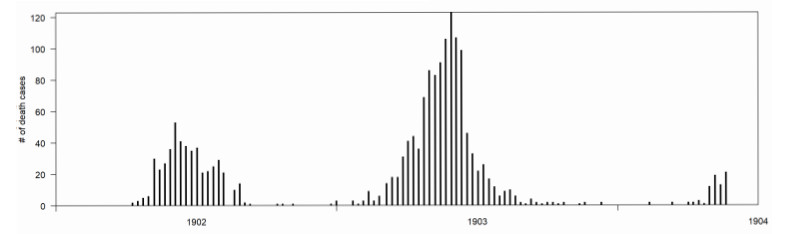
Identifying epidemic-driving factors through epidemiological modeling is a crucial public health strategy that has substantial policy implications for control and prevention initiatives. In this study, we employ dynamic modeling to investigate the transmission dynamics of pneumonic plague epidemics in Hong Kong from 1902 to 1904. Through the integration of human, flea, and rodent populations, we analyze the long-term changing trends and identify the epidemic-driving factors that influence pneumonic plague outbreaks. We examine the dynamics of the model and derive epidemic metrics, such as reproduction numbers, that are used to assess the effectiveness of intervention. By fitting our model to historical pneumonic plague data, we accurately capture the incidence curves observed during the epidemic periods, which reveals some crucial insights into the dynamics of pneumonic plague transmission by identifying the epidemic driving factors and quantities such as the lifespan of flea vectors, the rate of rodent spread, as well as demographic parameters. We emphasize that effective control measures must be prioritized for the elimination of fleas and rodent vectors to mitigate future plague outbreaks. These findings underscore the significance of proactive intervention strategies in managing infectious diseases and informing public health policies.
Citation: Salihu S. Musa, Shi Zhao, Winnie Mkandawire, Andrés Colubri, Daihai He. An epidemiological modeling investigation of the long-term changing dynamics of the plague epidemics in Hong Kong[J]. Mathematical Biosciences and Engineering, 2024, 21(10): 7435-7453. doi: 10.3934/mbe.2024327
[1] | Fangyuan Chen, Rong Yuan . Reconsideration of the plague transmission in perspective of multi-host zoonotic disease model with interspecific interaction. Mathematical Biosciences and Engineering, 2020, 17(5): 4422-4442. doi: 10.3934/mbe.2020244 |
[2] | Abdelheq Mezouaghi, Salih Djillali, Anwar Zeb, Kottakkaran Sooppy Nisar . Global proprieties of a delayed epidemic model with partial susceptible protection. Mathematical Biosciences and Engineering, 2022, 19(1): 209-224. doi: 10.3934/mbe.2022011 |
[3] | Quentin Griette, Jacques Demongeot, Pierre Magal . What can we learn from COVID-19 data by using epidemic models with unidentified infectious cases?. Mathematical Biosciences and Engineering, 2022, 19(1): 537-594. doi: 10.3934/mbe.2022025 |
[4] | Benjamin H. Singer, Denise E. Kirschner . Influence of backward bifurcation on interpretation of $R_0$ in a model of epidemic tuberculosis with reinfection. Mathematical Biosciences and Engineering, 2004, 1(1): 81-93. doi: 10.3934/mbe.2004.1.81 |
[5] | Yukihiko Nakata, Ryosuke Omori . The change of susceptibility following infection can induce failure to predict outbreak potential by $\mathcal{R}_{0}$. Mathematical Biosciences and Engineering, 2019, 16(2): 813-830. doi: 10.3934/mbe.2019038 |
[6] | Timothy C. Reluga, Jan Medlock, Alison Galvani . The discounted reproductive number for epidemiology. Mathematical Biosciences and Engineering, 2009, 6(2): 377-393. doi: 10.3934/mbe.2009.6.377 |
[7] | Oren Barnea, Rami Yaari, Guy Katriel, Lewi Stone . Modelling seasonal influenza in Israel. Mathematical Biosciences and Engineering, 2011, 8(2): 561-573. doi: 10.3934/mbe.2011.8.561 |
[8] | Avery Meiksin . Using the SEIR model to constrain the role of contaminated fomites in spreading an epidemic: An application to COVID-19 in the UK. Mathematical Biosciences and Engineering, 2022, 19(4): 3564-3590. doi: 10.3934/mbe.2022164 |
[9] | F. S. Vannucchi, S. Boccaletti . Chaotic spreading of epidemics in complex networks of excitable units. Mathematical Biosciences and Engineering, 2004, 1(1): 49-55. doi: 10.3934/mbe.2004.1.49 |
[10] | Tailei Zhang, Hui Li, Na Xie, Wenhui Fu, Kai Wang, Xiongjie Ding . Mathematical analysis and simulation of a Hepatitis B model with time delay: A case study for Xinjiang, China. Mathematical Biosciences and Engineering, 2020, 17(2): 1757-1775. doi: 10.3934/mbe.2020092 |
Identifying epidemic-driving factors through epidemiological modeling is a crucial public health strategy that has substantial policy implications for control and prevention initiatives. In this study, we employ dynamic modeling to investigate the transmission dynamics of pneumonic plague epidemics in Hong Kong from 1902 to 1904. Through the integration of human, flea, and rodent populations, we analyze the long-term changing trends and identify the epidemic-driving factors that influence pneumonic plague outbreaks. We examine the dynamics of the model and derive epidemic metrics, such as reproduction numbers, that are used to assess the effectiveness of intervention. By fitting our model to historical pneumonic plague data, we accurately capture the incidence curves observed during the epidemic periods, which reveals some crucial insights into the dynamics of pneumonic plague transmission by identifying the epidemic driving factors and quantities such as the lifespan of flea vectors, the rate of rodent spread, as well as demographic parameters. We emphasize that effective control measures must be prioritized for the elimination of fleas and rodent vectors to mitigate future plague outbreaks. These findings underscore the significance of proactive intervention strategies in managing infectious diseases and informing public health policies.
Plague is a zoonotic bacterial disease caused by the bacterium Yersinia pestis (or Y. pestis, formerly known as Pasteurella pestis) that affects humans and other mammals [1,2]. The disease is described as a disastrous cause of the black death that transpired around the middle of the 13th century and caused the death of millions of humans [1,2,3]. In recent years, major outbreaks occurred between 2010 and 2015 and affected over 3240 individuals, including more than 600 deaths [1]. The disease is endemic in Africa, Asia, South America, and Europe, with Peru, Madagascar, and the Democratic Republic of the Congo as the most affected countries, recently [1].
Plague is a zoonotic disease usually found in small mammals and their fleas and transmits between animals [4,5,6,7]. Humans can also be infected either by the bite of infected vector fleas, contact with infected items (or body fluids), or the intake of infected respiratory droplets [1,4]. The early 20th-century plague epidemics in Hong Kong were part of a global epidemic, with the Bombay outbreak in the 1890s identifying fleas as vectors for Yersinia pestis [4,8]. This identification was essential for developing effective strategies for plague prevention and management by targeting flea vectors and their rodent hosts. Plague can be a severe disease in humans, with a case-fatality ratio (CFR) ranging between 30–60% for the bubonic type and most fatal for the pneumonic type, especially when left untreated [1,2]. Until now, there has been no specific vaccine against plague infection. However, effective treatment against the disease's infection is currently available [1].
The third historically-recorded pandemic in China was introduced through Yunnan city in the 1850s and spread widely for the next few decades; however, it reached pandemic proportions in Hong Kong in 1894, killing over 2500 people and forcing the evacuation of roughly one-third of the city's population [3]. At various times from 1894 to 1904, the disease was reintroduced to the city via marine trade, killing thousands of people [3,9]. At the turn of the 20th century, most research on plague transmission involving rats, fleas, and humans was conducted by European and Japanese scientists in Hong Kong or using Hong Kong data [3,4,5,10] with emphasis on analyzing the correlation between rat infestations and plague and the many types of plague in rats [3,4].
In past centuries, plague dynamics have shifted dramatically between pandemics and years of relative inactivity. The 14th-century Black Death, a horrific pandemic that raced throughout Europe, serves as a stark illustration of the explosive nature of plague dynamics [11]. Thus, exploring long-term changing dynamics in plague epidemics could offer an intriguing insight into the intricate interaction of infectious agents, hosts, and the environment over prolonged durations.
Several epidemic modeling studies have been utilized to explore the dynamics of plague and related disease transmission (see, for instance, [4,5,6,7,12,13,14,15,16,17] and the references therein). In particular, Dean et al. [4] investigated the dynamics of plague transmission in Europe during the during the Black Death. They found that human ectoparasites, like body lice and human fleas, were more likely to have caused the rapidly developing epidemics than rats, especially in pre-industrial Europe. They also revealed that ectoparasite infections transpire with high infectivity in humans, which was in line with an experimental study by Boegler et al. [14]. Keeling et al. [5] employed a mechanistic model to study the transmission dynamics of bubonic plague in human, rat, and flea populations. They used sensitivity analysis to find some important parameters that are needed to control the plague. These include the equilibrium number of rat cases and the rate of infection during the first epizootic cycle. According to their study, they suggested that relatively small rodent populations can cause severe plague epidemics, which highlighted that timely intervention for vector control is a significant strategy. Nguyen et al. [6] studied the transmission dynamics of bubonic and pneumonic plague in Madagascar. They found that the estimated transmission rate from person to person was higher than the flea-to-human transmission rate. This further showed that person-to-person transmission should also be prioritized to effectively mitigate plague outbreaks.
In this study, we aim to extend previous research, such as those considered in [4,5], by incorporating demographic information, including birth and death rates, to enhance our understanding of the dynamics of plague epidemics in Hong Kong during the 1902–1904 outbreaks. Accurate modeling of disease transmission, especially over extended outbreak periods, is crucial for predicting disease spread and burden. Incorrect estimates of key epidemiological quantities, such as reproduction numbers and serial intervals, can lead to inaccurate predictions and responses to epidemics and pandemics. Our model considers the interplay of human, flea, and rodent populations to infer the long-term changing dynamics of the plague epidemic in Hong Kong. By fitting the model to historical plague mortality data from 1902 to 1904, we aim to elucidate the underlying drivers of the dynamics and identify effective measures for future epidemic preparedness. Our findings contribute to a deeper understanding of plague dynamics and inform strategies for mitigating the impact of future outbreaks. The paper is arranged as follows: the epidemic model is presented in Sections 2 and analyzed in Section 3 and sensitivity analysis are conducted in Section 4 brief discussion of results is provided in Section 5.
In this study, we analyzed the data for plague-induced mortality during nine outbreaks in Hong Kong between 1902 and 1904 [18]. The data were made available to the public through secondary sources, including published articles, books, and government reports. We digitized the data from these sources to cover the entire outbreak period. Unlike other historical records, such as the Eyam report, which exhibited two fatality peaks, the Hong Kong data provided a continuous record of weekly mortality figures. This allowed us to model the entire epidemic period more comprehensively. Figure 1 shows the plague weekly time series cases from the surveillance data for Hong Kong between 1902 and 1904 using the data obtained from [18].
The effective reproduction number (Reff) is an important epidemiological metric that measures the average number of secondary cases caused by an infectious individual in a partially susceptible population. In Figure 2, we estimate Reff based on the provided case data and utilize the serial interval (the time between successive cases in a transmission chain) for pneumonic plague, which was estimated from earlier studies to be around 2 to 4 days [19,20]. These estimations provide insights into the transmission potential of plague over time, incorporating uncertainty and smoothing to enhance clarity as well as aid in developing effective interventions.
In Figure 3, the time series spatial analysis illustrates the transmission dynamics of plague in Kowloon and Hong Kong Island during the 1902–1904 epidemics. Both locations exhibited similar transmission patterns, with rodents playing a significant role in disease spread. The spatial distribution of the plague outbreak significantly impacted its transmission across different geographical regions in Hong Kong. These patterns likely arose from a combination of factors, including human ecological conditions, migration, and vector dynamics. Plague transmission often propagated through trade routes, human movement, and environmental factors, leading to localized and clustered outbreaks. Understanding these spatial patterns is crucial for developing effective surveillance, control strategies, and forecasting future epidemics. By identifying these patterns, targeted interventions can be devised to curb disease transmission and protect vulnerable populations. These findings highlight the importance of incorporating spatial analysis into epidemiological modeling efforts to derive actionable insights that inform public health strategies. To further explore the spatial heterogeneity of plague transmission, identify high-risk areas, and uncover the key drivers of disease dynamics, future work will incorporate advanced spatial modeling techniques. This approach aims to enhance our ability to design effective mitigation plans and targeted interventions tailored to specific geographic settings, thereby improving our capacity to prevent and manage plague outbreaks and strengthen preparedness against unexpected epidemics or pandemics.
We employed a dynamic model to investigate the long-term changing dynamics of plague epidemics in Hong Kong from 1902 to 1904, drawing on insights from previous studies [4,5,15]. Our model, derived from [4], explores the interplay of human, flea, and rodent populations and incorporates demographic factors to infer shifts in plague epidemics while considering the population's homogeneous nature and the random distribution of vectors. Specifically, we extended the standard susceptible-exposed-infectious-removed (SEIR) epidemic model by integrating demographic information in humans and incorporating the logistic growth rate in the rodent population, a novel approach for analyzing Hong Kong's plague dynamics. This modification is particularly relevant for studying the relatively long period, during which demographic parameters play a crucial role in understanding plague transmission dynamics.
It is also critical to understand that the model considers different transmission pathways, rat to human and rat to rat via flea vector. Although the compartment of the flea vector was not explicitly present in the model, it is assumed to be included mechanistically as a reservoir that transfers the plague disease from dead rats to healthy rats or humans [4], we also noted that the higher the number of fleas in the population, the more plague infection. The ratio βrβh represents the rate of transmission from infected deceased rat (infected-dead rat or rat carcass) to susceptible rat and from infected rat carcass to susceptible humans, which is given by [4] based on the parameter values from Table 2. According to previous reports [15], the biting rate of an infectious flea is approximately 5 times more likely to cause an infection in susceptible rats than in susceptible humans. For more detail regarding the rat-flea model" formulation, see [4]. The total human population at time t, represented by Nh(t), is split into sub-compartments of susceptible, Sh(t), infected, Ih(t), recovered, Rh(t), and plagued-deceased, Dh(t), humans, such that
Nh(t)=Sh(t)+Ih(t)+Rh(t)+Dh. |
The total flea (vector) population at time t, represented by Nf(t), is split into two compartments: the average number of fleas living on a rat (Hf(t)) and the number of free infectious fleas (Ff(t)) that are searching for a host. Hence, we have
Nf(t)=Hf(t)+Ff(t). |
The total rat population at time t, represented by Nr(t), is divided into sub-compartments of susceptible Sr(t), infected Ir(t), recovered, Rr(t), and plague deceased, Dr(t), rats. Thus,
Nr(t)=Sr(t)+Ir(t)+Rr(t)+Dr. |
These definitions and the incorporation of demographic parameters allow for a comprehensive analysis of plague dynamics over the studied period. For more detailed information regarding the rat-flea model formulation, please refer to [4].
The plague model is epidemiologically described in Eq (2.1). The state variables and parameters listed in Tables 1 and 2, respectively, all of which are assumed to be positive, satisfy the next set of non-linear ordinary differential equations (ODEs).
dShdt=πh−βhShFfNh(e−aNr)−μhSh,dIhdt=βhShFfNh(e−aNr)−(γh+μh)Ih,dRhdt=ghγhIh−μhRh,dDhdt=(1−gh)γhIh,dHfdt=rfHf(1−HfKf)−μfHf,dFfdt=(μr+(1−gr)γr)IrHf−μfFf,dSrdt=μrNr−βrSrFfNr(1−e−aNr)−μrSr,dIrdt=βrSrFfNr(1−e−aNr)−(γr+μr)Ir,dRrdt=grγrIr−μrRr,dDrdt=(1−gr)γrIr. | (2.1) |
Variable | Interpretation |
Nh | Total population of humans |
Sh | Susceptible humans with risk of plague infection |
Ih | Infected humans with symptoms of plague infection |
Rh | Recovered humans |
Dh | Plague deceased-humans |
Nf | Total population of fleas |
Hf | Average number of fleas living on a rat |
Ff | Number of free infectious fleas searching for a host |
Nr | Total population of rats |
Sr | Susceptible rats |
Ir | Plague-infected rats |
Rr | Recovered rats |
Dr | Plague deceased-rats |
Parameter | Notation | Baseline value | Remark/Unit | Source(s) |
Transmission rate for humans | βh | 0.1001(0.01−0.2) | variable | [4] |
Transmission rate for rodents | βr | 0.5001(0.02−0.75) | variable | [4] |
Average infectious period in humans | 1/γh | 10(2.38−66.67) | day | [4] |
Average infectious period in rats | 1/γr | 5.2(1.59−100) | day | [4] |
Humans recovery rate | gh | 0.4(0.01−0.8) | dimensionless | [4] |
Rats recovery rate | gr | 0.1(0.01−0.5) | dimensionless | [4] |
Flea growth rate | rf | 0.0084(0.0001−0.05) | day−1 | [5] |
Fleas carrying capacity | Kf | 6(3.29−11.17) | dimensionless | [4,5] |
Flea searching efficiency | a | 0.004(0−1) | dimensionless | [5] |
Natural death rate of fleas | 1/μf | 5.0(3.23−100) | day | [4] |
Natural death rate of rats | 1/μr | 5.0(4.0−50.0) | day | [5] |
Natural death rate of humans | 1/μh | 1/(35×365)(1/(32×365)−1/(45×365)) | day−1 | [32,33] |
Recruitment rate of humans | πh | 42.27(32.88−46.23) | person \cdot day−1 | [32,33] |
It is worth mentioning that the susceptible flea population follows a logistic growth and is determined by the intrinsic growth rates, rfHf(1−HfKf). To further gain more epidemiological insight into the plague dynamics, we computed a basic reproduction number (R0), a key epidemiological metric that indicates the average number of secondary infections produced by one infected individual in a completely susceptible population It is used in evaluating disease transmission dynamics. For more details regarding R0 computation, see Appendix A.
Following previous approaches [21,22,23,24,25,26,27,28,29], we modeled the reported plague mortality cases in Hong Kong from 1902 to 1904 as a partially observed Markov process. The weekly reported cases, Ψi, were assumed to follow an over-dispersed Poisson process, modeled using a negative binomial (NB) distribution:
Ψi∼NB(mean=Γi,variance=Γi(1+τΓi)), |
where τ is an over-dispersion parameter to be estimated. The likelihood function for the i-th week of the j-th year, Li,j(⋅), represents the probability of the observed number of cases given the simulated cases Γi.
The overall log-likelihood, l, for the time series is:
l(Θ)=3∑j=1Φ∑i=1ln[Li,j(Ψi,j∣Ψ0,j,…,Ψi−1,j;Υ)], |
where Φ is the total number of weeks in an epidemic wave, and Υ represents the parameter vector to be estimated. We used iterated filtering and plug-and-play likelihood-based inference to find the maximum likelihood estimates (MLE) of Υ, implemented using the POMP package in R [30]. A fixed-time-step Euler-multinomial algorithm was used to simulate the ODE system.
To compare model performances, we used the Bayesian information criterion (BIC):
BIC=−2ln(ˆΥ)+Vln(U), |
where U is the number of data points, V is the number of parameters, and ˆΥ denotes the estimated parameters [31].
For the fitting process, we calibrated the model using both human and rodent mortality data from 1902 to 1904. Although the human and rodent epidemics exhibited similar temporal trends, they differed in magnitude. To account for seasonal variations, we employed a cubic spline approach. The number of nodes in the cubic spline, nm, was varied to find the optimal value that minimized the BIC. This approach allowed us to reconstruct the Γi series, revealing that the 1902–1904 plague dynamics in human and rodent populations shared similar patterns with seasonal variations. It is important to note that while seasonal variations were accounted for, explicit fluctuations in rodent population size were not modeled directly [27].
In Figure 4, we present the model-fitting results for plague-induced mortalities in Hong Kong between 1902 and 1904, encompassing both human and rodent populations. Using biologically plausible parameters, our epidemic model effectively captures the temporal patterns of the plague epidemics during this period. The fitting results closely align with the surveillance data, indicating that our model can accurately assess the transmission dynamics of the plague in Hong Kong and potentially in other regions. The parameters used in the model are detailed in Table 2.
To account for seasonal variations in the transmission factor and rodent susceptibility (which refers to the likelihood of rodents becoming infected when exposed to Yersinia pestis, influencing disease spread among the rodent population), we employed a cubic spline function with uniformly distributed nodes over the study period. The inset panels in Figure 4(a) display the profiles as functions of the number of nodes (nm). The optimal model, with the smallest BIC, was achieved with nm=7. Consequently, the main panels show the model fitting results with nm=7.
Figure 4 demonstrates that our model fits well with the reported plague cases from March 1902 to June 1904. The transmission factor (R0(t)) and rodent susceptibility are time-dependent, as depicted by the right vertical axis for the human and rodent populations, respectively. The outbreak began around April 1902, peaked in July, and showed a declining trend, reaching control by June 1904.
These findings have significant public health implications. The accurate fit of the model underscores the importance of considering both human and rodent populations in understanding plague dynamics. The seasonal fluctuations indicate that increased rodent activity and elevated transmission rates during certain periods contribute to the epidemic's peaks. From a public health perspective, this implies that interventions such as rodent control, flea eradication, and public health campaigns should be intensified during late spring and early summer when the risk is highest. By incorporating seasonal dynamics into the model, public health authorities can better predict periods of increased risk and allocate resources more efficiently, leading to more timely and effective interventions. Implementing this preventive strategy can reduce the incidence of plague and mitigate its impact on public health [27]. Overall, our investigation highlights the importance of integrated strategies for pest control, continuous surveillance, and adaptable public health measures to effectively control and prevent plague outbreaks.
As illustrated in Figure 5(a)–(c), our numerical simulations of the contour plots have uncovered a significant correlation between the R0 and certain crucial epidemic parameters generated from the model. The observed trend indicates that an increase in both the transmission rate from rodents to humans (βr) and the flea death rate (μf) leads to a substantial rise in R0 (see Figure 5. Similarly, in Figure 4(b), we found that an increase in βr and the rodent death rate (μr) causes an increase in R0, consequently increasing the epidemic's impact. However, Figure 5(c) revealed that an increase in μr and μf causes a decrease in R0. This further demonstrates the critical epidemiological metric of these parameters in relation to R0, which serves as an indicator of the potential for the plague to cause a serious epidemic. The results signify the influence of the transmission rate or contact rate of the disease, especially from rodents, fueling the likelihood of disease transmission per contact, logically contributing to an elevated R0. Therefore, the observed increase in R0 with rising βr and μf, or βr and μr, as well as the decrease in R0 with rising μr and μf, suggests a complex interplay between these epidemic parameters and the transmissibility of the plague in Hong Kong.
In this subsection, we performed a sensitivity analysis to determine the most sensitive parameter that should be prioritized in curtailing the spread of plague epidemics. We obtained the partial rank correlation coefficient (PRCC) for the sensitivity analysis by employing the same technique from previous studies [21,22,34,35]. In Figure 6, we show the results of the PRCC of the sensitivity analysis, which reveals key controllable epidemic parameters of the model (2.1). According to our results, the death rate of the flea vector (μf) and infectious period in rats (γr) are the most sensitive parameters of the model that need emphasis for effective control of plague outbreaks. The PRCC of the basic reproduction number, R0, was depicted in Figure 6 with the estimated parameters from the Table 2. The results further reveal that eliminating the plague vector by all means (e.g., adopting vector-borne disease control measures, such as the use of insecticides to kill the flea vector) is significant in mitigating plague epidemics.
Infectious disease modeling has played a crucial role in understanding epidemics, providing an effective way of examining disease transmission and assessing disease control and prevention strategies [6]. Plague is described as a notorious and calamitous disease that affects humans and mammals [4]. We employed a deterministic model that includes human, flea, and rodent populations, incorporating demographic information to explore the dynamics of plague transmission in Hong Kong during the 1902–1904 outbreaks. The model was analyzed and fitted using plague mortality data from Hong Kong from 1902 to 1904. Our investigation into the long-term impact of the changing dynamics during epidemics revealed intriguing insights into the complex interactions that occur over extended periods between infectious organisms, hosts, and the environment. We highlighted that similar future outbreaks could be accurately analyzed using the same model framework.
To accurately capture the transmission dynamics of the plague during the 1902–1904 epidemic in Hong Kong, we utilized both reported human mortality data and raw rodent mortality data as part of our model fitting process. The human mortality data, systematically collected from the streets by public health authorities, provided reliable weekly counts of fatalities. In contrast, the rodent mortality data, though raw and less precise by contemporary standards, were gathered through systematic searches and inspections conducted during the outbreak. By employing a cubic spline function, we were able to calibrate our model using these historical records, which provided robust estimates of rodent mortality trends in relation to human cases. It is important to note that the spline function modeled seasonal trends rather than explicit fluctuations in rodent population size. This approach aligns with established methodologies in historical epidemiological modeling, where the integration of reconstructed data plays a crucial role in understanding epidemic dynamics [4,5]. The inclusion of both human and rodent data allowed us to derive meaningful insights into the interaction between rodent populations and the plague pathogen, thereby reinforcing the validity of our findings and their implications for public health interventions.
In Figure 4, we demonstrated the correlation between the model and the reported cases of the plague epidemics in Hong Kong, spanning from April 1902 to June 1904. From the model fitting results, we assessed the dynamics of human and rodent populations using a time-varying transmission component and the susceptibility of the rodent population. Our results suggest that the reported epidemics were driven by a combination of human and rodent outbreaks, with a transmission rate that varied over time between fleas and humans, fleas and rodents, and vice versa. The results also indicate that the peak of plague epidemics in Hong Kong occurred during the midyear. Additionally, we found that the human transmission factor reached its maximum level between April and June annually, followed by a rapid decline. The susceptibility of rodents also peaked during the same period each year, then significantly decreased. Our findings provide a comprehensive overview of the annual occurrences of plague infection in both human and rodent populations. The escalating severity of the plague was likely due to a rise in the human transmission rate and heightened susceptibility of rodents each April during the 1902–1904 plague epidemic. We anticipate that transmission rates may be similar across different situations or regions.
However, additional data, especially concerning the rodent population (such as population size and the occurrence of illnesses), could be highly beneficial for further understanding the dynamics of the plague. According to our investigation, recurring trends in the data suggest that the fundamental causes of the plague epidemic may reflect similar tendencies throughout the outbreak period, potentially due to seasonal factors causing fluctuations as a result of changing conditions. We aim to explore more dynamics related to seasonal effects in future work, as understanding these effects is also crucial in understanding plague dynamics [36].
The proposed approach exhibits comparable goodness-of-fit in terms of log-likelihood values. The observed transmission among humans can be attributed to either an immediate outbreak among rodents, resulting in time-varying spillover, a consistent predominance of plague transmission, or a combination of both mechanisms. Our epidemiological data imply that plague transmission does not occur at a consistent rate; instead, it exhibits a punctuated equilibrium, with outbreaks happening in clusters followed by periods of silence. Several factors influence these fluctuating dynamics, including environmental circumstances, host immunity, genetic immunity evolution, and the presence of reservoirs and vectors [37].
Further numerical simulations were conducted, as depicted in Figures 5 and 6, to demonstrate the influence of key model parameters on overall plague transmission dynamics. These parameters include the mortality rate of flea vectors and the rate at which rodents transmit the disease. Our contour simulations (Figure 5) highlight the critical interplay between biological and ecological factors in driving plague epidemics. Our findings show that an increase in βr and a decrease in μf cause a substantial increase in R0 (see Figure 5(a)), indicating the need to target rodent-to-human transmission and manage flea populations. Interventions to reduce flea populations remain vital despite their high turnover. In Figure 5(b), the decrease in R0 with higher rodent mortality (μr) and a decrease in βr reveals that killing rodents might be a good strategy but may inadvertently increase human exposure to infected fleas, thereby emphasizing the need for integrated pest management strategies for effective control. Also, in Figure 5(c), the decrease in R0 with increasing μr and μf underscores the importance of rodent and flea longevity in sustaining the epidemic. These results highlight the necessity for comprehensive control measures to reduce both flea and rodent populations, informing proactive public health interventions to manage and prevent future plague outbreaks.
Furthermore, our sensitivity analysis results, presented in Figure 6, identify the death rate of the flea vector (μf) and the infectious period in rodents (γr) as the most critical parameters for controlling plague outbreaks. Targeting these parameters through interventions such as insecticide use is essential for effective epidemic control, highlighting the importance of vector management in public health strategies. Person-to-person transmission is crucial in the spread of a plague epidemic, particularly in cases of pneumonic plague, as illustrated in previous modeling studies. However, our present study primarily focuses on flea vector and rodent transmission dynamics relevant to the 1902–1904 plague epidemics in Hong Kong. In future studies, we intend to include this critical transmission mechanism (including person-to-person) to enhance the robustness and relevance of our model in both historical and contemporary contexts.
In summary, the main epidemiological findings from our study are as follows: (i) Estimation of the human transmission factor and rodent susceptibility during the plague epidemics in Hong Kong from 1902 to 1904. This finding highlights that the escalating severity of the plague was likely due to a rise in the human transmission rate and heightened susceptibility of rodents each April during the 1902–1904 plague epidemic. We anticipate that transmission rates may be similar across different regions. (ii) To our knowledge, no previous modeling study has analyzed plague transmission in Hong Kong using mortality data from 1902 to 1904 to identify the main transmission drivers during this period. Previous studies did not appropriately incorporate vital demographic factors, which are key to modeling the long-term dynamics of disease transmission. (iii) Additionally, through sensitivity analysis, we identified the key parameters that should be prioritized for effectively controlling plague transmission; this is crucial for future plague outbreaks and could provide valuable insights to public health practitioners and policymakers in designing suitable intervention measures. Furthermore, we also identified some crucial intervention measures that might be helpful for future epidemics.
Our investigation revealed that the long-term changing dynamics of plague epidemics reflect a complex interaction of variables that transcend temporal and spatial boundaries. Unraveling this complexity not only improves our understanding of historical pandemics but also enhances existing strategies for disease prevention and containment in a rapidly changing environment. Therefore, we emphasize, in particular, that controlling and eliminating flea and rodent vectors is crucial for preventing potential future plague outbreaks.
The authors declare they have not used Artificial Intelligence (AI) tools in the creation of this article. During the preparation of the final manuscript, the authors used grammar checker "QuillBot" in order to check and correct language errors and typos in the manuscript. After using this tool, the authors reviewed and edited the content as needed.
The authors are grateful to the handling editor and the anonymous reviewers for their invaluable comments and suggestions, which greatly contributed to improving the quality and clarity of our manuscript.
The authors declare that there is no conflict of interest. Daihai He is an Associate Editor for the journal but had no role in the handling, reviewing, or decision-making process for this manuscript in any capacity.
SSM and DH conceived the study and drafted the first manuscript. SSM, SZ, WM, AC, and DH carried out the investigation. All authors critically revised the manuscript and gave final approval for publication.
[1] | World Health Organization, Plague, Keyfacts, 2023. Available from: https://www.who.int/news-room/fact-sheets/detail/plague. |
[2] | Centers for Disease Control and Prevention, Plague, 2023. Available from: https://www.cdc.gov/plague/index.html. |
[3] | Statista, Number of deaths due to plague in Hong Kong during the Third Plague Pandemic from 1894 to 1902, 2023. Available from: https://www.statista.com/statistics/1115206/annual-plague-deaths-hong-kong-third-plague-pandemic/. |
[4] |
K. R. Dean, F. Krauer, L. Walløe, O. C. Lingjærde, B. Bramanti, N. Chr. Stenseth, et al., Human ectoparasites and the spread of plague in Europe during the Second Pandemic, Proc. Nat. Acad. Sci., 115 (2018), 1304–9. https://doi.org/10.1073/pnas.1715640115 doi: 10.1073/pnas.1715640115
![]() |
[5] |
M. J. Keeling, C. A. Gilligan, Bubonic plague: a metapopulation model of a zoonosis, Proc. R. Soc. Lond. B, 267 (2000), 2219–2230. https://doi.org/10.1098/rspb.2000.1272. doi: 10.1098/rspb.2000.1272
![]() |
[6] |
V. K. Nguyen, C. Parra-Rojas, E. A. Hernandez-Vargas, The 2017 plague outbreak in Madagascar: Data descriptions and epidemic modelling, Epidemics, 25 (2018), 20–25. https://doi.org/10.1016/j.epidem.2018.05.001 doi: 10.1016/j.epidem.2018.05.001
![]() |
[7] | World Health Organization, Plague manual: epidemiology, distribution, surveillance, and control, 1999. Available from: https://www.who.int/publications/i/item/WHO-CDS-CSR-EDC-99.2. |
[8] |
R. Yang, S. Atkinson, Z. Chen, Y. Cui, Z. Du, Y. Han, et al., Yersinia pestis and Plague: Some knowns and unknowns, Zoonoses (Burlington), 3 (2023), 5. https://doi.org/10.15212/zoonoses-2022-0040 doi: 10.15212/zoonoses-2022-0040
![]() |
[9] | Center for Health Protection, Hong Kong, Scientific committee on vector-borne diseases, situation of plague and prevention strategies, 2024. Available from: https://www.chp.gov.hk/files/pdf/diseases-situation_of_plague_and_prevention_strategie_r.pdf. |
[10] | E. H. Hankin, On the epidemiology of plague, Epidem. Infect., 5 (1905), 48–83. |
[11] |
R. Barbieri, M. Signoli, D. Chevé, C. Costedoat, S. Tzortzis, G. Aboudharam, et al., Yersinia pestis: the natural history of plague, Clin. Microbiol. Rev., 34 (2020), 10–128. https://doi.org/10.1128/CMR.00044-19 doi: 10.1128/CMR.00044-19
![]() |
[12] | R. J. Eisen, S. W. Bearden, A. P. Wilder, J. A. Montenieri, M. F. Antolin, K. L. Gage, Early-phase transmission of Yersinia pestis by unblocked fleas as a mechanism explaining rapidly spreading plague epizootics, PNAS, 103 (2006), 15380–15385. https://doi.org/10.1073pnas.0606831103/ |
[13] |
J. M. Girard, D. M. Wagner, A. J. Vogler, C. Keys, C. J. Allender, L. C. Drickamer, et al., Differential plague-transmission dynamics determine Yersinia pestis population genetic structure on local, regional, and global scales, PNAS, 101 (2004), 8408–8413. https://doi.org/10.1073/pnas.0401561101 doi: 10.1073/pnas.0401561101
![]() |
[14] |
K. A. Boegler, C. B. Graham, T. L. Johnson, J. A. Montenieri, R. J. Eisen, Infection prevalence, bacterial loads, and transmission efficiency in Oropsylla montana (Siphonaptera: Ceratophyllidae) one day after exposure to varying concentrations of Yersinia pestis in blood, J. Med. Entomol., 53 (2016), 674–680. https://doi.org/10.1093/jme/tjw004 doi: 10.1093/jme/tjw004
![]() |
[15] |
X. Didelot, L. K. Whittles, I. Hall, Model-based analysis of an outbreak of bubonic plague in Cairo in 1801, J. R. Soc. Interface, 14 (2017), 20170160. https://doi.org/10.1098/rsif.2017.0160 doi: 10.1098/rsif.2017.0160
![]() |
[16] |
S. Zhao, Z. Yang, S. S. Musa, J. Ran, M. K. C. Chong, M. Javanbakht, et al., Attach importance of the bootstrap t test against Student's t test in clinical epidemiology: a demonstrative comparison using COVID-19 as an example, Epidemiol. Infect., 149 (2021), e107. https://doi.org/10.1017/S0950268821001047 doi: 10.1017/S0950268821001047
![]() |
[17] |
K. R. Dean, F. Krauer, B. V. Schmid, Epidemiology of a bubonic plague outbreak in Glasgow, Scotland in 1900, R. Soc. open Sci., 6 (2019), 181695. https://doi.org/10.1098/rsos.181695 doi: 10.1098/rsos.181695
![]() |
[18] | W. Hunter, A Research into Epidemic and Epizootic Plague, Hong Kong: Noronha & Co., 1904. |
[19] |
H. Nishiura, M. Kakehashi, Real time estimation of reproduction numbers based on case notifications-Effective reproduction number of primary pneumonic plague, Trop. Med. Health, 33 (2005), 127–32. https://doi.org/10.2149/tmh.33.127 doi: 10.2149/tmh.33.127
![]() |
[20] | H. Nishiura, Backcalculation of the disease-age specific frequency of secondary transmission of primary pneumonic plague, preprint, arXiv: 0810.1606. |
[21] |
S. S. Musa, S. Zhao, D. Gao, Q. Lin, G. Chowell, D. He, Mechanistic modelling of the large-scale Lassa fever epidemics in Nigeria from 2016 to 2019, J. Theoret. Biol., 493 (2020), 110209. https://doi.org/10.1016/j.jtbi.2020.110209 doi: 10.1016/j.jtbi.2020.110209
![]() |
[22] |
S. Zhao, L. Stone, D. Gao, D. He, Modelling the large-scale yellow fever outbreak in Luanda, Angola, and the impact of vaccination, PLoS Neglet. Trop. Dis., 12 (2018), e0006158. https://doi.org/10.1371/journal.pntd.0006158 doi: 10.1371/journal.pntd.0006158
![]() |
[23] |
C. Breto, D. He, E. L. Ionides, A. A. King, Time series analysis via mechanistic models, Ann. Appl. Stat., 3 (2009), 319–348. http://dx.doi.org/10.1214/08-AOAS201 doi: 10.1214/08-AOAS201
![]() |
[24] | S. S. Musa, A. Tariq, L. Yuan, W. Haozhen, D. He, Infection fatality rate and infection attack rate of COVID-19 in South American countries, Infect. Dis. Poverty, 11 (2022). https://doi.org/10.1186/s40249-022-00961-5 |
[25] |
S. S. Musa, X. Wang, S. Zhao, S. Li, N. Hussaini, W. Wang, et al., The heterogeneous severity of COVID-19 in African countries: a modeling approach, Bull. Math. Biol., 84 (2022), 32. https://doi.org/10.1007/s11538-022-00992-x doi: 10.1007/s11538-022-00992-x
![]() |
[26] |
Q. Lin, Z. Lin, A. P. Y. Chiu, D. He, Seasonality of influenza A(H7N9) virus in China-fitting simple epidemic models to human cases, PLoS One, 11 (2016), e0151333. https://doi.org/10.1371/journal.pone.0151333 doi: 10.1371/journal.pone.0151333
![]() |
[27] |
D. He, E. L. Ionides, A. A. King, Plug-and-play inference for disease dynamics: measles in large and small populations as a case study, J. R. Soc. Interf., 7 (2010), 271–283. https://doi.org/10.1098/rsif.2009.0151 doi: 10.1098/rsif.2009.0151
![]() |
[28] |
D. He, S. Zhao, Q. Lin, S. S. Musa, L. Stone, New estimates of the Zika virus epidemic attack rate in Northeastern Brazil from 2015 to 2016: A modelling analysis based on Guillain-Barré Syndrome (GBS) surveillance data, PLoS Negl. Trop. Dis., 14 (2020), e0007502. https://doi.org/10.1371/journal.pntd.0007502 doi: 10.1371/journal.pntd.0007502
![]() |
[29] |
S. S. Musa, A. Tariq, L. Yuan, W. Haozhen, D. He, Infection fatality rate and infection attack rate of COVID-19 in South American countries, Infect. Dis. Poverty, 11 (2022), 42–52. https://doi.org/10.1186/s40249-022-00961-5 doi: 10.1186/s40249-022-00961-5
![]() |
[30] | Partially Observed Markov Process (POMP), The website of R package POMP: statistical inference for partially-observed Markov processes, 2018. Available from: https://kingaa.github.io/pomp/. |
[31] |
A. Camacho, S. Ballesteros, A. L. Graham, F. Carrat, O. Ratmann, B. Cazelles, Explaining rapid reinfections in multiple-wave influenza outbreaks: Tristan da Cunha 1971 epidemic as a case study, Proc. Biol. Sci., 278 (2011), 3635–3643. https://doi.org/10.1098/rspb.2011.0300 doi: 10.1098/rspb.2011.0300
![]() |
[32] | World Bank, World Bank data, Population, total (years) - Hong Kong SARS, China, 2020. Available from: https://data.worldbank.org/country/hong-kong-sar-china?view = chart. |
[33] | World Bank, World Bank data, Life expectancy at birth, total (years) - Hong Kong SAR, China, 2021. Available from: https://data.worldbank.org/indicator/SP.DYN.LE00.IN?locations = HK. |
[34] |
D. Gao, Y. Lou, D. He, T. C. Porco, Y. Kuang, G. Chowell, et al., Prevention and control of Zika as a mosquito-borne and sexually transmitted disease: a mathematical modeling analysis, Sci. Rep., 6 (2016), 28070. https://doi.org/10.1038/srep28070 doi: 10.1038/srep28070
![]() |
[35] |
D. He, S. Zhao, Q. Lin, S. S. Musa, L. Stone, New estimates of the Zika virus epidemic attack rate in Northeastern Brazil from 2015 to 2016: A modelling analysis based on Guillain-Barré Syndrome (GBS) surveillance data, PLoS Negl. Trop. Dis., 14 (2020), e0007502. https://doi.org/10.1371/journal.pntd.0007502 doi: 10.1371/journal.pntd.0007502
![]() |
[36] |
F. Krauer, H. Viljugrein, K. R. Dean, The influence of temperature on the seasonality of historical plague outbreaks, Proc. R. Soci. B., 288 (2021), 20202725. https://doi.org/10.1098/rspb.2020.2725 doi: 10.1098/rspb.2020.2725
![]() |
[37] |
J. Klunk, T. P. Vilgalys, C. E. Demeure, X. Cheng, M. Shiratori, J. Madej, et al., Evolution of immune genes is associated with the Black Death, Nature, 611 (2022), 312–319. https://doi.org/10.1038/s41586-022-05349-x doi: 10.1038/s41586-022-05349-x
![]() |
[38] |
P. van den Driessche, J. Watmough, Reproduction numbers and sub-threshold endemic equilibria for compartmental models of disease transmission, Math. Biosci., 180 (2002), 29–48, https://doi.org/10.1016/S0025-5564(02)00108-6 doi: 10.1016/S0025-5564(02)00108-6
![]() |
[39] |
O. Diekmann, J. Heesterbeek, J. Metz, On the definition and the computation of the basic reproduction ratio R0 in models for infectious diseases in heterogeneous populations, J. Math. Biol., 28 (1990), 365–382. https://doi.org/10.1007/BF00178324 doi: 10.1007/BF00178324
![]() |
[40] |
P. van den Driessche, Reproduction numbers of infectious disease models, Infect. Dis. Model., 2 (2017), 288–303. https://doi.org/10.1016/j.idm.2017.06.002 doi: 10.1016/j.idm.2017.06.002
![]() |
[41] |
S. S. Musa, S. Zhao, D. He, C. Liu, The long-term periodic patterns of global rabies epidemics among animals: A modeling analysis, Int. J. Bifur. Chaos, 30 (2020), 2050047. https://doi.org/10.1142/S0218127420500479 doi: 10.1142/S0218127420500479
![]() |
[42] |
S. S. Musa, S. Zhao, N. Hussaini, S. Usaini, D. He, Dynamics analysis of typhoid fever with public health education programs and final epidemic size relation, Results Appl. Math., 10 (2021), 100153. https://doi.org/10.1016/j.rinam.2021.100153 doi: 10.1016/j.rinam.2021.100153
![]() |
[43] |
S. S. Musa, S. Zhao, N. Hussaini, A. G. Habib, D. He, Mathematical modeling and analysis of meningococcal meningitis transmission dynamics, Int. J. Biomath., 13 (2020), 2050006. https://doi.org/10.1142/S1793524520500060 doi: 10.1142/S1793524520500060
![]() |
Variable | Interpretation |
Nh | Total population of humans |
Sh | Susceptible humans with risk of plague infection |
Ih | Infected humans with symptoms of plague infection |
Rh | Recovered humans |
Dh | Plague deceased-humans |
Nf | Total population of fleas |
Hf | Average number of fleas living on a rat |
Ff | Number of free infectious fleas searching for a host |
Nr | Total population of rats |
Sr | Susceptible rats |
Ir | Plague-infected rats |
Rr | Recovered rats |
Dr | Plague deceased-rats |
Parameter | Notation | Baseline value | Remark/Unit | Source(s) |
Transmission rate for humans | βh | 0.1001(0.01−0.2) | variable | [4] |
Transmission rate for rodents | βr | 0.5001(0.02−0.75) | variable | [4] |
Average infectious period in humans | 1/γh | 10(2.38−66.67) | day | [4] |
Average infectious period in rats | 1/γr | 5.2(1.59−100) | day | [4] |
Humans recovery rate | gh | 0.4(0.01−0.8) | dimensionless | [4] |
Rats recovery rate | gr | 0.1(0.01−0.5) | dimensionless | [4] |
Flea growth rate | rf | 0.0084(0.0001−0.05) | day−1 | [5] |
Fleas carrying capacity | Kf | 6(3.29−11.17) | dimensionless | [4,5] |
Flea searching efficiency | a | 0.004(0−1) | dimensionless | [5] |
Natural death rate of fleas | 1/μf | 5.0(3.23−100) | day | [4] |
Natural death rate of rats | 1/μr | 5.0(4.0−50.0) | day | [5] |
Natural death rate of humans | 1/μh | 1/(35×365)(1/(32×365)−1/(45×365)) | day−1 | [32,33] |
Recruitment rate of humans | πh | 42.27(32.88−46.23) | person \cdot day−1 | [32,33] |
Variable | Interpretation |
Nh | Total population of humans |
Sh | Susceptible humans with risk of plague infection |
Ih | Infected humans with symptoms of plague infection |
Rh | Recovered humans |
Dh | Plague deceased-humans |
Nf | Total population of fleas |
Hf | Average number of fleas living on a rat |
Ff | Number of free infectious fleas searching for a host |
Nr | Total population of rats |
Sr | Susceptible rats |
Ir | Plague-infected rats |
Rr | Recovered rats |
Dr | Plague deceased-rats |
Parameter | Notation | Baseline value | Remark/Unit | Source(s) |
Transmission rate for humans | βh | 0.1001(0.01−0.2) | variable | [4] |
Transmission rate for rodents | βr | 0.5001(0.02−0.75) | variable | [4] |
Average infectious period in humans | 1/γh | 10(2.38−66.67) | day | [4] |
Average infectious period in rats | 1/γr | 5.2(1.59−100) | day | [4] |
Humans recovery rate | gh | 0.4(0.01−0.8) | dimensionless | [4] |
Rats recovery rate | gr | 0.1(0.01−0.5) | dimensionless | [4] |
Flea growth rate | rf | 0.0084(0.0001−0.05) | day−1 | [5] |
Fleas carrying capacity | Kf | 6(3.29−11.17) | dimensionless | [4,5] |
Flea searching efficiency | a | 0.004(0−1) | dimensionless | [5] |
Natural death rate of fleas | 1/μf | 5.0(3.23−100) | day | [4] |
Natural death rate of rats | 1/μr | 5.0(4.0−50.0) | day | [5] |
Natural death rate of humans | 1/μh | 1/(35×365)(1/(32×365)−1/(45×365)) | day−1 | [32,33] |
Recruitment rate of humans | πh | 42.27(32.88−46.23) | person \cdot day−1 | [32,33] |