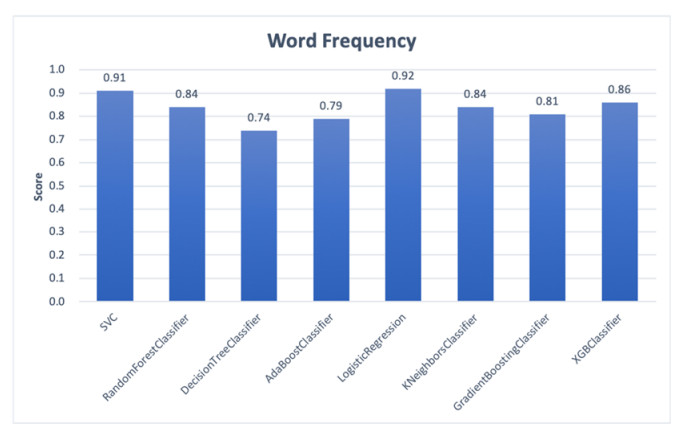
Recent decades have witnessed the rapid development of literary studies on gender and writing style. One of the common limitations of previous studies is that they analyze only a few texts, which some researchers have already pointed out. In this study, we attempt to find the features that best facilitate the classification of texts by authorial gender. Based on a corpus of 1113 classical fictions from the early 19th century to the early 20th century. Eight algorithms, including SVM, random forest, decision tree, AdaBoost, logistic regression, K-nearest neighbors, gradient boosting and XGBoost, are used to automatically select the features that are most useful for properly categorizing a text. We find that word frequency is the most important predictor for identifying authorial gender in classical fictions, achieving an accuracy rate of 92%. We also find that nationhood is not particularly impactful when dealing with authorial gender differences in classical fictions, as genderlectal variation is 'universal' in the English-speaking world.
Citation: Dan Zhu, Liru Yang, Xin Liang. Gender classification in classical fiction: A computational analysis of 1113 fictions[J]. Mathematical Biosciences and Engineering, 2022, 19(9): 8892-8907. doi: 10.3934/mbe.2022412
[1] | Zahra Movahedi Nia, Ali Ahmadi, Bruce Mellado, Jianhong Wu, James Orbinski, Ali Asgary, Jude D. Kong . Twitter-based gender recognition using transformers. Mathematical Biosciences and Engineering, 2023, 20(9): 15962-15981. doi: 10.3934/mbe.2023711 |
[2] | Natalya Shakhovska, Vitaliy Yakovyna, Valentyna Chopyak . A new hybrid ensemble machine-learning model for severity risk assessment and post-COVID prediction system. Mathematical Biosciences and Engineering, 2022, 19(6): 6102-6123. doi: 10.3934/mbe.2022285 |
[3] | Ansheng Ye, Xiangbing Zhou, Kai Weng, Yu Gong, Fang Miao, Huimin Zhao . Image classification of hyperspectral remote sensing using semi-supervised learning algorithm. Mathematical Biosciences and Engineering, 2023, 20(6): 11502-11527. doi: 10.3934/mbe.2023510 |
[4] | Lili Jiang, Sirong Chen, Yuanhui Wu, Da Zhou, Lihua Duan . Prediction of coronary heart disease in gout patients using machine learning models. Mathematical Biosciences and Engineering, 2023, 20(3): 4574-4591. doi: 10.3934/mbe.2023212 |
[5] | Jiang Xie, Junfu Xu, Celine Nie, Qing Nie . Machine learning of swimming data via wisdom of crowd and regression analysis. Mathematical Biosciences and Engineering, 2017, 14(2): 511-527. doi: 10.3934/mbe.2017031 |
[6] | Xianli Liu, Yongquan Zhou, Weiping Meng, Qifang Luo . Functional extreme learning machine for regression and classification. Mathematical Biosciences and Engineering, 2023, 20(2): 3768-3792. doi: 10.3934/mbe.2023177 |
[7] | Yingjian Yang, Wei Li, Yingwei Guo, Nanrong Zeng, Shicong Wang, Ziran Chen, Yang Liu, Huai Chen, Wenxin Duan, Xian Li, Wei Zhao, Rongchang Chen, Yan Kang . Lung radiomics features for characterizing and classifying COPD stage based on feature combination strategy and multi-layer perceptron classifier. Mathematical Biosciences and Engineering, 2022, 19(8): 7826-7855. doi: 10.3934/mbe.2022366 |
[8] | Chaofan Li, Qiong Liu, Kai Ma . DCCL: Dual-channel hybrid neural network combined with self-attention for text classification. Mathematical Biosciences and Engineering, 2023, 20(2): 1981-1992. doi: 10.3934/mbe.2023091 |
[9] | Liangyu Yang, Tianyu Shi, Jidong Lv, Yan Liu, Yakang Dai, Ling Zou . A multi-feature fusion decoding study for unilateral upper-limb fine motor imagery. Mathematical Biosciences and Engineering, 2023, 20(2): 2482-2500. doi: 10.3934/mbe.2023116 |
[10] | Ning Huang, Zhengtao Xi, Yingying Jiao, Yudong Zhang, Zhuqing Jiao, Xiaona Li . Multi-modal feature fusion with multi-head self-attention for epileptic EEG signals. Mathematical Biosciences and Engineering, 2024, 21(8): 6918-6935. doi: 10.3934/mbe.2024304 |
Recent decades have witnessed the rapid development of literary studies on gender and writing style. One of the common limitations of previous studies is that they analyze only a few texts, which some researchers have already pointed out. In this study, we attempt to find the features that best facilitate the classification of texts by authorial gender. Based on a corpus of 1113 classical fictions from the early 19th century to the early 20th century. Eight algorithms, including SVM, random forest, decision tree, AdaBoost, logistic regression, K-nearest neighbors, gradient boosting and XGBoost, are used to automatically select the features that are most useful for properly categorizing a text. We find that word frequency is the most important predictor for identifying authorial gender in classical fictions, achieving an accuracy rate of 92%. We also find that nationhood is not particularly impactful when dealing with authorial gender differences in classical fictions, as genderlectal variation is 'universal' in the English-speaking world.
The question of identifying and interpreting possible differences in linguistic styles between males and females has been discussed by linguistic researchers since the 1970s. One of the most distinguishing works from that time might be Lakoff's [1] 1973 Language and the Woman's Place. Since then, the linguistic landscape has already identified some 'universal' differences between males and females [2] For example, females are more likely than males to focus 'relationships' [3,4] and use facilitative tag questions [5] and they also use more compliments and apologies [6,7].
By the end of the last century, almost all studies on genderlectal variation focused on speech and other high-interaction linguistic modalities. Formal written texts, on the other hand, were often neglected since it was believed that they were written for a massive, unseen audience, lacking conversational and intonational cues that can be found only in speech. Some researchers [8,9] point out that there is no stylistic difference between male and female authors in their formal written texts. In the early 21st century, Argamon et al. [10] conducted a computer-based study on gender stylistics in formal written texts. It was a major breakthrough that they find male authors to focus on 'informativeness' and female authors on 'involvement' within formal written texts. From the perspective of computer science, the most important work on genderlectal variation is identifying the most effective predictors. Earlier corpus-based studies, on the other hand, often define word classes first and then use these classes to compare across genders.
There is another fitting example that shows that automatic prediction of authorial gender is more objective than other methods developed thus far. In 2011, Burger et al. [11] used 184,000 Twitter blog profiles to identify authorial gender based on gender metadata. The end result shows that the automatic prediction of authorial gender can achieve a higher rate of accuracy than the bare judgments of human raters. Other related studies [12] have also proven that genderlectal variation exists in science essays, political [13] and legislative [14] speeches, and poetry [15].
It seems that word choice always plays a crucial role in differentiating authorial genders. One notable study on this matter is by Newman et al. [16], who analyze the different word choices of male and female authors over 14,000 text files. He finds that women tend to use words that are associated with psychological and social processes, while men use more words that refer to object properties and impersonal topics. As Pennebaker [17] goes notes in another study, he finds that 'women use first person singular, cognitive, and social words more; men use articles more; and there are no meaningful differences between men and women for first person plural or positive emotion words'. Baker's [18] Using Corpora to Analyze Gender is another good example of this. In his second chapter, he uses the 'keywords technique' to produce lists of words that show the greatest difference in relative frequency.
Our study mainly focuses on identifying authorial gender in fictions. In regard to fiction creation, we point to Gustave Flaubert's [19] famous quote, 'It is a delicious thing to write, to be no longer yourself but to move in an entire universe of your own creating. Today, for instance, as man and woman, both lover and mistress, I rode in a forest on an autumn afternoon under the yellow leaves, and I was also the horses, the leaves, the wind, the words my people uttered, even the red sun that made them almost close their love-drowned eyes.' One might therefore worry that if authors can "get inside" the mind of a different gender, the gender difference would be reduced or even eliminated in such fiction texts. Luckily, this worry can be quelled with the help of Pennebaker [17], who finds that Shakespeare and Tarantino's male and female characters all use function words in a masculine style. In other words, Shakespeare and Tarantino failed to truly "get inside" the female mind when they were creating their female characters.
In fiction, one of the most important existing studies on authorial gender identification was conducted by Koppel et al. [20], who achieved an accuracy rate of approximately 80% based on function words and parts-of-speech. They also find that the accuracy of their technique does not differ very much between fiction and nonfiction. The most recent decade witnessed significant development in genderlectal variation studies based on computer science. Matt Jocker [21] takes gender as an individual genre in his stylometric analysis. According to his study, gender is 'a bit player' that accounts for approximately 8% of the overall results in classification tests and linear regression tests. Notably, he lists some words that are particularly favored by male and female authors and several authors who are particularly difficult to classify by gender based on their written works alone. Additionally, Rybicki's [22] 'Vive la difference: Tracing the (Authorial) Gender Signal by Multivariate Analysis of Word Frequencies' is considered 'a considerable step in the right direction' [23]. Rybicki conducts multivariate statistical analysis to find gender-sensitive words in 18th- and 19th-century English fictions. Notably, Rybicki also analyzes a large number of modern fictions and compares them to 18th- and 19th-century English fictions, indicating that the use of gender-sensitive words may begin to fade over time. Grayson, Mulvany, Wade, Meaney and Greene [24] explore genderlectal variation in 48 19th-century fictions from the perspective of embedded words, and their results correspond with those of Argamon et al. [10]. Weidman and O'Sullivan [23] also analyze gender-sensitive words across a selection of male and female authors based on the study by Rybicki [22]. They find that males seem to write somewhat similarly to other males, while females exhibit much greater changes in their gender-sensitive words.
Hoover [15] proposes that 'any study of the vocabulary of male and female poets would benefit from larger numbers of poets and larger samples, and many other configurations that address different contrasts are possible (e.g., nationality or historical period)'. Similarly, Grayson et al. [24] also mention that they wished they had a larger corpus for their embedding analysis. Mindful of these critiques, we apply two major improvements in our study. One is that we use a larger corpus of fiction texts, so our corpus is ten times larger (1113 fictions) than Jocker's (106 fictions). The other improvement is that we take 'nationality' into consideration, since the 'historical period' issue has already been well discussed by Rybicki [22] and Weidman and O'Sullivan [23].
It is also interesting to discuss some interesting questions from Rybicki [22] and Jocker [21]. As Rybicki [22] mentions, 'Little in terms of a theoretical basis that would explain why and how most frequent word frequencies usually work so much better in authorial attribution than any other features', so one of the objectives of this paper is to consider the features that best facilitate the classification of texts by author gender. In addition, this paper also tries to reidentify authorial gender in George Eliot's Middle March and Mary Elizabeth Braddon's works, which are particularly difficult to classify by author gender [21]. Last but not the least, similar to many genderlectal variation studies, this paper also identifies some word clusters that are particularly common in male- and female-authored texts.
As we explore the possible variation between male and female writing styles in classical English fictions, a large variety of algorithms are applied to identify several classes of typical lexical and syntactic features whose occurrences in texts differ distinctly according to authorial gender, in both US fictions and British fictions.
The greatest challenge in any gender study is perhaps the bias we live with. It has been argued that [25] many gender-based linguistics studies are methodologically flawed because they assume that differences exist first and then fish around to identify them. To circumvent aside such bias, large dataset studies have mushroomed with the help of modern computer science. Among the methods used by such studies, machine learning is one of the most reliable. As a newly adapted method in the field of digital humanities, we stand upon the shoulders of giants since computational linguistics is literally a myth at the end of the last century. In 1988, Potter mentions that 'until everything has been encoded, or until encoding is a trivial part of the work, the everyday critic will probably not consider computer treatments of texts' [26]. Since then, the path to digital humanities was not entirely free of obstacles due to 'some early concerns and several contemporary detractors' [21]. The year 2008 was full of crisis, and Gottschall has paralleled economic crisis with crisis of literary studies. In his book Literature, Science, and a New Humanities, he argues that literary studies have met their crisis and we must find new methods and new theoretical construct to make a solid ground for them [27]. Today, machine learning is often recognized as a highly objective method in gender-based text analysis, since experiment plays a crucial part as its solid foundation. The primary task of such experiment is to identify an unknown text based on known texts. The researchers use a set of known texts to 'train' the computer so that the computer can 'learn' the texts and build a specific model for them. After this is done, the computer can analyze new texts by placing it into the most similar of the previously defined categories that the model learned. At this stage, an 80% success rate in identifying authorial gender can usually be seen as a strong signal of accuracy [20,21].
We now have a clear understanding of the manipulation of machine learning in a genderlectal variation study. The readers can see, it is evident that the corpus and algorithm are two important factors owing to the essence of this research method.
We used a corpus consisting of 1113 fictions covering a large range of different genres. First, we checked The Cambridge History of The American Novel [28] and The Columbia History of the British Novel [29] and drew all the authors' names thoroughly from the index of these two books. Based on these authors' names, we downloaded as many texts as we could from DigiLibraries.com, given that these texts are freely available to the public for academic purposes.
At the time this study was performed, the corpus contained 781 male-authored fictions and 332 female-authored fictions. The fictions came from the early 19th century to the early 20th century. We excluded fictions that were published prior to the 19th century because few female authors were found before that time anyway. We also excluded fictions that were created by multiple authors of different genders (e.g., The Kempton-Wace Letters was authored by Jack London and Anna Strunsky). In such texts, the writers did not have a specific notion of the gender of their intended audiences so that any differences in the essence of texts reflect only the characteristics of the writers instead of those of the audiences.
The full dataset contains 102,846,113 words, and the average document length is 92,404 words. It has 15,266,486 sentence segments divided by the following punctuation marks: '; ' '.' '!' or '?'. It has 6,228,790 sentence segments divided by the following punctuation marks: '.' '!' or '?'.
We used 8 algorithms to automatically select the features that are most useful for properly categorizing a text. The use of multifactorial analysis is worth highlighting. The broad range of machine learning methods we use in this study have been proven reliable for text categorization. Specifically, a small number of labeled texts are used in a training corpus to construct the primary patterns of male- or female-authored texts. Then, these patterns are used to test the unlabeled texts to see if they are similar to the primary patterns. Ultimately, some have high levels of similarity and others low. In this way, some strong predictors for female authors can be found through machine learning. In other words, machine learning attempts to classify a work of unknown or disputed gender based on a training set of works whose author's gender is known.
The 8 algorithms that we apply in our study are typical in machine learning, including support vector machine, random forest, decision tree, AdaBoost algorithm, logistic regression, K-nearest neighbors, gradient boosting decision tree and XGBoost.
By calculating the frequency of each word in the corpus, we can identify the top 500 most frequent words. The 500 most frequent words are then used as 500 vectors. For each fiction, the number of words in each vector are calculated, 500 vectors (transverse) among 1113 fictions (vertical) are as follows:
After normalization, we have the following normalized 500 vectors (transverse) among 1113 fictions (vertical):
These vectors are categorized by male-authored fictions and female-authored fictions. The program is then trained on these two categories. In this stage, a process of iterative sampling is needed. An iterative experiment is conducted in which nine-tenths of the fictions in the dataset are selected at random for training a model, and the remaining fictions are withheld as 'test' data for classification. As the model simultaneously runs 8 kinds of algorithms, the tenfold cross-validation provides the resulting rates of error. These errors are recorded and then averaged to generate an overall estimate of accuracy for each category.
The average rates of accuracy are recorded in Figure 1. The cross-validation results indicate that logistic regression has the best results among all the algorithms in this study. Having achieved an average accuracy of 92%, 'word frequency' can be seen as a strong predictor of gender.
To investigate this matter thoroughly, we also conduct related experiments that use smaller numbers of vectors according to word importance instead of larger numbers of vectors according to word frequency. Our first step is to determine the importance of the 500 most frequent words by using the random forest algorithm (Table 1).
Word | Feature Importance |
happy | 0.015415813066918298 |
man | 0.013996896255355567 |
she | 0.011996387660753836 |
his | 0.011891455060731124 |
home | 0.011704461925716985 |
child | 0.011550413330974568 |
herself | 0.010310487749005634 |
always | 0.010101111537435687 |
her | 0.009470560413592646 |
never | 0.008365112290874992 |
became | 0.007798501713097518 |
already | 0.006989098645835062 |
mother | 0.006897225927142129 |
little | 0.006747038370581187 |
upon | 0.006172451248293137 |
… | … |
We choose the top 10, 20, 50 and 100 words according to word importance and use them as vectors in the 8 algorithms. Four relative experiments are then conducted by using the same method as in our first experiment (Figure 2).
We find that the average accuracy rates of word importance in the top 10, 20, 50 and 100 words are 82, 84, 86, and 87%, respectively. It is evident that the average accuracy rate is strongly associated with the number of vectors: The more vectors we use in our experiments, the higher the accuracy we can obtain. This is true at least in a corpus that contains 1113 fictions.
In addition, we realized that US authors and British authors may have different word frequencies in their fictions. To be rigorous, we therefore use US fictions and British fictions as 2 sub-corpuses to re-examine this matter.
We exclude authors from other nations, such as India and Canada, and those who lived in both Britain and the US. The sub-corpus of British fictions has 400 male-authored fictions and 196 female-authored fictions, while the subcorpus of US fictions has 307 male-authored fictions and 61 female-authored fictions. The end results are very similar to our first experiment (Figures 3 and 4), which tells us that nationhood (whether Britain or the United States) is not particularly important when identifying authorial gender in classical fictions.
There are a variety of part-of-speech (POS) taggers available for this kind of work, and all of them have advantages and disadvantages. The TextBlob POS tagger used in this research, for example, is known to be highly accurate; it has given 32 POS taggers for English vocabulary. Similar to the previous experiment, we use these predefined labels in the 8 algorithms. The TextBlob POS tagger reaches an accuracy rate of 80% in identifying authorial gender through logistic regression, although the TextBlob POS tagger might not be as sensitive to literary prose as another tagger trained on a corpus of classical fictions (Figure 5). A gender signal is evident here, given that at 80% accuracy, it is a strong signal.
To work specifically with sentence length, we divide our 1113 fictions into 15,266,486 sentence segments divided by the following punctuation '; ' '.' '!' '?' or ','. The number of words in each segment is calculated in Figure 6.
An overview of sentence length in these 15,266,486 segments tells us that most sentences contain 1–25 words. Based on this fact, we use 25 vectors for sentence length from 1 word to 25 words. These 25 vectors are then put into the 8 algorithms, similar to the first experiment.
The average rates of accuracy are recorded in Figure 7. The cross-validation results indicate that all the algorithms in this study have similar results. Sentence length has an accuracy of 70% in predicting authorial gender.
To investigate this matter thoroughly, we also use the punctuation marks '.' '!' or '?' to identify sentences in texts. The corpus has 6,228,790 sentence segments divided by such punctuation marks. Since most sentences contain 1–50 words, (Figure 8) we use 50 labels for sentence length from 1 to 50 words. Then, we repeat the experiment and obtain results that are very similar to those of the previous experiment (Figure 9).
From the two sets of experiments, we find that sentence length as a predictor has a success rate of 70% in identifying authorial gender.
To date, few studies have utilized predictor length in machine-learning-based text analysis. This study takes predictor length into the consideration as a possible gender predictor.
Statistics tell us that most words in this corpus contain 1–15 letters. Similar to what we do for sentence length as a predictor, we use 15 labels in the 8 algorithms. As a result, we find that word length as a predictor has an accuracy rate of 71% (Figure 10) in identifying authorial gender, which is almost the same as sentence length as a predictor.
The results of this study correspond with those of Argamon et al. [10], who have identified gender difference in language use from formal written texts. This study has also met its primary objectives. Our study clearly shows that word frequency is the most important predictor of authorial gender in classical fictions. Part-of-speech comes next: It is a strong signal but not as obvious as word frequency. In a corpus that contains 1113 fictions, a large number of vectors have high accuracy. Among the 8 algorithms, SVC and logistic regression outperform the other algorithms. The results reported in this paper are pleasantly surprising, as the highest accuracy rate reaches 92%. This is far beyond our expectations because our corpus covers various fictional genres and themes, not to mention authors with different backgrounds and upbringing. Nationhood is not particularly impactful when dealing with authorial gender differences in classical fictions, as genderlectal variation is 'universal' in the English-speaking world, at least from the early 19th century to the early 20th century.
Now, we can finally return to one of our initial questions: Can we find a way to identify authorial gender in George Eliot's Middle March and Mary Elizabeth Braddon's works? The answer is yes. We use 500 frequent words as 500 vectors and logistic regression as a trained algorithm to examine Middle March from Eliot and ten fictions from Braddon. These fictions are all from female authors (Figures 11 and 12, Table 2).
Fiction | Author | Female likelihood |
Henry Dunbar: A Novel | M. E. Braddon | 92% |
The Golden Calf | M. E. Braddon | 100% |
Birds of Prey | M. E. Braddon | 100% |
Lady Audley's Secret | M. E. Braddon | 100% |
Phantom Fortune, a Novel | M. E. Braddon | 97% |
London Pride, Or, When the World Was Younger | M. E. Braddon | 100% |
The Lovels of Arden | M. E. Braddon | 100% |
Fenton's Quest | M. E. Braddon | 95% |
Charlotte's Inheritance | M. E. Braddon | 90% |
Run to Earth: A Novel | M. E. Braddon | 98% |
Middle March | George Eliot | 100% |
Given that our corpus contains more than one hundred million words, it is also necessary to analyze male/female frequent word lists. Here, we should bear in mind that gender predictors may begin to fade over time [22]; what we discuss here are genderlectal variations from the early 19th century to the early 20th century.
Based on the top 800 most frequent words from male and female authors, this study identifies several gender-sensitive word clusters:
(1) Positive & negative emotion words
It is obvious that female authors overall use more positive emotion words than male authors (happy, smile, smiled, laugh, laughed, sweet, beautiful, bright, comfort, joy). Differences in word choices among negative emotion words are not as obvious as what we can observe in the 'positive emotion' word cluster. This result corroborates Augustine, Mehl and Larsen's findings, which indicate that 'women display a larger positivity bias in naturalistic speech' and the human tend to use more positive words than negative words [30].
(2) Number words
Male authors overall use more number words (one, two, three, four, five, six, ten, twenty, hundred, thousand) than female authors. This has already been pointed out by Pennebaker [17] and Rybicki [22]. What we want to add here is that the use of larger number words such as hundred and thousand is more common than the use of smaller number words.
(3) Temperature words
Female authors show more sensitivity to temperature words (cold, warm, hot, summer, and winter) than male authors do. This result is worth further studies because not so many researchers are aware of it.
(4) Family and social words
Expectedly, female authors use more terms of family members (family, husband, wife, brother, sister, uncle, aunt). In regard to social words, however, it is difficult to distinguish differences in word choice between male and female authors. Some social words, such as church and school, are more frequently used by female authors, while street and public are mainly used by male authors.
Gender shift might be one of the greatest challenges in differentiating authorial gender. In a recent study, Luoto [31] found that homosexual males can also produce some sorts of female-typical psycholinguistic outputs, which means that there exists a gray area between male-authored and female-authored fictions. However, it is not an easy task to resolve this problem, since sexual orientation was predominantly a dangerous topic in the 19th century, and the sexual orientation of individuals may also change over time.
Another limitation concerns the part-of-speech (POS) taggers used in our study. There are many POS taggers, such as TreeTagger, LingPipe, MorphAdorner and Stanford, available for this kind of work, and all of them have advantages and disadvantages. However, all these modern taggers are actually coded by humans because the tagger is produced from a certain trained corpus of (usually modern) texts. This is the same problem that Jocker encountered in his study [21].
The authors declare there is no conflict of interest.
[1] |
R. Lakoff, Language and woman's place, Lang. Soc., 2 (1973), 45–79. https://doi.org/10.1017/S0047404500000051 doi: 10.1017/S0047404500000051
![]() |
[2] |
J. Holmes, Women's talk: The question of sociolinguistic universals, Aust. J. Commun., 20 (1993), 125–149. https://doi.org/10.5588/pha.15.0018 doi: 10.5588/pha.15.0018
![]() |
[3] |
E. J. Aries, F. L. Johnson, Close friendship in adulthood: Conversational content between same-sex friends, Sex Roles, 9 (1983), 1183–1196. https://doi.org/10.1007/bf00303101 doi: 10.1007/bf00303101
![]() |
[4] | D. Tannen, Rethinking power and solidarity in gender and dominance, in Proceedings of the Annual Meeting of the Berkeley Linguistics Society, 16 (1990), 519. https://doi.org/10.3765/bls.v16i1.3433 |
[5] | J. Holmes, Women's language: A functional approach, Gen. Ling., 24 (1984), 149. |
[6] |
J. Holmes, Paying compliments: A sex-preferential positive politeness strategy, J. Pragmatics, 12 (1988), 445–465. https://doi.org/10.1016/0378-2166(88)90005-7 doi: 10.1016/0378-2166(88)90005-7
![]() |
[7] |
J. Holmes, Sex differences and apologies: One aspect of communicative competence, Appl. Ling., 10 (1989), 194–213. https://doi.org/10.1093/applin/10.2.194 doi: 10.1093/applin/10.2.194
![]() |
[8] |
C. L. Berryman-Fink, J. R. Wilcox, A multivariate investigation of perceptual attributions concerning gender appropriateness in language, Sex Roles, 9(1983), 663–681. https://doi.org/10.1007/BF00289796 doi: 10.1007/BF00289796
![]() |
[9] |
J. A. Simkins-Bullock, B. G. Wildman, An investigation into the relationship between gender and language, Sex Roles, 24 (1991), 149–160. https://doi.org/10.1007/BF00288888 doi: 10.1007/BF00288888
![]() |
[10] |
S. Argamon, M. Koppel, J. Fine, A. R. Shimoni, Gender, genre, and writing style in formal written texts, Text, 23 (2003), 321–346. https://doi.org/10.1515/text.2003.014 doi: 10.1515/text.2003.014
![]() |
[11] | J. D. Burger, J. C. Henderson, G. Kim, G. Zarrella, Discriminating gender on twitter, in Conference on Empirical Methods in Natural Language Processing, (2011), 1301–1309. Available from: https://dblp.uni-trier.de/rec/conf/emnlp/BurgerHKZ11.html. |
[12] | R. Sarawgi, K. Gajulapalli, Y. Choi, Gender attribution: Tracing stylometric evidence beyond topic and genre, in Proceedings of the Fifteenth Conference on Computational Natural Language Learning, (2011), 78–86. Available from: https://dblp.uni-trier.de/db/conf/conll/conll2011.html. |
[13] |
M. Dahllöf, Automatic prediction of gender, political affiliation, and age in Swedish politicians from the wording of their speeches—A comparative study of classifiability, Lit. Ling. Comput., 27 (2012), 139–153. https://doi.org/10.1093/llc/fqs010 doi: 10.1093/llc/fqs010
![]() |
[14] |
B. Yu, Language and gender in Congressional speech, Lit, Ling, Comput., 29 (2014), 118–132. https://doi.org/10.1093/llc/fqs073 doi: 10.1093/llc/fqs073
![]() |
[15] | D. L. Hoover, Textual analysis, in Literary Studies in the Digital Age (eds. K. M. Price and R. Siemens), 2013. Available from: http://dlsanthology.commons.mla.org/textual-analysis/. |
[16] |
M. L. Newman, C. J. Groom, L. D. Handelman, J. W. Pennebaker, Gender differences in language use: An analysis of 14,000 text samples, Discourse Processes, 45 (2008), 211–236. https://doi.org/10.1080/01638530802073712 doi: 10.1080/01638530802073712
![]() |
[17] | J. Pennebaker, The Secret Life of Pronouns: What Our Words Say about Us, Bloomsbury Press, London, (2011), 56. https://doi.org/10.1093/llc/fqt006 |
[18] | P. Baker, Using Corpora to Analyze Gender, Bloomsbury, London, 2014. https://doi.org/10.1016/j.system.2016.04.008 |
[19] | G. Flaubert, The Letters of Gustave Flaubert, 1830–1857, Harvard University Press, 1980. |
[20] |
M. Koppel, S. Argamon, A. R. Shimoni, Automatically categorizing written texts by author gender, Lit. Ling. Comput., 17 (2003), 401–412. https://doi.org/10.1093/llc/17.4.401 doi: 10.1093/llc/17.4.401
![]() |
[21] | M. Jockers, Macroanalysis: Digital Methods and Literary History, University of Illinois Press, Urbana, (2013), 93–99,133. https://doi.org/10.5406/illinois/9780252037528.001.0001 |
[22] |
J. Rybicki, Vive la difference: Tracing the (authorial) gender signal by multivariate analysis of word frequencies, Digital Scholarship Humanit., 31 (2016), 746–761. https://doi.org/10.1093/llc/fqv023 doi: 10.1093/llc/fqv023
![]() |
[23] |
S. G. Weidman, J. O'Sullivan, The limits of distinctive words: Re-evaluating literature's gender marker debate, Digital Scholarship Humanit., 33 (2018), 374–390. https://doi.org/10.1093/llc/fqx017 doi: 10.1093/llc/fqx017
![]() |
[24] | S. Grayson, M. Mulvany, K. Wade, G. Meaney, D. Greene, Exploring the role of gender in 19th century fiction through the lens of word embeddings, in 1st International Conference on Language, Data and Knowledge, (2017), 358–364. Available from: https://linkspringer.53yu.com/chapter/10.1007/978-3-319-59888-8_30. |
[25] |
V. Bergvall, Rethinking language and gender research: Theory and practice, J. Pragmatics, 29 (1996), 213–220. https://doi.org/10.1016/S0378-2166(97)82076-0 doi: 10.1016/S0378-2166(97)82076-0
![]() |
[26] |
R. Potter, Literary criticism and literary computing, Comput. Humanit., 22 (1988), 91–97. https://doi.org/10.2307/30200105 doi: 10.2307/30200105
![]() |
[27] | J, Gottschall, Literature, Science, and a New Humanities, Palgrave Macmillan, New York, 2008. https://doi.org/10.1057/9780230615595 |
[28] | L. Cassuto, C. V. Eby, B. Reiss, The Cambridge History of the American Novel, Cambridge University Press, 2011. https://doi.org/10.1017/CHOL9780521899079 |
[29] | J. Bender, D. David, M. Seidel, The Columbia History of the British Novel, Columbia University Press, 1994. https://doi.org/10.2307/3508695 |
[30] |
A. A. Augustine, M. R. Mehl, R. J. Larsen, A positivity bias in written and spoken English and its moderation by personality and gender, Social Psychol. Pers. Sci., 2 (2011), 508–515. https://doi.org/10.1177/1948550611399154 doi: 10.1177/1948550611399154
![]() |
[31] |
S. Luoto, Sexual dimorphism in language, and the gender shift hypothesis of homosexuality, Front. Psychol., 12 (2021), 1665. https://doi.org/10.3389/fpsyg.2021.639887 doi: 10.3389/fpsyg.2021.639887
![]() |
Word | Feature Importance |
happy | 0.015415813066918298 |
man | 0.013996896255355567 |
she | 0.011996387660753836 |
his | 0.011891455060731124 |
home | 0.011704461925716985 |
child | 0.011550413330974568 |
herself | 0.010310487749005634 |
always | 0.010101111537435687 |
her | 0.009470560413592646 |
never | 0.008365112290874992 |
became | 0.007798501713097518 |
already | 0.006989098645835062 |
mother | 0.006897225927142129 |
little | 0.006747038370581187 |
upon | 0.006172451248293137 |
… | … |
Fiction | Author | Female likelihood |
Henry Dunbar: A Novel | M. E. Braddon | 92% |
The Golden Calf | M. E. Braddon | 100% |
Birds of Prey | M. E. Braddon | 100% |
Lady Audley's Secret | M. E. Braddon | 100% |
Phantom Fortune, a Novel | M. E. Braddon | 97% |
London Pride, Or, When the World Was Younger | M. E. Braddon | 100% |
The Lovels of Arden | M. E. Braddon | 100% |
Fenton's Quest | M. E. Braddon | 95% |
Charlotte's Inheritance | M. E. Braddon | 90% |
Run to Earth: A Novel | M. E. Braddon | 98% |
Middle March | George Eliot | 100% |
Word | Feature Importance |
happy | 0.015415813066918298 |
man | 0.013996896255355567 |
she | 0.011996387660753836 |
his | 0.011891455060731124 |
home | 0.011704461925716985 |
child | 0.011550413330974568 |
herself | 0.010310487749005634 |
always | 0.010101111537435687 |
her | 0.009470560413592646 |
never | 0.008365112290874992 |
became | 0.007798501713097518 |
already | 0.006989098645835062 |
mother | 0.006897225927142129 |
little | 0.006747038370581187 |
upon | 0.006172451248293137 |
… | … |
Fiction | Author | Female likelihood |
Henry Dunbar: A Novel | M. E. Braddon | 92% |
The Golden Calf | M. E. Braddon | 100% |
Birds of Prey | M. E. Braddon | 100% |
Lady Audley's Secret | M. E. Braddon | 100% |
Phantom Fortune, a Novel | M. E. Braddon | 97% |
London Pride, Or, When the World Was Younger | M. E. Braddon | 100% |
The Lovels of Arden | M. E. Braddon | 100% |
Fenton's Quest | M. E. Braddon | 95% |
Charlotte's Inheritance | M. E. Braddon | 90% |
Run to Earth: A Novel | M. E. Braddon | 98% |
Middle March | George Eliot | 100% |