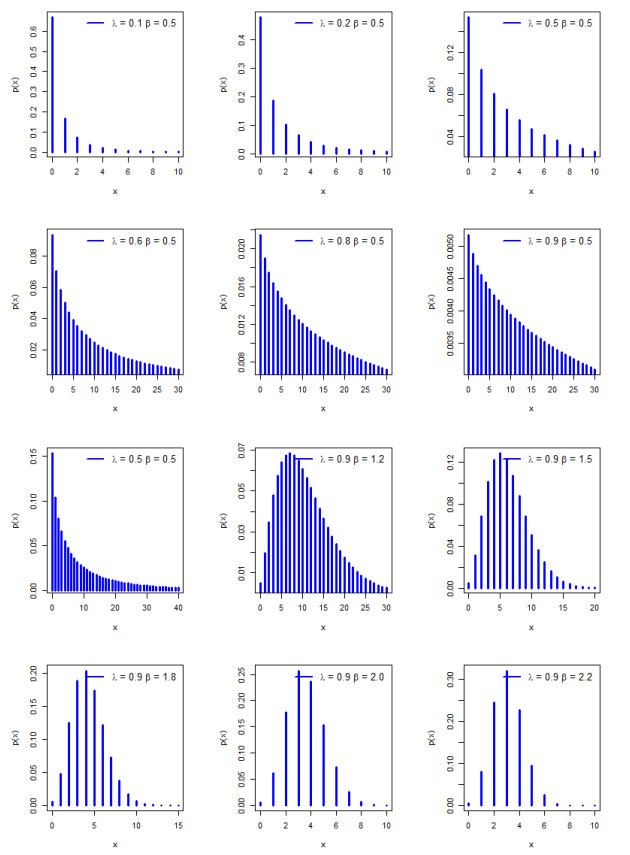
This paper mainly focuses on the dynamics behavior of a three-component chemotaxis system on alopecia areata
{ut=Δu−χ1∇⋅(u∇w)+w−μ1u2,x∈Ω,t>0,vt=Δv−χ2∇⋅(v∇w)+w+ruv−μ2v2,x∈Ω,t>0,wt=Δw+u+v−w,x∈Ω,t>0,∂u∂ν=∂v∂ν=∂w∂ν=0,x∈∂Ω,t>0,u(x,0)=u0(x), v(x,0)=v0(x), w(x,0)=w0(x),x∈Ω,
where Ω⊂Rn (n≥4) is a bounded convex domain with smooth boundary ∂Ω, the parameters χi, μi (i=1,2), and r are positive. We show that this system exists a globally bounded classical solution if μi(i=1,2) is large enough. This result extends the corresponding results which were obtained by Lou and Tao (JDE, 2021) to the higher-dimensional case.
Citation: Wenjie Zhang, Lu Xu, Qiao Xin. Global boundedness of a higher-dimensional chemotaxis system on alopecia areata[J]. Mathematical Biosciences and Engineering, 2023, 20(5): 7922-7942. doi: 10.3934/mbe.2023343
[1] | Hatim Solayman Migdadi, Nesreen M. Al-Olaimat, Maryam Mohiuddin, Omar Meqdadi . Statistical inference for the Power Rayleigh distribution based on adaptive progressive Type-II censored data. AIMS Mathematics, 2023, 8(10): 22553-22576. doi: 10.3934/math.20231149 |
[2] | M. G. M. Ghazal . Modified Chen distribution: Properties, estimation, and applications in reliability analysis. AIMS Mathematics, 2024, 9(12): 34906-34946. doi: 10.3934/math.20241662 |
[3] | Azam Zaka, Ahmad Saeed Akhter, Riffat Jabeen . The new reflected power function distribution: Theory, simulation & application. AIMS Mathematics, 2020, 5(5): 5031-5054. doi: 10.3934/math.2020323 |
[4] | H. M. Barakat, M. A. Alawady, I. A. Husseiny, M. Nagy, A. H. Mansi, M. O. Mohamed . Bivariate Epanechnikov-exponential distribution: statistical properties, reliability measures, and applications to computer science data. AIMS Mathematics, 2024, 9(11): 32299-32327. doi: 10.3934/math.20241550 |
[5] | Fiaz Ahmad Bhatti, G. G. Hamedani, Mashail M. Al Sobhi, Mustafa Ç. Korkmaz . On the Burr XII-Power Cauchy distribution: Properties and applications. AIMS Mathematics, 2021, 6(7): 7070-7092. doi: 10.3934/math.2021415 |
[6] | Ekramy A. Hussein, Hassan M. Aljohani, Ahmed Z. Afify . The extended Weibull–Fréchet distribution: properties, inference, and applications in medicine and engineering. AIMS Mathematics, 2022, 7(1): 225-246. doi: 10.3934/math.2022014 |
[7] | Hisham Mahran, Mahmoud M. Mansour, Enayat M. Abd Elrazik, Ahmed Z. Afify . A new one-parameter flexible family with variable failure rate shapes: Properties, inference, and real-life applications. AIMS Mathematics, 2024, 9(5): 11910-11940. doi: 10.3934/math.2024582 |
[8] | M. G. M. Ghazal, Yusra A. Tashkandy, Oluwafemi Samson Balogun, M. E. Bakr . Exponentiated extended extreme value distribution: Properties, estimation, and applications in applied fields. AIMS Mathematics, 2024, 9(7): 17634-17656. doi: 10.3934/math.2024857 |
[9] | Haidy A. Newer, Mostafa M. Mohie El-Din, Hend S. Ali, Isra Al-Shbeil, Walid Emam . Statistical inference for the Nadarajah-Haghighi distribution based on ranked set sampling with applications. AIMS Mathematics, 2023, 8(9): 21572-21590. doi: 10.3934/math.20231099 |
[10] | Alaa M. Abd El-Latif, Hanan H. Sakr, Mohamed Said Mohamed . Fractional generalized cumulative residual entropy: properties, testing uniformity, and applications to Euro Area daily smoker data. AIMS Mathematics, 2024, 9(7): 18064-18082. doi: 10.3934/math.2024881 |
This paper mainly focuses on the dynamics behavior of a three-component chemotaxis system on alopecia areata
{ut=Δu−χ1∇⋅(u∇w)+w−μ1u2,x∈Ω,t>0,vt=Δv−χ2∇⋅(v∇w)+w+ruv−μ2v2,x∈Ω,t>0,wt=Δw+u+v−w,x∈Ω,t>0,∂u∂ν=∂v∂ν=∂w∂ν=0,x∈∂Ω,t>0,u(x,0)=u0(x), v(x,0)=v0(x), w(x,0)=w0(x),x∈Ω,
where Ω⊂Rn (n≥4) is a bounded convex domain with smooth boundary ∂Ω, the parameters χi, μi (i=1,2), and r are positive. We show that this system exists a globally bounded classical solution if μi(i=1,2) is large enough. This result extends the corresponding results which were obtained by Lou and Tao (JDE, 2021) to the higher-dimensional case.
In numerous practical situations, the datasets are measured in the number of cycles, runs, and/or shocks the device sustains before its failure. For example, the number of voltage fluctuations, the lifetime of a discrete random variable (rv), and frequency of a device switched on/off, the life of a weapon is measured by the number of rounds fired before failure, and the number of completed cycles measures the life of the equipment. Further, the number of patients, number of deaths due to a disease/virus, and number of days a patient stays in a hospital ward. Various discrete probability models can be adopted to analyze such types of datasets.
The well-known traditional discrete probability models, including the negative binomial, geometric, and Poisson distributions have limitations to use due to their specific behavior such as the Poisson distribution that performs better with datasets having dispersion equal to average; the NB distribution is applicable for over-dispersed datasets. The real-life datasets may be over-dispersed or under-dispersed, so there is always a clear need for flexible discrete distributions to have a good resolution.
Several discretized forms of continuous distributions have been derived to model different count datasets in the last few decades. The most notable discretization approach in the literature is the survival discretizing approach which has gained much attention.
Let rv X follows a continuous distribution with survival function (sf) S(x). Using the survival discretization approach introduced by Kemp (2004), the probability mass function (pmf) of a discrete rv follows as
p(x)=P(X=x)=S(x)−S(x+1),x=0,1,2,3,… | (1) |
The survival discretization approach has been adopted to develop many discrete models. For example, the discrete normal [1], discrete Rayleigh [2], discrete half-normal [3], discrete Burr and discrete Pareto [4], discrete inverse-Weibull [5], new generalization of the geometric [6], discrete Lindley [7], generalized exponential type Ⅱ [8], discrete inverse-Rayleigh [9], two-parameter discrete Lindley [10], discrete log-logistic [11], discrete extended Weibull [12], exponentiated discrete-Lindley [13], discrete Burr-Hutke [14], discrete Marshall-Olkin Weibull [15], natural discrete-Lindley [16], discrete Bilal [17], discrete inverted Topp-Leone [18], uniform Poisson–Ailamujia [19], exponentiated discrete Lindley [20], discrete exponentiated Burr–Hatke [21], discrete Ramos-Louzada [22] and [23], and discrete type-Ⅱ half-logistic exponential [24].
The main goal of the present study is to introduce a new discrete distribution to model over-dispersed as well as under-dispersed datasets. The proposed distribution is called the discrete power-Ailamujia (DsPA) distribution. The mathematical properties of the DsPA distribution are derived and its parameters are estimated using the maximum likelihood method. Three real count datasets are fitted using the DsPA model and other competing discrete distributions. The DsPA distribution provides a better fit to the three datasets than some well-known discrete models according to the results of the simulation.
The paper is organized in the following sections. Section 2 is devoted to the derivation of the new DsPA distribution. Its mathematical properties are explored in Section 3. Section 4 is devoted to estimating the DsPA parameters and providing a comprehensive simulation study. The usefulness of the DsPA distribution is addressed in Section 5. Finally, we conclude the study in Section 6.
Jamal et al. [25] proposed a new continuous lifetime distribution called the power-Ailamujia distribution. Its probability density function and sf can be expressed as
f(x)=θ2βx2β−1e−θxβ,x≥0,θ,β>0 | (2) |
and
S(x)=(1+θxβ)e−θxβ,x≥0,θ,β>0, | (3) |
respectively.
Applying the survival discretization approach in (1), the rv X is said to have the DsPA distribution with parameters 0<λ<1 and β>0, if its sf takes the form
S(x;λ,β)=λ(x+1)β[1−(x+1)βlnλ],x∈N0, | (4) |
where λ=e−θ and N0={0,1,2,…,w} for 0<w<∞.
The corresponding cumulative distribution function (cdf) and pmf can be expressed as
F(x;λ,β)=1−λ(x+1)β[1−(x+1)βlnλ],x∈N0 | (5) |
and
Px(x;λ)=λxβ[1−xβlnλ]−λ(x+1)β[1−(x+1)βlnλ],x∈N0. | (6) |
Plots of the DsPA pmf, for various values of the parameters λ and β, are presented in Figure 1.
The hazard rate function (hrf) of the DsPA distribution can be expressed as
h(x;λ)=p(x)S(x)=λxβ[1−xβlnλ]λ(x+1)β[1−(x+1)βlnλ]−1,x∈N0 | (7) |
where h(x;λ)=P(x)S(x). Figure 2 shows the DsPA hrf plots for different values of λ and β.
The quantile function of the DsPA distribution reduces to
Q(u)=⌊1−[1−(x+1)βlnλ]λ(x+1)β⌋,0<u<1, |
where ⌊x⌋ denotes the integer part of x.
The reverse hrf (rhrf) of the DsPA distribution is defined as
r∗(x)=p(x)F(x)=λxβ[1−xβlnλ]−λ(x+1)β[1−(x+1)βlnλ]1−λ(x+1)β[1−(x+1)βlnλ],x∈N0, | (8) |
where r∗(x)=P(x)F(x). Figure 3 shows the DsPA rhrf plots for several values of λ and β.
The second failure rate of the DsPA distribution is expressed by
r∗∗(x)=log{S(x)S(x+1)}=log{λ(x+1)β[1−(x+1)βlnλ]λ(x+2)β[1−(x+2)βlnλ]},x∈N0. | (9) |
The recurrence relation of probabilities from the DsPA distribution has the form
P(x+1)P(x)=λ(x+1)β[1−(x+1)βlnλ]−λ(x+2)β[1−(x+2)βlnλ]λxβ[1−xβlnλ]−λ(x+1)β[1−(x+1)βlnλ]. | (10) |
Hence,
P(x+1)=λ(x+1)β[1−(x+1)βlnλ]−λ(x+2)β[1−(x+2)βlnλ]λxβ[1−xβlnλ]−λ(x+1)β[1−(x+1)βlnλ]P(x). |
In this section, we studied some mathematical properties of the DsPA distribution. In this section, we studied some mathematical properties of the DsPA distribution.
The probability generating function (pgf) of the DsPA distribution is given as follows
Gx(z)=1+(z−1)∞∑x=1zx−1(1−xβlnλ)λxβ, | (11) |
where Gx(z)=∑∞x=0zxP(x). The moment generating function (mgf) can be obtained by replacing z with ez in Eq (11). Thus, the mgf of the DsPA distribution can be expressed as
Mx(z)=1+(ez−1)∞∑x=1(ez)x−1(1−xβlnλ)λxβ. | (12) |
Thus, the first four moments of the DsPA distribution are
E(X)=∞∑x=1(1−xβlnλ)λxβ, | (13) |
E(X2)=∞∑x=1(2x−1)(1−xβlnλ)λxβ, |
E(X3)=∞∑x=1(3x2−3x+1)(1−xβlnλ)λxβ |
and
E(X4)=∞∑x=1(4x3−6x2+4x−1)(1−xβlnλ)λxβ. |
Using the above moments, the variance (σ2), coefficient of skewness (CS), and coefficient of kurtosis (CK) can be presented in closed-form expressions. Further, another classical concept, called dispersion index (DI). The DI is defined as a variance to mean ratio. If the DI value is less than 1, then the model is suitable for under-dispersed datasets. Conversely, if the DI is greater than 1, then it is suitable for over-dispersed datasets. Numerical values of the mean, E(X), σ2, CS, CK and DI are reported in Tables 1‒5.
β | λ | ||||||||
0.1 | 0.2 | 0.3 | 0.4 | 0.5 | 0.6 | 0.7 | 0.8 | 0.9 | |
0.5 | 0.7603 | 1.8909 | 3.6766 | 6.5446 | 10.966 | 16.848 | 22.277 | 22.458 | 12.187 |
1.0 | 0.3954 | 0.7529 | 1.1657 | 1.6848 | 2.3863 | 3.4156 | 5.1075 | 8.4629 | 18.452 |
1.5 | 0.3415 | 0.5827 | 0.8221 | 1.0907 | 1.4187 | 1.8533 | 2.4910 | 3.5895 | 6.2446 |
2.0 | 0.3313 | 0.5338 | 0.7085 | 0.8884 | 1.0967 | 1.3599 | 1.7259 | 2.3141 | 3.5954 |
2.5 | 0.3303 | 0.5230 | 0.6698 | 0.8012 | 0.9444 | 1.1259 | 1.3763 | 1.7634 | 2.5557 |
3.0 | 0.3303 | 0.5219 | 0.6619 | 0.7720 | 0.8721 | 0.9919 | 1.1725 | 1.4628 | 2.0209 |
β | λ | ||||||||
0.1 | 0.2 | 0.3 | 0.4 | 0.5 | 0.6 | 0.7 | 0.8 | 0.9 | |
0.5 | 2.7920 | 12.243 | 38.665 | 103.06 | 229.88 | 417.28 | 621.34 | 779.36 | 615.37 |
1.0 | 0.3901 | 0.8140 | 1.4378 | 2.4502 | 4.2371 | 7.7429 | 15.802 | 40.249 | 177.94 |
1.5 | 0.2475 | 0.3692 | 0.4988 | 0.6724 | 0.9299 | 1.3485 | 2.1203 | 3.8858 | 10.421 |
2.0 | 0.2236 | 0.2727 | 0.3017 | 0.3478 | 0.4232 | 0.5408 | 0.7369 | 1.1271 | 2.2935 |
2.5 | 0.2213 | 0.2517 | 0.2384 | 0.2287 | 0.2486 | 0.3033 | 0.3864 | 0.5260 | 0.8911 |
3.0 | 0.2212 | 0.2496 | 0.2252 | 0.1869 | 0.1626 | 0.1789 | 0.2448 | 0.3256 | 0.4729 |
β | λ | ||||||||
0.1 | 0.2 | 0.3 | 0.4 | 0.5 | 0.6 | 0.7 | 0.8 | 0.9 | |
0.5 | 4.6187 | 4.3533 | 3.9822 | 3.2831 | 2.4657 | 1.7555 | 1.2590 | 1.1461 | 2.0439 |
1.0 | 1.6228 | 1.4038 | 1.3664 | 1.3697 | 1.3821 | 1.3943 | 1.4037 | 1.4099 | 1.3511 |
1.5 | 0.9461 | 0.5856 | 0.5417 | 0.5744 | 0.6191 | 0.6586 | 0.6895 | 0.7124 | 0.7285 |
2.0 | 0.7459 | 0.1163 | -0.0092 | 0.1018 | 0.2223 | 0.2912 | 0.3312 | 0.3598 | 0.3836 |
2.5 | 0.7226 | -0.0655 | -0.4988 | -0.4984 | -0.1012 | 0.1683 | 0.1697 | 0.1591 | 0.1742 |
3.0 | 0.7218 | -0.0869 | -0.6648 | -1.0922 | -0.9663 | -0.0481 | 0.3705 | 0.0738 | 0.0476 |
β | λ | ||||||||
0.1 | 0.2 | 0.3 | 0.4 | 0.5 | 0.6 | 0.7 | 0.8 | 0.9 | |
0.5 | 41.471 | 36.784 | 28.534 | 18.030 | 10.188 | 5.7950 | 3.7130 | 3.1485 | 5.9942 |
1.0 | 5.9572 | 5.6739 | 5.7391 | 5.8240 | 5.8909 | 5.9380 | 5.9690 | 5.9875 | 5.4737 |
1.5 | 2.5630 | 2.7503 | 3.1474 | 3.3764 | 3.4912 | 3.5508 | 3.5857 | 3.6093 | 3.6262 |
2.0 | 1.6369 | 1.5891 | 2.4907 | 3.0833 | 3.1595 | 3.0954 | 3.0599 | 3.0514 | 3.0542 |
2.5 | 1.5246 | 1.0744 | 1.8108 | 3.2354 | 3.9993 | 3.4076 | 2.8767 | 2.8838 | 2.8977 |
3.0 | 1.5211 | 1.0098 | 1.4967 | 2.7867 | 4.9278 | 5.5909 | 3.4605 | 2.4008 | 2.8802 |
β | λ | ||||||||
0.1 | 0.2 | 0.3 | 0.4 | 0.5 | 0.6 | 0.7 | 0.8 | 0.9 | |
0.5 | 3.6720 | 6.4746 | 10.516 | 15.747 | 20.964 | 24.768 | 27.891 | 34.703 | 50.494 |
1.0 | 0.9866 | 1.0810 | 1.2335 | 1.4543 | 1.7756 | 2.2669 | 3.0939 | 4.7559 | 9.6437 |
1.5 | 0.7248 | 0.6337 | 0.6068 | 0.6165 | 0.6555 | 0.7276 | 0.8512 | 1.0826 | 1.6688 |
2.0 | 0.6749 | 0.5109 | 0.4258 | 0.3915 | 0.3859 | 0.3977 | 0.4269 | 0.4870 | 0.6379 |
2.5 | 0.6699 | 0.4813 | 0.3559 | 0.2854 | 0.2632 | 0.2694 | 0.2808 | 0.2983 | 0.3487 |
3.0 | 0.6697 | 0.4782 | 0.3402 | 0.2422 | 0.1865 | 0.1804 | 0.2088 | 0.2226 | 0.2340 |
From Tables 1‒5, we can conclude that the mean is an increasing function of λ and a decreasing function of β. It is clear that the skewness of the DsPA distribution can be positive or negative. The DI showing increasing behavior for larger values of the parameter λ and small values of β. Further, the DsPA distribution is suitable for over-dispersed and under-dispersed data sets.
The MRL function is a helpful reliability characteristic to model and analyze the burn-in and maintenance policies. Consider the rv X that has the cdf F(.). For a discrete rv, the MRL function is defined by
MRL=ε(i)=E(X−i|X≥i)=11−F(i−1,λ)w∑j=i+1[1−F(j−1,λ)],i∈N0, |
where N0={0,1,2,…,w} and 0<w<∞.
Then, the MRL of the DsPA model reduces to
MRL=11−F(i−1,λ,β)w∑j=i+1[1−F(j−1,λ,β)] |
=1[1−(i)βlnλ]λ(i)βw∑j=i+1[1−(j)βlnλ]λ(j)β |
=1[1−(i)βlnλ]λ(i)β[w∑j=i+1λ(j)β−lnλw∑j=i+1(j)βλ(j)β]. |
In this section, the parameters λ and β are estimated using the maximum likelihood (ML) method.
Suppose x1,…,xn be a random sample from the DsPA distribution with pmf (6). Then the log-likelihood function takes the form
L=1[1−(i)βlnλ]λ(i)βw∑j=i+1[1−(j)βlnλ]λ(j)β. | (14) |
Now, by differentiating (14) w.r.t λ and β, we can write
∂L∂λ=n∑i=1lnλ[(xi+1)2βλ(xi+1)β−xi2βλxiβ]λ{[1−xiβlnλ]λxiβ−[1−(xi+1)βlnλ]λ(xi+1)β}=0 | (15) |
and
∂L∂β=n∑i=1(lnλ)2[λ(xi+1)β(xi+1)2βln(xi+1)−λxiβxi2βlnxi]{[1−xiβlnλ]λxiβ−[1−(xi+1)βlnλ]λ(xi+1)β}=0. | (16) |
The ML estimates (MLEs) of λ and β follow from the above equation. Eqs (15) and (16) can be solved using iterative procedures such as Newton-Raphson. For this purpose, we use the maxLik function of R software [26].
In this section, we carried out a numerical simulation to access the performance of the ML estimation method. This assessment is done by generating N=10,000 samples using the qf of the DsPA model for different sample sizes n=10,20,50, and 100 and for several values of the parameters λ and β, where (λ,β)=(0.50,0.50),(0.50,2.0),(0.90,1.20),(0.90,2.0). The assessment is completed using absolute bias, mean relative errors (MREs), and mean square errors (MSEs) which are defined by
Bias(δ)=1NN∑i=1|ˆδi−δ|,MSE(δ)=1NN∑i=1(ˆδi−δ)2andMRE(δ)=1NN∑i=1ˆδiδi, |
where δ=(λ,β).
The simulation results for λ and β are reported in Tables 6‒8. The bias, MSE and MRE of the parameters λ and β are computed using the R program using the ML method. For all values of λ and β, the ML estimation approach illustrates the consistency property, that is, the MSEs and MREs decrease as n increases.
n | E(λ) | E(β) | Bias(λ) | Bias(β) | MSE(λ) | MSE(β) | MRE(λ) | MRE(β) |
10 | 0.4605 | 0.5804 | -0.0395 | 0.0804 | 0.0483 | 0.0333 | 0.0789 | 0.1609 |
20 | 0.4796 | 0.5370 | -0.0204 | 0.0370 | 0.0247 | 0.0111 | 0.0409 | 0.0740 |
50 | 0.4910 | 0.5143 | -0.0090 | 0.0143 | 0.0098 | 0.0033 | 0.0180 | 0.0286 |
100 | 0.4966 | 0.5065 | -0.0034 | 0.0065 | 0.0049 | 0.0015 | 0.0069 | 0.0130 |
200 | 0.4975 | 0.5037 | -0.0025 | 0.0037 | 0.0024 | 0.0007 | 0.0050 | 0.0074 |
n | E(λ) | E(β) | Bias(λ) | Bias(β) | MSE(λ) | MSE(β) | MRE(λ) | MRE(β) |
10 | 0.4654 | 2.3160 | -0.0346 | 0.3160 | 0.0490 | 0.5537 | 0.0692 | 0.1580 |
20 | 0.4809 | 2.1419 | -0.0191 | 0.1419 | 0.0243 | 0.1730 | 0.0382 | 0.0709 |
50 | 0.4905 | 2.0571 | -0.0095 | 0.0571 | 0.0097 | 0.0514 | 0.0191 | 0.0286 |
100 | 0.4970 | 2.0243 | -0.0030 | 0.0243 | 0.0050 | 0.0247 | 0.0060 | 0.0122 |
200 | 0.4984 | 2.0125 | -0.0016 | 0.0125 | 0.0024 | 0.0115 | 0.0032 | 0.0062 |
n | E(λ) | E(β) | Bias(λ) | Bias(β) | MSE(λ) | MSE(β) | MRE(λ) | MRE(β) |
10 | 0.8630 | 1.3915 | -0.0370 | 0.1915 | 0.0990 | 0.1973 | 0.0411 | 0.1596 |
20 | 0.8843 | 1.2834 | -0.0157 | 0.0834 | 0.0473 | 0.0630 | 0.0174 | 0.0695 |
50 | 0.8926 | 1.2310 | -0.0074 | 0.0310 | 0.0181 | 0.0190 | 0.0082 | 0.0258 |
100 | 0.8972 | 1.2154 | -0.0028 | 0.0154 | 0.0088 | 0.0086 | 0.0031 | 0.0129 |
200 | 0.8980 | 1.2076 | -0.0020 | 0.0076 | 0.0045 | 0.0043 | 0.0022 | 0.0063 |
From Tables 6‒8, we conclude that:
1. The estimates of λ and β close to their true values with the increase of n for all studied cases.
2. The MSEs for λ and β decrease with the increase of n for all studied cases.
3. The MREs for λ and β decrease with the increase of n for all studied cases.
n | E(λ) | E(β) | Bias(λ) | Bias(β) | MSE(λ) | MSE(β) | MRE(λ) | MRE(β) |
10 | 0.8690 | 2.3159 | -0.0310 | 0.3159 | 0.1005 | 0.5381 | 0.0344 | 0.1580 |
20 | 0.8785 | 2.1443 | -0.0215 | 0.1443 | 0.0454 | 0.1699 | 0.0239 | 0.0722 |
50 | 0.8941 | 2.0523 | -0.0059 | 0.0523 | 0.0183 | 0.0535 | 0.0065 | 0.0261 |
100 | 0.8957 | 2.0278 | -0.0043 | 0.0278 | 0.0089 | 0.0246 | 0.0048 | 0.0139 |
200 | 0.8980 | 2.0139 | -0.0020 | 0.0139 | 0.0044 | 0.0117 | 0.0022 | 0.0070 |
In this section, we illustrate the importance of the newly DsPA distribution by utilizing three real-life datasets. We shall compare the fits of the DsPA distribution with the following competing discrete distributions which are reported in Table 10.
Model | Abbreviation | pmf |
Discrete Bur-Ⅻ | DsBⅫ | P(x)=λln(1+xα)−λln(1+(1+x)α). |
Uniform Poisson–Ailamujia | UPA | P(x)=2λ(1+2λ)−x−1. |
Poisson | Poi | P(x)=e−λλxx!. |
Discrete-Pareto | DsPr | P(x)=e−λln(1+x)−e−λln(2+x). |
Discrete-Rayleigh | DsR | P(x)=e−x22λ2−e−(x+1)22λ2. |
Discrete inverse-Rayleigh | DsIR | P(x)=e−λ(1+x)2−e−λx2. |
Discrete Burr-Hutke | DsBH | P(x)=(1x+1−λx+2)λx. |
The fitted distributions are compared using the negative maximum log-likelihood (-Loglik.), Akaike information criterion (AIC), Bayesian information criterion (BIC), and the p-value of Kolmogorov–Smirnov test (KS p-value).
Dataset Ⅰ: The first dataset is about the failure times for a sample of 15 electronic components in an acceleration life test [27]. The data observations are: 1.0, 5.0, 6.0, 11.0, 12.0, 19.0, 20.0, 22.0, 23.0, 31.0, 37.0, 46.0, 54.0, 60.0 and 66.0.
Dataset Ⅱ: The second dataset is about the number of fires in Greece from July 1, 1998 to August 31, 1998. This dataset is studied [28]. The data observations are: 0, 0, 0, 0, 0, 0, 0, 0, 0, 0, 0, 0, 0, 0, 0, 0, 1, 1, 1, 1, 1, 1, 1, 1, 1, 1, 1, 1, 1, 2, 2, 2, 2, 2, 2, 2, 2, 2, 2, 2, 2, 2, 2, 3, 3, 3, 3, 3, 3, 3, 3, 3, 4, 4, 4, 4, 4, 4, 4, 4, 4, 4, 4, 5, 5, 5, 5, 5, 5, 5, 5, 5, 5, 5, 5, 5, 6, 6, 6, 6, 6, 6, 6, 6, 7, 7, 7, 7, 8, 8, 8, 8, 8, 8, 8, 8, 8, 9, 9, 9, 9, 9, 9, 10, 10, 10, 11, 11, 11, 11, 12, 12, 12, 12, 12, 12, 15, 15, 15, 15, 16, 20 and 43.
Dataset Ⅲ: The third dataset consists of 48 final mathematics examination marks for slow-paced students in the Indian Institute of Technology at Kanpur. The data is analyzed by [29]. The observations are: 29, 25, 50, 15, 13, 27, 15, 18, 7, 7, 8, 19, 12, 18, 5, 21, 15, 86, 21, 15, 14, 39, 15, 14, 70, 44, 6, 23, 58, 19, 50, 23, 11, 6, 34, 18, 28, 34, 12, 37, 4, 60, 20, 23, 40, 65, 19 and 31.
The MLEs of the competing discrete models, standard errors (SEs), and goodness-of-fit measures are listed in Tables 11‒13 for the three datasets, respectively. For visual comparisons, the P-P (probability–probability) plots of fitted distributions are displayed in Figures 4, 6 and 8 for the analyzed datasets, respectively. Furthermore, the estimated cdf, sf, hrf of the DsPA distribution are depicted in Figures 5, 7 and 9, respectively.
Model | λ | α | Measures | |||||
MLE | SEs | MLE | SEs | -Loglik. | AIC | BIC | KS p-value | |
DsBⅫ | 0.9839 | 0.0355 | 20.868 | 46.483 | 75.69 | 155.38 | 156.80 | 0.0150 |
UPA | 0.0182 | 0.0047 | - | - | 65.00 | 132.00 | 132.71 | 0.6734 |
Poi | 27.535 | 1.3548 | - | - | 151.21 | 304.41 | 305.12 | 0.0180 |
DsPr | 0.3283 | 0.0848 | - | - | 77.40 | 156.80 | 157.51 | 0.0097 |
DsR | 24.384 | 3.1487 | - | - | 66.39 | 134.79 | 135.50 | 0.4300 |
DsIR | 42.021 | 11.243 | - | - | 83.99 | 169.97 | 170.68 | 0.0000 |
DsBH | 0.9992 | 0.0076 | - | - | 91.37 | 184.74 | 185.44 | 0.0000 |
DsPA | 0.8886 | 0.0674 | 0.8588 | 0.1738 | 64.49 | 131.58 | 132.97 | 0.9500 |
Model | λ | α | Measures | |||||
MLE | SE | MLE | SE | -Loglik. | AIC | BIC | KS p-value | |
DsBⅫ | 0.7612 | 0.0427 | 2.5026 | 0.4870 | 373.39 | 750.79 | 756.41 | 0.0000 |
UPA | 0.0926 | 0.0090 | - | - | 341.14 | 684.28 | 687.09 | 0.0028 |
Poi | 5.3988 | 0.2095 | - | - | 467.83 | 937.65 | 940.47 | 0.0000 |
DsPr | 0.6046 | 0.0546 | - | - | 389.64 | 781.27 | 784.08 | 0.0000 |
DsR | 5.6792 | 0.2567 | - | - | 385.25 | 772.49 | 775.31 | 0.0000 |
DsIR | 3.9959 | 0.3995 | - | - | 412.72 | 827.44 | 830.25 | 0.0000 |
DsBH | 0.9836 | 0.0127 | - | - | 407.16 | 816.31 | 819.12 | 0.0000 |
DsPA | 0.5812 | 0.0407 | 0.7709 | 0.0562 | 340.33 | 684.67 | 690.29 | 0.2484 |
Model | λ | α | Measures | |||||
MLE | SE | MLE | SE | -Loglik. | AIC | BIC | KS p-value | |
DsBⅫ | 0.9382 | 0.1926 | 5.1500 | 16.5597 | 247.48 | 498.97 | 502.71 | 0.0000 |
UPA | 0.0193 | 0.0028 | - | - | 205.11 | 412.22 | 414.09 | 0.0174 |
Poi | 25.8950 | 0.7345 | - | - | 396.59 | 795.18 | 797.05 | 0.0000 |
DsPr | 0.3225 | 0.0466 | - | - | 215.18 | 504.36 | 506.23 | 0.0000 |
DsR | 22.7562 | 1.6427 | - | - | 201.89 | 405.79 | 407.66 | 0.0460 |
DsIR | 177.56 | 26.02 | - | - | 205.13 | 412.27 | 414.14 | 0.0000 |
DsBH | 0.9990 | 0.0046 | - | - | 297.68 | 597.35 | 599.22 | 0.0000 |
DsPA | 0.9409 | 0.0231 | 1.0621 | 0.1113 | 197.44 | 398.88 | 402.62 | 0.8102 |
The findings in Tables 11‒13 illustrate that the DsPA distribution provides a superior fit over other competing discrete models, since it has the lowest values for all measures and the largest K-S p-value.
In this study, a new one-parameter discrete model is proposed as a good alternative to some well-known discrete distributions. The newly introduced model is called the discrete-power-Ailamujia (DsPA) distribution. Some statistical properties of the DsPA distribution are derived. Its parameters are estimated by the maximum likelihood method. A simulation study is carried out to check the performance of the estimators. It is observed that the maximum likelihood method is efficient in estimating the DsPA parameters for large samples. Finally, three real-world datasets are analyzed to check the usefulness and applicability of the DsPA distribution. The goodness-of-fit measures and figures show that the DsPA distribution is a useful attractive alternative for competing discrete models.
This project was funded by the Deanship of Scientific Research (DSR) at King Abdulaziz University, Jeddah, Saudi Arabia under Grant No. (G: 047-662-1442). The authors, therefore, acknowledge with thanks DSR for technical and financial support.
The authors declare no conflict of interest.
[1] |
A. Dobreva, R. Paus, N. Cogan, Toward predicting the spatio-temporal dynamics of alopecia areata lesions using partial differential equation analysis, Bull. Math. Biol., 82 (2020), 1–32. https://doi.org/10.1007/s11538-020-00707-0 doi: 10.1007/s11538-020-00707-0
![]() |
[2] | A. Gilhar, A. Etzioni, R. Paus, Alopecia areata, N. Engl. J. Med., 366 (2012), 1515–1525. https://doi.org/10.1056/NEJMra1103442 |
[3] |
A. Luster, J. Ravetch, Biochemical characterization of a gamma interferon-inducible cytokine (IP-10), J. Exp. Med., 166 (1987), 1084–1097. https://doi.org/10.1084/jem.166.4.1084 doi: 10.1084/jem.166.4.1084
![]() |
[4] |
Y. Lou, Y. Tao, The role of local kinetics in a three-component chemotaxis model for Alopecia Areata, J. Differ. Equation, 305 (2021), 401–427. https://doi.org/10.1016/j.jde.2021.10.020 doi: 10.1016/j.jde.2021.10.020
![]() |
[5] |
Y. Tao, D. Xu, Combined effects of nonlinear proliferation and logistic damping in a three-component chemotaxis system for alopecia areata, Nonlinear Anal. Real World Appl., 66 (2022), 103517. https://doi.org/10.1016/j.nonrwa.2022.103517 doi: 10.1016/j.nonrwa.2022.103517
![]() |
[6] |
E. Keller, L. Segel, Initiation of slime mold aggregation viewed as an instability, J. Theoret. Biol., 26 (1970), 399–415. https://doi.org/10.1016/0022-5193(70)90092-5 doi: 10.1016/0022-5193(70)90092-5
![]() |
[7] |
M. Winkler, Aggregation vs. global diffusive behavior in the higher-dimensional Keller-Segel model, J. Differ. Equation, 248 (2010), 2889–2905. https://doi.org/10.1016/j.jde.2010.02.008 doi: 10.1016/j.jde.2010.02.008
![]() |
[8] |
G. Arumugam, J. Tyagi, Keller-Segel chemotaxis models: A review, Acta Appl. Math., 171 (2021), 1–82. https://doi.org/10.1007/s10440-020-00374-2 doi: 10.1007/s10440-020-00374-2
![]() |
[9] |
M. Winkler, Finite-time blow-up in the higher-dimensional parabolic-parabolic Keller-Segel system, J. Math. Pures Appl., 100 (2013), 748–767. https://doi.org/10.1016/j.matpur.2013.01.020 doi: 10.1016/j.matpur.2013.01.020
![]() |
[10] |
T. Xiang, On effects of sampling radius for the nonlocal Patlak-Keller-Segel chemotaxis model, Discrete Contin. Dyn. Syst., 34 (2014), 4911–4946. https://doi.org/10.3934/dcds.2014.34.4911 doi: 10.3934/dcds.2014.34.4911
![]() |
[11] |
T. Hillen, A. Potapov, The one-dimensional chemotaxis model: global existence and asymptotic profile, Math. Methods Appl. Sci., 27 (2004), 1783–1801. https://doi.org/10.1002/mma.569 doi: 10.1002/mma.569
![]() |
[12] |
H. Jin, T. Xiang, Chemotaxis effect vs. logistic damping on boundedness in the 2-D minimal Keller-Segel model, C. R. Math. Acad. Sci. Paris, 356 (2018), 875–885. https://doi.org/10.13140/RG.2.2.33597.36325 doi: 10.13140/RG.2.2.33597.36325
![]() |
[13] |
K. Osaki, T. Tsujikawa, A. Yagi, M. Mimura, Exponential attractor for a chemotaxis-growth system of equations, Nonlinear Anal., 51 (2002), 119–144. https://doi.org/10.1016/S0362-546X(01)00815-X doi: 10.1016/S0362-546X(01)00815-X
![]() |
[14] |
E. Nakaguchi, K. Osaki, Global solutions and exponential attractors of a parabolic-parabolic system for chemotaxis with subquadratic degradation, Discrete Contin. Dyn. Syst. Ser. B, 18 (2013), 2627–2646. https://doi.org/10.3934/dcdsb.2013.18.2627 doi: 10.3934/dcdsb.2013.18.2627
![]() |
[15] |
K. Lin, C. Mu, Global dynamics in a fully parabolic chemotaxis system with logistic source, Discrete Contin. Dyn. Syst., 36 (2016), 5025–5046. https://doi.org/10.3934/dcds.2016018 doi: 10.3934/dcds.2016018
![]() |
[16] |
T. Xiang, How strong a logistic damping can prevent blow-up for the minimal Keller-Segel chemotaxis system?, J. Math. Anal. Appl., 459 (2018), 1172–1200. https://doi.org/10.1016/j.jmaa.2017.11.022 doi: 10.1016/j.jmaa.2017.11.022
![]() |
[17] |
M. Winkler, Boundedness in the higher-dimensional parabolic-parabolic chemotaxis system with logistic source, Commun. Partial. Differ. Equation, 35 (2010), 1516–1537. https://doi.org/10.1080/03605300903473426 doi: 10.1080/03605300903473426
![]() |
[18] |
M. Winkler, How far do chemotaxis-driven forces influence regularity in the Navier-Stokes system?, Trans. Am. Math. Soc., 369 (2017), 3067–3125. http://dx.doi.org/10.1090/tran/6733 doi: 10.1090/tran/6733
![]() |
[19] |
M. Winkler, Global mass-preserving solutions in a two-dimensional chemotaxis-Stokes system with rotational flux components, J. Evol. Equation, 18 (2018), 1267–1289. https://doi.org/10.1007/s00028-018-0440-8 doi: 10.1007/s00028-018-0440-8
![]() |
[20] |
J. Zheng, An optimal result for global existence and boundedness in a three-dimensional Keller-Segel-Stokes system with nonlinear diffusion, J. Differ. Equation, 267 (2019), 2385–2415. https://doi.org/10.1016/j.jde.2019.03.013 doi: 10.1016/j.jde.2019.03.013
![]() |
[21] |
J. Zheng, A new result for the global existence (and boundedness) and regularity of a three-dimensional Keller-Segel-Navier-Stokes system modeling coral fertilization, J. Differ. Equation, 272 (2021), 164-202. https://doi.org/10.1016/j.jde.2020.09.029 doi: 10.1016/j.jde.2020.09.029
![]() |
[22] |
J. Zheng, Boundedness of solutions to a quasilinear parabolic-elliptic Keller-Segel system with logistic source, J. Differ. Equation, 259 (2015), 120-140. https://doi.org/10.1016/j.jde.2015.02.003 doi: 10.1016/j.jde.2015.02.003
![]() |
[23] |
J. Zheng, Eventual smoothness and stabilization in a three-dimensional Keller-Segel-Navier-Stokes system with rotational flux, Calc. Var. Partial Differ. Equations, 61 (2022), 52. https://doi.org/10.1007/s00526-021-02164-6 doi: 10.1007/s00526-021-02164-6
![]() |
[24] |
Y. Ke, J. Zheng, An optimal result for global existence in a three-dimensional Keller-Segel-Navier-Stokes system involving tensor-valued sensitivity with saturation, Calc. Var. Partial Differ. Equations, 58 (2019), 1–27. https://doi.org/10.1007/s00526-019-1568-2 doi: 10.1007/s00526-019-1568-2
![]() |
[25] |
N. Bellomo, A. Bellouquid, Y. Tao, M. Winkler, Toward a mathematical theory of Keller-Segel models of pattern formation in biological tissues, Math. Models Methods Appl. Sci., 25 (2015), 1663–1763. https://doi.org/10.1142/S021820251550044X doi: 10.1142/S021820251550044X
![]() |
[26] |
Y. Tian, D. Li, C. Mu, Stabilization in three-dimensional chemotaxis-growth model with indirect attractant production, C. R. Math. Acad. Sci. Paris, 357 (2019), 513–519. https://doi.org/10.1016/j.crma.2019.05.010 doi: 10.1016/j.crma.2019.05.010
![]() |
[27] |
C. Mu, W. Tao, Stabilization and pattern formation in chemotaxis models with acceleration and logistic source, Math. Biosci. Eng., 20 (2023), 2011–2038. https://doi.org/10.3934/mbe.2023093 doi: 10.3934/mbe.2023093
![]() |
[28] |
Y. Tao, M. Winkler, Taxis-driven Formation of Singular Hotspots in a May–Nowak Type Model for Virus Infection, SIAM J. Math. Anal., 53 (2021), 1411–1433. https://doi.org/10.1137/20M1362851 doi: 10.1137/20M1362851
![]() |
[29] |
T. Alzahrani, E. Raluca, T. Dumitru, Multiscale modelling of cancer response to oncolytic viral therapy, Math. Biosci., 310 (2019), 76–95. https://doi.org/10.1016/j.mbs.2018.12.018 doi: 10.1016/j.mbs.2018.12.018
![]() |
[30] |
N. Bellomo, K. Painter, Y. Tao, M. Winkler, Occurrence vs. Absence of taxis-driven instabilities in a may-nowak model for virus infection, SIAM J. Appl. Math., 79 (2019), 1990–2010. https://doi.org/10.1137/19M1250261 doi: 10.1137/19M1250261
![]() |
[31] |
Y. Tao, M. Winkler, Boundedness and decay enforced by quadratic degradation in a three-dimensional chemotaxis-fluid system, Z. Angew. Math. Phys., 66 (2015), 2555–2573. https://doi.org/10.1007/s00033-015-0541-y doi: 10.1007/s00033-015-0541-y
![]() |
[32] |
B. Hu, Y. Tao, To the exclusion of blow-up in a three-dimensional chemotaxis-growth model with indirect attractant production, Math. Models Methods Appl. Sci., 26 (2016), 2111–2128. https://doi.org/10.1142/S0218202516400091 doi: 10.1142/S0218202516400091
![]() |
[33] |
Y. Tao, M. Winkler, Large time behavior in a multidimensional chemotaxis-haptotaxis model with slow signal diffusion, SIAM J. Math. Anal., 47 (2015), 4229–4250. https://doi.org/10.1137/15M1014115 doi: 10.1137/15M1014115
![]() |
[34] |
D. Horstmann, M. Winkler, Boundedness vs. blow-up in a chemotaxis system, J. Differ. Equation, 215 (2005), 52–107. https://doi.org/10.1016/j.jde.2004.10.022 doi: 10.1016/j.jde.2004.10.022
![]() |
[35] |
Y. Tao, Z. Wang, Competing effects of attraction vs. repulsion in chemotaxis, Math. Models Methods Appl. Sci., 23 (2013), 1–36. https://doi.org/10.1142/S0218202512500443 doi: 10.1142/S0218202512500443
![]() |
[36] |
K. Fujie, A. Ito, M. Winkler, T. Yokota, Stabilization in a chemotaxis model for tumor invasion, Discrete Contin. Dyn. Syst., 36 (2016), 151-169. https://doi.org/10.3934/dcds.2016.36.151 doi: 10.3934/dcds.2016.36.151
![]() |
[37] |
P. Lions, Résolution de problemes elliptiques quasilinéaires, Arch. Ration. Mech. Anal., 74 (1980), 335–353. https://doi.org/10.1007/BF00249679 doi: 10.1007/BF00249679
![]() |
[38] |
R. Kowalczyk, Z. Szymańska, On the global existence of solutions to an aggregation model, J. Math. Anal. Appl., 343 (2008), 379–398. https://doi.org/10.1016/j.jmaa.2008.01.005 doi: 10.1016/j.jmaa.2008.01.005
![]() |
[39] | X. Bai, M. Winkler, Equilibration in a fully parabolic two-species chemotaxis system with competitive kinetics, Indiana Univ. Math. J., 65 (2016), 553–583. http://www.jstor.org/stable/26318163 |
[40] |
M. Porzio, V. Vespri, Hölder estimates for local solutions of some doubly nonlinear degenerate parabolic equations, J. Differ. Equation, 103 (1993), 146–178. https://doi.org/10.1006/jdeq.1993.1045 doi: 10.1006/jdeq.1993.1045
![]() |
[41] |
Y. Tao, M. Winkler, Boundedness and stabilization in a population model with cross-diffusion for one species, Proc. Lond. Math. Soc., 119 (2019), 1598–1632. https://doi.org/10.1112/plms.12276 doi: 10.1112/plms.12276
![]() |
[42] | G. Lieberman, Second order parabolic differential equations, World Sci., 1996 (1996). https://doi.org/10.1142/3302 |
1. | Ahmed Z. Afify, Muhammad Ahsan-ul-Haq, Hassan M. Aljohani, Abdulaziz S. Alghamdi, Ayesha Babar, Héctor W. Gómez, A new one-parameter discrete exponential distribution: Properties, inference, and applications to COVID-19 data, 2022, 34, 10183647, 102199, 10.1016/j.jksus.2022.102199 | |
2. | Mohamed S. Eliwa, Muhammad Ahsan-ul-Haq, Amani Almohaimeed, Afrah Al-Bossly, Mahmoud El-Morshedy, Barbara Martinucci, Discrete Extension of Poisson Distribution for Overdispersed Count Data: Theory and Applications, 2023, 2023, 2314-4785, 1, 10.1155/2023/2779120 | |
3. | Muhammad Ahsan-ul-Haq, On Poisson Moment Exponential Distribution with Applications, 2022, 2198-5804, 10.1007/s40745-022-00400-0 | |
4. | Muhammad Ahsan-ul-Haq, Javeria Zafar, A new one-parameter discrete probability distribution with its neutrosophic extension: mathematical properties and applications, 2023, 2364-415X, 10.1007/s41060-023-00382-z | |
5. | Amani Alrumayh, Hazar A. Khogeer, A New Two-Parameter Discrete Distribution for Overdispersed and Asymmetric Data: Its Properties, Estimation, Regression Model, and Applications, 2023, 15, 2073-8994, 1289, 10.3390/sym15061289 | |
6. | Refah Alotaibi, Hoda Rezk, Chanseok Park, Ahmed Elshahhat, The Discrete Exponentiated-Chen Model and Its Applications, 2023, 15, 2073-8994, 1278, 10.3390/sym15061278 | |
7. | Hassan M. Aljohani, Muhammad Ahsan-ul-Haq, Javeria Zafar, Ehab M. Almetwally, Abdulaziz S. Alghamdi, Eslam Hussam, Abdisalam Hassan Muse, Analysis of Covid-19 data using discrete Marshall–Olkinin Length Biased Exponential: Bayesian and frequentist approach, 2023, 13, 2045-2322, 10.1038/s41598-023-39183-6 | |
8. | Safar M. Alghamdi, Muhammad Ahsan-ul-Haq, Olayan Albalawi, Majdah Mohammed Badr, Eslam Hussam, H.E. Semary, M.A. Abdelkawy, Binomial Poisson Ailamujia model with statistical properties and application, 2024, 17, 16878507, 101096, 10.1016/j.jrras.2024.101096 | |
9. | Muhammad Ahsan-ul-Haq, Muhammad N. S. Hussain, Ayesha Babar, Laila A. Al-Essa, Mohamed S. Eliwa, Barbara Martinucci, Analysis, Estimation, and Practical Implementations of the Discrete Power Quasi‐Xgamma Distribution, 2024, 2024, 2314-4629, 10.1155/2024/1913285 | |
10. | Khlood Al-Harbi, Aisha Fayomi, Hanan Baaqeel, Amany Alsuraihi, A Novel Discrete Linear-Exponential Distribution for Modeling Physical and Medical Data, 2024, 16, 2073-8994, 1123, 10.3390/sym16091123 | |
11. | Seth Borbye, Suleman Nasiru, Kingsley Kuwubasamni Ajongba, Vladimir Mityushev, Poisson XRani Distribution: An Alternative Discrete Distribution for Overdispersed Count Data, 2024, 2024, 0161-1712, 10.1155/2024/5554949 | |
12. | Ahmed Sedky Eldeeb, Muhammad Ahsan-ul-Haq, Ayesha Babar, A new discrete XLindley distribution: theory, actuarial measures, inference, and applications, 2024, 17, 2364-415X, 323, 10.1007/s41060-023-00395-8 |
β | λ | ||||||||
0.1 | 0.2 | 0.3 | 0.4 | 0.5 | 0.6 | 0.7 | 0.8 | 0.9 | |
0.5 | 0.7603 | 1.8909 | 3.6766 | 6.5446 | 10.966 | 16.848 | 22.277 | 22.458 | 12.187 |
1.0 | 0.3954 | 0.7529 | 1.1657 | 1.6848 | 2.3863 | 3.4156 | 5.1075 | 8.4629 | 18.452 |
1.5 | 0.3415 | 0.5827 | 0.8221 | 1.0907 | 1.4187 | 1.8533 | 2.4910 | 3.5895 | 6.2446 |
2.0 | 0.3313 | 0.5338 | 0.7085 | 0.8884 | 1.0967 | 1.3599 | 1.7259 | 2.3141 | 3.5954 |
2.5 | 0.3303 | 0.5230 | 0.6698 | 0.8012 | 0.9444 | 1.1259 | 1.3763 | 1.7634 | 2.5557 |
3.0 | 0.3303 | 0.5219 | 0.6619 | 0.7720 | 0.8721 | 0.9919 | 1.1725 | 1.4628 | 2.0209 |
β | λ | ||||||||
0.1 | 0.2 | 0.3 | 0.4 | 0.5 | 0.6 | 0.7 | 0.8 | 0.9 | |
0.5 | 2.7920 | 12.243 | 38.665 | 103.06 | 229.88 | 417.28 | 621.34 | 779.36 | 615.37 |
1.0 | 0.3901 | 0.8140 | 1.4378 | 2.4502 | 4.2371 | 7.7429 | 15.802 | 40.249 | 177.94 |
1.5 | 0.2475 | 0.3692 | 0.4988 | 0.6724 | 0.9299 | 1.3485 | 2.1203 | 3.8858 | 10.421 |
2.0 | 0.2236 | 0.2727 | 0.3017 | 0.3478 | 0.4232 | 0.5408 | 0.7369 | 1.1271 | 2.2935 |
2.5 | 0.2213 | 0.2517 | 0.2384 | 0.2287 | 0.2486 | 0.3033 | 0.3864 | 0.5260 | 0.8911 |
3.0 | 0.2212 | 0.2496 | 0.2252 | 0.1869 | 0.1626 | 0.1789 | 0.2448 | 0.3256 | 0.4729 |
β | λ | ||||||||
0.1 | 0.2 | 0.3 | 0.4 | 0.5 | 0.6 | 0.7 | 0.8 | 0.9 | |
0.5 | 4.6187 | 4.3533 | 3.9822 | 3.2831 | 2.4657 | 1.7555 | 1.2590 | 1.1461 | 2.0439 |
1.0 | 1.6228 | 1.4038 | 1.3664 | 1.3697 | 1.3821 | 1.3943 | 1.4037 | 1.4099 | 1.3511 |
1.5 | 0.9461 | 0.5856 | 0.5417 | 0.5744 | 0.6191 | 0.6586 | 0.6895 | 0.7124 | 0.7285 |
2.0 | 0.7459 | 0.1163 | -0.0092 | 0.1018 | 0.2223 | 0.2912 | 0.3312 | 0.3598 | 0.3836 |
2.5 | 0.7226 | -0.0655 | -0.4988 | -0.4984 | -0.1012 | 0.1683 | 0.1697 | 0.1591 | 0.1742 |
3.0 | 0.7218 | -0.0869 | -0.6648 | -1.0922 | -0.9663 | -0.0481 | 0.3705 | 0.0738 | 0.0476 |
β | λ | ||||||||
0.1 | 0.2 | 0.3 | 0.4 | 0.5 | 0.6 | 0.7 | 0.8 | 0.9 | |
0.5 | 41.471 | 36.784 | 28.534 | 18.030 | 10.188 | 5.7950 | 3.7130 | 3.1485 | 5.9942 |
1.0 | 5.9572 | 5.6739 | 5.7391 | 5.8240 | 5.8909 | 5.9380 | 5.9690 | 5.9875 | 5.4737 |
1.5 | 2.5630 | 2.7503 | 3.1474 | 3.3764 | 3.4912 | 3.5508 | 3.5857 | 3.6093 | 3.6262 |
2.0 | 1.6369 | 1.5891 | 2.4907 | 3.0833 | 3.1595 | 3.0954 | 3.0599 | 3.0514 | 3.0542 |
2.5 | 1.5246 | 1.0744 | 1.8108 | 3.2354 | 3.9993 | 3.4076 | 2.8767 | 2.8838 | 2.8977 |
3.0 | 1.5211 | 1.0098 | 1.4967 | 2.7867 | 4.9278 | 5.5909 | 3.4605 | 2.4008 | 2.8802 |
β | λ | ||||||||
0.1 | 0.2 | 0.3 | 0.4 | 0.5 | 0.6 | 0.7 | 0.8 | 0.9 | |
0.5 | 3.6720 | 6.4746 | 10.516 | 15.747 | 20.964 | 24.768 | 27.891 | 34.703 | 50.494 |
1.0 | 0.9866 | 1.0810 | 1.2335 | 1.4543 | 1.7756 | 2.2669 | 3.0939 | 4.7559 | 9.6437 |
1.5 | 0.7248 | 0.6337 | 0.6068 | 0.6165 | 0.6555 | 0.7276 | 0.8512 | 1.0826 | 1.6688 |
2.0 | 0.6749 | 0.5109 | 0.4258 | 0.3915 | 0.3859 | 0.3977 | 0.4269 | 0.4870 | 0.6379 |
2.5 | 0.6699 | 0.4813 | 0.3559 | 0.2854 | 0.2632 | 0.2694 | 0.2808 | 0.2983 | 0.3487 |
3.0 | 0.6697 | 0.4782 | 0.3402 | 0.2422 | 0.1865 | 0.1804 | 0.2088 | 0.2226 | 0.2340 |
n | E(λ) | E(β) | Bias(λ) | Bias(β) | MSE(λ) | MSE(β) | MRE(λ) | MRE(β) |
10 | 0.4605 | 0.5804 | -0.0395 | 0.0804 | 0.0483 | 0.0333 | 0.0789 | 0.1609 |
20 | 0.4796 | 0.5370 | -0.0204 | 0.0370 | 0.0247 | 0.0111 | 0.0409 | 0.0740 |
50 | 0.4910 | 0.5143 | -0.0090 | 0.0143 | 0.0098 | 0.0033 | 0.0180 | 0.0286 |
100 | 0.4966 | 0.5065 | -0.0034 | 0.0065 | 0.0049 | 0.0015 | 0.0069 | 0.0130 |
200 | 0.4975 | 0.5037 | -0.0025 | 0.0037 | 0.0024 | 0.0007 | 0.0050 | 0.0074 |
n | E(λ) | E(β) | Bias(λ) | Bias(β) | MSE(λ) | MSE(β) | MRE(λ) | MRE(β) |
10 | 0.4654 | 2.3160 | -0.0346 | 0.3160 | 0.0490 | 0.5537 | 0.0692 | 0.1580 |
20 | 0.4809 | 2.1419 | -0.0191 | 0.1419 | 0.0243 | 0.1730 | 0.0382 | 0.0709 |
50 | 0.4905 | 2.0571 | -0.0095 | 0.0571 | 0.0097 | 0.0514 | 0.0191 | 0.0286 |
100 | 0.4970 | 2.0243 | -0.0030 | 0.0243 | 0.0050 | 0.0247 | 0.0060 | 0.0122 |
200 | 0.4984 | 2.0125 | -0.0016 | 0.0125 | 0.0024 | 0.0115 | 0.0032 | 0.0062 |
n | E(λ) | E(β) | Bias(λ) | Bias(β) | MSE(λ) | MSE(β) | MRE(λ) | MRE(β) |
10 | 0.8630 | 1.3915 | -0.0370 | 0.1915 | 0.0990 | 0.1973 | 0.0411 | 0.1596 |
20 | 0.8843 | 1.2834 | -0.0157 | 0.0834 | 0.0473 | 0.0630 | 0.0174 | 0.0695 |
50 | 0.8926 | 1.2310 | -0.0074 | 0.0310 | 0.0181 | 0.0190 | 0.0082 | 0.0258 |
100 | 0.8972 | 1.2154 | -0.0028 | 0.0154 | 0.0088 | 0.0086 | 0.0031 | 0.0129 |
200 | 0.8980 | 1.2076 | -0.0020 | 0.0076 | 0.0045 | 0.0043 | 0.0022 | 0.0063 |
n | E(λ) | E(β) | Bias(λ) | Bias(β) | MSE(λ) | MSE(β) | MRE(λ) | MRE(β) |
10 | 0.8690 | 2.3159 | -0.0310 | 0.3159 | 0.1005 | 0.5381 | 0.0344 | 0.1580 |
20 | 0.8785 | 2.1443 | -0.0215 | 0.1443 | 0.0454 | 0.1699 | 0.0239 | 0.0722 |
50 | 0.8941 | 2.0523 | -0.0059 | 0.0523 | 0.0183 | 0.0535 | 0.0065 | 0.0261 |
100 | 0.8957 | 2.0278 | -0.0043 | 0.0278 | 0.0089 | 0.0246 | 0.0048 | 0.0139 |
200 | 0.8980 | 2.0139 | -0.0020 | 0.0139 | 0.0044 | 0.0117 | 0.0022 | 0.0070 |
Model | Abbreviation | pmf |
Discrete Bur-Ⅻ | DsBⅫ | P(x)=λln(1+xα)−λln(1+(1+x)α). |
Uniform Poisson–Ailamujia | UPA | P(x)=2λ(1+2λ)−x−1. |
Poisson | Poi | P(x)=e−λλxx!. |
Discrete-Pareto | DsPr | P(x)=e−λln(1+x)−e−λln(2+x). |
Discrete-Rayleigh | DsR | P(x)=e−x22λ2−e−(x+1)22λ2. |
Discrete inverse-Rayleigh | DsIR | P(x)=e−λ(1+x)2−e−λx2. |
Discrete Burr-Hutke | DsBH | P(x)=(1x+1−λx+2)λx. |
Model | λ | α | Measures | |||||
MLE | SEs | MLE | SEs | -Loglik. | AIC | BIC | KS p-value | |
DsBⅫ | 0.9839 | 0.0355 | 20.868 | 46.483 | 75.69 | 155.38 | 156.80 | 0.0150 |
UPA | 0.0182 | 0.0047 | - | - | 65.00 | 132.00 | 132.71 | 0.6734 |
Poi | 27.535 | 1.3548 | - | - | 151.21 | 304.41 | 305.12 | 0.0180 |
DsPr | 0.3283 | 0.0848 | - | - | 77.40 | 156.80 | 157.51 | 0.0097 |
DsR | 24.384 | 3.1487 | - | - | 66.39 | 134.79 | 135.50 | 0.4300 |
DsIR | 42.021 | 11.243 | - | - | 83.99 | 169.97 | 170.68 | 0.0000 |
DsBH | 0.9992 | 0.0076 | - | - | 91.37 | 184.74 | 185.44 | 0.0000 |
DsPA | 0.8886 | 0.0674 | 0.8588 | 0.1738 | 64.49 | 131.58 | 132.97 | 0.9500 |
Model | λ | α | Measures | |||||
MLE | SE | MLE | SE | -Loglik. | AIC | BIC | KS p-value | |
DsBⅫ | 0.7612 | 0.0427 | 2.5026 | 0.4870 | 373.39 | 750.79 | 756.41 | 0.0000 |
UPA | 0.0926 | 0.0090 | - | - | 341.14 | 684.28 | 687.09 | 0.0028 |
Poi | 5.3988 | 0.2095 | - | - | 467.83 | 937.65 | 940.47 | 0.0000 |
DsPr | 0.6046 | 0.0546 | - | - | 389.64 | 781.27 | 784.08 | 0.0000 |
DsR | 5.6792 | 0.2567 | - | - | 385.25 | 772.49 | 775.31 | 0.0000 |
DsIR | 3.9959 | 0.3995 | - | - | 412.72 | 827.44 | 830.25 | 0.0000 |
DsBH | 0.9836 | 0.0127 | - | - | 407.16 | 816.31 | 819.12 | 0.0000 |
DsPA | 0.5812 | 0.0407 | 0.7709 | 0.0562 | 340.33 | 684.67 | 690.29 | 0.2484 |
Model | λ | α | Measures | |||||
MLE | SE | MLE | SE | -Loglik. | AIC | BIC | KS p-value | |
DsBⅫ | 0.9382 | 0.1926 | 5.1500 | 16.5597 | 247.48 | 498.97 | 502.71 | 0.0000 |
UPA | 0.0193 | 0.0028 | - | - | 205.11 | 412.22 | 414.09 | 0.0174 |
Poi | 25.8950 | 0.7345 | - | - | 396.59 | 795.18 | 797.05 | 0.0000 |
DsPr | 0.3225 | 0.0466 | - | - | 215.18 | 504.36 | 506.23 | 0.0000 |
DsR | 22.7562 | 1.6427 | - | - | 201.89 | 405.79 | 407.66 | 0.0460 |
DsIR | 177.56 | 26.02 | - | - | 205.13 | 412.27 | 414.14 | 0.0000 |
DsBH | 0.9990 | 0.0046 | - | - | 297.68 | 597.35 | 599.22 | 0.0000 |
DsPA | 0.9409 | 0.0231 | 1.0621 | 0.1113 | 197.44 | 398.88 | 402.62 | 0.8102 |
β | λ | ||||||||
0.1 | 0.2 | 0.3 | 0.4 | 0.5 | 0.6 | 0.7 | 0.8 | 0.9 | |
0.5 | 0.7603 | 1.8909 | 3.6766 | 6.5446 | 10.966 | 16.848 | 22.277 | 22.458 | 12.187 |
1.0 | 0.3954 | 0.7529 | 1.1657 | 1.6848 | 2.3863 | 3.4156 | 5.1075 | 8.4629 | 18.452 |
1.5 | 0.3415 | 0.5827 | 0.8221 | 1.0907 | 1.4187 | 1.8533 | 2.4910 | 3.5895 | 6.2446 |
2.0 | 0.3313 | 0.5338 | 0.7085 | 0.8884 | 1.0967 | 1.3599 | 1.7259 | 2.3141 | 3.5954 |
2.5 | 0.3303 | 0.5230 | 0.6698 | 0.8012 | 0.9444 | 1.1259 | 1.3763 | 1.7634 | 2.5557 |
3.0 | 0.3303 | 0.5219 | 0.6619 | 0.7720 | 0.8721 | 0.9919 | 1.1725 | 1.4628 | 2.0209 |
β | λ | ||||||||
0.1 | 0.2 | 0.3 | 0.4 | 0.5 | 0.6 | 0.7 | 0.8 | 0.9 | |
0.5 | 2.7920 | 12.243 | 38.665 | 103.06 | 229.88 | 417.28 | 621.34 | 779.36 | 615.37 |
1.0 | 0.3901 | 0.8140 | 1.4378 | 2.4502 | 4.2371 | 7.7429 | 15.802 | 40.249 | 177.94 |
1.5 | 0.2475 | 0.3692 | 0.4988 | 0.6724 | 0.9299 | 1.3485 | 2.1203 | 3.8858 | 10.421 |
2.0 | 0.2236 | 0.2727 | 0.3017 | 0.3478 | 0.4232 | 0.5408 | 0.7369 | 1.1271 | 2.2935 |
2.5 | 0.2213 | 0.2517 | 0.2384 | 0.2287 | 0.2486 | 0.3033 | 0.3864 | 0.5260 | 0.8911 |
3.0 | 0.2212 | 0.2496 | 0.2252 | 0.1869 | 0.1626 | 0.1789 | 0.2448 | 0.3256 | 0.4729 |
β | λ | ||||||||
0.1 | 0.2 | 0.3 | 0.4 | 0.5 | 0.6 | 0.7 | 0.8 | 0.9 | |
0.5 | 4.6187 | 4.3533 | 3.9822 | 3.2831 | 2.4657 | 1.7555 | 1.2590 | 1.1461 | 2.0439 |
1.0 | 1.6228 | 1.4038 | 1.3664 | 1.3697 | 1.3821 | 1.3943 | 1.4037 | 1.4099 | 1.3511 |
1.5 | 0.9461 | 0.5856 | 0.5417 | 0.5744 | 0.6191 | 0.6586 | 0.6895 | 0.7124 | 0.7285 |
2.0 | 0.7459 | 0.1163 | -0.0092 | 0.1018 | 0.2223 | 0.2912 | 0.3312 | 0.3598 | 0.3836 |
2.5 | 0.7226 | -0.0655 | -0.4988 | -0.4984 | -0.1012 | 0.1683 | 0.1697 | 0.1591 | 0.1742 |
3.0 | 0.7218 | -0.0869 | -0.6648 | -1.0922 | -0.9663 | -0.0481 | 0.3705 | 0.0738 | 0.0476 |
β | λ | ||||||||
0.1 | 0.2 | 0.3 | 0.4 | 0.5 | 0.6 | 0.7 | 0.8 | 0.9 | |
0.5 | 41.471 | 36.784 | 28.534 | 18.030 | 10.188 | 5.7950 | 3.7130 | 3.1485 | 5.9942 |
1.0 | 5.9572 | 5.6739 | 5.7391 | 5.8240 | 5.8909 | 5.9380 | 5.9690 | 5.9875 | 5.4737 |
1.5 | 2.5630 | 2.7503 | 3.1474 | 3.3764 | 3.4912 | 3.5508 | 3.5857 | 3.6093 | 3.6262 |
2.0 | 1.6369 | 1.5891 | 2.4907 | 3.0833 | 3.1595 | 3.0954 | 3.0599 | 3.0514 | 3.0542 |
2.5 | 1.5246 | 1.0744 | 1.8108 | 3.2354 | 3.9993 | 3.4076 | 2.8767 | 2.8838 | 2.8977 |
3.0 | 1.5211 | 1.0098 | 1.4967 | 2.7867 | 4.9278 | 5.5909 | 3.4605 | 2.4008 | 2.8802 |
β | λ | ||||||||
0.1 | 0.2 | 0.3 | 0.4 | 0.5 | 0.6 | 0.7 | 0.8 | 0.9 | |
0.5 | 3.6720 | 6.4746 | 10.516 | 15.747 | 20.964 | 24.768 | 27.891 | 34.703 | 50.494 |
1.0 | 0.9866 | 1.0810 | 1.2335 | 1.4543 | 1.7756 | 2.2669 | 3.0939 | 4.7559 | 9.6437 |
1.5 | 0.7248 | 0.6337 | 0.6068 | 0.6165 | 0.6555 | 0.7276 | 0.8512 | 1.0826 | 1.6688 |
2.0 | 0.6749 | 0.5109 | 0.4258 | 0.3915 | 0.3859 | 0.3977 | 0.4269 | 0.4870 | 0.6379 |
2.5 | 0.6699 | 0.4813 | 0.3559 | 0.2854 | 0.2632 | 0.2694 | 0.2808 | 0.2983 | 0.3487 |
3.0 | 0.6697 | 0.4782 | 0.3402 | 0.2422 | 0.1865 | 0.1804 | 0.2088 | 0.2226 | 0.2340 |
n | E(λ) | E(β) | Bias(λ) | Bias(β) | MSE(λ) | MSE(β) | MRE(λ) | MRE(β) |
10 | 0.4605 | 0.5804 | -0.0395 | 0.0804 | 0.0483 | 0.0333 | 0.0789 | 0.1609 |
20 | 0.4796 | 0.5370 | -0.0204 | 0.0370 | 0.0247 | 0.0111 | 0.0409 | 0.0740 |
50 | 0.4910 | 0.5143 | -0.0090 | 0.0143 | 0.0098 | 0.0033 | 0.0180 | 0.0286 |
100 | 0.4966 | 0.5065 | -0.0034 | 0.0065 | 0.0049 | 0.0015 | 0.0069 | 0.0130 |
200 | 0.4975 | 0.5037 | -0.0025 | 0.0037 | 0.0024 | 0.0007 | 0.0050 | 0.0074 |
n | E(λ) | E(β) | Bias(λ) | Bias(β) | MSE(λ) | MSE(β) | MRE(λ) | MRE(β) |
10 | 0.4654 | 2.3160 | -0.0346 | 0.3160 | 0.0490 | 0.5537 | 0.0692 | 0.1580 |
20 | 0.4809 | 2.1419 | -0.0191 | 0.1419 | 0.0243 | 0.1730 | 0.0382 | 0.0709 |
50 | 0.4905 | 2.0571 | -0.0095 | 0.0571 | 0.0097 | 0.0514 | 0.0191 | 0.0286 |
100 | 0.4970 | 2.0243 | -0.0030 | 0.0243 | 0.0050 | 0.0247 | 0.0060 | 0.0122 |
200 | 0.4984 | 2.0125 | -0.0016 | 0.0125 | 0.0024 | 0.0115 | 0.0032 | 0.0062 |
n | E(λ) | E(β) | Bias(λ) | Bias(β) | MSE(λ) | MSE(β) | MRE(λ) | MRE(β) |
10 | 0.8630 | 1.3915 | -0.0370 | 0.1915 | 0.0990 | 0.1973 | 0.0411 | 0.1596 |
20 | 0.8843 | 1.2834 | -0.0157 | 0.0834 | 0.0473 | 0.0630 | 0.0174 | 0.0695 |
50 | 0.8926 | 1.2310 | -0.0074 | 0.0310 | 0.0181 | 0.0190 | 0.0082 | 0.0258 |
100 | 0.8972 | 1.2154 | -0.0028 | 0.0154 | 0.0088 | 0.0086 | 0.0031 | 0.0129 |
200 | 0.8980 | 1.2076 | -0.0020 | 0.0076 | 0.0045 | 0.0043 | 0.0022 | 0.0063 |
n | E(λ) | E(β) | Bias(λ) | Bias(β) | MSE(λ) | MSE(β) | MRE(λ) | MRE(β) |
10 | 0.8690 | 2.3159 | -0.0310 | 0.3159 | 0.1005 | 0.5381 | 0.0344 | 0.1580 |
20 | 0.8785 | 2.1443 | -0.0215 | 0.1443 | 0.0454 | 0.1699 | 0.0239 | 0.0722 |
50 | 0.8941 | 2.0523 | -0.0059 | 0.0523 | 0.0183 | 0.0535 | 0.0065 | 0.0261 |
100 | 0.8957 | 2.0278 | -0.0043 | 0.0278 | 0.0089 | 0.0246 | 0.0048 | 0.0139 |
200 | 0.8980 | 2.0139 | -0.0020 | 0.0139 | 0.0044 | 0.0117 | 0.0022 | 0.0070 |
Model | Abbreviation | pmf |
Discrete Bur-Ⅻ | DsBⅫ | P(x)=λln(1+xα)−λln(1+(1+x)α). |
Uniform Poisson–Ailamujia | UPA | P(x)=2λ(1+2λ)−x−1. |
Poisson | Poi | P(x)=e−λλxx!. |
Discrete-Pareto | DsPr | P(x)=e−λln(1+x)−e−λln(2+x). |
Discrete-Rayleigh | DsR | P(x)=e−x22λ2−e−(x+1)22λ2. |
Discrete inverse-Rayleigh | DsIR | P(x)=e−λ(1+x)2−e−λx2. |
Discrete Burr-Hutke | DsBH | P(x)=(1x+1−λx+2)λx. |
Model | λ | α | Measures | |||||
MLE | SEs | MLE | SEs | -Loglik. | AIC | BIC | KS p-value | |
DsBⅫ | 0.9839 | 0.0355 | 20.868 | 46.483 | 75.69 | 155.38 | 156.80 | 0.0150 |
UPA | 0.0182 | 0.0047 | - | - | 65.00 | 132.00 | 132.71 | 0.6734 |
Poi | 27.535 | 1.3548 | - | - | 151.21 | 304.41 | 305.12 | 0.0180 |
DsPr | 0.3283 | 0.0848 | - | - | 77.40 | 156.80 | 157.51 | 0.0097 |
DsR | 24.384 | 3.1487 | - | - | 66.39 | 134.79 | 135.50 | 0.4300 |
DsIR | 42.021 | 11.243 | - | - | 83.99 | 169.97 | 170.68 | 0.0000 |
DsBH | 0.9992 | 0.0076 | - | - | 91.37 | 184.74 | 185.44 | 0.0000 |
DsPA | 0.8886 | 0.0674 | 0.8588 | 0.1738 | 64.49 | 131.58 | 132.97 | 0.9500 |
Model | λ | α | Measures | |||||
MLE | SE | MLE | SE | -Loglik. | AIC | BIC | KS p-value | |
DsBⅫ | 0.7612 | 0.0427 | 2.5026 | 0.4870 | 373.39 | 750.79 | 756.41 | 0.0000 |
UPA | 0.0926 | 0.0090 | - | - | 341.14 | 684.28 | 687.09 | 0.0028 |
Poi | 5.3988 | 0.2095 | - | - | 467.83 | 937.65 | 940.47 | 0.0000 |
DsPr | 0.6046 | 0.0546 | - | - | 389.64 | 781.27 | 784.08 | 0.0000 |
DsR | 5.6792 | 0.2567 | - | - | 385.25 | 772.49 | 775.31 | 0.0000 |
DsIR | 3.9959 | 0.3995 | - | - | 412.72 | 827.44 | 830.25 | 0.0000 |
DsBH | 0.9836 | 0.0127 | - | - | 407.16 | 816.31 | 819.12 | 0.0000 |
DsPA | 0.5812 | 0.0407 | 0.7709 | 0.0562 | 340.33 | 684.67 | 690.29 | 0.2484 |
Model | λ | α | Measures | |||||
MLE | SE | MLE | SE | -Loglik. | AIC | BIC | KS p-value | |
DsBⅫ | 0.9382 | 0.1926 | 5.1500 | 16.5597 | 247.48 | 498.97 | 502.71 | 0.0000 |
UPA | 0.0193 | 0.0028 | - | - | 205.11 | 412.22 | 414.09 | 0.0174 |
Poi | 25.8950 | 0.7345 | - | - | 396.59 | 795.18 | 797.05 | 0.0000 |
DsPr | 0.3225 | 0.0466 | - | - | 215.18 | 504.36 | 506.23 | 0.0000 |
DsR | 22.7562 | 1.6427 | - | - | 201.89 | 405.79 | 407.66 | 0.0460 |
DsIR | 177.56 | 26.02 | - | - | 205.13 | 412.27 | 414.14 | 0.0000 |
DsBH | 0.9990 | 0.0046 | - | - | 297.68 | 597.35 | 599.22 | 0.0000 |
DsPA | 0.9409 | 0.0231 | 1.0621 | 0.1113 | 197.44 | 398.88 | 402.62 | 0.8102 |