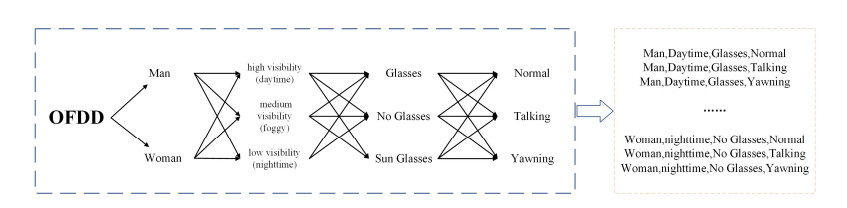
A modest review on stability investigations of numerical methods for systems of Itô-interpreted stochastic differential equations (SDEs) driven by m-dimensional Wiener processes W=(W1,W2,...,Wm) is presented in Rd. Since the problem of relevance of 1D test equations for multidimensional numerical methods has not completely been solved so far, we suggest to use the Krein-Perron-Frobenius theory of positive operators on positive cones of Rd×d, instead of classic stability functions with values in C1, which is only relevant for the very restricted case of "simultaneously diagonalizable" SDEs. Our main focus is put on the concept of asymptotic mean square and almost sure (a.s.) stability for systems with state-dependent noise (multiplicative case), and the concept of exact preservation of asymptotic probabilistic quantities for systems with state-independent noise (additive case). The asymptotic exactness of midpoint methods with any equidistant step size h is worked out in order to underline their superiority within the class of all drift-implicit, classic theta methods for multidimensional, bilinear systems of SDEs. Balanced implicit methods with appropriate weights can also provide a.s. exact, asymptotically stable numerical methods for pure diffusions. The review on numerical stability is based on "major breakthroughs" of research for systems of SDEs over the last 35 years, with emphasis on applicability to all dimensions d≥1.
Citation: Henri Schurz. A brief review on stability investigations of numerical methods for systems of stochastic differential equations[J]. Networks and Heterogeneous Media, 2024, 19(1): 355-383. doi: 10.3934/nhm.2024016
[1] | Yuhan Su, Shaoping Shen . Adaptive predefined-time prescribed performance control for spacecraft systems. Mathematical Biosciences and Engineering, 2023, 20(3): 5921-5948. doi: 10.3934/mbe.2023256 |
[2] | Na Zhang, Jianwei Xia, Tianjiao Liu, Chengyuan Yan, Xiao Wang . Dynamic event-triggered adaptive finite-time consensus control for multi-agent systems with time-varying actuator faults. Mathematical Biosciences and Engineering, 2023, 20(5): 7761-7783. doi: 10.3934/mbe.2023335 |
[3] | Filippo Cacace, Valerio Cusimano, Alfredo Germani, Pasquale Palumbo, Federico Papa . Closed-loop control of tumor growth by means of anti-angiogenic administration. Mathematical Biosciences and Engineering, 2018, 15(4): 827-839. doi: 10.3934/mbe.2018037 |
[4] | Su-na Zhao, Yingxue Cui, Yan He, Zhendong He, Zhihua Diao, Fang Peng, Chao Cheng . Teleoperation control of a wheeled mobile robot based on Brain-machine Interface. Mathematical Biosciences and Engineering, 2023, 20(2): 3638-3660. doi: 10.3934/mbe.2023170 |
[5] | Yixin Zhuo, Ling Li, Jian Tang, Wenchuan Meng, Zhanhong Huang, Kui Huang, Jiaqiu Hu, Yiming Qin, Houjian Zhan, Zhencheng Liang . Optimal real-time power dispatch of power grid with wind energy forecasting under extreme weather. Mathematical Biosciences and Engineering, 2023, 20(8): 14353-14376. doi: 10.3934/mbe.2023642 |
[6] | Balázs Csutak, Gábor Szederkényi . Robust control and data reconstruction for nonlinear epidemiological models using feedback linearization and state estimation. Mathematical Biosciences and Engineering, 2025, 22(1): 109-137. doi: 10.3934/mbe.2025006 |
[7] | Junyoung Jang, Kihoon Jang, Hee-Dae Kwon, Jeehyun Lee . Feedback control of an HBV model based on ensemble kalman filter and differential evolution. Mathematical Biosciences and Engineering, 2018, 15(3): 667-691. doi: 10.3934/mbe.2018030 |
[8] | Fang Zhu, Wei Liu . A novel medical image fusion method based on multi-scale shearing rolling weighted guided image filter. Mathematical Biosciences and Engineering, 2023, 20(8): 15374-15406. doi: 10.3934/mbe.2023687 |
[9] | Jingya Wang, Ye Zhu . L2−L∞ control for memristive NNs with non-necessarily differentiable time-varying delay. Mathematical Biosciences and Engineering, 2023, 20(7): 13182-13199. doi: 10.3934/mbe.2023588 |
[10] | Jiabao Gu, Hui Wang, Wuquan Li . Output-Feedback stabilization for stochastic nonlinear systems with Markovian switching and time-varying powers. Mathematical Biosciences and Engineering, 2022, 19(11): 11071-11085. doi: 10.3934/mbe.2022516 |
A modest review on stability investigations of numerical methods for systems of Itô-interpreted stochastic differential equations (SDEs) driven by m-dimensional Wiener processes W=(W1,W2,...,Wm) is presented in Rd. Since the problem of relevance of 1D test equations for multidimensional numerical methods has not completely been solved so far, we suggest to use the Krein-Perron-Frobenius theory of positive operators on positive cones of Rd×d, instead of classic stability functions with values in C1, which is only relevant for the very restricted case of "simultaneously diagonalizable" SDEs. Our main focus is put on the concept of asymptotic mean square and almost sure (a.s.) stability for systems with state-dependent noise (multiplicative case), and the concept of exact preservation of asymptotic probabilistic quantities for systems with state-independent noise (additive case). The asymptotic exactness of midpoint methods with any equidistant step size h is worked out in order to underline their superiority within the class of all drift-implicit, classic theta methods for multidimensional, bilinear systems of SDEs. Balanced implicit methods with appropriate weights can also provide a.s. exact, asymptotically stable numerical methods for pure diffusions. The review on numerical stability is based on "major breakthroughs" of research for systems of SDEs over the last 35 years, with emphasis on applicability to all dimensions d≥1.
Civil unmanned aerial vehicles (UAVs) are growing rapidly in the last few years, thanks to their agility, flexibility and superior mobility. These benefits enable civil UAVs to be applied in a wide range of fields, such as film and television, logistics and distribution, agriculture and plant protection, land surveying and mapping, energy and power line inspection.
Up to now, the Federal Aviation Administration (FAA) of the United States has issued 280,418 remote pilot licenses for UAVs [1] and 120,844 remote pilot licenses have been issued by the Civil Aviation Administration of China (CAAC) [2].
Fatigue has been proved to have a negative impact on people and reduce their work efficiency. N. Aung and P. Tewogbola [3] discussed the impact of emotional labor on the health of employees in the workplace, pointing out that such negative factors as burnout and fatigue will affect employees' emotions, not only reducing their work efficiency but also seriously affecting their physical and mental health. M. Hoang et al. [4] investigated the influence of call frequency, worry, fatigue and other negative factors on work efficiency. Studies [5,6,7] have consistently demonstrate a vigilance decrement of remote pilots about 20–35 minutes after task initiation and the dramatic increasement of fatigue after 12 hours working [8]. However, so far, there is no relevant work have applied in fatigue detection field for UAV remote pilot.
At present, the researches on fatigue detection are mainly concentrated in the field of traditional drivers. It is widely recognized that the fatigue can be detected by four different features, including physiological features [9], psychological features [10], speech features [11] and visual features [12]. Fatigue detection methods based on physiological features collect physiological signals and extract t electromyography signal and electrocardiograph signal through sensors [13]. Another natural idea is using psychological features. X. Li et al. [14] proposed a fatigue driving detection method based on psychoacoustic perceptual masking processing steps so as to the high fatigue sensitive frequency components in the speaking process can be highlighted. However, the above two kinds of methods are inapplicable to UAV remote pilot due to sensor intrusion [15,16]. The fatigue detection methods based on speech feature are only applicable to some specific scenarios with standard callouts and responses, and the conversation databases are limited or insufficient in quantity.
The methods based on visual features are the last kind of fatigue detection method. Some facial movement features always reflect the fatigue state, such as the number of blinks increases, the blinking speed becomes slower, the number of nods increases, and frequent yawing. Researchers have been working on techniques to detect the fatigue of driver based on these visual signs and those visual features based methods can be divided into two parts: based on expert rules or based on deep learning.
The first part of visual features based methods is fully rely on the rules design by experts. Those hand designed features and detection standards result in a slow detection speed and a low detection accuracy [17] for detecting driver fatigue. The second part of visual features based methods applies machine learning to detect driver fatigue. With the successful application of neural network architecture such as convolutional neural network in the field of computer vision, such as image classification, semantic segmentation and object detection [18]. In the field of face recognition, F. Liu et al. [19] comprehensively reviewed the existing deep learning based single sample face recognition methods, classifying them into virtual sample methods and generic learning methods, and discussed the advantages and the common defects of these methods. Y. Ed-doughmi et al. [20] proposed a fatigue detection method for drivers based on RNN; Z. Chang et al. [21] proposed a fatigue detection method based on Haar feature and extreme learning machine; R. Huang et al. [22] applied the functional calibration depth convolutional model RF-DCM to solve the problem of speaking and yawning distinguishing problems based on mouth feature fatigue detection; W. Gu et al. [23] employed multi-scale pooling for eye and mouth state detection, which was then applied in hierarchical CNN based model for driver fatigue detection. S. Dey et al. [24] used the positions of eyes, nose and mouth to extract the deep information, such as the eye aspect ratio (EAR), the mouth opening ratio and the nose length ratio, by a histogram of oriented gradient (HOG) with support vector machine (SVM) pretrained model. Z. Xiao et al. [25] proposed a method for driver fatigue detection by deep cascaded multi-task long short-term memory (LSTM) model. W. Liu et al. [26] proposed a driver fatigue detection model based on multiple facial features and two stream convolution neural network, which can combine static and dynamic information. L. Geng et al. [27] proposed a real time fatigue detection method based on morphology infrared features and deep learning, which solves the problem of detecting driver fatigue in an underlit scene. F. Liu et al. [28] introduced the integration of RGB-D camera and deep learning where Generative Adversarial Networks and multi-channel scheme are used to improve the performance, proving that the features extracted by depth learning method are effective for fatigue detection.
To sum up, the current fatigue detection methods are aimed at the traditional driver, while the fatigue detection for UAV remote pilot, to our best knowledge, has not yet been carried out and they are inapplicable to UAV remote pilot fatigue detection due to the following three reasons: 1) the visibility of driver's working space is invariable and is not affected seriously by the outside environment. However, UAV remote pilots often work outdoors, greatly affecting by the visibility. 2) remote pilots always need to communicate with the air traffic controller frequently, which means a higher speaking time as well as the speaking frequency, resulting in a confusion between "yawn" and "speaking" that is critical and must be determined in fatigue detection practice. 3) the robustness of multi-facial features. Depending on the change of head posture, common fatigue state features are easily lost.
In view of the above problems, this paper proposed a fatigue detection method for UAV remote pilot. In brief, the main contributions of this paper are summarized as follows.
1) We establish a UAV remote pilots fatigue detection dataset OFDD that consists of 48 videos with diverse condition, along with the thorough analysis and finding on our dataset.
2) We propose a fatigue detection method for UAV remote pilots, which integrates multiple facial fatigue features, especially the head posture, and temporal information to solve above challenge.
3) The experimental results show that this method not only performs well on the traditional driver fatigue detection dataset with accuracy rate of 97.05%, but also achieves the highest detection accuracy rate of 97.32% on our OFDD dataset.
The rest of this paper is organized as follows. In Section 2, we first briefly review the related dataset for fatigue detection. Then, the UAV remote pilot fatigue detection dataset OFDD is established and analyzed. In Section 3, based on our findings from our OFDD, we propose a fatigue detection method for UAV remote pilot. In Section 4, experimental results are given to verify the performance of our proposed method. In Section 5, several conclusions are drawn based on the proposed method.
At present, there are several public fatigue detection datasets available for traditional driver, such as YawDD yawning dataset released by the University of Ottawa [29], DriveFace driver driving scene facial feature dataset released by the University of California Irvine [30], CEW blinking dataset released by Nanjing University of Aeronautics and Astronautics [31] and NTHU-DDD driver sleepiness detection dataset of National Tsinghua University [32]. To the best of our knowledge, YawDD, DriveFace, CEW and NTHU-DDD are the most popular datasets for traditional driver fatigue detection, and they have been widely used in most recent driver fatigue detection work.
The YawDD yawning dataset is one of the earliest driver fatigue datasets. YawDD contains 322 videos captured by on board camera and consists of both male and female drivers, with and without glasses/sunglasses, from different ethnicities, and in 3 different situations: 1) normal driving (no talking), 2) talking or singing while driving, and 3) yawning while driving under natural and changing lighting conditions. For each video, the length ranges from 10 to 120 s. It is mainly used to develop and test fatigue detection algorithms and models, and can also be used for face and mouth recognition and tracking.
DriveFace dataset is a public image dataset that contains a sequence of object images when driving in a real scene. It consists of 606 samples, and the resolution of each sample is 640 × 480. It was obtained from 4 drivers (2 women and 2 men) on different days. These drivers have various face accessories, such as wearing glasses and beard.
CEW blink dataset is provided by Nanjing University of Aeronautics and Astronautics. It contains photos of the 2423 testers with the open and closed eyes, including individual differences and various environments, such as lighting, blur, and glasses.
NTHU-DDD is another driver sleepiness detection dataset established by National Tsinghua University. The whole dataset contains 36 subjects of different ethnicities recorded with and without wearing glasses under a variety of simulated driving scenarios, including normal driving, yawning, slow blinking, falling asleep, laughing, etc., under different illumination conditions. Further, the 90 videos in NTHU-DDD consist of two scenarios, including drowsiness related symptoms (yawning, nodding, slow blink rate) and non-drowsiness related actions (talking, laughing, looking at both sides), are each recorded about 1.5 minutes long.
Although these datasets have been widely used in driver fatigue detection, they are not designed for UAV remote pilot fatigue detection. Specifically, YawDD yawning dataset and DriveFace dataset are video dataset recorded by onboard cameras. The scenes captured are limited to the main driver's seat, while UAV remote pilots often work outdoors and have a wide range of activities. Besides, some datasets are mainly designed for eye state, such as CEW. However, UAV remote pilots sometimes need to wear sunglasses so as to the eyes will be blocked and we cannot access the fatigue feature. Additionally, remote pilots always need to communicate with the air traffic controller frequently, resulting in a continuous mouth pose variation, which cannot be found easily in above datasets.
As analyzed above, the existing fatigue detection datasets are designed for traditional drivers, and the working scenes as well as the contents of UAV remote pilots are quite different from those of traditional drivers. Up to now, to our best knowledge, there is no dataset for UAV remote pilot fatigue detection. In this work, we have established a new UAV remote pilot fatigue detection dataset (OFDD) to facilitate future research. The details about our dataset are discussed in the Section 2.1.
We present our OFDD dataset from aspects of category, procedure and peculiarity.
Category. As pointed out in the literature [33], the face is a non-rigid complex structure. Fatigue is determined by many factors, including posture, light, facial expression, etc. Therefore, in order to diversify the content of our OFDD and represent the different working states for UAV remote pilot, we make our OFDD UAV fatigue detection dataset from the perspectives of gender, lighting, occlusion and action, and the keyword hierarchy of video categories is shown in Figure 1.
In practice, we asked UAV remote pilots of different genders to wear glasses/sunglasses or not; then they were asked to work in three different mouth conditions: 1) keep their mouth closed (without speaking), 2) speaking, 3) yawning. Because the UAV remote pilots have to work in different visibility environment, we also recorded our video from three different visibility environment: low visibility (nighttime), medium visibility (foggy) and high visibility (daytime). The right part of in Figure 1 shows the scenes after all conditions combined, and there are 48 combined scenes with different conditions.
Procedure. In practice, the video is collected by Hikvision E12 camera, and the camera is set in front of the UAV remote pilot. The detailed setting includes 640 x 480 resolution, 24-bit true color (RGB) and 30 frames per second. In Figure 2, it is a partial picture of our OFDD dataset, which can be viewed from left to right under different visibility conditions. During the experiment, each participant was asked to free control the UAV during nine different situations with randomly sequence: 1) high visibility (daytime), no talking, 2) high visibility (daytime), talking, 3) high visibility (daytime), yawning, 4) medium visibility (fog), no talking, 5) medium visibility (fog), talking, 6) medium visibility (fog), yawning, 7) low visibility (nighttime), no talking, 8) low visibility (nighttime), talking and 9) low visibility (nighttime), yawning. Also, each participant was required to do the same things with normal glasses and sun glasses orderly. The combination of these completed stages helps to create a more realistic scene, distinguish these situations at the same time, and accurately detect the yawning posture. The length of each video ranges from 30 to 100 seconds, and after 48 videos were collected, with a total duration of 63 minutes and 14 seconds, the audio was deleted in order to reduce the volume.
Peculiarity. Our OFDD UAV remote pilot fatigue detection dataset has all the following important features.
1) Our OFDD dataset is the first UAV remote pilot fatigue detection dataset.
2) The subjects were UAV remote pilots working in different visibility.
3) The position of the camera and the angle of capturing the remote pilot are the same as those in the actual control monitoring system.
4) The remote pilot shall be photographed under various mouth opening conditions, such as normal (no talking), talking or yawning conditions and etc.
5) Face covers, such as wearing glasses and sunglasses, are considered to test the robustness of the algorithm.
6) The dataset is enough to be statistically significant.
In this section, we deeply mined our OFDD UAV remote pilot fatigue detection dataset, and further made statistics and analysis on each video. After that, we summarized the correlation and difference of fatigue features between traditional car driver and UAV remote pilot.
First, we investigated the relationship between multiple facial features and fatigue state in our OFDD dataset. We have counted the number of fatigue symptoms of our OFDD dataset, as shown in Figure 3, including blinking times, yawning times and nodding times. We can observe from Figure 3 that the number of fatigue symptoms of a fatigued UAV remote pilot is much greater than those of non-fatigued, which is in agreement with traditional car driver.
This result implies a high correlation between multiple facial features and fatigue of UAV remote pilot and we can further infer that the fatigue of UAV pilot can be identified by the frequency of three fatigue symptoms: blinking, nodding and yawning.
In addition, it is interesting to explore the difference between traditional driver fatigue dataset and UAV remote pilot fatigue dataset. We find that UAV remote pilots have two obvious differences compared with traditional car drivers: 1 Speaking frequency 2 Visibility condition. Specifically, we specially counted the speaking frequency under three different visibility conditions over OFDD and YawDD. As shown in Figure 4, the traditional driver is sitting in the driver seat (high visibility) and there is no data related to medium visibility (foggy) and low visibility (nighttime) scenes over YawDD dataset while UAV remote pilot need to work in all the above scenarios. Secondly, one also finds from Figure 4 that the speaking frequency of UAV remote pilots in OFDD dataset is significantly higher than that of traditional drivers in YawDD dataset. The reason may be that the UAV remote pilot always need to communicate with the air traffic controller frequently during working.
However, in the traditional driver fatigue detection, the opening and closing aspect ratio of driver's mouth is often used as the key element to judge fatigue, and the time sequence information do not be considered. This result implies the traditional fatigue detection method is not suitable for UAV remote pilots and temporal information should be introduced to distinguish yawning from speaking.
For detecting fatigue state of UAV remote pilot, we develop a new DNN based detection network YPS network. In Figure 5, our whole detection network can be divided into face detection module, multi-feature extraction module, and temporal fatigue decision module. According to the findings in Section 2, multiple facial fatigue features is highly correlated to the fatigue state, and the temporal information is essential for fatigue detection of UAV remote pilots. As such, we first regard two contiguous frames Xt and Xt−1 as the input of face detection module and the face detection module detects the human face through a state-of-the-art convolutional neural network. Then, the detected human faces serve as the input of multi-feature extraction module. After feature extraction, the temporal fatigue decision module integrates both the variety of different fatigue features and temporal information to make a final decision.
In face detection module, we employ the state-of-the-art detection network, YOLOv5 [34]. YOLOv5 has four different models including YOLOv5s, YOLOv5m, YOLOv5l and YOLOv5x. We choose YOLOv5s as our face detection model. YOLOv5s has four parts: input, backbone, neck and prediction and the inference time is about 0.007 s, that is 140 frame per second.
In practice, we respectively use the architecture of CSPDarknet53 with an SPP layer as our backbone, PANet as Neck. To further optimize the detection performance of human face, we follow the origin data enhancement framework of mosaic data enhancement and reset the anchor size to match the size of the network receptive field and the image scale. The details about our backbone choice and anchor setting are discussed in experiment.
We present our multi-feature extraction module from four subcomponents: face key point detection, eye fatigue feature, mouth fatigue feature and head posture fatigue feature.
First, following most of the existing fatigue detection technologies [35,36,37], we find the key point of the face. The number of face key points of traditional face key point detection methods includes 5 points, 68 points and 106 points, as shown in Figure 6. In practice, we choose 68 key points because 68 key points can accurately display the local features such as human eyes and mouth of a human head, reduce the operation time and improve the real time performance of face recognition [38].
As for the face key point detection model, we choose PFLD face key point detection model [39]. Besides, based on our finding in OFDD, we introduce temporal feature and propose a face key point detection model for UAV remote pilots. The architecture of face key point detection model is shown in Figure 7.
The L2 loss function of key point detection module can be expressed as
L2=1W∑Ww=1∑Nn=1[∑Cc=1ωcn∑Kk=1(1−cosθkn)]‖dwn‖22 | (1) |
where W is the number of samples; N is the number of feature points; ‖dmn‖ is the distance measurement of the n-th feature point in w samples; C is the number of different human faces (side face, front face, head up, head down, expression and occlusion); ωcn is the given weight corresponding to category C; K=3 is the three dimensions of face pose, which is namely yaw, pitch, roll angle (the higher the angle, the greater the weight); θ is the deviation value of predicted value in yaw angle, pitch angle and roll angle.
In order to better describe the eye state, the midpoint of key points 38 and 39, as shown in Figure 6(c), is used to represent the upper eyelid position of the left eye and the midpoint of key points 41 and 42 is used to represent the lower eyelid position of the left eye. As for the right eye, we use the midpoint of key points 44 and 45 to represent the upper eyelid position, and use the midpoint of key points 47 and 48 to represent the lower eyelid position. As a result, the eye state can be calculated by following formula.
E=dist(Elu,Eld)dist(Eolu,Eold)+dist(Eru,Erd)dist(Eoru,Eord) | (2) |
Elu=(x38+x392,y38+y392) | (3) |
Eld=(x41+x422,y41+y422) | (4) |
Eru=(x44+x452,y44+y452) | (5) |
Erd=(x47+x482,y47+y482) | (6) |
Among them, Elu, Eld, Eru and Erd respectively represent the upper and lower eyelid positions of the left and right eyes, which are calculated according to the coordinates of eye key points in the detection process of each frame; distolu, distold, distoru, distold represent the distance between the upper and lower eyelids in the initial state, which only needs to be calculated once in the initial state of the system. The value of distance is calculated by the Euclidean distance.
According to the findings in Section 2, the temporal information is essential for fatigue detection of UAV remote pilots, especially in distinguishing yawning and speaking with mouth fatigue feature.
There are usually three states of the mouth: normal, speaking and yawning. People yawn more frequently when they are tired. Therefore, the features of the mouth are also an important feature for judging fatigue. From the Section 2, it can be seen from the statistical data of OFDD dataset that the frequency of yawning can effectively reflect the fatigue state of UAV remote pilots. When the UAV remote pilot yawns due to fatigue, the mouth will open to the greatest extent, and the mouth will increase in height and decrease in width. In order to measure the fatigue state represented by these two indicators, the mouth aspect ratio M is introduced, and its calculation formula is
M=(|y51−y59|)+(|y53−y57|)2(x55−x49) | (7) |
where x49 and x55 are the abscissa of the two key points on the left and right of the mouth; y51, y53, y57 and y59 are the ordinates of the four key points above and below the mouth.
When determining the fatigue state according to the mouth state, we calculate the ratio between the origin distance between left and right corners of the mouth and the current distance between left and right corners of the mouth. If the ratio is greater than the given threshold value of 1.2, it is recognized as yawning.
In order to eliminate the interference of speaking and other actions, it is not reliable to judge the fatigue state only by the mouth state of current frame, so we introduce extra temporal information of mouth feature from last frame to improve the accuracy of judging the fatigue state. As a result, if the value of M is greater than the given threshold value of 1.2 for a continuous period of time (2S), it can be determined that the current UAV remote pilot is in a fatigue state.
People tend to nod their heads frequently or lower head their heads for a long time when they are in fatigue. Therefore, nodding (lower head) situation can be used as a fatigue characterization parameter. When people lower their heads, the distance from the midpoint of eyes to mouth in the photos taken by the camera will be significantly shorter than that when people raise their heads. Therefore, the change of this distance is used in this work as the change of nod (lower head) posture.
In Figure 6(c), the center position of left eye [xleft,yleft] and right eye [xright,yright] can be expressed as:
xleft=14(x38+x39+x41+x42)yleft=14(y38+y39+y41+y42)xright=14(x44+x45+x47+x48)yright=14(y44+y45+y47+y48) | (8) |
Then the linear fitting function of the center of both eyes is
x-xleftxright-xleft=y-yleftyright-yleft | (9) |
where xleft, xright, yleft and yright are the horizontal and vertical coordinates of the midpoint of the left and right eyes respectively; x, y is the horizontal and vertical coordinates of the linear function of the two eyes. When Eq (7) is transformed into standard Ax+By+C=0, the values of A, B, C are
{A=yright−yleftB=xleft−yrightC=xrightyleft−xleftyright | (10) |
Then, the distance from the midpoint of the two eyes of the face to the line is calculated by using point 52, that is, the distance d from the midpoint of the two eyes on the image to the mouth can be calculated by
d=|Ax52+By52+C√A2+B2|cos(pitch1) | (11) |
With reference to p80 standard [40], the threshold value of distance d is set to 0.8. In other words, when d≤0.8dmax, it can be determined that the driver is in a low head state. If the remote pilot hold bow state more than 5 s, it can be directly judged that the pilot is in the fatigue state. If the remote pilot hold less than 5 s, the percentage of bow state can be calculated by
H=tdownT×100% | (12) |
where tdown is the head lowering time; T is the total time; H is the head lowering frequency. The greater the value, the deeper the fatigue degree. H can also be used to determine the fatigue state. The Euler angle of the remote pilot's head posture is divided into pitch angle, yaw angle and roll angle, that is, the Euler angle of the remote pilot's head rotating around the x, y and z axes respectively. According to the research [41], the maximum angle range of the human head rotating around the X axis of the central axis is [-60.4°, 69.6°], the maximum range of rotating around the Y axis is [-90°, 75°], and the maximum range of rotating around the Z axis is [-40.9°, 36.3°]. Thus, the abnormal angle can be calculated according to the remote pilot's real time pitch, yaw and roll. In this paper, the judgment of abnormal angle refers to p80 standard [41] in PERCLOS, that is, when the remote pilot's head angle exceeds 80% of the normal range, it is determined as abnormal angle.
In temporal fatigue decision module, the cross-time temporal information integration procedure and multi-fatigue feature fusion SVM are proposed. In particular, the cross-time temporal information integration procedure can be used to encode temporal information from different frames and the multi-fatigue feature fusion SVM is developed to cumulate multi-fatigue feature and make a final decision.
The fatigued state of the human face can be comprehensively expressed according to the eyes (open, closed), mouth (closed, talking, yawning) and head yaw angle, pitch angle and roll angle. After the PFLD obtains 68 key points of the face, for the video image frames collected on the continuous time node sequence (Tt,Tt−1), each frame image can be used as a state according to the key points of the eye, mouth and head posture. Through 68 key points and attitude angles, the fatigue features E, M and H can be obtained according to Eqs (2), (7) and (12). As for the Euler angle, a standard face is defined firstly (the average value is taken from a stack of frontal faces), and 11 key points are fixed on the main plane of the face as the reference for all training faces. Then, using the corresponding 11 key points and the reference matrix of the estimated rotation matrix, the Euler angle is calculated by the rotation matrix.
Finally, combining the above features, we can get the following feature matrix (13).
[TtEtMtHyawtpitchtrolltTt−1Et−1Mt−1Hyawt−1pitcht−1rollt−1] | (13) |
where yaw, pitch and roll are the output vector of the PFLD auxiliary subnet (upper left blue box in Figure 7) to predict the human face posture during training.
Support vector machine (SVM) is a highly accurate and efficient supervised machine learning algorithm, which is mainly used to solve the data classification problem in the field of pattern recognition. After cumulating the deep fatigue features, this work uses SVM algorithm to establish a fatigue judgment model for UAV remote pilots.
Because the collected data of eye, mouth and head features are not regular, this kind of problem belongs to nonlinear classification problem. According to the time point when the characteristics change, the awake state and the fatigue state are distinguished. The identified fatigue characteristics of eyes, mouth and head are classified as fatigue state, and the classification label is 1; classify others as awake, and the classification label is 0.
In this section, our fatigue detection method of UAV remote pilot is tested, and a comparative experiment is carried out on OFDD dataset and other dataset to verify the effectiveness of the algorithm.
In our experiment, videos in 48 subcategories of OFDD UAV remote pilot fatigue detection dataset were randomly divided into training set, verification set and test set. Specifically, we intercepted continuous frame images of the video in the 48 subcategories of OFDD dataset. In order to balance the data in the experiment, we only clipped the first 30 s of videos in each subcategory for training. The resolution of each continuous frame image is 640 × 480, the horizontal resolution is 96 dpi, the vertical resolution is 96 dpi. In order to evaluate the performance of our proposed fatigue detection method for UAV remote pilots, we compared 10 other more effective fatigue detection methods. All experiments were conducted on a computer using Intel (R) core (TM) i7-4770. These include CPU@3.4 GHz, 16 GB ram and two NVIDIA GeForce GTX 3080 GPU.
The accuracy of face region and key point marking samples in the self-collected dataset is crucial to the accuracy of in depth learning. A picture is intercepted every 2 frames from the videos in the 48 subcategories in the OFDD dataset. A total of 21,860 frames of pictures are intercepted and the added face frame and key point positions are marked, like in Figure 8.
The setting of anchor is very important for face position. At present, most popular face detection algorithms use Anchor mechanism in the detection process, such as Face R-CNN [42], FDRNet [43], DSFD [44], RetinaFace [45], DFS [46], S3fd [47], FAN [48], etc. The anchor should cover the target to be detected, and the scale of the Anchor should match the size of the network receptive field and the image scale.
In order to ensure that human face is in the picture frame of the camera, the camera's angle of view should be as wide as possible, so there will be a lot of interference information in the background. In order to reduce the impact of other backgrounds, this paper sets an appropriate Anchor according to the receptive field of the human head and the scale of the feature map.
According to the Anchor setting method in DFS, FAN, the aspect ratio of the anchor is set to 1:1.5 in the three higher-level face detection modules FDM1, FDM2 and FDM3, while the aspect ratio of the anchor is set to 1:1 in the lower-level detection modules FDM4 and FDM5. This is because the smaller size face is closer to a square than the large-scale front face. In addition, each point in the feature map of each face detection module is set with three Anchors of different sizes to improve the detection accuracy. The setting of Anchor size and proportion in each level of feature map in the five detection modules is shown in Table 1.
Detection module | Stride | Anchor (width) | Aspect ratio |
FDM5 | 4 | 121,620 | 1:1 |
FDM4 | 8 | 243,240 | 1:1 |
FDM3 | 16 | 486,480 | 1:1.5 |
FDM2 | 32 | 96,128,160 | 1:1.5 |
FDM1 | 64 | 192,256,320 | 1:1.5 |
Where the stride indicates the relationship between the size of the current feature map and the input image. For example, if Stride is 4, the width and height of the current feature map are respectively 1/4 of the width and height of the input image. The corresponding Stride of the detection module determines its Anchor interval in the input image. For example, the Stride in DM5 is 4 and the Anchor size is 12 × 12, which Indicates that there is a 12 × 12 Anchor for every 4 pixels on the input image, and the ratio of anchors is 3–5 times that of Stride, which ensures that anchors of different scales have the same density on the image, and faces of various scales can roughly match the same number of anchors. In the training process, if the IOU of face annotation box and Anchor is greater than 0.5, it is considered that the box contains positive face samples. If the IOU is less than 0.3, it is considered that the box contains negative background samples without faces. If the IOU is between 0.3–0.5, it is ignored in the training process.
The labeled ODFF dataset is divided into training set, verification set and test set according to the ratio of 8:1:1. After introducing the training set data into the modified Anchor, yolov5 is trained. The trained model is fitted and verified with the validation set data, and then tested with the test dataset. The test identification results are shown in the table.
It can be seen from Table 2 that the recognition rate of face area reaches 99.5%, which is 1.2% higher than the original yolov5. This experimental result shows that our improved anchor setting for UAV remote pilot face detection is effective.
Face detection network | Number of face photos (piece) | Number of recognized faces (piece) | Recognition rate (%) |
Yolov5s | 21,860 | 21,488 | 98.3% |
Ours | 21,860 | 21,751 | 99.5% |
In this subsection, we compare the performance of different backbone in our UAV remote pilot fatigue detection model, including ResNest50 [49], MobileNetV2 [50], HRNetV2 [51] and EfficientNet [52]. Table 3 demonstrates the performance of different backbone.
Name | Params | Mean error | Failure rate |
ResNest-50 | 122.27 M | 0.046 | 0.038 |
MobileNetV2_0.25 | 1.09 M | 0.075 | 0.174 |
MobileNetV2_1.00 | 7.28 M | 0.065 | 0.127 |
HRNetV2 | 545.07 M | 0.066 | 0.125 |
EfficientNet-B0 | 16.67 M | 0.064 | 0.119 |
EfficientNet-B1 | 26.37 M | 0.075 | 0.149 |
EfficientNet-B2 | 30.85 M | 0.071 | 0.145 |
EfficientNet-B3 | 42.29 M | 0.099 | 0.136 |
EfficientNet-B4 | 68.34 M | 0.077 | 0.164 |
EfficientNet-B5 | 109.34 M | 0.094 | 0.173 |
EfficientNet-B6 | 156.34 M | 0.081 | 0.175 |
EfficientNet-B7 | 244.03 M | 0.081 | 0.196 |
The performance analysis of the backbone structure in the network structure is shown above. In the experiment, we tested 13 different versions of the backbone structure. Here, we mainly use the backbone parameter size, average error rate and failure rate to evaluate the performance of the adopted backbone structure. It can be seen from the results in Table 3 that the average error rate and failure rate of resneset50 are 0.046 and 0.038 respectively, reaching the lowest point in the experiment, but the parameters of the backbone structure are too large, about 122.27 M; the average error rate and failure rate of Blaze landmark are 0.069 and 0.131 respectively. The backbone parameter size is 7.52 M, and the first iteration time (seconds) is 0.171. The backbone parameter size is in the third place. The average error rate and failure rate of HRNetV2 are 0.066 and 0.125 respectively, but the parameter is 545.07 M. The big backbone structure is not suitable for actual deployment of UAV fatigue detection, so we choose efficient as the backbone of our model.
We also tested eight EfficientNet backbone structures from efficientnet-b0 to efficient-net-b7. The experimental results show that with the increase of backbone size, their average error rate and failure rate do not decrease significantly, while the backbone structure parameters increase gradually. The network backbone structure with the best performance in the experiment are MobileNetV2_0.25 and MobileNetV2_1.00, not only are the size relatively small, but also the average error rate and failure rate relatively low. For MobileNetV2_0.25 and MobileNetV2_1.00, although both are lightweight network backbone, but the average error rate and failure rate of MobileNetV2_1.00 are only 0.065 and 0.124, both better than MobileNetV2_0.25. These experimental results show that the error rate of MobileNetV2_1.00 selected in the paper is low, the detection accuracy is higher, and the comprehensive detection effect is better. The model used in this paper is used to detect the fatigue of UAV remote pilots, which is easy to be applied in low computing power devices such as mobile devices, and is suitable for the fatigue state detection of UAV remote pilots in outdoor scenes.
According to the statistical analysis of OFDD UAV remote pilot fatigue detection dataset, the fatigue state of UAV remote pilot is highly correlated to multi-facial fatigue characteristics, including eye fatigue feature, mouth fatigue feature and head posture fatigue feature, and also the temporal information is essential for fatigue detection of UAV remote pilots. In order to further verify the accuracy of our proposed method, we used eye feature parameter E, mouth feature parameter M, head feature parameter H, pitch angle pitch, yaw angle yaw and roll angle to perform ablation experiments. The results are shown in Table 4.
Fatigue characteristic parameters | Accuracy rate (%) |
E + pitch + yaw + roll | 93.26 |
M + pitch + yaw + roll | 90.13 |
H + pitch + yaw + roll | 86.76 |
E + M + H + pitch + yaw + roll | 97.32 |
It can be seen from Table 4 that when the eye feature parameter E is used to train the network with the angle of yaw, pitch and roll, the detection accuracy is 93.26%; when the mouth feature parameter M and yaw, pitch and roll angles are used to train the network, the detection accuracy is 90.13%; when the head feature parameters H and yaw, pitch and roll angles are used to train the network, the detection accuracy is 86.76%. It can be seen that when a single feature parameter is used for training, the eye feature parameter E has the highest accuracy rate, followed by the mouth feature parameter M, and finally the head feature parameter H. Ablation experiments show that eye feature parameters, mouth feature parameters and head feature parameters are all important for UAV remote pilot fatigue detection.
We conducted ablation experiments on face key point detection modules and compared five face key point detection modules: DSFD [44], RetinaFace [45], PFLD [39] (no auxiliary) and PFLD [39], where the first three model do not include head posture features (pitch + yaw + roll).
It can be seen from Table 5 that although the parameters and FLOP of DSFD and RetinaFace are very low, the accuracy of the face detection module is about 1 and 2% lower than that of PFLD (no auxiliary) respectively. For a PFLD module with the auxiliary, it can not only output 68 key points of face coordinates through the main network but also the yaw, pitch and roll attitude Euler angles of the head through its auxiliary network, which is helpful to improve the detection accuracy. As a result, PFLD module not only achieves the highest accuracy, but also has the smallest computational burden.
Module | Head Posture Feature | Backbone | Accuracy | Params (M) | Flops (G) |
DSFD | × | MobileNetV2_0.25 | 86.25 | 10.26 | 22.55 |
RetinaFace | × | MobileNetV2_0.25 | 87.78 | 8.43 | 18.34 |
PFLD (no auxiliary) | × | MobileNetV2_0.25 | 88.64 | 7.68 | 16.92 |
PFLD | √ | MobileNetV2_0.25 | 97.32 | 8.21 | 17.53 |
In this section, in order to verify the application particularity of UAV remote pilot fatigue detection and the superiority of the method proposed in this paper, we will compare the accuracy of our proposed method for UAV remote pilot fatigue detection with that of other traditional driver's fatigue detection methods. Including the LSTMs of literature [20], the Haar + ELM of literature [21], the RF-DCM of literature [22], the MSP-Net of literature [23], the HOG-SVM of literature [24], the FDRNet of literature [25] and the GFDN of literature [26]. The results are shown in Table 5.
In our experiments, we measure the performance of the algorithm with accuracy. As can be seen from Table 6, when did the experiment on our OFDD dataset, among all the comparison methods, the accuracy of LSTMs [20] is the lowest with 89.23% accuracy. The accuracy rates of Haar + ELM [21], RF-DCM [22], MSP-Net [23], HOG-SVM [24], FDRNet [25] and GFDN [26] are 92.71, 90.12, 93.69, 94.04, 94.61 and 94.88% respectively. Among all the experimental methods, the accuracy of the fatigue detection method for UAV remote pilots proposed by us is the highest, reaching 97.32%, 8.09% higher than LSTMs, and 2.44% higher than GFDN. The experimental results show that our method can detect the fatigue of UAV remote pilots with state-of-the-art performance. We hold the view that the main reasons for the superior performance of our method in OFDD UAV remote pilot fatigue detection dataset are as follows: 1) Our method adjusts the size of network input and anchor box in the face detection part, which improves the accuracy of face detection. 2) The PFLD module is fused to extract the features of eye, mouth and head features, which improves the extraction accuracy of face key points. 3) The temporal information is introduced to reduce the misjudgment of mouth movements such as speaking and improve the accuracy of fatigue detection.
Literature | Algorithm | Accuracy rate (%) |
[20] | LSTMs | 89.23 |
[21] | Haar + ELM | 92.71 |
[22] | RF-DCM | 90.12 |
[23] | MSP-Net | 93.69 |
[24] | HOG-SVM | 94.04 |
[25] | FDRNet | 94.61 |
[26] | GFDN | 94.88 |
Ours | Our method | 97.32 |
We selected some samples in OFDD dataset to analyze the detection results of different algorithms, shown in Figure 9 The first picture shows the normal working scene of a male remote pilot without glasses under daytime conditions. The second picture shows the normal working scene of a female remote pilot wearing sunglasses during the daytime. The third picture shows a male remote pilot without glasses yawning in fog. The fourth picture shows the working scene of a female remote pilot without glasses talking in fog. The fifth picture shows the normal working scene of a male remote pilot without glasses at night. The sixth picture shows the normal working scene of a female remote pilot wearing glasses at night.
It can be seen from Figure 9 that the LSTMs algorithm fails to detect in medium visibility (fog). We think the reason for the failure may be that LSTMs is a fatigue detection method based on human posture. However, in the fog scene, the remote pilots are far away from the camera and their body postures are not clear. Haar + ELM and RF-DCM do not perform well in the graph of yawning in foggy days. This can be caused by the above two algorithms are the fatigue detection methods for traditional drivers based on mouth features, which may mislead the decision in frequent speak scene.MSP-Net method successfully distinguishes yawning and speaking, but the detection effect is poor in the night scene. Although GFDN has successfully extracted the eye feature parameters in the scene of wearing glasses at night, this method fails in the scene of wearing sunglasses with serious masking. The fatigue detection method proposed in this paper is suitable for UAV remote pilots. In view of the frequent speech of UAV remote pilots and the working scene under high visibility, it combines the eye fatigue feature, mouth fatigue feature and head posture fatigue feature, and achieves the best detection accuracy in OFDD dataset.
In order to evaluate the generalization ability of our proposed method and further verify the effectiveness of our method. We conducted experiments on the public dataset YawDD dataset. The algorithm in this paper is compared with other latest fatigue detection algorithms in YawDD dataset, and the results are shown in Table 7.
Literature | Algorithm | Accuracy rate (%) |
[20] | LSTMs | 92.71 |
[21] | Haar + ELM | 93.90 |
[22] | RF-DCM | 89.42 |
[23] | MSP-Net | 95.36 |
[24] | HOG-SVM | 96.40 |
[25] | FDRNet | 98.96 |
[26] | GFDN | 97.06 |
Ours | Our method | 97.05 |
It can be seen from Table 7 that the detection accuracy of the method proposed in this paper is 97.15% in the public dataset of YawDD. The method proposed in this paper is higher than LSTMs [20], Haar + ELM [21], RF-DCM [22], MSP-Net [23] and HOG-SVM [24], but lower than FDRNet [25] and GFDN [26]. Considering that the traditional driver's working scene is relatively fixed and the working environment is relatively single, while the UAV remote pilot's working scene is complex and the environment is diverse, the main research and experimental object of the method proposed in this paper is the UAV remote pilot. The experimental results show that the algorithm can basically meet the fatigue detection of traditional drivers as well as the fatigue detection of UAV remote pilots.
The above experimental results show that, compared with the traditional fatigue driving detection algorithm, the fatigue detection algorithm based on deep learning can automatically extract the features of the input image, mine the deep information of the target image and do not rely on manual participation.
Summing up, compared with other in depth learning models, this paper effectively improves the recognition ability of fatigue characteristics of the model, improves the accuracy of fatigue detection, has strong robustness to the complex environment of the UAV remote pilot's working scene, and can basically realize the real time detection of the UAV remote pilot's fatigue state.
In addition, we additionally tested and analyzed the accuracy of different methods in different working scenarios. Specifically, in order to verify the application particularity of UAV remote pilot fatigue detection and the superiority of the method proposed in this paper, we will compare the accuracy of our UAV remote pilot fatigue detection method with other traditional pilot fatigue detection methods in the daytime, foggy day and nighttime.
It can be seen from Figure 10 that all algorithms have the best performance in daytime scenes, poor performance in nighttime scenes, and the worst performance in foggy scenes. This can be explained as: firstly, the lighting conditions in foggy days and at night are poor, which is not conducive to obtaining high precision face key points. Secondly, comparing with the nighttime, the sunglasses may block the eye features of remote pilots in foggy days.
As above analysis, we can draw a conclusion that the detection accuracy can keep rising by image enhancement module in foggy and nighttime scenes.
Based on the Yolov5 network, this paper integrates the PFLD module to detect the fatigue of UAV operators, which is convenient for application in low computing power devices such as mobile devices. The whole network input and the size of the anchor frame, and temporal feature is introduced to propose a fatigue detection method for UAV operators. This method not only performs well in the traditional motor vehicle driver data and achieves a detection success rate of 97.05%. And it achieves the highest detection success rate of 97.32% on the UAV operator's fatigue detection dataset OFDD. This method has good efficiency and accuracy, and can meet the requirements of UAV operator fatigue control detection based on visual features.
This research was funded by science and technology plan project of Sichuan Provincial Department of Science and Technology, grant number 21RKX0103. This research was funded by Civil Aviation Flight University of China "Smart Civil Aviation" special project, grant number ZHMH2022-005. This research was funded by key science and technology project of Civil Aviation Flight University of China, grant number ZJ2021-11. This research was funded by independent research project of civil aviation flight technology and flight safety key laboratory of Civil Aviation Flight University of China, grant number FZ2022ZZZ06.
The authors declare there is no conflict of interest.
[1] | E. Allen, Modeling With Itô Stochastic Differential Equations, Dordrecht: Springer, 2007. https://doi.org/10.1007/978-1-4020-5953-7 |
[2] | L. Arnold, Stochastic Differential Equations: Theory and Applications, 1974, (Reprinted by Dover Publications, 2014). |
[3] |
S. S. Artemiev, The mean square stability of numerical methods for solving stochastic differential equations, Russ. J. Numer. Anal. Math. Modelling, 9 (1994), 405–416. https://doi.org/10.1515/rnam.1994.9.5.405 doi: 10.1515/rnam.1994.9.5.405
![]() |
[4] | T. A. Averina, S. S. Artemiev, Numerical Analysis of Systems of Ordinary and Stochastic Differential Equations, Utrecht: De Gruyter, 1997. https://doi.org/10.1515/9783110944662 |
[5] |
K. Burrage, P. Burrage, T. Mitsui, Numerical solutions of stochastic differential equations—implementation and stability issues, J. Comput. Appl. Math., 125 (2000), 171–182. https://doi.org/10.1016/S0377-0427(00)00467-2 doi: 10.1016/S0377-0427(00)00467-2
![]() |
[6] |
K. Burrage, G. Lythe, Accurate stationary densities with partitioned numerical methods for stochastic differential equations, SIAM J. Numer. Anal., 49 (2009), 1601–1618. https://doi.org/10.1137/060677148 doi: 10.1137/060677148
![]() |
[7] |
J. Cui, J. Hong, D. Sheng, Density function of numerical solution of splitting AVF scheme for stochastic Langevin equation, Math. Comput., 91 (2022), 2283–2333. https://doi.org/10.1090/mcom/3752 doi: 10.1090/mcom/3752
![]() |
[8] |
R. D'Ambrosio, M. Moccaldi, B. Paternoster, Numerical preservation of long-term dynamics by stochastic two-step methods, Discrete. Cont. Dyn.-B, 23 (2018), 2763–2773. https://doi.org/10.3934/dcdsb.2018105 doi: 10.3934/dcdsb.2018105
![]() |
[9] |
M. A. Eissa, H. Zhang, Y. Xiao, Mean-square stability of split-step theta Milstein methods for stochastic differential equations, Math. Probl. Eng., 2018 (2018), 1–13. https://doi.org/10.1155/2018/1682513 doi: 10.1155/2018/1682513
![]() |
[10] | L. C. Evans, An Introduction to Stochastic Differential Equations, Providence: American Mathematical Society, 2014. https://doi.org/10.1090/mbk/082 |
[11] |
D. B. Hernandez, R. Spigler, A-stability of implicit Runge-Kutta methods for systems with additive noise, BIT, 32 (1992), 620–633. https://doi.org/10.1007/BF01994846 doi: 10.1007/BF01994846
![]() |
[12] |
D. B. Hernandez, R. Spigler, Convergence and stability of implicit Runge-Kutta methods for systems with multiplicative noise, BIT, 33 (1993), 654–669. https://doi.org/10.1007/BF01990541 doi: 10.1007/BF01990541
![]() |
[13] |
D. J. Higham, A-stability and stochastic mean-square stability, BIT, 40 (2000), 404–409. https://doi.org/10.1023/A:1022355410570 doi: 10.1023/A:1022355410570
![]() |
[14] |
D. J. Higham, Mean-square and asymptotic stability of the stochastic Theta method, SIAM J. Numer. Anal., 38 (2000), 753–769. https://doi.org/10.1137/S003614299834736X doi: 10.1137/S003614299834736X
![]() |
[15] |
D. J. Higham, X. Mao, A. M. Stuart, Exponential mean-square stability of numerical solutions to stochastic differential equations, LMS J. Comput. Math., 6 (2003), 297–313. https://doi.org/10.1112/S1461157000000462 doi: 10.1112/S1461157000000462
![]() |
[16] |
C. Huang, Exponential mean square stability of numerical methods for systems of stochastic differential equations, J. Comput. Appl. Math., 236 (2012), 4016–4026. https://doi.org/10.1016/j.cam.2012.03.005 doi: 10.1016/j.cam.2012.03.005
![]() |
[17] | S. Kanagawa, On the rate of convergence for Maruyama's approximate solutions of stochastic differential equations, Yokohama Math. J., 36 (1988), 79–85. |
[18] |
S. Kanagawa, The rate of convergence for approximate solutions of stochastic differential equations, Tokyo J. Math., 12 (1989), 33–48. https://doi.org/10.3836/TJM/1270133546 doi: 10.3836/TJM/1270133546
![]() |
[19] |
S. Kanagawa, Error estimation for the Euler-Maruyama approximate solutions of stochastic differential equations, Monte Carlo Methods Appl., 1 (1995), 165–171. https://doi.org/10.1515/mcma.1995.1.3.165 doi: 10.1515/mcma.1995.1.3.165
![]() |
[20] | S. Kanagawa, S. Ogawa, Numerical solution of stochastic differential equations and their applications, Sugaku Expositions, 18 (2005), 75–100. |
[21] | I. Karatzas, S. E. Shreve, Brownian Motion and Stochastic Calculus, New York: Springer, 1988. https://doi.org/10.1007/978-1-4684-0302-2 |
[22] | R. Z. Khas'miniskii, Stochastic Stability of Differential Equations, Sijthoff and Noerdhoff, 1980. |
[23] | P. E. Kloeden, E. Platen, Numerical Solutions of Stochastic Differential Equations, Berlin: Springer, 1992. https://doi.org/10.1007/978-3-662-12616-5 |
[24] | M. A. Krasnosel'skii, E. A. Lifshits, A. V. Sobolev, Positive Linear Systems: The Method of Positive Operators (in Russian), 1985. |
[25] |
A. Lang, A. Petersson, A. Thalhammer, Mean-square stability analysis of approximations of stochastic differential equations in infinite dimensions, BIT, 57 (2017), 963–990. https://doi.org/10.1007/s10543-017-0684-7 doi: 10.1007/s10543-017-0684-7
![]() |
[26] |
Y. Liu, Y. Niu, X. Cheng, Convergence and stability of the semi-tamed Milstein method for commutative stochastic differential equations with non-globally Lipschitz continuous coefficients, Appl. Math. Comput., 414 (2022), 126680. https://doi.org/10.1016/j.amc.2021.126680 doi: 10.1016/j.amc.2021.126680
![]() |
[27] |
J. C. Mattingly, A. M. Stuart, D. J. Higham, Ergodicity for SDEs and approximations: Locally Lipschitz vector fields and degenerate noise, Stoch. Proc. Appl., 101 (2002), 185–232. https://doi.org/10.1016/S0304-4149(02)00150-3 doi: 10.1016/S0304-4149(02)00150-3
![]() |
[28] | G. N. Milstein, Numerical Integration of Stochastic Differential Equations, Dordrecht: Springer, 1995. https://doi.org/10.1007/978-94-015-8455-5 |
[29] |
G. N. Milstein, E. Platen, H. Schurz, Balanced implicit methods for stiff stochastic differential equations, SIAM J. Numer. Anal., 35 (1998), 1010–1019. https://doi.org/10.1137/s0036142994273525 doi: 10.1137/s0036142994273525
![]() |
[30] | T. Mitsui, Stability analysis of numerical solution of stochastic differential equations, Kokyuroku (Res. Inst. Math. Sci., Kyoto Univ.), 850 (1995), 124–138. |
[31] |
E. Moro, H. Schurz, Boundary preserving semi-analytical numerical algorithms for stochastic differential equations, SIAM J. Sci. Comput., 29 (2007), 1525–1549. https://doi.org/10.1137/05063725X doi: 10.1137/05063725X
![]() |
[32] |
S. Ogawa, Some problems in the simulation of nonlinear diffusion processes, Math. Comput. Simulat., 38 (1995), 217–223. https://doi.org/10.1016/0378-4754(93)E0085-J doi: 10.1016/0378-4754(93)E0085-J
![]() |
[33] | B. Øksendal, Stochastic Differential Equations: An Introduction with Applications, 5 Eds., New York: Springer, 2000. |
[34] |
W. P. Petersen, Some experiments on numerical simulations of stochastic differential equations and a new algorithm, J. Comput. Phys., 113 (1994), 75–81 https://doi.org/10.1006/jcph.1994.1119 doi: 10.1006/jcph.1994.1119
![]() |
[35] |
E. Platen, An introduction to numerical methods for stochastic differential equations, Acta Numer., 8 (1999), 197–246. http://dx.doi.org/10.1017/S0962492900002920 doi: 10.1017/S0962492900002920
![]() |
[36] | P. Protter, Stochastic Integration and Differential Equations, Berlin: Springer, 1990. https://doi.org/10.1007/978-3-662-02619-9 |
[37] |
H. Ranjbar, L. Torkzadeh, D. Baleanu, K. Nouri, Simulating systems of Itô SDEs with split-step (α,β)-Milstein scheme, AIMS Math., 8 (2023), 2576–2590. https://doi.org/10.3934/math.2023133 doi: 10.3934/math.2023133
![]() |
[38] |
V. Reshniak, A. Q. M. Khaliq, D. A. Voss, G. Zhang, Split-step Milstein methods for multi-channel stiff stochastic differential systems, Appl. Numer. Math., 89 (2015), 1–23. https://doi.org/10.1016/j.apnum.2014.10.005 doi: 10.1016/j.apnum.2014.10.005
![]() |
[39] |
A. Rodkina, H. Schurz, Almost sure asymptotic stability of drift-implicit θ-methods for bilinear ordinary stochastic differential equations in R1, J. Comput. Appl. Math., 180 (2005), 13–31. https://doi.org/10.1016/j.cam.2004.09.060 doi: 10.1016/j.cam.2004.09.060
![]() |
[40] | Y. Saito, T. Mitsui, T-stability of numerical scheme for stochastic differential equations, In: R. P. Agarwal, Contributions in Numerical Mathematics, World Scientific Series in Applicable Analysis, 2 (1993), 333–344. https://doi.org/10.1142/9789812798886_0026 |
[41] |
Y. Saito, T. Mitsui, Stability analysis of numerical schemes for stochastic differential equations, SIAM J. Numer. Anal., 33 (1996), 2254–2267. https://doi.org/10.1137/S0036142992228409 doi: 10.1137/S0036142992228409
![]() |
[42] | Y. Saito, T. Mitsui, MS-stability analysis for numerical solutions of stochastic differential equations: Beyond single-step single dim, In: R. Jeltsch, T. T. Li, I. H. Sloan, Some Topics in Industrial and Applied Mathematics, Series in Contemporary Applied Mathematics, 8 (2007), 181–194. https://doi.org/10.1142/9789812709356_0010 |
[43] |
H. Schurz, Asymptotic mean square stability of an equilibrium point of some linear numerical solutions, Stoch. Anal. Appl., 14 (1996), 313–353. https://doi.org/10.1080/07362999608809442 doi: 10.1080/07362999608809442
![]() |
[44] | H. Schurz, Stability, Stationarity, and Boundedness of Implicit Numerical Methods for Stochastic Differential Equations and Applications, Berlin: Logos-Verlag, 1997. |
[45] |
H. Schurz, The invariance of asymptotic laws of linear stochastic systems under discretization, ZAMM-Z. Angew. Math. Me., 79 (1999), 375–382. https://doi.org/10.1002/(SICI)1521-4001(199906)79:6 doi: 10.1002/(SICI)1521-4001(199906)79:6
![]() |
[46] |
H. Schurz, Preservation of probabilistic laws through Euler methods for Ornstein-Uhlenbeck process, Stoch. Anal. Appl., 17 (1999), 463–486. https://doi.org/10.1080/07362999908809613 doi: 10.1080/07362999908809613
![]() |
[47] |
H. Schurz, Moment attractivity, stability, contractivity exponents of non-linear stochastic dynamical systems, Discrete Cont. Dyn.-A, 7 (2001), 487–515. https://doi.org/10.3934/dcds.2001.7.487 doi: 10.3934/dcds.2001.7.487
![]() |
[48] | H. Schurz, On moment-dissipative stochastic dynamical systems, Dynam. Syst. Appl., 10 (2001), 11–44. |
[49] | H. Schurz, Numerical analysis of SDEs without tears, In: V. Lakshmikantham, D. Kannan, Handbook of Stochastic Analysis, Boca Raton: CRC Press, 2002. https://doi.org/10.1201/9781482294705 |
[50] | H. Schurz, Stability of numerical methods for ordinary stochastic differential equations along Lyapunov-type and other functions with variable step sizes, Electron. T. Numer. Ana., 20 (2005), 27–49. |
[51] | H. Schurz, An axiomatic approach to numerical approximations of stochastic processes, Int. J. Numer. Anal. Mod., 3 (2006), 459–480. |
[52] |
H. Schurz, Almost sure asymptotic stability and convergence of stochastic Theta methods applied to systems of linear SDEs in Rd, Random Oper. Stoch. Equ., 19 (2011), 111–129. https://doi.org/10.1515/ROSE.2011.007 doi: 10.1515/ROSE.2011.007
![]() |
[53] | H. Schurz, Basic concepts of numerical analysis of stochastic differential equations explained by balanced implicit theta methods, In: Z. M. Mounir, D. V. Filatova, Stochastic Differential Equations and Processes, Springer Proceedings in Mathematics, Berlin: Springer, 7 (2012), 1–139. https://doi.org/10.1201/9781482294705 |
[54] |
H. Schurz, Numeric and dynamic B-stability, exact-monotone and asymptotic two-point behavior of theta methods for stochastic differential equations, J. Stoch. Anal., 2 (2021), 7. https://doi.org/10.31390/josa.2.2.07 doi: 10.31390/josa.2.2.07
![]() |
[55] | A. N. Shiryaev, Probability, New York: Springer, 1996. https://doi.org/10.1007/978-1-4757-2539-1 |
[56] | A. N. Shiryaev, R. Sh. Liptser, Theory of Martingales, Dordrecht: Springer, 1989. https://doi.org/10.1007/978-94-009-2438-3 |
[57] | D. Talay, Simulation of stochastic differential systems, In: P. Krée, W. Wedig, Probabilistic Methods in Applied Physics, Springer Lecture Notes in Physics, Berlin: Springer, 451 (1995), 54–96. xa https://doi.org/10.1007/978-94-009-2438-3 |
[58] | D. Talay, Simulation of stochastic processes and applications, In: R. D. Devore, A. Iserles, E. S Süli, Foundations of Computational Mathematics, Cambridge: Cambridge University Press, 284 (2001), 345–360. https://doi.org/10.1017/CBO9781107360198.013 |
[59] | D. Talay, Stochastic Hamiltonian systems: Exponential convergence to the invariant measure, and discretization by the implicit Euler scheme, Markov Process. Relat., 8 (2002), 163–198. |
[60] |
A. Tocino, M. J. Senosiain, Mean-square stability analysis of numerical schemes for stochastic differential systems, J. Comput. Appl. Math., 236 (2012), 2660–2672. https://doi.org/10.1016/j.cam.2012.01.002 doi: 10.1016/j.cam.2012.01.002
![]() |
[61] |
X. Wang, S. Gan, The tamed Milstein method for commutative stochastic differential equations with non-globally Lipschitz continuous coefficients, J. Differ. Equ. Appl., 19 (2013), 466–490. https://doi.org/10.1080/10236198.2012.656617 doi: 10.1080/10236198.2012.656617
![]() |
[62] |
L. Weng, W. Liu, Invariant measures of the Milstein method for stochastic differential equations with commutative noise, Appl. Math. Comput., 358 (2019), 169–176. https://doi.org/10.1016/j.amc.2019.04.049 doi: 10.1016/j.amc.2019.04.049
![]() |
[63] |
Z. Yin, S. Gan, An improved Milstein method for stiff stochastic differential equations, Adv. Differ. Equ.-NY, 2015 (2015), 369. https://doi.org/10.1186/s13662-015-0699-9 doi: 10.1186/s13662-015-0699-9
![]() |
[64] |
R. Yue, Q. Guo, W. Liu, X. Mao, The truncated Milstein method for stochastic differential equations with commutative noise, J. Comput. Appl. Math., 338 (2018), 298–310. https://doi.org/10.1016/j.cam.2018.01.014 doi: 10.1016/j.cam.2018.01.014
![]() |
Detection module | Stride | Anchor (width) | Aspect ratio |
FDM5 | 4 | 121,620 | 1:1 |
FDM4 | 8 | 243,240 | 1:1 |
FDM3 | 16 | 486,480 | 1:1.5 |
FDM2 | 32 | 96,128,160 | 1:1.5 |
FDM1 | 64 | 192,256,320 | 1:1.5 |
Face detection network | Number of face photos (piece) | Number of recognized faces (piece) | Recognition rate (%) |
Yolov5s | 21,860 | 21,488 | 98.3% |
Ours | 21,860 | 21,751 | 99.5% |
Name | Params | Mean error | Failure rate |
ResNest-50 | 122.27 M | 0.046 | 0.038 |
MobileNetV2_0.25 | 1.09 M | 0.075 | 0.174 |
MobileNetV2_1.00 | 7.28 M | 0.065 | 0.127 |
HRNetV2 | 545.07 M | 0.066 | 0.125 |
EfficientNet-B0 | 16.67 M | 0.064 | 0.119 |
EfficientNet-B1 | 26.37 M | 0.075 | 0.149 |
EfficientNet-B2 | 30.85 M | 0.071 | 0.145 |
EfficientNet-B3 | 42.29 M | 0.099 | 0.136 |
EfficientNet-B4 | 68.34 M | 0.077 | 0.164 |
EfficientNet-B5 | 109.34 M | 0.094 | 0.173 |
EfficientNet-B6 | 156.34 M | 0.081 | 0.175 |
EfficientNet-B7 | 244.03 M | 0.081 | 0.196 |
Fatigue characteristic parameters | Accuracy rate (%) |
E + pitch + yaw + roll | 93.26 |
M + pitch + yaw + roll | 90.13 |
H + pitch + yaw + roll | 86.76 |
E + M + H + pitch + yaw + roll | 97.32 |
Module | Head Posture Feature | Backbone | Accuracy | Params (M) | Flops (G) |
DSFD | × | MobileNetV2_0.25 | 86.25 | 10.26 | 22.55 |
RetinaFace | × | MobileNetV2_0.25 | 87.78 | 8.43 | 18.34 |
PFLD (no auxiliary) | × | MobileNetV2_0.25 | 88.64 | 7.68 | 16.92 |
PFLD | √ | MobileNetV2_0.25 | 97.32 | 8.21 | 17.53 |
Detection module | Stride | Anchor (width) | Aspect ratio |
FDM5 | 4 | 121,620 | 1:1 |
FDM4 | 8 | 243,240 | 1:1 |
FDM3 | 16 | 486,480 | 1:1.5 |
FDM2 | 32 | 96,128,160 | 1:1.5 |
FDM1 | 64 | 192,256,320 | 1:1.5 |
Face detection network | Number of face photos (piece) | Number of recognized faces (piece) | Recognition rate (%) |
Yolov5s | 21,860 | 21,488 | 98.3% |
Ours | 21,860 | 21,751 | 99.5% |
Name | Params | Mean error | Failure rate |
ResNest-50 | 122.27 M | 0.046 | 0.038 |
MobileNetV2_0.25 | 1.09 M | 0.075 | 0.174 |
MobileNetV2_1.00 | 7.28 M | 0.065 | 0.127 |
HRNetV2 | 545.07 M | 0.066 | 0.125 |
EfficientNet-B0 | 16.67 M | 0.064 | 0.119 |
EfficientNet-B1 | 26.37 M | 0.075 | 0.149 |
EfficientNet-B2 | 30.85 M | 0.071 | 0.145 |
EfficientNet-B3 | 42.29 M | 0.099 | 0.136 |
EfficientNet-B4 | 68.34 M | 0.077 | 0.164 |
EfficientNet-B5 | 109.34 M | 0.094 | 0.173 |
EfficientNet-B6 | 156.34 M | 0.081 | 0.175 |
EfficientNet-B7 | 244.03 M | 0.081 | 0.196 |
Fatigue characteristic parameters | Accuracy rate (%) |
E + pitch + yaw + roll | 93.26 |
M + pitch + yaw + roll | 90.13 |
H + pitch + yaw + roll | 86.76 |
E + M + H + pitch + yaw + roll | 97.32 |
Module | Head Posture Feature | Backbone | Accuracy | Params (M) | Flops (G) |
DSFD | × | MobileNetV2_0.25 | 86.25 | 10.26 | 22.55 |
RetinaFace | × | MobileNetV2_0.25 | 87.78 | 8.43 | 18.34 |
PFLD (no auxiliary) | × | MobileNetV2_0.25 | 88.64 | 7.68 | 16.92 |
PFLD | √ | MobileNetV2_0.25 | 97.32 | 8.21 | 17.53 |
Literature | Algorithm | Accuracy rate (%) |
[20] | LSTMs | 89.23 |
[21] | Haar + ELM | 92.71 |
[22] | RF-DCM | 90.12 |
[23] | MSP-Net | 93.69 |
[24] | HOG-SVM | 94.04 |
[25] | FDRNet | 94.61 |
[26] | GFDN | 94.88 |
Ours | Our method | 97.32 |
Literature | Algorithm | Accuracy rate (%) |
[20] | LSTMs | 92.71 |
[21] | Haar + ELM | 93.90 |
[22] | RF-DCM | 89.42 |
[23] | MSP-Net | 95.36 |
[24] | HOG-SVM | 96.40 |
[25] | FDRNet | 98.96 |
[26] | GFDN | 97.06 |
Ours | Our method | 97.05 |