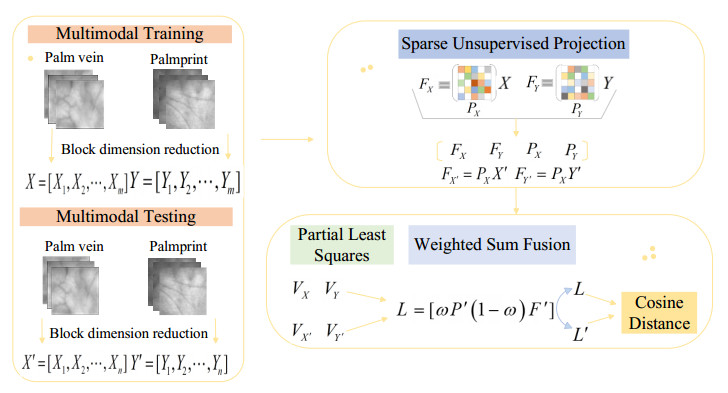
Biometric authentication prevents losses from identity misuse in the artificial intelligence (AI) era. The fusion method integrates palmprint and palm vein features, leveraging their stability and security and enhances counterfeiting prevention and overall system efficiency through multimodal correlations. However, most of the existing multi-modal palmprint and palm vein feature extraction methods extract only feature information independently from different modalities, ignoring the importance of the correlation between different modal samples in the class to the improvement of recognition performance. In this study, we addressed the aforementioned issues by proposing a feature-level joint learning fusion approach for palmprint and palm vein recognition based on modal correlations. The method employs a sparse unsupervised projection algorithm with a "purification matrix" constraint to enhance consistency in intra-modal features. This minimizes data reconstruction errors, eliminating noise and extracting compact, and discriminative representations. Subsequently, the partial least squares algorithm extracts high grayscale variance and category correlation subspaces from each modality. A weighted sum is then utilized to dynamically optimize the contribution of each modality for effective classification recognition. Experimental evaluations conducted for five multimodal databases, composed of six unimodal databases including the Chinese Academy of Sciences multispectral palmprint and palm vein databases, yielded equal error rates (EER) of 0.0173%, 0.0192%, 0.0059%, 0.0010%, and 0.0008%. Compared to some classical methods for palmprint and palm vein fusion recognition, the algorithm significantly improves recognition performance. The algorithm is suitable for identity recognition in scenarios with high security requirements and holds practical value.
Citation: Wei Wu, Yuan Zhang, Yunpeng Li, Chuanyang Li. Fusion recognition of palmprint and palm vein based on modal correlation[J]. Mathematical Biosciences and Engineering, 2024, 21(2): 3129-3145. doi: 10.3934/mbe.2024139
[1] | Xiao Ma, Xuemei Luo . Finger vein recognition method based on ant colony optimization and improved EfficientNetV2. Mathematical Biosciences and Engineering, 2023, 20(6): 11081-11100. doi: 10.3934/mbe.2023490 |
[2] | Hao Wang, Guangmin Sun, Kun Zheng, Hui Li, Jie Liu, Yu Bai . Privacy protection generalization with adversarial fusion. Mathematical Biosciences and Engineering, 2022, 19(7): 7314-7336. doi: 10.3934/mbe.2022345 |
[3] | Xiaoguang Liu, Meng Chen, Tie Liang, Cunguang Lou, Hongrui Wang, Xiuling Liu . A lightweight double-channel depthwise separable convolutional neural network for multimodal fusion gait recognition. Mathematical Biosciences and Engineering, 2022, 19(2): 1195-1212. doi: 10.3934/mbe.2022055 |
[4] | Yafeng Zhao, Xuan Gao, Junfeng Hu, Zhen Chen . Tree species identification based on the fusion of bark and leaves. Mathematical Biosciences and Engineering, 2020, 17(4): 4018-4033. doi: 10.3934/mbe.2020222 |
[5] | Zhijing Xu, Yang Gao . Research on cross-modal emotion recognition based on multi-layer semantic fusion. Mathematical Biosciences and Engineering, 2024, 21(2): 2488-2514. doi: 10.3934/mbe.2024110 |
[6] | Yongmei Ren, Xiaohu Wang, Jie Yang . Maritime ship recognition based on convolutional neural network and linear weighted decision fusion for multimodal images. Mathematical Biosciences and Engineering, 2023, 20(10): 18545-18565. doi: 10.3934/mbe.2023823 |
[7] | Yong Tian, Tian Zhang, Qingchao Zhang, Yong Li, Zhaodong Wang . Feature fusion–based preprocessing for steel plate surface defect recognition. Mathematical Biosciences and Engineering, 2020, 17(5): 5672-5685. doi: 10.3934/mbe.2020305 |
[8] | Chenkai Chang, Fei Qi, Chang Xu, Yiwei Shen, Qingwu Li . A dual-modal dynamic contour-based method for cervical vascular ultrasound image instance segmentation. Mathematical Biosciences and Engineering, 2024, 21(1): 1038-1057. doi: 10.3934/mbe.2024043 |
[9] | Cong Lin, Yiquan Huang, Wenling Wang, Siling Feng, Mengxing Huang . Lesion detection of chest X-Ray based on scalable attention residual CNN. Mathematical Biosciences and Engineering, 2023, 20(2): 1730-1749. doi: 10.3934/mbe.2023079 |
[10] | Hongmei Jin, Ning He, Zhanli Li, Pengcheng Yang . Micro-expression recognition based on multi-scale 3D residual convolutional neural network. Mathematical Biosciences and Engineering, 2024, 21(4): 5007-5031. doi: 10.3934/mbe.2024221 |
Biometric authentication prevents losses from identity misuse in the artificial intelligence (AI) era. The fusion method integrates palmprint and palm vein features, leveraging their stability and security and enhances counterfeiting prevention and overall system efficiency through multimodal correlations. However, most of the existing multi-modal palmprint and palm vein feature extraction methods extract only feature information independently from different modalities, ignoring the importance of the correlation between different modal samples in the class to the improvement of recognition performance. In this study, we addressed the aforementioned issues by proposing a feature-level joint learning fusion approach for palmprint and palm vein recognition based on modal correlations. The method employs a sparse unsupervised projection algorithm with a "purification matrix" constraint to enhance consistency in intra-modal features. This minimizes data reconstruction errors, eliminating noise and extracting compact, and discriminative representations. Subsequently, the partial least squares algorithm extracts high grayscale variance and category correlation subspaces from each modality. A weighted sum is then utilized to dynamically optimize the contribution of each modality for effective classification recognition. Experimental evaluations conducted for five multimodal databases, composed of six unimodal databases including the Chinese Academy of Sciences multispectral palmprint and palm vein databases, yielded equal error rates (EER) of 0.0173%, 0.0192%, 0.0059%, 0.0010%, and 0.0008%. Compared to some classical methods for palmprint and palm vein fusion recognition, the algorithm significantly improves recognition performance. The algorithm is suitable for identity recognition in scenarios with high security requirements and holds practical value.
Biometric recognition, due to its convenience and security, has replaced traditional identification methods and found widespread applications in various domains. Palmprint features are easy to collect and exhibit good stability. However, the accuracy of palmprint recognition [1] can be affected when the surface skin of the subject's palm is damaged, leading to incomplete feature information. Palm vein features, concealed beneath the epidermis, require collection under near-infrared light and are immune to theft via photography. Moreover, they enable liveness detection, making them a highly secure biometric feature. Nevertheless, the non-transparency, non-uniformity, and heterogeneity of the skin tissue covering the palm veins result in scattering of near-infrared light during imaging. This phenomenon can lead to unclear palm vein images in certain populations, thereby impacting the performance of palm vein recognition.
The fusion biometric recognition method of palmprint and palm vein not only leverages the stability of palmprint features but also capitalizes on the high security of palm vein features. This approach enhances the system's anti-counterfeiting capabilities while improving the system's recognition performance. It is a biometric fusion recognition method with high security and high user acceptance. Furthermore, we were inspired by the literature [2] and focused on the inter-modal correlation problem. The idea of joint learning was applied to feature extractions of palmprints and palm vein features.
At this stage, multimodal biometric recognition has gradually emerged as one of the primary focuses in the field of recognition. In this context, study [3] delves into the extraction of pixel difference vectors in multiple directions, achieving feature-level fusion by calculating differences between each pixel and its linear neighboring pixels in two modalities: palmprint and palm vein. Within literature [4], a deep scattering convolutional network is utilized to extract features from palmprints and palm veins, subsequently employing wavelet-based fusion. Moving on to literature [5], an effective fusion approach is employed, combining iris, face, and fingerprint features at the score level. This fusion technique integrates principal component analysis and local binary patterns. In literature [6], a structured robust and sparse least squares regression method is introduced, adaptively discriminating and recognizing fusion features from finger vein and finger knuckle print. In the realm of literature [7], a deep hash network is enlisted to extract binary templates for palmprint and palm vein features, followed by score-level fusion. For literature [8], key point detection and main line extraction are performed for hand geometry features and palmprint features, with corresponding points of the palmprint image detected through template-based matching for recognition. Finally, literature [9] employs Log-Gabor transform, histogram of oriented gradients (HOG), and local binary pattern (LBP) to extract features from palmprint and iris images, culminating in fractional level fusion.
At the level of biometric fusion, it can be categorized into two types based on fusion order: Pre-matching and post-matching. This includes sensor-level and feature-level fusion as pre-matching, and score-level, rank-level, and decision-level fusion as post-matching [10]. Feature-level fusion is capable of modeling biometric features from multiple dimensions and perspectives, effectively leveraging multimodal information. This helps mitigate errors and uncertainties introduced by noise, insufficient data, and non-robust features during single-feature extraction processes. Therefore, a palmprint and palm vein feature-level fusion recognition method based on joint learning is proposed. Due to the substantial amount of data involved in feature-level fusion, appropriate block-wise dimensionality reduction is applied to image features. This process aims to minimize the dimensions of the images while preserving the palmprint and palm vein features to the maximum extent. Subsequently, a sparse unsupervised projection algorithm with a "purification matrix" constraint is employed to perform dimensionality reduction and purification on the image features. This minimizes data reconstruction errors, eliminates redundant information in the subspace features, and extracts compact and discriminative feature representations. Following this, the partial least squares (PLS) algorithm is utilized to extract subspace features with high grayscale variation and intra-class correlation from each modality, promoting consistency in intra-class modality representation. The features extracted through joint learning enhance the stability of image features, emphasize the saliency of important information, and improve recognition performance. Finally, a weighted summation is employed to fuse the features extracted from palmprint and palm vein, jointly optimizing the contribution of each modality for classification recognition. The specific process is illustrated in Figure 1.
In Figure 1, X, Y, X′, and Y′ represent the training set and test set image matrices after preprocessing, chunking, and dimensionality reduction of palm lines and palm veins. Subsequently, X and Y undergo processing by the projection matrices PX and PY to obtain the new feature spaces FX and FY. The same projection matrices PX and PY are then applied to process the test set images, resulting in FX′ and FY′.The obtained feature matrices, namely FX, FY, FX′, and FY′, undergo supervised feature extraction using partial least squares, yielding the feature matrices VX, VY, VX′, and VY′ for fusion. L and L′ represent the training set and test set images. Additionally, L and L′ correspond to the fused features, while P′ and F′ in their fusion formulae represent two different modal features, respectively. The major contributions of the paper are:
i. Introducing a novel approach that addresses challenges in multi-modal palmprint and palm vein feature extraction. The method employs a sparse unsupervised projection algorithm with a "purification matrix" constraint, ensuring consistent intra-modal features in a shared expression space, minimizing data reconstruction errors, and enhancing feature representations.
ii. In this article, we utilized the partial least squares algorithm to extract high grayscale variance and category correlation subspaces from each modality. This promotes intra-modal representation consistency, improves the exploration of correlations among multi-modal samples, and thereby enhances recognition performance.
iii. We introduce a weighted sum strategy for dynamically optimizing each modality's contribution to classification recognition. Experimental evaluations on five multimodal databases validate its suitability for high-security identity recognition scenarios.
In summary, the contributions include a new feature-level fusion method, enhanced feature extraction, optimized inter-modal relationships, and effective fusion of palmprint and palm vein features, resulting in substantial improvements in recognition performance for multi-modal identity recognition scenarios.
In the realm of multimodal biometric recognition, the fusion of palmprint and palm vein features offers significant advantages. However, most of the existing multi-modal palmprint and palm vein feature extraction methods only extract feature information independently from different modalities, ignoring the importance of the correlation between different modal samples in the class to the improvement of recognition performance.
Existing biometric fusion recognition methods can be categorized into those based on traditional methods and those based on deep learning methods. In the realm of traditional methods, a study [11] proposed a cross-spectral matching system that extracts palmprint and palm vein features from the near-infrared (NIR) and visible light (RGB) spectral bands. Local binary coding is applied to palmprint features, and the NIR palm vein template is matched with the registered RGB palmprint template, with the fusion of similarity scores to enhance recognition performance. Another study [12] employed a kernel-based approach to extract facial features, Hough transform, and Daugman algorithm for left and right iris features, and Gabor filter banks for features of two thumbprints. The feature vectors are then mapped to the Reproducing Kernel Hilbert Space, followed by dimensionality reduction for feature fusion. In a different approach, a study [13] utilized low and high-frequency wavelet sub-bands to extract local and global information from palmprints and faces. A nearest-neighbor classifier is employed for sub-band recognition, and weighted majority voting is used to fuse the obtained categories. Last, a research effort [14] captured multimodal data from the same region of the hand using a single device. To obtain correlated information, fingerprint and finger vein features are decomposed into shared and private features, aiming to enhance complementarity.
In recognition methods based on deep learning, a study [15] proposed a wavelet-based fusion strategy for processing palmprint and palm vein images. Subsequently, deep scattering convolutional networks were employed for feature extraction and recognition. Another work [16] utilized a deep Hashing network (DHN) to extract binary templates for palmprint and palm vein authentication. This approach employed a spatial transformer network to overcome rotation and misalignment issues. By fusing features from different spectra, the method leveraged its advantages and improved recognition performance for fusion recognition. Addressing iris, palm vein, and finger vein modalities, a study [17] introduced a hybrid fusion model that captures typical features through a multi-ensemble structure. It utilized the distribution information of scores to assist decision-making, enhancing recognition accuracy and security. Additionally, a research effort [18] proposed a spatial and temporal multimodal fingerprint and finger vein network named FS-STMFPFV-Net, based on fingerprint and finger vein modalities. Independent learning for the two channels was achieved, enhancing resistance to image variations, and feature selection was performed using ReliefFS.
The traditional methods mentioned above use projection, encoding, and extraction of local global information to extract features, but ignore the importance of maximizing the correlation between different modal samples within a class during feature extraction to make the features more stable and discriminative, and to make the features more consistent in a common expression space for modal fusion recognition performance enhancement. On the other hand, deep learning methods, by increasing the depth of the feature extraction network, enhance the saliency of important features, making them more discriminative. However, this comes at the cost of increased complexity in the network model.
In this research, we employ a sparse unsupervised projection method constrained by a "purification matrix" to enhance intramodal features, minimizing data reconstruction errors and bolstering discriminative capabilities. Following this, the partial least squares algorithm discerns significant gray-scale variance and category relevance within each modality. A weighted sum is then applied to optimize each modality's contribution, ensuring precise classification results.
We summarize the paper as follows. Section 3 describes the derivation of the methods mentioned in this article. Section 4 creates five multimodal databases and performs performance experiments on the method. Section 5 summarizes the article.
In the image preprocessing stage, palmprint and palm vein images undergo denoising, contour extraction, determination of intersections and valley points, delineation of regions, and extraction of regions of interest (ROI) [19]. The ROIs obtained are of size 128pixel×128pixel. To achieve optimal recognition performance within a database containing 200 to 500 individuals, it is necessary to perform non-overlapping block-wise dimensionality reduction on the palmprint and palm vein ROI images.
In the feature extraction stage, due to the influence of environmental conditions, pose variations, and noise interference during image acquisition, it is necessary to suppress and eliminate various redundant information to the maximum extent in the feature extraction process. This ensures that the intra-class features are expressed with greater distinctiveness and consistency. The partial least squares (PLS) method, as a traditional feature extraction technique, excels in maximizing the correlation between the input feature matrix and identity information labels to extract features relevant to individual identity recognition. However, in the feature dimensionality reduction process, the PLS method does not adequately consider the sparsity of features, resulting in the extraction of features with potential redundancy. This leads to less compact feature representations, making it challenging to accurately differentiate between individuals. Additionally, the PLS method lacks a noise elimination mechanism during the feature extraction process, making it susceptible to noise interference in image data. This can hinder the improvement of intra-modal correlation and subsequently affect recognition performance.
To enhance the intra-class correlation between different modal samples, we propose a method called sparse unsupervised projection with partial least squares (SUPLS), introducing a sparse unsupervised projection (SUP) method with a "purification matrix". The "purification matrix" serves as a matrix aimed at eliminating noise information in image data while retaining useful information. Each element in the feature space of the "purification matrix" represents the weight or contribution of each feature in the samples. This method is applied to process the dimensionality-reduced image features. Through the "purification matrix", the dimensionality-reduced image features maintain sparsity while eliminating noise, preserving compact and distinctive feature representations, ensuring that intra-class features are more consistent in a shared expression space. Assuming the biological feature matrix is denoted as X and the "purification matrix" as F, the purified biological feature matrix XF is obtained through matrix multiplication. When selecting F, it is subjected to sparse constraints to ensure that the weights of noise information approach zero, thereby enhancing the saliency of important features.
The generation of the "purification matrix" is achieved by introducing constraints in the SUP method. Constraints are added during the projection process to minimize data reconstruction errors, eliminate noise, and ensure the consistency of intra-class feature representation.
The SUP method can maintain the sparsity of the output image features and extract compact and distinctive feature representations. Through sparse projection, it effectively eliminates noise interference in the data, improving the robustness and stability of features. Under the constraints of the SUP method, the PLS method is employed to extract supervised, dimensionality-reduced, and purified features. This fully utilizes individual identity information, addressing noise and redundant information interference. The method maximizes the correlation between the input feature matrix and identity information labels, thereby extracting features with discriminative and correlated characteristics. The specific derivation of the method is as follows:
Based on the unsupervised feature extraction method, we propose an optimization by introducing a weight allocation method in the sample space to capture the similarity between sample points. The weight allocation of sample points in the original sample space is represented as follows:
∑ni,j=1‖yi−yj‖22sij=2Tr(YLYT) | (1) |
where X=[x1,x2,...,xn]∈Rd×n represents the sample matrix, d represents the number of features, and n represents the number of samples. Y=PTX and yi=PTxi are the sample matrix and the ith sample after dimensionality reduction, respectively. Tr denotes the trace operation of the matrix, P∈Rd×d′(d′<d) is the projection matrix, d′ denotes the subspace dimension, and sij denotes the similarity between xi and xj. Building upon this, a "purification matrix" F∈Rn×d′ is introduced to eliminate noise in the data while preserving useful information. Here, λ represents the regularization parameter, and a higher value of λ indicates greater similarity between F and the projection matrix P, resulting in less noise removal. St=XTHX represents the global scatter matrix, while ‖P‖2,0=k denotes the number of non-zero rows in the projection matrix, which is equal to k. The representation is as follows:
minPTP=I,F12∑ni,j=1‖yi−yj‖22sij+λ‖XTP−F‖2F | (2) |
minPTP=I,‖P‖2,0=k,FTr(FTLF)+λ‖XTP−F‖2FTr(PTStP) | (3) |
minP,FTr(FTLF)+λTr(PTXXTP−2FPTX+FFT)Tr(PTStP)s.t.PTP=I,‖P‖2,0=k | (4) |
Upon performing dimensionality reduction and purification of the ROI image under the sparse unsupervised constraint with the "purification matrix", we obtain the corresponding subspace sample features F and the corresponding projection matrix P. The test image is then sparsely projected using the projection matrix P to obtain its subspace features. The subspace features of the training and test set images, which have undergone dimensionality reduction and purification under the sparse unsupervised constraint, are taken as the dependent variable matrix Y, while the image-category information is considered the independent variable matrix X. The relationship between the two is established to extract more discriminative features. The specific derivation is as follows:
In the context of biometric feature extraction, the independent variable matrix X consists of p image samples, and the dependent variable matrix Y consists of the corresponding class labels of the p image samples, denoted as X=(x1, x2,⋯,xp)TandY=(y1,y2,⋯,yp)T, respectively. Here, xt(t=1,2,⋯,p) represents the column vector formed by the t-th image. We extract the first pair of components from the variables X and Y, which are a linear combination of X and Y:
T1 = w11X1+w12X2+⋯+w1pXp=wT1X | (5) |
U1=v11Y1+v12Y2+⋯+v1qYq=vT1Y | (6) |
where T1 and U1 carry as much information as possible about the variantion in their respective matrices, and both need to satisfy the following conditions in order to maximize their correlation.
MAX(cov(T1,U1))=√var(T1)var(U1)r(T1,U1) | (7) |
In the above equation, cov(.) represents the covariance operator, var(.) represents the variance operator, and r(.) represents the correlation coefficient operator. Then, the score vectors for the first pair of components are calculated based on the standardized observation matrices X and Y, and linear regression is performed on the score vectors. The relationship model between X and Y is represented as follows:
Y=β1X1+β2X2+⋯+βmXm+Ym | (8) |
The above set of feature vectors β1,β2,⋯,βm represents a set of coordinate coefficients. The ROI image undergoes projection onto a set of vectors, resulting in coordinates that signify its position in the subspace. These coordinates form the basis for subsequent classification.
After feature extraction by the SUPLS method, the palmprint feature vector S and the palm vein feature vector Z in the multimodal image database can be obtained. In order to eliminate the adverse effects of the numerical imbalance between the two sets of features on the feature-level fusion, S and Z are standardized, respectively: Let S′=S/‖S‖, Z′=Z/‖Z‖, S and Z are transformed into unit vectors, S′ and Z′ are the standardized two modal features, and the double vertical lines represent the 2-norm. The combined feature vectors are as follows:
L=[ωZ′(1−ω)S′] | (9) |
where ω represents the weight, indicating the contribution of different modalities in the fusion process. The optimal value can be determined through experiments in the multimodal image database. After obtaining the fused feature, matching is performed by calculating the Euclidean distance between the p-th feature vector Lp and the q-th feature vector Lq extracted from the database. This distance can be denoted as:
Distancepq=|Lp−Lq| | (10) |
If the following condition is met:
Distance<t | (11) |
If it is determined that this pair of fused features originates from the same individual, it is accepted; otherwise, it is rejected. The parameter t represents a pre-defined threshold.
After conducting a search, it was discovered that there are limited publicly available databases capable of accommodating multiple hand-based features from the same individual simultaneously, such as palmprint, palm vein, fingerprint, knuckle pattern, finger vein, hand shape, etc. Therefore, we construct five multimodal databases comprising hand-based features, utilizing four unimodal palm vein databases and two unimodal palmprint databases. Table 1 provides the specific details of the utilized unimodal databases.
Databases | Traits | Subject | Sample | Total |
CASIA-P | Palmprint | 200 | 6 | 1200 |
Tongji-P | Palmprint | 600 | 20 | 12000 |
Tongji-V [20] | Palm-vein | 600 | 20 | 12000 |
CASIA-V [21] | Palm-vein | 200 | 6 | 1200 |
PolyU-NIR [22] | Palm-vein | 250 | 6 | 1500 |
Self-built | Palm-vein | 530 | 10 | 5300 |
The multimodal database CASIA-PV comprises two publicly available unimodal databases, derived from the CASIA multispectral palmprint database, for the extraction of palmprint and palm vein features from images captured at 460nm and 850nm wavelengths, respectively. These databases are then combined to form the new database, CASIA-PV. CASIA-P and CASIA-V contain palmprint and palm vein features obtained from the left and right hands of 100 individuals. Each individual has 6 images, and the features from the left and right hands are treated as separate individuals, resulting in a total of 1200 images for CASIA-P and CASIA-V. The region of interest (ROI) size is 128pixel×128pixel.
The multimodal database Tongji-PV consists of two publicly available unimodal databases: Tongji-P, which employs contact-based palmprint image acquisition and includes 12,000 palm vein images from 600 individuals, and Tongji-V, which adopts non-contact palm vein image acquisition with a light source wavelength of 940 nm and includes 12,000 palm vein images from 600 individuals aged between 20 and 50. The ROI region size for both databases is 128pixel×128pixel.
The multimodal database NIR-CAP consists of the PolyU-NIR (Hong Kong Polytechnic University Multispectral Palm Vein Database) and the CASIA-P (CASIA Palm Vein Database). The PolyU-NIR database acquires palm vein images under near-infrared (NIR) illumination, using a CCD camera and a high-power halogen light source as the contact-based acquisition device. The NIR-CAP database contains two databases, each with 200 individuals, and 6 samples per individual. The ROI region size is 128pixel×128pixel.
The multimodal database Self-built-CAP consists of a self-built database (Self-built) and a CASIA-P palmprint database (CASIA-P). The Self-built database was used to acquire non-contact palm pulse images from the left hand of 530 individuals aged between 20 and 50 years, with 10 images per individual, resulting in a total of 5300 images. The Self-built-CAP database contains two databases, each with 200 individuals, and 6 samples per individual. The ROI region size is 128pixel×128pixel.
The multimodal database NIR-TP contains the Hong Kong Polytechnic University multispectral palmprint database (PolyU-NIR) and the Tongji University palmprint database (Tongji-P). It consists of a total of 250 individuals from each of the two database, with 6 samples per individual and an ROI region size of 128pixel×128pixel. Table 2 presents the details of the used multimodal database.
Databases | Subject | Sample | Total | Image size |
CASIA-PV | 200 | 6 | 2400 | 128 × 128 |
Tongji-PV | 500 | 6 | 6000 | 128 × 128 |
NIR-CAP | 200 | 6 | 2400 | 128 × 128 |
Self-built-CAP | 200 | 6 | 2400 | 128 × 128 |
NIR-TP | 250 | 6 | 3000 | 128 × 128 |
To evaluate the accuracy of the proposed hand feature fusion recognition method, the training and test subsets are selected from each of the six multimodal databases, with approximately 50% of individuals randomly chosen from each database. The individuals in the training and test subsets are disjointed. Feature extraction and feature fusion are performed simultaneously on the test subset, followed by classification and matching with the training subset.
Recognition performance was evaluated using metrics such as the false rejection rate (FRR), false accept rate (FAR), correct recognition rate (CRR) [23], equal error rate (EER), and receiver operating characteristic (ROC) curves [24]. CRR is defined as:
CRR=NumberofexperimentsforcorrectidentificationofresultsTotalnumberofidentificationexperiments×100% |
EER is a comprehensive indicator of the performance of an identification system. In general, a smaller EER indicates superior performance in the identification system. The ROC curve visually depicts the variations in FAR and FRR as the discrimination threshold changes.
Before joint feature selection and extraction of sparse unsupervised projections, the image is first chunked to reduce the dimensionality, and we ensure that the major features are highlighted while reducing the number of image features. The sample dimension d1 after sparse processing and subspace denoising, the number of features selected k1, and the number of feature primes k generated after the image has been processed by partial least squares are adjusted, provided that the size of the chunks is in the range of 2 × 2, 4 × 4, and 8 × 8.
Based on findings from literature [25], it is recommended to choose the sample dimension (d1) and the number of features (k1) with the same value. Experimental results indicate that maintaining these values equal maximizes the positive impact on the overall procedure. In experiments, it is ensured that both values remain consistent and do not surpass the original sample dimension of the image. Similarly, from the literature [23], it can be seen that for chunking and dimensionality reduction in an image of size 128 × 128, when the chunking is 2 × 2, the chunking is too small, which will lead to a large amount of redundant information in the image features. This significantly increases the recognition time and incomplete dimensionality reduction. When the chunking is 8 × 8, the main texture information in the image features cannot be fully highlighted because the chunking is too large, leading to a lower recognition accuracy. Therefore, the 4 × 4 chunking standard was used for the experiment. The following parametric experiments were carried out using a Self-built database.
As the number of master elements (k) increases, the recognition rate gradually improves. The dimensionality reduction technique uses a 4 × 4 chunking standard, resulting in image features of size 32 × 32 after reduction. The number of sample dimensions (d1) and the number of selected features (k1) need to be controlled within the image sample dimensions, with 1000 being close to the upper limit. Table 3 shows that within this range, when the number of master elements reaches 300, the EER is 0.0028%, and the CRR is 100%. However, as the number of elements reaches 1000, further increases will lead to an increase in the number of features and a decrease in program efficiency.
Principal element (k) | Subspace sample dimension(d1)/ Number of feature selection(k1) | |||||||
400 | 500 | 600 | 700 | 800 | 900 | 1000 | ||
50 | EER (%) | 6.2743 | 6.2083 | 6.4043 | 6.6044 | 6.2124 | 6.4740 | 6.4043 |
CRR (%) | 97.45 | 97.62 | 96.76 | 97.54 | 98.45 | 96.81 | 96.55 | |
100 | EER (%) | 6.4043 | 6.2273 | 6.3418 | 6.4753 | 6.1839 | 6.2870 | 6.0895 |
CRR (%) | 97.65 | 96.66 | 97.25 | 97.65 | 96.99 | 98.86 | 96.6912 | |
150 | EER (%) | 3.3378 | 3.0536 | 2.7010 | 2.6702 | 2.7333 | 2.5918 | 2.5367 |
CRR (%) | 97.21 | 97.66 | 98.13 | 97.44 | 97.34 | 97.95 | 97.73 | |
200 | EER (%) | 0.8011 | 0.7907 | 0.5337 | 0.4680 | 0.4789 | 0.4090 | 0.4052 |
CRR (%) | 99.25 | 99.41 | 99.41 | 99.48 | 99.44 | 99.69 | 99.72 | |
250 | EER (%) | 0.2588 | 0.1366 | 0.1333 | 0.1334 | 0.1250 | 0.0787 | 0.0765 |
CRR (%) | 99.84 | 99.91 | 99.92 | 99.97 | 99.97 | 99.97 | 99.97 | |
300 | EER (%) | 0.0725 | 0.0444 | 0.0191 | 0.0129 | 0.0056 | 0.0045 | 0.0028 |
CRR (%) | 99.97 | 99.98 | 99.99 | 99.99 | 99.99 | 99.99 | 100.00 |
For the proposed method SUPLS in this paper, feature ablation experiments and module ablation experiments were conducted to verify the performance from PLS-based feature-level fusion recognition and SUPLS-based unimodal recognition, respectively, in which the EER display order was palm vein/palmprint in the unimodal experiments of SUPLS. The experimental results are shown in Tables 4 and 5 below.
Databases | CASIA-PV | Tongji-PV | NIR-CAP | Self-built-CAP | NIR-TP |
EER (%) | 0.8870/0.6667 | 0.8623/0.9447 | 0.5070/0.6667 | 0.4179/0.6667 | 0.8664/0.2810 |
Databases | CASIA-PV | Tongji-PV | NIR-CAP | Self-built-CAP | NIR-TP |
EER (%) | 0.2633/0.1123 | 0.7585/0.6667 | 0.1000/0.2633 | 0.5600/0.2633 | 0.1742/0.2400 |
To evaluate the performance of the proposed SUPLS method, we test it on a multimodal database and compares its performance with several classical methods commonly used in palmprint and palm vein recognition, including DCT_fusion [26], Pca+Lpp, DBM [27], 2DLDA [28], PLS [29], and JPCDA [30]. For the aforementioned methods, the palmprint and palm vein features from the multimodal database were extracted independently. The modal features were then normalized, and a weight-based fusion method was applied to obtain new fused features. The recognition performance was evaluated, and the experimental results are presented in Table 6 below. The ROC curves representing the recognition performance of the previously mentioned methods in the five multimodal fusion galleries are presented in Figures 3 to 7 below. In the four multimodal databases, our method improves the EER by 0.1494%, 0.1511%, 0.0195%, 0.0132%, and 0.0029%, respectively, compared to the other methods, which are more effective (JPCDA). The performance compared to the remaining other methods can be visualized from the ROC graphs in Figures 3 to 7, and the performance effect of our method remains stable.
DCT_fusion | Pca+Lpp | DBM | 2DLDA | PLS | JPCDA | SUPLS | |
CASIA-PV | 34.3667 | 28.3417 | 25.6738 | 30.6333 | 0.6598 | 0.1667 | 0.0173 |
Tongji-PV | 0.6667 | 1.2068 | 0.5384 | 1.0133 | 0.4728 | 0.1703 | 0.0192 |
NIR-CAP | 25.5000 | 12.4865 | 2.2654 | 26.3000 | 0.4912 | 0.0254 | 0.0059 |
Self-built-CAP | 25.2000 | 16.1667 | 7.6318 | 8.3057 | 0.4144 | 0.0142 | 0.0010 |
NIR-TP | 1.5581 | 8.0669 | 0.2709 | 2.5765 | 0.3200 | 0.0037 | 0.0008 |
In this paper, we propose a joint learning-based feature-level fusion recognition method for palmprints and palm veins. This method initially employs a sparse unsupervised projection algorithm with a "purification matrix" constraint to process palmprint and palm vein region-of-interest images. Subsequently, the use of partial least squares algorithm extracts subspaces with high grayscale variance and high category correlation from each modality, promoting the consistency of intra-modal representations. Finally, a weighted sum is applied to fuse palmprint and palm vein features, dynamically optimizing the contribution of each modality for classification recognition. Experimental evaluations conducted on five multimodal databases, composed of six unimodal databases, including the Chinese Academy of Sciences multispectral palmprint and palm vein databases, yielded EER of 0.0173%, 0.0192%, 0.0059%, 0.0010%, and 0.0008%. Both the stability of palmprint features and the high security of palm pulse features are utilised to increase the anti-counterfeiting function of the system. It can be widely used in authentication and access control of confidential information in artificial intelligence restaurants, unmanned hotels, smart banks, smart medical care, smart communities, traffic security checks, and other fields.
The authors declare that they have not used Artificial Intelligence (AI) tools in the creation of this article.
This research was funded by the National Natural Science Foundation of China (61991413), the China Postdoctoral Science Foundation (2019M651142), the Natural Science Foundation of Liaoning Province(2021-KF-12-07), and the Natural Science Foundation of Liaoning Province (2023-MS-322).
The authors declare that there are no conflicts of interest.
[1] |
S. P. Zhao, L. K. Fei, J. Wen, Multiview-learning-based generic palmprint recognition: A literature review, Mathematics, 11 (2023), 1261–1261. https://doi.org/10.3390/math11051261 doi: 10.3390/math11051261
![]() |
[2] |
S. Y. Li, B. Zhang, Joint discriminative sparse coding for robust hand-based multimodal recognition, IEEE Trans. Inf. Forensics and Secur., 16 (2021), 3186–3198. https://doi.org/10.1109/TIFS.2021.3074315 doi: 10.1109/TIFS.2021.3074315
![]() |
[3] | K. Zhang, H. Wang, C. Yu, M. Du, L. Tao, Class constraint-based discriminative features learning algorithm for palm print and palm vein fusion recognition, in 2022 7th International Conference on Signal and Image Processing (ICSIP), (2022), 275–280. https://doi.org/10.1109/ICSIP55141.2022.9886189 |
[4] | H. S. Kala, S. Kumar, R. B. Reddy, N. Shastry, R. Thakur, Contactless authentication device using palm vein and palm print fusion biometric technology for post covid world, in 2021 International Conference on Design Innovations for 3Cs Compute Communicate Control (ICDI3C), (2021), 281–285. https://doi.org/10.1109/ICDI3C53598.2021.00063 |
[5] | T. R. Yashavanth, M. Suresh, Performance analysis of multimodal biometric system using LBP and PCA, in 2023 International Conference on Recent Trends in Electronics and Communication (ICRTEC), (2023), 1–5. https://doi.org/10.1109/ICRTEC56977.2023.10111925 |
[6] | S. Li, B. Zhang, L. Wu, R. Ma, X. Ning, Robust and sparse least square regression for finger vein and finger knuckle print recognition, IEEE Trans. Inf. Forensics Secur., (2024), 2709–2719. https://doi.org/10.1109/TIFS.2024.3352389 |
[7] |
T. Wu, L. Leng, M. K. Khan, F. A. Khan, Palmprint-palmvein fusion recognition based on deep hashing network, IEEE Access, 9 (2021), 135816–135827. https://doi.org/10.1109/ACCESS.2021.3112513 doi: 10.1109/ACCESS.2021.3112513
![]() |
[8] | L. G. Oldal, A. Kovács, Hand geometry and palmprint-based authentication using image processing, in 2020 IEEE 18th International Symposium on Intelligent Systems and Informatics (SISY), (2020), 125–130. https://doi.org/10.1109/SISY50555.2020.9217068 |
[9] | C. Ramachandran, D. Sankar, Score level based fusion method for multimodal biometric recognition using palmprint and Iris, in 2020 Advanced Computing and Communication Technologies for High Performance Applications (ACCTHPA), (2020), 281–286. https://doi.org/10.1109/ACCTHPA49271.2020.9213216 |
[10] |
N. Bala, R. Gupta, A. Kumar, Multimodal biometric system based on fusion techniques: A review, Inf. Secur. J., 31(2022), 289–337. https://doi.org/10.1080/19393555.2021.1974130 doi: 10.1080/19393555.2021.1974130
![]() |
[11] |
S. C. Cho, B. S. Oh, K. A. Toh, Z. P. Lin, Extraction and cross-matching of palm-vein and palmprint from the RGB and the NIR spectrums for identity verification, IEEE Access, 8(2020), 4005–4021. https://doi.org/10.1109/ACCESS.2019.2963078 doi: 10.1109/ACCESS.2019.2963078
![]() |
[12] | H. S. Mohammad, A. D. Mohammad, S. Hamed, A hybrid approach for multimodal biometric recognition based on feature level fusion in reproducing Kernel Hilbert Space, in 2022 30th International Conference on Electrical Engineering (ICEE), (2022), 796–802. https://doi.org/10.1109/ICEE55646.2022.9827154 |
[13] | D. V. R. Devi, K. N. Rao, Decision level fusion schemes for a multimodal biometric system using local and global wavelet features, in 2020 IEEE International Conference on Electronics, Computing and Communication Technologies (CONECCT), (2020), 1–6. http://dx.doi.org/10.1109/conecct50063.2020.9198547 |
[14] | W. L. Yang, J. D. Huang, D. Luo, W. X. Kang, Efficient disentangled representation learning for multi-modal finger biometrics, Pattern Recognit., 145 (2024). https://doi.org/10.1016/j.patcog.2023.109944 |
[15] | H. S. Kala, K. Shreya, B. R. Rakshanda, S. Nishanth, T. Rounak, Contactless authentication device using palm vein and palmprint fusion biometric technology for post covid world, in 2021 International Conference on Design Innovations for 3Cs Compute Communicate Control (ICDI3C), (2021), 281–285. https://doi.org/10.1109/ICDI3C53598.2021.00063 |
[16] |
T. F. Wu, L. Leng, M. K. Khan, F. A. Khan, Palmprint-palmvein fusion recognition based on deep hashing network, IEEE Access, 9 (2021), 135816–135827. https://doi.org/10.1109/ACCESS.2021.3112513 doi: 10.1109/ACCESS.2021.3112513
![]() |
[17] |
C. Y. Zhou, J. Huang, F. Yang, Y. Q. Liu, A hybrid fusion model of iris, palm vein and finger vein for multi-biometric recognition system, Multimedia Tools Appl., 79 (2020), 1–22. https://doi.org/10.1007/s11042-020-08914-6 doi: 10.1007/s11042-020-08914-6
![]() |
[18] | S. A. Bala, Z. B. Abdullahi, C. Ponlawat, A. Muhammad, Sequence-wise multimodal biometric fingerprint and finger-vein recognition network (STMFPFV-Net), Intell. Syst. Appl., 19 (2023). https://doi.org/10.2139/ssrn.4432356 |
[19] |
W. Wu, Q. Wang, S. Q. Yu, Q. Luo, S. Lin, Z. Han, Y. D. Tang, Outside box and contactless palm vein recognition based on a wavelet denoising ResNet, IEEE Access, 9 (2021), 82471–82484. https://doi.org/10.1109/ACCESS.2021.3086811 doi: 10.1109/ACCESS.2021.3086811
![]() |
[20] | L. Zhang, L. Li, A. Yang, Y. Shen, M. Yang, Towards contactless palmprint recognition: A novel device, a new benchmark, and a collaborative representation based identification approach, Pattern Recognit., 69 (2017), 199–212. https://doi.org/10.1016/j.patcog.2017.04.016 |
[21] |
Y. Zhou, A. Kumar, Human identification using palm-vein images, IEEE Trans. Inf. Forensics Secur., 6 (2011), 1259–1274. https://doi.org/10.1109/TIFS.2011.2158423 doi: 10.1109/TIFS.2011.2158423
![]() |
[22] |
D. Zhang, Z. Guo, G. M. Lu, L. Zhang, W. M. Zuo, An online system of multispectral palmprint verification, IEEE Trans. Instrum. Measurement, 59 (2010), 480–490. https://doi.org/10.1109/TIM.2009.2028772 doi: 10.1109/TIM.2009.2028772
![]() |
[23] | W. Q. Yuan, W. Wu, S. Lin, H. Song, H. T. Zhang, Non-contact biometric recognition of palm vein based on block and partial least squares, Chinese J. Sci. Instrum., 34 (2013), 31–39. |
[24] |
B. Jay, K. Ajay, On estimating performance indices for biometric identification, Pattern Recognit., 42 (2009), 1803–1815. https://doi.org/10.1016/j.patcog.2008.10.004 doi: 10.1016/j.patcog.2008.10.004
![]() |
[25] |
J. Y. Wang, L. Wang, F. P. Nie, X. L. Li, Joint feature selection and extraction with sparse unsupervised projection, IEEE Trans. Neural Networks Learn. Syst., 34 (2021), 3071–3081. https://doi.org/10.1109/TNNLS.2021.3111714 doi: 10.1109/TNNLS.2021.3111714
![]() |
[26] |
E. Alhatami, U. A. Bhatti, M. X. Huang, S. L. Feng, Review and enhancement of discrete cosine transform (DCT) for medical image fusion, Springer Nat. Singapore, 614 (2023), 89–97. http://dx.doi.org/10.1007/978-981-19-9331-2_8 doi: 10.1007/978-981-19-9331-2_8
![]() |
[27] |
J. Almaghtuf, F. Khelifi, A. Bouridane, Fast and efficient difference of block means code for palmprint recognition, Mach. Vision Appl., 31 (2020), 478–487. https://doi.org/10.1007/s00138-020-01103-3 doi: 10.1007/s00138-020-01103-3
![]() |
[28] |
G. Manas, D. Aniruddha, Fractional-weighted entropy-based fuzzy G-2DLDA algorithm: A new facial feature extraction method, Multimedia Tools Appl., 82 (2022), 2689–2707. https://doi.org/10.1007/s11042-022-13328-7 doi: 10.1007/s11042-022-13328-7
![]() |
[29] |
A. Zainab, W. L. Jong, A. K. Muhammad, S. Muhammad, A. K. Sajid, R. Naveed, Optical character recognition (OCR) using partial least square (PLS) based feature reduction: an application to artificial intelligence for biometric identification, J. Enterp. Inf. Manage., 36 (2020), 767–789. https://doi.org/10.1108/JEIM-02-2020-0076 doi: 10.1108/JEIM-02-2020-0076
![]() |
[30] |
X. W. Zhao, J. Guo, F. P. Nie, L. Chen, Z. H. Li, H. X. Zhang, Joint principal component and discriminant analysis for dimensionality reduction, IEEE Trans. Neural Networks Learn. Syst., 31 (2020), 433–444. https://doi.org/10.1109/TNNLS.2019.2904701 doi: 10.1109/TNNLS.2019.2904701
![]() |
1. | Hou Tiantian, Jia Wei, Zhao Yang, Chuan Qin, Huiyu Zhou, 2024, Enhanced histogram of oriented lines for palmprint recognition, 9781510681514, 117, 10.1117/12.3035349 |
Databases | Traits | Subject | Sample | Total |
CASIA-P | Palmprint | 200 | 6 | 1200 |
Tongji-P | Palmprint | 600 | 20 | 12000 |
Tongji-V [20] | Palm-vein | 600 | 20 | 12000 |
CASIA-V [21] | Palm-vein | 200 | 6 | 1200 |
PolyU-NIR [22] | Palm-vein | 250 | 6 | 1500 |
Self-built | Palm-vein | 530 | 10 | 5300 |
Databases | Subject | Sample | Total | Image size |
CASIA-PV | 200 | 6 | 2400 | 128 × 128 |
Tongji-PV | 500 | 6 | 6000 | 128 × 128 |
NIR-CAP | 200 | 6 | 2400 | 128 × 128 |
Self-built-CAP | 200 | 6 | 2400 | 128 × 128 |
NIR-TP | 250 | 6 | 3000 | 128 × 128 |
Principal element (k) | Subspace sample dimension(d1)/ Number of feature selection(k1) | |||||||
400 | 500 | 600 | 700 | 800 | 900 | 1000 | ||
50 | EER (%) | 6.2743 | 6.2083 | 6.4043 | 6.6044 | 6.2124 | 6.4740 | 6.4043 |
CRR (%) | 97.45 | 97.62 | 96.76 | 97.54 | 98.45 | 96.81 | 96.55 | |
100 | EER (%) | 6.4043 | 6.2273 | 6.3418 | 6.4753 | 6.1839 | 6.2870 | 6.0895 |
CRR (%) | 97.65 | 96.66 | 97.25 | 97.65 | 96.99 | 98.86 | 96.6912 | |
150 | EER (%) | 3.3378 | 3.0536 | 2.7010 | 2.6702 | 2.7333 | 2.5918 | 2.5367 |
CRR (%) | 97.21 | 97.66 | 98.13 | 97.44 | 97.34 | 97.95 | 97.73 | |
200 | EER (%) | 0.8011 | 0.7907 | 0.5337 | 0.4680 | 0.4789 | 0.4090 | 0.4052 |
CRR (%) | 99.25 | 99.41 | 99.41 | 99.48 | 99.44 | 99.69 | 99.72 | |
250 | EER (%) | 0.2588 | 0.1366 | 0.1333 | 0.1334 | 0.1250 | 0.0787 | 0.0765 |
CRR (%) | 99.84 | 99.91 | 99.92 | 99.97 | 99.97 | 99.97 | 99.97 | |
300 | EER (%) | 0.0725 | 0.0444 | 0.0191 | 0.0129 | 0.0056 | 0.0045 | 0.0028 |
CRR (%) | 99.97 | 99.98 | 99.99 | 99.99 | 99.99 | 99.99 | 100.00 |
Databases | CASIA-PV | Tongji-PV | NIR-CAP | Self-built-CAP | NIR-TP |
EER (%) | 0.8870/0.6667 | 0.8623/0.9447 | 0.5070/0.6667 | 0.4179/0.6667 | 0.8664/0.2810 |
Databases | CASIA-PV | Tongji-PV | NIR-CAP | Self-built-CAP | NIR-TP |
EER (%) | 0.2633/0.1123 | 0.7585/0.6667 | 0.1000/0.2633 | 0.5600/0.2633 | 0.1742/0.2400 |
DCT_fusion | Pca+Lpp | DBM | 2DLDA | PLS | JPCDA | SUPLS | |
CASIA-PV | 34.3667 | 28.3417 | 25.6738 | 30.6333 | 0.6598 | 0.1667 | 0.0173 |
Tongji-PV | 0.6667 | 1.2068 | 0.5384 | 1.0133 | 0.4728 | 0.1703 | 0.0192 |
NIR-CAP | 25.5000 | 12.4865 | 2.2654 | 26.3000 | 0.4912 | 0.0254 | 0.0059 |
Self-built-CAP | 25.2000 | 16.1667 | 7.6318 | 8.3057 | 0.4144 | 0.0142 | 0.0010 |
NIR-TP | 1.5581 | 8.0669 | 0.2709 | 2.5765 | 0.3200 | 0.0037 | 0.0008 |
Databases | Traits | Subject | Sample | Total |
CASIA-P | Palmprint | 200 | 6 | 1200 |
Tongji-P | Palmprint | 600 | 20 | 12000 |
Tongji-V [20] | Palm-vein | 600 | 20 | 12000 |
CASIA-V [21] | Palm-vein | 200 | 6 | 1200 |
PolyU-NIR [22] | Palm-vein | 250 | 6 | 1500 |
Self-built | Palm-vein | 530 | 10 | 5300 |
Databases | Subject | Sample | Total | Image size |
CASIA-PV | 200 | 6 | 2400 | 128 × 128 |
Tongji-PV | 500 | 6 | 6000 | 128 × 128 |
NIR-CAP | 200 | 6 | 2400 | 128 × 128 |
Self-built-CAP | 200 | 6 | 2400 | 128 × 128 |
NIR-TP | 250 | 6 | 3000 | 128 × 128 |
Principal element (k) | Subspace sample dimension(d1)/ Number of feature selection(k1) | |||||||
400 | 500 | 600 | 700 | 800 | 900 | 1000 | ||
50 | EER (%) | 6.2743 | 6.2083 | 6.4043 | 6.6044 | 6.2124 | 6.4740 | 6.4043 |
CRR (%) | 97.45 | 97.62 | 96.76 | 97.54 | 98.45 | 96.81 | 96.55 | |
100 | EER (%) | 6.4043 | 6.2273 | 6.3418 | 6.4753 | 6.1839 | 6.2870 | 6.0895 |
CRR (%) | 97.65 | 96.66 | 97.25 | 97.65 | 96.99 | 98.86 | 96.6912 | |
150 | EER (%) | 3.3378 | 3.0536 | 2.7010 | 2.6702 | 2.7333 | 2.5918 | 2.5367 |
CRR (%) | 97.21 | 97.66 | 98.13 | 97.44 | 97.34 | 97.95 | 97.73 | |
200 | EER (%) | 0.8011 | 0.7907 | 0.5337 | 0.4680 | 0.4789 | 0.4090 | 0.4052 |
CRR (%) | 99.25 | 99.41 | 99.41 | 99.48 | 99.44 | 99.69 | 99.72 | |
250 | EER (%) | 0.2588 | 0.1366 | 0.1333 | 0.1334 | 0.1250 | 0.0787 | 0.0765 |
CRR (%) | 99.84 | 99.91 | 99.92 | 99.97 | 99.97 | 99.97 | 99.97 | |
300 | EER (%) | 0.0725 | 0.0444 | 0.0191 | 0.0129 | 0.0056 | 0.0045 | 0.0028 |
CRR (%) | 99.97 | 99.98 | 99.99 | 99.99 | 99.99 | 99.99 | 100.00 |
Databases | CASIA-PV | Tongji-PV | NIR-CAP | Self-built-CAP | NIR-TP |
EER (%) | 0.8870/0.6667 | 0.8623/0.9447 | 0.5070/0.6667 | 0.4179/0.6667 | 0.8664/0.2810 |
Databases | CASIA-PV | Tongji-PV | NIR-CAP | Self-built-CAP | NIR-TP |
EER (%) | 0.2633/0.1123 | 0.7585/0.6667 | 0.1000/0.2633 | 0.5600/0.2633 | 0.1742/0.2400 |
DCT_fusion | Pca+Lpp | DBM | 2DLDA | PLS | JPCDA | SUPLS | |
CASIA-PV | 34.3667 | 28.3417 | 25.6738 | 30.6333 | 0.6598 | 0.1667 | 0.0173 |
Tongji-PV | 0.6667 | 1.2068 | 0.5384 | 1.0133 | 0.4728 | 0.1703 | 0.0192 |
NIR-CAP | 25.5000 | 12.4865 | 2.2654 | 26.3000 | 0.4912 | 0.0254 | 0.0059 |
Self-built-CAP | 25.2000 | 16.1667 | 7.6318 | 8.3057 | 0.4144 | 0.0142 | 0.0010 |
NIR-TP | 1.5581 | 8.0669 | 0.2709 | 2.5765 | 0.3200 | 0.0037 | 0.0008 |