΩ,∂φ∂→n=0on∂Ω. |
Let ψ1 be the principal eigenfunction with respect to λ1(L,χ). From (EV2), we obtain
λ1(L,χ)=∫Ω−η|∇φ1|2+(−χ2Δβ−χ24η|∇β|2+(β(x)−c))φ21, |
where φ1=eχβ/2ηψ1. Since the principal eigenfunction is unique, φ1 is the principal eigenfunction with respect to λ1(ηΔ−χ2Δβ−χ24η|∇β|2+(β(x)−c)). Therefore, λ1(L,χ)=λ1(ηΔ−χ2Δβ−χ24η|∇β|2+(β(x)−c)). We will utilize the forms (EV1) and (EV2) in proving our results.
Before proving the theorems, we introduce some properties of the principal eigenvalue. The first property is well-known; the proof is omitted here (See [53]).
Lemma 3.1. Let λ1(ηΔ+g(x)) be the principal eigenvalue of
{ηΔψ+g(x)ψ=λψinΩ,∂ψ∂→n=0on∂Ω. | (3.2) |
Then,
(i) λ1(ηΔ+g(x)) is monotone decreasing function to η;
(ii) λ1(ηΔ+˜g(x))≥λ1(ηΔ+g(x)) for a function ˜g(x)≥g(x);
(iii) limη→0λ1(ηΔ+g(x))=maxx∈ˉΩg(x);
(iv) limη→∞λ1(ηΔ+g(x))=¯g.
Lemma 3.2. Let λ1(ηΔ+g(x)) be the principal eigenvalue of (3.2).
(i) If g(x)<0, then λ1(ηΔ+g(x))<0.
(ii) If ¯g>0, then λ1(ηΔ+g(x))>0.
(iii) If ∫Ωg(x)dx<0 and g(x) is positive somewhere, then there exists ˜η>0 such that λ1(ηΔ+g(x))≥0 if and only if η≤˜η. The equality holds when η=˜η.
Proof. (i) is obvious by the definition of the principal eigenvalue.
(ii) Suppose ∫Ωg(x)dx>0. From the definition of λ1(ηΔ+g(x)),
λ1(ηΔ+g(x))=supψ∈H1(Ω)∖{0},||ψ||2=1∫Ω−η|∇ψ|2+g(x)ψ2dx≥¯g>0. |
(iii) Let S:={x:g(x)>0}≠∅. Then, we can choose a test function ˜ψ with ||˜ψ||2=1 whose support is in S. Then, we obtain
λ1≥∫Ω−η|∇˜ψ|2+g(x)˜ψ2dx=∫S−η|∇˜ψ|2+g(x)˜ψ2dx>0 |
for sufficiently small η. Since λ1(ηΔ+g(x)) is monotone decreasing function to η and limη→∞λ1(ηΔ+g(x))=¯g<0, there exists ˜η such that if η>˜η, then λ1(ηΔ+g(x))<0, and if η<˜η, then λ1(ηΔ+g(x))>0, which is a desired result.
Now, we first give the proof Theorems 2.2 and 2.3.
Proof of Theorem 2.2. From the definition of the principal eigenvalue (3.1), we have
λ1(L,χ)=supψ∈H1(Ω)∖{0},∫Ωeχβ/ηψ2=1∫Ω−ηeχβ/η|∇ψ|2+(−c+β(x))eχβ/ηψ2<0 |
for c>maxˉΩβ.
Next, from (EV2) and ∂β∂→n=0, we obtain
λ1(L,χ)=λ1(ηΔ−χ2Δβ−χ24η|∇β|2+(β(x)−c))=∫Ω−η|∇φ1|2+(−χ2Δβ−χ24η|∇β|2+(β(x)−c))φ21, |
where φ1 is the principal eigenfunction with respect to λ1(ηΔ−χ2Δβ−χ24η|∇β|2+(β(x)−c)). Also, by the Green's first identity, we have
∫ΩΔβ(x)dx=∫∂Ω∂β∂→ndS=0. | (3.3) |
It follows from Eq (3.3) and Lemma 3.2 that
λ1(ηΔ−χ2Δβ−χ24η|∇β|2+(β(x)−c))>0forc<¯β−χ24η¯|∇β|2. |
Also, since Eq (3.1) and (EV2) are equivalent form of the principal eigenvalue,
λ1(ηΔ−χ2Δβ−χ24η|∇β|2+(β(x)−c))<0forc>maxˉΩβ. |
By Lemma 3.1 (ii), λ1(ηΔ−χ2Δβ−χ24η|∇β|2+(β(x)−c)) is monotone decreasing function to c. Then, we can find ˜c∈(¯β−χ24η¯|∇β|2,maxˉΩβ) such that λ1(ηΔ−χ2Δβ−χ24η|∇β|2+(β(x)−c))=0. By the monotonicity of λ1(L,χ) to c, (θ,0) is unstable when c<˜c, and (θ,0) is locally asymptotically stable when c>˜c.
Proof of Theorem 2.3. As in the proof of Theorem 2.2, it suffices to check the sign of λ1(L,χ). From the form Eq (3.1), (i) and (ii) are immediately obtained.
For (iii), we denote λ1(ηΔ−χ2Δβ−χ24η|∇β|2+(β(x)−c)) by ˜λ. Consider an eigenvalue problem as follows:
{ηΔφ+(−χ2Δβ−14ξ|∇β|2+(β(x)−c))φ=λφinΩ,∂φ∂→n=0on∂Ω. | (3.4) |
Then, the principal eigenvalue of Eq (3.4) can be written by
λ1(ηΔ−χ2Δβ−14ξ|∇β|2+(β(x)−c))=supφ∈H1(Ω)∖{0},||φ||2=1∫Ω−η|∇φ|2+(−χ2Δβ−14ξ|∇β|2+(β(x)−c))φ2, |
From Lemma 3.1 (ii),
˜λ>λ1(ηΔ−χ2Δβ−14ξ|∇β|2+(β(x)−c))if and only if η>ξχ2. | (3.5) |
Then, the results (iii) follows from Lemma 3.2.
(iv) Assume that c∈(¯β−14ξ¯|∇β|2,maxˉΩβ). By Lemma 3.2 (iii), there exists ˜η>0 depending on ξ and χ such that λ1(ηΔ−χ2Δβ−14ξ|∇β|2+(β(x)−c))<0 if and only if η>˜η. For ˜η<ξχ2, (˜η,ξχ2) is a non-empty interval. Then, it follows form (3.5) that ˜λ<0 when η∈(˜η,ξχ2), and thus (θ,0) is locally asymptotically stable. Similarly, for ˜η>ξχ2, (θ,0) is unstable when η∈(ξχ2,˜η).
Next, we prove Theorem 2.4.
Proof of Theorem 2.4. It can be proved similarly to Theorem 4.4 in [15]. For reader's convenience, we give the proof here. Since the principal eigenvalue of (2.2) is
λ1(L,χ)=supψ∈H1(Ω)∖{0},∫Ωeχβ/ηψ2=1∫Ω−ηeχβ/η|∇ψ|2+(−c+β(x))eχβ/ηψ2, |
we only need to find a function Ψ with ∫Ωeχβ/ηΨ2=1 such that
∫Ωηeχβ/η|∇Ψ|2<∫Ω(−c+β(x))eχβ/ηΨ2. | (3.6) |
From the assumption, a point x0∈ˉΩ exists satisfying β(x0)=maxˉΩβ and β(x0)−c≥δ for some δ>0. Let R1>0 be small such that β(x)−c≥12δ in BR1(x0)∩Ω, where BR1(x0) is a ball of radius R1 and centered at x0. We define β1=max(BR1(x0)∖BR1/2(x0))∩Ωβ and β2=minBR2(x0)∩Ωβ for R1,R2>0. Since the global maximum point is isolated,
β2≥12[β1+β(x0)]>β1 |
for sufficiently small R2 satisfying R2≤R1/2.
Define a function Φ∈C1(ˉΩ) as
Φ={1in BR1/2(x0)∩Ω,∈[0,1]in (BR1(x0)∖BR2(x0))∩Ω,0otherwise. |
If we put Ψ:=Φ/(∫Ωeχβ/ηΦ2)1/2 in Eq (3.6), the left-hand side becomes
∫Ωηeχβ/η|∇Ψ|2=η∫(BR1(x0)∖BR2(x0))∩Ωeχβ/η|∇Ψ|2≤C1∫(BR1(x0)∖BR2(x0))∩Ωeχβ/η≤C1|Ω|e(χ/η)β1, |
where C1 satisfies ||∇Ψ||∞≤C1. The right-hand side of Eq (3.6) is
∫Ω(−c+β(x))eχβ/ηΨ2=∫BR1(x0)∩Ω(−c+β(x))eχβ/ηΨ2≥e(χ/η)β2∫BR2(x0)∩Ω(−c+β(x))≥C2e(χ/η)β2 |
for some positive constant C2. Since C1 and C2 is independent of χ, Eq (3.6) holds if we choose χ large enough. Hence, we have a desired result.
Finally, we assume that β(x)=κm(x) for x∈Ω, which is the assumption in Theorem 2.5. Before proving the theorem, we give a known result in [14].
Lemma 3.3 (Cantrell et al. [14]). The integral ∫Ωθ∇θ⋅∇mdx is positive if Ω⊆R is an interval or if Ω⊆Rn is convex.
Then, we give the proof of Theorem 2.5.
Proof of Theorem 2.5. Let λ1(L,χ) be the principal eigenvalue with the form (EV1). Since β(x)=κm(x) for x∈Ω,
λ1(L,χ)=supφ∈H1(Ω)∖{0},||φ||2=1∫Ω−η|∇φ|2+κχφ∇φ⋅∇m+(−κ2χ24η|∇m|2+κm−c)φ2≥∫Ω−η|∇θ|2+κχθ∇θ⋅∇m+(−κ2χ24η|∇m|2+κm−c)θ2/∫Ωθ2dx=(Aχ2+Bχ+C)/∫Ωθ2dx, |
where A,B and C are given as Equation (2.4). Since B2−4AC>0, A<0 and B>0 by Lemma 3.3, the equation has two real roots χ1 and χ2 with at least one positive solution, say χ2. Then, Aχ2+Bχ+C>0 for χ1<χ<χ2. which implies (θ,0) is unstable for χ∈(max{χ1,0},χ2). We note that B2−4AC cannot be positive for c>maxˉΩβ, because (θ,0) is locally asymptotically stable (λ1<0) when c>maxˉΩβ. Hence, Theorem 2.5 is valid for c<maxˉΩβ.
In this section, we present the proofs of Theorems 2.6–2.8 which are about the existence and non-existence of the coexistence steady-states of Eq (2.5). Before proving the theorems, we introduce the fixed-point index theory.
Fixed-point index theory
Let E be a real Banach space and W⊂E a closed convex set. W is called a total wedge if αW⊂W for all α≥0 and ¯W∪(−W)=E. A wedge is said to be a cone if W∩(−W)={0}. For y∈W, we define Wy={x∈E:y+γx∈Wfor someγ>0} and Sy={x∈¯Wy:−x∈¯Wy}. Then, ¯Wy is a wedge containing W, y and −y, while Sy is a closed subspace of E containing y.
Let T be a compact linear operator on E satisfying T(¯Wy)⊂¯Wy. We say that T has property α on ¯Wy if there are t∈(0,1) and w∈¯Wy∖Sy such that w−tTw∈Sy. Let F:W→W is a compact operator with a fixed-point y∈W and F is Fréchet differentiable at y. Let L=F′(y) be the Fréchet derivative of F at y. Then, L maps ¯Wy into itself. For an open subset U⊂W, define indexW(F,U)=index(F,U,W)=degW(I−F,U,0), where I is the identity map. If y is an isolated fixed-point of F, then the fixed-point index of F at y in W is defined by indexW(F,y)=index(F,y,W)=index(F,U(y),W), where U(y) is a small open neighborhood of y in W.
The following theorem can be obtained from the results of [54,55,56].
Theorem 4.1. Assume that I−L is invertible on ¯Wy.
(i) If L has property α on ¯Wy, then indexW(F,y)=0.
(ii) If L does not have property α on ¯Wy, then indexW(F,y)=(−1)σ, where σ is the sum of multiplicities of all the eigenvalues of L which are greater than 1.
Lemma 4.2. Any coexistence state (u,w) of system (2.5) has an a priori estimate
u(x)≤Q1,w(x)≤Q2. |
where Q1=maxˉΩm,Q2=Q1maxˉΩβcminˉΩd.
Proof. Let (u,w) be a positive solution of (2.5). Since −μΔu≤u(m(x)−u), we obtain u≤maxˉΩm by maximum principle for elliptic.
Let w(x0)=maxˉΩw and h=χ/η. Then, by maximum principle for elliptic equation,
0≤β(x0)u(x0)u(x0)+d(x0)ehβ(x0)w(x0)−c≤maxˉΩβQ1Q1+minˉΩdw(x0)−c. |
Since
cminˉΩdw(x0)≤cQ1+cminˉΩdw(x0)≤maxˉΩβQ1, |
it implies that
maxˉΩw≤maxˉΩβcminˉΩdQ1. |
Notation 4.3.
(i) X:=C1N(ˉΩ)⨁C1N(ˉΩ) where C1N(ˉΩ):={ϕ∈C1(ˉΩ):∂ϕ∂→n=0on∂Ω}.
(ii) Q:=max{Q1,Q2}+1.
(iii) D:=DQ⨁DQ where DQ={ϕ∈C1N(ˉΩ):ϕ<QonˉΩ}.
(iv) W:=D0⨁D0 where D0={ϕ∈C1N(ˉΩ):0≤ϕonˉΩ}.
(v) D′:=D∩W.
For τ∈[0,1], define a positive compact operator Fτ:X→X by Fτ(u,w)=(−DΔ+P)−1[PI+τG](u,w)T where
G(u,w)T=(mu−u2−αueχβ/ηwu+d(x)eχβ/ηwχ∇β⋅∇w+βueχβ/ηwu+d(x)eχβ/ηw−cw),D=(μ00η). |
and P is a positive constant such that (2Q+maxΩαminΩd+maxΩβminΩd+c)Q+χ‖∇β‖∞‖∇w‖∞<P. Fτ is positive and compact operator. We denote F=F1. Then, (P') has a positive solution if and only if F has a positive fixed-point.
For the operator F, we first find the result for the index values, indexW(F,D′) and indexW(F,(0,0)). The following lemmas can be proved by similar argument to [57]; the proofs are omitted here.
Lemma 4.4. indexW(F,D′)=1.
Lemma 4.5. indexW(F,(0,0))=0.
Next, we calculate the indexW(F,(θ,0)). Remark that the principal eigenvalue λ1(L,χ) of Eq (2.2) is real, and the corresponding principal eigenfunction Ψ is positive. Since the principal eigenpair (λ1(L,χ),Ψ) satisfies Eq (2.2), (λ1(L,χ),Ψ) also satisfies the second equation of Eq (2.1):
λ1(L,χ)Ψ=ηΔΨ+χ∇β⋅∇Ψ+(−c+β(x))ΨinΩ. | (4.1) |
Lemma 4.6. If λ1(L,χ)>0, then indexW(F,(θ,0))=0.
Proof. By calculation, we have
¯W(θ,0)=C1N(¯Ω)⨁D0,S(θ,0)=C1N(¯Ω)⨁{0}. |
Define
L:=F′(θ,0)=(−DΔ+P)−1(m−2θ+P−α0χ∇β⋅∇+β−c+P). |
We first claim that I−L is invertible. Let L(ϕ,ψ)T=(ϕ,ψ)T∈¯W(θ,0).
{−μΔϕ=(m−2θ)ϕ−αψ−ηΔψ=χ∇β⋅∇ψ+(β−c)ψinΩ,∂ϕ∂→n=∂ψ∂→n=0on∂Ω. | (4.2) |
For ψ≥0, multiply the second equation of Eq (4.2) by eχβ/ηΨ and integrate over Ω, where Ψ is a principal eigenfunction of λ1(L,χ). Then, we obtain
0=∫Ωeχβ/ηΨ[ηΔψ+χ∇β⋅∇ψ]+eχβ/η(β−c)Ψψ=∫ΩΨ∇⋅[ηeχβ/η∇ψ]+eχβ/η(β−c)Ψψ=∫Ωψ∇⋅[ηeχβ/η∇Ψ]+eχβ/η(β−c)Ψψ=∫Ωeχβ/ηψ[ηΔΨ+χ∇β⋅∇Ψ+(β−c)Ψ]=λ1(L,χ)∫Ωeχβ/ηΨψ≥0, |
which implies ψ≡0. The last equality holds from Eq (4.1).
Since λ1(μΔ+(m−θ))=0 with the corresponding principal eigenfunction θ, λ1(μΔ+(m−2θ))<0 by Lemma 3.1 (ii). We multiply the first equation of Eq (4.2) by ϕ and integrate over Ω. Then, we have
0=∫Ωαϕψ=∫Ω−μ|∇ϕ|2+(m−2θ)ϕ2≤λ1(μΔ+(m−2θ))∫Ωϕ2≤0. |
Hence, ϕ≡0 and I−L is invertible.
Next, we show that L has a property α. Let r be the spectral radius of(−ηΔ+P)−1(χ∇β⋅∇+β−c+P). Then, the assumption λ1(L,χ)>0 implies that r>1. Then, there exist corresponding eigenfunction ξ exists in D0∖{0}. Take t=1/r∈(0,1), then
(ϕψ)−tL(ϕψ)=(00)∈S(θ,0)holdsfor(ϕψ)=(0ξ)∈¯W(θ,0)∖S(θ,0). |
Hence, L has property α. By Theorem 4.1 (i), we have indexW(F,(θ,0))=0.
Now, we give the proofs of Theorems 2.6–2.9. Theorems 2.7–2.9 can be proved in a similarly manner; we only give the proof of Theorem 2.6.
Proof of Theorem 2.6. Suppose that ∂β∂→n=0. From Theorem 2.2, there exists ˜c∈(¯β−χ24η¯|∇β|2,maxˉΩβ). Then, it follows from Lemma 4.4–4.6 that
1=indexW(F,D′)≠indexW(F,(0,0))+indexW(F,(θ,0))=0. |
Hence, Eq (2.5) has at least one coexistence state for c<˜c.
Next, we assume that c≥˜c. Suppose that (u,w) be the positive solution of Eq (2.5). Then, (u,w) satisfies
ηΔw+χ∇β⋅∇w+w(−c+β(x)uu+d(x)weχβ(x)/η)=0. | (4.3) |
Let ˜w:=w/(∫Ωeχβ/ηw2)1/2. If we multiply Eq (4.3) by eχβ/η˜w/(∫Ωeχβ/ηw2)1/2 and integrate over Ω, we have
0=∫Ω−ηeχβ/η|∇˜w|2+(β(x)uu+d(x)weχβ/η−c)eχβ/η˜w2=∫Ω−ηeχβ/η|∇˜w|2+(β(x)−c)eχβ/η˜w2+(β(x)uu+d(x)weχβ/η−β(x))eχβ/η˜w2≤λ1(L,χ)+∫Ω(β(x)uu+d(x)weχβ/η−β(x))eχβ/η˜w2. | (4.4) |
The last inequality holds by the definition of the principal eigenvalue λ1(L,χ). From Eq (4.4), we obtain
λ1(L,χ)≥∫Ω(β(x)−β(x)uu+d(x)weχβ/η)eχβ/η˜w2>0, |
which is a contradiction because λ1(L,χ)≤0 under the assumption c≥˜c. Hence, there is no positive solution of Eq (2.5) if c≥˜c.
In this section, we present some numerical simulations to verify the mathematical results of this paper. Throughout the simulations, the following parameters and functions are used:
β(x)=0.8α(x),d(x)=0.5+0.1sin(2πx),μ=0.01,u0(x)=0.5+0.2sin(2πx),v0(x)=0.2+0.1sin(2πx),Ω=[0,1]. |
We used the upwind scheme with the finite difference method, and the toleration of iteration is selected as 10−6. The simulation results presented show the important role of directional dispersal with taxis compared to the random dispersal when c,β and η are given.
Figure 1 shows that a large χ gives the predator with directional dispersal a survival advantage when c∈(¯β,maxˉΩβ). Theorem 2.4 implies that the (θ,0) is locally asymptotically stable when χ=0 for any given c and η, but a large χ allows (θ,0) to be unstable. This means that the strong taxis of predator can make them survive under the condition in which the predator with random dispersal cannot survive. Figure 1 shows that such dispersal makes the predator and prey coexist in the environment. It is noteworthy that, as can be seen in Figure 1, the density of the predator is concentrated on the local maximum of β, which is a feature of the species with directional dispersal.
In Figure 2, the case that β(x)=κm(x) is considered. If β(x) has at least one isolated global maximum point, we have a same result in Theorem 2.4 and Figure 1. Thus, we give β(x) as a combination of smoothstep function for Figure 2, which is the case when β has no isolated global maximum point on ¯Ω. Figure 2 shows that the properly chosen taxis sensitivity χ>0 can make the predator survive under the condition when the predator without taxis cannot survive. This represents the result of Theorem 2.5 that the directional dispersal is beneficial for the predator's survival when χ is in a proper region regardless of the assumption on β. This is a natural ecological result because the taxis of the predators not only makes them move towards the region with high hunting efficiency, but also the prey's favorable habitats where the density of the prey is large.
In this paper, we suggested the predator–prey model describing the phenomena that the predator moves with taxis toward the region with a high hunting efficiency. For the predator–prey model, most of the studies dealing with taxis have considered the prey-taxis [29,30,31,32,33,34]. Authors in [29] adopted the ratio-dependent functional responses with a spatially heterogeneous environment, which are the same as our model, and they showed that the prey taxis is always beneficial for the predator's survival. However, the predators cannot chase the prey directly because the model we established describes the situation that prey has evolved to avoid detection from the predator. Thus, we considered other type of predators' dispersal mechanism with taxis toward the location where the hunting efficiency is high using the spatial memory [43,44,45,46]. There are two cases of the rate at which predators capture the prey: proportional (β=κm) or not proportional (β≠κm) to the resources of prey. For the first case, a similar idea is introduced in [47,48,49] that the predators move in the direction of high prey's resources instead of tracking the prey directly, and [47] established the mathematical model of such dispersal and obtained some mathematical results. The other case can be followed from the prey's defensive mechanisms or environmental change from other external factors. This could make the predators go to a region with low prey density, which seems not helpful for the predators. In this paper, we investigated whether the defensive mechanism of the predators is advantageous to them for both cases.
Through this article, we determined the criteria and sufficient conditions for the local stability of the semi-trivial solution, which is closely related to the survival of the predators. Furthermore, the conditions for the existence of coexistence steady-state were obtained. We studied the stability and coexistence conditions for the three parameters: the death rate, diffusion, and taxis sensitivity. Finally, the obtained results were verified by using numerical simulations. From a biological perspective, the results for stability indicate that, when the predators have the spatial memory where the location is good to forage efficiently, the taxis toward the location could give a greater chance of invasion than when they disperse randomly (Theorems 2.2, 2.4 and 2.5). This implies that the directional movement toward the region with high foraging efficiency increases the fitness of predators. Consequently, such dispersal helps the predators to invade a region. If β has at least one isolated global maximum point, the dispersal is advantageous to the predator's survival when taxis is strong even though β is not proportional to m (Theorem 2.4). This represents that the dispersal mechanism also affects to the predators fitness regardless the distributions of β and m. When β has no isolated global maximum, the proper taxis sensitivity gives survival advantage to the predators (Theorem 2.5). This means that the properly chosen taxis sensitivity helps predators survive regardless of assumption on β when the predators have directional movement toward the location with abundant prey and high hunting efficiency (β is proportional to m). Moreover, as a dictum in ecology that "invasibility implies coexistence, " the taxis influences on the coexistence of predator and prey. This dispersal of predators could give them a greater possibility to coexist in the ecosystem than random dispersal (Theorems 2.6, 2.8 and 2.9). Thus, we conclude that the dispersal considered in this work plays a vital role in the survival of the predator and the coexistence in the predator–prey system.
This research was supported by Basic Science Research Program through the National Research Foundation of Korea (NRF) funded by the Ministry of Science, ICT & Future Planning (NRF-2015R1A2A2A01007013; NRF-2018R1C1B5039938).
All authors declare no conflicts of interest in this paper.
[1] | Navarro-Espinosa, J.A., Vaquero-Abellán, M., Perea-Moreno, A.J., Pedrós-Pérez, G., Aparicio-Martínez, P. and Martínez-Jiménez, M.P., The influence of technology on mental well-being of stem teachers at university level: Covid-19 as a stressor. International journal of environmental research and public health, 2021, 18(18): 9605. |
[2] | Thomas, L., Developing inclusive learning to improve the engagement, belonging, retention, and success of students from diverse groups. In Widening higher education participation, 2016,135‒159. Chandos Publishing. |
[3] | Meidelina, O., Saleh, A.Y., Cathlin, C.A. and Winesa, S.A., Transformational Leadership and Teacher Well-Being: A Systematic Review. Journal of Education and Learning (EduLearn), 2023, 17(3): 417‒424. |
[4] | Khansaheb, K.S.H.A., The Role of Artificial Intelligence in Enhancing Sustainability: The Case of UAE Smart Cities. BUiD Doctoral Research Conference 2023: Multidisciplinary Studies, 2024,235–242. https://doi.org/10.1007/978-3-031-56121-4_23 |
[5] | Halder Adhya, D., Al Bastaki, E.M., Suleymanova, S., Muhammad, N. and Purushothaman, A., Utilizing open educational practices to support sustainable higher education in the United Arab Emirates. Asian Association of Open Universities Journal, 2024. https://doi.org/10.1108/AAOUJ-07-2023-0086 |
[6] | Suresh, A., Jacobs, J., Clevenger, C., Lai, V., Tan, C., Martin, J.H., et al., Using AI to Promote Equitable Classroom Discussions: The TalkMoves Application. International conference on artificial intelligence in education, 2021,344–348. Cham: Springer International Publishing https://doi.org/10.1007/978-3-030-78270-2_61 |
[7] |
Yao, Y., Wang, P., Jiang, Y., Li, Q. and Li, Y., Innovative online learning strategies for the successful construction of student self-awareness during the COVID-19 pandemic: Merging TAM with TPB. Journal of Innovation & Knowledge, 2022, 7(4): 100252. https://doi.org/10.1016/j.jik.2022.100252 doi: 10.1016/j.jik.2022.100252
![]() |
[8] | Huo, L. and Sun, F., Application of Artificial Intelligence in Mental Health Education in Primary and Middle Schools. Proceedings of the 2nd Conference on Artificial Intelligence and Healthcare, 2021,306–312. https://doi.org/10.5220/0011368000003444 |
[9] | Luckin, R. and Holmes, W., Intelligence unleashed: An argument for ai in education, Pearson Education. 2016. |
[10] |
Chua, K.H. and Bong, W.K., Providing inclusive education through virtual classrooms: a study of the experiences of secondary science teachers in Malaysia during the pandemic. International Journal of Inclusive Education, 2024, 28(9): 1886–1903. https://doi.org/10.1080/13603116.2022.2042403 doi: 10.1080/13603116.2022.2042403
![]() |
[11] |
Shamsuddinova, S., Heryani, P. and Naval, M.A., Evolution to revolution: Critical exploration of educators' perceptions of the impact of Artificial Intelligence (AI) on the teaching and learning process in the GCC region. International Journal of Educational Research, 2024,125: 102326. https://doi.org/10.1016/j.ijer.2024.102326 doi: 10.1016/j.ijer.2024.102326
![]() |
[12] |
Javed, A.R., Shahzad, F., ur Rehman, S., Zikria, Y.B., Razzak, I., Jalil, Z., et al., Future smart cities: requirements, emerging technologies, applications, challenges, and future aspects. Cities, 2022,129: 103794. https://doi.org/10.1016/j.cities.2022.103794 doi: 10.1016/j.cities.2022.103794
![]() |
[13] |
Jerrim, J. and Sims, S., When is high workload bad for teacher wellbeing? Accounting for the non-linear contribution of specific teaching tasks. Teaching and Teacher Education, 2021,105: 103395. https://doi.org/10.1016/j.tate.2021.103395 doi: 10.1016/j.tate.2021.103395
![]() |
[14] |
Karkouti, I.M., Abu-Shawish, R.K. and Romanowski, M.H., Teachers' understandings of the social and professional support needed to implement change in Qatar. Heliyon, 2022, 8(1): e08818. https://doi.org/10.1016/j.heliyon.2022.e08818 doi: 10.1016/j.heliyon.2022.e08818
![]() |
[15] | Flamholtz, E. and Randle, Y., Leading Strategic Change, Cambridge University Press, 2008. https://doi.org/10.1017/CBO9780511488528 |
[16] | Skaalvik, E.M. and Skaalvik, S., Teacher Stress and Teacher Self-Efficacy: Relations and Consequences. In T. M. McIntyre, S. E. McIntyre, and D. J. Francis (Eds.), Educator Stress: An Occupational Health Perspective, 2017,101‒125. https://doi.org/10.1007/978-3-319-53053-6_5 |
[17] |
Akiba, M., Byun, S., Jiang, X., Kim, K. and Moran, A.J., Do Teachers Feel Valued in Society? Occupational Value of the Teaching Profession in OECD Countries. AERA Open, 2023, 9: 23328584231179184. https://doi.org/10.1177/23328584231179184 doi: 10.1177/23328584231179184
![]() |
[18] | Hui, Z., Khan, N.A. and Akhtar, M., AI-based virtual assistant and transformational leadership in social cognitive theory perspective: a study of team innovation in construction industry. International Journal of Managing Projects in Business, 2024, 1‒20. |
[19] |
Toropova, A., Myrberg, E. and Johansson, S., Teacher job satisfaction: the importance of school working conditions and teacher characteristics. Educational Review, 2021, 73(1): 71–97. https://doi.org/10.1080/00131911.2019.1705247 doi: 10.1080/00131911.2019.1705247
![]() |
[20] |
Heintzelman, S.J., Kushlev, K. and Diener, E, personalizing a positive psychology intervention improves well-being. Applied Psychology: Health and Well-Being, 2023, 15(4): 1271‒1292. https://doi.org/10.1111/aphw.12436 doi: 10.1111/aphw.12436
![]() |
[21] |
Guo, W., Li, W. and Tisdell, C.C., Effective pedagogy of guiding undergraduate engineering students solving first-order ordinary differential equations. Mathematics, 2021, 9(14): 1623. https://doi.org/10.3390/math9141623 doi: 10.3390/math9141623
![]() |
[22] |
Sun, R., Teulings, I. and Sauter, D., Why Being Social and Active Boosts Psychological Wellbeing: A Mediating Role of Momentary Positive Emotions. Social Psychological and Personality Science. 2023. https://doi.org/10.1177/19485506231218362 doi: 10.1177/19485506231218362
![]() |
[23] |
Xie, F. and Derakhshan, A., A Conceptual Review of Positive Teacher Interpersonal Communication Behaviors in the Instructional Context. Frontiers in Psychology, 2021, 12: 708490. https://doi.org/10.3389/fpsyg.2021.708490 doi: 10.3389/fpsyg.2021.708490
![]() |
[24] |
Billingsley, B., DeMatthews, D., Connally, K. and McLeskey, J., Leadership for Effective Inclusive Schools: Considerations for Preparation and Reform. Australasian Journal of Special and Inclusive Education, 2018, 42(01): 65–81. https://doi.org/10.1017/jsi.2018.6 doi: 10.1017/jsi.2018.6
![]() |
[25] |
Cann, R.F., Riedel-Prabhakar, R. and Powell, D., A Model of Positive School Leadership to Improve Teacher Wellbeing. International Journal of Applied Positive Psychology, 2021, 6(2): 195–218. https://doi.org/10.1007/s41042-020-00045-5 doi: 10.1007/s41042-020-00045-5
![]() |
[26] |
Du Plessis, A. and McDonagh, K., The out-of-field phenomenon and leadership for wellbeing: Understanding concerns for teachers, students and education partnerships. International Journal of Educational Research, 2021,106: 101724. https://doi.org/10.1016/j.ijer.2020.101724 doi: 10.1016/j.ijer.2020.101724
![]() |
[27] |
Nasir, M., Hasan, M., Adlim, A. and Syukri, M., Utilizing artificial intelligence in education to enhance teaching effectiveness. Proceedings of International Conference on Education, 2024, 2(1): 280–285. https://doi.org/10.32672/pice.v2i1.1367 doi: 10.32672/pice.v2i1.1367
![]() |
[28] | Sipahioglu, M., Empowering Teachers With Generative AI Tools and Support. Transforming Education With Generative AI: Prompt Engineering and Synthetic Content Creation, 2024,214–238. https://doi.org/10.4018/979-8-3693-1351-0.ch011 |
[29] |
Thornton, K., Leading through COVID-19: New Zealand secondary principals describe their reality. Educational Management Administration & Leadership, 2021, 49(3): 393–409. https://doi.org/10.1177/1741143220985110 doi: 10.1177/1741143220985110
![]() |
[30] |
Kern, M.L., Waters, L., Adler, A. and White, M., Assessing Employee Wellbeing in Schools Using a Multifaceted Approach: Associations with Physical Health, Life Satisfaction, and Professional Thriving. Psychology, 2014, 5(6): 500–513. https://doi.org/10.4236/psych.2014.56060 doi: 10.4236/psych.2014.56060
![]() |
[31] |
Yeh, C.S.H. and Barrington, R., Sustainable positive psychology interventions enhance primary teachers' wellbeing and beyond – A qualitative case study in England. Teaching and Teacher Education, 2023,125: 104072. https://doi.org/10.1016/j.tate.2023.104072 doi: 10.1016/j.tate.2023.104072
![]() |
[32] |
Goetz, T., Botes, E., Resch, L.M., Weiss, S., Frenzel, A.C. and Ebner, M., Teachers emotionally profit from positive school leadership: Applying the PERMA-Lead model to the control-value theory of emotions. Teaching and Teacher Education, 2024,141: 104517. https://doi.org/10.1016/j.tate.2024.104517 doi: 10.1016/j.tate.2024.104517
![]() |
[33] |
Seligman, M., PERMA and the building blocks of well-being. The Journal of Positive Psychology, 2018, 13(4): 333–335. https://doi.org/10.1080/17439760.2018.1437466 doi: 10.1080/17439760.2018.1437466
![]() |
[34] | Burns, J.M., Leadership, NY: Harper & Row, 1978. |
[35] |
Díaz, B. and Nussbaum, M., Artificial intelligence for teaching and learning in schools: The need for pedagogical intelligence. Computers & Education, 2024,217: 105071. https://doi.org/10.1016/j.compedu.2024.105071 doi: 10.1016/j.compedu.2024.105071
![]() |
[36] |
Popenici, S.A.D. and Kerr, S., Exploring the impact of artificial intelligence on teaching and learning in higher education. Research and Practice in Technology Enhanced Learning, 2017, 12(1): 22. https://doi.org/10.1186/s41039-017-0062-8 doi: 10.1186/s41039-017-0062-8
![]() |
[37] |
Su, J. and Yang, W., Artificial intelligence in early childhood education: A scoping review. Computers and Education: Artificial Intelligence, 2022, 3: 100049. https://doi.org/10.1016/j.caeai.2022.100049 doi: 10.1016/j.caeai.2022.100049
![]() |
[38] |
Ali, O., Murray, P.A., Momin, M., Dwivedi, Y.K. and Malik, T., The effects of artificial intelligence applications in educational settings: Challenges and strategies. Technological Forecasting and Social Change, 2024,199: 123076. https://doi.org/10.1016/j.techfore.2023.123076 doi: 10.1016/j.techfore.2023.123076
![]() |
[39] |
Ojha, A.K., Reflecting on management knowledge in India: Urgency to change the paradigm, decolonise and indigenise in the age of Artificial Intelligence. ⅡMB Management Review, 2024, 36(1): 7–20. https://doi.org/10.1016/j.iimb.2024.02.006 doi: 10.1016/j.iimb.2024.02.006
![]() |
[40] |
Adeleye, O.O., Eden, C.A. and Adeniyi, I.S., Innovative teaching methodologies in the era of artificial intelligence: A review of inclusive educational practices. World Journal of Advanced Engineering Technology and Sciences, 2024, 11(2): 069–079. https://doi.org/10.30574/wjaets.2024.11.2.0091 doi: 10.30574/wjaets.2024.11.2.0091
![]() |
[41] |
Ryan, R.M. and Deci, E.L., On Happiness and Human Potentials: A Review of Research on Hedonic and Eudaimonic Well-Being. Annual Review of Psychology, 2001, 52(1): 141–166. https://doi.org/10.1146/annurev.psych.52.1.141 doi: 10.1146/annurev.psych.52.1.141
![]() |
[42] |
Lester, L., Cefai, C., Cavioni, V., Barnes, A. and Cross, D., A Whole-School Approach to Promoting Staff Wellbeing. Australian Journal of Teacher Education, 2020, 45(2): 1–22. https://doi.org/10.14221/ajte.2020v45n2.1 doi: 10.14221/ajte.2020v45n2.1
![]() |
[43] | McLean, L. and Sandilos, L., Teachers' Well-Being: Sources, Implications, and Directions for Research. In Teachers' Well-Being: Sources, Implications, and Directions for Research, 2022. https://doi.org/10.4324/9781138609877-REE153-1 |
[44] | Falecki, D. and Mann, E., Practical Applications for Building Teacher WellBeing in Education. Cultivating Teacher Resilience, 2021,175–191. Springer Singapore. https://doi.org/10.1007/978-981-15-5963-1_11 |
[45] | O'Sullivan, C., Ryan, S. and O'Sullivan, L., Teacher Well-Being in Diverse School and Preschool Contexts. International perspectives on teacher well-being and diversity: Portals into innovative classroom practice, 2021,163–187. https://doi.org/10.1007/978-981-16-1699-0_8 |
[46] |
Corcoran, R.P. and O'Flaherty, J., Social and emotional learning in teacher preparation: Pre-service teacher well-being. Teaching and Teacher Education, 2022,110: 103563. https://doi.org/10.1016/j.tate.2021.103563 doi: 10.1016/j.tate.2021.103563
![]() |
[47] |
Pearce, A., Achieving Academic Satisfaction through the use of Education-Based Technologies: Strengthening Students Personal Well-being While Facing Online Learning Mandates and Digital Disparities. Global Journal of Human-Social Science, 2021, 21(5): 19–36. https://doi.org/10.34257/LJRHSSVOL21IS5PG21 doi: 10.34257/LJRHSSVOL21IS5PG21
![]() |
[48] |
Cabrera, V. and Donaldson, S.I., PERMA to PERMA+4 building blocks of well-being: A systematic review of the empirical literature. The Journal of Positive Psychology, 2024, 19(3): 510–529. https://doi.org/10.1080/17439760.2023.2208099 doi: 10.1080/17439760.2023.2208099
![]() |
[49] |
Eloff, I. and Dittrich, A.K., Understanding general pedagogical knowledge influences on sustainable teacher well-being: A qualitative exploratory study. Journal of Psychology in Africa, 2021, 31(5): 464–469. https://doi.org/10.1080/14330237.2021.1978166 doi: 10.1080/14330237.2021.1978166
![]() |
[50] |
Ausat, A.M.A., Shafiq, M.A., Wafik, D. and López, N.A.S., The Role of Transformational Leadership in Improving Employee Psychological Wellbeing: A Review. Apollo: Journal of Tourism and Business, 2024, 2(1): 148–157. https://doi.org/10.58905/apollo.v2i1.239 doi: 10.58905/apollo.v2i1.239
![]() |
[51] |
Daniëls, E., Hondeghem, A. and Dochy, F., A review on leadership and leadership development in educational settings. Educational Research Review, 2019, 27: 110–125. https://doi.org/10.1016/j.edurev.2019.02.003 doi: 10.1016/j.edurev.2019.02.003
![]() |
[52] | Litz, D. and Blaik-Hourani, R., Transformational Leadership and Change in Education. In Oxford Research Encyclopedia of Education, Oxford University Press, 2020. https://doi.org/10.1093/acrefore/9780190264093.013.631 |
[53] | Bass, B.M., From Transactional to Transformational Leadership: Learning to Share the Vision. In R. P. Vecchio (Ed.), Leadership: Understanding the Dynamics of Power and Influence in Organizations, Second Edition, 2007,302–317. University of Notre Dame Press. https://doi.org/10.2307/j.ctvpg85tk.29 |
[54] |
McCarthy, A.M., Maor, D., McConney, A. and Cavanaugh, C., Digital transformation in education: Critical components for leaders of system change. Social Sciences & Humanities Open, 2023, 8(1): 100479. https://doi.org/10.1016/j.ssaho.2023.100479 doi: 10.1016/j.ssaho.2023.100479
![]() |
[55] |
Venema-Steen, I., Southall, A. and Bortoli, A., Drawing on the Locus of Control Framework to Explore the Role of School Leaders in Teacher Well-Being. Journal of Educational Research and Practice, 2023, 13(1). https://doi.org/10.5590/JERAP.2023.13.1.11 doi: 10.5590/JERAP.2023.13.1.11
![]() |
[56] | Voelkel, R.H., Supporting Effective Professional Learning Communities Through Developing Increased Principal Leadership Capacity. Proceedings of the 2022 AERA Annual Meeting. 2022. https://doi.org/10.3102/1890357 |
[57] |
Cheng, E.C.K. and Wang, T., Leading digital transformation and eliminating barriers for teachers to incorporate artificial intelligence in basic education in Hong Kong. Computers and Education: Artificial Intelligence, 2023, 5: 100171. https://doi.org/10.1016/j.caeai.2023.100171 doi: 10.1016/j.caeai.2023.100171
![]() |
[58] |
Schmitz, M.L., Antonietti, C., Consoli, T., Cattaneo, A., Gonon, P. and Petko, D., Transformational leadership for technology integration in schools: Empowering teachers to use technology in a more demanding way. Computers & Education, 2023,204: 104880. https://doi.org/10.1016/j.compedu.2023.104880 doi: 10.1016/j.compedu.2023.104880
![]() |
[59] |
Raman, A., Don, Y. and Latif Kasim, A., The Relationship between Principals' Technology Leadership and Teachers' Technology Use in Malaysian Secondary Schools. Asian Social Science, 2014, 10(18): 30‒36. https://doi.org/10.5539/ass.v10n18p30 doi: 10.5539/ass.v10n18p30
![]() |
[60] |
Kern, M.L., Waters, L.E., Adler, A. and White, M.A., A multidimensional approach to measuring well-being in students: Application of the PERMA framework. The Journal of Positive Psychology, 2015, 10(3): 262–271. https://doi.org/10.1080/17439760.2014.936962 doi: 10.1080/17439760.2014.936962
![]() |
[61] |
Butler, J. and Kern, M.L., The PERMA-Profiler: A brief multidimensional measure of flourishing. International Journal of Wellbeing, 2016, 6(3): 1–48. https://doi.org/10.5502/ijw.v6i3.526 doi: 10.5502/ijw.v6i3.526
![]() |
[62] |
Mtebe, J.S., Mbwilo, B. and Kissaka, M.M., Factors Influencing Teachers' Use of Multimedia Enhanced Content in Secondary Schools in Tanzania. The International Review of Research in Open and Distributed Learning, 2016, 17(2): 65‒84. https://doi.org/10.19173/irrodl.v17i2.2280 doi: 10.19173/irrodl.v17i2.2280
![]() |
[63] |
Berkovich, I. and Eyal, O., Educational Leaders and Emotions. Review of Educational Research, 2015, 85(1): 129–167. https://doi.org/10.3102/0034654314550046 doi: 10.3102/0034654314550046
![]() |
[64] | Brown, T.A., Confirmatory factor analysis for applied research, Guilford publications, 2015. |
[65] |
Hau, K.T. Book Review of Structural Equation Modeling With LISREL, PRELIS, and SIMPLIS: Basic Concepts, Applications, and Programming. Structural Equation Modeling: A Multidisciplinary Journal, 2000, 7(4): 640–643. https://doi.org/10.1207/S15328007SEM0704_8 doi: 10.1207/S15328007SEM0704_8
![]() |
[66] | Mercer, S., Oberdorfer, P. and Saleem, M., Helping Language Teachers to Thrive: Using Positive Psychology to Promote Teachers' Professional Well-Being, Positive psychology perspectives on foreign language learning and teaching, 2016,213–229. https://doi.org/10.1007/978-3-319-32954-3_12 |
[67] |
Dreer, B., Teachers' well-being and job satisfaction: the important role of positive emotions in the workplace. Educational Studies, 2024, 50(1): 61–77. https://doi.org/10.1080/03055698.2021.1940872 doi: 10.1080/03055698.2021.1940872
![]() |
[68] |
Wassink, S.G., van Wingerden, J. and Poell, R.F., Correction: Meaningful work and resilience among teachers: The mediating role of work engagement and job crafting. PLOS ONE, 2022, 17(5): e0269347. https://doi.org/10.1371/journal.pone.0269347 doi: 10.1371/journal.pone.0269347
![]() |
[69] |
Polatcan, M., Özkan, P. and Bellibaş, M.Ş., Cultivating teacher innovativeness through transformational leadership and teacher agency in schools: the moderating role of teacher trust. Journal of Professional Capital and Community, 2024, 9(3): 227–242. https://doi.org/10.1108/JPCC-01-2024-0008 doi: 10.1108/JPCC-01-2024-0008
![]() |
[70] |
Brooks, M., Creely, E. and Laletas, S., Coping through the unknown: School staff wellbeing during the COVID-19 pandemic. International Journal of Educational Research Open, 2022, 3: 100146. https://doi.org/10.1016/j.ijedro.2022.100146 doi: 10.1016/j.ijedro.2022.100146
![]() |
[71] |
Orunbon, N.O. and Ibikunle, G.A., Principals' Toxic Leadership Behaviour and Teachers' Workplace Incivility in Public Senior Secondary Schools, Lagos State, Nigeria. EduLine: Journal of Education and Learning Innovation, 2023, 3(2): 202–213. https://doi.org/10.35877/454RI.eduline1717 doi: 10.35877/454RI.eduline1717
![]() |
1. | Rina Su, Chunrui Zhang, The generation mechanism of Turing-pattern in a Tree-grass competition model with cross diffusion and time delay, 2022, 19, 1551-0018, 12073, 10.3934/mbe.2022562 | |
2. | Wonhyung Choi, Kwangjoong Kim, Inkyung Ahn, Predation-induced dispersal toward fitness for predator invasion in predator–prey models, 2023, 17, 1751-3758, 10.1080/17513758.2023.2166133 | |
3. | Toan D. Ha, Vyacheslav G. Tsybulin, Pavel A. Zelenchuk, How to model the local interaction in the predator–prey system at slow diffusion in a heterogeneous environment?, 2022, 52, 1476945X, 101026, 10.1016/j.ecocom.2022.101026 | |
4. | T. D. Ha, V. G. Tsybulin, Multistability for a Mathematical Model of the Dynamics of Predators and Preys in a Heterogeneous Area, 2022, 68, 2413-3639, 509, 10.22363/2413-3639-2022-68-3-509-521 | |
5. | Wonhyung Choi, Kwangjoong Kim, Inkyung Ahn, Predator-prey models with prey-dependent diffusion on predators in spatially heterogeneous habitat, 2023, 525, 0022247X, 127130, 10.1016/j.jmaa.2023.127130 | |
6. | T. D. Ha, V. G. Tsybulin, Multistability for a Mathematical Model of the Dynamics of Predators and Prey in a Heterogeneous Area, 2024, 282, 1072-3374, 417, 10.1007/s10958-024-07185-y | |
7. | P. A. Zelenchuk, V. G. Tsybulin, Mathematical model of ideal free distribution in the predator-prey system, 2023, 69, 2949-0618, 237, 10.22363/2413-3639-2023-69-2-237-249 | |
8. | P. A. Zelenchuk, V. G. Tsybulin, Mathematical Model of Ideal Free Distribution in the Predator–Prey System, 2024, 1072-3374, 10.1007/s10958-024-07445-x |