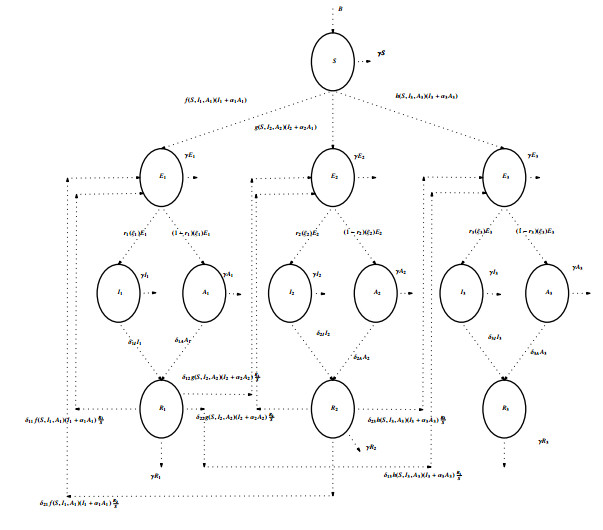
We investigate the behavior of a complex three-strain model with a generalized incidence rate. The incidence rate is an essential aspect of the model as it determines the number of new infections emerging. The mathematical model comprises thirteen nonlinear ordinary differential equations with susceptible, exposed, symptomatic, asymptomatic and recovered compartments. The model is well-posed and verified through existence, positivity and boundedness. Eight equilibria comprise a disease-free equilibria and seven endemic equilibrium points following the existence of three strains. The basic reproduction numbers R01, R02 and R03 represent the dominance of strain 1, strain 2 and strain 3 in the environment for new strain emergence. The model establishes local stability at a disease-free equilibrium point. Numerical simulations endorse the impact of general incidence rates, including bi-linear, saturated, Beddington DeAngelis, non-monotone and Crowley Martin incidence rates.
Citation: Manoj Kumar Singh, Anjali., Brajesh K. Singh, Carlo Cattani. Impact of general incidence function on three-strain SEIAR model[J]. Mathematical Biosciences and Engineering, 2023, 20(11): 19710-19731. doi: 10.3934/mbe.2023873
[1] | Arturo J. Nic-May, Eric J. Avila-Vales . Global dynamics of a two-strain flu model with a single vaccination and general incidence rate. Mathematical Biosciences and Engineering, 2020, 17(6): 7862-7891. doi: 10.3934/mbe.2020400 |
[2] | Matthew D. Johnston, Bruce Pell, David A. Rubel . A two-strain model of infectious disease spread with asymmetric temporary immunity periods and partial cross-immunity. Mathematical Biosciences and Engineering, 2023, 20(9): 16083-16113. doi: 10.3934/mbe.2023718 |
[3] | James M. Hyman, Jia Li . Differential susceptibility and infectivity epidemic models. Mathematical Biosciences and Engineering, 2006, 3(1): 89-100. doi: 10.3934/mbe.2006.3.89 |
[4] | Yanxia Dang, Zhipeng Qiu, Xuezhi Li . Competitive exclusion in an infection-age structured vector-host epidemic model. Mathematical Biosciences and Engineering, 2017, 14(4): 901-931. doi: 10.3934/mbe.2017048 |
[5] | Maia Martcheva, Mimmo Iannelli, Xue-Zhi Li . Subthreshold coexistence of strains: the impact of vaccination and mutation. Mathematical Biosciences and Engineering, 2007, 4(2): 287-317. doi: 10.3934/mbe.2007.4.287 |
[6] | Miller Cerón Gómez, Felipe Alves Rubio, Eduardo Ibarguen Mondragón . Qualitative analysis of generalized multistage epidemic model with immigration. Mathematical Biosciences and Engineering, 2023, 20(9): 15765-15780. doi: 10.3934/mbe.2023702 |
[7] | Abba B. Gumel, Baojun Song . Existence of multiple-stable equilibria for a multi-drug-resistant model of mycobacterium tuberculosis. Mathematical Biosciences and Engineering, 2008, 5(3): 437-455. doi: 10.3934/mbe.2008.5.437 |
[8] | Xue-Zhi Li, Ji-Xuan Liu, Maia Martcheva . An age-structured two-strain epidemic model with super-infection. Mathematical Biosciences and Engineering, 2010, 7(1): 123-147. doi: 10.3934/mbe.2010.7.123 |
[9] | Yoichi Enatsu, Yukihiko Nakata . Stability and bifurcation analysis of epidemic models with saturated incidence rates: An application to a nonmonotone incidence rate. Mathematical Biosciences and Engineering, 2014, 11(4): 785-805. doi: 10.3934/mbe.2014.11.785 |
[10] | Azmy S. Ackleh, Keng Deng, Yixiang Wu . Competitive exclusion and coexistence in a two-strain pathogen model with diffusion. Mathematical Biosciences and Engineering, 2016, 13(1): 1-18. doi: 10.3934/mbe.2016.13.1 |
We investigate the behavior of a complex three-strain model with a generalized incidence rate. The incidence rate is an essential aspect of the model as it determines the number of new infections emerging. The mathematical model comprises thirteen nonlinear ordinary differential equations with susceptible, exposed, symptomatic, asymptomatic and recovered compartments. The model is well-posed and verified through existence, positivity and boundedness. Eight equilibria comprise a disease-free equilibria and seven endemic equilibrium points following the existence of three strains. The basic reproduction numbers R01, R02 and R03 represent the dominance of strain 1, strain 2 and strain 3 in the environment for new strain emergence. The model establishes local stability at a disease-free equilibrium point. Numerical simulations endorse the impact of general incidence rates, including bi-linear, saturated, Beddington DeAngelis, non-monotone and Crowley Martin incidence rates.
The mathematical model is formulated to protect the environment and ease living beings' lives. Although humans have evolved to fight any circumstance, a state like an outbreak or pandemic still gets critically on our nerves. The biological reasons responsible for the spread of infection must be noted to decrease the hosts' mortality. The wheel of an infection period considers every aspect of the biological, social and physical environment. The genetic core is also a factor to be considered. The SIR (susceptible, infectious and recovered) compartmental model with a homogeneous host population was first studied by McKendrick and Kermack in 1927 [1]. Further, the McKendrick model was improved by adding an exposed compartment for the individuals during an incubation period. An incubation period is when the carrier does not show the symptoms of the infection. We have focused on the SEIAR model with two infectious compartments, including symptomatic and asymptomatic classes [2,3,4]. Symptomatic is the class of carriers comprising people showing symptoms within or after the incubation period. On the other hand, the asymptomatic class never shows any symptoms.
Strain is biologically defined as a phenotypically/genotypically distinctive group of isolates that depends on the typing scheme due to the emergence of strains and host immune changes [5,6]. Malaria, tuberculosis, dengue, influenza, Coronavirus and others have different variants. These variants are like plant branches that are somewhat different but have the same base properties. Variants may act differently in terms of resistance, rate of spread and vaccines. Biological data assures that the host's genetic changes and their response to infectious diseases could cause the genome sequencing of the infection. The multi-strain model [7,8,9,10,11] considers more than one variant of the infection, considering strains can coexist or compete with each other for their existence [4,12]. Here, we are considering three variants of the concerned, alpha, beta and delta, of the Coronavirus for the validation of our mathematical model [13,14,15].
Inter-agency assemblies like the Centers for Disease Control and Prevention (CDC) and the World Health Organization (WHO) focus on the quick classification of emergent mutations of viruses to observe the possible effect of viruses. To estimate the danger posed by variants of infectious diseases on the earth and recommend appropriate measures, experts estimate and measure the existing data to calculate the severity of the disease and capability of a blowout in hosts. The variants are categorized as variants being monitored (VBM), variant of interest (VOI), variant of concern (VOC) and variant of high consequence (VOHC) [16,17]. VBM comprise those variants whose statistics are directly connected to a severe infection or have amplified spread but are detected to have no long severity. These variants may have a low spread rate at this time. A VOI is a variant whose mutation has caused significant variation widely in the susceptible class. A VOI grows into a VOC if the spread rate increases and causes a devastating effect of the disease, while a VOHC is a stage when WHO informs other organizations about the infection's spread. A lineage is a cluster of related viruses with a common predecessor [18].
The incidence rate is the frequency of new infections of any disease considering susceptible populations arising in a certain period. The importance of the incidence rate and different kinds of nonlinear incidence functions [19,20,21,22,23] is formulated to explain and discuss the transmission rate of the infection amongst the host [24,25]. The global dynamics of the mathematical model for the bilinear [26,27,28,29], saturated [28,29,31], Beddington DeAngelis [32,33], fractional, non-monotonic [27] and Crowley Martin incidence function [34] was studied through SIR and SEIR models. Some mathematical models are studied with homogeneous mixture incidence function, while others consider heterogeneous mixture incidence function. O. Khyar and K. Allali formulated the SEIR model with two strains considering non-monotone and general incidence rates [27,35,36,37,38]. The main objective of the three-strain model with the generalized incidence function is to cover the class of all mentioned incidence rates and study the effect of incidence rate function (The choice of incidence rate plays an important role in the mathematical model) [39,40].
The model includes the vital dynamics where B is the recruitment rate, and 1/γ is the average life expectancy of the population. The basic model contains five variables, Si (susceptible), Ei (expected), Ii (infected), Ai (asymptotic) and Ri (recovered), where i=1,2 and 3 according to strain 1, strain 2 and strain 3, respectively. α1,α2 and α3 are the ratios of disease transmission rate by asymptomatic class of strain 1, strain 2 and strain 3, respectively. ξ1,ξ2 and ξ3 are the reciprocals of the latent/incubation periods for strain 1, strain 2 and strain 3, respectively. δ11,δ21,δ12,δ22,δ13,δ23 are multiplicative factors for δij, where i represents the rate of infection of individual Ri in recovery class by strain j. r1,r2 and r3 are the rates at which exposed individuals become symptomatic with strain 1, strain 2 and strain 3, respectively. δ1I,δ2I,δ3I,δ1A,δ2A,δ3A are the recovery rates of infectious and asymptomatic classes in accordance with the strains as mentioned in the subscripts of δ. f(S,I1,A1),g(S,I2,A2), and h(S,I3,A3) are generalized incidence functions which are assumed to be continuously differentiable in the interior of R3+ and satisfy the properties (2.1, 2.2 and 2.3). It is assumed in the mathematical model that at t=0 there was no infection in the host population. After the emergence of an infection, the first virus of concern is the first strain when t≤t1. At time t1, the new virus of concern, the second strain, emerges, for t1<t≤t2. At time t2, the third virus of concern is said to emerge. The emerging strain re-infection is not considered. [41]
f(0,I1,A1)=0,g(0,I2,A2)=0,h(0,I3,A3)=0,for allIj,Aj≥0,j=1,2,3. | (2.1) |
∂f(S,I1,A1)∂S>0,∂g(S,I2,A2)∂S>0,∂h(S,I3,A3)∂S>0,for allS>0,Ij,Aj≥0,j=1,2,3. | (2.2) |
∂f(S,I1,A1)∂I1≤0,∂g(S,I2,A2)∂I2≤0,∂h(S,I3,A3)∂I3≤0,for all S≥0,IjAj≥0,j=1,2,3. | (2.3) |
dSdt=B−f(S,I1,A1)(I1(t)+α1A1(t))−g(S,I2,A2)(I2(t)+α2A2(t))−h(S,I3,A3)(I3(t)+α3A3(t))−γS(t),dE1dt=f(S,I1,A1)(I1(t)+α1A1(t))+δ11f(S,I1,A1)(I1(t)+α1A1(t))R1(t)S(t)+δ21f(S,I1,A1)(I1(t)+α1A1(t))R2(t)S(t)−ξ1E1(t)−γE1(t),dI1dt=r1(ξ1)E1(t)−δ1II1(t)−γI1(t),dA1dt=(1−r1)(ξ1)E1(t)−δ1AA1(t)−γA1(t),dR1dt=δ1II1(t)+δ1AA1(t)−δ11f(S,I1,A1)(I1(t)+α1A1(t))R1(t)S(t)−δ12g(S,I2,A2)(I2(t)+α2A2(t))R1(t)S(t)−δ13h(S,I3,A3)(I3(t)+α3A3(t))R1(t)S(t)−γR1(t),dE2dt=g(S,I2,A2)(I2(t)+α2A2(t))+δ12g(S,I2,A2)(I2(t)+α2A2(t))R1(t)S(t)+δ22g(S,I2,A2)(I2(t)+α2A2(t))R2(t)S(t)−ξ2E2(t)−γE2(t),dI2dt=r2(ξ2)E2(t)−δ2II2(t)−γI2(t),dA2dt=(1−r2)(ξ2)E2(t)−δ2AA2(t)−γA2(t),dR2dt=δ2II2(t)+δ2AA2(t)−δ21f(S,I1,A1)(I1(t)+α1A1(t))R2(t)S(t)−δ22g(S,I2,A2)(I2(t)+α2A2(t))R2(t)S(t)−δ23h(S,I3,A3)(I3(t)+α3A3(t))R2(t)S(t)−γR2(t),dE3dt=h(S,I3,A3)(I3(t)+α3A3(t))+δ13h(S,I3,A3)(I3(t)+α3A3(t))R1(t)S(t)+δ23h(S,I3,A3)(I3(t)+α3A3(t))R2(t)S(t)−ξ3E3(t)−γE3(t),dI3dt=r3(ξ3)E3(t)−δ3II3(t)−γI3(t),dA3dt=(1−r3)(ξ3)E3(t)−δ3AA3(t)−γA3(t),dR3dt=δ3II3(t)+δ3AA3(t)−γR3(t).} | (2.4) |
According to the assumptions, the compartmental model (2.4) has all its associated parameters and variables non-negative. The model to fulfill its purpose, must satisfy the well-posedness, for which the existence, positivity and boundedness of the solution with the seed conditions, defined in the Theorem (1), are necessary.
Theorem 1. Let the seed conditions S0=(S(0),E1(0),I1(0),A1(0), R1(0), E2(0),I2(0),A2(0),R2(0),E3(0),I3(0), A3(0),R3(0)) be non-negative. Then, the solution ¯S={(S(t),E1(t),I1(t),A1(t),R1(t),E2(t),I2(t), A2(t),R2(t),E3(t),I3(t),A3(t),R3(t))} of the system of equations of model (2.4) exists, is non-negative and is bounded.
That is, ¯Π={¯S∈R13+,0≤N(t)≤max{N(0)+Bγ}} is the positively invariant feasible region.
Proof. The existence of the unique local solution of the mathematical model (2.4) can be stated by the fundamental theory of ordinary differential equations. To show the non-negativity of the solution, we must prove that any solution initiating from the non-negative region/orthant of R13+={¯S∈R13:S0≥0} resides in it globally.
Let us define
¯T=sup{t≥0:S0≥0}∈[0,t]. | (3.1) |
Claim that ¯T=+∞. Let us assume that ¯T is finite, so with the solution's property of being continuous, S(¯T)=0 or E1(¯T)=0 or I1(¯T)=0 or A1(¯T)=0 or R1(¯T)=0 or E2(¯T)=0 or I2(¯T)=0 or A2(¯T)=0 or R2(¯T)=0 or E3(¯T)=0 or I3(¯T)=0 or A3(¯T)=0 or R3(¯T)=0.
Let us assume S(¯T)=0 before any other variables become zero. Hence,
dS(¯T)dt=limt→¯T−S(¯T)−S(t)¯T−t=limt→¯T−−S(t)¯T−t≤0. | (3.2) |
From the leading equation of mathematical model (2.4) and Eq (2.1), we have
dS(¯T)dt=B−f(S(0),I1(¯T),A1(¯T))(I1(¯T)+α1A1(¯T))−g(S(0),I2(¯T),A2(¯T))(I2(¯T)+α2A2(¯T))−h(S(0),I3(¯T),A3(¯T))(I3(¯T)−α3A3(¯T))−γS(¯T)=B−f(0,I1(¯T),A1(¯T))(I1(¯T)+α1A1(¯T))−g(0,I2(¯T),A2(¯T))(I2(¯T)+α2A2(¯T))−h(0,I3(¯T),A3(¯T))(I3(¯T)+α3A3(¯T))−γS(0)=B>0. | (3.3) |
This contradicts the above Eq (3.2). Now, assuming E1(¯T) as zero, before any other variables become zero. Hence,
dE1(¯T)dt=limt→¯T−E1(¯T)−E1(t)¯T−t=limt→¯T−−E1(t)¯T−t≤0. | (3.4) |
From the second equation of mathematical model (2.4) and Eq (2.2), we have
dE1(¯T)dt=f(S,I1,A1)(I1(¯T)+α1A1(¯T))+δ11f(S,I1,A1)(I1(¯T)+α1A1(¯T))R1(¯T)S(¯T)+δ21f(S,I1,A1)(I1(¯T)+α1A1(¯T))R2(¯T)S(¯T)−ξ1E1(¯T)−γE1(¯T)=f(S,I1,A1)(I1(¯T)+α1A1(¯T))+δ11f(S,I1,A1)(I1(¯T)+α1A1(¯T))R1(¯T)S(¯T)+δ21f(S,I1,A1)(I1(¯T)+α1A1(¯T))R2(¯T)S(¯T)>0. | (3.5) |
This contradicts the above Eq (3.4). Similarly, we can find for I1,A1,R1, E2,I2,A2,R2,I3,A3,R3. Hence, ¯T could not have a finite value. Thus, the positivity of the solution of model (2.4) is confirmed.
For boundedness of the solution, consider the total population N(t) that is equal to the sum of all the compartments of the mathematical model. The time derivative of the total population found with the help of system of Eq (2.4) is
dN(t)dt=B−γN(t). | (3.6) |
Therefore,
N(t)=Bγ+(N(0)−Bγ)e(−γt). | (3.7) |
For time 0≤t<∞, we find that N(t)≤Bγ+N(0). This states the boundedness of N(t), which confirms the boundedness of ¯S. Hence, the local solution of the mathematical model (2.4) is established to have uniqueness, non-negativity and boundedness property globally.
This subsection studies the basic reproduction number. The basic reproduction denoted as R0 is the number measuring the secondary infections caused due to one susceptible host in the entire period of infection [42]. It plays a crucial role to decide the risk behind any disease to bloom out. We determine the basic reproduction number by the next generation matrix FV−1 where F is the Jacobian matrix of the new infections at time t2, with the co-existence of all the three strains together. The matrix V is the Jacobian matrix of infection transfer to other classes that is non-singular at time t2. The spectral radius of the next generation matrix results in the basic reproduction number. The three-strain epidemic model has three eigenvalues of the next generation matrix R01, R02 and R03. The basic reproduction number R0 here is the maximum of the three for the co-existence of all the three strains at time t2 at an endemic equilibrium point (˜S,~E1,~I1,~A1,~R1,~E2,~I2,~A2,~R2,~E3,~I3,~A3,~R3).
F=(0F1F20000000000000000000000000000G1G20000000000000000000000000000H1H2000000000000000000), |
V=(m100000000−r1ξ1m20000000ξ1(r1−1)0m3000000000m400000000−r2ξ2m50000000ξ2(r2−1)0m6000000000m700000000−r3ξ3m80000000ξ3(r3−1)0m9). |
Here,
F1=(f(˜S,~I1,~A1)+∂f(˜S,~I1,~A1)∂I1(~I1+α1~A1))(˜S+δ11~R1+δ21~R2)˜S, |
F2=(α1f(˜S,~I1,~A1)+∂f(˜S,~I1,~A1)∂A1(~I1+α1~A1))(˜S+δ11~R1+δ21~R2)˜S, |
G1=(g(˜S,~I2,~A2)+∂g(˜S,~I2,~A2)∂I2(~I2+α2~A2))(˜S+δ12~R1+δ22~R2)˜S, |
G2=(α2g(˜S,~I2,~A2)+∂g(˜S,~I2,~A2)∂A2(~I2+α2~A2))(˜S+δ12~R1+δ22~R2)˜S, |
H1=(h(˜S,~I3,~A3)+∂h(˜S,~I3,~A3)∂I3(~I3+α3~A3))(˜S+δ13R1+δ23R2)˜S, |
H2=(α3h(˜S,~I3,~A3)+∂h(˜S,~I3,~A3)∂A3(~I3+α3~A3))(˜S+δ13~R1+δ23~R2)˜S, |
m1=ξ1+γ,m2=δ1I+γ,m3=δ1A+γ,m4=ξ2+γ,m5=δ2I+γ, |
m6=δ2A+γ,m7=ξ2+γ,m8=δ3I+γ,m9=δ3A+γ. |
We know that ρ(FV−1)=R0=max{R01,R02,R03}, with ρ as spectral radius and R01,R02,R03 values as in Eq (3.8). Here, the R0 represents the emergence of the third strain in the environment.
R01=ξ1(F1m3r1+F2α1m2(1−r1))m1m2m3,R02=ξ2(G1m6r2+G2α2m5(1−r2))m4m5m6,R03=ξ3(H1m9r3+H2α3m8(1−r3))m7m8m9. | (3.8) |
R01=RI01+RA01,R02=RI02+RA02,R03=RI03+RA03,RI01=F1ξ1m3r1m1m2m3,RA01=F2ξ1m2(1−r1)m1m2m3,RI02=G1ξ2m6r2m4m5m6,RA02=G2ξ2m5(1−r2)m4m5m6,RI03=H1ξ3m9r3m7m8m9,RA03=H2ξ3m8(1−r3)m7m8m9. |
The basic reproduction number at the DFE=E0 is denoted as R(E0)=max{R01(E0),R02(E0),R03(E0)}. It describes the spread of infections by an individual at the initial stage.
R01(E0)=f(Bγ,0,0)ξ1(m3r1+α1m2(1−r1))m1m2m3,R02(E0)=g(Bγ,0,0)ξ2(m6r2+α2m5(1−r2))m4m5m6,R03(E0)=h(Bγ,0,0)ξ3(m9r3+α3m8(1−r3))m7m8m9. | (3.9) |
Theorem 2. The mathematical model (2.4) has disease free equilibrium E0 and seven endemic equilibrium points ϵs1,ϵs2,ϵs3,ϵs12,ϵs23, ϵs13, ϵs123. These endemic equilibrium points exists in accordance with some conditions. [27,43]
(i) The ϵs1 is the first strain equilibrium which exists when R01>1.
(ii) The ϵs2 is the second strain equilibrium which exists when R02>1.
(iii) The ϵs3 is the third strain equilibrium which exists when R03>1.
(iv) The ϵs12 is the endemic equilibrium which exists when R01>1 and R02>1.
(v) The ϵs23 is the endemic equilibrium which exists when R02>1 and R03>1.
(vi) The ϵs13 is the endemic equilibrium which exists when R01>1 and R03>1.
(vii) The ϵs123 is the endemic equilibrium which exists when R01>1,R02>1 and R03>1.
Proof. For calculating the steady states of the mathematical model (2.4), we equate dSdt,dE1dt,dI1dt,dA1dt,dR1dt,dE2dt,dI2dt,dA2dt,dR2dt,dE3dt,dI3dt,dA3dt and dR3dt to zero. The disease free equilibrium is the stage when it is considered that there is zero infection in the environment, also assumed as t=0 stage. The case when there is no infection is I1=0, A1=0, I2=0, A2=0, I3=0 and A3=0. Hence, the equilibrium point is named as the disease free equilibrium, denoted as E0=(Bγ,0,0,0,0,0,0,0,0,0,0,0,0).
(ⅰ) The case when there is an infection due to first strain, that is, I1≠0, A1≠0, I2=0, A2=0, I3=0 and A3=0. Here, the endemic equilibrium is ϵs1=(Ss1,Es1,1,Is1,1,As1,1,Rs1,1,0,0,0,0,0,0,0,0). Here, Ss1=Bγ−f(Ss1,Is1,1,As1,1)(Is1,1+α1As1,1)γ, Es1,1=(B−γSs1)(Ss1+δ11Rs1,1)Ss1m1, Is1,1=r1ξ1m2Es1,1, As1,1=(1−r1)ξ1m3Es1,1, Rs1,1=(δ1IIs1,1+δ2AAs1,1)Ss1δ11(B−γSs1)+γSs1.
(ⅱ) The case when there is an infection due to second strain, that is, I1=0, A1=0, I2≠0, A2≠0, I3=0 and A3=0. Here, the endemic equilibrium is ϵs2=(Ss1,0,0,0,0,Es2,2,Is2,2,As2,2,Rs2,2,0,0,0,0). Here, Ss2=Bγ−g(Ss2,Is2,2,As2,2)(Is2,2+α2As2,2)γ, Es2,2=(B−γSs2)(Ss2+δ22Rs2,2)Ss2m4, Is2,2=r2ξ2m5Es2,2, As2,2=(1−r2)ξ2m6Es2,2, Rs2,2=(δ2IIs2,2+δ2AAs2,2)Ss2δ22(B−γSs2)+γSs2.
(ⅲ) The case when there is an infection due to third strain in the environment, that is, I1=0, A1=0, I2=0, A2=0, I3≠0 and A3≠0. Here, the endemic equilibrium is ϵs3=(Ss3,0,0,0,0,0,0,0,0,Es3,3,Is3,3,As3,3,Rs3,3). Here, Ss3=Bγ−h(Ss3,Is3,3,As3,3)(Is3,3+α3As3,3)γ, Es3,3=(B−γSs3)m4, Is3,3=r3ξ3m8Es3,3, As3,3=(1−r3)ξ3m9Es3,3, Rs3,3=(δ3IIs3,3+δ3AAs3,3)Ss3γ.
(ⅳ) The case when there is an infection due to the first and second strain surviving in the environment that is, I1≠0, A1≠0, I2≠0, A2≠0, I3=0 and A3=0. Here, the endemic equilibrium is ϵs12=(Ss12,Es12,1,Is12,1, As12,1,Rs12,1,Es12,2,Is12,2,As12,2,Rs12,2,0,0,0,0). Here, Ss12=Bγ−f(Ss12,Is12,1,As12,1)(Is12,1+α1As12,1)γ−g(Ss12,Is12,2,As12,2)(Is12,2+α2As12,2)γ, Es12,1=f(Ss12,Is12,1,As12,1)(Ss12+δ11Rs12,1+δ21Rs12,2)(Is12,1+α1As12,1)Ss12m1, Is12,1=r1ξ1m2Es12,1, As12,1=(1−r1)ξ1m3Es12,1, Rs12,1=(δ1IIs12,1+δ2AAs12,1)Ss12δ11f(Ss12,Is12,1,As12,1)(Is12,1+α1As12,1)+δ12g(Ss12,Is12,2,As12,2)(Is12,2+α2As12,2)+γSs1, Es12,2=g(Ss12,Is12,2,As12,2)(Ss12+δ12Rs12,1+δ22Rs12,2)(Is12,2+α2As12,2)Ss12m4, Is12,2=r2ξ2m5Es12,2, As12,2=(1−r2)ξ2m6Es12,2, Rs12,2=(δ2IIs12,2+δ2AAs12,2)Ss2δ21f(Ss12,Is12,1,As12,1)(Is12,1+α1As12,1)+δ22g(Ss12,Is12,2,As12,2)(Is12,2+α2As12,2)+γSs12.
(ⅴ) The case when there is an infection due to first and second strain surviving in the environment, that is, I1=0, A1=0, I2≠0, A2≠0, I3≠0 and A3≠0. Here, the endemic equilibrium is ϵs23=(Ss23,0,0,0,0,Es23,2,Is23,2,As23,2,Rs23,2,Es23,3,Is23,3,As23,3,Rs23,3). Here, Ss23=Bγ−g(Ss23,Is23,2,As23,2)(Is23,2+α2As23,2)γ−h(Ss23,Is23,3,As23,3)(Is23,3+α3As23,3)γ, Es23,2=g(Ss23,Is23,2,As23,2)(Ss23+δ22Rs23,2)(Is23,2+α2As23,2)Ss23m4, Is23,2=r2ξ2m5Es23,2, As23,2=(1−r2)ξ2m6Es23,2, Rs23,2=(δ2IIs23,2+δ2AAs23,2)Ss23δ22g(Ss23,Is23,2,As23,2)(Is23,2+α2As23,2)+δ23h(Ss23,Is23,1,As23,1)(Is23,3+α3As23,3)+γSs23, Es23,3=h(Ss23,Is23,3,As23,3)(Ss23+δ23Rs23,2)(Is23,3+α3As23,3)Ss23m7, Is23,3=r3ξ3m8Es23,3, As23,1=(1−r3)ξ3m9Es23,3, Rs23,3=δ3IIs23,3+δ3AAs23,3γ.
(ⅵ) The case when there is an infection due to first and second strain surviving in the environment, that is, I1≠0, A1≠0, I2=0, A2=0, I3≠0 and A3≠0. Here, the endemic equilibrium is ϵs13=(Ss13,Es13,1,Is13,1, As13,1,Rs13,1,0,0,0,0,Es13,3,Is13,3,As13,3,Rs13,3). Here, Ss13=Bγ−f(Ss13,Is13,1,As13,1)(Is13,1+α1As13,1)γ−h(Ss13,Is13,3,As13,3)(Is13,3+α3As13,3)γ, Es13,1=f(Ss13,Is13,1,As13,1)(Ss13+δ11Rs13,1)Ss13m1, Is13,1=r1ξ1m2Es13,1, As13,1=(1−r1)ξ1m3Es13,1, Rs13,1=(δ1IIs13,1+δ2AAs13,1)Ss13δ11f(Ss13,Is13,1,As13,1)(Is13,1+α1As13,1)+δ13h(Ss13,Is13,3,As13,3)(Is13,3+α3As13,3)+γSs13, Es13,3=h(Ss13,Is13,3,As13,3)(Ss13+δ13Rs13,2)Ss13m7, Is13,3=r3ξ3m8Es13,3, As13,1=(1−r3)ξ3m9Es13,3, Rs13,3=δ3IIs13,3+δ3AAs13,3γ.
(ⅶ) The case when there is infection due to first, second and third strain in the environment, that is, I1≠0, A1≠0, I2≠0, A2≠0, I3≠0 and A3≠0. Here, the endemic equilibrium is ϵs123=(˜S,~E1,~I1,~A1,~R1,~E2,~I2,~A2,~R2,~E3,~I3,~A3,~R3). Here, ˜S=Bγ−f(˜S,~I1,~A1)(~I1+α1~A1)γ−g(˜S,~I2,~A2)(~I2+α2~A2)γ−h(˜S,~I3,~A3)(~I3+α3~A3)γ, ~E1=f(˜S,~I1,~A1)(˜S+δ11~R1+δ21~R2)(~I1+α1~A1)˜Sm1, ~I1=r1ξ1m2~E1, ~A1=(1−r1)ξ1m3~E1, ~R1=(δ1I~I1+δ1A~A1)˜Sδ11f(˜S,~I1,~A1)(~I1+α1~A1)+δ12g(˜S,~I2,~A2)(~I2+α2~A2)+δ13h(˜S,~I3,~A3)(~I3+α3~A3)+γ˜S, ~E2=g(˜S,~I2,~A2)(˜S+δ12~R1+δ22~R2)(~I2+α2~A2)˜Sm4, ~I2=r2ξ2m5~E2, ~A2=(1−r2)ξ2m6~E2, ~R2=(δ2I~I2+δ2A~A2)˜Sδ21f(˜S,~I1,~A1)(~I1+α1~A1)+δ22g(˜S,~I2,~A2)(~I2+α2~A2)+δ23h(˜S,~I3,~A3)(~I3+α3~A3)+γ˜S, ~E3=h(˜S,~I3,~A3)(˜S+δ13~R1+δ23~R2)(~I3+α3~A3)˜Sm7, ~I3=r3ξ3m8~E3, ~A3=(1−r3)ξ3m9~E3, ~R3=δ3I~I3+δ3A~A3γ.
This subsection discusses the local stability of disease free equilibrium (DFE). The Jacobian matrix of order thirteen of the system (2.4) is in Eq (3.10).
A=(ai,j),where i≤13,j≤13. | (3.10) |
The appendix section contains all the non zero terms of the matrix A. It is hard to determine the further dynamics with the matrix A due to the course of constraints.
Theorem 3. The disease free equilibrium (DFE) of the mathematical model (2.4) is locally asymptotic stable if the basic reproduction R(E0) is less than one and unstable for greater than one.
Proof. The Jacobian JDFE of the mathematical model (2.4) at the initial stage when t=0, the infection is not spread (disease free equilibrium) is,
(−γ0−F−α1F00−G−α2G00−H−α3H00−m1Fα1F0000000000ξ1r1−m200000000000ξ1(1−r1)0−m300000000000δIδA−γ0000000000000−m4Gα2G0000000000ξ2r2−m500000000000ξ2(1−r2)0−m600000000000δ2Iδ2A−γ0000000000000−m7Hα3H0000000000ξ3r3−m800000000000ξ3(1−r3)0−m900000000000δ3Iδ3A−γ). |
The above matrix JDFE has the characteristic polynomial as,
(γ+λ)4(α1(−F)ξ1(r1−1)(λ+m2)−(λ+m3)(−Fξ1r1+λ2+λm2+m1(λ+m2)))(α2(−G)ξ2(r2−1)(λ+m5)−(λ+m6)(−Gξ2r2+λ2+λm5+m4(λ+m5)))(α3(−H)ξ3(r3−1)(λ+m8)−(λ+m9)(−Hξ3r3+λ2+λm8+m7(λ+m8))) | (3.11) |
Here, F=f(Bγ,0,0),G=g(Bγ,0,0),H=h(Bγ,0,0). From the characteristic polynomial, four eigenvalues of Eq (3.11) are real and negative, and the other nine are the zeros of Eq (3.12).
(A0λ3+A1λ2+A2λ+A3)(B0λ3+B1λ2+B2λ+B3)(C0λ3+C1λ2+C2λ+C3)=0 | (3.12) |
Here, A0=1,A1=m1+m2+m3,A2=m1m2(1−RI01)+m1m3(1−RA01)+m2m3,A3=m1m2m3(1−R01),B0=1,B1=m4+m5+m6,B2=m4m6(1−RA02)+m4m5(1−RI02)+m5m6,B3=m4m5m6(1−R02),C0=1,C1=m7+m8+m9,C2=m7m9(1−RA03)+m7m8(1−RI03)+m8m9, and C3=m7m8m9(1−R03).
By the Liénard Chipart criterion, a polynomial has all its zeros with negative real part if A0>0,A1>0,A2>0,A3>0,B0>0,B1>0,B2>0,B3>0,C0>0,C1>0,C2>0,C3>0,A1A2−A0A3>0,B1B2−B0B3>0, and C1C2−C0C3>0. It is clear that the conditions hold if R01<1,R02<1 and R02<1.
As A1A2−A0A3=(m21(m3(1−RA01)+m2(1−RI01)) +m1(m3m2(−RA01−RI01+R01+2)+m23(1−RA01)+m22(1−RI01))+m2m3 (m2+m3)), B1B2−B0B3=(m24(m6(1−RA02)+m5(1−RI02))+m4(m6m5(−RA02−RI02+R02+2)+m26(1−RA02)+m25(1−RA02))+m5m6(m5+m6)), and C1C2−C0C3=(m27(m9(1−RA03)+m8(1−RI03))+m7(m9m8(−RA03−RI03+R03+2)+m29(1−RA03)+m28(1−RI03))+m8m9(m8+m9)).
Evidently all the thirteen eigenvalues of the Jacobian at disease free equilibrium have real part negative if and only if R01<1,R02<1 and R02<1. This indicates asymptotic local stability of mathematical model (2.4) with the basic reproduction number less than one and instability otherwise.
This section studies importance and the effect of each compartment with different incidence rates (transfer of the infection). Considering the three incidence functions f(S,I1,A1),g(S,I2,A2) and h(S,I3,A3) have an impact that cannot be neglected and further clarifies the need to choose the perfect incidence rate in the mathematical model.
Bilinear incidence rate β∗Susceptible is used when the susceptible compartment has direct linear impact on the transmission, and all other compartments play a negligible effect. Beddington DeAngelis function β∗Susceptible1+ζ1∗Susceptible+ζ2∗Infected+ζ3∗Asymptomatic shows the nonlinear impact of the infected, asymptomatic and susceptible class on the transmission of the disease. Non-monotone function β∗Susceptible1+ζ1∗Infected2 or β∗Susceptible1+ζ1∗Asymptomatic2, Crowley Martin function (β∗Susceptible)/(1+ζ1∗Susceptible+ζ2∗Infected+ζ3∗Asymptomatic+ζ1ζ2∗Infected∗Susceptible+ζ1∗ζ3∗Susceptible∗Asymptomatic+ζ2∗ζ3∗Infected∗Asymptomatic), saturated function β∗Susceptible1+ζ1∗Susceptible or β∗Susceptible1+ζ2∗Infected or β∗Susceptible1+ζ3∗Asymptomatic and specific nonlinear function (β∗Susceptible)/(1+ζ1∗Susceptible+ζ2∗Infected+ζ3∗Asymptomatic+ζ4∗Infected∗Susceptible+ζ5∗Susceptible∗Asymptomatic+ζ6∗Infected∗Asymptomatic) all describe the non-linear relationship of the susceptible, infected and asymptomatic compartments in one or another way. The definition of the parameter used in these incidence rates also makes them different in one or another way.
Figure 2 is the result of the simulation with initial values of the variables as {81000,7000,112,100,10,6000,203,105,60,5200,100,100,10} with hypothetical values of parameters as α1=0.79,α2=0.85,α3=0.78,γ=2,δ11=0.57,δ12=0.39,δ22=0.3,δ13=0.4,δ21=0.59,δ23=0.459,δ1I=0.52,δ1A=0.848,δ2I=0.94,δ2A=0.68,δ3I=0.88,δ3A=0.548,r1=0.7,r2=0.67,r3=0.7,ξ1=0.45,ξ2=0.29,ξ3=0.27,B=2∗N(0), and the parameters included for different choices of incidence rates (f(S,I1,A1),g(S,I2,A2) and h(S,I3,A3)) vary.
Figure 2(a) has parametric values as β1=0.88,β2=0.8 and β3=0.9 whose graphical plot shows how there is sudden decrease in the susceptible class S(t) and rise in the exposed class E3(t). The decrease in the exposed class of strain 1 and strain 2 is also observed while the infected class of the third strain rises. Figure 2(b) has the parametric values β1=0.8,β2=0.76,β3=0.88,ζ1=0.012,ζ2=0.152,ζ3=0.035,ζ4=0.012,ζ5=0.11,ζ6=0.032,ζ7=0.014,ζ8=0.022,ζ9=0.112. The decline in the number of individuals in the susceptible class and the wave in number of individuals in the exposed class are easily noticed at the time span of one of all strains. There is a rise in the number of infected and asymptomatic class individuals as well. Figure 2(c) has the parametric values β1=0.87,β2=0.79,β3=0.85,ζ1=0.018,ζ2=0.07,ζ3=0.0128. The line plot shows a sudden decrease in the susceptible class at time 0.1, and at this period exposed classes of all the strain rises, and a little jump in infected class of respected strains are observed. Figure 2(d) has the parametric values β1=0.77,β2=0.9,β3=0.75,ζ1=0.012,ζ2=0.02,ζ3=0.01 with the graphical plot similar to Figure 2(c) but large variation in the number of susceptible and exposed class individuals. Figure 2(e) has the parametric values β1=0.77,β2=0.9,β3=0.75,ζ1=0.03,ζ2=0.04,ζ3=0.135,ζ4=0.028 with the line plot observed to have a rapid decrease in the susceptible class at time 0.1, and at this period exposed classes of strain 1 rises. Also there is a jump in infected class and asymptomatic infected class of strain 1. Figure 2(f) has the parametric values β1=0.77,β2=0.8,β3=0.85,ζ1=0.016,ζ2=0.37,ζ3=0.02,ζ4=0.015,ζ5=0.024,ζ6=0.01388,ζ7=0.027,ζ8=0.0262,ζ9=0.0125,ζ10=0.012, and the plot has an increase in the susceptible class individuals and a fall in the exposed classes of all the strains for the same, and a negligible increase in the infected individuals is also observed. Figure 2(g) has the parametric values β1=0.787,β2=0.8,β3=0.87,ζ1=0.04,ζ2=0.03,ζ3=0.06261, and it is similar to Figure 2(f). Here, the plot indicates the certain increase in the susceptible class individuals from time 0.2 to 0.6 and decrement in the exposed classes of all the strains for the same. An increase in the infected individuals is also observed. Figure 2(h) has the parametric values β1=0.7,β2=0.85,β3=0.75,ζ1=0.033,ζ2=0.025,ζ3=0.016. The two-dimensional line plot shows a sudden decrease in the susceptible class at time almost near to zero, and at this period exposed classes of all the strain rise and a little jump in the number of individuals in the other classes of respected strains is marked. Figure 2(i) has the parametric values β1=0.88,β2=0.9,β3=0.92,ζ1=0.02,ζ2=0.25,ζ3=0.014 with the line plot observed to have a large decrease in the susceptible class at one third time of 0.2, and at this period exposed classes of all the strains rises. Huge variation in the exposed classes of the strains is noticed in the time span of zero to two. Also there is a jump in infected class and asymptomatic infected class of all strains. Figure 2(j) has the parametric values β1=0.8,β2=0.9,β3=0.88,ζ1=0.0327,ζ2=0.014,ζ3=0.035,ζ4=0.018,ζ5=0.016,ζ6=0.014,ζ7=0.01,ζ8=0.0145,ζ9=0.019,ζ10=0.014,ζ11=0.0217,ζ12=0.019,ζ13=0.0038,ζ14=0.014,ζ15=0.0156,ζ16=0.0245,ζ17=0.0128,ζ18=0.017 and is very largely similar to Figure 2(f), and the same dynamics are observed.
Figure 3 interprets the impact of the incidence rates according to the cases in Table 1 on the total number of infected individuals remarkably. Case (a), case (d), case (i), case (e) and case (h) shows the high rise in the number of infected classes. Meanwhile, case (g) and case (j) have a small rise. Case (c) and case (f) show an increase in the number of total infected individuals at the time of 0.25 and then decrease. Case (b) also increases the total number of infected individuals slowly from a time-span of zero to one. The importance of choosing an incidence rate could lead the mathematical model to interpret different scenarios clearly.
Case | f(S,I1,A1) | g(S,I2,A2) | h(S,I3,A3) |
(a) | β1S | β2S | β3S |
(b) | β1S1+ζ1S+ζ2I1+ζ3A1 | β2S1+ζ4S+ζ5I2+ζ6A2 | β3S1+ζ7S+ζ8I3+ζ9A3 |
(c) | β1S1+ζ1I21 | β2S1+ζ2I22 | β3S1+ζ3I23 |
(d) | β1S1+ζ1A21 | β2S1+ζ2A22 | β3S1+ζ3A23 |
(e) | β1S1+ζ1S | β2S1+ζ2S | β3S1+ζ3S |
(f) | β1S1+ζ1I1 | β2S1+ζ2I2 | β3S1+ζ3I3 |
(g) | β1S1+ζ1A1 | β2S1+ζ2A2 | β3S1+ζ3A3 |
(h) | β1S/(1+ζ1S+ζ2I1 | β2S/(1+ζ4S+ζ5I2 | β3S/(1+ζ7S+ζ8I3 |
+ζ3A1+ζ1ζ2SI1 | +ζ6A2+ζ4ζ5SI2 | +ζ9A3+ζ7ζ8SI3 | |
+ζ1ζ3SA1+ζ2ζ3I1A1) | +ζ5ζ6I2A2+ζ4ζ6SA2) | +ζ8ζ9I3A3+ζ7ζ9SA3) | |
(i) | β1S/(1+ζ1S+ζ2I1 | β2S/(1+ζ7S+ζ8I2 | β3S/(1+ζ13S+ζ14I3 |
+ζ3A1+ζ4SI1 | +ζ9A2+ζ10SI2 | +ζ15A3+ζ16SI3 | |
+ζ5SA1+ζ6I1A1) | +ζ11I2A2+ζ12SA2) | +ζ17I3A3+ζ18SA3) | |
(j) | β1S1+ζ1I1 | β2S1+ζ2S+ζ3I2+ζ4A2 | β3S1+ζ5A23 |
Predictions made with the mathematical models help to control the forthcoming dangerous situations and handle them smartly. An epidemiological model (2.4) has been formulated to understand the transmission behavior of the multi-strain model with respect to the choice of incidence rate function numerically. An evaluation of the following mathematical and epidemiological results of the proposed model has been discussed in the paper:
(i) The solutions of the system of thirteen differential Eq (2.4) is non-negative and bounded for all times t>0, when initial data is non-negative (Theorem (1)). Thus, the formulated mathematical model (2.4) is mathematically and epidemiologically well-posed in Section 2.
(ii) The three-strain epidemic model has the basic reproduction number R0, the maximum of the three R01, R02 and R03. R0 in Section 3.2. The reproduction number R0 defines the number of new infections at the time of emerging of a new strain. The three-strain model has a disease-free equilibrium (DFE) with local-asymptotic stability whenever the associated basic reproduction number R0 (E0) is lesser than a unit (Theorem 3). Biologically, if the reproduction number is less than a unit, an infectious host will get exposed to less than a host in the tenure of his infection, leading to contraction in the number of cases of the infection.
(iii) The proposed model has eight endemic equilibrium according to the strain influence and its impact in Section 3.2. The existence of the strains and their nature of co-existence and competence leads to the scenarios discussed in the Theorem (3).
(iv) The effect of incidence rate choice leads to different effects on the number of infected persons. Each incidence rate type has equal importance and definition defined in Section 4. Biologically in the transmission of the infection different compartments plays role differently that is described through incidence rate in the differential equation modeling. In both epidemic and virus dynamics models, this general incidence function can represent a wide range of potential incidence functions. The general incidence at which people transition from the class of susceptible individuals to the class of infective individuals has been the focus of such epidemiological models. These common incidences have primarily been modeled using functional responses of the bilinear and Holling types [21,29]. Such tactics may involve treatments, vaccines, isolation and educational campaigns for epidemic diseases [44,45]. Mathematical models are now crucial tools for understanding how infectious diseases spread and are managed. Incidence function decides the effect of the variables and parameters on the virus spread; hence, it is important to consider the type of function needed according to the flowchart of the infection.
Incidence rates play a crucial role in the epidemiological model formulation. The rapid appearance of any disease can be governed totally by the incidence rate equal to the affected host population in the tenure. Choosing correct incidence rate during the mathematical model formulation could give accurate predictions. Every infectious disease mutates following the host and environment, which shows the importance of multi-strain mathematical models. A variant of an infectious disease can form an epidemic or pandemic during the period.
The authors declare they have not used artificial intelligence (AI) tools in the creation of this article.
The authors have no words of conflict.
Entries of the Jacobian matrix of order thirteen associated with the system (2.4) are
a1,1=−γ−∂f(S,I1,A1)∂S(I1+α1A1)−∂g(S,I2,A2)∂S(I2+α2A2)−∂h(S,I3,A3)∂S(I3+α3A3),a1,3=−∂f(S,I1,A1)∂I1(I1+α1A1)−f(S,I1,A1),a1,4=−∂f(S,I1,A1)∂A1(I1+α1A1)−α1f(S,I1,A1),a1,7=−∂g(S,I2,A2)∂I2(I2+α2A2)−g(S,I2,A2),a1,8=−∂g(S,I2,A2)∂A2(I2+α2A2)−α2g(S,I2,A2),a1,11=−∂h(S,I3,A3)∂I3(I3+α3A3)−h(S,I3,A3),a1,12=−∂h(S,I3,A3)∂A3(I3+α3A3)−α3h(S,I3,A3),a2,1={∂f(S,I1,A1)∂S(1+δ11R1S+δ12R2S)−f(S,I1,A1)(δ11R1S2+δ12R2S2)}(I1+α1A1),a2,2=−ξ1−γ,a2,3=∂f(S,I1,A1)∂I1(1+δ11R1S+δ21R2S)(I1+α1A1)+f(S,I1,A1)(1+δ11R1S+δ21R2S),a2,4=∂f(S,I1,A1)∂A1(1+δ11R1S+δ21R2S)(I1+α1A1)+α1f(S,I1,A1)(1+δ11R1S+δ21R2S),a2,5=δ11f(S,I1,A1)(I1+α1A1)S,a3,2=r1ξ1,a3,3=−δ1I−γ,a4,2=(1−r1)ξ1,a4,4=−δ1A−γ,a5,1=R1S{δ11f(S,I1,A1)(I1+α1A1)+δ12g(S,I2,A2)(I2+α2A2)+δ13h(S,I3,A3)(I3+α3A3)−δ11∂f(S,I1,A1)∂S(I1+α1A1)−δ12∂g(S,I2,A2)∂S(I2+α2A2)−δ13∂h(S,I3,A3)∂S(I3+α3A3)},a5,3=−δ1Iδ11R1S{∂f(S,I1,A1)∂I1(I1+α1A1)+f(S,I1,A1)},a5,4=−δ1Iδ11R1S{∂f(S,I1,A1)∂A1(I1+α1A1)+α1f(S,I1,A1)},a5,5=−{δ11f(S,I1,A1)(I1+α1A1)+δ12g(S,I2,A2)(I2+α2A2)+δ13h(S,I3,A3)(I3+α3A3)}/S−γ,a5,7=−δ12R1S{∂g(S,I2,A2)∂I2(I2+α2A2)+g(S,I2,A2)},a5,8=−δ12R1S{∂g(S,I2,A2)∂A2(I2+α2A2)+α2g(S,I2,A2)},a5,11=−δ13R1S{∂h(S,I3,A3)∂I3(I3+α3A3)+h(S,I3,A3)},a5,12=−δ13R1S{∂h(S,I3,A3)∂A3(I3+α3A3)+α3h(S,I3,A3)},a6,1={∂g(S,I2,A2)∂S(1+δ21R1S+δ22R2S)−g(S,I2,A2)(δ21R1S2+δ22R2S2)}(I2+α2A2),a6,5=δ12g(S,I2,A2)(I2+α2A2)S,a6,6=−ξ2−γ,a6,7=∂g(S,I2,A2)∂I2(1+δ12R1S+δ22R2S)(I2+α2A2)+g(S,I2,A2)(1+δ12R1S+δ22R2S),a6,8=∂g(S,I2,A2)∂A2(1+δ12R1S+δ22R2S)(I2+α2A2)+α2g(S,I2,A2)(1+δ12R1S+δ22R2S),a6,9=δ22g(S,I2,A2)(I2+α2A2)S,a7,6=r2ξ2,a7,7=−δ2I−γ,a8,6=(1−r2)ξ2,a8,8=−δ2A−γ,a9,1=R2S{δ21f(S,I1,A1)(I1+α1A1)+δ22g(S,I2,A2)(I2+α2A2)+δ23h(S,I3,A3)(I3+α3A3)−δ21∂f(S,I1,A1)∂S(I1+α1A1)−δ22∂g(S,I2,A2)∂S(I2+α2A2)−δ23∂h(S,I3,A3)∂S(I3+α3A3)},a9,3=−δ21R2S{∂f(S,I1,A1)∂I1(I1+α1A1)+f(S,I1,A1)},a9,4=−δ21R2S{∂f(S,I1,A1)∂A1(I1+α1A1)+α1f(S,I1,A1)},a9,7=−δ2Iδ22R2S{∂g(S,I2,A2)∂I2(I2+α2A2)+g(S,I2,A2)},a9,8=−δ2Aδ22R2S{∂g(S,I2,A2)∂A2(I2+α2A2)+α2g(S,I2,A2)},a9,9=−{δ21f(S,I1,A1)(I1+α1A1)+δ22g(S,I2,A2)(I2+α2A2)+δ23h(S,I3,A3)(I3+α3A3)}/S−γ,a9,11=−δ23R2S{∂h(S,I3,A3)∂I3(I3+α3A3)+h(S,I3,A3)},a9,12=−δ23R2S{∂h(S,I3,A3)∂A3(I3+α3A3)+α3h(S,I3,A3)},a10,1=(∂h(S,I3,A3)∂S(1+δ13R1S+δ23R2S)−h(S,I3,A3)(δ13R1S2+δ23R2S2))(I3+α3A3),a10,5=δ13h(S,I3,A3)(I3+α3A3)S,a10,9=δ23h(S,I3,A3)(I3+α3A3)S,a10,10=−ξ3−γ,a10,11=∂h(S,I3,A3)∂I3(1+δ13R1S+δ23R2S)(I3+α3A3)+h(S,I3,A3)(1+δ13R1S2+δ23R2S2),a10,12=∂h(S,I3,A3)∂I3(1+δ13R1S+δ23R2S)(I3+α3A3)+h(S,I3,A3)(1+δ13R1S2+α3δ23R2S2),a11,10=r3ξ3,a11,11=−δ3I−γ,a12,10=(1−r3)ξ3,a12,12=−δ3A−γ,a13,11=δ3I,a13,12=δ3A,a13,13=−γ. |
[1] |
W. O. Kermack, A. G. McKendrick, A contribution to the mathematical theory of epidemics, Proc. R. Soc. Lond. A., 115 (1927), 700–721. https://doi.org/10.1098/rspa.1927.0118 doi: 10.1098/rspa.1927.0118
![]() |
[2] | J. Murray, Mathematical Biology, Springer-Verlag, Heidelberg, 1 (2002). |
[3] | F. Brauer, C. C. Chavez, Z. Feng, Mathematical Models in Epidemiology, Springer, 32 (2019). https://doi.org/10.1007/978-1-4939-9828-9 |
[4] |
S. Garmer, R. Lynn, D. Rossi, A. Capaldi, Multistrain infections in metapopulations, Spora, 1 (2015), 17–27. https://doi.org/10.30707/SPORA1.1Garmer doi: 10.30707/SPORA1.1Garmer
![]() |
[5] |
E. Cirulli, D. Goldstein, Uncovering the roles of rare variants in common disease through whole-genome sequencing, Nat. Rev. Genet., 11 (2010), 415–425. https://doi.org/10.1038/nrg2779 doi: 10.1038/nrg2779
![]() |
[6] | M. Cascella, M. Rajnik, A. Aleem, Features, Evaluation, and Treatment of Coronavirus (COVID-19), StatPearls Publishing, 2022. |
[7] |
Z. Yaagoub, K. Allali, Global stability of multi-strain SEIR epidemic model with vaccination strategy, Math. Comput. Appl., 28 (2023), 9. https://doi.org/10.3390/mca28010009 doi: 10.3390/mca28010009
![]() |
[8] |
T. Sardar, I. Ghosh, X. Rodó, J. Chattopadhyay, A realistic two-strain model for MERS-CoV infection uncovers the high risk for epidemic propagation, PLoS Neglected Trop. Dis., 14 (2020), e0008065. https://doi.org/10.1371/journal.pntd.0008065 doi: 10.1371/journal.pntd.0008065
![]() |
[9] |
M. A. Kuddus, E. S. McBryde, A. I. Adekunle, M. T. Meehan, Analysis and simulation of a two-strain disease model with nonlinear incidence, Chaos Solitons Fractals, 155 (2022). https://doi.org/10.1016/j.chaos.2021.111637 doi: 10.1016/j.chaos.2021.111637
![]() |
[10] |
V. P. Bajiya, J. P. Tripathi, V. Kakkar, J. Wang, G. Sun, Global dynamics of a multi-group SEIR epidemic model with infection age, Chin. Ann. Math. Ser. B, 42 (2021), 833–860. https://doi.org/10.1007/s11401-021-0294-1 doi: 10.1007/s11401-021-0294-1
![]() |
[11] |
E. F. Arruda, S. S. Das, C. M. Dias, D. H. Pastore, Modelling and optimal control of multi strain epidemics, with application to COVID-19, PLoS ONE, 16 (2021). https://doi.org/10.1371/journal.pone.0257512 doi: 10.1371/journal.pone.0257512
![]() |
[12] | S. Ghosh, M. Banerjee, A multi-strain model for COVID-19, in Applied Analysis, Applied Analysis, Optimization and Soft Computing, 2022. https://doi.org/10.1007/978-981-99-0597-3_10 |
[13] |
S. W. X. Ong, C. J. Chiew, L. W. Ang, T. Mak, L. Cui, M. P. H. S. Toh, et al., Clinical and virological features of severe acute respiratory syndrome coronavirus 2 (SARS-CoV-2) variants of concern: A retrospective cohort study comparing B.1.1.7 (Alpha), B.1.351 (Beta), and B.1.617.2 (Delta), Clin. Infect. Dis., 75 (2021), e1128–e1136. https://doi.org/10.1093/cid/ciab721 doi: 10.1093/cid/ciab721
![]() |
[14] |
M. Massard, R. Eftimie, A. Perasso, B. Saussereau, A multi-strain epidemic model for COVID-19 with infected and asymptomatic cases: application to French data, J. Theor. Biol., 545 (2022), 111117. https://doi.org/10.1016/j.jtbi.2022.111117 doi: 10.1016/j.jtbi.2022.111117
![]() |
[15] |
Z. Abreu, G. Cantin, C. J. Silva, Analysis of a COVID-19 compartmental model: a mathematical and computational approach, Math. Biosci. Eng., 18 (2021), 7979–7998. https://doi.org/10.3934/mbe.2021396 doi: 10.3934/mbe.2021396
![]() |
[16] | World Health Organization, Coronavirus World Health Organization 19. Available from: https://www.who.int/health-topics/coronavirus. |
[17] | Center for Disease Control and prevention (CDC). Available from: https://www.cdc.gov/coronavirus/2019-ncov/variants/variant-classifications.html |
[18] | National Center for Biotechnology Information (NCBI). Available from: https://www.ncbi.nlm.nih.gov/activ. |
[19] |
G. Rohith, K. B. Devika, Dynamics and control of COVID-19 pandemic with nonlinear incidence rates, Nonlinear Dyn., 101 (2020), 2013–2026. https://doi.org/10.1007/s11071-020-05774-5 doi: 10.1007/s11071-020-05774-5
![]() |
[20] |
S. Ruan, W. Wang, Dynamical behavior of an epidemic model with a nonlinear incidence rate, J. Diff. Equation, 188 (2003), 135–163. https://doi.org/10.1016/S0022-0396(02)00089-X doi: 10.1016/S0022-0396(02)00089-X
![]() |
[21] |
H. W. Hethcote, P. van den Driessche, Some epidemiological models with nonlinear incidence, J. Math. Biol., 29 (1991), 271–287. https://doi.org/10.1007/bf00160539 doi: 10.1007/bf00160539
![]() |
[22] |
G. Huang, Y. Takeuchi, W. Ma, D. Wei, Global stability for delay SIR and SEIR epidemic models with nonlinear incidence rate, Bull. Math. Biol., 72 (2010), 1192–1207. https://doi.org/10.1007/s11538-009-9487-6 doi: 10.1007/s11538-009-9487-6
![]() |
[23] |
W. M. Liu, S. A. Levin, Y. Iwasa, Influence of nonlinear incidence rates upon the behavior of SIRS epidemiological models, J. Math. Biol., 23 (1986), 187–204. https://doi.org/10.1007/BF00276956 doi: 10.1007/BF00276956
![]() |
[24] |
J. Arino, C. C. McCluskey, Effect of a sharp change of the incidence function on the dynamics of a simple disease, J. Bio. Dyn., 4 (2010), 490–505. https://doi.org/10.1080/17513751003793017 doi: 10.1080/17513751003793017
![]() |
[25] |
N. Bellomo, R. Bingham, M. A. J. Chaplain, G. Dosi, G. Forni, D. A. Knopoff, et al., A multiscale model of virus pandemic: Heterogeneous interactive entities in a globally connected world, Math. Models Methods Appl. Sci., 30 (2020), 1591–1651. https://doi.org/10.1142/s0218202520500323 doi: 10.1142/s0218202520500323
![]() |
[26] |
J. J. Wang, J. Z. Zhang, Z. Jin, Analysis of an SIR model with bilinear incidence rate, Nonlinear Anal. Real World Appl., 11 (2010), 2390–2402. https://doi.org/10.1016/j.nonrwa.2009.07.012 doi: 10.1016/j.nonrwa.2009.07.012
![]() |
[27] |
O. Khyar, K. Allali, Global dynamics of a multi-strain SEIR epidemic model with general incidence rates: application to COVID-19 pandemic, Nonlinear Dyn., (2020), 1–21. https://doi.org/10.1007/s11071-020-05929-4 doi: 10.1007/s11071-020-05929-4
![]() |
[28] |
X. Liu, L. Yang, Stability analysis of an SEIQV epidemic model with saturated incidence rate, Nonlinear Anal. Real World Appl., 13 (2012), 2671–2679. https://doi.org/10.1016/j.nonrwa.2012.03.010 doi: 10.1016/j.nonrwa.2012.03.010
![]() |
[29] | A. Kaddar, On the dynamics of a delayed SIR epidemic model with a modified saturated incidence rate, Electron. J. Differ. Equation, 13 (2009), 1–7. |
[30] |
Y. Zhao, D. Jiang, The threshold of a stochastic SIRS epidemic model with saturated incidence, Appl. Math. Lett., 34 (2014), 90–93. https://doi.org/10.3934/dcdsb.2015.20.1277 doi: 10.3934/dcdsb.2015.20.1277
![]() |
[31] |
X. Q. Liu, S. M. Zhong, B. D. Tian, F. X. Zheng, Asymptotic properties of a stochastic predator-prey model with Crowley–Martin functional response, J. Appl. Math. Comput., 43 (2013), 479–490. https://doi.org/10.1007/s12190-013-0674-0 doi: 10.1007/s12190-013-0674-0
![]() |
[32] |
J. R. Beddington, Mutual interference between parasites or predators and its effect on searching efficiency, J. Anim. Ecol., 44 (1975), 331–341. https://doi.org/10.2307/3866 doi: 10.2307/3866
![]() |
[33] |
R. S. Cantrell, C. Cosner, On the dynamics of predator-prey models with the Beddington–DeAngelis functional response, J. Math. Anal. Appl., 257 (2001), 206–222. https://doi.org/10.1006/JMAA.2000.7343 doi: 10.1006/JMAA.2000.7343
![]() |
[34] |
X. Zhou, J. Cui, Global stability of the viral dynamics with Crowley–Martin functional response, Bull. Korean Math. Soc., 48 (2011), 555–574. https://doi.org/10.1002/mma.3078 doi: 10.1002/mma.3078
![]() |
[35] |
K. Hattaf, N. Yousfi, A. Tridane, Mathematical analysis of a virus dynamics model with general incidence rate and cure rate, Nonlinear Anal. Real World Appl., 13 (2012), 1866–1872. https://doi.org/10.1016/j.nonrwa.2011.12.015 doi: 10.1016/j.nonrwa.2011.12.015
![]() |
[36] |
K. Hattaf, M. Mahrouf, J. Adnani, N. Yousfi, Qualitative analysis of a stochastic epidemic model with specific functional response and temporary immunity, Phys. A., 490 (2018), 591–600. https://doi.org/10.1016/j.physa.2017.08.043 doi: 10.1016/j.physa.2017.08.043
![]() |
[37] |
K. Hattaf, N. Yousfi, Global dynamics of a delay reaction–diffusion model for viral infection with specific functional response, Comput. Appl. Math., 34 (2015), 807–818. https://doi.org/10.1007/s40314-014-0143-x doi: 10.1007/s40314-014-0143-x
![]() |
[38] |
K. Hattaf, N. Yousfi, A. Tridane, Stability analysis of a virus dynamics model with general incidence rate and two delays, Appl. Math. Comput., 221 (2013), 514–521. https://doi.org/10.1016/j.amc.2013.07.005 doi: 10.1016/j.amc.2013.07.005
![]() |
[39] | M. K. Singh, Anjali, Mathematical modeling and analysis of seqiahr model: Impact of quarantine and isolation on COVID-19, App. App. Math., 17 (2022), 146–171. |
[40] |
S. Sharma, V. Volpert, M. Banerjee, Extended SEIQR type model for covid-19 epidemic and data analysis, Math. Biosci. Eng., (2020), 7562–7604. https://doi.org/10.3934/mbe.2020386 doi: 10.3934/mbe.2020386
![]() |
[41] |
P. Kim, S. M. Gordon, M. M. Sheehan, M. B. Rothberg, Duration of severe acute respiratory syndrome Coronavirus 2 natural immunity and protection against the Delta variant: A retrospective cohort study, Clin. Infect. Dis., 75 (2022), 185–190. https://doi.org/10.1093/cid/ciab999 doi: 10.1093/cid/ciab999
![]() |
[42] |
P. Van den Driessche, J. Watmough, Reproduction numbers and sub-threshold endemic equilibria for compartmental models of disease transmission, Math. Biosci., 180 (2002), 29–48. https://doi.org/10.1016/s0025-5564(02)00108-6 doi: 10.1016/s0025-5564(02)00108-6
![]() |
[43] |
D. Bentaleb, S. Amine, Lyapunov function and global stability for a two-strain SEIR model with bilinear and non-monotone, Int. J. Biomath., 12 (2019). https://doi.org/10.1142/S1793524519500219 doi: 10.1142/S1793524519500219
![]() |
[44] |
F. G. Ball, E. S. Knock, P. D. O'Neil, Control of emerging infectious diseases using responsive imperfect vaccination and isolation, Math. Biosci., 216 (2008), 100–113. https://doi.org/10.1016/j.mbs.2008.08.008 doi: 10.1016/j.mbs.2008.08.008
![]() |
[45] | C. Castilho, Optimal control of an epidemic through educational campaigns, Electron. J. Differ. Equation, (2006), 1–11. |
Case | f(S,I1,A1) | g(S,I2,A2) | h(S,I3,A3) |
(a) | β1S | β2S | β3S |
(b) | β1S1+ζ1S+ζ2I1+ζ3A1 | β2S1+ζ4S+ζ5I2+ζ6A2 | β3S1+ζ7S+ζ8I3+ζ9A3 |
(c) | β1S1+ζ1I21 | β2S1+ζ2I22 | β3S1+ζ3I23 |
(d) | β1S1+ζ1A21 | β2S1+ζ2A22 | β3S1+ζ3A23 |
(e) | β1S1+ζ1S | β2S1+ζ2S | β3S1+ζ3S |
(f) | β1S1+ζ1I1 | β2S1+ζ2I2 | β3S1+ζ3I3 |
(g) | β1S1+ζ1A1 | β2S1+ζ2A2 | β3S1+ζ3A3 |
(h) | β1S/(1+ζ1S+ζ2I1 | β2S/(1+ζ4S+ζ5I2 | β3S/(1+ζ7S+ζ8I3 |
+ζ3A1+ζ1ζ2SI1 | +ζ6A2+ζ4ζ5SI2 | +ζ9A3+ζ7ζ8SI3 | |
+ζ1ζ3SA1+ζ2ζ3I1A1) | +ζ5ζ6I2A2+ζ4ζ6SA2) | +ζ8ζ9I3A3+ζ7ζ9SA3) | |
(i) | β1S/(1+ζ1S+ζ2I1 | β2S/(1+ζ7S+ζ8I2 | β3S/(1+ζ13S+ζ14I3 |
+ζ3A1+ζ4SI1 | +ζ9A2+ζ10SI2 | +ζ15A3+ζ16SI3 | |
+ζ5SA1+ζ6I1A1) | +ζ11I2A2+ζ12SA2) | +ζ17I3A3+ζ18SA3) | |
(j) | β1S1+ζ1I1 | β2S1+ζ2S+ζ3I2+ζ4A2 | β3S1+ζ5A23 |
Case | f(S,I1,A1) | g(S,I2,A2) | h(S,I3,A3) |
(a) | β1S | β2S | β3S |
(b) | β1S1+ζ1S+ζ2I1+ζ3A1 | β2S1+ζ4S+ζ5I2+ζ6A2 | β3S1+ζ7S+ζ8I3+ζ9A3 |
(c) | β1S1+ζ1I21 | β2S1+ζ2I22 | β3S1+ζ3I23 |
(d) | β1S1+ζ1A21 | β2S1+ζ2A22 | β3S1+ζ3A23 |
(e) | β1S1+ζ1S | β2S1+ζ2S | β3S1+ζ3S |
(f) | β1S1+ζ1I1 | β2S1+ζ2I2 | β3S1+ζ3I3 |
(g) | β1S1+ζ1A1 | β2S1+ζ2A2 | β3S1+ζ3A3 |
(h) | β1S/(1+ζ1S+ζ2I1 | β2S/(1+ζ4S+ζ5I2 | β3S/(1+ζ7S+ζ8I3 |
+ζ3A1+ζ1ζ2SI1 | +ζ6A2+ζ4ζ5SI2 | +ζ9A3+ζ7ζ8SI3 | |
+ζ1ζ3SA1+ζ2ζ3I1A1) | +ζ5ζ6I2A2+ζ4ζ6SA2) | +ζ8ζ9I3A3+ζ7ζ9SA3) | |
(i) | β1S/(1+ζ1S+ζ2I1 | β2S/(1+ζ7S+ζ8I2 | β3S/(1+ζ13S+ζ14I3 |
+ζ3A1+ζ4SI1 | +ζ9A2+ζ10SI2 | +ζ15A3+ζ16SI3 | |
+ζ5SA1+ζ6I1A1) | +ζ11I2A2+ζ12SA2) | +ζ17I3A3+ζ18SA3) | |
(j) | β1S1+ζ1I1 | β2S1+ζ2S+ζ3I2+ζ4A2 | β3S1+ζ5A23 |