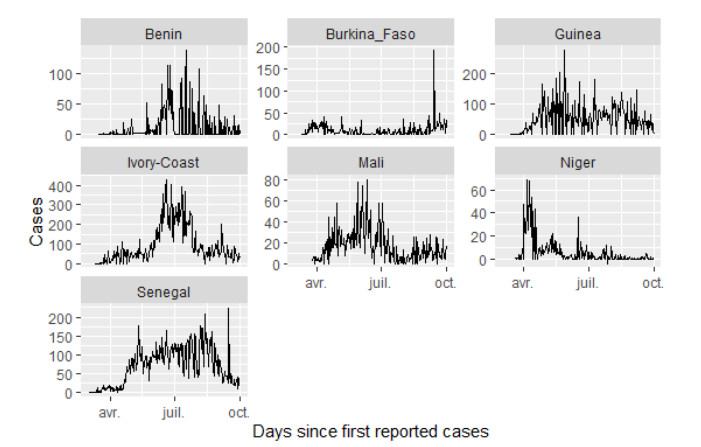
Bayesian flexible multilevel nonlinear models (FMNLMs) are powerful tools to analyze infectious disease data with asymmetric and unbalanced structures, such as varying epidemic stages across countries. However, the robustness of these models can be undermined by poorly designed estimation methods, particularly due to uncertainties in prior distributions and initial values. This study investigates how varying levels of prior informativeness can influence the model convergence, parameter estimation, and computation time in a Bayesian flexible multilevel nonlinear model (FMNLM). A simulation study was conducted to evaluate the impact of modifying prior assumptions on posterior estimates and their subsequent effects on the interpretations. The framework was applied to COVID-19 data from Francophone West Africa. The results indicate that accurate, informative priors enhance the prediction performance with minimal impact on the computation time. Conversely, non-informative or inaccurate priors for nonlinear parameters led to lower convergence rates and a reduced recovery accuracy, although they may remain viable in standard multilevel nonlinear models.
Citation: Olaiya Mathilde Adéoti, Aliou Diop, Romain Glèlè Kakaï. Bayesian inference and impact of parameter prior specification in flexible multilevel nonlinear models in the context of infectious disease modeling[J]. Mathematical Biosciences and Engineering, 2025, 22(4): 897-919. doi: 10.3934/mbe.2025032
[1] | Sara Y. Del Valle, J. M. Hyman, Nakul Chitnis . Mathematical models of contact patterns between age groups for predicting the spread of infectious diseases. Mathematical Biosciences and Engineering, 2013, 10(5&6): 1475-1497. doi: 10.3934/mbe.2013.10.1475 |
[2] | David J. Gerberry . An exact approach to calibrating infectious disease models to surveillance data: The case of HIV and HSV-2. Mathematical Biosciences and Engineering, 2018, 15(1): 153-179. doi: 10.3934/mbe.2018007 |
[3] | Yendry N. Arguedas, Mario Santana-Cibrian, Jorge X. Velasco-Hernández . Transmission dynamics of acute respiratory diseases in a population structured by age. Mathematical Biosciences and Engineering, 2019, 16(6): 7477-7493. doi: 10.3934/mbe.2019375 |
[4] | M. C. J. Bootsma, K. M. D. Chan, O. Diekmann, H. Inaba . Separable mixing: The general formulation and a particular example focusing on mask efficiency. Mathematical Biosciences and Engineering, 2023, 20(10): 17661-17671. doi: 10.3934/mbe.2023785 |
[5] | Cunjuan Dong, Changcheng Xiang, Wenjin Qin, Yi Yang . Global dynamics for a Filippov system with media effects. Mathematical Biosciences and Engineering, 2022, 19(3): 2835-2852. doi: 10.3934/mbe.2022130 |
[6] | Jinlong Lv, Songbai Guo, Jing-An Cui, Jianjun Paul Tian . Asymptomatic transmission shifts epidemic dynamics. Mathematical Biosciences and Engineering, 2021, 18(1): 92-111. doi: 10.3934/mbe.2021005 |
[7] | Mahmudul Bari Hridoy . An exploration of modeling approaches for capturing seasonal transmission in stochastic epidemic models. Mathematical Biosciences and Engineering, 2025, 22(2): 324-354. doi: 10.3934/mbe.2025013 |
[8] | Rajanish Kumar Rai, Arvind Kumar Misra, Yasuhiro Takeuchi . Modeling the impact of sanitation and awareness on the spread of infectious diseases. Mathematical Biosciences and Engineering, 2019, 16(2): 667-700. doi: 10.3934/mbe.2019032 |
[9] | Qiuyi Su, Jianhong Wu . Impact of variability of reproductive ageing and rate on childhood infectious disease prevention and control: insights from stage-structured population models. Mathematical Biosciences and Engineering, 2020, 17(6): 7671-7691. doi: 10.3934/mbe.2020390 |
[10] | Samuel Bronstein, Stefan Engblom, Robin Marin . Bayesian inference in epidemics: linear noise analysis. Mathematical Biosciences and Engineering, 2023, 20(2): 4128-4152. doi: 10.3934/mbe.2023193 |
Bayesian flexible multilevel nonlinear models (FMNLMs) are powerful tools to analyze infectious disease data with asymmetric and unbalanced structures, such as varying epidemic stages across countries. However, the robustness of these models can be undermined by poorly designed estimation methods, particularly due to uncertainties in prior distributions and initial values. This study investigates how varying levels of prior informativeness can influence the model convergence, parameter estimation, and computation time in a Bayesian flexible multilevel nonlinear model (FMNLM). A simulation study was conducted to evaluate the impact of modifying prior assumptions on posterior estimates and their subsequent effects on the interpretations. The framework was applied to COVID-19 data from Francophone West Africa. The results indicate that accurate, informative priors enhance the prediction performance with minimal impact on the computation time. Conversely, non-informative or inaccurate priors for nonlinear parameters led to lower convergence rates and a reduced recovery accuracy, although they may remain viable in standard multilevel nonlinear models.
Multilevel nonlinear models, also known as nonlinear mixed-effects models (NLMMs), offer a sophisticated approach to data analysis by accounting for both within- and between-subject variability. These models are particularly well-suited to leverage the richness of repeated measurements. Over the past few decades, there has been growing interest in applying NLMMs to infectious disease modeling to gain a more comprehensive understanding of disease dynamics and to improve control strategies. This interest is particularly driven by the need to account for substantial heterogeneity across countries when conducting in-depth analyses of micro-infection dynamics.
Estimation methods may lack robustness if not carefully designed. To enhance the application of these models in infectious disease analyses, Bayesian methods have been proposed as a valuable framework to incorporate a priori information more rigorously. This approach flexibly addresses sparse and unbalanced longitudinal data from individual subjects [1]. However, a common concern with Bayesian methods is their sensitivity to various aspects of the modeling process, including prior distributions and initial values [2]. A sensitivity analysis serves as a fundamental tool to investigate the model uncertainty. Prior distributions play a critical role in determining the extent to which subjective opinions influence the model estimation. As [3] noted, the effect of a prior distribution depends on factors such as its degree of informativeness and the sample size. This study builds on recent developments in Bayesian flexible multilevel nonlinear models (FMNLMs) for infectious disease analyses [3,4]. Specifically, it examines how prior informativeness and accuracy, along with the sample or cluster size, affect the parameter estimates in FMNLMs. The focus is on understanding whether strong but inaccurate subjective beliefs about epidemic parameters can significantly impact estimation outcomes.
The literature on the specific impact of different prior distributions in infectious disease modeling remains limited. This investigation aims to provide insights into the consequences of using informative prior distributions in this specific context. We focus on two flexible models, namely the scale mixtures of skew-normal nonlinear mixed model (SMSN-NLMM) and the semi-nonparametric nonlinear mixed model (SNP-NLMM), to examine how varying levels of prior informativeness can influence the model convergence, parameter estimation, and computation time through a simulation study. Starting from completely diffuse prior distributions, we introduce reasonable modifications to the prior assumptions, recompute the posterior quantities of interest, and assess whether these changes significantly affect the interpretations or conclusions.
In this framework, we assume that the random terms (random effects and residuals) follow either a scale mixtures of skew-normal (SMSN) distribution [5,6,7] or a semi-nonparametric (SNP) distribution [8,9]. Using specific epidemic data characteristics, such as sample size, cluster size, or reporting frequency, we evaluate the empirical performance of these models in recovering the epidemic parameters under varying levels of prior informativeness.
This paper is structured as follows: in Section 2, we introduce a case study of infectious disease dynamic models and describe the real dataset used in this analysis; Section 3 provides a concise summary of the multivariate flexible multilevel nonlinear models (SMSN-NLMM and SNP-NLMM) and their associated Bayesian estimation procedures; Section 4 presents a simulation study to evaluate the methodology; in Section 5, we apply the proposed methodology to the COVID-19 dataset described in Section 2, thus showcasing its utility and presenting the results; and finally, Section 6 presents a discussion of the findings and their implications before a short summary of the key findings in the conclusion.
Infectious disease outbreak data, such as reported cases and deaths, are collected within countries or regions. Countries that experience outbreaks earlier tend to accumulate more data compared to those in the same region that experience outbreaks later. To prepare for potential outbreaks, countries that have not yet reached the peak often leverage existing data from neighboring countries to model the average patterns and predict the trajectory of the epidemic. Additionally, the level of surveillance and preparedness against outbreaks varies across countries, thus leading to a diverse range of responses that can influence the epidemiological parameters. Sub-notification is a significant challenge in accurately understanding the true number of infections in the population due to the varying testing capacities between countries.
Figure 1 presents example cases, showing the reported number of daily COVID-19 cases across different countries. It displays daily reported cases from the first identified cases until October 1, 2020, in seven West African countries (Benin, Burkina Faso, Ivory Coast, Guinea, Mali, Niger, and Senegal). These countries were selected for comparison and discussion, as previously chosen by [10] to examine the epidemic dynamics in the region. The authors emphasized that these countries demonstrate the varying dynamics of the pandemic, despite implementing different government measures within the same geographical area.
The datasets can be accessed via the link https://github.com/CSSEGISandData/COVID-19/ provided by Johns Hopkins University in collaboration with the Center for Systems Science and Engineering (see [11]).
It is worth noting that, in contrast to countries in regions such as America or Europe, where cases have been reported daily since the first identified case, the Francophone West African countries presented here experienced several consecutive days with zero detected cases, despite being at different stages of the pandemic curve. The different stages of disease spread are crucial information that should be incorporated into the model. Moreover, infectious disease data often exhibit skewness, outliers, or heavy-tailed behavior [2,12,13]. For instance, Figure 2a shows the density curve of residuals from reported cases, thus revealing significant skewness in the reported responses. It is important to note that these reported responses have been subjected to linear transformations, as proposed in [13], yij=zij/kz, where zij is the number of reported cases at the ith country and the jth day since the first reported case, and kz is chosen to be the sample standard deviation from the country data, which is the smaller one in the observed data. Non-normality is also confirmed by the Q-Q plot of the fitted residuals in Figure 2b. To characterize skewness with heavy tails, which often appear in such epidemic data, we will develop a Bayesian flexible parametric (SMSN-NLMM) or semi-nonparametric (SNP-NLMM) multilevel nonlinear models in conjunction with Model 3.1 under the assumption of SMSN and SNP distributions. That is, we assume that both εi and bi either follow a multivariate SMSN distribution as proposed by [5,6,13] and [7] or a SNP as introduced by [8] and [9]. These methodologies jointly accommodate the different stages of the diseases while borrowing information from the different time series and considering the epidemic data structure to provide a more robust and reliable fit and prediction.
NLMMs can be applied across various fields beyond infectious disease modeling. In this section, we focus on their application to infectious disease data. Specifically, we treat subjects as either countries or regions within a country.
Let us consider n independent countries (or regions of a country), each with mi measurements from the ith country (or region of a country). Let yij denote the observed response and xij represent the vector of within-subject covariates for the jth measurements (j=1,...,mi) from the ith country (i=1,...,n). The response vector for the ith country is denoted as yi=(yi1,...,yimi)⊤. Next, let us define a scalar-valued differentiable nonlinear function μ(xij|ϕi) that describes the average trajectory of the response. The general nonlinear mixed-effects model is expressed as follows [14] :
yi=μ(ϕi,xi)+εi, | (3.1) |
where εi is a ni−dimensional vector of independent within-country errors. The mixed effects parameter vector ϕi is modeled as follows [14] :
ϕi=Aiβ+Bibi. | (3.2) |
In Eq (3.2), Ai is a design matrix of size (r, p) for the fixed effects that possibly depends on elements of xi, and Bi is a design matrix of size (r, q) for the random effects that allow the incorporation, for instance, of "time-varying" covariates in the random effects with r, which represents the number of subject-specific parameters included in the nonlinear function. β is a p-dimensional locator vector of fixed-effects. bi is a q-dimensional vector of random-effects (assumed to be mutually independent across subjects and independent of the within-country errors εi) associated with the ith country.
The random-effects vector bi has the density function gb(.|θ) and the conditional distribution of yi given bi, and the covariate vector xij has the density function fY(.|bi,θ). The estimation of the parameter vector θ (which contains β and distribution parameters) of NLMMs is commonly achieved through maximization of the marginal likelihood L(.|y) of the observed data Y=(y⊤1,...,y⊤n)⊤ as follows:
L(θ|Y)=n∏i=1∫fy(yi|bi,θ)gb(bi|θ)dbi. | (3.3) |
Both gb(.|θ) and fY(.|bi,θ) are often assumed to be multivariate normal densities; however, the observed data may not support these assumptions.
The parametric flexible multilevel nonlinear model is the nonlinear mixed effects model in which the random terms (random effects and errors) follow a scale mixture of skew-normal (SMSN) distributions. It's expressed as follows: yi=μi(ϕi)+ϵi; ϕi=Aiβ+Bibi with
ϵij∼SMSN1(−η,ω2,λ,ν);bi∼SMSNq(−ηb,Ωb,λb,νb), | (3.4) |
where η and ηb are location shifts used to zero means of residual errors and random effects, respectively, ω2 and Ωb are the scale parameters that know the degrees of freedom (ν and νb) and the shape parameters (λ and λb) to effectively capture skewness and heavy-tailed behavior. Meanwhile, under a mixed effects design with n subjects and mi measurements for the ith subject, the SMSN-NLMM is given for the jth outcome Yij (i=1,⋯,n; j=1,⋯,mi) as follows:
(Yij∣xij,ϕi)ind∼SMSN1(ξij,ω2,λ,ν),biind∼SMSNq(ξb,Ωb,λb,νb), | (3.5) |
with E(Yij∣xij,ϕi)=μ(xij∣ϕi)=μij; Var(Yij∣ϕi)=σ2; E(bi)=0; Var(bi)=Σb. Let κ be the mixture variable associated to response yij with the cumulative distribution function Hκ(ν) and the density function hκ(ν) and U, the mixture variable associated to random effect vector bi with cumulative distribution function Hu(νb), and the density function hu(νb), whereas κet=E{κ−t/2}<∞ (t=1,2) and Ut=E{U−t/2}<∞ (t=1,2). Then, the expectation of the response, and the residual variance, and the variance-covariance matrix of random effects are expressed as follows [7]: ξij=μij−cκe1δe; ω2=κ−1e2(σ2+c2κ2e1δ2e); ξb=−cU1δb and Ωb=U−12(Σb+c2U21δbδb⊤) with c=√2/π.
A key feature of SMSN-NLMM is that it can be formulated within a flexible hierarchical representation that is useful to easily write implementable STAN or BUGS codes and for analytical derivations. While referring to [15] and [16], it follows from (3.4) that
(Yij∣ϕi,κij,tij)ind∼N1(μij+(tijκ−1/2ij−cκe1)δe,κ−1ijˉω2), | (3.6a) |
κijind∼Hκ(ν) and tijind∼HN(0,1), | (3.6b) |
(bi∣Ui,si)ind∼Nq((siu−1/2i−cU1)δb,u−1iˉΩb), | (3.6c) |
Uiind∼Hu(νb) and siind∼HN(0,1), | (3.6d) |
where δe=ωλ(1+λ2)−1/2, δb=(1+λ⊤bλb)−1/2Ω1/2bλb, ˉω2=ω2−δ2e, ˉΩb=Ωb−δbδ⊤b. t and s are positive standard half normal random variables (absolute value of a standard normal random variables) denoted HN(0,1).
For instance, candidate shape parameters are δe and δb. Meanwhile, the parameters to be estimated are the fixed effects β⊤, the shape parameters δe and δb, the degrees of freedom ν and νb, and the variance components σ2 and Σb. The parameters ν and νb of the mixture variables control the shape of the SMSN distributions with δe and δb, especially the skewness and the excess of kurtosis. Taking Y as the complete data (observed data with a missing data), the complete likelihood function associated with Y under the hierarchical representation can be expressed using Bayes Theorem as follows:
L(θ|Y)=n∏i=1{{ni∏j=1f(yij|bi,κij,tij,ui,si)}fb(bi|ui,si)ni∏j=1(fκi(κij)fti(tij))fUi(ui)fsi(si)} | (3.7) |
where the notation fx(x) refers to the density function of x. θ=(β⊤,σ2,ϱ⊤,ρ⊤,δe,δb,ν,νb)⊤ takes ϱ⊤, ρ⊤ as a random effects dispersion parameters matrix (Σb is supposed to be unstructured) diagonal elements and off diagonal elements, respectively. κi=(κi1,...,κimi)⊤ and Ti=(ti1,...,timi)⊤.
SNP-NLMM is the nonlinear mixed effects model in which the random terms (random effects and errors) follow SNP distributions. The SNP-NLMM is expressed as follows: yi=μi(ϕi)+ϵi; ϕi=Aiβ+Bibi with
ϵij∼SNP(−η,R2,ψ,K);bi∼SNPq(−ηb,RbR⊤b,ψb,K), | (3.8) |
where the subscript i represents the subject index, η and ηb are the location shifts used to zero means of error and random effects, respectively, a shape vector ψ∈[−π/2,π/2], and a scale matrix Σ>0 (positive definite) with Cholesky factor R (RRT=Σ) and integer valued expansion order K. Here, we assume that the residual and the random effects are mutually independent across i, and write the following:
yij=μij+R(Zij−η), | (3.9) |
bi=Rb(Zbi−ηb), | (3.10) |
where R is the residual dispersion parameter, Rb is a (q×q) upper triangular scale matrix for random effects, and Zij∼SSNP(ψ,K) and Zbi∼SSNPq(ψb,K) are standard SNP variables.
SSNPq(ψ,K) defined in the sequel denotes a standard SNP variable with a null location vector and the identity scale (SNPq(0;Iq;λ;K)). A standard SSNPq(ψ,K) variable z has a density of the following form:
h(z⏐ψ,K)=P2K(z|ψ)ϕq(z), | (3.11) |
where PK(.|ψ) is a multivariate polynomial of order K. Let α be a multi-index, i.e., a q×1 vector with nonnegative integer elements: α=(α1,...,αq)⊤. Let |α|=∑qk=1αk and zα=∏qk=1zαkk. Then, the K order multivariate polynomial can be written as follows:
PK(z|λ)=K∑|α|=0aαzα. | (3.12) |
Therefore, the densities of response and random effects can be expressed as follows:
fY(yij|bi,ψ,R)=P2K(zij;ψ)ϕ1(yij|η,R2), | (3.13) |
fb(bi|ψb,Rb)=P2K(Zbi;ψb)ϕq(bi|ηb,RbR⊤b), | (3.14) |
where zij=R−1(yij−μij)+η, and zbi=R−1bbi+ηb; ϕq(.|γb,Σb) is q-variate normal density with mean γb and covariance matrix RbR⊤b knowing Σb=RbR⊤b. Thus, following [17], E(Yij)=μij+η−σE(Zij); var(Yij)=σ2var(Zij); E(bi)=ηb+Σ1/2bE(Zi) and var(bi)=Σbvar(Zi), where E(Zij)=E(UP2K(U;ψ)) and var(Zij)=E(U2P2K(U;ψ))−E2(Zij) and U∼N(0,1). The marginal likelihood for θ=(β⊤,R,Rb,ψ,ψb)⊤ for a fixed K is as folows:
L(θ|Y)=n∏i=1{{ni∏j=1fY(yij|bi,ψ,R)}fb(bi|ψb,Rb)}, | (3.15) |
where b=(b⊤1,…,b⊤m)⊤, y=(y1⊤,...,y⊤m)⊤, and yi = (yi1,...,yimi)⊤, i=1,...,n. Moreover, the SNP-NLMM exploits the SNP approach to represent the density, where the degree of flexibility is controlled by the parameter K, which is chosen via inspection of the standard information criteria. Thus, the distribution density is parameterized in terms of a finite set of parameters θ, where the dimension of θ (through ψ and ψb) depends on K. When K=0, the semi-nonparametric density reduces to a standard q-variate normal density, (3.13 and 3.14) are Nni(η,R2) and Nq(ηb,RbR⊤b) with η=μij and R2=σ2, so that the usual normal specification for NLMMs is a special case [9]. The approach is widely recommended for routine use in situations where a departure from the usual normal assumption for the random terms is either present or suspected. Often, K = 1 or 2 is sufficient to represent an adequate departure from normality [17]. Most studies considered the performance for varying the expansion order parameter K and confirmed this underlined proposition [8,9,18,19]. A larger K gives more flexibility to represent the random terms distribution; however, choosing a K that is too large will result in an inefficient representation [17]. For facilitation and comparison purposes in this study, we just considered the SNP-NLMM with K = 1.
To complete the Bayesian specification, we need to consider prior distributions for all the unknown model parameters, (β⊤,σ2,τ⊤,ρ⊤,δe,δ⊤b,ν,νb,ψ,ψb)⊤.
In this study, a Bayesian approach is adopted, thereby considering different levels of informative priors by fixing the mean hyperparameter for the prior distribution to represent relatively close, moderate, or distant values from the corresponding population value of the model parameters. These variations are specifically applied to the model's mixed effects parameters, particularly the fixed effects, while all other parameters (such as precision, scale, and structural model parameters) are completely assigned to diffuse priors, as described by [20]. Therefore, the primary factors manipulated in this study are the mean hyperparameter and the variance hyperparameter for each nonlinear parameter. A detailed description of these factors is provided below.
In Bayesian modeling, the elements of the parameter vector θ are assumed to be independent. Consequently, the joint prior distribution (π(θ)) for all unknown parameter densities can be expressed for the SMSN-NLMM and SNP-NLMM models, respectively, as follows:
π(θ)=π(β)π(σ2)π(Σ)π(δ0)π(δ0b)π(ν)π(νb), | (3.16) |
π(θ)=π(β)π(σ2)π(Σ)π(ψ)π(ψb). | (3.17) |
By knowing the models for the observed data and the prior distributions for the unknown model parameters, a statistical inference for the parameters can be carried out using their posterior distributions under the Bayesian framework. Given the observed data, the joint posterior density of the parameter vector θ is expressed as follows:
π(θ,bi|y)=L(θ|y)π(θ). | (3.18) |
In general, expression (3.18) does not have a closed form, and MCMC procedures can be used to sample based on (3.18) using the Hamiltonian Monte Carlo (HMC) algorithm. This latest is implemented in the Stan program along with the Bayesian Regression Models (brms) procedure [21,22]. This approach is particularly advantageous, as it allows the developed models to be implemented using the rstan package in R, which is a freely available software [23]. In this study, the tools and techniques are situated within a Bayesian framework and rely on the HMC algorithm implemented through rstan. This approach offers a practical alternative to the computationally expensive traditional MCMC methods, thus making it a more efficient option for Bayesian inference [4].
Considering the potentially asymmetric and skewed nature of infectious disease data [16], a natural extension of the modeling approach is to adopt a nonlinear mixed-effects model where the random components (residuals and random effects) are assumed to follow a flexible distribution. One such distribution is the skew Student-t, a specific case of the SMSN-NLMM framework [12,13], which incorporates the degrees of freedom (ν) and the shape parameters (δ) to capture skewness and heavy-tailed behavior:
Yij|bi∼SMSN1(μij−η,ω2y,δ,ν), bi∼SMSNq(−ηb,Ωb,δb,νb) |
where μij=μij(ϕi) is a non-linear function with the individual parameter vector
ϕi=(ϕ1iϕ2iϕ3iϕ4i)=Xiβ+bi, |
η and ηb are location shifts used to zero means of residual errors and random effects, respectively. The intra-individual regression function considered is the derivative of the generalized logistic used to model COVID-19 death curves from American countries [13], as defined by the following:
μ(xij;ϕi)=ϕ1ϕ3iϕ4exp{−ϕ3ixij}(ϕ2i+exp{−ϕ3ixij})ϕ4+1 | (4.1) |
In this equation, ϕ2i=exp{β2+b2i}, ϕ3i=exp{β3+b3i}, and ϕk=exp{βk}, for k=1;4, with the exponential transformation being used to ensure positiveness of the parameters; xij=tij is the time of observation j (1≤j≤ni) on individual i (1≤i≤n). The nonlinear mixed parameters is particularly express as follows: ϕi=exp(Xiβ+bi).
Referring to [24], random effects are incorporated into (4.1) to facilitate a multivariate approach and enable information sharing across different time series. The parameter ϕ3 controls the infection rate, while ϕ4 acts as an asymmetry parameter. Additionally, ϕ1, ϕ2, and ϕ4 influence the curve's asymptote, with the total expected cases given by ϕ1ϕϕ42. The peak of the curve occurs at the time point t=−ln(ϕ2/ϕ4)ϕ3. The initial infection rate, ϕ3, of an epidemic is a critical measure of disease transmission, as it is often utilized to estimate the basic reproduction number R0. This key epidemiological parameter, which represents the average number of secondary infections caused by a single infected individual in a fully susceptible population, is calculated as R0=eϕ3T [25].
The parameters of the epidemic model are treated as random variables and assigned specific probability distributions. To generate data from the epidemic model, each parameter was initially simulated from its respective distribution (see population values in Table 1). The nonlinear parameters fixed effect population values are presented in the table with their standard errors in the parenthesis. Then, the resulting parameter values were used to simulate the dataset. These predefined distributions serve as benchmarks to evaluate the fitted posterior distributions derived from the simulated dataset.
Parameters | Population values | Variance Hyperparameter Levels | ||||
10.00% Variance | 20.00% Variance | 50.00% Variance | ||||
Mean Hyperparameter Level: 1SD | ||||||
β1 | 1.98 (10−1) | N(1.535,0.198) | N(1.351,0.396) | N(0.985,0.990) | ||
β2 | -1.07 (10−1) | N(−1.397,0.107) | N(−1.533,0.214) | N(−1.801,0.535) | ||
β3 | -2.89 (10−1) | N(−3.428,0.289) | N(−3.650,0.578) | N(−4.092,1.445) | ||
β4 | 1.87 (10−1) | N(1.438,0.187) | N(1.258,0.374) | N(0.903,0.935) | ||
σ | 3.8 | |||||
Ω1 | 0.721 | |||||
Ω2 | 0.589 | |||||
Ω12 | -0.097 | |||||
δ & δb | 0.1 | |||||
ν & νb | 1.98 | |||||
Mean Hyperparameter Level: 3SD | ||||||
β1 | 1.98 (10−1) | N(0.645,0.198) | N(0.092,0.396) | N(−1.005,0.990) | ||
β2 | -1.07 (10−1) | N(−2.051,0.107) | N(−2.458,0.214) | N(−3.264,0.535) | ||
β3 | -2.89 (10−1) | N(−4.503,0.289) | N(−5.171,0.578) | N(−6.496,1.445) | ||
β4 | 1.87 (10−1) | N(0.573,0.187) | N(0.035,0.374) | N(−1.031,0.935) | ||
σ | 3.8 | |||||
Ω1 | 0.721 | |||||
Ω2 | 0.589 | |||||
Ω12 | -0.097 | |||||
δ & δb | 0.1 | |||||
ν & νb | 1.98 | |||||
Mean Hyperparameter Level: 5SD | ||||||
β1 | 1.98 (10−1) | N(−0.245,0.198) | N(−1.166,0.396) | N(−2.995,0.990) | ||
β2 | -1.07 (10−1) | N(−2.706,0.107) | N(−3.383,0.214) | N(−4.727,0.535) | ||
β3 | -2.89 (10−1) | N(−5.578,0.289) | N(−6.691,0.578) | N(−8.900,1.445) | ||
β4 | 1.87 (10−1) | N(−0.292,0.187) | N(−1.188,0.374) | N(−2.965,0.935) | ||
σ | 3.8 | |||||
Ω1 | 0.721 | |||||
Ω2 | 0.589 | |||||
Ω12 | -0.097 | |||||
δ & δb | 0.1 | |||||
ν & νb | 1.98 |
We simulated M datasets under the assumption that specific infectious disease measurements were reported daily over two timeframes: a maximum of 1 month for 6 clusters (representing a small dataset) and a maximum of 1 month for 12 clusters (representing a large dataset), thereby taking clusters for countries or areas of the country. A limit of quantification for the reported cases was set at 0.0 individuals, meaning any simulated measurements below this threshold were adjusted to 0.0. The parameter values used in the simulations were from estimates obtained through a nonlinear least squares (NLS) estimation for fixed effects and the maximum likelihood estimation proposed by [13] applied to some Latin American countries COVID-19 data (Table 1).
The primary focus of this paper has been to examine the effects of the varying degrees of (in) accurate prior distributions placed on parameters within the context of FMNLM. Meanwhile, it involves manipulating the mean (and variance) hyperparameter for the nonlinear parameter priors. Specifically, informative priors were only placed only on the nonlinear parameters (β∼Np(β0,Σ0); β0, Σ0 are constituted of the defined hyperparameters) in this study (Table 1), while all other parameters were given completely diffuse priors (refering to [20], [12] and [19]).
Following the systematic evaluation framework of [3], the mean hyperparameter term was determined as a function of the fixed variance hyperparameter. Specifically, the mean hyperparameter was decreased by either 1 standard deviation (SD), 3SD, or 5SD based on the fixed variance hyperparameter term. However, the levels of variance hyperparameters were systematically set, taking either 10.00% (relatively low variance), 20.00% (relatively moderate variance), or 50.00% (relatively high variance) of the corresponding population value. Meanwhile, this setup resulted in nine distinct prior settings: 1SD10, 1SD20, 1SD50, 3SD10, 3SD20, 3SD50, 5SD10, 5SD20, and 5SD50, where αSDγ denotes an αSD mean hyperparameter and a γ% variance hyperparameter level. This method was deemed the most straightforward and practical to define the critical conditions of interest in this investigation, thus giving the infeasibility of exploring all possible combinations of mean and variance hyperparameter conditions. We refer to [3] for further details.
Table 1 provides a summary of the population model values and their corresponding priors across all mean and variance hyperparameter levels.
As an example, all mean hyperparameter levels for the first parameter are illustrated in Figure 3. This figure demonstrates the prior distributions relative to the population value for all nine hyperparameter levels considered in this study.
For the population value V, the corresponding variance hyperparameters are computed as follows: Var1=⏐V⏐∗10/100, Var2=⏐V⏐∗20/100, Var3=⏐V⏐∗50/100, with the corresponding SDs: SD1=√Var1 for 10%, SD2=√Var2 for 20%, and SD3=√Var3 for 50%. For example, the mean hyperparameters for 10% variance are as follows: V−(1∗SD1), V−(3∗SD1), and V−(5∗SD1) for 1SD, 3SD, and 5SD, respectively.
Under each of the simulation scenarios, we fitted the Bayesian flexible semi-nonparametric multilevel nonlinear models and the Bayesian flexible parametric multilevel nonlinear models in addition to the actual normal NLMM to analyse the characteristics of each fitting model.
The Hamiltonian Monte-Carlo (HMC) algorithm has been proposed to be suitable for such a modeling approach [4]. Meanwhile, we took advantage of brms within the STAN interface in the R software [23] for the analysis. The population parameters were initialized at the values obtained through the nlme package setting and the random effects were initialized to zero. The scale parameters were initialized to 1, while the correlation, shape, and skewness parameters were initialized to zero. We ran 3 chains of 400 iterations for each simulated dataset, where we kept 200 iterations per chain for the posterior sample and the first 200 iterations were considered to ensure convergence (i.e., warm-up = 200 iterations). We obtained a posterior sample of L = 600 replications for each simulated dataset, where the trace plots of some simulated datasets were examined to check the convergence of the HMC algorithm based on the rstan package. In its initial conception, the latest package considered neither the SMSN family nor the SNP distribution of a random terms specification. However, we refer to the standard N-NLMM stan code to write the specific flexible stan codes by adding the required parameters following their stochastic representations (3.6 and 3.13).
We assessed how different priors affect the model convergence rate, parameter estimation, and computation time. We relied on the effective sample size ˆnESS and its relative Gelman-Rubin statistics (classic split-Rhat) ˆR to assess the impact of the prior information on the speed of convergence. Then, we computed the mean of the effective sample sizes and ˆR over the M simulated datasets for each population parameter θ under each prior scenario. In each simulation condition, the larger the mean of ˆnESS quantities are, the better the posterior distribution is estimated, and an ˆR indicator close to 1 shows a good convergence of the chains.
For the assessment of the impact of the prior information on the model estimation, we obtained the parameter estimate empirical standard error (^SEk=[ˆθ(r)k−Eθk]), the average standard error (^ASEk), the mean squared error (^MSEk), and the recovery ratios (RRk) for each parameter based on the converged simulation replications (see Table 2). The recovery ratios (RR) represent ratios of the estimate/population, where values closer to 1.0 indicate a proper logistic parameter [3], while the recovery quantity CR(^HDI)k is the proportion of datasets for which the simulation mean value is included in the Bayesian highest posterior density interval [26,27]. For the ^MSEk, the values far from 0.00, indicated that the population values were not properly recovered. Additionally, we recorded the estimation time (in minutes) for each replication, and then computed the average estimation time (AET) for all the converged replications.
Performance measure | Formula | Role |
ˉθk: | M−1∑Mr=1ˆθ(r)k | Parameters mean estimation |
ˉ˜θk: | M−1∑Mr=1˜θ(r)k | Parameters median estimation |
^MSEk: | (M)−1∑Mr=1[ˆθ(r)k−Eθk]2 | Conformity of posterior mean |
^ASEk: | (M−1∑Mr=1[^SE(r)k]) | Precision on an estimate |
RRk: | (M)−1∑Mr=1[ˆθ(r)k/Eθk] | Precision and accuracy of an estimate |
CR(^HDI)k: | M−1∑Mr=11{θk∈^HDIrk} | Evaluate aptitude of confidence intervals to contain Eθk |
mean(ˆnESS): | M−1∑Mr=1ˆnrESS | Measure of sample effectiveness |
mean(ˆR): | M−1∑Mr=1ˆRr | diagnostic tool of good convergence |
The sensitivity to the prior information was assessed, and we checked the conventional convergence diagnostics tools provided by Stan software. First, we validated the estimation of Bayesian flexible multilevel nonlinear models parameters using the HMC algorithm through a simulation study. We assessed the estimators accuracy in terms of the average standard errors (ASE) and the mean of squared errors (MSE), and handled incertitude using ratios of the estimate/population, where values closer to 1.0 indicate proper epidemic parameters recovery. The Bayesian flexible multilevel nonlinear models showed different sensitivities compared to the standard normal nonlinear mixed model.
Figure 5 shows the convergence appreciation based on the mean effective sample size for BFMNLM curve modeling with different nonlinear parameter priors. This figure clearly shows that the levels of non-informativeness of the priors harm the model convergence. The mean effective sample size over the 200 simulated datasets of parameters were varied (Figure 5). For parameters ϕ1, ϕ2, ϕ3, and ϕ4 fixed effects knowing the informativeness level, the more accurate the prior was, the faster the convergence was, translating into higher effective sample sizes under all models (for SMSN-NLMM: mean(ˆnESS(β1)) = (136, 90, 8); mean(ˆnESS(β2)) = (487,180, 45); mean(ˆnESS(β3)) = (116, 28, 11); mean(ˆnESS(β4)) = (292, 46, 21) for 1SD10, 1SD20 and 1SD50, respectively). On the other hand (knowing prior accuracy level), the more informative the prior was, the faster the convergence was, translating into higher effective sample sizes for SMSN-NLMM (mean(ˆnESS(β1,β2,β3,β4)) = (136,487,116,292)) under the highly informative prior (1SD10) against (146,196,170,179) under the weakly informative prior (3SD10) and (19, 83, 83, 56) for a highly weak informative prior while the contrast should be concluded on the standard multilevel nonlinear model (N-NLMM). The less informative the prior was, the faster the convergence was translating into higher effective sample sizes (mean(ˆnESS(β1,β2,β3,β4)) = (450,556,454,315)) under the non-informative prior (5SD10) against (mean(ˆnESS(β1,β2,β3,β4)) = (168,291,129, 87)), under the weakly informative prior (3SD10), and (123,321, 84, 33) under the highly informative prior (1SD10). The informative prior seemed to be the most favorable to good convergence for flexible multilevel nonlinear models, as also confirmed by Gelman Rubin statistic (mean(ˆR(β2,β3)) = (1.01, 1.09)) under the highly informative prior (1SD10) against (mean(ˆR(β2,β3)) = (1.20, 1.10)) and under the weakly informative prior (3SD10). However, the weakly (or non) informative prior seemed to be the most favorable to good convergence for the standard multilevel nonlinear model as mean(ˆR(β2,β3)) = (0.99, 1.02) under the non-informative prior (5SD10) against (mean(ˆR(β2,β3)) = (1.02, 1.61)), under the weakly informative prior (3SD10), and (1.03, 1.48) under the highly informative prior (1SD10).
For each model, the computation time remained stable from one prior scenario to another (Figure 4), thus suggesting a low impact of the prior on the time consumption. It's noteworthy that the SMSN-NLMM is about two time more time-consuming in all scenarios compared to SNP-NLMM and N-NLMM. The average estimation time (min-max) in minutes was 59.7 (55–72) of all scenarios combined for SMSN-NLMM, 36.3 (31–41) for SNP-NLMM, and 28.3 (20–34) for standard multilevel nonlinear model.
Tables 3–5 contain two pieces of information aimed at quantifying the difference between the parameter estimates and the population value. First, the differences between the estimated nonlinear parameters proportions and the population proportions are presented in terms of ratios, which were defined earlier. Next, the difference between the estimated model parameters and the population values was quantified in terms of the MSE and the ASE where the closer the value was to zero, the more appreciable the estimates.
Parameters | Measures | N-NLMM | SMSN-NLMM | SNP-NLMM | ||||||||
10% | 20% | 50% | 10% | 20% | 50% | 10% | 20% | 50% | ||||
β1 | ASE | 0.679 | 0.953 | 3.415 | 0.144 | 0.639 | 1.538 | 1.128 | 1.771 | 1.823 | ||
MSE | 0.120 | 0.057 | 3.044 | 0.024 | 0.101 | 0.269 | 0.176 | 0.564 | 0.853 | |||
RR | 0.546 | 0.785 | -0.866 | 0.797 | 0.789 | 0.452 | 0.628 | 0.078 | 0.260 | |||
β2 | ASE | 0.011 | 0.160 | 0.159 | 0.006 | 0.043 | 0.147 | 0.027 | 0.112 | 0.395 | ||
MSE | 0.008 | 0.025 | 0.069 | 0.012 | 0.021 | 0.044 | 0.010 | 0.023 | 0.060 | |||
RR | 1.271 | 1.453 | 1.773 | 1.325 | 1.437 | 1.615 | 1.305 | 1.442 | 1.569 | |||
β3 | ASE | 0.130 | 0.652 | 1.907 | 0.092 | 0.213 | 0.426 | 0.367 | 1.356 | 0.929 | ||
MSE | 0.003 | 0.090 | 0.265 | 0.015 | 0.031 | 0.082 | 0.028 | 0.109 | 0.357 | |||
RR | 0.948 | 1.168 | 0.691 | 0.866 | 1.188 | 1.028 | 0.956 | 0.915 | 0.850 | |||
β4 | ASE | 0.538 | 0.805 | 1.705 | 0.016 | 0.070 | 0.177 | 1.523 | 1.723 | 1.570 | ||
MSE | 0.095 | 0.295 | 0.204 | 0.035 | 0.025 | 0.345 | 0.120 | 0.272 | 0.332 | |||
RR | 1.437 | 1.522 | 1.003 | 0.681 | 0.733 | 0.824 | 1.184 | 0.685 | 1.208 | |||
σ | ASE | 0.000 | 0.000 | 0.000 | 0.140 | 0.205 | 0.494 | 0.002 | 0.001 | 0.002 | ||
MSE | 0.143 | 0.143 | 0.143 | 0.007 | 0.008 | 0.025 | 0.143 | 0.143 | 0.143 | |||
Ω1 | ASE | 0.518 | 0.419 | 0.416 | 0.047 | 0.056 | 0.244 | 0.513 | 0.343 | 0.692 | ||
MSE | 0.062 | 0.102 | 0.079 | 0.018 | 0.029 | 0.031 | 0.111 | 0.040 | 0.039 | |||
Ω2 | ASE | 0.493 | 0.789 | 0.956 | 0.420 | 0.252 | 0.216 | 0.552 | 0.964 | 0.814 | ||
MSE | 0.158 | 0.078 | 0.300 | 0.045 | 0.117 | 0.109 | 0.194 | 0.415 | 0.121 |
Parameters | Measures | N-NLMM | SMSN-NLMM | SNP-NLMM | ||||||||
10% | 20% | 50% | 10% | 20% | 50% | 10% | 20% | 50% | ||||
β1 | ASE | 0.696 | 1.695 | 3.906 | 0.084 | 0.571 | 2.465 | 1.358 | 1.819 | 3.967 | ||
MSE | 0.428 | 1.709 | 4.667 | 0.196 | 0.186 | 0.349 | 0.286 | 0.697 | 3.105 | |||
RR | -0.025 | -0.768 | -1.605 | 0.297 | 0.416 | 0.320 | 0.293 | -0.148 | -0.539 | |||
β2 | ASE | 0.011 | 0.101 | 0.054 | 0.027 | 0.037 | 0.099 | 0.108 | 0.083 | 0.350 | ||
MSE | 0.098 | 0.182 | 0.483 | 0.098 | 0.193 | 0.515 | 0.088 | 0.177 | 0.381 | |||
RR | 1.928 | 2.252 | 3.053 | 1.929 | 2.298 | 3.121 | 1.857 | 2.244 | 2.782 | |||
β3 | ASE | 0.198 | 0.904 | 2.110 | 0.037 | 0.094 | 1.060 | 1.153 | 1.609 | 2.271 | ||
MSE | 0.184 | 0.338 | 0.484 | 0.206 | 0.395 | 0.093 | 0.040 | 0.170 | 0.022 | |||
RR | 1.461 | 0.739 | 0.493 | 1.496 | 1.686 | 1.231 | 1.111 | 0.802 | 0.943 | |||
β4 | ASE | 0.741 | 1.046 | 1.715 | 0.026 | 0.108 | 0.454 | 1.385 | 1.366 | 2.012 | ||
MSE | 0.495 | 0.567 | 0.316 | 0.158 | 0.279 | 0.743 | 0.196 | 0.559 | 0.055 | |||
RR | 0.298 | 0.322 | 0.541 | 0.326 | 0.111 | -0.346 | 0.889 | 0.408 | 1.038 | |||
σ | ASE | 0.000 | 0.000 | 0.000 | 0.302 | 0.217 | 0.325 | 0.001 | 0.001 | 0.000 | ||
MSE | 0.143 | 0.143 | 0.143 | 0.005 | 0.001 | 0.053 | 0.143 | 0.1435 | 0.143 | |||
Ω1 | ASE | 0.590 | 0.496 | 0.397 | 0.270 | 0.114 | 0.218 | 0.542 | 0.532 | 0.137 | ||
MSE | 0.449 | 0.067 | 0.043 | 0.013 | 0.014 | 0.020 | 0.013 | 0.077 | 0.024 | |||
Ω2 | ASE | 0.504 | 0.621 | 0.905 | 0.368 | 0.433 | 0.235 | 0.961 | 0.795 | 1.192 | ||
MSE | 0.503 | 0.834 | 0.509 | 0.195 | 0.154 | 0.131 | 0.408 | 0.573 | 0.235 |
Parameters | Measures | N-NLMM | SMSN-NLMM | SNP-NLMM | ||||||||
10% | 20% | 50% | 10% | 20% | 50% | 10% | 20% | 50% | ||||
β1 | ASE | 0.418 | 1.608 | 3.890 | 0.068 | 0.406 | 1.160 | 0.957 | 1.166 | 1.516 | ||
MSE | 1.081 | 1.219 | 7.045 | 0.515 | 0.895 | 1.752 | 0.560 | 1.384 | 0.290 | |||
RR | -0.565 | -0.643 | -2.107 | -0.145 | -0.496 | -0.736 | -0.003 | -0.508 | -0.886 | |||
β2 | ASE | 0.005 | 0.093 | 0.091 | 0.026 | 0.037 | 0.173 | 0.106 | 0.190 | 0.804 | ||
MSE | 0.267 | 0.519 | 1.374 | 0.262 | 0.529 | 1.464 | 0.214 | 0.419 | 1.101 | |||
RR | 2.528 | 3.125 | 4.462 | 2.513 | 3.150 | 4.568 | 2.347 | 2.827 | 3.973 | |||
β3 | ASE | 0.030 | 1.704 | 2.018 | 0.064 | 0.189 | 1.318 | 1.201 | 1.087 | 1.584 | ||
MSE | 0.633 | 0.235 | 0.524 | 0.694 | 1.387 | 1.102 | 0.182 | 0.669 | 0.289 | |||
RR | 1.870 | 1.363 | 0.542 | 1.911 | 2.287 | 1.670 | 1.143 | 0.541 | 1.319 | |||
β4 | ASE | 0.335 | 1.072 | 1.154 | 0.060 | 0.388 | 0.851 | 1.177 | 0.783 | 1.521 | ||
MSE | 0.741 | 0.670 | 0.659 | 0.448 | 0.930 | 0.982 | 0.442 | 0.868 | 0.304 | |||
RR | -0.192 | 0.209 | 0.314 | -0.132 | -0.629 | -0.614 | 0.375 | -0.018 | 1.354 | |||
σ | ASE | 0.000 | 0.000 | 0.000 | 0.368 | 0.331 | 0.174 | 0.001 | 0.001 | 0.001 | ||
MSE | 0.143 | 0.143 | 0.143 | 0.012 | 0.011 | 0.016 | 0.143 | 0.143 | 0.143 | |||
Ω1 | ASE | 0.255 | 1.211 | 0.442 | 0.712 | 0.701 | 0.372 | 0.849 | 0.464 | 0.449 | ||
MSE | 1.026 | 0.274 | 0.074 | 0.051 | 0.023 | 0.018 | 0.183 | 0.023 | 0.050 | |||
Ω2 | ASE | 0.083 | 0.656 | 0.535 | 0.365 | 0.409 | 0.301 | 0.577 | 0.508 | 0.849 | ||
MSE | 0.817 | 0.820 | 0.659 | 0.092 | 0.235 | 0.104 | 0.663 | 0.830 | 0.181 |
Figure 6 shows the parameters estimates for different models along with the true parameters values, while Tables 3–5 present corresponding average standard error (^ASEk), the ^MSEk, and the RR for all models parameters. The first general result is that under the accurate informative prior, parametric flexible multilevel nonlinear models (SMSN-NLMM) recover better epidemic nonlinear parameters, as shown with values closer to the true simulation values (Figure 6). Tables 4 and 5 present results for the 3SD and 5SD mean hyperparameter levels, respectively, and show that the nonlinear parameters were overall poorly recovered with SMSN-NLMM.
However, the SNP-NLMM had facility in recovering epidemic parameters in case of such inaccurate informative priors (cases of 50% variance hyperparameters) or weakly informative priors (cases of 3SD and 5SD mean hyperparameters), where nonlinear parameters were consistently underestimated (Figure 6). Given the same mean hyperparameter setting, the uncertainty in estimates increased with the variance hyperparameters values (RRβ1=(0.797,0.789,0.452); ^ASEβ1=(0.144,0.639,1.538) for 10%, 20%, and 50% variance, respectively). However, the more the mean hyperparameter value is far from the true value, the more the estimates uncertainty is pronounced (RRβ1=(0.8,0.3,−0.1); ^MSEβ1=(0.02,0.30,0.52) for 1SD, 3SD and 5SD mean respectively with 10% variance). These specific results are as indicated in the case of SMSN-NLMM for the SNP-NLMM and N-NLMM models (see Tables 3–5).
When considering an increased number of clusters, the results in Table 6 show the outperformance (estimates) of SMSN-NLMM on other models when more time consumption as known. Meanwhile, the results for varying the mean and variance hyperparameters are as the case of six clusters (SMSN-NLMM: RRβ2=(1.331,1.505,1.861); RRβ3=(0.865,1.020,0.969); SNP-NLMM: RRβ2=(1.671,1.791,2.067); RRβ3=(1.064,1.192,1.109); N-NLMM: RRβ2=(1.279,1.496,2.204); RRβ3=(0.723,0.797,0.478) for 10%, 20%, and 50% variance, respectively).
Models | . | 1SD10 | 1SD20 | 1SD50 | |||||||||||
β1 | β2 | β3 | β4 | β1 | β2 | β3 | β4 | β1 | β2 | β3 | β4 | ||||
N-NLMM | RR | 0.32 | 1.27 | 0.72 | 0.98 | -0.09 | 1.49 | 0.79 | 0.82 | -0.83 | 2.20 | 0.85 | 0.87 | ||
MSE | 0.28 | 0.01 | 0.10 | 0.26 | 0.53 | 0.03 | 0.09 | 0.18 | 2.68 | 0.18 | 0.32 | 0.32 | |||
AET | 31.99 | 32.12 | 22.42 | ||||||||||||
SMSN-NLMM | RR | 0.90 | 1.33 | 0.86 | 0.66 | 1.51 | 1.51 | 1.02 | 0.55 | 0.88 | 1.86 | 0.97 | 0.12 | ||
MSE | 0.01 | 0.01 | 0.02 | 0.04 | 0.12 | 0.03 | 0.01 | 0.08 | 0.59 | 0.08 | 0.02 | 0.34 | |||
AET | 57.71 | 55.72 | 56.67 | ||||||||||||
SNP-NLMM | RR | 0.88 | 1.67 | 1.06 | 1.36 | 1.03 | 1.79 | 1.19 | 1.35 | 0.89 | 2.08 | 1.11 | 1.40 | ||
MSE | 0.07 | 0.06 | 0.08 | 0.17 | 0.01 | 0.08 | 0.09 | 0.12 | 0.08 | 0.14 | 0.12 | 0.19 | |||
AET | 39.35 | 34.67 | 38.08 |
As described earlier, we considered the case of seven Francophone West African countries while estimating the average behavior of countries in the population and the variability among and within them during the first 7 months of the pandemic based on the studied approach.
Our modeling strategy was to fit the studied flexible multilevel nonlinear models and present epidemic interpretations of the results. We referred to our simulation result and Figure 2 to highlight that the flexible multilevel nonlinear models (SMSN-NLMM) with informative prior is more suitable model to the considered COVID-19 data. The code for the application of the proposed Bayesian flexible multilevel nonlinear models, with other necessary r codes, stan codes, and the considered COVID-19 data in the case of this study, is available through the GitHub link https://github.com/mathildeadeoti/BFMNLM-and-Prior. Figure 7 shows the fitting output, while the Table 7 presents keys epidemiological statistics that indicate the dynamics of COVID-19 in the seven west African countries and per country.
Cluster | ϕ1 | ϕ2 | ϕ3 | ϕ4 | TEC | EPT | R0 | First case |
Benin | 7.377 | 0.410 | 0.022 | 4.250 | 3846 | 105 | 1.167 | 16/03/2020 |
Burkina Faso | 0.389 | 0.015 | 4798 | 153 | 1.115 | 10/03/2020 | ||
Guinea | 0.327 | 0.020 | 10,111 | 127 | 1.150 | 13/03/2020 | ||
Ivory-Coast | 0.292 | 0.024 | 16,297 | 110 | 1.840 | 11/03/2020 | ||
Mali | 0.400 | 0.031 | 4255 | 74 | 1.249 | 25/03/2020 | ||
Niger | 0.448 | 0.025 | 2644 | 90 | 1.890 | 20/03/2020 | ||
Senegal | 0.291 | 0.023 | 16,607 | 118 | 1.172 | 02/03/2020 |
The figure confirmed how likely the model fits the data trajectory better by considering the inter-variability of countries. Table 7 includes the estimated total number of cases (TEC:Total_Est_Cases) at the end of the pandemic, the estimated peak time (EPT:Est_Peak_Time), and the basic reproduction numbers (R0) based on flexible multilevel nonlinear models (SMSN-NLMM) with the informative prior. It showed a great heterogeneity in the region in terms of epidemic dynamic (Total_Est_Cases, Est_Peak_Time, etc.). This is clearly understandable as the population density and testing efforts were not homogeneous [28]. In most countries, the model estimated an average of one new case of infection caused by an infected individual during the infectious period (R0≈1). The estimated total number of cases (Total_Est_Cases) serves as a reference to determine whether one country is probably at the end of the pandemic or not, while Est_Peak_Time indicates whether the worst phase has passed.
The results show that the final sizes of the epidemic were estimated to be relatively larger in Senegal, Ivory-Coast, and Guinea compared to all other countries with an estimated number of 16,607, 16,297, and 10,111 cases, respectively, before expecting the end of the pandemic. For the remaining countries, namely Burkina-Faso, Mali, Benin, and Niger, the epidemic reached its final size at 4798, 4255, 3846, and 2644 reported cases, respectively. The peak time was estimated at 74, 90,105,110,118, and 127 days from the first detected case date for Mali, Niger, Benin, Ivory-Coast, Senegal, and Guinea, respectively, whereas it was relatively larger for Burkina-Faso (around 153 days). This means that the epidemic progression is relatively slower in the latter country compared to the former (as confirmed in the fitted trajectories on Figure 7). In general, the estimated peak times range along with the observed true peak time, while their estimated reported peak sizes accounted for less than 1% of their population size, on average (Table 7, Figure 7). This underlined that all the considered countries would reach their peak with less than 1% of their population being affected.
The basic usefulness of the approach was confirmed from the estimates of the parameters (ˆϕk) of the logistic dynamic of the model. The result corroborate the assumption on the data, as the estimated ˆϕ4≫1 indicates a right skewness for the West African countries pandemic data, thus suggesting that the increased phase occurs much faster than the decrease phase in many of the countries.
While compartmental models and other statistical tools are most commonly used in epidemic modeling [16,29,30], NLMMs have received particular attention due to their flexibility in handling the heterogeneous and unbalanced repeated measures data that can arise in infectious disease modeling. Although flexible multilevel nonlinear curve modeling has been proposed, especially in the Bayesian approach, the effect of the parameter prior distribution has not been fully studied.
A sensitivity analysis procedure relatively to the specification of priors can assess the sensitivity of the posterior densities to the choice of prior distributions [31]. Informative priors can have an impact on estimates, even if little methodological research has directly focused on this matter, as previously reported [3]. Basically, this process is a means to investigate the robustness of a model by systematically changing priors to assess the impact of those changes. That is, this paper simply reconsidered the proposed Bayesian multilevel nonlinear models by making reasonable modifications to the prior assumptions, recomputing the posterior quantities of interest, and observing whether they have changed in a way that significantly affects the resulting interpretations or conclusions.
Our results corroborate with the one of [32] for the fact that outliers influence the computation time. Therefore, the proposed model based on the scale mixture of skew normal distribution is more time consuming than the standard. Additionally, the results clearly showed that the levels of non-informativeness of the priors harmed the model convergence. For logistics parameters ϕ1, ϕ2, ϕ3, and ϕ4 fixed effects knowing informativeness level, the more accurate the prior, the faster the convergence translated into higher effective sample sizes for the studied flexible multilevel nonlinear model (SMSN-NLMM). Therefor, the informative prior seemed to be the most favorable to a good convergence for flexible multilevel nonlinear models as confirmed by the Gelman Rubin statistic, while the weakly (or non) informative prior would be the most favorable to a good convergence for either the semi-nonparametric nonlinear mixed model or the standard multilevel nonlinear model.
The analysis of such complex epidemic data requires more consideration due to some inherent features: countries at different stages of pandemic, outliers in the response, and the specific case of Francophone West African countries considered in this study, with several consecutive days with zero detected cases mainly due to their test capacity. As a flexible multilevel modeling, the fitted curve (Figure 7) showed an increased sensitivity to outliers and variations of the epidemic stages; this can either positively or negatively influence the analysis or interpretations. It's advisable to consider estimates and computable key epidemic parameters for rational analyses of the fitting trajectories.
One of the advantages of the Bayesian approach is that the uncertainty in all parameter values is taken into account through the use of prior distributions [33]. However, priors have also been pinpointed as being one of the main drawbacks to this framework as a result of the inherent subjectivity that is coupled with choosing prior distributions and the corresponding hyperparameters. Meanwhile, the actual impact of prior distributions is an important research topic to explore within Bayesian estimation. Although (non) informative priors can have a large impact on the estimates (see, e.g., [34]), little methodological research has directly focused on this matter. Most researches explore a feature of the sample size used for an estimation in the data analysis within any estimation framework. However, this issue of sample sizes is closely tied to the use of prior distributions within the Bayesian estimation. Research has indicated that even noninformative priors can impact the estimates using larger sample sizes. Then, it is important to never underestimate the impact of a prior distribution, regardless of the sample size, as previously underlined [35]. Meanwhile, this paper endorsed the use of the proposed methodology that jointly accommodated the different stages of the diseases while borrowing information of the different time series to provide a more robust and reliable fit and prediction. With such a joint accommodation, we strongly believe that this methodology of Bayesian flexible multilevel nonlinear models can provide more reliable and meaningful predictions that allow policymakers around the world to make effective decisions.
Bayesian flexible multilevel nonlinear models are powerful tools to analyze infectious disease data with asymmetric and unbalanced structures, such as varying epidemic stages across countries. This study investigated how varying levels of prior informativeness can influence the model convergence, parameter estimation, and computation time in a Bayesian FMNLM. The key finding from this study is outlined as follows:
- Outliers influence the computation time, thus leading to more time consuming of flexible multilevel nonlinear model, though the levels of informativeness of the priors do not harm model computation time;
- The results clearly show that the levels of non-informativeness of the priors harm the model convergence;
- Knowing the informativeness level, the more accurate the prior, the faster the convergence for the studied flexible multilevel nonlinear model (SMSN-NLMM);
- The weakly (or non) informative prior is the most favorable to a good convergence of either the semi-nonparametric nonlinear mixed model or the standard multilevel nonlinear model;
- Under an accurate informative prior, the parametric flexible multilevel nonlinear models (SMSN-NLMM) recover the epidemic nonlinear parameters better;
- Giving the same mean hyperparameter setting, the uncertainty in estimates increases with the variance hyperparameters values;
- The cluster size or number of countries/areas have no impact on the estimates of Bayesian FMNLM.
The authors declare they have not used Artificial Intelligence (AI) tools in the creation of this article.
OMA acknowledges the support from Deutscher Akademischer Austauschdienst German Academic Exchange Service (DAAD) through the programme In-Country/In-Region Scholarship. OMA is also grateful to the Centre d'Excellence d'Afrique en Sciences Mathématiques, Informatique et Applications (CEA-SMIA) for supporting his works. RGK acknowledges the Sub-Saharan Africa Advanced Consortium for Biostatistics (SSACAB) Phase Ⅱ.
OMA and RGK conceptualized the problem. OMA and AD designed the Methodology. RGK and AD administrated the project. OMA did the resource and software handling. RGK and AD supervised the project. The original draft writing and editing involved all the authors.
The authors declare there is no conflict of interest.
[1] |
Y. Huang, D. Liu, H. Wu, Hierarchical bayesian methods for estimation of parameters in a longitudinal HIV dynamic system, Biometrics, 62 (2006), 413–423. https://doi.org/10.1111/j.1541-0420.2005.00447.x doi: 10.1111/j.1541-0420.2005.00447.x
![]() |
[2] |
Y. Huang, G. Dagne, Skew-normal bayesian nonlinear mixed-effects models with application to aids studies, Stat. Med., 29 (2010), 2384–2398. https://doi.org/10.1002/sim.3996 doi: 10.1002/sim.3996
![]() |
[3] |
S. Depaoli, The impact of inaccurate "informative" priors for growth parameters in bayesian growth mixture modeling, Struct. Equation Model. Multidiscip. J., 21 (2014), 239–252. https://doi.org/10.1080/10705511.2014.882686 doi: 10.1080/10705511.2014.882686
![]() |
[4] |
M. Kerioui, F. Mercier, J. Bertrand, C. Tardivon, R. Bruno, J. Guedj, et al., Bayesian inference using hamiltonian monte-carlo algorithm for nonlinear joint modeling in the context of cancer immunotherapy, Stat. Med., 39 (2020), 4853–4868. https://doi.org/10.1002/sim.8756 doi: 10.1002/sim.8756
![]() |
[5] |
M. D. Branco, D. K. Dey, A general class of multivariate skew-elliptical distributions, J. Multivar. Anal., 79 (2001), 99–113. https://doi.org/10.1006/jmva.2000.1960 doi: 10.1006/jmva.2000.1960
![]() |
[6] |
C. Meza, F. Osorio, R. De la Cruz, Estimation in nonlinear mixed-effects models using heavy-tailed distributions, Stat. Comput., 22 (2012), 121–139. https://doi.org/10.1007/s11222-010-9212-1 doi: 10.1007/s11222-010-9212-1
![]() |
[7] |
C. F. Tovissodé, A. Diop, R. Glèlè Kakaï, Inference in skew generalized t-link models for clustered binary outcome via a parameter-expanded EM algorithm, Plos One, 16 (2021), e0249604. https://doi.org/10.1371/journal.pone.0249604 doi: 10.1371/journal.pone.0249604
![]() |
[8] |
D. Zhang, M. Davidian, Linear mixed models with flexible distributions of random effects for longitudinal data, Biometrics, 57 (2001), 795–802. https://doi.org/10.1111/j.0006-341X.2001.00795.x doi: 10.1111/j.0006-341X.2001.00795.x
![]() |
[9] |
J. Chen, D. Zhang, M. Davidian, A Monte Carlo EM algorithm for generalized linear mixed models with flexible random effects distribution, Biostatistics, 3 (2002), 347–360. https://doi.org/10.1093/biostatistics/3.3.347 doi: 10.1093/biostatistics/3.3.347
![]() |
[10] |
E. Bonnet, O. Bodson, F. Le Marcis, A. Faye, N. Sambieni, F. Fournet, et al., The COVID-19 pandemic in Francophone West Africa: from the first cases to responses in seven countries, BMC Public Health, 21 (2021), 1–17. https://doi.org/10.1186/s12889-021-11529-7 doi: 10.1186/s12889-021-11529-7
![]() |
[11] | E. Dong, H. Du, L. Gardner, An interactive web-based dashboard to track COVID-19 in real time, Lancet Infect. Dis., 20 (2020), 533–534. |
[12] |
V. H. Lachos, D. Bandyopadhyay, D. K. Dey, Linear and nonlinear mixed-effects models for censored HIV viral loads using normal/independent distributions, Biometrics, 67 (2011), 1594–1604. https://doi.org/10.1111/j.1541-0420.2011.01586.x doi: 10.1111/j.1541-0420.2011.01586.x
![]() |
[13] |
F. L. Schumacher, C. S. Ferreira, M. O. Prates, A. Lachos, V. H. Lachos, A robust nonlinear mixed-effects model for COVID-19 death data, Stat. Interface, 14 (2021), 49–57. https://doi.org/10.4310/20-SII637 doi: 10.4310/20-SII637
![]() |
[14] |
M. J. Lindstrom, D. M. Bates, Nonlinear mixed effects models for repeated measures data, Biometrics, (1990), 673–687. https://doi.org/10.2307/2532087 doi: 10.2307/2532087
![]() |
[15] |
V. H. Lachos, L. M. Castro, D. K. Dey, Bayesian inference in nonlinear mixed-effects models using normal independent distributions, Comput. Stat. Data Anal., 64 (2013), 237–252. https://doi.org/10.1016/j.csda.2013.02.011 doi: 10.1016/j.csda.2013.02.011
![]() |
[16] |
O. M. Adéoti, S. Agbla, A. Diop, R. G. Kakaï, Nonlinear mixed models and related approaches in infectious disease modeling: A systematic and critical review, Infect. Dis. Modell., 10 (2024), 110–128. https://doi.org/10.1016/j.idm.2024.09.001 doi: 10.1016/j.idm.2024.09.001
![]() |
[17] | J. Chen, D. Zhang, M. Davidian, A Monte Carlo EM algorithm for generalized linear mixed models with flexible random effects distribution, Ph.D. thesis, Graduate Faculty of North Carolina State University, 2001. |
[18] | M. Davidian, D. M. Giltinan, Nonlinear Models for Repeated Measurement Data, CRC press, 62 (1995). |
[19] |
M. Davidian, A. R. Gallant, The nonlinear mixed effects model with a smooth random effects density, Biometrika, 80 (1993), 475–488. https://doi.org/10.1093/biomet/80.3.475 doi: 10.1093/biomet/80.3.475
![]() |
[20] |
J. P. Hobert, G. Casella, The effect of improper priors on Gibbs sampling in hierarchical linear mixed models, J. Am. Stat. Assoc., 91 (1996), 1461–1473. https://doi.org/10.1080/01621459.1996.10476714 doi: 10.1080/01621459.1996.10476714
![]() |
[21] |
P. C. Bürkner, brms: An R package for bayesian multilevel models using Stan, J. Stat. Software, 80 (2017), 1–28. https://doi.org/10.18637/jss.v080.i01 doi: 10.18637/jss.v080.i01
![]() |
[22] | T. Stan Development, Rstan: the r interface to stan, R packages 2.17.3. (2018). |
[23] | R. C. Team, R: A language and environment for statistical computing (version 3.1. 2). vienna, austria. r foundation for statistical computing; 2014, 2019. |
[24] | C. Team, Covidlp: short and long term prediction for COVID-19, Departamento de Estatıstica, UFMG, Brazil, 2020. Available from: http://est.ufmg.br/covidlp/home/en. |
[25] |
J. Wallinga, M. Lipsitch, How generation intervals shape the relationship between growth rates and reproductive numbers, Proc. R. Soc. B, 274 (2007), 599–604. https://doi.org/10.1098/rspb.2006.3754 doi: 10.1098/rspb.2006.3754
![]() |
[26] |
D. Makowski, M. S. Ben-Shachar, D. Lüdecke, bayestestR: Describing effects and their uncertainty, existence and significance within the bayesian framework, J. Open Source Software, 4 (2019), 1541. https://doi.org/10.21105/joss.01541 doi: 10.21105/joss.01541
![]() |
[27] |
J. M. Curran, An introduction to bayesian credible intervals for sampling error in DNA profiles, Law Probab. Risk, 4 (2005), 115–126. https://doi.org/10.1093/lpr/mgi009 doi: 10.1093/lpr/mgi009
![]() |
[28] |
S. H. Honfo, H. B. Taboe, R. G. Kakaï, Modeling COVID-19 dynamics in the sixteen West African countries, Sci. Afr., 18 (2022), e01408. https://doi.org/10.1016/j.sciaf.2022.e01408 doi: 10.1016/j.sciaf.2022.e01408
![]() |
[29] |
L. Tang, Y. Zhou, L. Wang, S. Purkayastha, L. Zhang, J. He, et al., A review of multi-compartment infectious disease models, Int. Stat. Rev., 88 (2020), 462–513. https://doi.org/10.1111/insr.12402 doi: 10.1111/insr.12402
![]() |
[30] |
J. E. Gnanvi, K. V. Salako, G. B. Kotanmi, R. Glèlè Kakaï, On the reliability of predictions on COVID-19 dynamics: A systematic and critical review of modelling techniques, Infect. Dis. Modell., 6 (2021), 258–272. https://doi.org/10.1016/j.idm.2020.12.008 doi: 10.1016/j.idm.2020.12.008
![]() |
[31] |
R. E. Kass, L. Wasserman, The selection of prior distributions by formal rules, J. Am. Stat. Assoc., 91 (1996), 1343–1370. https://doi.org/10.1080/01621459.1996.10477003 doi: 10.1080/01621459.1996.10477003
![]() |
[32] |
X. Tong, Z. Ke, Assessing the impact of precision parameter prior in bayesian non-parametric growth curve modeling, Front. Psychol., 12 (2021), 624588. https://doi.org/10.3389/fpsyg.2021.624588 doi: 10.3389/fpsyg.2021.624588
![]() |
[33] |
P. C. Lambert, A. J. Sutton, P. R. Burton, K. R. Abrams, D. R. Jones, How vague is vague? A simulation study of the impact of the use of vague prior distributions in MCMC using WinBUGS, Stat. Med., 24 (2005), 2401–2428. https://doi.org/10.1002/sim.2112 doi: 10.1002/sim.2112
![]() |
[34] |
Z. Zhang, F. Hamagami, L. Wang, J. R. Nesselroade, K. J. Grimm, Bayesian analysis of longitudinal data using growth curve models, Int. J. Behav. Dev., 31 (2007), 374–383. https://doi.org/10.1177/0165025407077764 doi: 10.1177/0165025407077764
![]() |
[35] |
R. Natarajan, C. E. McCulloch, Gibbs sampling with diffuse proper priors: A valid approach to data-driven inference?, J. Comput. Graphical Stat., 7 (1998), 267–277. https://doi.org/10.1080/10618600.1998.10474776 doi: 10.1080/10618600.1998.10474776
![]() |
Parameters | Population values | Variance Hyperparameter Levels | ||||
10.00% Variance | 20.00% Variance | 50.00% Variance | ||||
Mean Hyperparameter Level: 1SD | ||||||
β1 | 1.98 (10−1) | N(1.535,0.198) | N(1.351,0.396) | N(0.985,0.990) | ||
β2 | -1.07 (10−1) | N(−1.397,0.107) | N(−1.533,0.214) | N(−1.801,0.535) | ||
β3 | -2.89 (10−1) | N(−3.428,0.289) | N(−3.650,0.578) | N(−4.092,1.445) | ||
β4 | 1.87 (10−1) | N(1.438,0.187) | N(1.258,0.374) | N(0.903,0.935) | ||
σ | 3.8 | |||||
Ω1 | 0.721 | |||||
Ω2 | 0.589 | |||||
Ω12 | -0.097 | |||||
δ & δb | 0.1 | |||||
ν & νb | 1.98 | |||||
Mean Hyperparameter Level: 3SD | ||||||
β1 | 1.98 (10−1) | N(0.645,0.198) | N(0.092,0.396) | N(−1.005,0.990) | ||
β2 | -1.07 (10−1) | N(−2.051,0.107) | N(−2.458,0.214) | N(−3.264,0.535) | ||
β3 | -2.89 (10−1) | N(−4.503,0.289) | N(−5.171,0.578) | N(−6.496,1.445) | ||
β4 | 1.87 (10−1) | N(0.573,0.187) | N(0.035,0.374) | N(−1.031,0.935) | ||
σ | 3.8 | |||||
Ω1 | 0.721 | |||||
Ω2 | 0.589 | |||||
Ω12 | -0.097 | |||||
δ & δb | 0.1 | |||||
ν & νb | 1.98 | |||||
Mean Hyperparameter Level: 5SD | ||||||
β1 | 1.98 (10−1) | N(−0.245,0.198) | N(−1.166,0.396) | N(−2.995,0.990) | ||
β2 | -1.07 (10−1) | N(−2.706,0.107) | N(−3.383,0.214) | N(−4.727,0.535) | ||
β3 | -2.89 (10−1) | N(−5.578,0.289) | N(−6.691,0.578) | N(−8.900,1.445) | ||
β4 | 1.87 (10−1) | N(−0.292,0.187) | N(−1.188,0.374) | N(−2.965,0.935) | ||
σ | 3.8 | |||||
Ω1 | 0.721 | |||||
Ω2 | 0.589 | |||||
Ω12 | -0.097 | |||||
δ & δb | 0.1 | |||||
ν & νb | 1.98 |
Performance measure | Formula | Role |
ˉθk: | M−1∑Mr=1ˆθ(r)k | Parameters mean estimation |
ˉ˜θk: | M−1∑Mr=1˜θ(r)k | Parameters median estimation |
^MSEk: | (M)−1∑Mr=1[ˆθ(r)k−Eθk]2 | Conformity of posterior mean |
^ASEk: | (M−1∑Mr=1[^SE(r)k]) | Precision on an estimate |
RRk: | (M)−1∑Mr=1[ˆθ(r)k/Eθk] | Precision and accuracy of an estimate |
CR(^HDI)k: | M−1∑Mr=11{θk∈^HDIrk} | Evaluate aptitude of confidence intervals to contain Eθk |
mean(ˆnESS): | M−1∑Mr=1ˆnrESS | Measure of sample effectiveness |
mean(ˆR): | M−1∑Mr=1ˆRr | diagnostic tool of good convergence |
Parameters | Measures | N-NLMM | SMSN-NLMM | SNP-NLMM | ||||||||
10% | 20% | 50% | 10% | 20% | 50% | 10% | 20% | 50% | ||||
β1 | ASE | 0.679 | 0.953 | 3.415 | 0.144 | 0.639 | 1.538 | 1.128 | 1.771 | 1.823 | ||
MSE | 0.120 | 0.057 | 3.044 | 0.024 | 0.101 | 0.269 | 0.176 | 0.564 | 0.853 | |||
RR | 0.546 | 0.785 | -0.866 | 0.797 | 0.789 | 0.452 | 0.628 | 0.078 | 0.260 | |||
β2 | ASE | 0.011 | 0.160 | 0.159 | 0.006 | 0.043 | 0.147 | 0.027 | 0.112 | 0.395 | ||
MSE | 0.008 | 0.025 | 0.069 | 0.012 | 0.021 | 0.044 | 0.010 | 0.023 | 0.060 | |||
RR | 1.271 | 1.453 | 1.773 | 1.325 | 1.437 | 1.615 | 1.305 | 1.442 | 1.569 | |||
β3 | ASE | 0.130 | 0.652 | 1.907 | 0.092 | 0.213 | 0.426 | 0.367 | 1.356 | 0.929 | ||
MSE | 0.003 | 0.090 | 0.265 | 0.015 | 0.031 | 0.082 | 0.028 | 0.109 | 0.357 | |||
RR | 0.948 | 1.168 | 0.691 | 0.866 | 1.188 | 1.028 | 0.956 | 0.915 | 0.850 | |||
β4 | ASE | 0.538 | 0.805 | 1.705 | 0.016 | 0.070 | 0.177 | 1.523 | 1.723 | 1.570 | ||
MSE | 0.095 | 0.295 | 0.204 | 0.035 | 0.025 | 0.345 | 0.120 | 0.272 | 0.332 | |||
RR | 1.437 | 1.522 | 1.003 | 0.681 | 0.733 | 0.824 | 1.184 | 0.685 | 1.208 | |||
σ | ASE | 0.000 | 0.000 | 0.000 | 0.140 | 0.205 | 0.494 | 0.002 | 0.001 | 0.002 | ||
MSE | 0.143 | 0.143 | 0.143 | 0.007 | 0.008 | 0.025 | 0.143 | 0.143 | 0.143 | |||
Ω1 | ASE | 0.518 | 0.419 | 0.416 | 0.047 | 0.056 | 0.244 | 0.513 | 0.343 | 0.692 | ||
MSE | 0.062 | 0.102 | 0.079 | 0.018 | 0.029 | 0.031 | 0.111 | 0.040 | 0.039 | |||
Ω2 | ASE | 0.493 | 0.789 | 0.956 | 0.420 | 0.252 | 0.216 | 0.552 | 0.964 | 0.814 | ||
MSE | 0.158 | 0.078 | 0.300 | 0.045 | 0.117 | 0.109 | 0.194 | 0.415 | 0.121 |
Parameters | Measures | N-NLMM | SMSN-NLMM | SNP-NLMM | ||||||||
10% | 20% | 50% | 10% | 20% | 50% | 10% | 20% | 50% | ||||
β1 | ASE | 0.696 | 1.695 | 3.906 | 0.084 | 0.571 | 2.465 | 1.358 | 1.819 | 3.967 | ||
MSE | 0.428 | 1.709 | 4.667 | 0.196 | 0.186 | 0.349 | 0.286 | 0.697 | 3.105 | |||
RR | -0.025 | -0.768 | -1.605 | 0.297 | 0.416 | 0.320 | 0.293 | -0.148 | -0.539 | |||
β2 | ASE | 0.011 | 0.101 | 0.054 | 0.027 | 0.037 | 0.099 | 0.108 | 0.083 | 0.350 | ||
MSE | 0.098 | 0.182 | 0.483 | 0.098 | 0.193 | 0.515 | 0.088 | 0.177 | 0.381 | |||
RR | 1.928 | 2.252 | 3.053 | 1.929 | 2.298 | 3.121 | 1.857 | 2.244 | 2.782 | |||
β3 | ASE | 0.198 | 0.904 | 2.110 | 0.037 | 0.094 | 1.060 | 1.153 | 1.609 | 2.271 | ||
MSE | 0.184 | 0.338 | 0.484 | 0.206 | 0.395 | 0.093 | 0.040 | 0.170 | 0.022 | |||
RR | 1.461 | 0.739 | 0.493 | 1.496 | 1.686 | 1.231 | 1.111 | 0.802 | 0.943 | |||
β4 | ASE | 0.741 | 1.046 | 1.715 | 0.026 | 0.108 | 0.454 | 1.385 | 1.366 | 2.012 | ||
MSE | 0.495 | 0.567 | 0.316 | 0.158 | 0.279 | 0.743 | 0.196 | 0.559 | 0.055 | |||
RR | 0.298 | 0.322 | 0.541 | 0.326 | 0.111 | -0.346 | 0.889 | 0.408 | 1.038 | |||
σ | ASE | 0.000 | 0.000 | 0.000 | 0.302 | 0.217 | 0.325 | 0.001 | 0.001 | 0.000 | ||
MSE | 0.143 | 0.143 | 0.143 | 0.005 | 0.001 | 0.053 | 0.143 | 0.1435 | 0.143 | |||
Ω1 | ASE | 0.590 | 0.496 | 0.397 | 0.270 | 0.114 | 0.218 | 0.542 | 0.532 | 0.137 | ||
MSE | 0.449 | 0.067 | 0.043 | 0.013 | 0.014 | 0.020 | 0.013 | 0.077 | 0.024 | |||
Ω2 | ASE | 0.504 | 0.621 | 0.905 | 0.368 | 0.433 | 0.235 | 0.961 | 0.795 | 1.192 | ||
MSE | 0.503 | 0.834 | 0.509 | 0.195 | 0.154 | 0.131 | 0.408 | 0.573 | 0.235 |
Parameters | Measures | N-NLMM | SMSN-NLMM | SNP-NLMM | ||||||||
10% | 20% | 50% | 10% | 20% | 50% | 10% | 20% | 50% | ||||
β1 | ASE | 0.418 | 1.608 | 3.890 | 0.068 | 0.406 | 1.160 | 0.957 | 1.166 | 1.516 | ||
MSE | 1.081 | 1.219 | 7.045 | 0.515 | 0.895 | 1.752 | 0.560 | 1.384 | 0.290 | |||
RR | -0.565 | -0.643 | -2.107 | -0.145 | -0.496 | -0.736 | -0.003 | -0.508 | -0.886 | |||
β2 | ASE | 0.005 | 0.093 | 0.091 | 0.026 | 0.037 | 0.173 | 0.106 | 0.190 | 0.804 | ||
MSE | 0.267 | 0.519 | 1.374 | 0.262 | 0.529 | 1.464 | 0.214 | 0.419 | 1.101 | |||
RR | 2.528 | 3.125 | 4.462 | 2.513 | 3.150 | 4.568 | 2.347 | 2.827 | 3.973 | |||
β3 | ASE | 0.030 | 1.704 | 2.018 | 0.064 | 0.189 | 1.318 | 1.201 | 1.087 | 1.584 | ||
MSE | 0.633 | 0.235 | 0.524 | 0.694 | 1.387 | 1.102 | 0.182 | 0.669 | 0.289 | |||
RR | 1.870 | 1.363 | 0.542 | 1.911 | 2.287 | 1.670 | 1.143 | 0.541 | 1.319 | |||
β4 | ASE | 0.335 | 1.072 | 1.154 | 0.060 | 0.388 | 0.851 | 1.177 | 0.783 | 1.521 | ||
MSE | 0.741 | 0.670 | 0.659 | 0.448 | 0.930 | 0.982 | 0.442 | 0.868 | 0.304 | |||
RR | -0.192 | 0.209 | 0.314 | -0.132 | -0.629 | -0.614 | 0.375 | -0.018 | 1.354 | |||
σ | ASE | 0.000 | 0.000 | 0.000 | 0.368 | 0.331 | 0.174 | 0.001 | 0.001 | 0.001 | ||
MSE | 0.143 | 0.143 | 0.143 | 0.012 | 0.011 | 0.016 | 0.143 | 0.143 | 0.143 | |||
Ω1 | ASE | 0.255 | 1.211 | 0.442 | 0.712 | 0.701 | 0.372 | 0.849 | 0.464 | 0.449 | ||
MSE | 1.026 | 0.274 | 0.074 | 0.051 | 0.023 | 0.018 | 0.183 | 0.023 | 0.050 | |||
Ω2 | ASE | 0.083 | 0.656 | 0.535 | 0.365 | 0.409 | 0.301 | 0.577 | 0.508 | 0.849 | ||
MSE | 0.817 | 0.820 | 0.659 | 0.092 | 0.235 | 0.104 | 0.663 | 0.830 | 0.181 |
Models | . | 1SD10 | 1SD20 | 1SD50 | |||||||||||
β1 | β2 | β3 | β4 | β1 | β2 | β3 | β4 | β1 | β2 | β3 | β4 | ||||
N-NLMM | RR | 0.32 | 1.27 | 0.72 | 0.98 | -0.09 | 1.49 | 0.79 | 0.82 | -0.83 | 2.20 | 0.85 | 0.87 | ||
MSE | 0.28 | 0.01 | 0.10 | 0.26 | 0.53 | 0.03 | 0.09 | 0.18 | 2.68 | 0.18 | 0.32 | 0.32 | |||
AET | 31.99 | 32.12 | 22.42 | ||||||||||||
SMSN-NLMM | RR | 0.90 | 1.33 | 0.86 | 0.66 | 1.51 | 1.51 | 1.02 | 0.55 | 0.88 | 1.86 | 0.97 | 0.12 | ||
MSE | 0.01 | 0.01 | 0.02 | 0.04 | 0.12 | 0.03 | 0.01 | 0.08 | 0.59 | 0.08 | 0.02 | 0.34 | |||
AET | 57.71 | 55.72 | 56.67 | ||||||||||||
SNP-NLMM | RR | 0.88 | 1.67 | 1.06 | 1.36 | 1.03 | 1.79 | 1.19 | 1.35 | 0.89 | 2.08 | 1.11 | 1.40 | ||
MSE | 0.07 | 0.06 | 0.08 | 0.17 | 0.01 | 0.08 | 0.09 | 0.12 | 0.08 | 0.14 | 0.12 | 0.19 | |||
AET | 39.35 | 34.67 | 38.08 |
Cluster | ϕ1 | ϕ2 | ϕ3 | ϕ4 | TEC | EPT | R0 | First case |
Benin | 7.377 | 0.410 | 0.022 | 4.250 | 3846 | 105 | 1.167 | 16/03/2020 |
Burkina Faso | 0.389 | 0.015 | 4798 | 153 | 1.115 | 10/03/2020 | ||
Guinea | 0.327 | 0.020 | 10,111 | 127 | 1.150 | 13/03/2020 | ||
Ivory-Coast | 0.292 | 0.024 | 16,297 | 110 | 1.840 | 11/03/2020 | ||
Mali | 0.400 | 0.031 | 4255 | 74 | 1.249 | 25/03/2020 | ||
Niger | 0.448 | 0.025 | 2644 | 90 | 1.890 | 20/03/2020 | ||
Senegal | 0.291 | 0.023 | 16,607 | 118 | 1.172 | 02/03/2020 |
Parameters | Population values | Variance Hyperparameter Levels | ||||
10.00% Variance | 20.00% Variance | 50.00% Variance | ||||
Mean Hyperparameter Level: 1SD | ||||||
β1 | 1.98 (10−1) | N(1.535,0.198) | N(1.351,0.396) | N(0.985,0.990) | ||
β2 | -1.07 (10−1) | N(−1.397,0.107) | N(−1.533,0.214) | N(−1.801,0.535) | ||
β3 | -2.89 (10−1) | N(−3.428,0.289) | N(−3.650,0.578) | N(−4.092,1.445) | ||
β4 | 1.87 (10−1) | N(1.438,0.187) | N(1.258,0.374) | N(0.903,0.935) | ||
σ | 3.8 | |||||
Ω1 | 0.721 | |||||
Ω2 | 0.589 | |||||
Ω12 | -0.097 | |||||
δ & δb | 0.1 | |||||
ν & νb | 1.98 | |||||
Mean Hyperparameter Level: 3SD | ||||||
β1 | 1.98 (10−1) | N(0.645,0.198) | N(0.092,0.396) | N(−1.005,0.990) | ||
β2 | -1.07 (10−1) | N(−2.051,0.107) | N(−2.458,0.214) | N(−3.264,0.535) | ||
β3 | -2.89 (10−1) | N(−4.503,0.289) | N(−5.171,0.578) | N(−6.496,1.445) | ||
β4 | 1.87 (10−1) | N(0.573,0.187) | N(0.035,0.374) | N(−1.031,0.935) | ||
σ | 3.8 | |||||
Ω1 | 0.721 | |||||
Ω2 | 0.589 | |||||
Ω12 | -0.097 | |||||
δ & δb | 0.1 | |||||
ν & νb | 1.98 | |||||
Mean Hyperparameter Level: 5SD | ||||||
β1 | 1.98 (10−1) | N(−0.245,0.198) | N(−1.166,0.396) | N(−2.995,0.990) | ||
β2 | -1.07 (10−1) | N(−2.706,0.107) | N(−3.383,0.214) | N(−4.727,0.535) | ||
β3 | -2.89 (10−1) | N(−5.578,0.289) | N(−6.691,0.578) | N(−8.900,1.445) | ||
β4 | 1.87 (10−1) | N(−0.292,0.187) | N(−1.188,0.374) | N(−2.965,0.935) | ||
σ | 3.8 | |||||
Ω1 | 0.721 | |||||
Ω2 | 0.589 | |||||
Ω12 | -0.097 | |||||
δ & δb | 0.1 | |||||
ν & νb | 1.98 |
Performance measure | Formula | Role |
ˉθk: | M−1∑Mr=1ˆθ(r)k | Parameters mean estimation |
ˉ˜θk: | M−1∑Mr=1˜θ(r)k | Parameters median estimation |
^MSEk: | (M)−1∑Mr=1[ˆθ(r)k−Eθk]2 | Conformity of posterior mean |
^ASEk: | (M−1∑Mr=1[^SE(r)k]) | Precision on an estimate |
RRk: | (M)−1∑Mr=1[ˆθ(r)k/Eθk] | Precision and accuracy of an estimate |
CR(^HDI)k: | M−1∑Mr=11{θk∈^HDIrk} | Evaluate aptitude of confidence intervals to contain Eθk |
mean(ˆnESS): | M−1∑Mr=1ˆnrESS | Measure of sample effectiveness |
mean(ˆR): | M−1∑Mr=1ˆRr | diagnostic tool of good convergence |
Parameters | Measures | N-NLMM | SMSN-NLMM | SNP-NLMM | ||||||||
10% | 20% | 50% | 10% | 20% | 50% | 10% | 20% | 50% | ||||
β1 | ASE | 0.679 | 0.953 | 3.415 | 0.144 | 0.639 | 1.538 | 1.128 | 1.771 | 1.823 | ||
MSE | 0.120 | 0.057 | 3.044 | 0.024 | 0.101 | 0.269 | 0.176 | 0.564 | 0.853 | |||
RR | 0.546 | 0.785 | -0.866 | 0.797 | 0.789 | 0.452 | 0.628 | 0.078 | 0.260 | |||
β2 | ASE | 0.011 | 0.160 | 0.159 | 0.006 | 0.043 | 0.147 | 0.027 | 0.112 | 0.395 | ||
MSE | 0.008 | 0.025 | 0.069 | 0.012 | 0.021 | 0.044 | 0.010 | 0.023 | 0.060 | |||
RR | 1.271 | 1.453 | 1.773 | 1.325 | 1.437 | 1.615 | 1.305 | 1.442 | 1.569 | |||
β3 | ASE | 0.130 | 0.652 | 1.907 | 0.092 | 0.213 | 0.426 | 0.367 | 1.356 | 0.929 | ||
MSE | 0.003 | 0.090 | 0.265 | 0.015 | 0.031 | 0.082 | 0.028 | 0.109 | 0.357 | |||
RR | 0.948 | 1.168 | 0.691 | 0.866 | 1.188 | 1.028 | 0.956 | 0.915 | 0.850 | |||
β4 | ASE | 0.538 | 0.805 | 1.705 | 0.016 | 0.070 | 0.177 | 1.523 | 1.723 | 1.570 | ||
MSE | 0.095 | 0.295 | 0.204 | 0.035 | 0.025 | 0.345 | 0.120 | 0.272 | 0.332 | |||
RR | 1.437 | 1.522 | 1.003 | 0.681 | 0.733 | 0.824 | 1.184 | 0.685 | 1.208 | |||
σ | ASE | 0.000 | 0.000 | 0.000 | 0.140 | 0.205 | 0.494 | 0.002 | 0.001 | 0.002 | ||
MSE | 0.143 | 0.143 | 0.143 | 0.007 | 0.008 | 0.025 | 0.143 | 0.143 | 0.143 | |||
Ω1 | ASE | 0.518 | 0.419 | 0.416 | 0.047 | 0.056 | 0.244 | 0.513 | 0.343 | 0.692 | ||
MSE | 0.062 | 0.102 | 0.079 | 0.018 | 0.029 | 0.031 | 0.111 | 0.040 | 0.039 | |||
Ω2 | ASE | 0.493 | 0.789 | 0.956 | 0.420 | 0.252 | 0.216 | 0.552 | 0.964 | 0.814 | ||
MSE | 0.158 | 0.078 | 0.300 | 0.045 | 0.117 | 0.109 | 0.194 | 0.415 | 0.121 |
Parameters | Measures | N-NLMM | SMSN-NLMM | SNP-NLMM | ||||||||
10% | 20% | 50% | 10% | 20% | 50% | 10% | 20% | 50% | ||||
β1 | ASE | 0.696 | 1.695 | 3.906 | 0.084 | 0.571 | 2.465 | 1.358 | 1.819 | 3.967 | ||
MSE | 0.428 | 1.709 | 4.667 | 0.196 | 0.186 | 0.349 | 0.286 | 0.697 | 3.105 | |||
RR | -0.025 | -0.768 | -1.605 | 0.297 | 0.416 | 0.320 | 0.293 | -0.148 | -0.539 | |||
β2 | ASE | 0.011 | 0.101 | 0.054 | 0.027 | 0.037 | 0.099 | 0.108 | 0.083 | 0.350 | ||
MSE | 0.098 | 0.182 | 0.483 | 0.098 | 0.193 | 0.515 | 0.088 | 0.177 | 0.381 | |||
RR | 1.928 | 2.252 | 3.053 | 1.929 | 2.298 | 3.121 | 1.857 | 2.244 | 2.782 | |||
β3 | ASE | 0.198 | 0.904 | 2.110 | 0.037 | 0.094 | 1.060 | 1.153 | 1.609 | 2.271 | ||
MSE | 0.184 | 0.338 | 0.484 | 0.206 | 0.395 | 0.093 | 0.040 | 0.170 | 0.022 | |||
RR | 1.461 | 0.739 | 0.493 | 1.496 | 1.686 | 1.231 | 1.111 | 0.802 | 0.943 | |||
β4 | ASE | 0.741 | 1.046 | 1.715 | 0.026 | 0.108 | 0.454 | 1.385 | 1.366 | 2.012 | ||
MSE | 0.495 | 0.567 | 0.316 | 0.158 | 0.279 | 0.743 | 0.196 | 0.559 | 0.055 | |||
RR | 0.298 | 0.322 | 0.541 | 0.326 | 0.111 | -0.346 | 0.889 | 0.408 | 1.038 | |||
σ | ASE | 0.000 | 0.000 | 0.000 | 0.302 | 0.217 | 0.325 | 0.001 | 0.001 | 0.000 | ||
MSE | 0.143 | 0.143 | 0.143 | 0.005 | 0.001 | 0.053 | 0.143 | 0.1435 | 0.143 | |||
Ω1 | ASE | 0.590 | 0.496 | 0.397 | 0.270 | 0.114 | 0.218 | 0.542 | 0.532 | 0.137 | ||
MSE | 0.449 | 0.067 | 0.043 | 0.013 | 0.014 | 0.020 | 0.013 | 0.077 | 0.024 | |||
Ω2 | ASE | 0.504 | 0.621 | 0.905 | 0.368 | 0.433 | 0.235 | 0.961 | 0.795 | 1.192 | ||
MSE | 0.503 | 0.834 | 0.509 | 0.195 | 0.154 | 0.131 | 0.408 | 0.573 | 0.235 |
Parameters | Measures | N-NLMM | SMSN-NLMM | SNP-NLMM | ||||||||
10% | 20% | 50% | 10% | 20% | 50% | 10% | 20% | 50% | ||||
β1 | ASE | 0.418 | 1.608 | 3.890 | 0.068 | 0.406 | 1.160 | 0.957 | 1.166 | 1.516 | ||
MSE | 1.081 | 1.219 | 7.045 | 0.515 | 0.895 | 1.752 | 0.560 | 1.384 | 0.290 | |||
RR | -0.565 | -0.643 | -2.107 | -0.145 | -0.496 | -0.736 | -0.003 | -0.508 | -0.886 | |||
β2 | ASE | 0.005 | 0.093 | 0.091 | 0.026 | 0.037 | 0.173 | 0.106 | 0.190 | 0.804 | ||
MSE | 0.267 | 0.519 | 1.374 | 0.262 | 0.529 | 1.464 | 0.214 | 0.419 | 1.101 | |||
RR | 2.528 | 3.125 | 4.462 | 2.513 | 3.150 | 4.568 | 2.347 | 2.827 | 3.973 | |||
β3 | ASE | 0.030 | 1.704 | 2.018 | 0.064 | 0.189 | 1.318 | 1.201 | 1.087 | 1.584 | ||
MSE | 0.633 | 0.235 | 0.524 | 0.694 | 1.387 | 1.102 | 0.182 | 0.669 | 0.289 | |||
RR | 1.870 | 1.363 | 0.542 | 1.911 | 2.287 | 1.670 | 1.143 | 0.541 | 1.319 | |||
β4 | ASE | 0.335 | 1.072 | 1.154 | 0.060 | 0.388 | 0.851 | 1.177 | 0.783 | 1.521 | ||
MSE | 0.741 | 0.670 | 0.659 | 0.448 | 0.930 | 0.982 | 0.442 | 0.868 | 0.304 | |||
RR | -0.192 | 0.209 | 0.314 | -0.132 | -0.629 | -0.614 | 0.375 | -0.018 | 1.354 | |||
σ | ASE | 0.000 | 0.000 | 0.000 | 0.368 | 0.331 | 0.174 | 0.001 | 0.001 | 0.001 | ||
MSE | 0.143 | 0.143 | 0.143 | 0.012 | 0.011 | 0.016 | 0.143 | 0.143 | 0.143 | |||
Ω1 | ASE | 0.255 | 1.211 | 0.442 | 0.712 | 0.701 | 0.372 | 0.849 | 0.464 | 0.449 | ||
MSE | 1.026 | 0.274 | 0.074 | 0.051 | 0.023 | 0.018 | 0.183 | 0.023 | 0.050 | |||
Ω2 | ASE | 0.083 | 0.656 | 0.535 | 0.365 | 0.409 | 0.301 | 0.577 | 0.508 | 0.849 | ||
MSE | 0.817 | 0.820 | 0.659 | 0.092 | 0.235 | 0.104 | 0.663 | 0.830 | 0.181 |
Models | . | 1SD10 | 1SD20 | 1SD50 | |||||||||||
β1 | β2 | β3 | β4 | β1 | β2 | β3 | β4 | β1 | β2 | β3 | β4 | ||||
N-NLMM | RR | 0.32 | 1.27 | 0.72 | 0.98 | -0.09 | 1.49 | 0.79 | 0.82 | -0.83 | 2.20 | 0.85 | 0.87 | ||
MSE | 0.28 | 0.01 | 0.10 | 0.26 | 0.53 | 0.03 | 0.09 | 0.18 | 2.68 | 0.18 | 0.32 | 0.32 | |||
AET | 31.99 | 32.12 | 22.42 | ||||||||||||
SMSN-NLMM | RR | 0.90 | 1.33 | 0.86 | 0.66 | 1.51 | 1.51 | 1.02 | 0.55 | 0.88 | 1.86 | 0.97 | 0.12 | ||
MSE | 0.01 | 0.01 | 0.02 | 0.04 | 0.12 | 0.03 | 0.01 | 0.08 | 0.59 | 0.08 | 0.02 | 0.34 | |||
AET | 57.71 | 55.72 | 56.67 | ||||||||||||
SNP-NLMM | RR | 0.88 | 1.67 | 1.06 | 1.36 | 1.03 | 1.79 | 1.19 | 1.35 | 0.89 | 2.08 | 1.11 | 1.40 | ||
MSE | 0.07 | 0.06 | 0.08 | 0.17 | 0.01 | 0.08 | 0.09 | 0.12 | 0.08 | 0.14 | 0.12 | 0.19 | |||
AET | 39.35 | 34.67 | 38.08 |
Cluster | ϕ1 | ϕ2 | ϕ3 | ϕ4 | TEC | EPT | R0 | First case |
Benin | 7.377 | 0.410 | 0.022 | 4.250 | 3846 | 105 | 1.167 | 16/03/2020 |
Burkina Faso | 0.389 | 0.015 | 4798 | 153 | 1.115 | 10/03/2020 | ||
Guinea | 0.327 | 0.020 | 10,111 | 127 | 1.150 | 13/03/2020 | ||
Ivory-Coast | 0.292 | 0.024 | 16,297 | 110 | 1.840 | 11/03/2020 | ||
Mali | 0.400 | 0.031 | 4255 | 74 | 1.249 | 25/03/2020 | ||
Niger | 0.448 | 0.025 | 2644 | 90 | 1.890 | 20/03/2020 | ||
Senegal | 0.291 | 0.023 | 16,607 | 118 | 1.172 | 02/03/2020 |