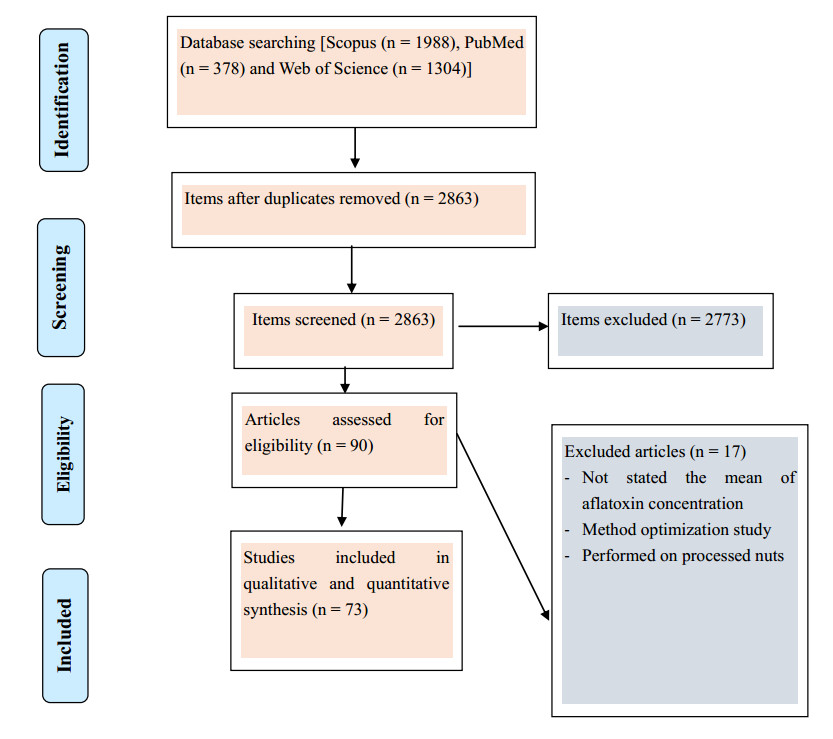
We consider a volume constraint problem for the nonlocal doubly nonlinear parabolic equation, called the nonlocal p-Sobolev flow, and introduce a nonlinear intrinsic scaling, converting a prototype nonlocal doubly nonlinear parabolic equation into the nonlocal p-Sobolev flow. This paper is dedicated to Giuseppe Mingione on the occasion of his 50th birthday, who is a maestro in the regularity theory of PDEs.
Citation: Masashi Misawa, Kenta Nakamura, Yoshihiko Yamaura. A volume constraint problem for the nonlocal doubly nonlinear parabolic equation[J]. Mathematics in Engineering, 2023, 5(6): 1-26. doi: 10.3934/mine.2023098
[1] | Sanjaykumar Kinge, B. Sheela Rani, Mukul Sutaone . Restored texture segmentation using Markov random fields. Mathematical Biosciences and Engineering, 2023, 20(6): 10063-10089. doi: 10.3934/mbe.2023442 |
[2] | Rafsanjany Kushol, Md. Hasanul Kabir, M. Abdullah-Al-Wadud, Md Saiful Islam . Retinal blood vessel segmentation from fundus image using an efficient multiscale directional representation technique Bendlets. Mathematical Biosciences and Engineering, 2020, 17(6): 7751-7771. doi: 10.3934/mbe.2020394 |
[3] | WenJun Zhou, Tianfei Wang, Yuhang He, Shenghua Xie, Anguo Luo, Bo Peng, Lixue Yin . Contrast U-Net driven by sufficient texture extraction for carotid plaque detection. Mathematical Biosciences and Engineering, 2023, 20(9): 15623-15640. doi: 10.3934/mbe.2023697 |
[4] | Xin Yi, Chen Peng, Zhen Zhang, Liang Xiao . The defect detection for X-ray images based on a new lightweight semantic segmentation network. Mathematical Biosciences and Engineering, 2022, 19(4): 4178-4195. doi: 10.3934/mbe.2022193 |
[5] | Macarena Boix, Begoña Cantó . Using wavelet denoising and mathematical morphology in the segmentation technique applied to blood cells images. Mathematical Biosciences and Engineering, 2013, 10(2): 279-294. doi: 10.3934/mbe.2013.10.279 |
[6] | Yue Li, Hongmei Jin, Zhanli Li . A weakly supervised learning-based segmentation network for dental diseases. Mathematical Biosciences and Engineering, 2023, 20(2): 2039-2060. doi: 10.3934/mbe.2023094 |
[7] | Ansheng Ye, Xiangbing Zhou, Kai Weng, Yu Gong, Fang Miao, Huimin Zhao . Image classification of hyperspectral remote sensing using semi-supervised learning algorithm. Mathematical Biosciences and Engineering, 2023, 20(6): 11502-11527. doi: 10.3934/mbe.2023510 |
[8] | Yafei Liu, Linqiang Yang, Hongmei Ma, Shuli Mei . Adaptive filter method in Bendlet domain for biological slice images. Mathematical Biosciences and Engineering, 2023, 20(6): 11116-11138. doi: 10.3934/mbe.2023492 |
[9] | Chao Zhao, Bing Li, KaiYuan Guo . Adaptive enhancement design of non-significant regions of a Wushu action 3D image based on the symmetric difference algorithm. Mathematical Biosciences and Engineering, 2023, 20(8): 14793-14810. doi: 10.3934/mbe.2023662 |
[10] | Tingxi Wen, Hanxiao Wu, Yu Du, Chuanbo Huang . Faster R-CNN with improved anchor box for cell recognition. Mathematical Biosciences and Engineering, 2020, 17(6): 7772-7786. doi: 10.3934/mbe.2020395 |
We consider a volume constraint problem for the nonlocal doubly nonlinear parabolic equation, called the nonlocal p-Sobolev flow, and introduce a nonlinear intrinsic scaling, converting a prototype nonlocal doubly nonlinear parabolic equation into the nonlocal p-Sobolev flow. This paper is dedicated to Giuseppe Mingione on the occasion of his 50th birthday, who is a maestro in the regularity theory of PDEs.
Nuts are one of the foods consumed by a vast range of ages. These foods are good sources of nutrients, including essential amino acids, proteins, minerals, vitamins, and fatty acids essential in health. Research by the European Cancer and Nutrition Research (EPIC) found that the average consumption of nuts in ten European countries (the United Kingdom, Greece, France, Italy, Netherlands, Germany, Germany, Spain, Norway, and Denmark) was 30.8 g/day [1]. A similar study in the United States showed that the average consumption of nuts was 11.75 g/day. In contrast, the average nut consumption in Australia was 4.6 g/day. Based on nutrition surveys worldwide, 8.9 of nuts g per day can be considered the average consumption of nuts globally, which could notably vary by country/region [2,3]. Relating, in European countries, almost 15% of daily food is consists of nuts or one type of nut. Consumption of nuts in different age groups is typical due to strengthening the immune system, especially in children and the elderly. Besides, any contaminants and mycotoxins in nuts consumed by those groups have remained a significant concern [3,4,5].
The presence of aflatoxin in nuts is a primary concern of consumers and regulatory systems [6,7]. In this regard, several studies have been performed to determine the concentration of aflatoxins on different nuts (walnuts, pistachios, almonds, Brazil nuts, etc.) [8,9]. Various factors such as lack of crop care during growth and pre-harvest, improper harvesting methods, and post-harvest processes (sorting, drying, packaging, storage, and transportation) potentially affect the presence and occurrence of aflatoxins. In addition, geographically, climate change in temperature and humidity can create a favorable environment for fungal growth and aflatoxin production[10,11]. In general, due to a lack or weakness of GMP (good manufacturing practices), the risk of food contamination by mycotoxin-producing fungi like Aspergillus flavus, Aspergillus parasiticus, and Aspergillus nomius increases[12,13].
Aflatoxins contamination in nuts is a critical defect due to its heat-resistant nature [14,15]. Studies have shown that food processing techniques are not efficient in eliminating aflatoxin [16]. The European Commission Rapid Alert System for Food and Feed (RASEF) adopts several alerts or notifications for identifying aflatoxin contaminations in nuts originating from different countries annually [17].
According to epidemiological studies, a strong link between AFB1 consumption and cancer occurrence has been documented [18]. The International Agency for Research on Cancer (IARC) categorized AFB1 in group1 [19]. In addition, AFB1 has been considered a teratogen, mutagen, immune system modulator, and growth suppressant contaminant [20,21,22]. Other types of aflatoxin, including aflatoxin G1 (AFG1), aflatoxin B2 (AFB2), and aflatoxin G2 (AFG2), are classified in group 2 as a possible carcinogen for humans [19,23,24].
Currently, strict regulations for aflatoxins have been employed by many countries to promote the safety of nuts as high-risk contaminated food products. Mainly, these standards were noteworthy for exporting and importing nuts worldwide [25]. Furthermore, monitoring programs have been implemented in countries regarding the public health risks associated with aflatoxins ingestion via the consumption of different nuts [8,26,27,28]. According to the identification of aflatoxin as a hazard, its risk assessment involves determining nut samples' concentrations and consumption data [29].
The probabilistic assessment is considered a helpful tool for assessing cancer risk in various human body organs, especially the liver, due to exposure to AFB1. The health risk related to aflatoxins from nuts can be determined by considering carcinogenic risk and daily consumption[30]. The Monte Carlo simulation (MCS), a valuable model, could meet the uncertainty of health risks[31]. In this method, the amplitude values of the variables that are randomly considered in the health risk assessment equation are obtained until the predicted distribution indicates the uncertainty of the input variables.
To our knowledge, there is no comprehensive review of systematic review to assess aflatoxin levels in nuts. Therefore, our aim is to conduct a systematic review and healh risk assessment of aflatoxins by international studies based on the available evidence on the levels of aflatoxins B1, B2, G1, G2 and the total aflatoxins in different nut samples (such as walnuts, pistachios, almonds, Brazil nuts, etc.).
The fitting studies were explored in PubMed, Web of Science, and Scopus, from 2000 to June 12, 2020. The used keywords for the systematic search were: (aflatoxin OR mycotoxin OR mycotoxins) AND (nuts OR peanut OR walnut OR almond OR pistachio OR hazelnut) AND (incidence OR level OR prevalence OR concentration OR contamination).
Two researchers reviewed the title and abstract of articles and chose them according to: (1) published in the English language; (2) reporting the concentration of aflatoxins in nuts; (3) investigated nuts including peanuts, almond, walnut, pistachio, hazelnuts, Brazil nut or other types of nuts; and (4) didn't report aflatoxin concentration as a range. The excluded articles included the studies which had not matched the criteria.
Two authors extracted data from qualified articles. Any conflicts were discussed to reach an agreement. The data were documented from each study according to the first author's name, sampling country, publication year, analysis method, type of nut, the mean and standard deviation of aflatoxin concentration, aflatoxin type (AFB1 and AFT), and standard error. Regarding nuts, the main subgroups were peanuts, pistachio, walnut, almond, Brazil nuts, and hazelnut. In addition, other nuts such as cashew nuts, Betelnut, areca nut, Macadamia, Pinhol, Pecan, Assorted nuts, mixed nuts, etc., were categorized into other nuts.
The Chronic Daily Intake (CDI) of AFB1 from nuts was determined by Equation (1) [32]:
CDI=C×IRi×EDi×EFiBW×AT | (1) |
This is assumed that nuts are consumed 365 d/year, C is the content of AFB1 in nuts (ng/g) which determined as mean of AFB1 in countries; IRi as the global daily intake of nuts were noticed as g/day (8.9 g/day) [2]; ED is the time-period (70 years); EFi is the exposure frequency (350 days/year); [33,34]; BWi is the average body weight (70 kg) and AT is the mean time life [35,36,37,38].
The margin of exposure (MOE) was used to evaluate the carcinogenic risk of AFB1 for humans based on consumption of nuts (Equation (2)). The MOE was counted by dividing the benchmark dose lower confidence limit (BMDL) by CDI [39].
MoE=BMDLCDI | (2) |
BMDL is equal to 870 ng/Kg b.w. per day for AFB1 [40], and CDI is the chronic daily intake (ng/kg bw/day). MOE values of more than 10000 were considered a low concern for public health[41].
The carcinogenic (MOE) of AFB1 was estimated by Monte Carlo (MC) simulation (Crystal Ball v 11.1.2.4.600 software, Oracle, Decisioneering, Denver, CO, USA) [42]. The simulation was carried out using notified indexes, and the MC model was stated for 10,000 repetitions. Furthermore, 95th percentiles of MOE were selected for risk assessment [43,44].
In real-life applications, multivariate statistical methods may be employed in food microbiology studies for visualizing the similarities/differences between samples in a two/three-dimensional aspect plane based on the different factors [45,46]. The HCA is the most widely employed tool to investigate similarities and concealed schema among samples where associations on data and clusters are unclear, in different samples, fungal metabolites details sets, and various nut types are the targets of these methods [47,48,49]. The HCA was performed to evaluate the correlation between the type and aflatoxin content in different nuts by applying SPSS software version 18.
The literature searches and study selection process are shown in Figure 1. At initial systematic search, 3670 eligible studies were determined. Of these, 807 articles were duplicated, and another 2773 were not qualified according to the title and abstract. Finally, 90 papers were reviewed, and 73 provided sufficient information for the systematic review. The reasons for excluding are mentioned in (Figure 1).
Thirty-nine studies (53.42%) were conducted in Asia (Bahrain, Bangladesh, China, India, Iran, Japan, Korea, Malaysia, Saudi Arabia, Pakistan, South Korea, Taiwan, Thailand, Turkey, and Yemen); 16 studies (21.91%) in Africa (Cameroon, Congo, Côte d'Ivoire, Egyptian, Ghana, Morocco, Nigeria, South-western Uganda, and Zambia); 10 studies (13.69%) in Europe (Cyprus, Greece, Italy, Poland, Southern Haiti, and Spain) and 8 studies (10.95%) in South America (Brazil and Argentina). Distribution of studies included in the present review across different countries were as follows: Brazil (7) > Malaysia (6) > Iran (6) ~ Turkey (6) > Pakistan (4) > Spain (3) ~ Italy (3) ~ Saudi Arabia (3) ~ Nigeria (3) > China (2) ~ Taiwan (2) ~ Korea (2) ~ Egyptian (2) ~ Cameroon (2) ~ South-western Uganda (2) ~ Ghana (2) ~ Zambia (2) > Argentina (1) ~ Bahrain (1) ~ Bangladesh (1) ~ South Korea (1) ~ Thailand (1) ~ Yemen (1) ~ Congo (1) ~ Côte d'Ivoire (1) ~ Morocco (1) ~ Cyprus (1) ~ Greece (1) ~ Poland (1) ~ Southern Haiti (1) ~ Argentina (1) ~ India (1) ~ Japan (1).
The rank order of the used techniques for quantification of aflatoxin based on the number of studies was high-performance liquid chromatography (HPLC) (44 article, 60.27%) > thin-layer chromatography (TLC) (13 article, 17.8%) > enzyme-linked immunosorbent assay (ELISA) (8 article, 10.95%) > liquid chromatography-mass spectrometry (LC/MS/MS) (4 article, 5.47%) ~ and other methods (UPLC-MS/MS, LC-UV, MFC, MIC, RRLC-MS/MS) (4 article, 5.47%).
Among the reviewed articles, most studies were performed on peanuts. The sharing of studies investigated on nut subtypes were shown in Figure 2.
Considering the mean concentration of AFB1 in peanut samples gathered from different countries, the observed rank order was as follows: Argentina (530 µg/kg) > Congo (163.22 µg/kg) > Nigeria (110.95 µg/kg) > South-western Uganda (103.10 µg/kg) > Bangladesh (93.10 µg/kg) > Southern Haiti (58.7 µg/kg) > Zambia (51.10 µg/kg) > Cameroon (47.00 µg/kg) > Italy (42.06 µg/kg) > Brazil (31.00 µg/kg) > Ghana (18.20 µg/kg) > Malaysia (17.26 µg/kg) > China (6.48 µg/kg) > Pakistan (5.90 µg/kg) > Saudi Arabia (4.86 µg/kg) > Côte d'Ivoire (4.80 µg/kg) > South Korea (4.07 µg/kg) > Korea (3.31 µg/kg) > Cyprus (3.00 µg/kg) > Taiwan (1.56 µg/kg) > Turkey (0.96 µg/kg) > Spain (0.91 µg/kg) > Thailand (0.85 µg/kg) > Morocco (0.17 µg/kg) > Iran (0.00 µg/kg) ~ Japan (0.00 µg/kg) (Figure 3a). The final mean concentration of AFB1 in Peanuts was (37.82 µg/kg) (Table 1 Supplementary).
Country | CDI Percentiles |
|||
5% | 50% | 75% | 95% | |
Argentina | 4.39E-2 | 6.42E-2 | 7.57E-2 | 9.65E-2 |
Bahrain | 2.01E-3 | 3.06E-3 | 3.58E-3 | 4.56E-3 |
Bangladesh | 4.99E-3 | 7.36E-3 | 8.74E-3 | 1.09E-2 |
Brazil | 1.99E-3 | 2.96E-3 | 3.51E-3 | 4.44E-3 |
USA | 5.80E-4 | 8.80E-4 | 1.05E-3 | 1.34E-3 |
Cameroon | 3.80E-3 | 5.65E-3 | 6.69E-3 | 8.77E-3 |
China | 5.93E-4 | 8.76E-4 | 1.03E-3 | 1.29E-3 |
Congo | 1.33E-2 | 1.98E-2 | 2.36E-2 | 2.99E-2 |
Cyprus | 5.45E-4 | 8.09E-4 | 9.44E-4 | 1.22E-3 |
south Africa | 8.66E-4 | 1.30E-3 | 1.54E-3 | 1.92E-3 |
Egypt | 7.65E-4 | 1.14E-3 | 1.35E-3 | 1.71E-3 |
Ghana | 1.46E-3 | 2.20E-3 | 2.57E-3 | 3.28E-3 |
India | 7.62E-3 | 1.13E-2 | 1.30E-2 | 1.67E-2 |
Iran | 2.52E-3 | 3.83E-3 | 4.55E-3 | 5.71E-3 |
The mean concentrations of AFT in peanut samples gathered from different countries were as follows: Congo (197.11 µg/kg) > Nigeria (148.75 µg/kg) > South-western Uganda (96.10 µg/kg) > Southern Haiti (70.20 µg/kg) > Italy (45.36 µg/kg) > Malaysia (35.99 µg/kg) > Ghana (27.67 µg/kg) > Turkey (22.08 µg/kg) > Spain (20.73 µg/kg) > Brazil (16.99 µg/kg) > Saudi Arabia (13.33 µg/kg) > Iran (9.94 µg/kg) > Yemen (8.88 µg/kg) > Taiwan (8.20 µg/kg) > Cameroon (6.50 µg/kg) > Pakistan (6.26 µg/kg) > Korea (4.08 µg/kg) > Thailand (1.43 µg/kg) > Zambia (0.43 µg/kg) > Morocco (0.30 µg/kg) > Japan (0.00 µg/kg) (Figure 3a). The final mean concentration of AFT in Peanuts was (43.93 µg/kg) (Table 1 Supplementary).
The mean concentration of AFB1 in Pistachio samples from different countries was: Taiwan (233.60 µg/kg) > Morocco (158.00 µg/kg) > Iran (64.65 µg/kg) > Bahrain (24.90 µg/kg) > Cyprus (8.20 µg/kg) > Pakistan (5.60 µg/kg) > Saudi Arabia (5.42 µg/kg) > Turkey (4.55 µg/kg) > Korea (1.68 µg/kg) > Italy (1.29 µg/kg) > Spain (0.00 µg/kg) (Figure 3b). The final mean concentration of AFB1 in pistachio was (39.44 µg/kg) (Table 2 Supplementary).
Country | MOE Percentiles |
|||
5% | 50% | 75% | 95% | |
Argentina | 9.11 | 13.71 | 16.07 | 21.24 |
Bahrain | 189.61 | 286.41 | 343.96 | 438.91 |
Bangladesh | 75.14 | 116.99 | 136.67 | 175.36 |
Brazil | 193.02 | 296.80 | 351.51 | 447.83 |
USA | 628.94 | 975.49 | 1152.10 | 1505.80 |
Cameroon | 102.16 | 155.70 | 186.68 | 238.66 |
China | 637.79 | 993.10 | 1200.60 | 1526.20 |
Congo | 28.53 | 43.35 | 52.36 | 67.39 |
Cyprus | 664.64 | 1071.10 | 1263.50 | 1588.70 |
South Africa | 433.60 | 654.31 | 785.12 | 1017.20 |
Egypt | 506.92 | 767.02 | 927.33 | 1176.20 |
Ghana | 252.82 | 392.07 | 462.82 | 606.49 |
India | 47.86 | 75.71 | 91.40 | 117.01 |
Iran | 144.63 | 224.35 | 268.18 | 357.23 |
The order of mean concentration of AFT in Pistachio was: Taiwan (245.60 µg/kg) > Morocco (163.00 µg/kg) > Iran (56.95 µg/kg) > Bahrain (27.30 µg/kg) > Spain (21.40 µg/kg) > Saudi Arabia (16.60 µg/kg) > Pakistan (5.13 µg/kg) > Turkey (4.95 µg/kg) > Korea (1.70 µg/kg) > Italy (1.47 µg/kg) > Malaysia (0.00 µg/kg) (Figure 3b). The final mean concentration of AFT in pistachio was (35.59 µg/kg) (Table 2 Supplementary).
The mean concentration of AFB1 in almond samples from different countries was according to following rank order: Cyprus (32.90 µg/kg) > Pakistan (4.05 µg/kg) > Saudi Arabia (1.97 µg/kg) > Turkey (0.05 µg/kg) > Iran (0.00 µg/kg) ~ Korea (0.00 µg/kg) ~ Spain (0.00 µg/kg) (Figure 3c). The final mean concentration of AFB1 in almond was (3.93 µg/kg) (Table 3 Supplementary).
Nuts | Total sample number | AFT mean µg/kg | AFT range µg/kg | AFT median µg/kg | MRL µg/kg | %Studies with AFT mean < ML µg/kg | %Studies with AFT mean MRL-50 µg/kg | %Studies with AFT mean 51–200 µg/kg | %Studies with AFT mean > 200 µg/kg |
Peanuts | 4737 | 40.87 | Nd-530 | 13.25 | 15 | 61.60 | 17.85 | 16.96 | 3.57 |
Pistachios | 11552 | 37.52 | Nd-245.60 | 7.20 | 10 | 71.42 | 11.90 | 9.52 | 7.14 |
Almonds | 517 | 3.54 | Nd-32.90 | 3.10 | 10 | 90.62 | 9.37 | 0.00 | 0.00 |
Hazelnuts | 293 | 17.33 | 0.2–124 | 2.10 | 10 | 76.92 | 7.69 | 15.38 | 0.00 |
Brazil nuts | 173 | 4.61 | 0.88–36.90 | 3.00 | 10 | 92.30 | 7.69 | 0.00 | 0.00 |
AFT: Aflatoxin total (sum of aflatoxin B1, B2, G1 and G2). MRL: Maximum Residue Limits (MRLs) for aAflatoxin adopted by Codex Alimentarius. Nd: Not detected. |
Also regarding AFT in almond the fallowing order was: Turkey (3.57 µg/kg) > Pakistan (3.38 µg/kg) > Saudi Arabia (2.20 µg/kg) > China (1.16 µg/kg) > Iran (0.00 µg/kg) ~ Korea (0.00 µg/kg) (Figure 3c). The final mean concentration of AFT in almond was (3.16 µg/kg) (Table 3 Supplementary).
The data showed that the mean concentration of AFB1 in hazelnut samples from different countries was: Italy (28.15 µg/kg) > Turkey (2.97 µg/kg) > Spain (0.51 µg/kg) (Figure 3d). The final mean concentration of AFB1 in hazelnut was (10.54 µg/kg) (Table 4 Supplementary).
The mean concentration of AFT in hazelnut was determined as: Italy (41.71 µg/kg) > Turkey (19.60 µg/kg) > Saudi Arabia (2.50 µg/kg) > China (2.10 µg/kg) (Figure 3d). The final mean concentration of AFT in hazelnut was (24.13 µg/kg) (Table 4 Supplementary).
The mean concentration of AFB1 in walnut samples monitored in different countries was: Morocco (360 µg/kg) > Iran (9.00 µg/kg) > Pakistan (4.01 µg/kg) > Turkey (0.34 µg/kg) > Saudi Arabia (0.00 µg/kg) ~ South Korea (0.00 µg/kg) ~ Cyprus (0.00 µg/kg) ~ Korea (0.00 µg/kg) ~ Spain (0.00 µg/kg) (Figure 3e). The final mean concentration of AFB1 in walnut was (22.23 µg/kg) (Table 5 Supplementary).
Also regarding AFT in walnut the obtained order was: Morocco (730 µg/kg) > Iran (13.04 µg/kg) > Turkey (5.99 µg/kg) > Pakistan (4.44 µg/kg) > Saudi Arabia (2.05 µg/kg) > China (0.86 µg/kg) > Korea (0.00 µg/kg) ~ Malaysia (0.00 µg/kg) (Figure 3e). The final mean concentration of AFT in walnut was (44.84 µg/kg) (Table 5 Supplementary).
As investigated in studies the mean concentration of AFB1 in Brazil nut samples from different countries, the rank order was: Brazil (3.71 µg/kg) > Cyprus (3.00 µg/kg) (Figure 3f). The final mean concentration of Aflatoxin B1 in Brazil nuts was (3.35 µg/kg) (Table 6 Supplementary).
Also, regarding AFT in Brazil nut the order was: Brazil (3.94 µg/kg) > Malaysia (0.88 µg/kg) (Figure 3f). The final mean concentration of AFT in Brazil nut was (5.87 µg/kg) (Table 6 Supplementary).
Considering the mean concentration of AFB1 in other nuts samples the observed order was: Betelnut (62.38 µg/kg) > Sliced raw areca nut (10.86 µg/kg) > Assorted nuts (6.68 µg/kg) > Mixed nuts (5.70 µg/kg) > Cashew nuts (2.69 µg/kg) > Tigernuts (2.40 µg/kg) > Nuts Cocktail (0.33 µg/kg) > Pinhol (0.04 µg/kg) > Macadamia nut (0.00 µg/kg) ~ Pecan (0.00 µg/kg) (Figure 3). The final mean concentration of AFB1 in other nuts was (7.38 µg/kg) (Table 7 Supplementary).
Also regarding AFT in other nuts the fallowing order was: Chilgoza pine nut (493.91 µg/kg) > Mixed nuts (11.50 µg/kg) > Raw areca nuts (8.90 µg/kg) > Tigernuts (8.67 µg/kg) > Assorted nuts (7.89 µg/kg) > Cashew nuts (7.12 µg/kg) > Macadamia nut (0.00 µg/kg) ~ Pecan (0.00 µg/kg) ~ Pine nuts (0.00 µg/kg). The final mean concentration of AFT in other nuts was (30.52 µg/kg) (Table 7 Supplementary).
Hierarchical clustering was used to understand the relationships among the AFT, aflatoxin type, and nut type in different countries. Cluster analysis showed the grouping of accessions between two significant clusters and two sub-clusters (Figure 4). The first cluster includes the aflatoxin type and the nut type, containing two sub-groups. The second cluster only includes AFT. The aflatoxin type and nut type in different countries were closer, indicating that these variables had similar trends in different samples.
The exposure assessment of AFB1 through nuts consumption was simulated by the Monte Carlo approach, as shown in Table 1. The results for AFB1 revealed that Argentina had a higher mean intake (6.42E μg kg bw−1 day−1) compared to the other countries.
Occurrence and risk characterization by a margin of exposure of AFB1 was calculated using a probabilistic approach. The rank order of countries based on the country in term of margin of exposure value was Argentina > Congo > India > Bangladesh > Cameroon > Iran > Bahrain > Brazil > Ghana > south Africa > Egypt > USA > China > Cyprus (Table 2), which the MOE of the consumers in some countries was considerably below the safety margin of hepatic carcinogen (MoE > 10,000).
The present systematic review study updates the aflatoxins content status in nuts, including peanut, pistachio, walnut, hazelnut, Brazil nut, almond, and other nuts from different origins and markets. According to include studies, AFB1was the predominant notified mycotoxin in nut samples. In addition, in a more studied data series, the mean concentration of AFT was similar to AFB1 content, confirming the importance of AFB1 as the main Aflatoxin associated with nuts. However, each nut's susceptibility is different as peanut and pistachio were the most notified contaminated commodities from the nut category in reviewed studies. In total, a wide diverse mean level range of aflatoxin was reported in nuts samples from a different area. Also, from all reviewed studies, the levels of aflatoxins in countries were discussed by different standard maximum levels. Among the analytical methods developed to quantify aflatoxins, the HPLC and TLC were the most common methods widely used in all research.
Aflatoxin contamination is a critical defect in nuts and tree nuts. Due to nut type variability, different cultivation/production zones, and altered agriculture practice, the concentration of aflatoxin dramatically varies from place to place, from year to year also vary among the nuts cultivars [50,51]. Besides increasing public knowledge about food safety, international action has focused on monitoring food hazards. Aflatoxin is one of the notified hazards considered a significant food safety issue[52]. Therefore, crucially establishing a tolerance limit for aflatoxin contamination has tremendous importance[53,54]. The regulations have been set in many countries from past decades, yet newer rules are being established. The details about the maximum level of aflatoxins in high-risk food commodities such as nuts and sampling/analyzing protocols have been revised and republished. In this base, the comparing of aflatoxins content is complex from country to country[11]. From the joint of FAO/WHO, Codex Alimentarius is the central committee that sets principal standards for contaminant and natural toxicants in food and feed.
The Codex standard states that the maximum levels (ML) of AFT for peanut, pistachio, hazelnut, almond, and Brazil nut for processing is 15 µg/kg. For pistachio, hazelnut, almond, and Brazil nut for direct human consumption (ready to eat) is 10 µg/kg (Table 3). Also, the criteria for sampling and quantification methods are defined by the Codex standard [55]. Many countries accepted and followed the Codex legislation as global standards for aflatoxin occurrence evaluation. Comparing this standard, the final mean level of AFT in all nuts except in peanuts (13.25 µg/kg) was lower than the maximum residue limit (10 µg/kg). Some studies compare the data with the European Union (EU), establishing its maximum residue limit (MRL) for aflatoxin in nuts. The EU legislation seems to be harmonized with Codex in some parts and be stricter than codex ones in others. In addition, the USA, China, Australia, Canada, and Japan have been issued the regulatory MRLs for aflatoxin and Guidance for the producer industry [11].
The aflatoxin occurrence data are significantly varied beyond the world. Also, some countries are owing limited or lack available data about aflatoxin contamination in food and nuts. Furthermore, several prevalence/ incidence data are from official monitoring programs, mainly agreeing with the MRLs. Therefore, the data series has skewness and is often lower than aflatoxin's actual level at the farm [11,36]. A report from EFSA (2007) regarding aflatoxin analysis in 34,326 samples from EU countries revealed that Brazil nut, pistachio, and spices were the highest contaminated samples. Of 168 samples containing more than 200 µg/kg, 110 samples belonged to pistachios, 30 samples to Brazil nuts, 23 samples to peanuts, 3 samples to other nuts, and two samples to almonds [40].
The aflatoxin occurrences evaluation in our study indicated a heterogeneous aflatoxin distribution in nuts. In most studies, mean values were low, a few were high, and some were very high. Also, high mean concentration values were reported by several studies such as 730 µg/kg AFT for walnut (Juan 2008), 530 µg/kg AFB1 for peanut [56], 493.91 µg/kg AFT for nut [12], 440 µg/kg AFT for peanut [57], 360 µg/kg AF B1 for walnut (Juan 2008). As highlighted in table 3, the median was lower than the mean in all studied nut types. All these findings imply a remarkable variation in aflatoxin concentration as a contaminant.
Although aflatoxin contamination originates from the farm and continues to the consumer table, preventive strategies predominantly constitute pre-to post-harvest. However, effective safety management is needed to minimize and control this hazard in nuts. Other aspects that impact the result of aflatoxin determination in research are sampling method, analyses, detection method, the condition of sample preservation, climate. Furthermore, a glance at the country of the study illustrated that in most cases, the nut samples are originated and imported from other countries; hence the fowling of importing/exporting legislation could indirectly affect contamination.
The attainability of statistics about the content and occurrence of Aflatoxin in various nuts could provide necessary information for health risk assessment and exposure assessment. It is such an influential role to evaluate and check Aflatoxin in food. The main directly or indirectly path of population exposure to Aflatoxin is via diets. There are three intervention actions, including agricultural, dietary, and clinical intervention [58]—all these interventions are designed to diminish aflatoxin exposure. Generally, the risk evaluation study is an estimating process that can predict the adverse effect for individuals/populations. In some countries, the margin of exposure indexes derived from the consumption of peanuts, pistachios, almonds, hazelnuts, walnut, brazil nuts, and other nuts was considerably lower than the safe margin of 10,000. These findings point to a potential concern that world population health may be a global health problem.
In this context, rare studies have been assessed the risk of aflatoxin exposure in nuts. Liu and Wu (2010) estimated that Malaysians had been exposed to AFB1 ranging from 15–140 ng/kg body weight/day, reflecting 91–857% of cancer incidence related to dietary Aflatoxin [58]. In contrast, Sabran et al. (2012) reported 26.20 ng/kg body weight/day for dietary AFB1 exposure, contributing to 13.50 % of liver cancer in Malaysia [59].
In another study by Foerster (2020), the margin of exposure was reported ranging from 1133 to 8500. The results showed a public health concern for Aflatoxin in food commodities consumed in Chile [41].
Zhung et al. (2020) studied the probabilistic risk of exposure to AFB1 in Guangzhou (China) [60]. The mean of MOE was estimated at 100 to 1000 for Guangzhou residents.
A probabilistic risk assessment of AFB1 level in walnuts in Iran showed more than 50% of samples containing AFB1 at level range from 0.80–14.50 µg/kg. Low carcinogenic risk concluded for Iranians [61]. Health risk characterization for dietary exposure to AFB1 in food samples (maize, rice, peanut, and sesame) from Vietnam provinces revealed different liver cancer risks. The results pointed out AFB1 was the most detected and distributed mainly in samples, leading to 0.23, 0.65, and 21.0 liver cancer per 100,000 adults annually [62].
The rate of the population facing unwanted exposure to Aflatoxin and then liver cancer consequence are unclear. Therefore, risk assessment information helps estimate the aflatoxin exposure magnitude [29].
In aflatoxin exposure, like other pollutants, the leading considered population groups are children and adults. For drawing the exposure scenario, probabilistic models are an efficient tool. In this field, the simulation methods provide a chance to percept the validity and accuracy of estimation.
The health risk assessment demonstrated that some countries, such as Argentina, Congo, India, Bangladesh, and Cameroon, are at greater risk. Therefore, preventive strategies for reducing the aflatoxin level in nuts should be more practiced. Accordingly, the main limitation in exposure characterization is data censoring from occurrence studies and the heterogeneity of aflatoxin levels, also reliable biomarkers for detecting Aflatoxin in urine [29,63].
In this systematic review study, the concentration of aflatoxins was screened in different nuts samples, including peanuts, pistachios, walnuts, almonds, hazelnuts, Brazil nuts, and other nuts in different nuts countries. Besides, the health risk related to aflatoxin content was assessed, and the correlation between the type and amount of Aflatoxin with nut samples was evaluated by hierarchical cluster visualization. The mean concentration of AFB1 and AFT in nuts was significant in different nut types and between countries. Even though more than 60% of studies reported AFT mean concentration in the subgroups of nuts lower than the maximum allowable level set by Codex Alimentarius, risk characterization in some countries was considerably lower than the safe margin of exposure. According to determined chronic daily intake and margin of aflatoxin exposure in nut samples from all included studies, it was found that we are facing a global health problem. Since nuts are widely consumed in different consumer groups, it is necessary to emphasize strict control measures to prevent contamination of these foods with aflatoxins.
No separate funding was necessary for undertaking this systematic review.
All authors confirm that this manuscript has no conflicts of interest.
[1] |
B. Abdellaoui, A. Attar, R. Bentifour, I. Peral, On fractional p-Laplacian parabolic problem with general data, Ann. Mat. Pura Appl., 197 (2018), 329–356. https://doi.org/10.1007/s10231-017-0682-z doi: 10.1007/s10231-017-0682-z
![]() |
[2] |
E. Acerbi, N. Fusco, Regularity for minimizers of nonquadratic functionals: the case 1<p<2, J. Math. Anal. Appl., 140 (1989), 115–135. https://doi.org/10.1016/0022-247X(89)90098-X doi: 10.1016/0022-247X(89)90098-X
![]() |
[3] |
H. W. Alt, S. Luckhaus, Quasilinear elliptic-parabolic differential equations, Math. Z., 183 (1983), 311–341. https://doi.org/10.1007/BF01176474 doi: 10.1007/BF01176474
![]() |
[4] |
A. Banerjee, P. Garain, J. Kinnunen, Some local properties of subsolutons and supersolutions for a doubly nonlinear nonlocal parabolic p-Laplace equation, Ann. Mat. Pura Appl., 201 (2022), 1717–1751. https://doi.org/10.1007/s10231-021-01177-4 doi: 10.1007/s10231-021-01177-4
![]() |
[5] |
V. Bögelein, F. Duzaar, P. Marcellini, C. Scheven, Doubly nonlinear equations of porous medium type, Arch. Rational Mech. Anal., 229 (2018), 503–545. https://doi.org/10.1007/s00205-018-1221-9 doi: 10.1007/s00205-018-1221-9
![]() |
[6] |
V. Bögelein, F. Duzaar, P. Marcellini, Parabolic systems with p,q-growth: a variational approach, Arch. Rational Mech. Anal., 210 (2013), 219–267. https://doi.org/10.1007/s00205-013-0646-4 doi: 10.1007/s00205-013-0646-4
![]() |
[7] |
V. Bögelein, F. Duzaar, R. Korte, C. Scheven, The higher integrability of weak solutions of porous medium systems, Adv. Nonlinear Anal., 8 (2019), 1004–1034. https://doi.org/10.1515/anona-2017-0270 doi: 10.1515/anona-2017-0270
![]() |
[8] |
V. Bögelein, F. Duzaar, J. Kinnunen, C. Scheven, Higher integrability for doubly nonlinear parabolic systems, J. Math. Pures Appl., 143 (2020), 31–72. https://doi.org/10.1016/j.matpur.2020.06.009 doi: 10.1016/j.matpur.2020.06.009
![]() |
[9] |
V. Bögelein, F. Duzaar, N. Liao, On the Hölder regularity of signed solutions to a doubly nonlinear equation, J. Funct. Anal., 281 (2021), 109173. https://doi.org/10.1016/j.jfa.2021.109173 doi: 10.1016/j.jfa.2021.109173
![]() |
[10] |
V. Bögelein, F. Duzaar, N. Liao, L. Shätzler, On the Hölder regularity of signed solutions to a doubly nonlinear equation, part II, Rev. Mat. Iberoam., 39 (2022), 1005–1037. https://doi.org/10.4171/RMI/1342 doi: 10.4171/RMI/1342
![]() |
[11] |
V. Bögelein, N. Dietrich, M. Vestberg, Existence of solutions to a diffusive shallow medium equation, J. Evol. Equ., 21 (2021), 845–889. https://doi.org/10.1007/s00028-020-00604-y doi: 10.1007/s00028-020-00604-y
![]() |
[12] |
S. Biagi, S. Dipierro, E. Valdinoci, E. Vecchi, Mixed local and nonlocal elliptic operators: regularity and maximum principles, Commun. Part. Diff. Eq., 47 (2022), 585–629. https://doi.org/10.1080/03605302.2021.1998908 doi: 10.1080/03605302.2021.1998908
![]() |
[13] |
S. Biagi, E. Vecchi, S. Dipierro, E. Valdinoci, Semilinear elliptic equations involving mixed local and nonlocal operators, P. Roy. Soc. Edinb. A, 151 (2021), 1611–1641. https://doi.org/10.1017/prm.2020.75 doi: 10.1017/prm.2020.75
![]() |
[14] | S. Biagi, S. Dipierro, E. Valdinoci, E. Vecchi, A Faber-Krahn inequality for mixed local and nonlocal operators, JAMA, 2023. https://doi.org/10.1007/s11854-023-0272-5 |
[15] |
L. Brasco, D. Gómez-Castro, J. L. Vázquez, Characterisation of homogeneous fractional Sobolev spaces, Calc. Var., 60 (2021), 60. https://doi.org/10.1007/s00526-021-01934-6 doi: 10.1007/s00526-021-01934-6
![]() |
[16] |
L. Brasco, E. Lindgren, M. Strömqvist, Continuity of solutions to a nonlinear fractional diffusion equation, J. Evol. Equ., 21 (2021), 4319–4381. https://doi.org/10.1007/s00028-021-00721-2 doi: 10.1007/s00028-021-00721-2
![]() |
[17] |
L. Brasco, A. Salort, A note on homogeneous Sobolev spaces of fractional order, Ann. Mat. Pura Appl., 198 (2019), 1295–1330. https://doi.org/10.1007/s10231-018-0817-x doi: 10.1007/s10231-018-0817-x
![]() |
[18] |
A. Di Castro, T. Kuusi, G. Palatucci, Nonlocal Harnack inequalities, J. Funct. Anal., 267 (2014), 1807–1836. https://doi.org/10.1016/j.jfa.2014.05.023 doi: 10.1016/j.jfa.2014.05.023
![]() |
[19] |
A. Di Castro, T. Kuusi, G. Palatucci, Local behavior of fractional p-minimizers, Ann. Inst. Henri Poincare, 33 (2016), 1279–1299. https://doi.org/10.1016/j.anihpc.2015.04.003 doi: 10.1016/j.anihpc.2015.04.003
![]() |
[20] | C. De Filippis, G. Mingione, Gradient regularity in mixed local and nonlocal problems, Math. Ann., 2022. https://doi.org/10.1007/s00208-022-02512-7 |
[21] | S. Dipierro, E. P. Lippi, E. Valdinoci, (Non)local logistic equations with Neumann conditions, Ann. Inst. Henri Poincare, 2021. https://doi.org/10.4171/aihpc/57 |
[22] |
M. Giaquinta, G. Modica, Remarks on the regularity of the minimizers of certain degenerate functionals, Manuscr. Math., 57 (1986), 55–99. https://doi.org/10.1007/BF01172492 doi: 10.1007/BF01172492
![]() |
[23] | P. Grisvard, Elliptic problems in nonsmooth domains, Society for Industrial and Applied Mathematics, 1985. |
[24] |
J. Kinnunen, P. Lindqvist, Pointwise behaviour of semicontinuous supersolutions to a quasilinear parabolic equation, Ann. Mat. Pura Appl., 185 (2006), 411–435. https://doi.org/10.1007/s10231-005-0160-x doi: 10.1007/s10231-005-0160-x
![]() |
[25] | N. Kato, M. Misawa, K. Nakamura, Y. Yamaura, Existence for doubly nonlinear fractional p-Laplacian equations, arXiv, 2021. https://doi.org/10.48550/arXiv.2305.00661 |
[26] | M. Kassmann, R. W. Schwab, Regularity results for nonlocal parabolic equations, Riv. Math. Univ. Parma (N.S.), 5 (2014), 183–212. |
[27] |
T. Kuusi, M. Misawa, K. Nakamura, Regularity estimates for the p-Sobolev flow, J. Geom. Anal., 30 (2020), 1918–1964. https://doi.org/10.1007/s12220-019-00314-z doi: 10.1007/s12220-019-00314-z
![]() |
[28] |
T. Kuusi, M. Misawa, K. Nakamura, Global existence for the p-Sobolev flow, J. Differ. Equations, 279 (2021), 245–281. https://doi.org/10.1016/j.jde.2021.01.018 doi: 10.1016/j.jde.2021.01.018
![]() |
[29] | T. Kuusi, G. Palatucci, Recent developments in nonlocal theory, Warsaw: De Gruyter Open Poland, 2017. https://doi.org/10.1515/9783110571561 |
[30] |
N. Liao, L. Schätzler, On the Hölder regularity of signed solutions to a doubly nonlinear equation. Part III, International Mathematics Research Notices, 2022 (2022), 2376–2400. https://doi.org/10.1093/imrn/rnab339 doi: 10.1093/imrn/rnab339
![]() |
[31] |
J. M. Mazón, J. D. Rossi, J. Toledo, Fractional p-Laplacian evolution equations, J. Math. Pures Appl., 105 (2016), 810–844. https://doi.org/10.1016/j.matpur.2016.02.004 doi: 10.1016/j.matpur.2016.02.004
![]() |
[32] |
G. Mingione, V. Rǎdulescu, Recent developments in problems with nonstandard growth and nonuniform ellipticity, J. Math. Anal. Appl., 501 (2021), 125197. https://doi.org/10.1016/j.jmaa.2021.125197 doi: 10.1016/j.jmaa.2021.125197
![]() |
[33] |
M. Misawa, K. Nakamura, Intrinsic scaling method for doubly nonlinear equations and its application, Adv. Calc. Var., 16 (2023), 259–297. https://doi.org/10.1515/acv-2020-0109 doi: 10.1515/acv-2020-0109
![]() |
[34] |
M. Misawa, K. Nakamura, Existence of a sign-changing weak solution to doubly nonlinear parabolic equations, J. Geom. Anal., 33 (2023), 33. https://doi.org/10.1007/s12220-022-01087-8 doi: 10.1007/s12220-022-01087-8
![]() |
[35] |
K. Nakamura, Local boundedness of a mixed local-nonlocal doubly nonlinear equation, J. Evol. Equ., 22 (2022), 75. https://doi.org/10.1007/s00028-022-00832-4 doi: 10.1007/s00028-022-00832-4
![]() |
[36] |
K. Nakamura, Harnack's estimate for a mixed local-nonlocal doubly nonlinear parabolic equation, Calc. Var., 62 (2023), 40. https://doi.org/10.1007/s00526-022-02378-2 doi: 10.1007/s00526-022-02378-2
![]() |
[37] |
E. Di Nezza, G. Palatucci, E. Valdinoci, Hitchhiker's guide to the fractional Sobolev space, Bull. Sci. Math., 136 (2012), 521–573. https://doi.org/10.1016/j.bulsci.2011.12.004 doi: 10.1016/j.bulsci.2011.12.004
![]() |
[38] |
D. Puhst, On the evolutionary fractional p-Laplacian, Appl. Math. Res. eXpress, 2015 (2015), 253–273. https://doi.org/10.1093/amrx/abv003 doi: 10.1093/amrx/abv003
![]() |
[39] |
S. Sturm, Existence of weak solutions of doubly nonlinear parabolic equations, J. Math. Anal. Appl., 455 (2017), 842–863. https://doi.org/10.1016/j.jmaa.2017.06.024 doi: 10.1016/j.jmaa.2017.06.024
![]() |
[40] |
M. Strömqvist, Local boundedness of solutions to non-local parabolic equations modeled on the fractional p-Laplacian, J. Differ. Equations, 266 (2019), 7948–7979. https://doi.org/10.1016/j.jde.2018.12.021 doi: 10.1016/j.jde.2018.12.021
![]() |
[41] |
M. Strömqvist, Harnack's inequality for parabolic nonlocal equations, Ann. Inst. Henri Poincare, 36 (2019), 1709–1745. https://doi.org/10.1016/j.anihpc.2019.03.003 doi: 10.1016/j.anihpc.2019.03.003
![]() |
[42] |
J. L. Vázquez, The Dirichlet problem for the fractional p-Laplacian evolution equation, J. Differ. Equations, 260 (2016), 6038–6056. https://doi.org/10.1016/j.jde.2015.12.033 doi: 10.1016/j.jde.2015.12.033
![]() |
1. | Amr Farouk, Adel Gabr Abdel-Razek, Karolina Gromadzka, Ahmed Noah Badr, Prevention of Aflatoxin Occurrence Using Nuts-Edible Coating of Ginger Oil Nanoemulsions and Investigate the Molecular Docking Strategy, 2022, 11, 2223-7747, 2228, 10.3390/plants11172228 | |
2. | Banafsheh Bagheri Marzooni, Gholamreza Jahed Khaniki, Nabi Shariatifar, Ebrahim Molaee-Aghaee, Assessment of aflatoxins in some pistachio cultivars supplied in bulk in Tehran food stores, 2022, 0306-7319, 1, 10.1080/03067319.2022.2098479 | |
3. | Hassan Rasouli, Fatemeh Dehghan Nayeri, Reza Khodarahmi, May phytophenolics alleviate aflatoxins-induced health challenges? A holistic insight on current landscape and future prospects, 2022, 9, 2296-861X, 10.3389/fnut.2022.981984 | |
4. | Paola Sánchez-Bravo, Luis Noguera-Artiaga, Vicente M. Gómez-López, Ángel A. Carbonell-Barrachina, José A. Gabaldón, Antonio J. Pérez-López, Impact of Non-Thermal Technologies on the Quality of Nuts: A Review, 2022, 11, 2304-8158, 3891, 10.3390/foods11233891 | |
5. | Tareq M. Osaili, Wael A.M. Bani Odeh, Manar Al Ayoubi, Ahmed A.S.A. Al Ali, Maryam S. Al Sallagi, Reyad S. Obaid, Vaidehi Garimella, Fatema Saeed Bin Bakhit, Richard Holley, Nada El Darra, Occurrence of aflatoxins in nuts and peanut butter imported to UAE, 2023, 24058440, e14530, 10.1016/j.heliyon.2023.e14530 | |
6. | María José González Muñoz, Houda Berrada Ramdani, Sonia Marín Sillué, Francisco José, Morales Navas, Silvia Pichardo Sánchez, Ana María Rivas Velasco, Report of the Scientific Committee of the Spanish Agency for Food Safety and Nutrition (AESAN) on the prospection of chemical hazards of interest in food safety in Spain (2), 2023, 1, 29401399, 10.2903/sp.efsa.2023.FR-0004 | |
7. | Cüneyt Çakir, Emre Turan, Atilla Şimşek, The effects of bentonite and activated charcoal treatments on aflatoxin content (AFB1, AFB2, AFG1, and AFG2) and physicochemical characteristics of hazelnut milk, 2023, 17, 2193-4126, 5256, 10.1007/s11694-023-02024-1 | |
8. | Parnian Samimi, Ramin Aslani, Ebrahim Molaee-Aghaee, Parisa Sadighara, Nabi Shariatifar, Gholamreza Jahed Khaniki, Sibel Ozcakmak, Zahra Reshadat, Determination and risk assessment of aflatoxin B1 in the kernel of imported raw hazelnuts from Eastern Azerbaijan Province of Iran, 2024, 14, 2045-2322, 10.1038/s41598-024-57422-2 | |
9. | Tahira Sultana, Khafsa Malik, Naveed Iqbal Raja, Zia-Ur-Rehman Mashwani, Asma Hameed, Riaz Ullah, Ali S. Alqahtani, , Aflatoxins in Peanut (Arachis hypogaea): Prevalence, Global Health Concern, and Management from an Innovative Nanotechnology Approach: A Mechanistic Repertoire and Future Direction, 2024, 9, 2470-1343, 25555, 10.1021/acsomega.4c01316 |
Country | CDI Percentiles |
|||
5% | 50% | 75% | 95% | |
Argentina | 4.39E-2 | 6.42E-2 | 7.57E-2 | 9.65E-2 |
Bahrain | 2.01E-3 | 3.06E-3 | 3.58E-3 | 4.56E-3 |
Bangladesh | 4.99E-3 | 7.36E-3 | 8.74E-3 | 1.09E-2 |
Brazil | 1.99E-3 | 2.96E-3 | 3.51E-3 | 4.44E-3 |
USA | 5.80E-4 | 8.80E-4 | 1.05E-3 | 1.34E-3 |
Cameroon | 3.80E-3 | 5.65E-3 | 6.69E-3 | 8.77E-3 |
China | 5.93E-4 | 8.76E-4 | 1.03E-3 | 1.29E-3 |
Congo | 1.33E-2 | 1.98E-2 | 2.36E-2 | 2.99E-2 |
Cyprus | 5.45E-4 | 8.09E-4 | 9.44E-4 | 1.22E-3 |
south Africa | 8.66E-4 | 1.30E-3 | 1.54E-3 | 1.92E-3 |
Egypt | 7.65E-4 | 1.14E-3 | 1.35E-3 | 1.71E-3 |
Ghana | 1.46E-3 | 2.20E-3 | 2.57E-3 | 3.28E-3 |
India | 7.62E-3 | 1.13E-2 | 1.30E-2 | 1.67E-2 |
Iran | 2.52E-3 | 3.83E-3 | 4.55E-3 | 5.71E-3 |
Country | MOE Percentiles |
|||
5% | 50% | 75% | 95% | |
Argentina | 9.11 | 13.71 | 16.07 | 21.24 |
Bahrain | 189.61 | 286.41 | 343.96 | 438.91 |
Bangladesh | 75.14 | 116.99 | 136.67 | 175.36 |
Brazil | 193.02 | 296.80 | 351.51 | 447.83 |
USA | 628.94 | 975.49 | 1152.10 | 1505.80 |
Cameroon | 102.16 | 155.70 | 186.68 | 238.66 |
China | 637.79 | 993.10 | 1200.60 | 1526.20 |
Congo | 28.53 | 43.35 | 52.36 | 67.39 |
Cyprus | 664.64 | 1071.10 | 1263.50 | 1588.70 |
South Africa | 433.60 | 654.31 | 785.12 | 1017.20 |
Egypt | 506.92 | 767.02 | 927.33 | 1176.20 |
Ghana | 252.82 | 392.07 | 462.82 | 606.49 |
India | 47.86 | 75.71 | 91.40 | 117.01 |
Iran | 144.63 | 224.35 | 268.18 | 357.23 |
Nuts | Total sample number | AFT mean µg/kg | AFT range µg/kg | AFT median µg/kg | MRL µg/kg | %Studies with AFT mean < ML µg/kg | %Studies with AFT mean MRL-50 µg/kg | %Studies with AFT mean 51–200 µg/kg | %Studies with AFT mean > 200 µg/kg |
Peanuts | 4737 | 40.87 | Nd-530 | 13.25 | 15 | 61.60 | 17.85 | 16.96 | 3.57 |
Pistachios | 11552 | 37.52 | Nd-245.60 | 7.20 | 10 | 71.42 | 11.90 | 9.52 | 7.14 |
Almonds | 517 | 3.54 | Nd-32.90 | 3.10 | 10 | 90.62 | 9.37 | 0.00 | 0.00 |
Hazelnuts | 293 | 17.33 | 0.2–124 | 2.10 | 10 | 76.92 | 7.69 | 15.38 | 0.00 |
Brazil nuts | 173 | 4.61 | 0.88–36.90 | 3.00 | 10 | 92.30 | 7.69 | 0.00 | 0.00 |
AFT: Aflatoxin total (sum of aflatoxin B1, B2, G1 and G2). MRL: Maximum Residue Limits (MRLs) for aAflatoxin adopted by Codex Alimentarius. Nd: Not detected. |
Country | CDI Percentiles |
|||
5% | 50% | 75% | 95% | |
Argentina | 4.39E-2 | 6.42E-2 | 7.57E-2 | 9.65E-2 |
Bahrain | 2.01E-3 | 3.06E-3 | 3.58E-3 | 4.56E-3 |
Bangladesh | 4.99E-3 | 7.36E-3 | 8.74E-3 | 1.09E-2 |
Brazil | 1.99E-3 | 2.96E-3 | 3.51E-3 | 4.44E-3 |
USA | 5.80E-4 | 8.80E-4 | 1.05E-3 | 1.34E-3 |
Cameroon | 3.80E-3 | 5.65E-3 | 6.69E-3 | 8.77E-3 |
China | 5.93E-4 | 8.76E-4 | 1.03E-3 | 1.29E-3 |
Congo | 1.33E-2 | 1.98E-2 | 2.36E-2 | 2.99E-2 |
Cyprus | 5.45E-4 | 8.09E-4 | 9.44E-4 | 1.22E-3 |
south Africa | 8.66E-4 | 1.30E-3 | 1.54E-3 | 1.92E-3 |
Egypt | 7.65E-4 | 1.14E-3 | 1.35E-3 | 1.71E-3 |
Ghana | 1.46E-3 | 2.20E-3 | 2.57E-3 | 3.28E-3 |
India | 7.62E-3 | 1.13E-2 | 1.30E-2 | 1.67E-2 |
Iran | 2.52E-3 | 3.83E-3 | 4.55E-3 | 5.71E-3 |
Country | MOE Percentiles |
|||
5% | 50% | 75% | 95% | |
Argentina | 9.11 | 13.71 | 16.07 | 21.24 |
Bahrain | 189.61 | 286.41 | 343.96 | 438.91 |
Bangladesh | 75.14 | 116.99 | 136.67 | 175.36 |
Brazil | 193.02 | 296.80 | 351.51 | 447.83 |
USA | 628.94 | 975.49 | 1152.10 | 1505.80 |
Cameroon | 102.16 | 155.70 | 186.68 | 238.66 |
China | 637.79 | 993.10 | 1200.60 | 1526.20 |
Congo | 28.53 | 43.35 | 52.36 | 67.39 |
Cyprus | 664.64 | 1071.10 | 1263.50 | 1588.70 |
South Africa | 433.60 | 654.31 | 785.12 | 1017.20 |
Egypt | 506.92 | 767.02 | 927.33 | 1176.20 |
Ghana | 252.82 | 392.07 | 462.82 | 606.49 |
India | 47.86 | 75.71 | 91.40 | 117.01 |
Iran | 144.63 | 224.35 | 268.18 | 357.23 |
Nuts | Total sample number | AFT mean µg/kg | AFT range µg/kg | AFT median µg/kg | MRL µg/kg | %Studies with AFT mean < ML µg/kg | %Studies with AFT mean MRL-50 µg/kg | %Studies with AFT mean 51–200 µg/kg | %Studies with AFT mean > 200 µg/kg |
Peanuts | 4737 | 40.87 | Nd-530 | 13.25 | 15 | 61.60 | 17.85 | 16.96 | 3.57 |
Pistachios | 11552 | 37.52 | Nd-245.60 | 7.20 | 10 | 71.42 | 11.90 | 9.52 | 7.14 |
Almonds | 517 | 3.54 | Nd-32.90 | 3.10 | 10 | 90.62 | 9.37 | 0.00 | 0.00 |
Hazelnuts | 293 | 17.33 | 0.2–124 | 2.10 | 10 | 76.92 | 7.69 | 15.38 | 0.00 |
Brazil nuts | 173 | 4.61 | 0.88–36.90 | 3.00 | 10 | 92.30 | 7.69 | 0.00 | 0.00 |
AFT: Aflatoxin total (sum of aflatoxin B1, B2, G1 and G2). MRL: Maximum Residue Limits (MRLs) for aAflatoxin adopted by Codex Alimentarius. Nd: Not detected. |