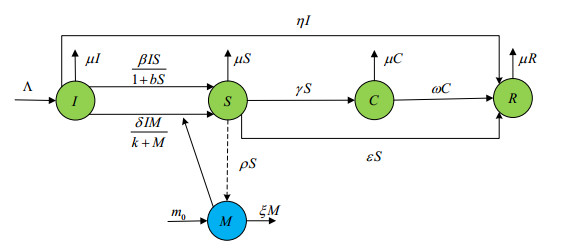
Ovarian cancer (OC) represents a significant challenge in the realm of gynecological cancers, characterized by poor survival rates and complex etiology. This review delves into the genetic, environmental, and hormonal factors underpinning OC development, shedding light on both well-established contributors and emerging influences. We reviewed scientific databases searching for OC genetic and epigenetic factors and included studies based on their relevancy. As a result of exploring ovarian carcinogenesis, this systematic review contains data collected from 102 works. The role of prominent genetic players, such as BRCA mutations and DNA repair mechanisms, underscores the intricate landscape of OC susceptibility. Furthermore, we explore Li-Fraumeni and Lynch syndrome, which result in a heightened predisposition to OC development. Hormonal factors such as estrogen, progesterone, and androgens are also discussed in detail. Environmental alterations, ranging from lifestyle influences to microbiome dysbiosis add layers of complexity to OC pathogenesis. Lifestyle factors such as obesity, alcohol consumption, and physical activity intersect with genetic and epigenetic pathways, shaping the risk landscape for OC. This review provides a nuanced understanding of the multifactorial nature of OC through a meticulous examination of current literature, emphasizing the need for holistic approaches to prevention, diagnosis, and treatment.
Citation: Alicja Florczak, Aleksandra Królikowska, Mateusz Mazurek, Sławomir Woźniak, Zygmunt Domagała. Most relevant genetic factors in ovarian cancer development[J]. AIMS Molecular Science, 2025, 12(1): 1-25. doi: 10.3934/molsci.2025001
[1] | Peter W. Bates, Jianing Chen, Mingji Zhang . Dynamics of ionic flows via Poisson-Nernst-Planck systems with local hard-sphere potentials: Competition between cations. Mathematical Biosciences and Engineering, 2020, 17(4): 3736-3766. doi: 10.3934/mbe.2020210 |
[2] | Yiwei Wang, Mingji Zhang . Finite ion size effects on I-V relations via Poisson-Nernst-Planck systems with two cations: A case study. Mathematical Biosciences and Engineering, 2024, 21(2): 1899-1916. doi: 10.3934/mbe.2024084 |
[3] | Dandan Fan, Dawei Li, Fangzheng Cheng, Guanghua Fu . Effects of congestion charging and subsidy policy on vehicle flow and revenue with user heterogeneity. Mathematical Biosciences and Engineering, 2023, 20(7): 12820-12842. doi: 10.3934/mbe.2023572 |
[4] | Tien-Wen Sung, Wei Li, Qiaoxin Liang, Chuanbo Hong, Qingjun Fang . Research on charging behavior of electric vehicles based on multiple objectives. Mathematical Biosciences and Engineering, 2023, 20(9): 15708-15736. doi: 10.3934/mbe.2023700 |
[5] | Chii-Dong Ho, Jr-Wei Tu, Hsuan Chang, Li-Pang Lin, Thiam Leng Chew . Optimizing thermal efficiencies of power-law fluids in double-pass concentric circular heat exchangers with sinusoidal wall fluxes. Mathematical Biosciences and Engineering, 2022, 19(9): 8648-8670. doi: 10.3934/mbe.2022401 |
[6] | Virginia González-Vélez, Amparo Gil, Iván Quesada . Minimal state models for ionic channels involved in glucagon secretion. Mathematical Biosciences and Engineering, 2010, 7(4): 793-807. doi: 10.3934/mbe.2010.7.793 |
[7] | Mette S. Olufsen, Ali Nadim . On deriving lumped models for blood flow and pressure in the systemic arteries. Mathematical Biosciences and Engineering, 2004, 1(1): 61-80. doi: 10.3934/mbe.2004.1.61 |
[8] | Guichen Lu, Zhengyi Lu . Permanence for two-species Lotka-Volterra cooperative systems with delays. Mathematical Biosciences and Engineering, 2008, 5(3): 477-484. doi: 10.3934/mbe.2008.5.477 |
[9] | Bindu Kumari, Chandrashekhar Sakode, Raghavendran Lakshminarayanan, Prasun K. Roy . Computational systems biology approach for permanent tumor elimination and normal tissue protection using negative biasing: Experimental validation in malignant melanoma as case study. Mathematical Biosciences and Engineering, 2023, 20(5): 9572-9606. doi: 10.3934/mbe.2023420 |
[10] | Suqing Lin, Zhengyi Lu . Permanence for two-species Lotka-Volterra systems with delays. Mathematical Biosciences and Engineering, 2006, 3(1): 137-144. doi: 10.3934/mbe.2006.3.137 |
Ovarian cancer (OC) represents a significant challenge in the realm of gynecological cancers, characterized by poor survival rates and complex etiology. This review delves into the genetic, environmental, and hormonal factors underpinning OC development, shedding light on both well-established contributors and emerging influences. We reviewed scientific databases searching for OC genetic and epigenetic factors and included studies based on their relevancy. As a result of exploring ovarian carcinogenesis, this systematic review contains data collected from 102 works. The role of prominent genetic players, such as BRCA mutations and DNA repair mechanisms, underscores the intricate landscape of OC susceptibility. Furthermore, we explore Li-Fraumeni and Lynch syndrome, which result in a heightened predisposition to OC development. Hormonal factors such as estrogen, progesterone, and androgens are also discussed in detail. Environmental alterations, ranging from lifestyle influences to microbiome dysbiosis add layers of complexity to OC pathogenesis. Lifestyle factors such as obesity, alcohol consumption, and physical activity intersect with genetic and epigenetic pathways, shaping the risk landscape for OC. This review provides a nuanced understanding of the multifactorial nature of OC through a meticulous examination of current literature, emphasizing the need for holistic approaches to prevention, diagnosis, and treatment.
ovarian cancer;
Li-Fraumeni syndrome;
progesterone receptor;
polycystic ovary syndrome;
combined oral contraceptive pills;
double-strand break repair;
lipopolysaccharides
A rumor refers to unsubstantiated information, which has the potential to rapidly and extensively disseminate through various communication channels [1]. In the past, rumors were disseminated through oral communication as well as various forms of print media, radio broadcasts, and other mediums. Contemporary social networks can function as an appropriate platform for the rumor propagation, while also satisfying individuals' informational needs [2]. For example, individuals' excessive salt consumption can be attributed to the panic of the Fukushima nuclear accident-related rumor. There have been numerous rumors that emerged a range of adverse impacts on the world since the outbreak of COVID-19 [3]. The security of individuals and their belongings is significantly compromised due to these rumors. Therefore, it is imperative to grasp the rules of communicating with scientists and create effective methods to intervene, defend, and prevent rumors from spreading.
Since rumor spreading is the analogy to the disease's transmission among individuals, many scholars employ similar rumor models. Early studies on rumor propagation focused a tiny community through individual interaction. According to rumor propagation, the crowd can be classified into three categories: ignorant, spreader, and stifler. In 1964, Daley and Kendall proposed the classic DK rumor-spreading model, which is the first cabin model in rumor propagation [4]. Furthermore, in 1973, Maki and Thompson proposed the MT model as an extension of the DK model [5]. According to the MT model, once interpersonal communication occurs, the former will no longer propagate. According to several scholars, the complexity of rumor propagation processes cannot be adequately captured by DK and MK models across various network settings, especially in the context of large-scale social networks. Zanette proposed a conceptual framework to simulate the dynamics of rumor propagation in small-world networks [6]. By integrating the MK model and the SIR model, Moreno et al. developed a novel rumor propagation model, demonstrating that network topologies can significantly influence the spread of rumors on scale-free networks [7]. To gain a deeper comprehension of rumor propagation, an increasing number of factors are being taken into account through the development of more rational models; for instance, hesitating mechanisms [8], inhibiting mechanisms, and attitude adjustment [9], etc. The innovations of these scholars, to a certain extent, promote rumor propagation to advance research under a variety of changing factors.
Nowadays, given the multiple channels of rumor propagation, individuals can quickly obtain information on social media platforms, thereby complicating the regulation of rumor propagation. Due to the significant damage caused by rumors, several control measures aimed at minimizing losses have been proposed by scholars; for instance, optimal control strategies [10], time-dependent controller [11], event-triggered control [12], and so on. In reality, when rumors prevail on social media platforms, the media coverage disseminates information to debunk these rumors and intervene in individuals' behavior, thereby mitigating social panic and upholding social harmony. Cheng et al. incorporated a nonlinear factor into the contact rate by integrating optimal control and time delay [13]. Huo et al. introduced a nonlinear function to effectively capture the impact of misinformation and media reports on the spread of rumors [14]. Pan et al. investigated a novel rumor model that integrated media coverage and rumor refuters [15]. Further on, Guo et al. proposed two models considering media coverage and a refutation mechanism, respectively [16,17]. Numerous mathematical models are available to describe how media coverage affects rumor propagation. Therefore, our proposed model must account for the impact of media coverage on the dissemination of rumors among individuals. Evidently, the aforementioned models have predominantly explored the propagation mechanism of rumors in an integer order.
However, the dynamic behavior of rumors is influenced by historical information. Fractional calculus, as an extension of integral calculus, provides innovative approaches for characterizing the memory effects, which is a generalization of integral calculus. Fractional order Caputo derivatives, extensively employed in various fields such as science, biology, engineering, computer science, chaos theory, and others, have witnessed significant advancements in recent decades [18,19,20,21,22,23,24,25]. The literature offers a wide range of models described by fractional derivatives, such as the SIR or SIRS model [26,27], the SEI model [28], the SEIR model [29,30,31], the SIQR model [32] and the SEIQRS model [33]. Rumor diffusion is similar to virus propagation and can be effectively modeled using fractional calculus. Singh proposed the dynamics of a rumor propagation in a social network, utilizing the Atangana-Baleanu derivative [34]. Ye et al. investigated a fractional-order reaction-diffusion rumor model in two linguistic contexts [35]. The impact of memory was investigated, and the findings clearly demonstrate that fractional calculus can be employed to more accurately characterize the process of rumor propagation.
Revisiting the findings of the previous study, we establish an Ignorants-Spreaders-Calmness-Removers-Media Reports (ISCRM) rumor propagation model with media reports under the Caputo sense by incorporating the memory effect. The subsequent sections of this manuscript are organized as follows: In Section 2, we provide a concise overview of fundamental definitions pertaining to fractional calculus. In Section 3, we propose an enhanced fractional-order ISCRM rumor model. In Section 4, the local and global asymptotic stability of the equilibria is investigated. In Section 5, the optimal control problem is addressed. In Section 6, numerical simulation is examined. In Section 7, the paper culminates with the presentation of our findings.
Various definitions exist regarding fractional derivatives, with the Caputo sense being provided as follows [36].
Definition 2.1. For Riemann-Liouville fractional integration with respect to order α, the function f(t) is presented as
t0Iαtf(t)=D−αtf(t)=1Γ(α)∫tt0f(τ)(t−τ)1−αdτ, | (2.1) |
where α∈R+, t0 represents the initial time, and Γ(⋅), which is established by Γ(z)=∫∞t0tz−1e−tdt, represents the Gamma function.
Definition 2.2. Define l−1<α⩽l, l∈N. The Riemann-Liouville fractional derivative with respect to order α is presented as [36]
RLt0Dαtf(t)=dαf(t)dtα=1Γ(m−α)dldtl∫tt0f(τ)(t−τ)α−l+1dτ, | (2.2) |
Remark 2.1. The combination of Definitions 2.1 and 2.2 yields RLt0Dαt(t0Iαtf(t))=f(t), giving the Riemann-Liouville fractional integrations and derivatives with respect to order α.
Definition 2.3. The Caputo fractional derivative with respect to order α is presented as follows:
Ct0Dαtf(t)={1Γ(l−α)∫tt0fl(τ)(t−τ)α−l+1dτ, l−1<α<l,dldtlf(t),α=l, | (2.3) |
where l denotes the smallest integer number such that α<l.
Remark 2.2. The equivalence between the definitions of Riemann-Liouville fractional integration and Caputo fractional integration are the same. Therefore, from Definitions 2.1 and 2.3, we obtain
Ct0Dαt(t0Iαtf(t))=f(t), | (2.4) |
giving the Caputo fractional integrations and derivatives with respect to order α.
In the subsequent sections of this paper, the notation Dα represents the Riemann-Liouville fractional derivative.
Lemma 2.1. Provide a definition for a function f(t) and a nonzero interval t∈(0,T), the condition |f(t)|⩾F1, where F1>0, is a constant. Next, a positive constant is given L=F1TαΓ(1+α). Thus, the inequality 0Iαt1|f(t)|⩾L is held for t∈(0,t1), t1⩾T.
Proof. The equality is evidently apparent:
0Iαt1|f(t)|=1Γ(α)∫t10|f(τ)|(t−τ)1−αdτ. | (2.5) |
By referring to Eq (2.1), which defines fractional integration, the value of 0Iαt1|f(t)| can be determined. Due to the condition |f(t)|⩾F1, for at least one nonzero interval t∈(0,T), it acquires
0Iαt1|f(t)|=1Γ(α)∫t10|f(τ)|(t−τ)1−αdτ⩾1Γ(α)∫T0|f(τ)|(t−τ)1−αdτ⩾F1Γ(α)∫T01(t−τ)1−αdτ=F1TαΓ(1+α). | (2.6) |
Therefore, the inequality 0Iαt1|f(t)|⩾F1TαΓ(1+α) holds for f(t).
Definition 2.4. Let F(τ) be the Laplace transform of the function f(t). Then, the Caputo fractional derivative Laplace transform is provided as [37]
L[Dαf(t)]=ταF(τ)−n−1∑i=0f(i)(0)τα−n−1, n−1<α⩽n, n∈N. | (2.7) |
Definition 2.5. The expressions for the Mittag-Leffler functions in both one-parameter and two-parameter forms are provided as [36]
Eα(z)=∞∑k=0zkΓ(αk+1), Eα,l(z)=∞∑k=0zkΓ(αk+l), | (2.8) |
where z,α,β∈C.
Theorem 2.1. [36] Consider the autonomous linear fractional-order equation Dαx=Ax, x(0)=x0, where 0<α<1, x∈Rn and A∈Rn×n. The asymptotic stability of this system implies that
|arg(eigA)|⩾απ2. | (2.9) |
In this scenario, each component of the state's decays toward 0 as t−α.
Theorem 2.2. [38] Let x=0 denote an equilibrium point for the equilibrium of the non-autonomous fractional-order equation
Dαx(t)=f(x,t), | (2.10) |
where f(x,t) satisfies the Lipschitz constant l>0 and α∈(0,1). Provided that it constitutes a Lyapunov function V(t,x(t)) and class-K functions α1, α2 and α3 satisfying the condition
α1(‖x‖)⩽V(t,x)⩽α2(‖x‖), DβV(t,x)⩽α3(‖x‖), |
where β∈(0,1), the equilibrium point of Eq (2.10) is asymptotic stable.
The proposed framework in this section is an Ignorants-Spreaders-Calmness-Removers-Media Reports (ISCRM). All of the crowd are divided into four groups: Ignorants are those who are unfamiliar with the rumors but are willing to believe them, denoted by I(t); Spreaders are those who acquire the rumors and spread them, denoted by S(t); Calmness are those who calm down before they refrain from disseminating rumors [39], denoted by C(t); Removers are those who are aware of the rumors and either refute them or have become disinterested in rumor, denoted by R(t). Since rumor propagation varies over time, media reports will undergo changes over time. We employ a quantization method to measure the impact of media reports, denoted by M(t).
The rules for rumor propagation are as follows:
(ⅰ) When an ignorant contacts a spreader, it will be affected and become a spreader with the exposure rate of β/(1+bS), which reflects the reduced amount of contact rate due to the scale of the rumor propagation. β denotes the rumor contact rate and b is the measure of inhibition.
(ⅱ) δ/(k+M) represents the influence of the media reports, where δ>1 and k⩾0. δ/k means the highest availability of media influence effect. δ represents the rate of contact between the ignorant and the media reports and k is the effect of media reports. γ represents calm down rate of the spreader. ε and ω represent the forgetting rates.
(ⅲ) We define that the immigrate rate is Λ and the emigrate rate is μ in our study. Furthermore, η represents the influence of rumors under the rumor control measures. m0 represents the initial value of the media reports. ρ represents the influence of rumor propagation on media reports. The parameters of the model are assumed to be constant and positive.
The mathematical dynamics of rumor propagation under the Caputo sense is derived based on the propagation rules in Figure 1 as follows:
DαI(t)=Λ−βI(t)S(t)1+bS(t)−δI(t)M(t)k+M(t)−ηI(t)−μI(t),DαS(t)=βI(t)S(t)1+bS(t)+δI(t)M(t)k+M(t)−γS(t)−εS(t)−μS(t),DαC(t)=γS(t)−ωC(t)−μC(t),DαR(t)=ηI(t)+εS(t)+ωC(t)−μR(t),DαM(t)=m0+ρS(t)−ξM(t), | (3.1) |
where the initial conditions are denoted by (I(0),S(0),C(0),R(0),M(0))=(I0,S0,C0,R0,M0).
In this model, the non-negative initial conditions of Eq (3.1) are obtained as
I(0)=I0⩾0, S(0)=S0⩾0, C(0)=C0⩾0, R(0)=R0⩾0, M(0)=M0⩾0. | (3.2) |
Theorem 3.1. The solution of the Caputo sense Eq (3.1) subject to the conditions (3.2) is non-negative within the closed set
Ω={(I,S,C,R,M)∈R4+:N(t)=I(t)+S(t)+C(t)+R(t)⩽Λμ,M(t)⩽m0ξ+ρΛμξ}. |
Proof. For Caputo fractional derivatives (3.1), one obtains
{DαI(t)|I(t)=0=Λ⩾0,DαS(t)|S(t)=0=δI(t)M(t)k+M(t)⩾0,DαS(t)|S(t)=0=γS(t)⩾0,DαR(t)|R(t)=0=ηI(t)+εS(t)+ωC(t)⩾0,DαM(t)|M(t)=0=m0+ρS(t)⩾0. | (3.3) |
Based on the Laplace transform, it can be demonstrated that I,S,C,R,M⩾0, t∈[t0,T). Additionally, the total population is determined as N(t)=I(t)+S(t)+C(t)+R(t). Thus, adding the first four equations of Eq (3.1), we obtain
C0DαtN(t)=Λ−μ(I(t)+S(t)+C(t)+R(t))=Λ−μN(t). |
By employing the Laplace transform, we obtain N(t)⩽N(0)Eα(−μtα)+Λμ(1−Eα(−μtα)). Eα(−μtα) is the Mittag-Leffler function of parameter α. Since 0⩽Eα(−μtα)⩽1, we obtain N(t)⩽Λμ+N(0). Subsequently, by applying the fifth equation of Eq (3.1), we obtain DαM(t)⩽m0+ρΛμ−ξM(t), and then, 0⩽M(t)⩽m0ξ+ρΛμξ−M(0), where M(0) denotes the initial value of the message of media reports. Thus, sup. This implies that is bounded, thus and are bounded in
So, all solutions of Eq (3.1) are non-negative. Now, we will show that equation has a unique solution.
Theorem 3.2. For any given initial condition , Eq (3.1) has a unique solution .
Proof. The approach employed in [40] is adopted to validate the existence and uniqueness.
Let as follows:
Furthermore, for any given , a function can be constructed as follows:
where
Therefore, satisfies the Lipschitz condition with any initial condition . Equations (3.1) always have a unique solution.
In order to explore the rumor-free and rumor-permanence equilibrium points of the fractional order Eq (3.1), we solve the following equations:
By denoting the aforementioned Eq (3.1) with , the rumor-free equilibrium point can be calculated as: By employing the next generation approach [41], the basic reproduction number is calculated. If , the original equation can be reformulated as
where
The Jacobian matrices of and at the rumor-free equilibrium , respectively, are
The next-generation matrix for Eq (3.1) is presented as follows:
Subsequently, the basic reproduction number of Eq (3.1) is determined:
The following theorem is utilized to analyze the local and global stability of the two equilibrium points.
Theorem 4.1. Equation (3.1) exhibit local stability of the rumor-free equilibrium if , and if it is unstable.
Proof. The stability of the equilibrium point is investigated by examining the Jacobian matrix of Eq (3.1).
The characteristic polynomial of is derived as follows:
(4.1) |
If all roots of possess negative real parts, the rumor-free equilibrium can be considered asymptotically stable.
When , we obtain
and
(4.2) |
It is obvious that and are negatives roots and thus, the real component of each of Eq (4.2) roots plays a crucial role.
According to Eq (4.1), it defines
Evidently, we obtain
(4.3) |
When , we acquire
(4.4) |
Thus, is deducted from Eq (4.2) by adding to Eq (4.4), and we obtain
(4.5) |
From Eq (4.5), we obtain
(4.6) |
By adding the parameter to Eq (4.6), we obtain
(4.7) |
Therefore, is deduced based on Eq (4.7). After evaluation of different coefficients of Eq (4.2), and are obtained. According to the Routh-Hurwitz conditions [42], all eigenvalues of have negative real part. The condition is satisfied and the rumor-free equilibrium is locally asymptotically stable if , and unstable if .
Theorem 4.2. The rumor-free equilibrium of Eq (3.1) is globally asymptotically stable if .
Proof. Let , with positive constants and , serve as a Lyapunov function
By differentiating the Lyapunov function with respect to time, we obtain
(4.8) |
where Substituting , and in Eq (3.1) into Eq (4.8) yields
(4.9) |
From Eq (4.9), we obtain
Equating the coefficient to zero, we obtain
At the rumor-free equilibrium , and , we obtain . By considering and substituting and into Eq (4.9), the obtained result is as follows:
If , then . Specially, if and only if . Therefore, the largest compact invariant set in is the singleton set . According to LaSalle's invariance principle [43], is globally asymptotically stable in if .
Theorem 4.3. If and , Eq (3.1) exhibits local stability of rumor-permanence equilibrium .
Proof. Equation (3.1) at the rumor-permanence equilibrium can be further simplified as follows:
The Jacobian matrix evaluated at the rumor-permanence equilibrium persistence is presented in [16] as
where
The corresponding characteristic equation can be derived as
(4.10) |
where
For , and are all negative, while and are positive. Subsequently, we obtain
Obviously, it is . If , then . According to the Routh-Hurwitz criterion [42], it can be inferred that all eigenvalues of the eigenequation in Eq (4.10) possess negative real parts.
Therefore, the rumor-permanence equilibrium is proven to be locally asymptotically stable and Theorem 4.3 is proven.
The objective of our study is to identify the optimal control method and approach in order to minimize the overall cost associated with rumor spreading. The feasible intervention method aims to minimize the dissemination of rumors by individuals. The primary objective of fractional optimal control is to mitigate the relative density of individuals who spread rumors. Furthermore, the consideration of cost control measures is imperative in light of the current circumstances. Therefore, the optimal control strategy for Eq (5.1) is proposed, and its investigation can be facilitated through the application of Pontryagin's maximum principle [44].
Equation (3.1), incorporating the control mechanism, is thus formulated as
(5.1) |
where the control strengths to the rumor propagation are represented by and through education or government management. The objective function of the optimal control is formulated as
(5.2) |
where and are the non-negative weights related to and in Eq (5.2). and are relative to cost of the measures.
To determine the optimal interventions proposed in Eq (5.1),
(5.3) |
where and are the upper bounds of and , respectively. Optimal controls and satisfy .
We derive the optimal controls for minimizing the rumor propagation at minimal cost. Consequently, the following Lagrangian function is obtained:
(5.4) |
where are adjoint variable functions.
Theorem 5.1. There exists an optimal control with the solution corresponding to the state equation in Eq (5.1) and the adjoint variables , we obtain
with boundary conditions According to the adjoint variables and , we differentiate the Hamiltonian in Eq (5.2) with respect to each variable.
Proof. From the adjoint variables and , we differentiate the Hamiltonian in Eq (5.2) with respect to each variable:
Moreover, the optimal control of the control variables set is attained by partial differential equation as follows:
For
For
For
Therefore,
The uniqueness of the optimal control has been established based on the boundedness and adjoint variables. The standard control with a bound is obtained as follows:
The sensitivity of the parameters in the rumor propagation model is investigated in this section. The following conditions are satisfied:
In conclusion, , , and are sensitive, and , analogous to and are not sensitive. Sensitivity analysis shows that it is possible to reduce the rumor contact rate and parameters and , thus lowering the value of .
In this section, we present the fractional Euler method [45], which is employed in solving fractional-order differential equations and obtaining a discretized equation, as described below:
for where . The fractional Euler method is employed for the subsequent numerical solutions of the fractional-order differential equations.
In this section, we conduct numerical simulations to validate the findings presented in the preceding sections. The initial values are considered as and . Additionally, the parametric values are listed in Table 1. Obviously, the basic reproduction number is . Figure 2 provides graphs of the evolution of (a) Ignorants, (b) Spreaders, (c) Calmness, (d) Removers, and (e) Media Reports in the rumor-free case for Eq (3.1), employing various values of the differentiation parameter With the decrease of the order , the convergence rate of the rumor propagation curve slows down and eventually tends to stabilize, which shows the memory effect. From the perspective of public opinion dissemination, it implies that such rumors tend to persist for an extended duration. Moreover, it demonstrates an anomalous propagation pattern at a lower order , characterized by an exponentially rapid growth in the initial phase as depicted in Figure 3(b). Meanwhile, the subsequent phase exhibits a deceleration. When the order is , the fractional-order differential equation turns into an integer-order one.
Parameter | Value | Parameter | Value |
0.4 | 0.4 | ||
0.1 | 0.2 | ||
0.2 | 0.5 | ||
0.5 | 0.2 | ||
0.5 | 0.2 | ||
0.3 | 0.05 | ||
0.5 |
The initial values are also set to and . Furthermore, the parametric values are provided in Table 2. The calculation result of the basic reproduction number is . The results corresponding to the simulation of the rumors case are presented in Figure 3 using various values of the differentiation parameter The convergence rates of rumor propagation vary depending on the order value . The impact of the fractional order is observed through numerical simulation in Figure 3, from which it is demonstrated that reducing the value can enhance the stability range of model (3.1). The larger the order , the more rapidly the curve approaches stability in the rumor-permanence case. Therefore, it is imperative to consistently and promptly disseminate scientific knowledge while actively debunking rumors in order to effectively suppress rumor propagation.
Parameter | Value | Parameter | Value |
1.2 | 0.4 | ||
0.6 | 0.2 | ||
0.5 | 0.6 | ||
1 | 0.6 | ||
1 | 0.2 | ||
0.3 | 0.3 | ||
0.5 |
To illustrate the effectiveness of the media reports, for the basic reproduction number is established with the parametric values in Table 2. The media reports have an impact on Eq (3.1) governing fractional-order as illustrated in Figure 4. The peak of the Spreaders group decreases as the value of increases, as shown in Figure 4(b). The statement suggests that there is a positive correlation between the level of media reports and the influence on rumor propagation. Therefore, employing appropriate media strategies can effectively suppress the rumor propagation.
To demonstrate the effectiveness of the fractional optimal control strategy, for the basic reproduction number is established with the parametric values in Table 2. Figure 5 shows the populations of and , with or without control mechanism. Compared with Eq (5.1), with or without control strategy, the populations of the Ignorants and the Spreaders are significantly influenced. This implies that implementing an effective control mechanism can significantly restrict the rumor diffusion.
A Caputo sense-based ISCRM rumor propagation model incorporating media reports is proposed. The existence of positive solutions is rigorously established in this study, and the equilibrium solutions are determined for both the rumor-free equilibrium and rumor-permanence equilibrium, along with their local properties. The rumor-free equilibrium is locally and globally asymptotically stable if . The instability of the unique rumor-permanence equilibrium exists when , and for , it is locally asymptotically stable. The analytical research was validated by conducting numerical simulations, encompassing a range of fractional orders and initial compartment sizes. In the case of , stability is rapidly achieved at lower fractional orders, whereas in the case of , the attainment of stability is rapidly achieved at higher orders. The significance of fractional analysis has been underscored by the achievement of significantly improved and more realistic outcomes. Additionally, a comprehensive analysis of the impact of media reports and an optimal control strategy is presented. The effectiveness of rumor diffusion prevention can be significantly enhanced through the implementation of a control strategy and media reports, thereby mitigating the risk associated with rumor propagation. In future research, the investigation of time delay effects [46] and stochastic factors [47,48] could be expanded within the framework of complex networks in Caputo sense systems.
The authors declare they have not used Artificial Intelligence (AI) tools in the creation of this article.
The authors declare that they have no known competing financial interests or personal relationships that could have appeared to influence the work reported in this paper.
[1] |
Gupta S, Rajappa S, Advani S, et al. (2021) Prevalence of BRCA1 and BRCA2 mutations among patients with ovarian, primary peritoneal, and fallopian tube cancer in India: A multicenter cross-sectional study. JCO Glob Oncol 7: 849-861. https://doi.org/10.1200/GO.21.00051 ![]() |
[2] | Ovarian Cancer Research AllianceOvarian cancer (2024). Available from: https://ocrahope.org/get-the-facts/statistics/ |
[3] |
Cabasag CJ, Fagan PJ, Ferlay J, et al. (2022) Ovarian cancer today and tomorrow: A global assessment by world region and Human Development Index using GLOBOCAN 2020. Int J Cancer 151: 1535-1541. https://doi.org/10.1002/ijc.34002 ![]() |
[4] | Ovarian Cancer StatisticsKey Statistics for Ovarian Cancer (2024). Available from: https://www.cancer.org/cancer/types/ovarian-cancer/about/key-statistics.html |
[5] |
Toss A, Tomasello C, Razzaboni E, et al. (2015) Hereditary ovarian cancer: Not only BRCA 1 and 2 Genes. Biomed Res Int 2015: 341723. https://doi.org/10.1155/2015/341723 ![]() |
[6] |
Avramenko AS, Flanagan JM (2023) An epigenetic hypothesis for ovarian cancer prevention by oral contraceptive pill use. Clin Epigenet 15: 165. https://doi.org/10.1186/S13148-023-01584-9 ![]() |
[7] |
Domański J, Domagala Z, Simmons JE, et al. (2023) Terra Incognita in anatomical museology–A literature review from the perspective of evidence-based care. Ann Anat 245: 152013. https://doi.org/10.1016/J.AANAT.2022.152013 ![]() |
[8] |
Mateusz M, Oliwier P, Mateusz D, et al. (2024) Decoding the mysteries of the obturator nerve. J Anat Soc India 73: 64-69. https://doi.org/10.4103/JASI.JASI_122_23 ![]() |
[9] |
Domagała D, Data K, Szylle H, et al. (2024) Cellular, molecular and clinical aspects of aortic aneurysm—vascular physiology and pathophysiology. Cells 13: 274. https://doi.org/10.3390/CELLS13030274 ![]() |
[10] |
Xu K, Yang S, Zhao Y (2017) Prognostic significance of BRCA mutations in ovarian cancer: an updated systematic review with meta-analysis. Oncotarget 8: 285-302. https://doi.org/10.18632/ONCOTARGET.12306 ![]() |
[11] | Casaubon JT, Kashyap S, Regan JP BRCA1 and BRCA2 mutations (2023). Available from: https://www.ncbi.nlm.nih.gov/books/NBK470239/ |
[12] | Varol U, Kucukzeybek Y, Alacacioglu A, et al. (2018) BRCA genes: BRCA 1 and BRCA 2. J BUON 23: 862-866. |
[13] |
Venkitaraman AR Cancer susceptibility and the functions of BRCA1 and BRCA2. Cell 108: 171-182. https://doi.org/10.1016/S0092-8674(02)00615-3 ![]() |
[14] |
Vergote I, González-Martínet A, Ray-Coquardal I, et al. (2022) European experts consensus: BRCA/homologous recombination deficiency testing in first-line ovarian cancer. Ann Oncol 33: 276-287. https://doi.org/10.1016/j.annonc.2021.11.013 ![]() |
[15] |
Tutt ANJ, Lord CJ, Mccabe N, et al. (2005) Exploiting the DNA repair defect in BRCA mutant cells in the design of new therapeutic strategies for cancer. Cold Spring Harb Symp Quant Biol 70: 139-148. https://doi.org/10.1101/SQB.2005.70.012 ![]() |
[16] |
Doig KD, Fellowes AP, Fox SB (2023) Homologous Recombination Repair Deficiency: An Overview for Pathologists. Modern Pathol 36: 100049. https://doi.org/10.1016/j.modpat.2022.100049 ![]() |
[17] |
McCarthy N (2005) A positive defect. Nat Rev Cancer 5: 333. https://doi.org/10.1038/nrc1618 ![]() |
[18] | Villani A, Frebourg T, Malkin D (2021) Li-fraumeni syndrome. The hereditary basis of childhood cancer. Cham: Springer 1-21. https://doi.org/10.1007/978-3-030-74448-9_1 |
[19] |
Guha T, Malkin D (2017) Inherited TP53 mutations and the Li-fraumeni syndrome. Cold Spring Harb Perspect Med 7: a026187. https://doi.org/10.1101/CSHPERSPECT.A026187 ![]() |
[20] |
Sorrell AD, Espenschied CR, Culver JO, et al. (2013) Tumor protein p53 (TP53) testing and Li-Fraumeni Syndrome. Mol Diagn Ther 17: 31-47. https://doi.org/10.1007/S40291-013-0020-0 ![]() |
[21] |
Kumamoto T, Yamazaki F, Nakano Y, et al. (2021) Medical guidelines for Li–Fraumeni syndrome 2019, version 1.1. Int J Clin Oncol 26: 2161-2178. https://doi.org/10.1007/S10147-021-02011-W ![]() |
[22] | Genes by Name. Available from: https://www.facingourrisk.org/info/hereditary-cancer-and-genetic-testing/hereditary-cancer-genes-and-risk/genes-by-name |
[23] |
Ryan NA, McMahon RF, Ramchander NC, et al. (2021) Lynch syndrome for the gynaecologist. TOG 23: 9-20. https://doi.org/10.1111/TOG.12706 ![]() |
[24] |
Underkofler KA, Ring KL (2023) Updates in gynecologic care for individuals with lynch syndrome. Front Oncol 13: 1127683. https://doi.org/10.3389/FONC.2023.1127683 ![]() |
[25] |
Bansidhar BJ, Silinsky J (2012) History and Pathogenesis of Lynch Syndrome. Clin Colon Rectal Surg 25: 63-66. https://doi.org/10.1055/S-0032-1313776 ![]() |
[26] |
McRonald FE, Pethick J, Santaniello F, et al. (2024) Identification of people with Lynch syndrome from those presenting with colorectal cancer in England: baseline analysis of the diagnostic pathway. Eur J Hum Genet 32: 529-538. https://doi.org/10.1038/s41431-024-01550-w ![]() |
[27] |
Hampel H, De La Chapelle A (2011) The search for unaffected individuals with Lynch syndrome: Do the ends justify the means?. Cancer Prev Res (Phila) 4: 1-5. https://doi.org/10.1158/1940-6207.CAPR-10-0345 ![]() |
[28] |
Grindedal EM, Renkonen-Sinisalo L, Vasen H, et al. (2010) Survival in women with MMR mutations and ovarian cancer: A multicentre study in Lynch syndrome kindreds. J Med Genet 47: 99-102. https://doi.org/10.1136/JMG.2009.068130 ![]() |
[29] |
Broaddus RR, Lynch HT, Chen L, et al. (2006) Pathologic features of endometrial carcinoma associated with HNPCC: A comparison with sporadic endometrial carcinoma. Cancer 106: 87-94. https://doi.org/10.1002/CNCR.21560 ![]() |
[30] |
Christianson CA, Powell KP, Hahn SE, et al. (2012) The use of a family history risk assessment tool within a community health care system: views of primary care providers. J Genet Couns 21: 652-661. https://doi.org/10.1007/S10897-011-9479-1 ![]() |
[31] |
Crosbie EJ, Ryan NA, Arends MJ, et al. (2019) The Manchester International Consensus Group recommendations for the management of gynecological cancers in Lynch syndrome. Genet Med 21: 2390-2400. https://doi.org/10.1038/S41436-019-0489-Y ![]() |
[32] | Weiss JM, Gupta S, Burke C, et al. (2021) NCCN guidelines® insights: Genetic/familial high-risk assessment: Colorectal, version 1.2021. J Natl Compr Canc Netw 19: 1122-1132. https://doi.org/10.1164/jnccn.2021.0048 |
[33] |
Burn J, Sheth H, Elliott F, et al. (2020) Cancer prevention with aspirin in hereditary colorectal cancer (Lynch syndrome), 10-year follow-up and registry-based 20-year data in the CAPP2 study: a double-blind, randomised, placebo-controlled trial. Lancet 395: 1855-1863. ![]() |
[34] |
Burton AM, Peterson SK, Marani SK, et al. (2010) Health and lifestyle behaviors among persons at risk for Lynch syndrome. Cancer Causes Control 21: 513-521. https://doi.org/10.1007/S10552-009-9482-0 ![]() |
[35] |
Kurose K, Zhou XP, Araki T, et al. (2001) Frequent loss of PTEN expression is linked to elevated phosphorylated Akt levels, but not associated with p27 and cyclin D1 expression, in primary Epithelial ovarian carcinomas. Am J Pathol 158: 2097-2106. https://doi.org/10.1016/S0002-9440(10)64681-0 ![]() |
[36] |
Li X, Miao C, Wang L, et al. (2023) Estrogen promotes Epithelial ovarian cancer cells proliferation via down-regulating expression and activating phosphorylation of PTEN. Arch Biochem Biophys 743: 109662. https://doi.org/10.1016/J.ABB.2023.109662 ![]() |
[37] | Syed V, Ho SM (2003) Progesterone-induced apoptosis in immortalized normal and malignant human ovarian surface epithelial cells involves enhanced expression of FasL. Oncogene 2003 22: 6883-6890. https://doi.org/10.1038/sj.onc.1206828 |
[38] |
Syed V, Ulinski G, Mok SC, et al. (2002) Reproductive hormone-induced, STAT3-mediated interleukin 6 action in normal and malignant human ovarian surface epithelial cells. JNCI: J Natl Cancer I 94: 617-629. https://doi.org/10.1093/JNCI/94.8.617 ![]() |
[39] |
Sarwar S, Alamro A, Huq F, et al. (2022) Insights into the role of epigenetic factors determining the estrogen response in estrogen-positive ovarian cancer and prospects of combining epi-drugs with endocrine therapy. Front Genet 13: 812077. https://doi.org/10.3389/FGENE.2022.812077 ![]() |
[40] |
Zhou B, Sun Q, Cong R, et al. (2008) Hormone replacement therapy and ovarian cancer risk: A meta-analysis. Gynecol Oncol 108: 641-651. https://doi.org/10.1016/j.ygyno.2007.12.003 ![]() |
[41] |
Mungenast F, Thalhammer T (2014) Estrogen biosynthesis and action in ovarian cancer. Front Endocrinol 5: 192. https://doi.org/10.3389/FENDO.2014.00192 ![]() |
[42] |
Choi JH, Wong AST, Huang HF, et al. (2007) Gonadotropins and ovarian cancer. Endocr Rev 28: 440-461. https://doi.org/10.1210/ER.2006-0036 ![]() |
[43] |
Yang H, Dai H, Li L, et al. (2019) Age at menarche and epithelial ovarian cancer risk: A meta-analysis and Mendelian randomization study. Cancer Med 8: 4012-4022. https://doi.org/10.1002/CAM4.2315 ![]() |
[44] | Nowak-Markwitz E, Spaczyński M (2012) Ovarian cancer–modern approach to its origin and histogenesis. Ginekol Pol 83: 454-457. |
[45] |
Kujawa KA, Lisowska KM (2015) Rak jajnika–od biologii do kliniki. Postepy Hig Med Dosw 69: 1275-1290. ![]() |
[46] | Ochman K Utrata heterozygotyczności loci sprzężonych z genami BRCA1 i BRCA2 w rakach jajnika (2006). |
[47] |
Moorman PG, Havrilesky LJ, Gierisch JM, et al. (2013) Oral contraceptives and risk of ovarian cancer and breast cancer among high-risk women: A systematic review and meta-analysis. J Clin Oncol 31: 4188-4198. https://doi.org/10.1200/JCO.2013.48.9021 ![]() |
[48] |
Merritt MA, Riboli E, Murphy N, et al. (2015) Reproductive factors and risk of mortality in the European prospective investigation into cancer and nutrition; a cohort study. BMC Med 13: 252. https://doi.org/10.1186/s12916-015-0484-3 ![]() |
[49] | Syed V, Ulinski G, Mok SC, et al. (2001) Expression of gonadotropin receptor and growth responses to key reproductive hormones in normal and malignant human ovarian surface epithelial cells. Cancer Res 61: 6768-6776. |
[50] |
Ye Q, Cai W, Zheng Y, et al. (2014) ERK and AKT signaling cooperate to translationally regulate survivin expression for metastatic progression of colorectal cancer. Oncogene 33: 1828-1839. https://doi.org/10.1038/ONC.2013.122 ![]() |
[51] |
Modugno F (2004) Ovarian cancer and polymorphisms in the androgen and progesterone receptor genes: A HuGE review. Am J Epidemiol 159: 319-335. https://doi.org/10.1093/AJE/KWH046 ![]() |
[52] |
Pearce CL, Wu AH, Gayther SA, et al. (2008) Progesterone receptor variation and risk of ovarian cancer is limited to the invasive endometrioid subtype: results from the ovarian cancer association consortium pooled analysis. Br J Cancer 98: 282-288. https://doi.org/10.1038/SJ.BJC.6604170 ![]() |
[53] |
Terry KL, De Vivo I, Titus-Ernstoff L, et al. (2005) Genetic variation in the progesterone receptor gene and ovarian cancer risk. Am J Epidemiol 161: 442-451. https://doi.org/10.1093/AJE/KWI064 ![]() |
[54] |
Leite DB, Junqueira MG, de Carvalho CV, et al. (2008) Progesterone receptor (PROGINS) polymorphism and the risk of ovarian cancer. Steroids 73: 676-680. https://doi.org/10.1016/J.STEROIDS.2008.02.005 ![]() |
[55] |
Runnebaum IB, Wang-Gohrke S, Vesprini D, et al. (2001) Progesterone receptor variant increases ovarian cancer risk in BRCA1 and BRCA2 mutation carriers who were never exposed to oral contraceptives. Pharmacogenetics 11: 635-638. https://doi.org/10.1097/00008571-200110000-00010 ![]() |
[56] |
Throwba P.K H, Unnikrishnan L, Pangath M, et al. (2022) The epigenetic correlation among ovarian cancer, endometriosis and PCOS: A review. Crit Rev Oncol Hematol 180: 103852. https://doi.org/10.1016/J.CRITREVONC.2022.103852 ![]() |
[57] |
Reid BM, Permuth JB, Sellers TA (2017) Epidemiology of ovarian cancer: A review. Cancer Biol Med 14: 9-32. https://doi.org/10.20892/J.ISSN.2095-3941.2016.0084 ![]() |
[58] |
Troisi R, Bjørge T, Grotmol T, et al. (2018) The role of pregnancy, perinatal factors, and hormones in maternal cancer risk: A review of the evidence. J Intern Med 283: 430-445. https://doi.org/10.1111/JOIM.12747 ![]() |
[59] |
Tsilidis KK, Allen NE, Key TJ, et al. (2011) Oral contraceptive use and reproductive factors and risk of ovarian cancer in the European Prospective Investigation into Cancer and Nutrition. Br J Cancer 105: 1436-1442. https://doi.org/10.1038/bjc.2011.371 ![]() |
[60] |
Toufakis V, Katuwal S, Pukkala E, et al. (2021) Impact of parity on the incidence of ovarian cancer subtypes: a population-based case-control study. Acta Oncol 60: 850-855. https://doi.org/10.1080/0284186X.2021.1919754 ![]() |
[61] |
Ramírez-de-Arellano A, Villegas-Pineda JC, Hernández-Silva CD, et al. (2021) The relevant participation of prolactin in the genesis and progression of gynecological cancers. Front Endocrinol 12: 747810. https://doi.org/10.3389/FENDO.2021.747810 ![]() |
[62] |
Lurie G, Wilkens LR, Thompson PJ, et al. (2008) Combined oral contraceptive use and epithelial ovarian cancer risk: Time-related effects. Epidemiology 19: 237-243. https://doi.org/10.1097/EDE.0B013E31816334C5 ![]() |
[63] | Samkari AA, Alsulami M, Bataweel L, et al. (2022) Body microbiota and its relationship with benign and malignant breast tumors: A systematic review. Cureus 14: e25473. https://doi.org/10.7759/CUREUS.25473 |
[64] |
Reitano E, de'Angelis N, Gavriilidis P, et al. (2021) Oral bacterial microbiota in digestive cancer patients: A systematic review. Microorganisms 9: 2585. https://doi.org/10.3390/microorganisms9122585 ![]() |
[65] |
Yu X, Shi Y, Yuan R, et al. (2023) Microbial dysbiosis in oral squamous cell carcinoma: A systematic review and meta-analysis. Heliyon 9: e13198. https://doi.org/10.1016/J.HELIYON.2023.E13198 ![]() |
[66] |
Mun LS, Lum SW, Sze GKY, et al. (2021) Association of microbiome with oral squamous cell carcinoma: A systematic review of the metagenomic studies. Int J Environ Res Public Health 18: 7224. https://doi.org/10.3390/IJERPH18147224 ![]() |
[67] |
Banerjee S, Tian T, Wei Z, et al. (2017) The ovarian cancer oncobiome. Oncotarget 8: 36225-36245. https://doi.org/10.18632/ONCOTARGET.16717 ![]() |
[68] |
Hu X., et al. (2023) Gut microbiota dysbiosis promotes the development of epithelial ovarian cancer via regulating Hedgehog signaling pathway. Gut Microbes 15: 2221093. https://doi.org/10.1080/19490976.2023.2221093 ![]() |
[69] |
Siddiqui R, Makhlouf Z, Alharbi AM, et al. (2022) The gut microbiome and female health. Biology 11: 1683. https://doi.org/10.3390/BIOLOGY11111683 ![]() |
[70] |
Sipos A, Ujlaki G, Mikó E, et al. (2021) The role of the microbiome in ovarian cancer: mechanistic insights into oncobiosis and to bacterial metabolite signaling. Mol Med 27: 33. https://doi.org/10.1186/S10020-021-00295-2 ![]() |
[71] |
Nené NR, Reisel D, Leimbach A, et al. (2019) Association between the cervicovaginal microbiome, BRCA1 mutation status, and risk of ovarian cancer: A case-control study. Lancet Oncol 20: 1171-1182. https://doi.org/10.1016/S1470-2045(19)30340-7 ![]() |
[72] |
Łaniewski P, Ilhan ZE, Herbst-Kralovetz MM (2020) The microbiome and gynaecological cancer development, prevention and therapy. Nat Rev Urol 17: 232-250. https://doi.org/10.1038/S41585-020-0286-Z ![]() |
[73] |
Lu YC, Yeh WC, Ohashi PS (2008) LPS/TLR4 signal transduction pathway. Cytokine 42: 145-151. https://doi.org/10.1016/J.CYTO.2008.01.006 ![]() |
[74] |
Bertani B, Ruiz N (2018) Function and biogenesis of lipopolysaccharides. EcoSal Plus 8: 1-19. https://doi.org/10.1128/ECOSALPLUS.ESP-0001-2018 ![]() |
[75] |
Cazzaniga M, Cardinali M, Di Pierro F, et al. (2022) Ovarian microbiota, ovarian cancer and the underestimated role of HPV. Int J Mol Sci 23: 16019. https://doi.org/10.3390/IJMS232416019 ![]() |
[76] |
Gulve N, Rudel T (2019) Chlamydia trachomatis and human herpesvirus 6 infections in ovarian cancer—Casual or causal?. PLoS Pathog 15: e1008055. https://doi.org/10.1371/JOURNAL.PPAT.1008055 ![]() |
[77] |
Pathak S, Wilczyński JR, Paradowska E (2020) Factors in oncogenesis: Viral infections in ovarian cancer. Cancers 12: 561. https://doi.org/10.3390/CANCERS12030561 ![]() |
[78] |
Gonzalez SL, Stremlau M, He X, et al. (2001) Degradation of the retinoblastoma tumor suppressor by the human papillomavirus type 16 E7 oncoprotein is important for functional inactivation and is separable from proteasomal degradation of E7. J Virol 75: 7583-7591. https://doi.org/10.1128/JVI.75.16.7583-7591.2001 ![]() |
[79] |
Liu X, Clements A, Zhao K, et al. (2006) Structure of the human papillomavirus E7 oncoprotein and its mechanism for inactivation of the retinoblastoma tumor suppressor. J Biol Chem 281: 578-586. https://doi.org/10.1074/JBC.M508455200 ![]() |
[80] |
Paradowska E, Jabłońska A, Studzińska M, et al. (2019) Detection and genotyping of CMV and HPV in tumors and fallopian tubes from epithelial ovarian cancer patients. Sci Rep 9: 19935. https://doi.org/10.1038/s41598-019-56448-1 ![]() |
[81] |
Malisic E, Jankovic R, Jakovljevic K (2012) Detection and genotyping of human papillomaviruses and their role in the development of ovarian carcinomas. Arch Gynecol Obstet 286: 723-728. https://doi.org/10.1007/S00404-012-2367-6 ![]() |
[82] |
Svahn MF, Faber MT, Christensen J, et al. (2014) Prevalence of human papillomavirus in epithelial ovarian cancer tissue. A meta-analysis of observational studies. Acta Obstet Gyn Scan 93: 6-19. https://doi.org/10.1111/AOGS.12254 ![]() |
[83] |
Olsen CM, Green AC, Whiteman DC, et al. (2007) Obesity and the risk of epithelial ovarian cancer: A systematic review and meta-analysis. Eur J Cancer 43: 690-709. https://doi.org/10.1016/J.EJCA.2006.11.010 ![]() |
[84] |
Urbute A, Frederiksen K, Kjaer SK (2022) Early adulthood overweight and obesity and risk of premenopausal ovarian cancer, and premenopausal breast cancer including receptor status: Prospective cohort study of nearly 500,000 Danish women. Ann Epidemiol 70: 61-67. https://doi.org/10.1016/J.ANNEPIDEM.2022.03.013 ![]() |
[85] |
Liu ZY, Gao X, Zhu S, et al. (2019) Dietary inflammatory index and risk of gynecological cancers: A systematic review and meta-analysis of observational studies. J Gynecol Oncol 30: e32. https://doi.org/10.3802/JGO.2019.30.E23 ![]() |
[86] |
Ding N, Zhan J, Shi Y, et al. (2022) Obesity in children and adolescents and the risk of ovarian cancer: A systematic review and dose–response meta-analysis. PLoS One 17: e0278050. https://doi.org/10.1371/JOURNAL.PONE.0278050 ![]() |
[87] |
Friedenreich CM, Ryder-Burbidge C, McNeil J (2021) Physical activity, obesity and sedentary behavior in cancer etiology: Epidemiologic evidence and biologic mechanisms. Mol Oncol 15: 790-800. https://doi.org/10.1002/1878-0261.12772 ![]() |
[88] |
Baczewska M, Bojczuk K, Kołakowski A, et al. (2021) Obesity and energy substrate transporters in ovarian cancer—Review. Molecules 26: 1659. https://doi.org/10.3390/MOLECULES26061659 ![]() |
[89] | Khanlarkhani N, Azizi E, Amidi F, et al. (2022) Metabolic risk factors of ovarian cancer: A review. JBRA Assist Reprod 26: 335-347. https://doi.org/10.5935/1518-0557.20210067 |
[90] |
Kolomeyevskaya N, Eng KH, Khan ANH, et al. (2015) Cytokine profiling of ascites at primary surgery identifies an interaction of tumor necrosis factor-α and interleukin-6 in predicting reduced progression-free survival in epithelial ovarian cancer. Gynecol Oncol 138: 352-357. https://doi.org/10.1016/J.YGYNO.2015.05.009 ![]() |
[91] | Ray A, Fornsaglio J, Dogan S, et al. (2024) Gynaecological cancers and leptin: A focus on the endometrium and ovary. Facts Views Vis Obgyn 10: 5-18. |
[92] |
Larsson SC, Spyrou N, Mantzoros CS (2022) Body fatness associations with cancer: Evidence from recent epidemiological studies and future directions. Metabolism 137: 155326. https://doi.org/10.1016/J.METABOL.2022.155326 ![]() |
[93] |
Wu D, Yang H, Winham SJ, et al. (2018) Mediation analysis of alcohol consumption, DNA methylation, and epithelial ovarian cancer. J Hum Genet 63: 339-348. https://doi.org/10.1038/S10038-017-0385-8 ![]() |
[94] |
Cvetkovic D (2003) Early events in ovarian oncogenesis. Reprod Biol Endocrinol 1: 68. https://doi.org/10.1186/1477-7827-1-68 ![]() |
[95] |
Liu S, Feng S, Du F, et al. (2023) Association of smoking, alcohol, and coffee consumption with the risk of ovarian cancer and prognosis: a mendelian randomization study. BMC Cancer 23: 256. https://doi.org/10.1186/S12885-023-10737-1 ![]() |
[96] |
Cook LS, Leung AC, Swenerton K, et al. (2016) Adult lifetime alcohol consumption and invasive epithelial ovarian cancer risk in a population-based case-control study. Gynecol Oncol 140: 277-284. https://doi.org/10.1016/j.ygyno.2015.12.005 ![]() |
[97] |
Tanha K, Mottaghi A, Nojomi M, et al. (2021) Investigation on factors associated with ovarian cancer: An umbrella review of systematic review and meta-analyses. J Ovarian Res 14: 153. https://doi.org/10.1186/S13048-021-00911-Z ![]() |
[98] |
Pelucchi C, Bosetti C, Galeone C, et al. (2015) Dietary acrylamide and cancer risk: An updated meta-analysis. Int J Cancer 136: 2912-2922. https://doi.org/10.1002/IJC.29339 ![]() |
[99] |
Kotsopoulos J, Vitonis AF, Terry KL, et al. (2009) Coffee intake, variants in genes involved in caffeine metabolism, and the risk of epithelial ovarian cancer. Cancer Causes Control 20: 335-344. https://doi.org/10.1007/S10552-008-9247-1 ![]() |
[100] |
Pan JY, Haile RW, Templeton A, et al. (2018) Worldwide practice patterns in Lynch syndrome diagnosis and management, based on data from the international mismatch repair consortium. Clin Gastroenterol H 16: 1901-1910. https://doi.org/10.1016/j.cgh.2018.04.025 ![]() |
[101] |
Harris HR, Terry KL (2016) Polycystic ovary syndrome and risk of endometrial, ovarian, and breast cancer: A systematic review. Fertil Res Pract 2: 14. https://doi.org/10.1186/S40738-016-0029-2 ![]() |
[102] |
Rosenthal AN, Fraser LS, Philpott S, et al. (2017) Evidence of stage shift in women diagnosed with ovarian cancer during phase II of the United Kingdom familial ovarian cancer screening study. J Clin Oncol 35: 1411-1420. https://doi.org/10.1200/JCO.2016.69.9330 ![]() |
1. | Hong Li, Zhantao Li, Chaohong Pan, Jie Song, Mingji Zhang, Cubic-like Features of I–V Relations via Classical Poisson–Nernst–Planck Systems Under Relaxed Electroneutrality Boundary Conditions, 2024, 13, 2075-1680, 790, 10.3390/axioms13110790 | |
2. | Xiangshuo Liu, Jie Song, Lijun Zhang, Mingji Zhang, Roles Played by Critical Potentials in the Study of Poisson–Nernst–Planck Models with Steric Effects Under Relaxed Neutral Boundary Conditions, 2025, 14, 2075-1680, 69, 10.3390/axioms14010069 |
Parameter | Value | Parameter | Value |
0.4 | 0.4 | ||
0.1 | 0.2 | ||
0.2 | 0.5 | ||
0.5 | 0.2 | ||
0.5 | 0.2 | ||
0.3 | 0.05 | ||
0.5 |
Parameter | Value | Parameter | Value |
1.2 | 0.4 | ||
0.6 | 0.2 | ||
0.5 | 0.6 | ||
1 | 0.6 | ||
1 | 0.2 | ||
0.3 | 0.3 | ||
0.5 |