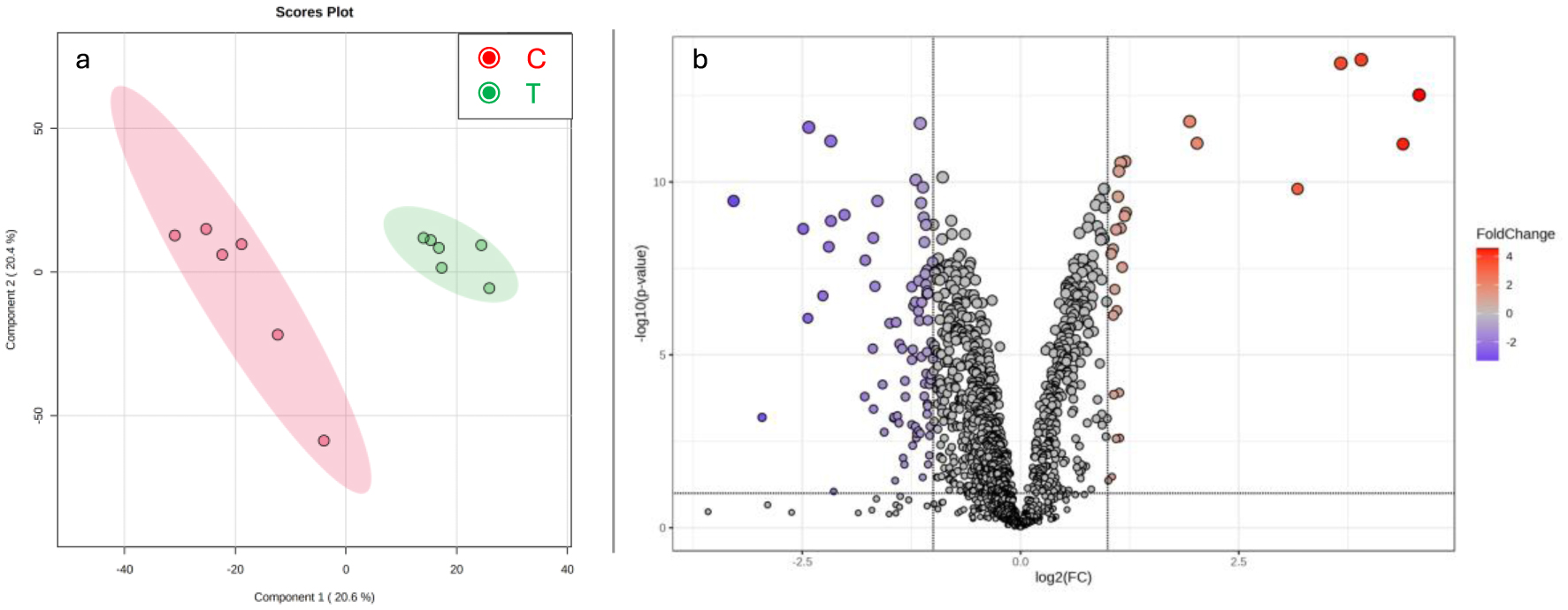
Neural tube defects (NTDs) are common congenital anomalies formed by the incomplete closure of the neural tube during early embryonic development. This study aims to identify potential plasma biomarkers associated with NTDs using an untargeted metabolomics approach, thereby enhancing the understanding of the disease pathogenesis and facilitating early diagnosis. Peripheral venous blood samples were obtained from 21 healthy controls and 20 individuals diagnosed with NTDs. Untargeted metabolomic profiling was performed using Quadrupole Time-of-Flight Liquid Chromatography Mass Spectrometry (Q-TOF LC/MS) in positive ion mode. Data processing was conducted using the XCMS package in R, thus identifying significant metabolites based on a fold change greater than 1.5 and a p-value less than 0.05. The analysis revealed significant alterations in several metabolites in NTD cases compared to the healthy controls. Notably, changes were observed in arachidonic acid and prostaglandins (lipid metabolism), choline (methylation processes), amino acids such as leucine, phenylalanine, and lysine (amino acid metabolism), thyrotropin-releasing hormone, vitamin D3, cob(II)alamin, and galactosylsphingosine (sphingolipid metabolism). The identified metabolites may play a role in the pathogenesis of NTDs and hold potential as early diagnostic biomarkers.
Citation: Şule Özel, Ozan Kaplan, Bilge Başak Fidan, Mustafa Çelebier, Yaprak Engin-Üstün. Altered plasma levels of arachidonic acid, choline, amino acids, and sphingolipids signal neural tube defects[J]. AIMS Molecular Science, 2025, 12(1): 99-112. doi: 10.3934/molsci.2025006
[1] | Stephen Schultz, Dario Siniscalco . Endocannabinoid system involvement in autism spectrum disorder: An overview with potential therapeutic applications. AIMS Molecular Science, 2019, 6(1): 27-37. doi: 10.3934/molsci.2019.1.27 |
[2] | Chisato Kinoshita, Koji Aoyama, Toshio Nakaki . microRNA as a new agent for regulating neuronal glutathione synthesis and metabolism. AIMS Molecular Science, 2015, 2(2): 124-143. doi: 10.3934/molsci.2015.2.124 |
[3] | Francisco dos Santos Farias, Vicente Paulo da Costa Neto, Cleriston Correia da Silva Souza, Daniela Vieira Chaves, Aurenivia Bonifácio . Differential metabolic rearrangements improve biomass and enhance the tolerance of two dwarf cashew (Anacardium occidentale L.) genotypes to salt stress. AIMS Molecular Science, 2024, 11(2): 206-220. doi: 10.3934/molsci.2024012 |
[4] | Navid J. Ayon . Features, roles and chiral analyses of proteinogenic amino acids. AIMS Molecular Science, 2020, 7(3): 229-268. doi: 10.3934/molsci.2020011 |
[5] | Davide Lovisolo, Marianna Dionisi, Federico A. Ruffinatti, Carla Distasi . Nanoparticles and potential neurotoxicity: focus on molecular mechanisms. AIMS Molecular Science, 2018, 5(1): 1-13. doi: 10.3934/molsci.2018.1.1 |
[6] | Geovanni Alberto Ruiz-Romero, Carolina Álvarez-Delgado . Effects of estrogens in mitochondria: An approach to type 2 diabetes. AIMS Molecular Science, 2024, 11(1): 72-98. doi: 10.3934/molsci.2024006 |
[7] | Teresa M. Fonovich . Phospholipid synthetic and turnover pathways elicited upon exposure to different xenobiotics. AIMS Molecular Science, 2020, 7(3): 211-228. doi: 10.3934/molsci.2020010 |
[8] | Yutaka Takihara, Ryuji Otani, Takuro Ishii, Shunsuke Takaoka, Yuki Nakano, Kaori Inoue, Steven Larsen, Yoko Ogino, Masashi Asai, Sei-ichi Tanuma, Fumiaki Uchiumi . Characterization of the human IDH1 gene promoter. AIMS Molecular Science, 2023, 10(3): 186-204. doi: 10.3934/molsci.2023013 |
[9] | Amena W. Smith, Swapan K. Ray, Arabinda Das, Kenkichi Nozaki, Baerbel Rohrer, Naren L. Banik . Calpain inhibition as a possible new therapeutic target in multiple sclerosis. AIMS Molecular Science, 2017, 4(4): 446-462. doi: 10.3934/molsci.2017.4.446 |
[10] | Irene M. Waita, Atunga Nyachieo, Daniel Chai, Samson Muuo, Naomi Maina, Daniel Kariuki, Cleophas M. Kyama . Genetic polymorphisms in eostrogen and progesterone receptor genes in Papio anubis induced with endometriosis during early stage of the disease. AIMS Molecular Science, 2021, 8(1): 86-97. doi: 10.3934/molsci.2021007 |
Neural tube defects (NTDs) are common congenital anomalies formed by the incomplete closure of the neural tube during early embryonic development. This study aims to identify potential plasma biomarkers associated with NTDs using an untargeted metabolomics approach, thereby enhancing the understanding of the disease pathogenesis and facilitating early diagnosis. Peripheral venous blood samples were obtained from 21 healthy controls and 20 individuals diagnosed with NTDs. Untargeted metabolomic profiling was performed using Quadrupole Time-of-Flight Liquid Chromatography Mass Spectrometry (Q-TOF LC/MS) in positive ion mode. Data processing was conducted using the XCMS package in R, thus identifying significant metabolites based on a fold change greater than 1.5 and a p-value less than 0.05. The analysis revealed significant alterations in several metabolites in NTD cases compared to the healthy controls. Notably, changes were observed in arachidonic acid and prostaglandins (lipid metabolism), choline (methylation processes), amino acids such as leucine, phenylalanine, and lysine (amino acid metabolism), thyrotropin-releasing hormone, vitamin D3, cob(II)alamin, and galactosylsphingosine (sphingolipid metabolism). The identified metabolites may play a role in the pathogenesis of NTDs and hold potential as early diagnostic biomarkers.
Birth defects constitute a significant global health issue, often arising from unknown causes or a combination of factors [1]. In human embryonic development, the neural tube originates from brain tissue and extends downward to form the spinal cord. This structure completes its development between the second and third weeks after fertilization. Disruptions in its development, for any reason, can cause portions of the tube to remain open, thus leading to neural tube defects (NTDs) [2]. They are both common and critical and impact numerous newborns worldwide. For instance, Tan et al. reported a birth defect prevalence of 23.99 cases per 1000 live births in Singapore between 1994 and 2000 [3]. This study also highlighted an increasing trend in terminations due to birth defects, accompanied by a decreasing trend in congenital anomalies among live births [3].
Busby et al. reported that 8913 babies or fetuses with NTDs (anencephaly, spina bifida, or encephalocele) were identified among 9,273,212 births across 16 countries between 1980 and 2001 [4]. The prevalence of NTDs decreased in the United Kingdom and Ireland from 45 per 10,000 births in the 1980s to 10–15 per 10,000 in the 1990s. However, in the rest of Europe, the prevalence remained close to 10 per 10,000 births during the same period [4]. To prevent NTDs, some governments have implemented public health policies promoting folic acid supplementation [5]. Although the pathogenesis of NTDs and their relationship with folate metabolism are well understood [6], early diagnoses have evolved from techniques such as amniography [7] and ultrasound [8] to identifying biomarkers for prenatal diagnoses of NTDs based on omic techniques [9].
Advancements in analytical technologies, particularly mass spectrometry (MS) and nuclear magnetic resonance (NMR) spectroscopy, have propelled metabolomics to the forefront of systems biology. Metabolomics can be broadly divided into targeted and untargeted approaches. Targeted metabolomics quantifies a predefined set of metabolites, which are often related to a specific pathway or disease. In contrast, untargeted metabolomics aims to detect and quantify as many metabolites as possible without prior selection, making it particularly valuable for discovering novel biomarkers and identifying unexpected metabolic alterations linked to diseases [10],[11].
In this paper, we aim to identify significant metabolic differences that may provide insights into the pathogenesis of NTDs by analyzing and comparing the plasma metabolite profiles of 21 healthy controls and 20 individuals with NTDs. Identifying altered metabolites will enhance our understanding of metabolic disruptions associated with NTDs and contribute to the development of non-invasive biomarkers for early detection. Ultimately, these findings may facilitate early diagnoses and individuals affected by this condition. This study aims to identify potential plasma biomarkers for the diagnosis of NTDs using an untargeted metabolomics approach with Quadrupole Time-of-Flight Liquid Chromatography Mass Spectrometry (Q-TOF LC/MS).
This single-center, prospective study enrolled pregnant women and healthy controls aged 20-40 years. All the participants were examined at the Zekai Tahir Burak Women's Health, Health Application, and Research Center, which is affiliated with the Ministry of Health of the Republic of Türkiye. Ethical approval was obtained from the institutional review board of the hospital, and informed consent was obtained from all participants before inclusion in the study. The study population consisted of 21 healthy control samples (Group C) and 20 plasma samples from cases diagnosed with NTDs (Group T). The study population was determined based on specific inclusion and exclusion criteria to ensure the reliability and validity of the findings.
The inclusion criteria are as follows: the study included second trimester pregnant women aged between 20 and 40 years who had a diagnosis of NTD in the fetus.
The exclusion criteria are as follows: the study excluded pregnant women with genetic or systemic diseases or on medication, a history of metabolic or autoimmune diseases before pregnancy, pregnancies that resulted from in vitro fertilization or intracytoplasmic sperm injection, multiple pregnancies, women who had previous pregnancies affected by NTDs or complicated pregnancies, individuals unwilling to participate in the study, and participants with incomplete or insufficient clinical data.
All the participants provided blood samples in a fasting state during their routine hospital visits, thereby minimizing the potential influence of immediate nutritional intake on their metabolomic profiles. Given the numerous factors which influence metabolomic levels, the nutritional status of the participants was not considered a primary confounder, as all groups were selected to ensure a homogeneous study population.
Peripheral venous blood (3 mL) was drawn from each participant into tubes containing ethylenediaminetetraacetic acid (EDTA) to prevent coagulation. The samples were centrifuged at 3000 rpm for 10 minutes to separate the plasma. The plasma samples were immediately frozen at −80 °C until the analysis to preserve the metabolite integrity. On the day of the experiment, the plasma samples were thawed at room temperature. For each group (Groups C and T), 200 µL of the individual plasma samples were pooled into 15 mL Falcon conical tubes (Fisher Scientific, New Hampshire, USA) and thoroughly vortexed to ensure homogeneity. The pooled samples served as quality control (QC) samples, which monitor the consistency and reliability of the analytical process. Both the pooled and individual samples underwent metabolite extraction through protein precipitation using methanol. Specifically, 200 µL of plasma was mixed with 600 µL of methanol in an Eppendorf tube and vortexed to ensure complete mixing. The mixture was centrifuged at 9000 rpm at 4 °C for 30 minutes to pellet the precipitated proteins. The supernatant, which contained the metabolites, was carefully collected and dried using a vacuum centrifuge (9 °C, Labconco CentriVap Benchtop Vacuum Concentrator, Kansas City, USA). The dried extracts were reconstituted in 300 µL of a 1:1 (v/v) mixture of acetonitrile and water. All solvents used were LC-MS grade (Sigma-Aldrich, Missouri, USA) to ensure high purity for mass spectrometry analysis.
A mass spectrometry analysis was performed using an Agilent 6530 Q-TOF LC/MS system (Agilent Technologies, Santa Clara, CA). The mass spectrometer operated in positive ion mode, thus enhancing the ionization of most metabolites. The MS scan range was set between 100 and 1700 m/z to capture a broad spectrum of low-molecular-weight metabolites. The instrument parameters included a column temperature of 35 °C, a drying gas temperature of 350 °C, and a capillary voltage of 4000 V. The auto MS/MS mode was employed for metabolite identification and to facilitate structural elucidation.
A chromatographic separation was performed using an Agilent Zorbax C18 column (1.8 µm, 50 x 2.1 mm). It is widely used for metabolomics due to its high resolution and efficiency in separating a broad range of metabolites. A gradient elution method was employed, thereby utilizing a mobile phase comprised of water (solvent A) and acetonitrile (ACN, solvent B), both containing 0.1% formic acid to enhance the ionization efficiency. The gradient program began with 10% ACN at a flow rate of 0.20 mL/min, which was linearly increased to 90% ACN by the 10th minute. After reaching this maximum, the ACN ratio was gradually reduced to 10% by the 17th minute. The composition was maintained at 10% ACN until the 25th minute, followed by a 5-minute post-run to ensure that the column was ready for subsequent injections. All samples were injected in duplicate, and the injection order was randomized to mitigate any potential instrument drift or batch effects during the analysis. Using a C18 column and gradient elution with acetonitrile ensures the practical separation of both polar and non-polar metabolites, thus providing a comprehensive metabolomic profiling. This method, with optimized flow rates and gradient timings, aligns with the best practices in metabolomics literature for achieving high reproducibility and resolution. Then, the raw data were processed using XCMS.
Peak detection, alignment, and grouping were performed using the XCMS package in the R programming environment, which is widely utilized for processing untargeted metabolomics data [12]. To optimize the parameters for peak picking and grouping in XCMS, we employed the Isotopologue Parameter Optimization (IPO) software. IPO automates the optimization process by utilizing the design of experiments and natural selection algorithms to fine-tune XCMS parameters specific to our dataset [13]. After processing with XCMS, the resulting peak lists were exported to Microsoft Excel for a further analysis. To ensure the reliability of the detected peaks and to eliminate false positives, we conducted a regression analysis based on the consecutive dilution series prepared from the pooled QC samples. Specifically, we examined the correlation between the peak intensities (y) and the corresponding dilution factors (x) as reported in previous studies [14]. Peaks that exhibited a strong linear relationship with the dilution factors (R² > 0.90) were considered reliable and were retained for a further analysis, as they demonstrated consistent behaviors proportional to the dilutions [14]. Peaks that did not meet this criterion were discarded to reduce the likelihood of including artifacts or inconsistent signals. The peak intensities were normalized using the total ion current (TIC) normalization method, which involves dividing each peak area by the sum of all peak areas in that sample. This approach compensates for variations in the sample concentration and the instrument response, thereby enhancing the data comparability across samples [15]. Then, the normalized data were subjected to a statistical analysis to identify the significant differences between the NTD cases and the healthy controls.
The statistical evaluations were conducted using a univariate analysis. A two-tailed Student's t-test was applied to compare the mean metabolite levels between the two groups. Metabolites that exhibited a fold change (FC) greater than 1.5 and a p-value less than 0.05 were considered significantly altered. These thresholds commonly strike a balance between the sensitivity and the specificity in detecting meaningful changes. Then, the significantly altered metabolites were annotated and identified using MetaboAnalyst 5.0, which is an online tool that facilitates metabolomic data analyses, metabolite identification, and pathway mapping [16]. By combining rigorous data processing methods with statistical analyses grounded in established metabolomics practices, we aimed to ensure the reliability and biological relevance of the metabolites identified as potential biomarkers for NTDs. This comprehensive approach enhances the validity of our findings and aligns with the best practices in the field.
From a clinical perspective, the metabolomic profiling of plasma samples from the NTD cases (Group T) and the healthy controls (Group C) revealed significant metabolic alterations. The Principal Component Analysis (PCA) Plot in Figure 1a visualizes the metabolic differences between the NTD cases (Group T) and the healthy controls (Group C). The clear separation between Group T (green) and Group C (red) shows distinct metabolic profiles between the two groups, thus indicating a significant alteration in the metabolic pathways associated with NTD. The shaded regions further emphasize the clustering within each group, with minimal overlap, thus suggesting the robustness of the metabolomic differences. The volcano plot in Figure 1b shows the log2 fold change (FC) of metabolites between the NTD cases and the controls plotted against the -log10 p-value. Metabolites with higher fold changes are located to the right (positive values), while those with reduced levels in the NTD cases are to the left (negative values). The color scale indicates the magnitude of the fold change, with red representing highly upregulated metabolites in the NTD cases and blue representing downregulated ones. Statistically significant metabolites (FC > |1.5| or log2 FC > |0.585|, p < 0.05) are located in the outer regions of the plot. These metabolites are potential biomarkers for NTD and warrant further investigation.
A comprehensive analysis detected 3558 distinct peaks across the study population. Of these, 386 metabolites exhibited statistically significant differences between the NTD cases and the controls, with a fold change (FC) greater than 1.5 and a p-value less than 0.05. These findings indicate the presence of specific metabolic disruptions in the NTD cases, which may have potential diagnostic and prognostic values. The putatively identified metabolites implicated in key metabolic pathways such as lipid metabolism, amino acid metabolism, and sphingolipid biosynthesis are listed in Table 1. This data suggests a potential role for these metabolites in the pathogenesis of NTDs, thus highlighting their relevance as possible biomarkers for early diagnoses or risk stratification in clinical settings.
Metabolite | Matched Form | HMDB code | |
1 | Choline | M[1+] | HMDB0000097 |
2 | L-Leucine or L-Isoleucine | M-NH3+H[1+] | HMDB0000687 |
3 | L-Phenylalanine | M-HCOOH+H[1+] | HMDB0000159 |
4 | L-Lysine | M-NH3+H[1+] | HMDB0000182 |
5 | Thyrotropin-releasing hormone | M+2H[2+] | HMDB0060080 |
6 | N6-Acetyl-L-lysine | M+Na[1+] | HMDB0000206 |
7 | Prostaglandin D2, E2, A2, J2, or B2 | M-H4O2+H[1+] | HMDB0001403 |
8 | Arachidonic acid | M+Na[1+] | HMDB0001043 |
9 | Aspartylglycosamine | M+H[1+] | HMDB0000489 |
10 | Vitamin D3 (Calcitriol) | M-HCOOH+H[1+] | HMDB0001903 |
11 | Adenylsuccinic acid | M-CO2+H[1+] | HMDB0000536 |
12 | Galactosylsphingosine | M-H4O2+H[1+] | HMDB0000648 |
13 | Delta 8,14-Sterol | M+NaCl[1+] | HMDB0006928 |
14 | LysoPC(O-18:0) | M[1+] | HMDB0011149 |
15 | Estriol-16-Glucuronide | M+HCOONa[1+] | HMDB0006766 |
16 | Coproporphyrin I or II | M[1+] | HMDB0000643 |
17 | Ganglioside GA2 (d18:1/12:0) | M-CO+H[1+] | HMDB0004888 |
18 | Galabiosylceramide (d18:1/9Z-18:1) | M+NaCl[1+] | HMDB0004832 |
19 | Cob(II)alamin | M-H2O+H[1+] | HMDB0006316 |
A study published by Dheen et al. about NTD overviewed the relationship between NTD and embryos during diabetic pregnancies [17]. Jawerbaum and Gonzalez reported that hyperglycemia can provoke cellular damage by increasing the production of reactive oxygen species (ROS), altering the arachidonic acid metabolism, and enhancing the generation of nitric oxide [18]. In our experiments, the levels of two metabolites (arachidonic acid and prostaglandin) involved in the arachidonic acid metabolism were significantly altered between Group T and Group C. Some early studies promote the use of Myo-inositol and prostaglandins to reverse the glucose inhibition of neural tube fusion in cultured mouse embryos, as well as the relationship between diabetes mellitus and prostaglandin E2 levels [19]–[21]. Recent studies also confirm the usage of inositol for the prevention of NTDs [22].
In a study published by Mills et al., it was reported that maternal betaine and choline concentrations are not strongly associated with NTD risk since the mean plasma total choline concentrations in the NTD and control groups did not significantly differ [23]. However, Shaw et al. reported that the dietary choline intake was associated with reduced NTD risks [24]. In our experiments, we observed a significant difference (FC: 1.80) between Group C and T. Therefore, the choline concentration in the plasma must be evaluated in different populations to highlight the importance of choline usage in pregnancy or this unique metabolite as a biomarker for NTD.
L-Leucine is a branched-chain amino acid, and it is mainly involved in stress, energy, and muscle metabolism. In an early study published by Rosenquist et al, it was reported that avian embryos treated with leucine experienced normal neural tube closure including the lumbar region [25]. Although the changes in the concentration of such amino acids are related to many other disease conditions, the difference in the leucine or iso-leucine concentration between the groups (FC: 1.73) in our experimental model encourages us to consider the leucine or iso-leucine concentration as a predictor of NTD. Leucine's regulatory role in cellular growth through mTORC1 suggests that it may act as a critical factor during neural tube development by influencing the proliferation and differentiation of embryonic cells [26]. The concentrations of the other amino acids, phenylalanine and lysine, were altered by FC: 1.61 and FC: 2.05, respectively. Denno et al. reported that excess phenylalanine has been shown to increase neural tube openings during embryo development. Particularly high levels of phenylalanine may contribute to NTD development by altering the neurotransmitter levels and disrupting the developmental signaling pathways [27]. They conducted a study on the amino acid levels in the plasma and amniotic fluid of women with a genetic risk of embryo developmental impairments and a previous history of aberrant chromosomal segregation or NTDs. In these women, the plasma levels of proline, leucine, and valine were increased by more than 50%. In the amniotic fluid samples from the NTD group, the levels of lysine, serine, and leucine were decreased in women over 35 years of age [28],[29]. These results were correlated with ours.
Abnormalities in the thyroid hormone and prolactin were reported in NTD [30]. The thyrotropin-releasing hormone levels for working groups (might. Groups C and T) differed (FC: 1.81), and this result may be considered in further clinical studies. The maternal serum vitamin D levels in pregnancies complicated by NTDs and in healthy pregnant women were compared, and the mean maternal serum vitamin D3 level was 6.2 ± 5.0 ng/mL in the study group and 9.1 ± 7.3 ng/mL in the control group (p = 0.071) [31]. In our experimental population, the results were similar to the reported ones, but with a higher fold change (FC: 3.94), consistent with the reported ones. In such a situation, vitamin D3 cannot be proposed as a biomarker; however, it should be considered as a parameter to understand the pathogenesis of the disease and prevent NTD.
In a study published by Groenen et al., 1H NMR spectroscopy of amniotic fluids from spina bifida fetuses and controls was examined. Succinic acid, glutamine, creatine, and creatinine were verified as potential biomarkers based on the 1H NMR results [32]. In the study conducted by Zheng et al., a combined ultra-high performance liquid chromatography with quadrupole time-of-flight mass spectrometry (UPLC-Q-TOF-MS) and gas chromatography-time of flight mass spectrometry (GC–TOF MS) approach was used to analyze the serum metabolome of mothers with NTDs, thus revealing significant alterations in tricarboxylic acid (TCA) cycle metabolites. In the first trimester, the succinic acid level was found to be increased (FC = 1.50; P = 0.0762) in the NTD group; however, this increase was not statistically significant. In contrast, the metabolic product of succinic acid and fumaric acid was significantly decreased (FC = 0.56; P < 0.05). Similarly, in the same study, a dibutyl phthalate (DBP)-induced animal model also showed an increase in the succinic acid levels (FC = 1.70; P < 0.05) and a decrease in the fumaric acid levels (FC = 0.63; P < 0.05). DBP is known to be an inhibitor of succinate dehydrogenase (SDH), and the inhibition of this enzyme has been reported to cause impairments in the mitochondrial energy production [33]. In our Q-TOF LC/MS results, the adenylsuccinic acid level was found to be different between the test and control groups. Prospective cohort studies should be conducted in large patient groups to clinically validate these biomarkers and determine the specific changes in the adenylsuccinic acid and vitamin D levels in individuals with NTDs. Since succinic acid is an intermediate product of the TCA cycle, its direct measurement may not fully reflect the overall metabolic processes. Therefore, the evaluation of the succinic acid levels should be considered in conjunction with other metabolites of the TCA cycle and within the appropriate context. For this purpose, targeted metabolomic analyses and enzyme-linked biochemical assays can be utilized. Additionally, to assess the sensitivity and specificity of these biomarkers, diagnostic accuracy analyses should be performed using statistical methods, such as a Receiver Operating Characteristic (ROC) curve analysis, in conjunction with metabolomic analyses.
The relationship between vitamin B12 estimated deficiency and NTD have been well-established for a long time [34]. According to the Human Metabolome Database (HMDB), cob(II)alamin, also known as vitamin B12 or co(i)-cobalamine, falls within the class of organic compounds referred to as cobalamin derivatives. In the present work, the cob(II)alamin concentrations were statistically different between the groups. The vitamin B12 insufficiency and cob(II)alamin plasma concentration may be correlated to monitor the disease progression or to gain a deeper understanding of the disease pathogenesis.
In this context, examining changes in sphingolipid metabolism products may help better understand the mechanisms of NTD formation and contribute to the development of early diagnostic and preventive strategies. Sphingolipid metabolism plays a critical role in the successful implantation of the embryo into the uterus [35]. Sphingolipids have been demonstrated to regulate various processes, including angiogenesis, cell migration, apoptosis, immunomodulation, and cellular differentiation. Additionally, sphingolipids are crucial bioactive molecules involved in cellular signaling, membrane integrity, and apoptosis, all of which are essential for embryonic development [36]. Disruptions in the sphingolipid metabolism have been associated with various developmental disorders, including congenital NTDs [37],[38]. The essential role of sphingosine kinases in neural and vascular development, as well as the role of sphingosine 1-phosphate in neural signaling and function, have been reported in previous studies [39],[40]. In an eye-catching study published in 1999, it was reported that some mycotoxin called fumonisins caused NTD in South Texas [41]. As we know from published results, fumonisin B1 inhibits sphingosine (sphinganine) N-acyltransferase and de novo sphingolipid biosynthesis [42]. Interestingly, the galactosylsphingosine concentration in our study population differed between the groups (FC: 2.48). Therefore, we propose that galactosylsphingosine may be regarded as a biomarker for NTD; however, targeted metabolomic studies on an expanded population, in addition to clinical evaluation for group homogeneity, must be performed to verify this hypothesis. In a study published by Liu et al., it was observed that the detected glycerolipids, glycerophospholipids, sphingolipids, and carboxylic acids were different in polycystic ovary syndrome. The follicular dynamics of glycerophospholipid and sphingolipid metabolisms were discussed [43]. Galabiosylceramide and ganglioside are sphingolipids, and in the present study, their concentrations were determined to be different among the groups, except for galactosylsphingosine concentration. These findings suggest that the sphingolipid metabolism could be a valuable target to identify biomarkers associated with NTDs, as an untargeted approach, such as the one used in the present study, is not capable of screening the entire sphingolipid metabolism under experimental conditions.
Since the 1940s, it has been recognized that teratogens and vitamin deficiencies can trigger birth defects [44]. A well-known example affects the impact of vitamin A deficiencies on maternal and child health [45]. While vitamin A is an essential dietary nutrient, the excessive intake of retinoids by pregnant women can lead to teratogenesis [46]. Similarly, vitamin D is crucial not only for bone health but also for a wide range of physiological functions within the body. Fetal skeletal growth requires additional calcium and increased maternal vitamin D support during pregnancy, underscoring the longstanding recognition of vitamin D and micronutrients, such as calcium, as vital during gestation [47],[48].
Recent studies have confirmed the role of folic acid in preventing NTDs, as well as the association between folate and clinical vitamin B12 deficiencies with infertility or recurrent spontaneous abortion [49]. The role of vitamin deficiencies in birth defects has long been recognized. In particular, folic acid and vitamin B12 deficiencies have been associated with NTDs. In addition to vitamin deficiencies, studies on the metabolic effects of NTDs have suggested a potential link between NTDs, lipid metabolism, and steroid hormone biosynthesis.
In our experiments, some metabolites (HMDB codes are as follows: HMDB0006928, HMDB0011149, HMDB0006766, HMDB0000643, HMDB0004888, and HMDB0004832) listed in Table 1 matched with the peaks under the experimental conditions and were involved in lipid metabolism and steroid hormone biosynthesis. These results must be logically considered to answer the question of whether NTD causes problems in steroid hormone biosynthesis or whether some secondary effects of having problems in steroid hormone biosynthesis can lead to NTD.
Cob(II)alamin (FC: 1.68 in our experimental conditions), an inactive form of vitamin B12, plays a crucial role in and the conversion of homocysteine to methionine. This pathway is essential for DNA synthesis and methylation, and disruptions in it have been linked to NTD.
The findings suggest significant changes in the arachidonic acid and prostaglandin levels in NTDs, thus indicating their potential as early diagnostic biomarkers. Inositol and prostaglandins could mitigate glucose inhibition of neural tube fusion. Variations in choline and amino acids, such as leucine, phenylalanine, and lysine, were noted, thus suggesting their potential role in the risk for NTD. Thyroid hormone and vitamin D3 levels also significantly differed. Cob(II)alamin's role in homocysteine metabolism highlights its importance. Sphingolipid metabolism, especially galactosylsphingosine, may also be relevant. These metabolites could serve as biomarkers, but further targeted studies are needed for validation.
In light of the findings from the study of Nasri et al. [50], which emphasized the roles of docosahexaenoic acid (DHA), eicosapentaenoic acid (EPA), and arachidonic acid (ARA) in NTDs, our results further underscore the importance of lipid metabolism in NTD pathogenesis. Similar to their observations of altered ARA levels, we identified significant changes in metabolites associated with arachidonic acid metabolism, including prostaglandins, thus reinforcing the hypothesis that disruptions in fatty acid metabolism may be a key contributor to the risk of NTD. They highlight the interaction between lipid metabolism and essential micronutrients, such as folate and vitamin D. This finding parallels our results, which show variations in the choline and vitamin D3 levels between the NTD cases and the controls. This connection between lipid metabolism and nutrient deficiencies adds another layer of complexity to the metabolic processes underlying NTDs.
Our study has several limitations that should be acknowledged. First, the small sample size reduces the statistical power and may limit the generalizability of the findings. Therefore, our data should be viewed as preliminary or hypothesis-generating rather than conclusive. Second, although we employed an untargeted metabolomics approach, many of the significantly altered metabolites require validation, and further targeted analyses are necessary. Increasing the number of participants in future studies and confirming metabolite identities through a targeted analysis will be essential to validate and expand upon these initial results.
In conclusion, this study identified several potential plasma biomarkers for NTDs through untargeted metabolomics, including arachidonic acid, prostaglandin, choline, leucine, phenylalanine, lysine, thyrotropin-releasing hormone, vitamin D3, cob(II)alamin, and galactosylsphingosine. The level differences between the NTD-affected and control groups suggest their potential relevance in the pathogenesis and early diagnosis of NTDs. However, the putative nature of these identifications necessitates further targeted validation studies to confirm their utility and reliability as biomarkers. Future research should focus on expanding the population studies and incorporating targeted metabolomics to enhance the accuracy and clinical applicability of these findings.
Concept: ŞÖ, YEÜ, MÇ; Sample collection: ŞÖ, YEÜ; LC-MS/MS Analysis: BBF, OK; Data analysis: BBF, OK; Writing Manuscript: ŞÖ, MÇ; Supervising: ŞÖ, MÇ, YEÜ.
The authors declare they have not used Artificial Intelligence (AI) tools in the creation of this article. Grammarly's complete edition was used to edit the language.
[1] |
Nelson K, Holmes LB (1989) Malformations due to presumed spontaneous mutations in newborn infants. N Engl J Med 320: 19-23. https://doi.org/10.1056/NEJM198901053200104 ![]() |
[2] |
Greene NDE, Copp AJ (2014) Neural tube defects. Annu Rev Neurosci 37: 221-242. https://doi.org/10.1146/annurev-neuro-062012-170354 ![]() |
[3] | Tan KH, Tan TYT, Tan J, et al. (2005) Birth defects in Singapore: 1994–2000. Singapore Med J 46: 545-552. |
[4] |
Busby A, Abramsky L, Dolk H, et al. (2005) Preventing neural tube defects in Europe: Population based study. BMJ 330: 574-575. ![]() |
[5] |
Cornel MC, De Smit DJ, De Jong-van den Berg LTW (2005) Folic acid—the scientific debate as a base for public health policy. Reprod ToxicoL 20: 411-415. https://doi.org/10.1016/j.reprotox.2005.03.015 ![]() |
[6] | Pulikkunnel ST, Thomas SV (2005) Neural tube defects: Pathogenesis and folate metabolism. J Assoc Physicians India 53: 127-135. |
[7] | Mennuti MT, Moranz JG, Schwarz RH, et al. (1977) Amniography for the early detection of neural tube defects. Obstet Gynecol 49: 25-30. |
[8] |
Blumenfeld Z, Siegler E, Bronshtein M (1993) The early diagnosis of neural tube defects. Prenat Diagn 13: 863-871. https://doi.org/10.1002/pd.1970130910 ![]() |
[9] |
Huang W, Gu H, Yuan Z (2022) Identifying biomarkers for prenatal diagnosis of neural tube defects based on “omics”. Clin Genet 101: 381-389. https://doi.org/10.1111/cge.14087 ![]() |
[10] |
López-López A, López-Gonzálvez A, Barker-Tejeda TC, et al. (2018) A review of validated biomarkers obtained through metabolomics. Expert Rev Mol Diagn 18: 557-575. https://doi.org/10.1080/14737159.2018.1481391 ![]() |
[11] |
Jacob M, Lopata AL, Dasouki M, et al. (2019) Metabolomics toward personalized medicine. Mass Spectrom Rev 38: 221-238. https://doi.org/10.1002/mas.21548 ![]() |
[12] |
Forsberg EM, Huan T, Rinehart D, et al. (2018) Data processing, multi-omic pathway mapping, and metabolite activity analysis using XCMS Online. Nat Protoc 13: 633-651. https://doi.org/10.1038/nprot.2017.151 ![]() |
[13] |
Libiseller G, Dvorzak M, Kleb U, et al. (2015) IPO: A tool for automated optimization of XCMS parameters. BMC Bioinformatics 16: 118. https://doi.org/10.1186/s12859-015-0562-8 ![]() |
[14] | Kaplan O, Çelebier M (2020) Evaluation of recent data processing strategies on Q-TOF LC/MS based untargeted metabolomics. Mass Spectrometry Lett 11: 1-5. https://doi.org/10.5478/MSL.2020.11.1.1 |
[15] |
Sysi-Aho M, Katajamaa M, Yetukuri L, et al. (2007) Normalization method for metabolomics data using optimal selection of multiple internal standards. BMC Bioinformatics 8: 93. https://doi.org/10.1186/1471-2105-8-93 ![]() |
[16] |
Pang Z, Chong J, Zhou G, et al. (2021) MetaboAnalyst 5.0: narrowing the gap between raw spectra and functional insights. Nucleic Acids Res 49: W388-W396. https://doi.org/10.1093/nar/gkab382 ![]() |
[17] |
Dheen ST, Tay SSW, Boran J, et al. (2009) Recent studies on neural tube defects in embryos of diabetic pregnancy: An overview. Current Med Chem 16: 2345-2354. https://doi.org/10.2174/092986709788453069 ![]() |
[18] |
Jawerbaum A, Gonzalez E (2005) The role of alterations in arachidonic acid metabolism and nitric oxide homeostasis in rat models of diabetes during early pregnancy. Curr Pharm Des 11: 1327-1342. https://doi.org/10.2174/1381612053507503 ![]() |
[19] |
Baker L, Piddington R, Goldman A (1990) Myo-inositol and prostaglandins reverse the glucose inhibition of neural tube fusion in cultured mouse embryos. Diabetologia 33: 593-596. https://doi.org/10.1007/BF00400202 ![]() |
[20] |
Reece EA, Khandelwal M, Wu YK, et al. (1997) Dietary intake of myo-inositol and neural tube defects in offspring of diabetic rats. Am J Obstet Gynecol 176: 536-539. https://doi.org/10.1016/S0002-9378(97)70543-X ![]() |
[21] |
Piddington R, Joyce J, Dhanasekaran P, et al. (1996) Diabetes mellitus affects prostaglandin E2 levels in mouse embryos during neurulation. Diabetologia 39: 915-920. https://doi.org/10.1007/BF00403910 ![]() |
[22] |
Greene NDE, Leung KY, Copp AJ (2017) Inositol, neural tube closure and the prevention of neural tube defects. Birth Defects Res 109: 68-80. https://doi.org/10.1002/bdra.23533 ![]() |
[23] |
Mills JL, Fan R, Brody LC, et al. (2014) Maternal choline concentrations during pregnancy and choline-related genetic variants as risk factors for neural tube defects. Am J Clin Nutr 100: 1069-1074. https://doi.org/10.3945/ajcn.113.079319 ![]() |
[24] | Shaw GM, Carmichael SL, Yang W, et al. (2004) Periconceptional dietary intake of choline and betaine and neural tube defects in offspring. Am J Epidemiol 160: 102-109. https://doi.org/10.1093/aje/kwh187 |
[25] | Rosenquist TH, Ratashak SA, Selhub J (1996) Homocysteine induces congenital defects of the heart and neural tube: Effect of folic acid. Proc Natl Acad Sci USA 93: 15227-15232. https://doi.org/10.1073/pnas.93.26.15227 |
[26] | Saxton RA, Knockenhauer KE, Wolfson RL, et al. (2016) Structural basis for leucine sensing by the Sestrin2-mTORC1 pathway. Science 351: 53-58. https://doi.org/10.1126/science.aad2087 |
[27] |
Denno KM, Sadler T (1990) Phenylalanine and its metabolites induce embryopathies in mouse embryos in culture. Teratology 42: 565-570. https://doi.org/10.1002/tera.1420420513 ![]() |
[28] | Duczyńska N, Cabalska B, Nowaczewska I, et al. (1990) Evaluation of amino acids in plasma and amniotic fluid of women from genetic risk groups. Probl Med Wieku Rozwoj 16: 103-115. |
[29] |
Gamero-Estevez E, Baumholtz AI, Ryan AK (2018) Developing a link between toxicants, claudins and neural tube defects. Reprod Toxicol 81: 155-167. https://doi.org/10.1016/j.reprotox.2018.08.008 ![]() |
[30] |
Bereket A (2008) Endocrine aspects of neural tube defects. The Spina Bifida.Springer 423-428. https://doi.org/10.1007/978-88-470-0651-5_34 ![]() |
[31] |
Daglar K, Tokmak A, Kirbas A, et al. (2016) Maternal serum vitamin D levels in pregnancies complicated by neural tube defects. J Matern Fetal Neonatal Med 29: 298-302. https://doi.org/10.3109/14767058.2014.999037 ![]() |
[32] | Groenen PMW, Engelke UF, Wevers RA, et al. (2004) High-resolution 1H NMR spectroscopy of amniotic fluids from spina bifida fetuses and controls. Eur J Obstet Gynecol Reprod Biol 112: 16-23. https://doi.org/10.1016/s0301-2115(03)00279-3 |
[33] |
Zheng X, Su M, Pei L, et al. (2011) Metabolic signature of pregnant women with neural tube defects in offspring. J Proteome Res 10: 4845-4854. https://doi.org/10.1021/pr200666d ![]() |
[34] |
Thompson MD, Cole DEC, Ray JG (2009) Vitamin B-12 and neural tube defects: The Canadian experience. Am J Clin Nutr 89: 697S-701S. https://doi.org/10.3945/ajcn.2008.26947B ![]() |
[35] |
Kaneko-Tarui T, Zhang L, Austin KJ, et al. (2007) Maternal and embryonic control of uterine sphingolipid-metabolizing enzymes during murine embryo implantation. Biology of reproduction 77: 658-665. https://doi.org/10.1095/biolreprod.107.061044 ![]() |
[36] |
Guo L, Geng X, Ma L, et al. (2013) Sphingosine-1-phosphate inhibits ceramide-induced apoptosis during murine preimplantation embryonic development. Theriogenology 80: 206-211. https://doi.org/10.1016/j.theriogenology.2013.04.016 ![]() |
[37] |
Lumsangkul C, Tso KH, Fan YK, et al. (2021) Mycotoxin fumonisin B1 interferes sphingolipid metabolisms and neural tube closure during early embryogenesis in brown tsaiya ducks. Toxins 13: 743. https://doi.org/10.3390/toxins13110743 ![]() |
[38] |
Marasas WFO, Riley RT, Hendricks KA, et al. (2004) Fumonisins disrupt sphingolipid metabolism, folate transport, and neural tube development in embryo culture and in vivo: a potential risk factor for human neural tube defects among populations consuming fumonisin-contaminated maize. J Nutr 134: 711-716. https://doi.org/10.1093/jn/134.4.711 ![]() |
[39] |
Mizugishi K, Yamashita T, Olivera A, et al. (2005) Essential role for sphingosine kinases in neural and vascular development. Mol Cell Biol 25: 11113-11121. https://doi.org/10.1128/MCB.25.24.11113-11121.2005 ![]() |
[40] |
Milstien S, Gude D, Spiegel S (2007) Sphingosine 1—phosphate in neural signalling and function. Acta Paediatr 96: 40-43. https://doi.org/10.1111/j.1651-2227.2007.00206.x ![]() |
[41] |
Hendricks K (1999) Fumonisins and neural tube defects in South Texas. Epidemiology 10: 198-200. https://doi.org/10.1097/00001648-199903000-00022 ![]() |
[42] |
Merrill AH, van Echten G, Wang E, et al. (1993) Fumonisin B1 inhibits sphingosine (sphinganine) N-acyltransferase and de novo sphingolipid biosynthesis in cultured neurons in situ. J Biol Chem 268: 27299-27306. ![]() |
[43] |
Liu L, Yin TL, Chen Y, et al. (2018) Follicular dynamics of glycerophospholipid and sphingolipid metabolisms in polycystic ovary syndrome patients. J Steroid Biochem Mol Biol 185: 142-149. https://doi.org/10.1016/j.jsbmb.2018.08.008 ![]() |
[44] |
Webster WS (1988) The use of animal models in understanding human teratogens. Cong Anom 28: 295-302. ![]() |
[45] |
Radhika MS, Bhaskaram P, Balakrishna N, et al. (2002) Effects of vitamin A deficiency during pregnancy on maternal and child health. BJOG 109: 689-693. https://doi.org/10.1111/j.1471-0528.2002.01010.x ![]() |
[46] |
Soprano DR, Soprano KJ (1995) Retinoids as teratogens. Annu Rev Nutr 15: 111-132. https://doi.org/10.1146/annurev.nu.15.070195.000551 ![]() |
[47] |
Lewis S, Lucas RM, Halliday J, et al. (2010) Vitamin D deficiency and pregnancy: From preconception to birth. Mol Nutr Food Res 54: 1092-1102. https://doi.org/10.1002/mnfr.201000044 ![]() |
[48] |
Gernand AD, Schulze KJ, Stewart CP, et al. (2016) Micronutrient deficiencies in pregnancy worldwide: Health effects and prevention. Nat Rev Endocrinol 12: 274-289. https://doi.org/10.1038/nrendo.2016.37 ![]() |
[49] |
Molloy AM, Kirke PN, Brody LC, et al. (2008) Effects of folate and vitamin B12 deficiencies during pregnancy on fetal, infant, and child development. Food Nutr Bull 29: S101-S111. https://doi.org/10.1177/15648265080292S114 ![]() |
[50] |
Nasri K, Ben Jamaa N, Gaigi SS, et al. (2024) Docosahexaenoic acid, eicosapentaenoic acid, arachidonic acid, and neural tube defects in Tunisian population. Birth Defects Res 116: e2372. https://doi.org/10.1002/bdr2.2372 ![]() |
Metabolite | Matched Form | HMDB code | |
1 | Choline | M[1+] | HMDB0000097 |
2 | L-Leucine or L-Isoleucine | M-NH3+H[1+] | HMDB0000687 |
3 | L-Phenylalanine | M-HCOOH+H[1+] | HMDB0000159 |
4 | L-Lysine | M-NH3+H[1+] | HMDB0000182 |
5 | Thyrotropin-releasing hormone | M+2H[2+] | HMDB0060080 |
6 | N6-Acetyl-L-lysine | M+Na[1+] | HMDB0000206 |
7 | Prostaglandin D2, E2, A2, J2, or B2 | M-H4O2+H[1+] | HMDB0001403 |
8 | Arachidonic acid | M+Na[1+] | HMDB0001043 |
9 | Aspartylglycosamine | M+H[1+] | HMDB0000489 |
10 | Vitamin D3 (Calcitriol) | M-HCOOH+H[1+] | HMDB0001903 |
11 | Adenylsuccinic acid | M-CO2+H[1+] | HMDB0000536 |
12 | Galactosylsphingosine | M-H4O2+H[1+] | HMDB0000648 |
13 | Delta 8,14-Sterol | M+NaCl[1+] | HMDB0006928 |
14 | LysoPC(O-18:0) | M[1+] | HMDB0011149 |
15 | Estriol-16-Glucuronide | M+HCOONa[1+] | HMDB0006766 |
16 | Coproporphyrin I or II | M[1+] | HMDB0000643 |
17 | Ganglioside GA2 (d18:1/12:0) | M-CO+H[1+] | HMDB0004888 |
18 | Galabiosylceramide (d18:1/9Z-18:1) | M+NaCl[1+] | HMDB0004832 |
19 | Cob(II)alamin | M-H2O+H[1+] | HMDB0006316 |
Metabolite | Matched Form | HMDB code | |
1 | Choline | M[1+] | HMDB0000097 |
2 | L-Leucine or L-Isoleucine | M-NH3+H[1+] | HMDB0000687 |
3 | L-Phenylalanine | M-HCOOH+H[1+] | HMDB0000159 |
4 | L-Lysine | M-NH3+H[1+] | HMDB0000182 |
5 | Thyrotropin-releasing hormone | M+2H[2+] | HMDB0060080 |
6 | N6-Acetyl-L-lysine | M+Na[1+] | HMDB0000206 |
7 | Prostaglandin D2, E2, A2, J2, or B2 | M-H4O2+H[1+] | HMDB0001403 |
8 | Arachidonic acid | M+Na[1+] | HMDB0001043 |
9 | Aspartylglycosamine | M+H[1+] | HMDB0000489 |
10 | Vitamin D3 (Calcitriol) | M-HCOOH+H[1+] | HMDB0001903 |
11 | Adenylsuccinic acid | M-CO2+H[1+] | HMDB0000536 |
12 | Galactosylsphingosine | M-H4O2+H[1+] | HMDB0000648 |
13 | Delta 8,14-Sterol | M+NaCl[1+] | HMDB0006928 |
14 | LysoPC(O-18:0) | M[1+] | HMDB0011149 |
15 | Estriol-16-Glucuronide | M+HCOONa[1+] | HMDB0006766 |
16 | Coproporphyrin I or II | M[1+] | HMDB0000643 |
17 | Ganglioside GA2 (d18:1/12:0) | M-CO+H[1+] | HMDB0004888 |
18 | Galabiosylceramide (d18:1/9Z-18:1) | M+NaCl[1+] | HMDB0004832 |
19 | Cob(II)alamin | M-H2O+H[1+] | HMDB0006316 |