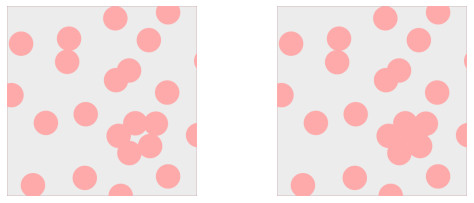
We studied stochastic homogenization of a quasi-linear parabolic partial differential equation (PDE) with nonlinear microscopic Robin conditions on a perforated domain. The focus of our work lies in the underlying geometry that does not allow standard stochastic homogenization techniques to be applied directly. Instead, we introduced a concept of regularized homogenization: We proved homogenization on a regularized but still random geometry and demonstrated afterwards that the form of the homogenized equation was independent from the regularization, though the explicit values of the coefficients depended on the regularization. Then, we passed to the regularization limit to obtain the anticipated limit equation where the coefficients were finally independent from the intermediate regularizations. We provided evidence that the regularized homogenization and the classical stochastic homogenization coincided on geometries that indeed allowed stochastic homogenization. Furthermore, we showed that Boolean models of Poisson point processes were covered by our approach.
Citation: Martin Heida, Benedikt Jahnel, Anh Duc Vu. Regularized homogenization on irregularly perforated domains[J]. Networks and Heterogeneous Media, 2025, 20(1): 165-212. doi: 10.3934/nhm.2025010
[1] | Martin Heida . Stochastic homogenization on perforated domains Ⅰ – Extension Operators. Networks and Heterogeneous Media, 2023, 18(4): 1820-1897. doi: 10.3934/nhm.2023079 |
[2] | Arianna Giunti . Convergence rates for the homogenization of the Poisson problem in randomly perforated domains. Networks and Heterogeneous Media, 2021, 16(3): 341-375. doi: 10.3934/nhm.2021009 |
[3] | Daniel Peterseim . Robustness of finite element simulations in densely packed random particle composites. Networks and Heterogeneous Media, 2012, 7(1): 113-126. doi: 10.3934/nhm.2012.7.113 |
[4] | Patrizia Donato, Olivier Guibé, Alip Oropeza . Corrector results for a class of elliptic problems with nonlinear Robin conditions and $ L^1 $ data. Networks and Heterogeneous Media, 2023, 18(3): 1236-1259. doi: 10.3934/nhm.2023054 |
[5] | Hakima Bessaih, Yalchin Efendiev, Florin Maris . Homogenization of the evolution Stokes equation in a perforated domain with a stochastic Fourier boundary condition. Networks and Heterogeneous Media, 2015, 10(2): 343-367. doi: 10.3934/nhm.2015.10.343 |
[6] | Shin-Ichiro Ei, Toshio Ishimoto . Effect of boundary conditions on the dynamics of a pulse solution for reaction-diffusion systems. Networks and Heterogeneous Media, 2013, 8(1): 191-209. doi: 10.3934/nhm.2013.8.191 |
[7] | Catherine Choquet, Ali Sili . Homogenization of a model of displacement with unbounded viscosity. Networks and Heterogeneous Media, 2009, 4(4): 649-666. doi: 10.3934/nhm.2009.4.649 |
[8] | Brahim Amaziane, Leonid Pankratov, Andrey Piatnitski . Homogenization of variational functionals with nonstandard growth in perforated domains. Networks and Heterogeneous Media, 2010, 5(2): 189-215. doi: 10.3934/nhm.2010.5.189 |
[9] | Manuel Friedrich, Bernd Schmidt . On a discrete-to-continuum convergence result for a two dimensional brittle material in the small displacement regime. Networks and Heterogeneous Media, 2015, 10(2): 321-342. doi: 10.3934/nhm.2015.10.321 |
[10] | Iryna Pankratova, Andrey Piatnitski . Homogenization of convection-diffusion equation in infinite cylinder. Networks and Heterogeneous Media, 2011, 6(1): 111-126. doi: 10.3934/nhm.2011.6.111 |
We studied stochastic homogenization of a quasi-linear parabolic partial differential equation (PDE) with nonlinear microscopic Robin conditions on a perforated domain. The focus of our work lies in the underlying geometry that does not allow standard stochastic homogenization techniques to be applied directly. Instead, we introduced a concept of regularized homogenization: We proved homogenization on a regularized but still random geometry and demonstrated afterwards that the form of the homogenized equation was independent from the regularization, though the explicit values of the coefficients depended on the regularization. Then, we passed to the regularization limit to obtain the anticipated limit equation where the coefficients were finally independent from the intermediate regularizations. We provided evidence that the regularized homogenization and the classical stochastic homogenization coincided on geometries that indeed allowed stochastic homogenization. Furthermore, we showed that Boolean models of Poisson point processes were covered by our approach.
Soon after the groundbreaking introduction of stochastic homogenization by Papanicolaou and Varadhan [22] and Kozlov [12], research developed a natural interest in the homogenization of randomly perforated domains. A good summary of the existing methods up to 1994 can be found in [13]. By the same time, Zhikov [28] provided a homogenization result for linear parabolic equations on stationary randomly perforated domains. Bourgeat et al. [27] introduced a concept of two-scale convergence in the mean for stochastic problems, where the two-scale limit is performed simultaneously over all realizations. This method was the first to apply the two-scale convergence idea to a stochastic setting, but it was not capable to deal with nonlinearities that require strong convergence of solutions (see also [10]). Hence, we cannot benefit of this approach in our work. It then became silent for a decade. In [30], Zhikov and Piatnitsky reopened the case by introducing stochastic two-scale convergence as a generalization of [20,1,29] to the stochastic setting, particularly to random measures that comprise random perforations and random lower-dimensional structures in a natural way. The method was generalized to various applications in discrete and continuous homogenization [16,3,4] and recently also to an unfolding method [19,10].
Concerning the homogenization on randomly perforated domains, there seems to be few results in the literature, with [7,5,23] being the closest related work from the PDE point of view. We emphasize that there is a further discipline in stochastic homogenization, studying critical regimes of scaling for holes in a perforated domain of the Stokes equation; see [6] and references therein.
In this work, we focus on the geometric aspects in the homogenization of quasi-linear parabolic equations and go beyond any recent assumptions on the random geometry. Given ε>0, we consider a bounded domain Q⊂Rd perforated by a random set Gε and write Qε:=Q∖Gε. Typically, Gε≈εG where G is a stationary random set and Gε is additionally regularized close to ∂Q [7,5,23]. Also, we assume that Qε is connected, for simplicity of calculations and presentation, and our geometric model will be regularized in such a way; see also Remark 5.
We then study the following PDE on Qε for the time interval I=[0,T]:
∂tuε−∇⋅(A(uε)∇uε)=fin I×QεA(uε)∇uε⋅ν=0on I×∂QA(uε)∇uε⋅ν=εh(uε)on I×∂Qε∖∂Quε(0,x)=u0(x)in Qε | (1.1) |
with ν being the outer normal vector.
In case of a fully linear PDE, i.e., h(⋅)=const and A(⋅)=const, this problem was homogenized already in the aforementioned [28] and later reconsidered in [30]. In this linear case, one benefits from the regularity of the limit solution and the weak convergence of the ε-solutions that is given a priori.
However, the nonlinear case is more difficult. Weak convergence of solutions is no longer sufficient. Thus, one needs to establish strong convergence of the uε. As we will discuss below, a lack of a uniformly continuous family of extension operators or, more generally, a degeneration of the homogenized matrix will cause the whole argumentation to break down. Hence, typical assumptions in the literature, such as minimal smoothness (see Definition 23) of G and uniform boundedness of the hole sizes, ensure the existence of uniformly bounded extension operators Uε,∙:W1,2(Qε)→W1,2(Q) [7]. This in turn implies weak compactness of Uε,∙uε in W1,2(Q), a property of uttermost importance to pass to the homogenization limit in the nonlinear terms. Other approaches are conceivable, e.g., exploiting the Frechet–Riesz–Kolmogorov compactness theorem, but in application the prerequisites are hard to prove.
If all limit passages go through, the homogenized limit as ε→0 reads for some positive definite matrix A(G) as
C1,(G)∂tu−div(A(u)A(G)∇u)−C2,(G)h(u)=C1,(G)fin I×QA(u)A(G)∇u⋅ν=0on I×∂Qu(0,x)=C1,(G)u0(x)in Q, | (1.2) |
which represents the macroscopic behavior of our object. We note at this point that positivity of A(G) is, in general, nontrivial but can be shown for minimally smooth domain examples (see Sections 8 and 9).
Unfortunately, canonical perforation models are neither minimally smooth nor is the size of their holes uniformly bounded. Our toy model of choice will be the Boolean model ΞXpoi:=¯⋃x∈XpoiBr(x) (see Definition 1) driven by a Poisson point process Xpoi. It clearly reveals the following general issues for the homogenization analysis:
i) ΞX∁poi=Rd∖ΞXpoi is not connected due to areas that are encircled.
ii) Two distinct balls can lie arbitrarily close to each other or – in case they intersect – have arbitrary small overlap. This implies that
● the connected components in ΞXpoi develop arbitrarily large local Lipschitz constants: Two balls of equal radius intersecting at an angle α have the Lipschitz constant tan((π−α)/2) at the points of intersection, and
● there is no δ>0 such that for every p∈∂ΞX∁poi the surface Bδ(p)∩∂ΞX∁poi is a graph of a function: If x,y∈Xpoi with |x−y|=2r+η and |p−x|=r, |p−y|=r+η, Bδ(p)∩∂ΞXpoi can be a graph only if δ<η.
The first issue can be fixed by considering a "filled-up model" ⊟Xpoi in Definition 1. Furthermore, we will see that – under mild regularity conditions – the procedure of filling up a perforation does not change the effective conductivity A(G) at all (Theorem 58). Unfortunately, the second issue poses an actual problem. In a recent work [9], one of the authors has shown that in some cases an extension operator Uε,∙:W1,p(Qε)→W1,q(Q), 1≤q<p, can be constructed for some geometries including the Boolean model (strictly speaking, this was shown for an extension from the balls to the complement in the percolation case). However, [9] also suggests that the Boolean model for the Poisson point process requires p>2 in order for Uε,∙ to be properly defined for some q≥1.
Due to these severe analytical difficulties, we need other approaches to the problem. We call our approach 'regularized homogenization' and it consists of an approximation of the random geometry G by Gn, performing homogenization, and afterward letting n→∞. In our particular example, it consists of the following steps:
i) Given a general stationary ergodic (admissible) random point process X, we construct a regularization X(n):=FnX (see Definition 3) such that the set ⊟X(n) is uniformly minimally smooth for given n∈N.
ii) Given n∈N, we perform homogenization for the smoothed geometry ⊟X(n) instead of ⊟X (see Lemma 7).
iii) We pass to the limit n→∞ along a subsequence to obtain the anticipated homogenized limit problem (see Theorem 56), where the coefficients are independent from the intermediate regularizations (Corollary 39). This happens under the assumption that ⊟X∁ is statistically connected (see Definition 15).
iv) We show that the Poisson point process in the subcritical regime is a valid example for our general homogenization result (see Section 9).
We are thus in a position to prove an indirect homogenization result. This seems to us an appropriate intermediate step on the way to a full homogenization result, which may be achieved in the future using further developed hom ogenization techniques based on a better understanding of the interaction of geometry and homogenization. Let us note that we focus on fixed radii r>0 for the sake of presentation. In fact, random radii pose no issue for the procedure as briefly mentioned in Remark 18 as long as the remaining conditions are satisfied.
This paper is structured as follows:
● In Section 3, we introduce the core objects and state the main result. This includes the thinned point processes X(n) and its filled-up Boolean model ⊟X(n).
● In Section 4, we prove relevant properties of the thinning map and the thinned point processes, most importantly, minimal smoothness of ⊟X(n) (Theorem 25) and ⊟X(n)→⊟X in a certain sense (Lemma 29).
● Section 5 deals with the cell solutions and the definition of the effective conductivity A.
● The homogenization theory for minimally smooth holes is sketched in Section 6 on the basis of stochastic two-scale convergence. Due to the considerations in Section 5, the underlying probability space is a compact separable metric space.
● In Section 7, we show that the homogenized solutions to Eq (1.2) for G=⊟X(n) converge and that their limit is a solution to the anticipated limit problem for G=⊟X.
● Section 8 establishes a criterion for statistical connectedness (nondegeneracy of the effective conductivity A) using percolation channels. We follow the ideas in [13, Chapter 9] where a discrete model was considered.
● In Section 9, we show that the Poisson point process Xpoi is indeed admissible, which follows from readily available percolation results. Showing statistical connectedness of ⊟X∁poi is much harder. We do so using the criterion established in Section 8 and a version of [11, Theorem 11.1]. As the original [11, Theorem 11.1] is a statement about percolation channels on the Z2-lattice, we need to adjust both the statement and the proof to our setting.
General notation
● M(Rd): Space of Radon measures on Rd equipped with the vague topology
● S(Rd)⊂M(Rd): Space of boundedly finite point clouds/point measures in Rd
● A∁: Complement of a set A
● B(X): Borel-σ-algebra of the topological space X
● Ld: d-dimensional Lebesgue-measure
● Hd: d-dimensional Hausdorff-measure
● Hd⌞A: Restriction of Hd to A, i.e. Hd⌞A(B):=Hd(B∩A)
● o:=0Rd∈Rd: Origin in Rd
● 1A: Indicator/characteristic function of a set A
Specific notation introduced later
● Br(A): Open r-neighborhood around A. (Definition 1)
● Ξx and ⊟x: Boolean model of x and its filled version (Definition 1)
● Cx(x): Cluster of x in x∈S(Rd) (Definition 3)
● x(n) for x∈S(Rd): x(n)=Fnx with thinning map Fn (Definition 3)
● Qεx and Jε(Q,x): Perforated domain and index set generating perforations (Definition 4)
● τx:M(Rd)→M(Rd): Shift-operator in M(Rd) (Definition 8)
● λ(μ): Intensity of random measure μ (Definition 8)
● μx: Hd−1 restricted to ∂⊟x (Definition 30)
● A and αA: Effective conductivity and smallest eigenvalue of A (Definition 37)
● U and T: Extension and trace operators (Theorem 43 and Theorem 51)
● με: Scaled measure (Assumption 46)
We start by introducing some concepts from the theory of point processes. We will not formulate the concepts in full generality but only as general as needed for our purpose. Let d≥2 and let S(Rd) be the set of boundedly finite point clouds in Rd (i.e., point clouds without accumulation points) and M(Rd) the space of Radon measures with the vague topology, that is, the smallest topology on M(Rd) such that
μ↦∫Rdfdμ |
is continuous for every f∈C∞c(Rd). Every x∈S(Rd) can be identified with a Borel measure through the correspondence
x(A)=∑x∈xδx(A). |
Hence, we identify S(Rd)⊂M(Rd).
Our perforation model of interest is the Boolean model driven by a point cloud x∈S(Rd). While it is a natural way to generate perforations, we require its complement to be connected for suitable x. Hence, the perforation Ξx needs to be filled up in order to remove all finite-sized connected components from its complement. These can be easily identified as they do not admit a path of infinite diameter.
Definition 1 (Boolean model Ξ of a point cloud and filled-up model ⊟ (see Figure 1)). Let x∈S(Rd). The Boolean model of x for a radius r>0 is
Ξx:=¯⋃x∈xBr(x)=¯Br(x), |
where Br(x) is the open ball of radius r around x and Br(A):=⋃x∈ABr(x). We define the filled-up Boolean model ⊟x of x for radius r through its complement, i.e.,
⊟x∁:={x∈Rd|∃γ:[0,∞)→Ξx∁continuous andγ(0)=x,lim supt→∞|γ(t)|=∞}. |
Remark 2. We observe that
Ξ(x+x)=Ξ(x)+xand⊟(x+x)=⊟x+x. |
As discussed in the introduction, we need to "smoothen" the geometry in order to be able to apply standard homogenization methods. Given a Lipschitz domain P⊂Rd, we define for p∈∂P
δ(p):=12supδ′>0{∂P is Lipschitz-graph in Bδ′(p)}, |
and because δ:∂P→R≥0 is continuous [9], we can define for bounded P
δ(P):=minp∈∂Pδ(p). |
Definition 3 (Thinning maps Fn (see Figure 2)). Let x∈S(Rd) be a point cloud. We denote the cluster of x in x by
Cx(x):={y∈x|∃path from x to y inside Ξx}. |
We set
F1,nx:={x∈x|∀y∈x:d(x,y)∉(0,1n)∪(2r−1n,2r+1n)}, | (3.1) |
F2,nx:={x∈x|#Cx(x)≤n,δ(Br(Cx(x)))≥1n}, | (3.2) |
and define the thinning map Fn
Fn:S(Rd)→S(Rd),x(n):=Fnx:=(F2,n∘F1,n)x. |
Fn can be understood as a generalization of the classical Matérn construction [15,26]. For an arbitrary x∈S(Rd), we see that (⊟x(n))∁ is always minimally smooth (see Definition 23). Furthermore, if X is a stationary point process (as defined later), then the same holds for X(n)=FnX. We note that Fn is in general not monotone in n, i.e., Fmx⊄Fnx for m≤n.
Given a scale ε>0, we define the perforation domain Qε such that the perforations have some minimal distance from the boundary ∂Q:
Definition 4 (Perforation of domain Qε). Let x∈S(Rd). We set
Jε(x,Q):={x∈x|dist(εCx(x),Q∁)>2εr},Gεx:=ε⊟(Jε(x,Q)) |
as well as the perforated domain
Qεx:=Q∖Gεx. |
One quickly verifies that Qεx(n) is minimally smooth (Definition 23); see Theorem 25.
Remark 5. By construction, our perforation model Gεx:=ε⊟(Jε(x,Q)) ensures connectedness of its complement Rd∖Gεx, resp., of Qεx. We prefer this approach of "filling the holes of the holes" because it allows for an easy use of extension operators in a way that we can safely apply compact embeddings. Otherwise, once Rd∖Gεx becomes disconnected, we would have to rely on additional regularity assumptions on the initial data in those holes, which is then carried on by the evolution equation and would lead to additional tedious calculations. Otherwise, we would have to introduce a second PDE on the holes with suitable coupling boundary conditions. While we do not claim that a treatment of such a situation is impossible, we claim that the additional effort would not be justified by the additional expected insight.
We make the following parameter assumptions on our partial differential Eq (1.1).
Assumption 6 (Parameters of PDE). Let I=[0,T]⊂R and Q⊂Rd be a bounded, connected open domain. We assume that
● u0∈W1,2(Q)
● f∈L2(I;L2(Q))
● h:R→R is Lipschitz continuous with Lipschitz constant Lh
● A:R→R is continuous with 0<inf(A) and sup(A)<∞.
Generalized time derivatives will always be considered under the evolution triple W1,2(Q)↪L2(Q)↪W1,2(Q)∗ or W1,2(Qεx(n))↪L2(Qεx(n))↪W1,2(Qεx(n))∗ in the case of a perforated domain Qεx(n).
Lemma 7 (Solution to PDE for minimally smooth holes). Let x∈S(Rd) and n∈N. Under Assumption 6, we have on Qεx(n): There exists a weak solution uε∈L2(I;W1,2(Qεx(n))) with generalized time derivative ∂tuε∈L2(I;W1,2(Qεx(n))∗) to Eq (1.1) on Qεx(n) instead of Qε.
This uε satisfies for some C>0 independent of ε depending only on Q, n, f and u0 but not on ε
esssupt∈I‖uε(t)‖L2(Qεx(n))+‖uε‖L2(I;W1,2(Qεx(n)))≤C‖∂tuε‖L2(I;W1,2(Qεx(n))∗)≤C. |
The proof is given in Section 6 (Theorem 53).
The next step is passing to the limit . We do so in the case of being a realization of a stationary ergodic point process as defined below:
Definition 8 (Random measure and shift-operator ). A random measure is a random variable with values in . It induces a probability distribution on . Given the continuous map
(3.3) |
a random measure is stationary if for every and every . In line with the above setting, a random point process is a random measure with , and one quickly verifies that is stationary if for every , , and bounded open , it holds
We call a stationary random measure ergodic if the -algebra of -invariant sets is trivial under its distribution .
Remark 9 (Compatibility of thinning with shifts). The thinning map is compatible with the shift , i.e., on
Lemma 10 (Homogenized PDE for minimally smooth domains). Let be a stationary ergodic point process and be fixed. For almost every realization of , we have under Assumption 6:
For , let be a solution to Eq (1.1) on instead of . Given any sequence , we find a subsequence (still denoted as ) and corresponding with , such that strongly in for some with generalized time derivative . This is a weak solution to
(3.4) |
with constants depending on the distribution of and being a symmetric positive semi-definite matrix – the so-called effective conductivity based on the event that the origin is not covered by (see Definition 37).
Proof. This is shown in Section 6 (Theorem 55) using two-scale convergence.
A more general result, which immediately is implied by the proof of the previous lemma is the following Lemma 11. There are many random geometries that do not have an extension operator but still an extension operator for some , see [9]. For such geometries, the desired homogenization result could be established without the bypass that we will take below. However, results in [9] will provide sufficient conditions for homogenization, but they do not provide necessary conditions.
Lemma 11. Assume that is such that there exists such that for every family with , there exists a , such that and such that is pre-compact in . Then, every convergent subsequence of the solutions of Eq (1.1) converges to a weak solution of Eq (3.5) given below.
Remark 12 (Estimate). Observe that the situation in Lemma 7 provides us with an estimate on the solutions , and the compact operator is a combination of the extension operator for minimally smooth domains and the standard Sobolev embedding.
Remark 13 (Compactness). It has to be noted that the claim of compactness is somewhat natural and for most nonlinearities, even necessary. Indeed, unless the nonlinearities are monotone operators, we are not aware of an existence theory for equations of Type (1.1), which would not rely on compactness of a sequence of approximate solutions in some -space. However, if existence theory is already heavily relying on this compactness assumption, we have no hope that homogenization could cope with less.
When it comes to the final homogenization result, we will need the following assumptions on the point process .
Definition 14 (Admissible point process). We call a point cloud admissible the following holds (with from Definition 1):
i) Equidistance Property: .
ii) Finite Clusters: For every , we have .
A stationary ergodic boundedly finite point process is called admissible if its realizations are almost surely admissible.
Definition 15 (Statistical connectedness). The random set is statistically connected if the effective conductivity (Definition 37) based on the event that the origin is covered by is strictly positive definite.
In our setting of Boolean models of admissible point processes, the procedure of filling up a perforation does not change its effective conductivity (Theorem 58). In particular, is statistically connected if, and only if, the same holds for .
Remark 16 (On statistical connectedness). Let us note that the existence of an infinite connected component in is a necessary requirement for , as can be seen later in Section 8. There, we also give a criterion for statistical connectedness: the existence of sufficiently many so-called percolation channels. The procedure is based on [13, Lemma 9.7], which we adjust to the continuum setting.
We may now state the main theorem of this work.
Theorem 17 (Homogenized limit for admissible point processes). Let be an admissible point process and statistically connected. Under Assumption 6, we have for almost every realization of : For every , let be a homogenized limit in Lemma 10. For every subsequence of , we can extract a subsequence such that there exists a with generalized time-derivative
and is a weak solution to
(3.5) |
with constants only depending on the distribution of and being a symmetric positive definite matrix – the so-called effective conductivity based on the event that the origin is not covered by (Definition 37). The limit may depend on the chosen subsequence if the solution to Eq (3.5) is not unique. Otherwise, the whole sequence converges to .
Proof. This is proved in Theorem 56.
Regarding the derivation of Eq (3.5), we only need local convergence of (Lemma 29). Hence, Eq (3.5) is independent of the thinning procedure as long as local convergence is satisfied.
Remark 18 (Random radii). For simplicity, we have chosen the Boolean model with fixed radius as our underlying model. One can easily generalize the procedure to random independent radii. Given a marked point process whose marks represent the radius of the ball around , we need to adjust Point 1 in Definition 14 accordingly, i.e.,
The thinning maps (Definition 3) also need to be modified to ensure minimal smoothness (Definition 23), e.g., balls with especially large/small radii need to be removed. Eq (3.1) has the purpose to ensure that two balls in the regularized geometry either have a minimal distance or, if they intersect, the intersection angle is bounded away from . This also has to be modified depending on radii and of two intersecting balls. Local convergence is preserved if all clusters remain finite. Hence, the rest of our procedure essentially follows as is under the assumption of .
Remark 19 (Homogenization procedure). For fixed , solutions to Eq (1.1) exist for admissible as for large enough (Lemma 29). If is a realization of some admissible point process , then this is still not sufficient to pass to the limit . The missing regularity of still prevents us from establishing a priori estimates. All in all, our procedure yields the following diagram:
![]() |
(3.6) |
Statistical connectedness of is crucial to establish -estimates for . This indicates that the direct limit passing might only rely on the statistical connectedness property, but we cannot answer that as of now. On the other hand, if the assumptions of Lemma 11 hold, then the diagram commutes.
In order to demonstrate that the class of point process satisfying our assumptions is not empty, we show in Section 9 that the Poisson point process is indeed suitable for our framework. We obtain the following.
Theorem 20 (Admissibility and statistical connectedness for ). In the subcritical regime (see Assumption 62), we have for the Poisson point process that
● is an admissible point process.
● is statistically connected.
While admissibility is easily proven, statistical connectedness is much harder to deal with. Most of Section 9 is dedicated to this proof. It also builds up on Section 8 in which we show that so-called percolation channels yield statistical connectedness.
In what follows, we collect examples where stochastic homogenization is well understood and where our new ansatz of regularized stochastic homogenization is consistent in the sense that the Diagram (3.6) commutes for these examples.
Let be a stationary ergodic random set and let be jointly stationary sets with where and where pointwise as . We then define as before and additionally . Then, by definition, and we have the following result.
Theorem 21. Under the above assumptions, let be extended by to and .
i) For each , assume that as . Then, the solutions of the problem
(3.7) |
satisfy as , where is the solution to Eq (3.7) on .
ii) For each , there exists a positive semi-definite such that as implies in , where solves
iii) If as , then and weakly in . Note from Statement 2 that solves
In other words, Diagram 3.6 commutes for linear equations.
We now sketch the homogenization of Eq (1.1) on a geometry that is not minimally smooth but allows for homogenization. This sample geometry was introduced in [9]: We start from a Poisson point process and erase all points that are closer to each other than a given distance threshold . We then construct the Delaunay triangulation and assign a pipe of random diameter with a distribution to every edge, for all . Furthermore, we enrich the geometry by the balls of radius around the remaining points. This union of pipes and balls is stationary and ergodic and we then define as the intersection of with the system of balls and pipes scaled by a factor . In three dimensions, the complement of is pathwise connected and unbounded, but in two dimensions, the complement consists of bounded sets, where there is no upper bound on the diameter of these sets. Also, there is no upper bound on the local Lipschitz constant.
Writing for the functions in with value zero on then, in [9, Theorem 1.15], it is shown that, for every , there almost-surely exists an extension operator that is continuous with depending only on the random geometry, such that, for every and , it holds that
(3.8) |
An inspection of the proof of [9, Theorem 1.7] reveals that it also holds for therein, that is, there exists a constant , independent from , such that
(3.9) |
The important insight is that is bounded in and hence, pre-compact in , which in turn yields two-scale pre-compactness of the traces , in a similar way as below. Thus, we can argue as in our proof of Lemma 10 to obtain the respective homogenization result. The needed two-scale convergence methods have been introduced in [8].
On the other hand, in , we can regularize the geometry by filling up holes with a diameter larger than a prescribed threshold and we can additionally prescribe a minimal thickness for the pipes. Since the generating points of the Delaunay triangulation have a minimal mutual distance, the filling of large holes provides minimal smoothness. However, Lemma 10 guarantees homogenization. Together with the proof of Theorem 17, we again find that Diagram 3.6 commutes.
In the previous example, we explicitly constructed the approximation in a way that . However, this is not strictly necessary. The following result shows that the homogenization limits would also exist and look the same as above, as long as the distributions of several geometric quantities do not worsen (significantly), with the respective conditions to be taken from [9].
Theorem 22. Let be jointly stationary sets with where pointwise as . Assume further that there exists , independent of and , such that Eq (3.8) holds for every and . Then, the assertion in Theorem 21 still holds.
Without going further into detail, we mention that our main results in form of Lemma 10 and Theorem 56 can be reproduced for any family of geometries satisfying Eqs (3.8) and (3.9) using Theorem 22.
It is currently not clear whether the Boolean model introduced above has a family of extension operators such as in the pipe example. Furthermore, if such a family does exist, then the diagram commutes. Hence, we propose the approximation method to derive an educated guess for a homogenized model.
We first establish some properties of , most importantly the minimal smoothness of .
Definition 23 (Minimal smoothness [25]). An open set is called minimally smooth with constants if we may cover by a countable sequence of open sets such that
i) .
ii) .
iii) For every , agrees (in some Cartesian system of coordinates) with the graph of a Lipschitz function whose Lipschitz semi-norm is at most .
Lemma 24 (Uniform on individual clusters). Let be an admissible point cloud. Then, for every
Proof. Let and assume for some . Then, there must be some with . This together with bounded finiteness gives such that , in particular . This contradicts the equidistance property of .
The thinning maps have been constructed just to yield the following theorem.
Theorem 25 (Minimal smoothness of thinned point clouds). For every , both and are minimally smooth with , . Furthermore, every connected component of or has diameter less than .
Proof. It remains to verify the estimate on . Let and . Then the Lipschitz constant at the intersection of the two balls and is less than .
Theorem 26 (Further properties of ). The set of admissible point clouds is measurable in the vague -algebra. Given , it holds that is measurable, is compact in the vague topology and the following three properties of are equivalent:
i) ,
ii) ,
iii) Eqs (4.1) and (4.2) hold:
(4.1) |
(4.2) |
Proof. implies since , and vice versa, implies by definition of . By construction of it follows that Eqs (4.1) and (4.2) hold if, and only if, .
Consider the space of (non-simple) counting measures , i.e.,
We see, e.g., in [2], that
● and are both measurable w.r.t. the Borel--algebra of .
● and is closed in . In particular, is also complete under the Prokhorov metric.
Now is pre-compact because of the characterization of pre-compact sets in the vague topology: For every bounded open , it holds that with depending only on . It remains to show that is closed as a subset of . Let be a converging sequence with limit . One checks that (4.1) (namely ) ensures , e.g., in a procedure similar to the proof of [2, Lemma 9.1.V]. We observe that for every , there exist such that , as . This implies by a limit in Eq (4.1) that still satisfies Eq (4.1).
For , one checks that Eq (4.1) (namely ) implies .
Let and let with sequences , . Given , let such that for all and it holds . Then there exists such that and is a Lipschitz graph in the ball for every . Hence is a Lipschitz graph in the ball . Because the Lipschitz regularity of changes continuously under slight shifts of the balls, there exists such that for and is a Lipschitz graph in . Since is arbitrary, we find is Lipschitz graph in for every , implying . Since this holds for every , we conclude Eq (4.2) and is compact.
To see that is measurable, consider for
and define
We check that is a closed subset inside (repeat the arguments above), i.e., . In particular, is measurable w.r.t. the vague topology of . Similarly, one shows that is closed as a subset inside . Again, this shows that is measurable. Consider now the measurable sets
We see that
i) if, and only if, for all , it holds .
ii) if, and only if, for every , it holds that and .
Therefore,
is measurable.
To see that is measurable, recall from Definition 3. It, therefore, suffices to show that the following maps are measurable:
For , consider the evaluation by , i.e.,
If , we observe the upper semi-continuity of
We have lower semi-continuity for since . Therefore with is measurable in the cases and and hence, in general. Since the vague topology is generated by , we conclude that and are measurable.
Remark 27 (Fine details of Theorem 26).
● For , we have that
● is not upper semi-continuous on (in contrast to ): The condition that is crucial to ensure that clusters do not change sizes.
Definition 28. We define the events that the origin is not covered by the filled-up Boolean model, i.e.,
This gives us, for , that
We will later consider the effective conductivities based on these events.
Lemma 29 (Approximation properties). Let be an admissible point cloud.
i) For every bounded domain , there exists an such that for every
ii) For every bounded domain , there exists an such that for every
iii) There exists an such that for every :
In particular, only consists of non-admissible point clouds.
Proof. i) Boundedness of implies that there are only finitely many mutually disjoint clusters , that intersect with . Furthermore, because and because of Property 1 of admissible point clouds, we know
and Lemma 24 yields
This implies the first statement.
ii) By making larger, we may assume for some . For ,
only has finitely many connected components . Take one of these connected components and suppose it lies in . Then, it has to be encircled by finitely many balls in . Let be large enough such that all these lie in . Then, . We may do so for every . Take
For every , the connected components of and are identical since . Therefore, we get the claim
iii) This is a direct consequence of Point 2. If , then for every but . Therefore, cannot be admissible by Point 2.
Definition 30 (Surface measure of ). We define the surface measure for
Note that .
Definition 31 (Intensity of random measure). Given a stationary random measure , we define its intensity
We define the intensity of point processes by identifying them as random measures.
Lemma 32 (Convergence of intensities). Let be an admissible stationary point process with finite intensity . Then,
Proof. "Almost surely" is to be understood w.r.t. the distribution of .
i) By Lemma 29, we have almost surely as . Dominated convergence with majorant yields
ii) Again, by Lemma 29, we have almost surely , in particular
Dominated convergence yields, again, convergence of intensities.
Remark 33 (Local convergence). The convergence in Lemma 29 is much stronger than what is actually needed to prove the convergence of intensities. Indeed, we could prove convergence even for so-called tame and local functions , among which the intensity is just one special case .
The structure as in Definition 8 is a dynamical system:
Definition 34 (Dynamical system, stationarity, ergodicity). Let be a separable metric probability space. A dynamical system is a family of measurable mappings satisfying
● Group property: and for any .
● Measure preserving: For any and any , we have .
● Continuity: The map , is continuous w.r.t. the product topology on .
is called ergodic if the -algebra of -invariant sets is trivial under .
Our practical setting will always be some , but we will still work with abstract dynamical systems in Sections 5 and 6. In this context, let us mention that continuity of was not needed in the first place [22], but turned out to be very useful in the proofs concerning two-scale convergence [30]. Since we frequently use results from [30] and since we get continuity of for free in our applied setting, we will simply rely on this property.
Let be a dynamical system. We write . The dynamical system introduces a strongly continuous group action on through with the independent generators
with domain where is the canonical Euclidean basis. Introducing
and the gradient , we can define the space of potential vector fields
Defining , we find with that
(5.1) |
because is a separable metric [8] where
For measurable, we define
Definition 35 (Cell solutions). Let be a separable metric probability space with dynamical system and let . We notice that for every unit vector , the map
is a bounded linear functional on the Hilbert space . Using the Riesz representation theorem, we obtain a unique such that or equivalently
is called the -th cell solution. The cell solutions satisfy
and can be grouped in the matrix
Remark 36. We observe that by definition, is the minimizer in of the functional
(5.2) |
Definition 37 (Effective conductivity ). Let be the cell solution on . The effective conductivity based on the event is defined as
(5.3) |
with being the identity matrix. We observe for the entries of that
(5.4) |
We write for its smallest eigenvalue.
Lemma 38 (Convergence of cell solutions). Let and such that -almost everywhere as and
● either for every , or
● there exist , independent from and continuous operators such that, for every and every , it holds that .
Then, the sequence of cell solutions to the cell problem on satisfies
where is the -th cell solution on
Proof. We first check that the limit satisfies and then .
i) In both cases, the a priori estimate yields an -weakly convergent subsequence of after extending to the whole of via 0. Let . We have -almost everywhere, so dominated convergence yields
while weak convergence yields
We also have
which implies
Therefore, with :
ii) In both cases of the lemma, the space is closed and convex, so it is also weakly closed. We construct a weakly converging sequence in that converges to . Since , we find such that
Since , we get
Note that is a bounded sequence that is weakly converging to because for every we find by dominated convergence that strongly in . Therefore, we also obtain
In the first case of the lemma, , so we get that . In the second case, we obtain and hence . Since being a -function is distinguished from being a pure -function only by the Condition (5.1), the integrability of then yields .
Corollary 39 (Convergence of effective conductivities). Let and such that -almost surely and
● either for every , or
● there exist , independent from and continuous operators such that, for every and every , it holds that .
Let be the effective conductivity of and be the effective conductivity of . Then,
Proof. This follows from Eq (5.4) and weak convergence .
Remark 40 (Variational formulation). There is another way to define : For , (see Definition 35) is the unique minimizer to
and therefore
This equality is related to Theorem 57.
We will prove both theorems at once. The first part follows using the weak formulation, weak convergence of both and as as well as standard PDE arguments. The second part is proved in [30] using our notation and also before in [28]. The convergence of in Part three is given by Corollary 39. Hence, it only remains to prove the convergence of .
When it comes to the latter, we have to distinguish between three cases:
i) There exists such that, for every and every , it holds
Then, the proof is straightforward using the resulting uniform estimates on .
ii) : Then, convergence follows after assuming that is uniformly bounded as well as testing with . This will eventually lead to an estimate
Afterward, we may use an approximation argument for bounded in using a standard mollifier: with . Then,
iii) along some . Then, these form a linear subspace and we may restrict our testing functions to a dependence in the orthogonal direction, only. More precisely, we can consider testing functions with support concentrated on the orthogonal plane to as .
In Section 6, we will use two-scale convergence to homogenize Eq (1.1) for fixed . This process is more convenient to handle if the underlying probability space is compact. Here we show that we may take as the underlying probability space instead of .
Lemma 41. Let be a distribution on and let with the push-forward measure . Recall and let . Let be the cell solutions on and the cell solutions on for their respective dynamical systems. Then, for every , it holds that
(5.5) |
Lemma 42 (Properties of pull-back functions). Let , be dynamical systems, measurable such that and such that for every
(5.6) |
Then, the following holds: For every , we have with . If , then . If , then . is called the pull-back of .
Proof. Due to , we immediately obtain for arbitrary measurable and its pull-back
(5.7) |
Therefore and (5.6) yields . For , follows from and checking
for -almost every and every on a bounded domain .
Proof of Lemma 41. We use Lemma 42 for with , where is the shift-operator on and is the Borel--algebra generated by the vague topology, and again with shift-operator and Borel--algebra. Let be the pull-back of according to Lemma 42. We see , so has support in . Let with . The pull-back of satisfies and hence, . We observe with the pull-back . This implies . Eq (5.7) yields Eq (5.5).
We first collect all the tools needed to prove the homogenization result for minimally smooth domains (Lemma 10).
Theorem 43 (Extending beyond holes and trace operator). There exists a constant depending only on and such that the following holds: Assume that is a bounded Lipschitz domain with Lipschitz constant , and such that for every it holds . Then, there exists an extension operator
such that and
(6.1) |
Furthermore, there exists a trace operator
such that for every and
(6.2) |
Proof. For every with , the set is minimally smooth with and, . Furthermore, the connected components of have a diameter less than . The existence of satisfying Eq (6.1) follows from [5, Lemma 2.4] (actually, this was pointed out before by [7, Section 3], but the implications there are not obvious). The existence of satisfying Eq (6.2) is provided in [9].
Definition 44 (Stationary and ergodic random measures). A random measure with underlying dynamical system is called stationary if
for every measurable , , and -almost every . is called ergodic if it is stationary and is ergodic.
Definition 44 is compatible with Definition 8 given in Section 3 by considering the canonical underlying probability space with being the distribution of .
Theorem 45 (Palm theorem (for finite intensity) [17]). Let be a stationary random measure with underlying dynamical system of finite intensity .
Then, there exists a unique finite measure on such that for every measurable and either or :
For arbitrary with , we have that
in particular, . Furthermore, for every and , the ergodic limit
(6.3) |
holds for -almost every . We call the Palm measure of .
For the rest of this subsection, we use the following assumptions.
Assumption 46. is a compact metric space with a probability measure and continuous dynamical system . Furthermore, is a stationary ergodic random measure with Palm measure . We define .
According to [30] (by an application of Eq (6.3)), almost every is typical, i.e., for such an , it holds for every that
Definition 47 (Two-scale convergence). Let Assumption 46 hold and let be typical. Let be a sequence and let such that
and such that for every ,
(6.4) |
Then, is said to be (weakly) two-scale convergent to , written .
Remark 48 (Extending the space of test functions).
● For , let . The original proof of the following Lemma 49 in [30] shows that we can equally define two-scale convergence additionally claiming Eq (6.4) has to hold for every and , where . In particular, given a fixed , we can w.l.o.g. say that two-scale convergence of implies .
● Using a standard approximation argument, we can extend the class of test functions from to , provided is uniformly continuous w.r.t. the Lebesgue measure. Then, strong -convergence implies two-scale convergence for .
Lemma 49 ([30, Lemma 5.1]). Let Assumption 46 hold. Let be typical and be a sequence of functions such that for some independent of . Then, there exists a subsequence of and such that and
(6.5) |
Theorem 50 (Two-scale convergence in [30]). Under Assumption 46, for every typical the following holds: If for all and if
then, there exists a with weakly in and there exists such that weakly in two scales.
Due to Theorem 26, the set is compact, hence the above two-scale convergence method can be applied for the stationary ergodic point process taking values in only. To be more precise, we consider the compact metric probability space and a random variable such that and have the same distribution. By the considerations made in Subsection 5.4, they will both result in the same partial differential equation.
Theorem 51 (Extension and trace estimates on for ). Let be a bounded domain, be fixed. Let be an admissible point process with values in . For almost every realization of , we have: Let and be defined according to Definition 4.
i) There exists a depending only on and and a family of extension and trace operators
such that for every it holds
ii) If is a sequence satisfying , then, there exists a and a subsequence still indexed by such that weakly in and there exists such that
where . Furthermore, for some depending only on and
(6.6) |
Proof. i) follows from using Theorem 43 on and rescaling the inequalities (6.1) and (6.2).
ii) is a bit more lengthy. The existence of a subsequence and and such that and follows from Theorem 50. We observe that and . Therefore, (Remark 48(i)). Furthermore, we observe with such that we have and
Therefore, strongly in , , and hence, taking any arbitrary , , we find
which means that and have the same two-scale limit
Due to the absolutely bounded diameter of the connected components of , there exists a domain big enough such that, with the notation of Definition 4,
Now let be the canonical extension operator satisfying
Reapplying Theorem 43 to the trace on , we find for some constant independent from and but depending on , , and and varying from line to line:
as desired.
Due to the perforations, cannot be embedded in a common space in a convenient way for the application of the Aubin–Lions theorem. Hence, we use the following general characterization of compact sets.
Theorem 52 (Characterization of compact sets in [24, Theorem 1]). Let be a Banach space, and . is relatively compact in if, and only if,
(6.7) |
(6.8) |
where is the shift by .
We can now establish the existence of a solution for fixed to our partial differential equation.
Theorem 53 (Existence of solution on perforated domains and a priori estimate). Let . Under Assumption 6 and with as defined in Definition 4, we have that there exists a solution , with generalized time derivative , to Eq (1.1), i.e.,
(6.9) |
which satisfies the following a priori estimates for small enough
(6.10) |
where
is from Theorem 51 depending only on and and where is independent of .
Proof. We will only sketch the proof. There are 3 main steps: Deriving a priori estimates, existence of Galerkin solutions and the limit passing.
i) Testing Eq (6.9) with and using
yields
The a priori estimate then follows from the Gronwall inequality and the trace estimate in Theorem 51. For the a priori estimate in , one simply uses
ii) Let be a family of finite-dimensional vector spaces, . Solutions to Eq (1.1) exist in , that is,
where is the orthogonal projection. This can be shown via, e.g., [21, Theorem 3.7] which yields solutions for some . In this reference, their corresponds to the parameters of the Galerkin approximation, i.e., with being fixed orthonormal base vectors. These solutions satisfy the a priori estimate in Eq (6.10) as well as
(6.11) |
in particular, we may extend the solution to the whole time interval (with still uniform bounds in ).
iii) The a priori estimates yield a -weakly convergent subsequence to some . Theorem 52 and Eq (6.11) imply pre-compactness of as well as pre-compactness of , see Remark 54. Testing with functions in and passing to the limit finishes the proof since is dense in .
Remark 54 (Procedure of Simon's theorem). We will use Simon's theorem (Theorem 52) on multiple occasions. The general procedure will always be the same. We will exemplaily prove the following result: Let , be some bounded Lipschitz-domain and the trace operator. For each , let with generalized time-derivative via . Assume that
for either the situation that with and uniformly continuous injective maps or for the situation that . We further claim for almost every . Then,
are relatively compact.
Exemplarily proof for the procedure of Simon's theorem. We need to show Conditions (6.7) and (6.8) from Theorem 52.
i) Condition (6.7) usually relies on compactness results for the stationary setting. Since
compactness of yields pre-compactness of , so we have shown Condition (6.7).
ii) Condition (6.8) will additionally require some a priori estimate on . We have
as elements of . Using the Cauchy–Schwarz inequality twice, we get for :
Compactness of implies that for every , there exists a such that
Therefore,
The estimate is independent of the chosen , and Condition (6.8) holds.
We have shown both conditions and concluded.
We can now pass to the limit for the homogenized system. Some extra care has to be taken since , especially in the boundary term. However, we show that the difference becomes negligible for the two-scale convergence as .
Theorem 55 (Homogenized system for ). Let be a stationary ergodic point process with values in . Recall the surface measure from Definition 30
Under Assumption 6, we have for almost every realization of and with as defined in Definition 4: Let be a solution to Eq (6.9) and let be given as in Theorem 51. There exists a with generalized time derivative such that for a subsequence
and is a (not necessarily unique) solution to
(6.12) |
with being the effective conductivity based on the event defined in Definition 37. Furthermore, satisfies the following a priori estimates
for
Proof. The a priori estimates in Eq (6.10) and Theorem 51 tell us that
for some and where the two-scale convergence is with respect to the Lebesgue measure . The uniform bound for in Eq (6.10) together with Theorem 52 yields (for yet another subsequence)
(6.13) |
compared to, e.g., Remark 54.
For with and with , we use as a test function and pass to the limit using two-scale convergence. Furthermore, we use
(6.14) |
which we will prove below. We then obtain the two equations
(6.15) |
(6.16) |
The second equation holds true for every choice of and as above if we make the standard ansatz (see the remark following this proof), where are the cell solutions from Definition 35 for and being the distribution of . Plugging this information into the first equation yields (6.12). The a priori estimate follows from testing Eq (6.12) with and the Gronwall inequality (see, e.g., the proof of Theorem 53). It only remains to prove Eq (6.14).
Now, we show the first part of Eq (6.14). By Remark 48, we know that
Using dominated convergence and Eq (6.13) yields a subsequence such that in for every . Using test functions and , we observe that , so .
The second part of Eq (6.14) is more difficult. Given and , we set and find
We will show that all these terms go to as . Due to the Lipschitz continuity of and Stampacchia's lemma, we find
Furthermore, strongly in and weakly in implies that in the same topologies. as in Definition 4 fulfills . Eq (6.6) together with the strong convergence of tells us
which already shows convergence in the first summand. Similar considerations to the proof of Eq (6.6) tell us that is a bounded linear operator, so we can consider the trace not only on but even for clusters close to the boundary. We have, with changing from line to line but independent of ,
where
We observe that as , i.e., point-wise -almost everywhere. We know that strongly in , so dominated convergence yields that the second summand also converges to .
The third summand follows from two-scale convergence, i.e., for almost every .
Remark (On the special solution of Eq (6.16)). Our special ansatz as a solution to Eq (6.16) for a fixed, given has a long tradition, where we mention [5] for perforated domains or the more "historical" papers [22,30]. For readers familiar with periodic homogenization, let us mention that due to being problem (6.16) is the probabilistic equivalent of
Now given a , we are looking for a solution to
for arbitrary and with . Since functions of the form are dense in for with (by definition of ), this equation actually has to hold for arbitrary . By the fundamental lemma of calculus of variations, this solution must satisfy for almost every and almost every
Next, we see that is indeed a solution since the are cell solutions
On the other hand, such a solution is unique: First, observe that functions of the form are dense in . Then, we consider the coercive bilinear form on :
Lax–Milgram tells us that the solution to
is unique, which is equivalent to
which justifies the standard ansatz.
Theorem 17 is a consequence of the following.
Theorem 56 (Main theorem: homogenized limit of admissible point processes). Let be a stationary ergodic admissible point process with distribution such that is statistically connected. Under Assumption 6, let be a homogenized solution from Theorem 55 for the thinned point process .
For any subsequence of , we are able to extract yet another subsequence that converges to a . This is a (not necessarily unique) weak solution to the initial value problem
Here is the effective conductivity defined in Definition 37 based on the event , , and is the intensity of . Furthermore, with being the smallest eigenvalue of and being the Lipschitz constant of
where
Proof. We note that from Theorem 55 is defined with cell solutions on and the push-forward measure . We use the pull-back result from Lemma 41 to obtain a representation of in terms of and the original probability distribution.
Lemmas 32 and 29 and Corollary 39 yield respectively
for and . From the a priori estimates in Theorem 55, we furthermore find
and Aubin–Lions (or more general, Theorem 52) yields pre-compactness. These uniform bounds together with compactness arguments yield the existence of with generalized time derivative , such that for a subsequence
as well as
From here we conclude.
In this chapter, we will establish a criterion for to be statistically connected (Definition 15), that is Theorem 60. To be precise, we will show that
as all other directions can be shown analogously via rotation. The procedure will be based on [13, Chapter 9]. The matrix corresponds to the matrix there. We will also see that is statistically connected if is statistically connected.
Notation. Given a fixed admissible point process , we write in this section
Most arguments work for more general random perforations and their filled-up versions as long as has no infinite connected component (Theorem 58 needs additionally that almost surely, the bounded connected components of have non-zero distance to the infinite connected components). We refrain from doing so since we would need to introduce the notion of stationary random sets and the main focus here lies on point processes.
The following theorem gives us a different point of view on the effective conductivity :
Theorem 57 (Variational formulation [13, Theorem 9.1]). For every ergodic admissible point process, we have almost surely and for every :
where is the effective conductivity based on the event .
The first observation we can make is that the effective conductivity depends monotonously on the domain: The larger the set of holes, the lower the effective conductivity. The question arises in which cases this term becomes 0. This should only happen if is "insufficiently connected". Intuitively, we want , but at the same time, needs to be at the boundaries. If our region is badly connected, we can hide large gradients inside the holes, see, e.g., Figure 3. As in [13], we will see that the existence of sufficiently many "channels" connecting the left to the right side of a box will ensure . Before we do that, we establish an important fact:
We have defined statistical connectedness (Definition 15) via the filled-up Boolean model . Unfortunately, filling up holes is non-local (depending on the size of holes), which is troublesome on the stochastic side. However, an analogue of [13, Lemma 9.7] tells us that the effective conductivity of both the Boolean model and its filled-up version are the same.
Theorem 58 (Filling up holes preserves the effective conductivity). For every ergodic admissible point process, we have almost surely
where the infimum is over .
Proof. As mentioned before, this is a variation of [13, Lemma 9.7] fitted to our purpose. Let
● be the set of islands (i.e., connected components in of finite diameter) of diameter that intersect but do not lie inside .
● be the set of islands of diameter that do not completely lie inside and that are encircled by a -cluster of size larger than .
All the islands in and belong to connected components of different from (the unique unbounded connected component). Since is admissible, almost surely they all have non-zero distance to . Therefore, the following infimum decomposes, with all the infima being over
with
and where the second equality comes from the fact that filling up islands that lie completely inside does not change the value of the infimum. Now, we observe
● for fixed , so and
● denoting by all islands of diameter greater than , we have almost surely
All islands are of finite size, yielding . Hence, .
Choosing sufficiently large finishes the proof.
Definition 59 (Percolation channels (see Figure 4)). Fix a . We consider the lattice and the cube with corner
and call two vertices neighbors if their -distance is equal to .
We call open if
An open left-right crossing of is called a percolation channel in , i.e.,
i) are neighbors for each ,
ii) all the are open, and
iii) and .
We define the quantity (depending on the random and on )
and the tube corresponding to the path as
Statistical connectedness of then reads as follows:
Theorem 60 (Percolation channels imply conductivity). For almost every realization of an ergodic admissible point process, we have for
In particular, the effective conductivity is strictly positive if almost surely
(8.1) |
Proof. This is an analogue of [13, Theorem 9.11] and relies on defining a suitable vector field inside channels on . We want to satisfy the following
● for every inside the tube and outside.
● is parallel to except on corners as well as and .
● for .
● For the standard normal vector to :
(8.2) |
Thus, the vector field was deliberately chosen such that the Gauß divergence theorem only yields contributions from the "starting" and "ending" surfaces. Figure 5 illustrates how can be chosen to satisfy these properties.
The rest is simple. Take disjoint nonself-intersecting channels in . Set
Then,
For a fixed tube , we have
Therefore,
and so
Passing to the finishes the proof.
Remark 61 ( and bottom-top crossings). Let be the minimal number of open vertices that a -bottom-top crossing of must have. It turns out that in
(see Lemma 73). We will use this to show Eq (8.1) for the Poisson point process .
The driving force behind this work has been a stationary Poisson point process . It is known that the Poisson point process is ergodic (even mixing) and its high spatial independence makes it the canonical random point process. As pointed out before though, gives rise to numerous analytical issues which prevent the usage of the usual homogenization tools.
The main theorem (Theorem 56) tells us that homogenization is still reasonable for highly irregular filled-up Boolean models driven by admissible point processes .
It is known for that there exists some critical radius such that
● only consists of finite clusters for (subcritical regime) and
● has a unique infinite cluster for (supercritical regime).
The behavior at criticality is still a point of research. For details, we refer to [14] for the Poisson point process and [18, Chapter 3] for the Boolean model .
We will see in the subcritical regime that
i) is an ergodic admissible point process and
ii) is statistically connected, which is equivalent to being statistically connected (see Theorem 58).
We therefore make the following assumption for the rest of this section:
Assumption 62 (subcritical regime). We assume that
Remark 63 (Scaling relation). has the following scaling relation
The Mecke–Slivnyak theorem tells us that the Palm probability measure (Theorem 45) of a stationary Poisson point process is just a Poisson point process with a point added in the origin. This gives us the following lemma:
Lemma 64 (Equidistance property). The stationary Poisson point process satisfies the equidistance property for arbitrary , i.e.,
Proof. This follows from using the Palm theorem Theorem 45 on
and the Mecke–Slivnyak theorem [14, Theorem 9.4].
Corollary 65 ( is admissible). Under Assumption 62, is an admissible point process.
Proof. is not just ergodic, but even mixing (see [14, Theorem 8.13]). The equidistance property has been proven in Corollary 64. Finiteness of clusters follows from the subcritical regime (Assumption 62).
Proving the statistical connectedness of (Definition 15) is much harder and does not immediately follow from readily available results. Our procedure is as follows:
i) We employ the criterion from Section 8. Therefore, we will check that there are sufficiently many percolation channels for .
ii) Using the spatial independence of the Poisson point process , we show that it is sufficient to only consider -dimensional slices.
iii) We show the statement in using ideas in [11, Chapter 11]. There, the result has been proven for certain iid fields on planar graphs, including .
Additionally to Assumption 62, we need sufficient discretization for the percolation channels:
Assumption 66 (Sufficient scaling). Let be large enough such that, for the critical radius ,
e.g., .
Definition 67 (Recap and random field ). Recall Definition 59, most importantly
as well as the notion of percolation channels for and
We define the random field
We say that is blocked if and open if (this is consistent with Definition 59).
Theorem 68 (Percolation channels of the Poisson point process). Under Assumptions 62 and 66, there is a such that Eq (8.1) holds, i.e.,
In particular, is statistically connected (see Theorem 60).
The rest of the section deals with the proof of Theorem 68. It will follow as a direct consequence of Lemma 70 (reduction to ) and Lemma 74 (main result for ) which are given later.
For disjoint and events only depending on inside , we know that is an independent family. This is one of the striking properties of a Poisson point process and we will heavily make use of it. The Boolean model for radius still retains this property in a slightly weaker form and correspondingly the random field :
Lemma 69 (Independence in large distances). Let such that
(9.1) |
Then, and are independent.
Proof. is only affected by points of inside
The same holds for and we check that Eq (9.1) implies .
Lemma 70 (-dimensional percolation channels imply channel property for ). For , we define (compare to Definition 59)
If there are such that for some ,
(9.2) |
then there exists a such that
(This proof heavily relies on the independence structure of the Poisson point process, i.e., Lemma 69.)
Proof. is stationary, so for distinct ,
Let . By Lemma 69, the events on are independent from the events on . Therefore, is an iid family of Bernoulli random variables with parameter . Then,
By Eq (9.2) and the law of large numbers, we get
Setting , we obtain Eq (8.1) after checking
which finishes the proof.
Remark 71. Spatial independence is needed to move from to . The strong independence properties of allow far weaker conditions on (positive probability) than on (probability 1). Either way, Lemma 74 shows that drops exponentially in .
As shown before, we may limit ourselves to a fixed lattice . Therefore, we will often suppress the "anchor point" and just act like we are in . Our random field from Definition 67 is then by abuse of notation
Definition 72 (Vertical crossings). Consider the -lattice, that is are neighbors if .
An -bottom-top crossing in is called a vertical crossing. We call a path blocked if all its vertices are blocked. We define the quantity
(The percolation channels lie on the -graph, while the vertical crossings lie on the -graph.)
We may work with single vertical crossings instead of collections of percolation channels:
Lemma 73 (Percolation channels vs vertical crossings (see Figure 6)). It holds that
Proof. See the proof of [11, Theorem 11.1] based on Menger's theorem and [11, Proposition 2.2].
The main work is proving the following equivalent of [11, Proposition 11.1]:
Lemma 74 (Open vertices in vertical crossings). Under Assumptions 62 and 66, there are such that
in particular,
The proof relies on a reduction scheme of the path . We divide into several segments which must either contain an open vertex or contain a blocked path of large diameter. Since we are in the subcritical regime, the probability of such paths decreases exponentially in their diameter:
Lemma 75 (Diameter of blocked paths). Let . Under Assumption 62 and as in Assumption 66, there are such that
where
Proof. Consider the Boolean model for radius , i.e.,
Let be a blocked path in containing with diameter . Since is blocked and (Assumption 66), we find for every some such that
and therefore
Connecting all the by a straight line, we obtain a continuous path inside . In particular, they all belong to the same -cluster. Then,
since the occurrence of large clusters drops exponentially in the diameter ([18, Lemma 2.4]).
Let . As pointed out before, we follow the procedure in [11, Proposition 11.1] adjusted to the continuum setting. We define for and as
The idea is to break up the path into multiple segments (see Figure 7). In each segment, we can either reduce by or employ Lemma 75. We set
These boxes are defined so that the following holds: For fixed , we have by Lemma 69 that the random variables and are independent. That means the state of the vertices in is independent from the state of the vertices in . Additionally, we define the probability
The key inequality for the iteration in is the following
(9.3) |
for whenever .
Proof of Eq (9.3). Consider the event that for some , we find a path that has at most open vertices, i.e.,
Now assume that the event happens. Take a path with and at most of the being open. Let be the last index with . This exists since , so has to pass by to reach . For this , we know that completely lies in . Since does not happen, it must have open vertices. is a path from to that is blocked everywhere except its end. Therefore,
As previously mentioned, the events in and are independent from each other. This gives us
Now,
concludes the proof of Eq (9.3).
Observe that the reduction in can only happen until , so more -terms have to show up at some point. Since any path has diameter of at least , Lemma 75 tells us that for independent of and . Choose large such that
For simplicity, we introduce
and rewrite Eq (9.3) into
(9.4) |
We now iteratively use Eq (9.4) up to times as it is only applicable when . All the are summed over and all the over .
We iterate as long as ; otherwise, we stop for and land in the second summand. Only matters since whenever . Also, observe that can only happen if
since we "gain" at most to the second component in each .
Let be large enough such that
Then,
Since was arbitrary, we get
Now we finally make use of . Setting and , the claim follows:
All results of the publication have resulted from joint discussions. They can therefore not be attributed separately to the authors, but are to be regarded as joint achievements.
The authors declare they have not used Artificial Intelligence (AI) tools in the creation of this article.
The authors thank the two anonymous reviewers for their valuable comments that helped to improve the manuscript. BJ and ADV received support from the Leibniz Association within the Leibniz Junior Research Group on Probabilistic Methods for Dynamic Communication Networks as part of the Leibniz Competition (grant no. J105/2020).
The authors declare there is no conflict of interest.
[1] |
G. Allaire, Homogenization and two-scale convergence, SIAM J. Math. Anal., 23 (1992), 1482–1518. https://doi.org/10.1137/0523084 doi: 10.1137/0523084
![]() |
[2] | D. J. Daley, D. Vere-Jones, An Introduction to the Theory of Point Processes, Volume II: General Theory and Structure, Springer Science & Business Media, New York, 2008. |
[3] |
A. Faggionato, Random walks and exclusion processes among random conductances on random infinite clusters: Homogenization and hydrodynamic limit, Electron. J. Probab., 13 (2008), 2217–2247. https://doi.org/10.1214/EJP.v13-591 doi: 10.1214/EJP.v13-591
![]() |
[4] |
F. Flegel, M. Heida, M. Slowik, Homogenization theory for the random conductance model with degenerate ergodic weights and unbounded-range jumps, Ann. Inst. H. Poincaré Probab. Statist., 55 (2019), 1226–1257. https://doi.org/10.1214/18-aihp917 doi: 10.1214/18-aihp917
![]() |
[5] |
B. Franchi, M. Heida, S. Lorenzani, A mathematical model for Alzheimer's disease: An approach via stochastic homogenization of the Smoluchowski equation, Commun. Math. Sci., 18 (2020), 1105–1134. https://doi.org/10.4310/CMS.2020.v18.n4.a10 doi: 10.4310/CMS.2020.v18.n4.a10
![]() |
[6] |
A. Giunti, R. M. Höfer, Convergence of the pressure in the homogenization of the Stokes equations in randomly perforated domains, J. Differ. Equ., 320 (2022), 399–418. https://doi.org/10.1016/j.jde.2022.03.003 doi: 10.1016/j.jde.2022.03.003
![]() |
[7] |
N. Guillen, I. Kim, Quasistatic droplets in randomly perforated domains, Arch. Ration. Mech. Anal., 215 (2015), 211–281. https://doi.org/10.1007/s00205-014-0777-2 doi: 10.1007/s00205-014-0777-2
![]() |
[8] | M. Heida, Precompact probability spaces in applied stochastic homogenization, 2021. https://doi.org/10.20347/WIAS.PREPRINT.2852 |
[9] |
M. Heida, Stochastic homogenization on perforated domains I–Extension operators, Networks Heterogen. Media, 18 (2023), 1820–1897. https://doi.org/10.3934/nhm.2023079 doi: 10.3934/nhm.2023079
![]() |
[10] |
M. Heida, S. Neukamm, M. Varga, Stochastic homogenization of -convex gradient flows, Discrete Contin. Dyn. Syst. - Ser. S, 14 (2021), 427–453. https://doi.org/10.3934/dcdss.2020328 doi: 10.3934/dcdss.2020328
![]() |
[11] | H. Kesten, Percolation Theory for Mathematicians, Springer, Boston: Birkhäuser, 1982. https://doi.org/10.1007/978-1-4899-2730-9 |
[12] |
S. M. Kozlov, Averaging of random operators, Sbornik Math., 151 (1979), 188–202. https://doi.org/10.1070/SM1980v037n02ABEH001948 doi: 10.1070/SM1980v037n02ABEH001948
![]() |
[13] | S. M. Kozlov, O. A. Oleinik, V. V. Zhikov, Homogenization of Differential Operators and Integral Functionals, Springer Science & Business Media, Germany, 1994. https://doi.org/10.1007/978-3-642-84659-5 |
[14] | G. Last, M. Penrose, Lectures on the Poisson process, Cambridge University Press, UK, 7 (2017). |
[15] | B. Matérn, Spatial variation, Springer-Verlag, Berlin, 36 (1986), 151. https://doi.org/10.1007/978-1-4615-7892-5 |
[16] |
P. Mathieu, A. Piatnitski, Quenched invariance principles for random walks on percolation clusters, Proc. R. Soc. A, 463 (2007), 2287–2307. https://doi.org/10.1098/rspa.2007.1876 doi: 10.1098/rspa.2007.1876
![]() |
[17] |
J. Mecke, Stationäre zufällige Maße auf lokalkompakten abelschen Gruppen, Z. Wahrscheinlichkeitstheorie verw. Geb., 9 (1967), 36–58. https://doi.org/10.1007/BF00535466 doi: 10.1007/BF00535466
![]() |
[18] | R. Meester, R. Roy, Continuum Percolation, Cambridge University Press, UK, 119 (1996). |
[19] |
S. Neukamm, M. Varga, Stochastic unfolding and homogenization of spring network models, Multiscale Model. Simul., 16 (2018), 857–899. https://doi.org/10.1137/17M1141230 doi: 10.1137/17M1141230
![]() |
[20] |
G. Nguetseng, A general convergence result for a functional related to the theory of homogenization, SIAM J. Math. Anal., 20 (1989), 608–623. https://doi.org/10.1137/0520043 doi: 10.1137/0520043
![]() |
[21] | D. O'Regan, Existence Theory for Nonlinear Ordinary Differential Equations, Kluwer Academic Publishers Group, Dordrecht, 398 (1997). https://doi.org/10.1007/978-94-017-1517-1 |
[22] | G. C. Papanicolaou, S. R. S. Varadhan, Boundary value problems with rapidly oscillating random coefficients, Colloq. Math. Soc. János Bolyai, 27 (1981), 835–873. |
[23] |
A. Piatnitski, M. Ptashnyk, Homogenization of biomechanical models of plant tissues with randomly distributed cells, Nonlinearity, 33 (2020), 5510–5542. https://doi.org/10.1088/1361-6544/ab95ab doi: 10.1088/1361-6544/ab95ab
![]() |
[24] |
J. Simon, Compact sets in the space , Ann. Mat. Pura Appl., 146 (1986), 65–96. https://doi.org/10.1007/BF01762360 doi: 10.1007/BF01762360
![]() |
[25] | E. M. Stein, Singular Integrals and Differentiability Properties of Functions, Princeton university press, New Jersey, USA, 30 (1970). |
[26] | D. Stoyan, W. S. Kendall, J. Mecke, Stochastic Geometry and Its Applications, John Wiley & Sons, Hoboken, New Jersey, 1987. |
[27] |
S. Wright, A. Mikelic, A. Bourgeat, Stochastic two-scale convergence in the mean and applications, J. Reine Angew. Math., 456 (1994), 19–51. https://doi.org/10.1515/crll.1994.456.19 doi: 10.1515/crll.1994.456.19
![]() |
[28] |
V. V. Zhikov, Averaging in perforated random domains of general type, Math. Notes, 53 (1993), 30–42. https://doi.org/10.1007/BF01208520 doi: 10.1007/BF01208520
![]() |
[29] |
V. V. Zhikov, On an extension of the method of two-scale convergence and its applications, Sbornik Math., 191 (2000), 973–1014. https://doi.org/10.1070/SM2000v191n07ABEH000491 doi: 10.1070/SM2000v191n07ABEH000491
![]() |
[30] |
V. V. Zhikov, A. L. Pyatniskii, Homogenization of random singular structures and random measures, Izv. Math., 70 (2006), 19–67. https://doi.org/10.1070/IM2006v070n01ABEH002302 doi: 10.1070/IM2006v070n01ABEH002302
![]() |