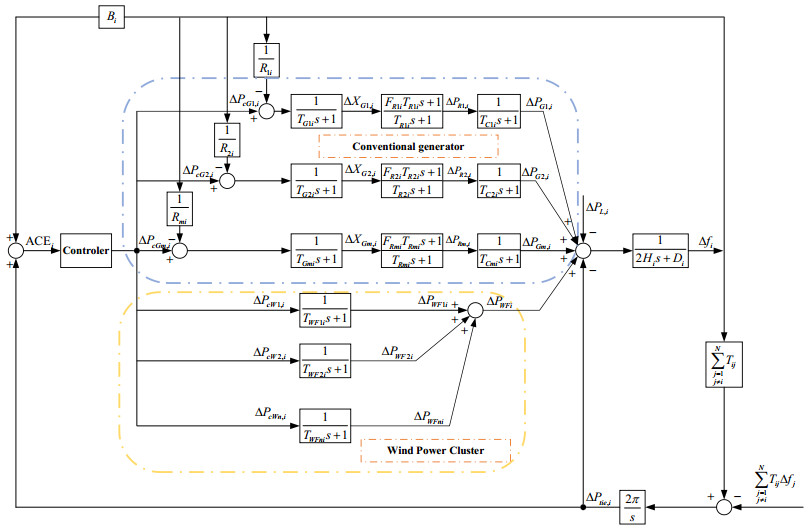
The increasing integration of large-scale wind power aggravates the difficulty of maintaining system frequency deviations in a certain range. The frequency regulation pressure of conventional generators increases, which requires wind farms to participate in system frequency regulation. In this paper, a multi-area interconnected power system frequency response model with wind power is established. Based on the frequency response model, the state space model of regional interconnected power system is presented. Then, the wind power variogram characteristics are introduced for estimating wind power variations in different time-scales. By predicting the wind power variations in AGC time-scale, a strategy of wind farm participating in AGC system is proposed and performed based on model predictive control (MPC). The control strategy makes the conventional units and wind farms to participate in AGC system coordinately. Simulation results are provided which verifies the feasibility and validity of the proposed strategy.
Citation: Qi Wang, Yufeng Guo, Dongrui Zhang, Yingwei Wang, Ying Xu, Jilai Yu. Research on wind farm participating in AGC based on wind power variogram characteristics[J]. Mathematical Biosciences and Engineering, 2022, 19(8): 8288-8303. doi: 10.3934/mbe.2022386
[1] | Yixin Zhuo, Ling Li, Jian Tang, Wenchuan Meng, Zhanhong Huang, Kui Huang, Jiaqiu Hu, Yiming Qin, Houjian Zhan, Zhencheng Liang . Optimal real-time power dispatch of power grid with wind energy forecasting under extreme weather. Mathematical Biosciences and Engineering, 2023, 20(8): 14353-14376. doi: 10.3934/mbe.2023642 |
[2] | Rami Al-Hajj, Gholamreza Oskrochi, Mohamad M. Fouad, Ali Assi . Probabilistic prediction intervals of short-term wind speed using selected features and time shift dependent machine learning models. Mathematical Biosciences and Engineering, 2025, 22(1): 23-51. doi: 10.3934/mbe.2025002 |
[3] | Xiaoqiang Dai, Kuicheng Sheng, Fangzhou Shu . Ship power load forecasting based on PSO-SVM. Mathematical Biosciences and Engineering, 2022, 19(5): 4547-4567. doi: 10.3934/mbe.2022210 |
[4] | Sue Wang, Jing Li, Saleem Riaz, Haider Zaman, Pengfei Hao, Yiwen Luo, Alsharef Mohammad, Ahmad Aziz Al-Ahmadi, NasimUllah . Duplex PD inertial damping control paradigm for active power decoupling of grid-tied virtual synchronous generator. Mathematical Biosciences and Engineering, 2022, 19(12): 12031-12057. doi: 10.3934/mbe.2022560 |
[5] | Jian Fang, Na Li, Chenhe Xu . A nonlocal population model for the invasion of Canada goldenrod. Mathematical Biosciences and Engineering, 2022, 19(10): 9915-9937. doi: 10.3934/mbe.2022462 |
[6] | Sue Wang, Chaohong Zhou, Saleem Riaz, Xuanchen Guo, Haider Zaman, Alsharef Mohammad, Ahmad Aziz Al-Ahmadi, Yasser Mohammed Alharbi, NasimUllah . Adaptive fuzzy-based stability control and series impedance correction for the grid-tied inverter. Mathematical Biosciences and Engineering, 2023, 20(2): 1599-1616. doi: 10.3934/mbe.2023073 |
[7] | Tangsheng Zhang, Hongying Zhi . A fuzzy set theory-based fast fault diagnosis approach for rotators of induction motors. Mathematical Biosciences and Engineering, 2023, 20(5): 9268-9287. doi: 10.3934/mbe.2023406 |
[8] | Yunfeng Liu, Guowei Sun, Lin Wang, Zhiming Guo . Establishing Wolbachia in the wild mosquito population: The effects of wind and critical patch size. Mathematical Biosciences and Engineering, 2019, 16(5): 4399-4414. doi: 10.3934/mbe.2019219 |
[9] | Lihe Liang, Jinying Cui, Juanjuan Zhao, Yan Qiang, Qianqian Yang . Ultra-short-term forecasting model of power load based on fusion of power spectral density and Morlet wavelet. Mathematical Biosciences and Engineering, 2024, 21(2): 3391-3421. doi: 10.3934/mbe.2024150 |
[10] | Mo'tassem Al-Arydah, Robert Smith? . Controlling malaria with indoor residual spraying in spatially heterogenous environments. Mathematical Biosciences and Engineering, 2011, 8(4): 889-914. doi: 10.3934/mbe.2011.8.889 |
The increasing integration of large-scale wind power aggravates the difficulty of maintaining system frequency deviations in a certain range. The frequency regulation pressure of conventional generators increases, which requires wind farms to participate in system frequency regulation. In this paper, a multi-area interconnected power system frequency response model with wind power is established. Based on the frequency response model, the state space model of regional interconnected power system is presented. Then, the wind power variogram characteristics are introduced for estimating wind power variations in different time-scales. By predicting the wind power variations in AGC time-scale, a strategy of wind farm participating in AGC system is proposed and performed based on model predictive control (MPC). The control strategy makes the conventional units and wind farms to participate in AGC system coordinately. Simulation results are provided which verifies the feasibility and validity of the proposed strategy.
The objective of combating climate change has forced governments and other agencies around the world to set plans to transform the conventional power system into low-carbon power systems [1]. This process presents a unique opportunity for the rapid development of renewable energy sources (RES) such as wind power. However, it also poses enormous technical challenges for power systems, especially from a viewpoint of frequency stability [2,3,4].
One of the reasons is that wind turbine generator (WTG) is connected by electronic devices. Most WTGs inherently provide either no inertial response or frequency regulation. In addition, the massive deployment of WTG is realized by displacement of large numbers of conventional generators. The deployment of WTGs would lead to further deterioration in both system frequency control and inertial response [5]. Therefore, to ensure a secure transition to future low-carbon electricity systems with high penetration of wind power, two requirements are established for power system frequency response. (i) Conventional generators should provide more flexible frequency regulation service for a wider frequency deviation ranges. (ii) The WTGs should undertake more responsibility for power system frequency response. Various frequency regulation control strategies for WTGs have been proposed to help WTGs operating like conventional power generators as in references [6,7,8,9,10]. Compared with conventional generators, the unpredictable and intermittent nature of wind power should be considered. Therefore, the participation of WTGs in power system frequency control needs to be further studied and new techniques required to be developed.
In our previous work [11], a variogram function is proved to be a useful tool to depict the variation characteristics of wind power, after which a three-parameter power-law model is established for estimating wind power variations. In [12], we use the three-parameter power-law model to predict the wind power variations in AGC control time-scale. An AGC feedforward control strategy for conventional generators is proposed. In [12], the AGC power set value can be advancingly adjusted before the wind power variation really occurs, based on the anticipated wind power variations. Then, the AGC units can respond in advance to match the imbalance between generation and load to improve system operational performance. However, the variation rates in generation are larger now with the increasing integration of wind power generation [13]. This leads to the fact that conventional AGC units may not be able to follow these variations as tightly as desired, which also results in the increase of system frequency deviations. As a result, the coordination feedforward control of both WTGs and conventional AGC units has a significant impact on system operational performance.
MPC is a new computer control algorithm proposed in the field of industrial process control in the 1970s. It is widely used in various fields because it is convenient for modeling with good dynamic performance and stability [14]. In recent years, stochastic MPC [15], centralized MPC [16], decentralized MPC [17], and distributed MPC [18] and other improved algorithms are used in frequency control of multi-area interconnected power systems. However, the above methods are mainly focused on the controlling of conventional generators, where wind farms are actively participating. In this paper, MPC is chosen as the control algorithm to realize the feedforward control for both WTGs and conventional AGC units. Two problems are mainly focused. The first one is to predicate the variations of wind power more accurately. The second one is to use the wind power prediction information to make wind power generators provide more stable and flexible frequency response capacities. To solve these problems, variations of wind power is predicted based on variogram function and used in MPC controller to improve system frequency response performance.
This paper is organized as follows. Section 2 presents the frequency response model of regional power system with wind power. Section 3 proposes a strategy of wind farm participating in AGC based on the characteristics of wind power variations. Section 4 presents simulations and the performance of the control strategy under different conditions. Conclusions are presented in Section 5.
In this paper, the WTGs are considered participating in AGC system. Then, a multi-area frequency response model with large scale wind power is established.
Consider that the interconnected power system is composed of areas. The frequency response model of area is shown in Figure 1 [19].
In this paper, the wind farm is considered as one equivalent wind farm in the AGC model since the control strategy is presented with respect to a wind-farm level. The detailed state equation of AGC system for area is as follows.
(1) |
(2) |
(3) |
(4) |
(5) |
(6) |
In this paper, is selected as the output of area . The output equation can be obtained as follow:
(7) |
where, is the area frequency deviation coefficient. is the frequency deviation of area i. is the exchange power deviation of the tie-line. is the variation of thermal generator governor position. is power variation of re-heat turbine. and are the output power of thermal generators and wind farms respectively. and are active power control command signals respectively. is load fluctuations in the i-th area.
From the frequency response model in Fig.1, the state space model of area i can be obtained by (1)–(7).
(8) |
where, is the state variable, control variable, disturbance variable and output variable respectively. is the corresponding state matrix, control matrix, disturbance matrix and output matrix of area i. and is the state interaction matrix and control interaction matrix respectively.
From the above analysis, the state variable consists of , , , , and :
(9) |
The control variable of area , , is composed of all the power control signals that participating in AGC, namely:
(10) |
The disturbance variable is defined as the load disturbance of area , whose expression is ; The output variable is considered as the regional deviation signal ACE, whose expression is .
The state matrix of area , , can be obtained by (1)–(10):
(11) |
The control matrix is
(12) |
The disturbance matrix is
(13) |
The output matrix is
(14) |
It is noting that, only , while other elements are 0 in . And that all the elements in are 0.
With the state space model of area , the state space model of inter-connected power system can be obtained as follows:
(15) |
where, are the state variable, control variable and disturbance variable of the inter-connected power system.
(16) |
The state matrix of the inter-connected power system is as follows:
(17) |
(18) |
where, and .
In the latest Chinese national standard technical regulations (GB /t19963-2021) for wind farm integration, wind farms are supposed to participate in power system frequency regulation and peak load regulation. This section focuses on the strategy of wind farm participating in powers system AGC.
Normally, the active power control command of the wind farm is set lower than its available power (maximum wind power that can be generated by wind turbine) to ensure the active power control capability. However, the above method is built on the assumptions that the actual power (the measured wind power) of the wind farm can track the power control command. In actual operation process, the available power of wind farm cannot reach the active power control command within a certain period due to the uncertainty and variability of wind power. At such time, the actual power generated by the wind farm would instead be the available power. The actual output curve of a wind farm in one day is shown in Figure 2.
In Figure 2, it is assumed that the active power control command of the wind farm is 35MW and remains unchanged. It can be seen that the available power of the wind farm is higher than the active power control command during the time period of 00:30–04:15, 04:40–05:25, 10:30–13:45 and 17:20–23:55. At these time periods, the actual generated power of the wind farm tracks the active power control command; In other periods, the available power of the wind farm is lower than the active power control command, and the actual output power of the wind farm is instead the real available power.
The above analysis shows that the wind farm output power should be regarded as an additional system disturbance when the available power of the wind farm is lower than the active power control command. Otherwise, the wind farm can participate in the system AGC when the available power of the wind farm is greater than the control command.
As a new computer control algorithm for industrial control process, MPC has the characteristics of high robustness, good control effect, strong adaptive ability and low requirement for model accuracy. In this paper, the AGC control strategy is proposed based on MPC controller. The overall control method is shown in Figure 3.
The core idea of this strategy is:
a) The total active power command of AGC system is calculated by MPC controller according to system frequency deviation and tie-line power flow;
b) The situation of each wind farm, i.e., whether they can participate in frequency regulation, is judged by wind power prediction;
c) Online rolling optimization is performed considering the output state of thermal generators and wind farms. After which, the active power control commands of wind farm and thermal generator are calculated.
It is noting that the control time step of AGC system is about 30s-1min, while the time-scale of ultra-short-term wind power prediction is 5–15 min [20]. The wind power prediction data of 5–15min scale could not be used as the control signal of AGC system. One of the solution is to generate a wind power prediction data with a time resolution of 1 min based on 5–15 minute wind power prediction data. The common algorithm is autoregressive integrated moving average model (ARIMA). However, the increasing wind power penetration leads to the fact that conventional AGC units may not be able to follow these variations as tightly as desired. Therefore, a more accurate and shorter time-scale prediction data may lead both the conventional units and wind farms to participate AGC system better. With the above analysis, it is necessary for the wind farm to provide wind power prediction data with a time scale of less than 1 min.
Based on the variation characteristics of wind power, this paper proposed a method to estimate the variations of wind power with a time scale of 5 s–1 min based on the 15 min wind power prediction data.
The variogram of the wind power output during an interval from to with duration is defined as
(19) |
Let
(20) |
where, is hourly average wind power with 15 min sampling interval; is the maximum power of wind farm. and are per-unit values.
With the above definition, variation intensity is defined as follows:
(21) |
is an index to measure the change intensity of wind power.
By curve fitting, a three-power law model is presented as follows:
(22) |
where and are parameters of the power law model.
With (21) and (22), the variogram of wind power can be predicted when is obtained by real-time measurement.
Suppose that the is available. Then the variance of wind power can be predicted using the following equation:
(23) |
In (23), represents the absolute value of wind power variance. The plus-minus sign of is another important component, which can be obtained by
(24) |
where is the wind power moving average in the time interval . is the total number of sampling points.
From (24), the predicted variation of wind farm output power can be expressed as
(25) |
With (25), the prediction value of wind power at is obtained
(26) |
From (25), the variation of wind power at can be predicted by . The predicted wind power variation sequence can be recorded as:
(27) |
In Section 2, the state space model of (8) and (15) are continuous state space model. Using the zero-order holder discretization method, the discrete state space model of system (8) and (15) can be obtained as follows:
(28) |
(29) |
Where, , , , , , , and is the corresponding discrete variable respectively; , , , , , , , , and is the discrete matrix respectively.
Let be the prediction time domain, be the control time domain; and is set as the state vector and output vector of time predicted for time ; is set as the control vector of time predicted for time .
Based on the above analysis, the objective function of the interconnected power system is:
(30) |
where, is the objective function for time ; and is the diagonal weighting matrix for output variable and control variable respectively.
System active power balance constraint is expressed as:
(31) |
Active power output constraints of thermal generators is:
(32) |
Climbing rate constraint of thermal generators is:
(33) |
Tie-line power deviation constraint is:
(34) |
Due to the error of wind power prediction, the prediction sequence of each wind farm is corrected after each optimization to compensate the error in real time.
The compensation strategy is as follows: The predicted wind power value at the next time interval is corrected according to the measured active power value of the wind farms.
For example, is considered as the prediction wind power for time predicted at time . is the measured wind power at time . The corrected wind power can be written as:
(35) |
is the corrected wind power for time . The influence of wind power prediction error on control effect is reduced by this method.
The control strategy proposed in Section 2 is studied by a three area inter-connected power system. It is assumed that the installed capacity and generator deployment of each region is consistent. Each area has thermal generators and wind farms, and the total installed capacity of each area is 3352 MW, where thermal AGC generator capacity is 1946 MW and wind power installed capacity 1406 MW. GRCs of different type AGC units are 1.5, 2 and 4 per minute, respectively. The control time step of MPC is set as 1 min, while the prediction time domain is and the control time domain .
Load curve of each area is shown in Figure 4. Curves are drawn by actual measured data from 00:00 to 24:00. The load forecasting curve is obtained by 15-min average from actual load data. It is noting that, the load data of each area is assumed to be the same. The difference between each area is the control mode of AGC system.
The actual wind power curve and the forecasting wind power curve are shown in Figure 5. It is noting that, the forecasting wind power curve in Figure 5 is calculated based on the wind power variograms with a temporal lag of 30 s. The forecasting accuracy is much better than that of the 15 min wind power prediction.
In order to compare the performance of different AGC strategies, three control modes are proposed in this section for in-depth analysis. Different control modes are list as follows:
Control Mode I: MPC according to wind power prediction based on wind power variogram characteristics (MPC+VC);
Control Mode II: MPC according to ultra-short-term wind power prediction based on ARMIA (MPC+UST);
Control Mode III: Conventional PI control of AGC system;
The frequency deviation curves of three regions are shown in Figure 6. Figure 6(a) is the frequency deviation curve of area 1 with Control Mode I. Figure 6(b) is the frequency deviation curve of area 2 with Control Mode II and Figure 6(c) is the frequency deviation curve of area 3 with Control Mode III.
It can be seen from Figure 6 that each control mode can maintain the frequency deviation of each area at –0.1 ~ 0.1 Hz. With wind farms participate in AGC system, the frequency deviation is smaller than that of the conventional PI control of thermal generators. The control mode with prediction information based on wind power variogram characteristics has a better performance than the control mode with ultra-short-term wind power prediction information.
In order to verify that the wind power variogram characteristics based method can improve the accuracy of wind power prediction and make rational use of the reserve capacity of wind farms, comparative studies are performed. The simulation results of thermal generator output power by variogram characteristic based wind power prediction method and normal ultra-short-term wind power prediction method are selected. The results are shown in Figure 7.
In Figure 7, the output power curve of thermal generator by MPC + VC control mode is smoother than that of thermal generator by MPC + UST control mode. The active power control amplitude and control frequency of thermal power generators decreases when the wind farm participates in AGC. The participation of wind farm can well alleviate the frequency regulation pressure of thermal power generators.
This paper proposes an AGC control strategy with wind power participation based on wind power variogram characteristics. Through simulation verifications, the following conclusions are obtained:
1) Based on the wind power prediction data of AGC control time-scale, wind farm have more flexible reserves to enable the wind farm to participate in AGC system. The frequency stability of the power system with large-scale wind power is effectively improved.
2) With the three-parameter power-law model, the variation of wind power in future can be predicted and taken as the prediction model. The actual available power of wind farm is compared with the predicted wind power, and the prediction error of is corrected to reduce the impact on AGC system.
3) Simulation results show that with this new strategy, frequency deviations under wind power variations can be effectively decreased. The control strategy makes both conventional AGC generators and wind farms act in advance to race against time, and therefore reduce system frequency regulation pressure.
Authors declare no conflict of interest.
[1] |
X. Chen, M. B. Mcelroy, Q. Wu, Y. Shu, Y. Xue, Transition towards higher penetration of renewables: an overview of interlinked technical, environmental and socio-economic challenges, J. Modern Power Syst. Clean Energy, 7 (2019), 1-8. https://doi.org/10.1007/s40565-018-0438-9 doi: 10.1007/s40565-018-0438-9
![]() |
[2] |
C. Rahmann, S. I. Chamas, R. Alvarez, H. Chavez, D. Ortiz-Villalba, Y. Shklyarskiy, Methodological approach for defining frequency related grid requirements in low-carbon power systems, IEEE Access, 8 (2020), 161929-161942. https://doi.org/10.1109/ACCESS.2020.3021307 doi: 10.1109/ACCESS.2020.3021307
![]() |
[3] |
Y. Fang, S. Zhao, E. Du, S. Li, Z. Li, Coordinated operation of concentrating solar power plant and wind farm for frequency regulation, J. Modern Power Syst. Clean Energy, 9 (2021), 751-759. https://doi.org/10.35833/MPCE.2021.000060 doi: 10.35833/MPCE.2021.000060
![]() |
[4] |
Z. Zheng, J. Li, H. Sang. A hybrid invasive weed optimization algorithm for the economic load dispatch problem in power systems, Math. Biosci. Eng., 16 (2019), 2775-2794. https://doi.org/10.3934/mbe.2019138 doi: 10.3934/mbe.2019138
![]() |
[5] |
N. Nguyen, J. Mitra, An analysis of the effects and dependency of wind power penetration on system frequency regulation, IEEE Trans. Sustain. Energ., 7 (2016), 354-363. https://doi.org/10.1109/TSTE.2015.2496970 doi: 10.1109/TSTE.2015.2496970
![]() |
[6] |
H. Ye, W. Pei, Z. Qi, Analytical modeling of inertial and droop responses from a wind farm for short-term frequency regulation in power systems, IEEE Trans. Power Syst., 31 (2016), 3414-3423. https://doi.org/10.1109/TPWRS.2015.2490342 doi: 10.1109/TPWRS.2015.2490342
![]() |
[7] |
Y. Wu, W. Yang, Y. Hu, P. Q. Dzung, Frequency regulation at a wind farm using time-varying inertia and droop controls, IEEE Trans. Ind. Appl., 55 (2019), 213-224. https://doi.org/10.1109/TIA.2018.2868644 doi: 10.1109/TIA.2018.2868644
![]() |
[8] |
H. Luo, Z. Hu, H. Zhang, H. Chen, Coordinated active power control strategy for deloaded wind turbines to improve regulation performance in AGC, IEEE Trans. Power Syst., 34 (2019), 98-108. https://doi.org/10.1109/TPWRS.2018.2867232 doi: 10.1109/TPWRS.2018.2867232
![]() |
[9] |
Z. Wang, W. Wu, Coordinated control method for DFIG-based wind farm to provide primary frequency regulation service, IEEE Trans. Power Syst., 33 (2018), 2644-2659. https://doi.org/10.1109/TPWRS.2017.2755685 doi: 10.1109/TPWRS.2017.2755685
![]() |
[10] |
M. A. Kamarposhti, I. Colak, K. Eguchi, Optimal energy management of distributed generation in micro-grids using artificial bee colony algorithm, Math. Biosci. Eng., 18 (2021), 7402-7418. https://doi.org/10.3934/mbe.2021366 doi: 10.3934/mbe.2021366
![]() |
[11] |
J. Liu, G. Ren, J. Wan, Y. Guo, D. Yu, Variogram time-series analysis of wind speed, Renewable Energy, 99 (2016), 483-491. https://doi.org/10.1016/j.renene.2016.07.013 doi: 10.1016/j.renene.2016.07.013
![]() |
[12] |
Y. Guo, Q. Wang, D. Zhang, J. Wan, D. Yu, J. Yu, Anticipatory AGC control strategy based on wind power variogram characteristic, IET Renewable Power Gen., 14 (2020), 1124-1133. https://doi.org/10.1049/iet-rpg.2019.0723 doi: 10.1049/iet-rpg.2019.0723
![]() |
[13] |
J. Kiviluoma, H. Holttinen, D. Weir, R. Scharff, L. Soder, N. Menemenlis, et al., Variability in large-scale wind power generation, Wind Energy, 19 (2016), 1649-1665. https://doi.org/10.1002/we.1942 doi: 10.1002/we.1942
![]() |
[14] |
C. Wang, J. Tang, B. Jiang, Z. Wu. Sliding-mode variable structure control for complex automatic systems: a survey, Math. Biosci. Eng., 19 (2022), 2616-2640. https://doi.org/10.3934/mbe.2022120 doi: 10.3934/mbe.2022120
![]() |
[15] |
H. Zhao, Q. Wu, Q. Guo, H. Sun, Y. Xue, Distributed model predictive control of a wind farm for optimal active power controlpart ii: implementation with clustering-based piece-wise affine wind turbine model, IEEE Trans. Sustain. Energ., 6 (2015), 840-849. https://doi.org/10.1109/TSTE.2015.2418281 doi: 10.1109/TSTE.2015.2418281
![]() |
[16] |
H. Jiang, J. Lin, Y. Song, D. J. Hill, MPC-based frequency control with demand-side participation: a case study in an isolated wind-aluminum power system, IEEE Trans. Power Syst., 30 (2015), 3327-3337. https://doi.org/10.1109/TPWRS.2014.2375918 doi: 10.1109/TPWRS.2014.2375918
![]() |
[17] |
X. Kong, X. Liu, L. Ma and K. Y. Lee, Hierarchical distributed model predictive control of standalone wind/solar/battery power system, IEEE Trans. Syst. Man Cybernetics Syst., 49 (2019), 1570-1581. https://doi.org/10.1109/TSMC.2019.2897646 doi: 10.1109/TSMC.2019.2897646
![]() |
[18] |
J. C. Sánchez, O. Marjanovic, M. Barnes, P. R. Green, Secondary model predictive control architecture for VSC-HVDC networks interfacing wind power, IEEE Trans. Power Del., 35 (2020), 2329-2341. https://doi.org/10.1109/TPWRD.2020.2966325 doi: 10.1109/TPWRD.2020.2966325
![]() |
[19] |
S. Desai, N. R. Sabar, R. Alhadad, A. Mahmood, Naveen Chilamkurti, Mitigating consumer privacy breach in smart grid using obfuscation-based generative adversarial network, Math. Biosci. Eng., 19 (2022), 3350-3368. https://doi.org/10.3934/mbe.2022155 doi: 10.3934/mbe.2022155
![]() |
[20] |
F. M. Butt, L. Hussain, A. Mahmood, K. Lone, Artificial intelligence based accurately load forecasting system to forecast short and medium-term load demands, Math. Biosci. Eng., 18 (2021), 400-425. https://doi.org/10.3934/mbe.2021022 doi: 10.3934/mbe.2021022
![]() |