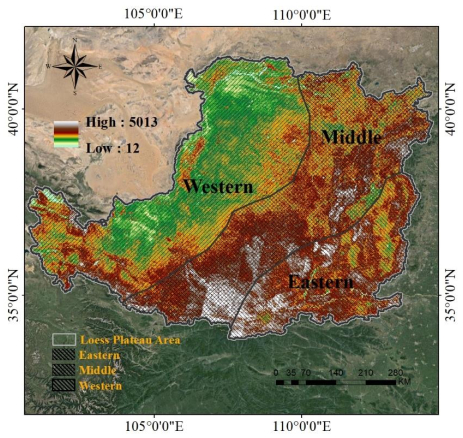
This study aimed to assess the impact of land consolidation projects and climate change on changes in vegetation in the Loess Plateau during 2012–2021. The study also explored the impacts of human activities and climate change on the ecological quality of the Loess Plateau during this period. The spatial and temporal normalized difference combined meteorological monitoring data, project data, and normalized difference vegetation index (NDVI) data that was used to create the vegetation index dataset spanning from 2012–2021. The study discussed and assessed the effectiveness of the project, revealing the following results: 1) A significant increase was observed in the vegetation index of the Loess Plateau region from 2012 to 2021, with an upward trend of 0.0024 per year (P < 0.05). 2) Contributions to changes in vegetation attributed to climatic factors and the anthropogenic factors of the ditch construction project were 82.74 and 17.62%, respectively, with climatic factors dominating and the degree of response of the ditch construction project increasing annually. 3) In the Loess Plateau, climatic variables dominated changes in vegetation. However, land consolidation projects in vegetation factors played a key role in changes in vegetation, and the degree of influence was gradually increasing.
Citation: Hui Kong, Liangyan Yang, Dan Wu, Juan Li, Shenglan Ye. Ditch control and land reclamation promote vegetation recovery in Loess Plateau[J]. Mathematical Biosciences and Engineering, 2024, 21(3): 3784-3797. doi: 10.3934/mbe.2024168
[1] | Shannon Dixon, Nancy Huntly, Priscilla E. Greenwood, Luis F. Gordillo . A stochastic model for water-vegetation systems and the effect of decreasing precipitation on semi-arid environments. Mathematical Biosciences and Engineering, 2018, 15(5): 1155-1164. doi: 10.3934/mbe.2018052 |
[2] | Jing Yang, Guo Xie, Yanxi Yang, Qijun Li, Cheng Yang . A multilevel recovery diagnosis model for rolling bearing faults from imbalanced and partially missing monitoring data. Mathematical Biosciences and Engineering, 2023, 20(3): 5223-5242. doi: 10.3934/mbe.2023242 |
[3] | Christopher Middlebrook, Xiaoying Wang . A mathematical model between keystone species: Bears, salmon and vegetation. Mathematical Biosciences and Engineering, 2023, 20(9): 16628-16647. doi: 10.3934/mbe.2023740 |
[4] | Jichun Li, Gaihui Guo, Hailong Yuan . Nonlocal delay gives rise to vegetation patterns in a vegetation-sand model. Mathematical Biosciences and Engineering, 2024, 21(3): 4521-4553. doi: 10.3934/mbe.2024200 |
[5] | Zixiao Xiong, Xining Li, Ming Ye, Qimin Zhang . Finite-time stability and optimal control of an impulsive stochastic reaction-diffusion vegetation-water system driven by L$ {\rm \acute{e}} $vy process with time-varying delay. Mathematical Biosciences and Engineering, 2021, 18(6): 8462-8498. doi: 10.3934/mbe.2021419 |
[6] | Linhao Xu, Donald L. DeAngelis . Effects of initial vegetation heterogeneity on competition of submersed and floating macrophytes. Mathematical Biosciences and Engineering, 2024, 21(10): 7194-7210. doi: 10.3934/mbe.2024318 |
[7] | Xiaomei Bao, Canrong Tian . Turing patterns in a networked vegetation model. Mathematical Biosciences and Engineering, 2024, 21(11): 7601-7620. doi: 10.3934/mbe.2024334 |
[8] | Longting Ding, Yuan Li, Zhanchuang Han, Mengyuan Zhang, Xuancang Wang, Lu He . The effect of RAP content on fatigue damage property of hot reclaimed asphalt mixtures. Mathematical Biosciences and Engineering, 2024, 21(2): 3037-3062. doi: 10.3934/mbe.2024135 |
[9] | Lingling Li, Congbo Li, Li Li, Ying Tang, Qingshan Yang . An integrated approach for remanufacturing job shop scheduling with routing alternatives. Mathematical Biosciences and Engineering, 2019, 16(4): 2063-2085. doi: 10.3934/mbe.2019101 |
[10] | Sarita Bugalia, Jai Prakash Tripathi, Hao Wang . Mathematical modeling of intervention and low medical resource availability with delays: Applications to COVID-19 outbreaks in Spain and Italy. Mathematical Biosciences and Engineering, 2021, 18(5): 5865-5920. doi: 10.3934/mbe.2021295 |
This study aimed to assess the impact of land consolidation projects and climate change on changes in vegetation in the Loess Plateau during 2012–2021. The study also explored the impacts of human activities and climate change on the ecological quality of the Loess Plateau during this period. The spatial and temporal normalized difference combined meteorological monitoring data, project data, and normalized difference vegetation index (NDVI) data that was used to create the vegetation index dataset spanning from 2012–2021. The study discussed and assessed the effectiveness of the project, revealing the following results: 1) A significant increase was observed in the vegetation index of the Loess Plateau region from 2012 to 2021, with an upward trend of 0.0024 per year (P < 0.05). 2) Contributions to changes in vegetation attributed to climatic factors and the anthropogenic factors of the ditch construction project were 82.74 and 17.62%, respectively, with climatic factors dominating and the degree of response of the ditch construction project increasing annually. 3) In the Loess Plateau, climatic variables dominated changes in vegetation. However, land consolidation projects in vegetation factors played a key role in changes in vegetation, and the degree of influence was gradually increasing.
Vegetation is considered a crucial component of terrestrial ecosystems. It is susceptible to environmental changes and serves as an indicator of changes in the regional ecological environment. Vegetation cover helps prevent erosion and reduces the flow of water and sediment [1]. Tracking the dynamic changes of vegetation on a broad scale in real time is challenging when using the existing measurement methods because of the geographical and temporal variations in regional changes in vegetation [2]. Remote sensing technology has gradually been applied in monitoring large-scale changes in the ecological environment. The normalized difference vegetation index (NDVI), first introduced by Rouse in 1973, is the most traditional and efficient indicator for vegetation coverage and growth. It is frequently employed to monitor large-scale dynamic changes in vegetation [3]. Climate change and land reclamation are the main factors affecting regional changes in vegetation [4]. The impact of ditch management on the ecological environment has deepened with the rapid growth of society and the economy, steadily developing into a determining factor that cannot be disregarded in regional changes in vegetation [5]. For instance, Cao's finding showing that incorrect afforestation in regions with little precipitation and high potential evapotranspiration may actually worsen ecosystem deterioration [6], wind erosion, and environmental degradation, has also received much attention [7]. Quantitative evaluation methods for ditch reclamation mainly include the regression model method and residual method. The regression model method is subjective in selecting the influencing factors and only assumes different relationships between vegetation and various driving factors. It is difficult to avoid the difference between factors [8]. Collinearity and spatial autocorrelation are incompatible. However, it is impossible to distinguish between how land reclamation and climate change affect vegetation [9]. The residual method involves calculating the difference between the simulated changes in vegetation in areas without human disturbance and the observed changes, providing an indirect estimate of the impact of ditch reclamation. Currently, the residual method model is most frequently used to distinguish between ditch reclamation and climate change [10]. The conclusions vary across studies due to differences in research periods and focal points. Sun et al. demonstrated that the Loess Plateau's vegetation coverage showed an increasing trend from 1982 to 1999. Yuan et al. examined the temporal and spatial variations in the vegetation coverage of the Loess Plateau from 2000 to 2010. He et al. studied the changes in vegetation cover in the Loess Plateau for 32 years and found that the vegetation cover had a relatively slow growth trend during the study period. Despite this, they suggested that this trend will remain essentially unchanged with continuous improvement in the future. However, a significant growth trend has been observed after 2005. In terms of influencing factors, Shi et al. showed that climatic factors contributed 45.78% to NDVI changes in the Loess Plateau, and ditch reclamation contributed 54.22%. Long-term NDVI trends are more sensitive to climate change than short-term trends. Li et al. reported that vegetation improved in areas with annual precipitation > 400 mm in the central region, while no obvious change in vegetation was noted in relatively arid areas [11].
This study used GIMMS NDVI 3g v1.0 and MODIS NDVI datasets to construct a long-term NDVI dataset spanning from 2012 to 2021 through pixel-by-pixel univariate linear regression. The goal was to analyze the temporal and spatial variations in vegetation cover over 11 years in the Loess Plateau [12]. Regional mapping of NDVI influencing factors was conducted to separate the effects of climate change and ditch reclamation on changes in vegetation cover by using the residual technique and employing correlation and regression analyses [13]. The vegetation cover in the Loess Plateau has significantly increased recently, with ongoing ditch reclamation initiatives in the central, eastern, and western regions of the watershed. However, it is unclear whether these changes in the watershed are directly related to the ditch reclamation project. The implementation effect of the ditch reclamation project area is beneficial. This study addressed the dynamic changes in vegetation in the area of the ditch control land reclamation project based on the analysis results of the dynamic changes in vegetation in the Loess Plateau and its influencing factors. The aim was to provide a reference for the subsequent formulation of relevant policies [14].
Most areas in the Loess Plateau (96°–119°E, 32°–42°N) were semi-arid and arid areas, with congenitally insufficient water resources. The average temperature was 2.68℃, with substantial regional temperature fluctuations and less than 450 mm of average annual precipitation. In contrast, high and low temperatures were recorded in the southeast and northwest areas, respectively. Most of the areas of ditch control and land reclamation projects were located in the central, eastern, and western regions. Forest vegetation types in the Loess Plateau included pine, cypress, and birch forests. Pine forests were widely distributed and were the most common type of forest vegetation in the region. The pine forests played a significant role in soil and water conservation (Figure 1).
The NDVI datasets used in this study were obtained from the MODIS MOD13Q1 (https://ladsweb.modaps.eosdis.nasa.gov/search/) and GIMMS NDVI3gv1.0 databases (https://www.nasa.gov/). The GIMMS NDVI collection offered NDVI data with an 8-km spatial resolution and a 15-day temporal range from 2012 to 2021. This study used the data from 2012 to 2021. The MOD1S dataset offered NDVI data with a spatial resolution of 250 m and a temporal resolution of 16 days from 2012 to the present.
The "Monthly Value Dataset of China's Surface Climate Data" from the China Meteorological Data Network provided information on precipitation and temperature. In this study, 218 sites in and around the Loess Plateau were selected (Figure 1), and their latitude and longitude details were obtained. Monthly precipitation and monthly average temperature data of these sites from 2012 to 2021 were also obtained.
The geographical distribution dataset of China's forestry engineering was selected to represent the data for the areas of ditch control and land reclamation projects on the resource and environmental data cloud platform of the Chinese Academy of Sciences.
The NDVI dataset from 2012 to 2021 was constructed for the Loess Plateau using a pixel-by-pixel univariate linear regression model based on the GIMMS NDVI3g and MODIS NDVI data. The changes in the temporal and spatial distribution of NDVI were then assessed using the least squares method in residual and partial correlation analyses. The impact of climatic variables and land reclamation on NDVI variations were assessed using the trend analysis technique.
The GIMMS NDVI3g V1.0 dataset was converted into a.tif format using the R language. Both NDVI datasets underwent preprocessing steps, including coordinate transformation, data cropping, and maximum value extraction. The monthly and annual NDVI datasets were combined using the maximum value composite method. The MODIS NDVI was resampled to 8 km, with specific resampling methods described in ENVI 5.3. The inverse distance weighting method was used to interpolate the station data for the monthly average temperature and monthly precipitation data obtained by the meteorological station. The spatial resolution of the grid after interpolation processing was 8 km. Preprocessing, such as geographic coordinate conversion and cropping, was performed on the acquired data of the areas of the ditch control and land reclamation project to delineate the project scope in the central, eastern, and western regions.
As the two datasets are from different sensors and show certain differences in spectral response, this study conducted a consistency test on the two datasets. The overlapping period data of GIMMS and MODIS were selected to test the pixel-by-pixel univariate linear regression model, constructing a model for monthly NDVI for the period 2001–2008 and a verification model for the period 2017–2021. The new NDVI dataset was then constructed using the regression model and named the GIMMS–MODIS NDVI. The formula used for the pixel-by-pixel univariate linear regression model is as follows:
Gi=λ+μVi+ϵi | (1) |
μ=∑ni=1(Gi−¯V)(Gi−¯G)∑ni=1(Vi−¯V)2 | (2) |
λ=¯G-μ¯V | (3) |
where λ; μ is the parameter; εi is the random error; n is the research period; i represents the month; Gi is the GIMMS NDVI value of the ith month; Vi is the MODIS NDVI value of the ith month; G is the average value of the monthly GIMMS NDVI data at the corresponding pixel; and V is the average value of the monthly GIMMS NDVI data at the corresponding pixel.
The ordinary least square (OLS) method was used to assess the spatial fluctuation of NDVI from 2012 to 2021. The slope of the OLS curve was calculated using the equation:
θslop=n×∑ni=1i×Xi−∑ni=1i∑ni=1Xin×∑ni=1i2−(∑ni=1i)2 | (4) |
where θslop is the regression coefficient, i represents the year, Xi represents the NDVI value of the corresponding year in year i, and n is the research period that reflects the trend of the variable. θslop values between 0 and 1 indicate whether the NDVI is showsan upward or downward trend. The significance of the changing trend results was assessed using the F-significance test.
By adjusting for additional factors, partial correlation analysis describes the correlation between two variables among several variables [15]. Compared with the correlation coefficient, the partial correlation coefficient is more accurate and reliable. The correlation between climatic variables and NDVI was investigated in this study using partial correlation analysis, and the calculation results were subjected to the T-significance test.
A widely used method for quantitatively separating the impacts of ditch maintenance and climate change on changes in vegetation is the NDVI residual approach [16]:
ψ=NDVIreal−NDVIpre | (5) |
NDVIpre=α×P+β×T+ϕ | (6) |
where α is the regression coefficient of NDVI and precipitation; β is the regression coefficient of NDVI and air temperature; φ is the regression constant; P and T are the annual average total precipitation and annual average temperature, respectively; NDVIreal is the observed value in remote sensing images; and NDVIpre is the predicted value of NDVI obtained by regression analysis.
ψ > 0 indicates that land reclamation has a positive impact on NDVI changes;
ψ < 0 indicates that ditch reclamation has a negative impact on NDVI changes; and ψ = 0 indicates that ditch reclamation has a relatively weak impact on NDVI changes.
Based on the residual analysis results, the overall impact of ditch management on NDVI is as follows [17]:
CH=ψ/NDVIreal×100% | (7) |
The overall impact of climate change on NDVI is:
CN=1−CH | (8) |
The influencing factors in regions with significant changes in NDVI were categorized into six groups based on the results of significant changes in NDVI, the partial correlation analysis between climatic factors, and NDVI and their significance levels, as well as the trend and significance levels of multi-year residuals (Table 1).
Driver | NDVI change trend (P < 0.05) | Correlation between NDVI and climatic factors | Variation trend of NDVI residuals | ||
Significant increase in NDVI caused by climate and ditch reclamation | + | + | P < 0.05 | + | P < 0.05 |
Significant increase in NDVI due to climatic factors | + | + | P < 0.05 | P > 0.05 | |
Significant increase in NDVI dominated by ditch reclamation | + | P > 0.05 | + | P < 0.05 | |
Significant decline in NDVI caused by climate and ditch reclamation | – | – | P < 0.05 | – | P < 0.05 |
Significant decline in NDVI due to climatic factors | – | – | P < 0.05 | P > 0.05 | |
Significant decline in NDVI dominated by ditch reclamation | – | P > 0.05 | – | P < 0.05 | |
*Note: The "+" in the table refers to a positive correlation of the two factors and the "-" refers to a negative correlation of the two factors. |
Figure 2 displays the monthly GMMS NDVI and MODIS NDVI variation trends from 2012 to 2021. The figure shows that the overall fluctuation trends of the two datasets were consistent, mirroring the regional annual vegetation growth phenology similarly.
The data from April to October for the years 2012 to 2017 were chosen based on the pixel scale, and this dataset was used to validate the regression model with additional data from the same months in the period from 2017 to 2021. The study represents the results of fitting GIMMS NDVI and MODIS NDVI, as well as GIMMS NDVI and modified MODIS NDVI (GIMMS–MODIS NDVI), for the period from April to October, spanning from 2017 to 2021. The root-mean-square error (RMSE) between GIMMS and MODIS NDVI was 0.0321, the bias was 0.001, and the goodness of fit (R2) was 0.7754. The RMSE between GIMMS–MODIS NDVI and GIMMS NDVI was 0.0183, the bias was 0.0003, and the goodness of fit (R2) was 0.8536. MODIS NDVI and GIMMS NDVI data exhibited a better fitting effect after regression model modification.
The annual average NDVI in the Loess Plateau increased substantially between 2012 and 2021, with an overall trend of 0.0024 per year. The growth trend of NDVI was relatively slow from 2012 to 2017, whereas it was relatively fast from 2017 to 2021. NDVI fluctuated between 0.49 and 0.64 during the study period, with the lowest (0.49) in 2012 and the highest (0.64) in 2017. Figure 1 illustrates the regional distribution of NDVI in the Loess Plateau, which exhibited a steadily diminishing spatial distribution from south to north. The distribution of the Kubuqi Desert and bare rock in the west and north contrasted with the distribution of the Qinghai–Tibet Plateau, Loess Plateau, Fenhe River basin, and Weihe River basin in the east and south, where vegetation cover was often good. The slope of NDVI change for the Loess Plateau from 2012 to 2021, as shown in Figure 3, indicates that 17.32% of the region's pixels exhibited a declining trend during the past 11 years, whereas 82.68% exhibited an increasing trend, with 75.99% of them experiencing significant changes. In general, the low-value and medium-value areas increased, while the high-value area deceased. Although the substantial increase in area was primarily concentrated in the middle and north of the basin, the significant degradation area was dispersed primarily in the west and south of the basin.
The Loess Plateau experienced an increase in annual precipitation, average temperature, and warm, humid conditions over the last 11 years. The increasing trend in annual precipitation was 0.608 6 mm per year, and the annual temperature was 0.042 1℃/a (Figure 5). Geographically, the water and heat resources of the basin gradually diminished from south to north, with significant resources in the southeast and fewer in the northwest.
The results of the correlation between NDVI and climatic variables are shown in Figure 4. The partial correlation coefficients, which ranged from 0.27 to 0.99 with an average of 0.83 in calculating precipitation and NDVI, were all positive. Of these, 99.67% of the pixels passed the significance test (P = 0.05). The correlation coefficients between temperature and NDVI ranged from −0.97 to 0.85, with an average of −0.24. Of these, 53.53% of pixels passed the significance test with a P value of 0.05, whereas 23.41% of pixels displayed positive correlation and 76.59% displayed negative correlation. Precipitation and NDVI exhibited a substantial positive association, and average temperature and NDVI had a significant negative correlation. Precipitation had a bigger overall impact on NDVI than the average temperature. Spatially, precipitation was significantly correlated with the basin as a whole, and the correlation was mainly positive. Compared with other regions, the correlation was stronger in the western and central regions. In the central and eastern regions, a substantial negative correlation was observed between temperature and NDVI, whereas no such correlation existed in the other regions.
The combined contribution of ditch land reclamation factors and climatic factors to NDVI change was 17.26%, with climatic factors accounting for 82.74%, according to the separation of the impacts of climate change and ditch land reclamation shown in Figure 5. Among the influencing factors of NDVI change, climate change played a dominant role. Spatially, the level of overall impact of the two influencing factors exhibited heterogeneity. The impact of ditching and land reclamation gradually decreased from north to south, with the highest distribution in the Northern Kubuqi Desert and some western areas, and the lowest distribution in the southwest Qinghai–Tibet Plateau. In contrast, climatic factors exhibited an opposing trend. Only in the northwest part of the basin did ditching and land reclamation have a higher impact on NDVI, while other regional climatic factors were dominant.
The contribution rate of land reclamation was 0.127 per year (P < 0.05) and that of climatic factors was −0.136 per year (P < 0.05). The impact of land reclamation gradually enhanced, while that of climatic factors gradually weakened. In terms of spatial distribution, the overall contribution of drainage land reclamation declined in 31.93% of the areas, whereas the contribution of climatic factors increased, with the majority of the increase occurring in the northeast of the basin. Compared with drainage and land reclamation, primarily dispersed in the south, west, and north of the basin, 68.10% of the total contribution from regional climatic components exhibited a decreasing trend (Figure 6).
The climatic factors of 99.98% of the pixels in the Loess Plateau exhibited a substantial correlation with NDVI based on the results of the correlation analysis and significance test of climatic factors and NDVI, which are depicted by two results in Table 1. This study did not find a substantial increase or decrease in the dominant NDVI of ditch reclamation. The four categories into which the regional influencing factors of significant changes in NDVI are grouped as follows: the significant increase in NDVI caused by climate and ditching land, the significant increase in NDVI caused by climatic factors, the significant decrease in NDVI caused by climatic factors, and the significant decrease in NDVI caused by climatic factors. Figure 6 depicts the zoning of influencing factors in the regions of the Loess Plateau that had large NDVI changes between 2012 and 2021. Notably, 4.87% of the areas experienced significant vegetation degradation because of climatic factors, 61.16% of the areas underwent significant vegetation recovery because of climatic factors, and 1.11% of the areas underwent significant vegetation degradation because of both climate and land reclamation factors. The vegetation recovery area dominated by climate and land reclamation factors accounted for 32.86%.
The Loess Plateau was divided into the eastern, middle, and western regions. Table 2 shows the statistics of NDVI changes and the impact of influencing factors in these three regions. The vegetation coverage rate in these three regions all showed an increasing trend, which was higher than the average, indicating that vegetation recovery was obvious in the range involved in the ditch reclamation project. The implementation of ditch reclamation projects significantly improved regional vegetation coverage, a factor that could not be ignored in regional vegetation restoration. Meanwhile, residual values were positive in the central and southern regions of the Loess Plateau, and the overall impact of ditch reclamation has consistently strengthened during the study period.
Hong ditch stretched engineering | In the west | In the east | In the middle |
Average annual NDVI | 0.672 | 0.541 | 0.37 |
NDVI change slope (per a-1) | 0.004 | 0.005 | 0.006 |
Residual value | 0.001 | 0.0033 | 0.0034 |
Impact of climatic factors (%) | 86.23 | 83.55 | 77.26 |
Ditch-building contribution (%) | 13.77 | 16.45 | 22.74 |
Slope of change in level of impact of ditch reclamation (per a-1) | 0.1102 | 0.0725 | 0.1231 |
Climatic factors dominated NDVI significantly increased the regional proportion (%) | 65.7 | 66.5 | 67.2 |
Climatic factors and land reclamation jointly led NDVI to increase the regional proportion significantly (%) | 34.5 | 31.9 | 32.8 |
Table 2 presents the statistics of NDVI change and the impact of influencing factors in the drainage land reclamation project area. The values indicate that the vegetation recovery was evident in the drainage land reclamation project area; the vegetation in all three engineering areas showed an increasing trend, which was higher than the basin average. Meanwhile, the residual values were all positive in the engineering area, and the overall impact of ditch land reclamation was continuously strengthened in the study period. This indicates that the ditch land reclamation project significantly improves regional vegetation coverage, a factor that cannot be ignored for regional vegetation restoration.
The western region had the best vegetation coverage, followed by the eastern and central regions. Although NDVI in the western region did not exhibit a significant growth trend during the research period, it did in the eastern and central regions (P 0.05). In terms of influencing factors, ditch land reclamation positively affected vegetation in the three study regions, with the central region contributing the most, followed by the eastern region, and the western region contributing the least. In all three study regions, a 60% increase was found in the amount of vegetation that had been significantly restored by climate change. Climatic factors significantly impacted vegetation in the ditch land reclamation engineering areas more than the ditch land reclamation factors, which played a dominant role; however, the contribution of ditch land reclamation increased during the study period.
1) The NDVI of the Loess Plateau displayed a significant upward trend of 0.0024 per year from 2012 to 2021 (P < 0.05). Spatial patterns of the NDVI values of the Loess Plateau indicate a declining trend in the high-value area and an increasing trend in the low- and medium-value areas, with 17.32% of the image elements' NDVI exhibiting a declining trend and 82.68% exhibiting an increasing trend. Significantly increased trends were primarily found in areas with low NDVI values, such as the central and northern parts of the basin, whereas significantly degraded areas were primarily found in regions with high NDVI values, such as the western and southern parts of the basin.
2) In the Loess Plateau, the correlation coefficients for precipitation and air temperature with NDVI were 0.83 (P < 0.05) and –0.24, respectively. The watershed's heavy reliance on precipitation was related to the fact that it was located in a semi-arid and arid environment with few water supplies. Precipitation had a substantial positive correlation with vegetation in the basin. In contrast, mean temperature had a significantly positive correlation with vegetation mainly in the central and eastern parts of the basin, with no correlation in the other regions.
3) During the 11-year period, the overall impact of climatic factors on NDVI changes in the Loess Plateau was 82.74%, while that of ditch reclamation to NDVI changes was 17.26%. The percentage of places in the Loess Plateau where climatic variables considerably dominated vegetation regrowth was 61.16%. The percentage of regions in the Loess Plateau where climate and ditch reclamation in vegetation elements considerably dominated vegetation recovery was 32.86%. In the Loess Plateau, climatic variables dominate changes in vegetation. However, ditch reclamation in vegetation factors plays a key role in changes in vegetation, and the degree of influence is gradually increasing.
The authors declare they have not used Artificial Intelligence (AI) tools in the creation of this article.
We would like to thank you for following the instructions above very closely in advance. Supported by Funded by Development Program of Shaanxi under Grant (2022ZDLNY02-10) and Soil moisture inversion study of the Loess Plateau dry plateau based on SAR radar data(DJNY2022-23).
The authors declare there is no conflict of interest.
[1] |
F. Ji, D. Han, L. Yan, S. Yan, J. Zha, J. Shen, Assessment of benthic invertebrate diversity and river ecological status along an urbanized gradient using environmental DNA metabarcoding and a traditional survey method, Sci. Total Environ., 806 (2022), 150587. https://doi.org/10.1016/j.scitotenv.2021.150587 doi: 10.1016/j.scitotenv.2021.150587
![]() |
[2] |
X. Yao, Y. Cao, G. Zheng, A. T. Devlin, J. S. Khim, Use of life cycle assessment and water quality analysis to evaluate the environmental impacts of the bioremediation of polluted water. Sci. Total Environ., 761 (2021), 143260. https://doi.org/10.1016/j.scitotenv.2020.143260 doi: 10.1016/j.scitotenv.2020.143260
![]() |
[3] |
R. Pascual-Rico, Z. Morales-Reyes, N. Aguilera-Alcalá, A. Olszańska, E. Sebastián-González, R. Naidoo, et al., Usually hated, sometimes loved: A review of wild ungulates' contributions to people, Sci. Total Environ., 801 (2021), 149652. https://doi.org/10.1016/j.scitotenv.2021.149652 doi: 10.1016/j.scitotenv.2021.149652
![]() |
[4] |
H. Liu, G. Liu, W. Xing, Functional traits of submerged macrophytes in eutrophic shallow lakes affect their ecological functions, Sci. Total Environ., 760 (2021), 143332. https://doi.org/10.1016/j.scitotenv.2020.143332 doi: 10.1016/j.scitotenv.2020.143332
![]() |
[5] |
Z. Q. Gao, J. Y. Liu, D. F. Zhuang, The relations analysis between ecological environmental quality of Chinese land resources and population, Can. J. Remote Sens., 1 (1999), 66–70. https://doi.org/10.11834/jrs.19990110 doi: 10.11834/jrs.19990110
![]() |
[6] |
D. Jarvis, N. Stoeckl, S. Larson, D. Grainger, J. Addison, A. Larson, The learning generated through indigenous natural resources management programs increases quality of life for indigenous people–improving numerous contributors to Wellbeing, Ecol. Econ., 180 (2021), 106899. https://doi.org/10.1016/J.ECOLECON.2020.106899 doi: 10.1016/J.ECOLECON.2020.106899
![]() |
[7] |
P. Huang, C. Ma, A. Zhou, Assessment of groundwater sustainable development considering geo-environment stability and ecological environment: a case study in the Pearl River Delta, China, Environ. Sci. Pollut. R, 29 (2021), 18010–18035. https://doi.org/10.1007/s11356-021-16924-6 doi: 10.1007/s11356-021-16924-6
![]() |
[8] |
N. Chai, X. Yi, J. Xiao, T. Liu, Y. Liu, L. Deng, Spatiotemporal variations, sources, water quality and health risk assessment of trace elements in the Fen River, Sci. Total Environ., 757 (2021), 143882. https://doi.org/ 10.1016/j.scitotenv.2020.143882 doi: 10.1016/j.scitotenv.2020.143882
![]() |
[9] |
T. Zhai, J. Wang, Y. Fang, Y. Qin, L. Huang, Y. Chen, Assessing ecological risks caused by human activities in rapid urbanization coastal areas: Towards an integrated approach to determining key areas of terrestrial-oceanic ecosystems preservation and restoration, Sci. Total Environ., 708 (2020), 135153. https://doi.org/10.1016/j.scitotenv.2019.135153 doi: 10.1016/j.scitotenv.2019.135153
![]() |
[10] |
F. Shi, S. Liu, Y. Sun, Y. An, S. Zhao, Y. Liu, et al., Ecological network construction of the heterogeneous agro-pastoral areas in the upper Yellow River basin, Agr. Ecosyst. Environ., 302 (2020), 107069. https://doi.org/10.1016/j.agee.2020.107069 doi: 10.1016/j.agee.2020.107069
![]() |
[11] |
J. Li, Study on the impact of seashore leisure behavior on ecological environment based on water quality analysis, J. Coastal. Res., 115 (2020), 193. https://doi.org/10.2112/JCR-SI115-060.1 doi: 10.2112/JCR-SI115-060.1
![]() |
[12] |
M. Höglind, D. Cameron, T. Persson, X. Huang, M. V. Oijen, BASGRA_N: A model for grassland productivity, quality and greenhouse gas balance, Ecol. Model., 417 (2020), https://doi.org/10.1016/j.ecolmodel.2019.108925 doi: 10.1016/j.ecolmodel.2019.108925
![]() |
[13] |
Y. X. Gao, Study on water resources carrying capacity of ChangjiArea based on water quality-ecological system, Ground Water, 26 (2019) 11000–11011, https://doi.org/10.1007/s11356-019-04414-9 doi: 10.1007/s11356-019-04414-9
![]() |
[14] |
G. Sun, S. J. Hoff, B. C. Zelle, M. A. Nelson, Forecasting daily source air quality using multivariate statistical analysis and radial basis function networks, J. Air Waste Manage., 58 (2019), 1571–1578. https://doi.org/10.3155/1047-3289.58.12.1571 doi: 10.3155/1047-3289.58.12.1571
![]() |
[15] |
L. C. Evans, R. M. Sibly, P. Thorbek, I. Sims, T. H. Oliver, R. J. Walters, Quantifying the effectiveness of agri-environment schemes for a grassland butterfly using individual-based models, Ecol. Model., 411 (2019), 108798. https://doi.org/10.1016/j.ecolmodel.2019.108798 doi: 10.1016/j.ecolmodel.2019.108798
![]() |
[16] |
S. R. Corsi, L. A. De Cicco, D. L. Villeneuve, B. R. Blackwell, K. A. Fay, G. T. Ankley, et al., Prioritizing chemicals of ecological concern in Great Lakes tributaries using high-throughput screening data and adverse outcome pathways, Sci. Total Environ., 686 (2019), 995–1009. https://doi.org/10.1016/j.scitotenv.2019.05.457 doi: 10.1016/j.scitotenv.2019.05.457
![]() |
[17] |
D. Saadoud, M. Hassani, F. J. M. Peinado, M. S. Guettouche, Application of fuzzy logic approach for wind erosion hazard mapping in Laghouat region (Algeria) using remote sensing and GIS, Aeolian Res., 32 (2018), 762–781. https://doi.org/10.3390/sym11060762 doi: 10.3390/sym11060762
![]() |
Driver | NDVI change trend (P < 0.05) | Correlation between NDVI and climatic factors | Variation trend of NDVI residuals | ||
Significant increase in NDVI caused by climate and ditch reclamation | + | + | P < 0.05 | + | P < 0.05 |
Significant increase in NDVI due to climatic factors | + | + | P < 0.05 | P > 0.05 | |
Significant increase in NDVI dominated by ditch reclamation | + | P > 0.05 | + | P < 0.05 | |
Significant decline in NDVI caused by climate and ditch reclamation | – | – | P < 0.05 | – | P < 0.05 |
Significant decline in NDVI due to climatic factors | – | – | P < 0.05 | P > 0.05 | |
Significant decline in NDVI dominated by ditch reclamation | – | P > 0.05 | – | P < 0.05 | |
*Note: The "+" in the table refers to a positive correlation of the two factors and the "-" refers to a negative correlation of the two factors. |
Hong ditch stretched engineering | In the west | In the east | In the middle |
Average annual NDVI | 0.672 | 0.541 | 0.37 |
NDVI change slope (per a-1) | 0.004 | 0.005 | 0.006 |
Residual value | 0.001 | 0.0033 | 0.0034 |
Impact of climatic factors (%) | 86.23 | 83.55 | 77.26 |
Ditch-building contribution (%) | 13.77 | 16.45 | 22.74 |
Slope of change in level of impact of ditch reclamation (per a-1) | 0.1102 | 0.0725 | 0.1231 |
Climatic factors dominated NDVI significantly increased the regional proportion (%) | 65.7 | 66.5 | 67.2 |
Climatic factors and land reclamation jointly led NDVI to increase the regional proportion significantly (%) | 34.5 | 31.9 | 32.8 |
Driver | NDVI change trend (P < 0.05) | Correlation between NDVI and climatic factors | Variation trend of NDVI residuals | ||
Significant increase in NDVI caused by climate and ditch reclamation | + | + | P < 0.05 | + | P < 0.05 |
Significant increase in NDVI due to climatic factors | + | + | P < 0.05 | P > 0.05 | |
Significant increase in NDVI dominated by ditch reclamation | + | P > 0.05 | + | P < 0.05 | |
Significant decline in NDVI caused by climate and ditch reclamation | – | – | P < 0.05 | – | P < 0.05 |
Significant decline in NDVI due to climatic factors | – | – | P < 0.05 | P > 0.05 | |
Significant decline in NDVI dominated by ditch reclamation | – | P > 0.05 | – | P < 0.05 | |
*Note: The "+" in the table refers to a positive correlation of the two factors and the "-" refers to a negative correlation of the two factors. |
Hong ditch stretched engineering | In the west | In the east | In the middle |
Average annual NDVI | 0.672 | 0.541 | 0.37 |
NDVI change slope (per a-1) | 0.004 | 0.005 | 0.006 |
Residual value | 0.001 | 0.0033 | 0.0034 |
Impact of climatic factors (%) | 86.23 | 83.55 | 77.26 |
Ditch-building contribution (%) | 13.77 | 16.45 | 22.74 |
Slope of change in level of impact of ditch reclamation (per a-1) | 0.1102 | 0.0725 | 0.1231 |
Climatic factors dominated NDVI significantly increased the regional proportion (%) | 65.7 | 66.5 | 67.2 |
Climatic factors and land reclamation jointly led NDVI to increase the regional proportion significantly (%) | 34.5 | 31.9 | 32.8 |