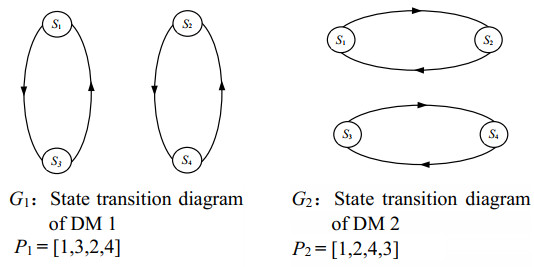
A mathematical model for decision maker's preference prediction in environmental governance conflict is established based on the graph model for conflict resolution. The rapid economic development in many countries, over the past decades, has caused serious environmental pollution. Sewage companies are the main source of contamination since they are always wavering on the issue of environmental governance because of their profit-seeking nature. Environmental management departments cannot grasp the offending company preferences accurately. The problem of how to obtain decision maker's preference in environmental governance conflict is studied in this paper. The mathematical model established in this paper can obtain a preference set of one decision maker on the promise that the ideal conflict outcome and preference of the other decision makers are known. Then, preference value distribution information entropy is introduced to mine the preference information contained in the preference set, which means that it is possible to get the preference information of conflict opponent at their own ideal conflict outcome. All of these preference sets provide the possibility to choose the appropriate coping strategies and lead the conflict to the direction that some decision makers want. Finally, the effectiveness and superiority of the preference prediction analysis method is verified through a case study of "Chromium Pollution in Qujing County" which took place in China. The preference prediction analysis method in this paper can provide decision making support for the decision makers in environmental governance from strategic level.
Citation: Jinshuai Zhao, Baohua Yang. Preference prediction analysis based on graph model for environmental governance conflict[J]. Mathematical Biosciences and Engineering, 2022, 19(6): 6141-6156. doi: 10.3934/mbe.2022287
[1] | Saswati Biswas, Pankaj Kumar Tiwari, Yun Kang, Samares Pal . Effects of zooplankton selectivity on phytoplankton in an ecosystem affected by free-viruses and environmental toxins. Mathematical Biosciences and Engineering, 2020, 17(2): 1272-1317. doi: 10.3934/mbe.2020065 |
[2] | Jianquan Guo, Guanlan Wang, Mitsuo Gen . Green closed-loop supply chain optimization strategy considering CER and incentive-compatibility theory under uncertainty. Mathematical Biosciences and Engineering, 2022, 19(9): 9520-9549. doi: 10.3934/mbe.2022443 |
[3] | Zheng Liu, Lingling Lang, Lingling Li, Yuanjun Zhao, Lihua Shi . Evolutionary game analysis on the recycling strategy of household medical device enterprises under government dynamic rewards and punishments. Mathematical Biosciences and Engineering, 2021, 18(5): 6434-6451. doi: 10.3934/mbe.2021320 |
[4] | Eunjae Choi, Yoosang Park, Jongsun Choi, Jaeyoung Choi, Libor Mesicek . Forecasting of garlic price based on DA-RNN using attention weight of temporal fusion transformers. Mathematical Biosciences and Engineering, 2023, 20(5): 9041-9061. doi: 10.3934/mbe.2023397 |
[5] | Yangyan Zeng, Yidong Zhou, Wenzhi Cao, Dongbin Hu, Yueping Luo, Haiting Pan . Big data analysis of water quality monitoring results from the Xiang River and an impact analysis of pollution management policies. Mathematical Biosciences and Engineering, 2023, 20(5): 9443-9469. doi: 10.3934/mbe.2023415 |
[6] | Shan Huang, Khor Teik Huat, Yue Liu . Study on the influence of Chinese traditional culture on corporate environmental responsibility. Mathematical Biosciences and Engineering, 2023, 20(8): 14281-14305. doi: 10.3934/mbe.2023639 |
[7] | Irina Kareva, Faina Berezovkaya, Georgy Karev . Mixed strategies and natural selection in resource allocation. Mathematical Biosciences and Engineering, 2013, 10(5&6): 1561-1586. doi: 10.3934/mbe.2013.10.1561 |
[8] | Irfanullah Khan, Asif Iqbal Malik, Biswajit Sarkar . A distribution-free newsvendor model considering environmental impact and shortages with price-dependent stochastic demand. Mathematical Biosciences and Engineering, 2023, 20(2): 2459-2481. doi: 10.3934/mbe.2023115 |
[9] | Rui Huang, Hao Xiao, Guoqin Pan . Authority decentralization and air pollution reduction: Evidence from China. Mathematical Biosciences and Engineering, 2022, 19(12): 14212-14231. doi: 10.3934/mbe.2022661 |
[10] | Hexiao Hu, Yalian Zhang, Chen Yao, Xin Guo, Zhijing Yang . Research on cost accounting of enterprise carbon emission (in China). Mathematical Biosciences and Engineering, 2022, 19(11): 11675-11692. doi: 10.3934/mbe.2022543 |
A mathematical model for decision maker's preference prediction in environmental governance conflict is established based on the graph model for conflict resolution. The rapid economic development in many countries, over the past decades, has caused serious environmental pollution. Sewage companies are the main source of contamination since they are always wavering on the issue of environmental governance because of their profit-seeking nature. Environmental management departments cannot grasp the offending company preferences accurately. The problem of how to obtain decision maker's preference in environmental governance conflict is studied in this paper. The mathematical model established in this paper can obtain a preference set of one decision maker on the promise that the ideal conflict outcome and preference of the other decision makers are known. Then, preference value distribution information entropy is introduced to mine the preference information contained in the preference set, which means that it is possible to get the preference information of conflict opponent at their own ideal conflict outcome. All of these preference sets provide the possibility to choose the appropriate coping strategies and lead the conflict to the direction that some decision makers want. Finally, the effectiveness and superiority of the preference prediction analysis method is verified through a case study of "Chromium Pollution in Qujing County" which took place in China. The preference prediction analysis method in this paper can provide decision making support for the decision makers in environmental governance from strategic level.
The industrialization process has achieved rapid development in the past 30 years around the world. At the same time, the problem of environmental pollution is extremely serious. The total discharge of pollutants far exceeds the environmental capacity, and major environmental pollution accidents occur frequently in many countries [1–4]. Identifying and quantifying planetary boundaries that must not be transgressed could help prevent human activities causing unacceptable environmental change [5]. Nebasifu et al. [6] investigated a systematic review of articles from academic and policy publications over the last few decades, and they found that there are paradoxical gaps in resilient environmental governance. Xu et al. [7] studied the structural conflict under the new green dilemma. They concluded that the inequality of global production system suppresses the effects of subsidy competition and erodes the economic foundation of global climate governance. Obviously, environmental governance is a collective matter involving multiple stakeholders, and it is inevitable that many complex contradictions and conflicts will be generated. How to effectively resolve these conflicts requires decision makers (DMs) to make better decisions with the help of decision support systems [8]. Most of the existing environmental governance decision support systems are based on classical game theory. However, classical game theory puts too much emphasis on inherent game rules and has strong assumptions on sharing knowledge. Raiffa et al. [9] pointed out that the assumptions in classical game theory are too strict, so it cannot be widely used. Such strict restrictions make it difficult to apply this fine theory to practice.
Conflict analysis is a method for decision making analysis of conflict behaviors which are difficult to quantitatively describe. It was developed in recent years on the basis of classical game theory and meta-game theory. It avoids too many assumptions as criticized in the classical game theory, and has achieved great success in the past 20 years. However, the existing conflict theories are mainly based on that the preferences of conflict DMs are known. While, in the process of conflicts in environmental governance, it is very difficult to fully grasp the opponent's real preference of the possible outcomes. Because of the autonomy of DMs, the inconsistency of interest claims, and the dynamics in the process of environmental governance [10], it is impossible for any DM in the environmental governance conflict to actively convey the true preferences of the other DMs. That is, the key preferences among DMs in the pollution control conflict may be kept secret to each other [11]. Therefore, in general, DMs in conflict mainly rely on their own subjective cognition to get their opponents' preferences. DM's subjective cognition often has certain limitations. That will cause decision mistake and pay a huge price due to the incomplete grasp of the opponent's preferences. Therefore, how to design a method which can analyze the preference information of opponents roundly and profoundly to make up for the limitations of subjective cognition is of great significance for the DMs in the environmental governance conflict, especially for the government to choose appropriate coping strategies [12] and to guide the conflict to develop towards an ideal conflict outcome in the process of environmental governance conflict [13].
The research on the incompleteness of DMs' preference perception in conflict has always received extensive attention from academic areas. For example, Hipel et al. [14] first proposed the idea of information gap for water resources management, which was used to describe the information asymmetry between the two sides of the conflict. Then, they established an information gap model and applied the research results to the water resource conflict in the Middle East, and gave strategic decision suggestions. On the basis of the information gap model of preference perception uncertainty, Song et al. [15] established the equilibrium outcome based on hyper-game theory, and analyzed the robustness of the results. Subsequently, to handle the ranking method of multiple feasible outcomes of preference uncertainty, research has been completed with respect to degree of freedom [16], probability [17] and attitude [18], as well as the competition and cooperation behavior of DMs in conflict [19]. In addition, literatures [20−23] focused on the balanced outcome of strategic conflict under cognitive difference information, conflict resolution methods for emergent conflict events, sensitivity analysis of DMs in conflict, and conflict resolution method based on negotiation, respectively.
The above research results analyze the cognitive dissonance that may exist between DMs of conflict, and make decisions or coping strategies for this error hypothesis. So far, the research in this field has demonstrated a lack of strong theoretical basis and systematic cognitive model to support the choice of coping strategies. Sakakibara et al. [24] first proposed the inverse problem based on the Graph Model for Conflict Resolution (GMCR) [25], and tried to verify the assumption by using the trail and error method. However, it has been proved that this method has heavy logic workload and cannot be done by computer, which makes it difficult to apply. Based on GMCR and with the help of high-performance computer, Kinsara et al. [26] proposed a method to obtain the preference information of DMs by a brute force method, which can be used for negotiation and third-party mediation. However, the disadvantage of this method is that the amount of computation increases exponentially with the increase of algorithm size, and eventually the algorithm goes into an infinite loop. That is because the number exceeds the scope of computer representation. Treml et al. [27] developed an innovative approach using graph theory for socio-ecological conflict. The method has the potential to assist management efforts in prioritizing and strengthening governance to effectively safeguard ecological processes across multiple jurisdictions. Kinimonth et al. [28] presented a conceptual model based on graph theory. The conceptual model provides a novel approach to the structural analysis of socio-ecological systems.
A new mathematical theoretical model for obtaining information about DMs' preferences in environmental governance conflicts is proposed in this paper based on GMCR. The model assumes that one DM in environmental governance is very clear about their own preference order and ideal conflict outcome. Starting from all feasible preference orders of the opponent in conflict, the existence of the opponent's minimum preference value is verified, which means that there are stable preference orders in the ideal conflict outcome for the opponent. Then, the corresponding preference order set is found. Finally, information entropy is introduced to mine the preference information of the opponent in the preference order set, so as to give coping strategies in the process of conflict evolution. The specific innovations mainly include: 1) The relative real preference order set of the opponent is obtained by determining the minimum preference value which satisfies stability. 2) The definition of preference value distribution information entropy is given. The information quantity in preference order set can be mined by this definition. DMs can be given appropriate conflict coping strategies according to their own interests.
The remainder of this paper is organized as follows. Section 2 introduces the basic concept of GMCR. Section 3 develops a cognitive model of conflict opponent's preference in environmental governance. In the subsequent section, a case study of a conflict in China is utilized to demonstrate the advantages of the cognitive model. The final section contains overall comments and insights to put the research into perspective.
GMCR is a method for solving conflict which is proposed by the Canadian conflict analysis research team [25]. It is developed on the basis of game theory. Its characteristic is based on relative preference, which is simpler and more flexible than the quantitative analysis of game theory. Meanwhile, through analyzing DMs, feasible states, options, state transition diagram and preferences, the conflict equilibrium solution is obtained under different stability constraints. In GMCR, the graph model is represented by a directed graph, which uses a complete directed graph to represent the feasible states and state transition of each DM. State transition means that when other DMs keep the strategy selected, the remaining DM can consider changing the strategy to change the outcome of the conflict. Since the research in this article is based on GMCR, the basic concept of GMCR is given first.
Definition 1 (GMCR): GMCR is represented by a four groups structure V={N,S,P,G}, where
1) N={1,2,⋯,n} represents the set of DMs, and n is the total number of DMs.
2) S={s1,s2,⋯,sm} denotes the set of feasible states, and m is the number of feasible states.
3) P={P1,P2,⋯,Pn} is called the preference set of the DMs, where Pi represents the preference information of DM i.
4) G={G1,G2,⋯,Gn} is a non-empty, finite set, called state transition directed graph. Gi=<S,Ai>, for each DM i, Ai represents DM i's set of oriented arcs, which contains the transition between feasible states controlled by DM i and the arrow of the arc points to the reachable state that can be transferred from the original state.
Definition 2 (Preference order): After DM i∈N rank all feasible states from inferior to superior by the degree of preference, the order position of each state is represented by the vector Pi=[k1,k2,⋯,km]. Pi is called the preference order of DM i, where kl is the preference value of DM i of state sl, denoted as Pi(sl)=kl, kl is an integer between [1,l], and l≤m. When the elements in preference set Pi are different from each other, the preference of DM i is called strictly ordered preference.
Definition 3 (Reachable list):Ri(s) is DM i's one-step reachable set from state s, called reachable list. For DM i∈N,
Ri(s)={sq∈S|(s,sq)∈Ai} | (1) |
Where (s,sq)∈Ai represents that there is a directed arc from state s to state sq.
Definition 4 (Improvement reachable list):R+i(s) is the DM i's one-step improved reachable set from state s, called the improved reachable list. For DM i∈N,
R+i(s)={sq∈S|(s,sq)∈Ai&Pi(s)<Pi(sq)} | (2) |
For example, the graph model of the classic "Chicken Game" is shown in Figure 1, where N={1,2}, S={s1,s2,s3,s4}, P={P1,P2}, G={G1,G2}, R1(s3)={s1}, R+1(s3)=∅.
Definitions 1–4 are the four basic definitions of GMCR. For detailed explanations of these definitions, please refer to [25]. Then, according to the number of state transition steps taken by the DMs and their attitudes towards risk, GMCR gives the definition of sequential (SEQ) stability that conforms to the actual conflict situation.
Definition 5 (SEQ): For DM i∈N and state s∈S, state s is SEQ iff for every s1∈R+i(s) there exists at least one s2∈R+N−i(s1) with Pi(s)>Pi(s2), which is denoted as s∈SSEQi. When all DMs in set N satisfy SEQ stability, state s is called SEQ equilibrium.
SEQ stability believes that the opponent will counterattack all their unilateral improvements, and the counterattack will reduce their own preference value and increase the opponent's preference value. It describes the DMs' prediction of the two-step stability of the conflict, which is in line with the characteristics that each DMs' strategy in each step is aimed at promoting personal interests. It can perfectly describe the strategic conflict in the real world. When n=2, the set N in the Definition 5 reduces to N={i,j}, i and j are opponents in conflict, and R+N−i reduces to R+j. In this article, we just investigate the two DMs conflict.
In a conflict, usually all DMs involved in it have a certain estimate of the final outcome about the conflict. That is, the final outcome of the conflict is "known". For example, in environmental governance, everyone involved will understand that pollution will be treated as a result. However, the polluting enterprises are prone to fluke and do not actively deal with their own pollutants due to the environment itself has a certain ability to solve pollution. This makes themselves comply with the environmental regulations of the government and the supervision of environmental non-governmental organizations in the most self-interest way. It will inevitably lead to that the final realization of environmental governance is a long process. In environmental governance of China, the participants have different understandings on how to achieve the conflict outcome of "pollution control" and how much they hope to spend to achieve this outcome. If the participants' perception of the outcome in environmental governance conflicts is regarded as the preferences of the participants, then in the resolution of environmental governance conflicts, a very important question is what are the true preferences of the conflict opponent? Only by obtaining the preferences of the conflict opponents, we can master the initiative in the conflict game and make the outcome develop in the direction of the predetermined situation. In order to solve this problem, this section uses the definition of SEQ stability on the premise that the final outcome is known, and infers all preference orders that satisfy a stable outcome inversely. At the same time, the opponent's true intentions are extracted from these preference order data sets.
Definition 6 (Ideal conflict outcome of DM i): For DM i∈N, state s∈S, the state s∗i with the largest preference value is defined as:
Pi(s∗i)=maxs∈S{Pi(s)} | (3) |
Then state s∗i is the ideal conflict outcome of DM i. The rest of the feasible conflict outcomes are non-ideal.
Definition 7 (Preference order set): The set of all preference orders of DM i satisfying SEQ stability in a certain state s is called preference order set, which is represented by Qi(s).
For any conflict, each DM is very clear about his own preference order and ideal conflict outcome. Obviously, ideal conflict outcome satisfies the DM's SEQ stability. While the information of his opponent is unknown. The root of the conflict is that the DMs have different views towards the final outcome of the conflict, and each DM wants the opponent to be stable in his own ideal conflict outcome. Therefore, the research on the preference order of its opponent which satisfying stable in the ideal conflict outcome can better reflect the opponent's bottom line, which is conducive to the final resolution of the conflict.
According to the definition of SEQ stability, the following two conclusions are obviously established:
i) when Pj(s∗i)=1, DM j does not have a preference order satisfies SEQ stable in state s∗i, that is Qj(s∗i)=∅.
ii) when Pj(s∗i)=m, all the preferences order of DM j satisfy SEQ stable in state s∗i.
The above two conclusions give two extreme situations in conflict. Conclusion i) reflects that since DMs i and j in the game have serious disagreements on the conflict outcome s∗i, it is impossible to have equilibrium in s∗i at any stage of the conflict. Conclusion ii) describes that the ideal conflict outcome between DMs i and j is the same, and the conflict no longer exists.
Given that DMs i and j are conflict opponents, their ideal conflict outcome must be inconsistent. If DM i wants DM j to be stable in DM i's ideal conflict outcome, the preference order value of DM j in his ideal conflict outcome must be increased.Assuming Pj(s∗i)=k, then to what extent does the value of k increase, DM j in the conflict has a preference order that satisfies SEQ stability in s∗i. That is to say, getting the minimum value of k for DM j that satisfies the stability of SEQ in s∗i is very important.
Lemma 1 (K-value existence theorem): It is known that the preference order Pi of DM i in the conflict and its ideal conflict outcome s∗i. Let Pj(s∗i)=k, then there must be a k value of DM j in state s∗i that satisfies the stability of SEQ.
Proof: Because k is the preference value of DM j in state s∗i, its value is an integer between [1, m]. Considering the extreme case, if k=m, then any counteraction from DM i will reduce DM j's preference value. So, DM j refuses to move away from state s∗i. According to Definition 5, DM j satisfies SEQ stability in state s∗i.
Lemma 1 proves the existence of k value. Theorem 1 gives a method to obtain the minimum kof SEQ stability by using 0-1 programming.
In order to increase the readability of the article, the main symbols and descriptions used in the following are shown below.
X=[x1,x2,⋯,xm] is an m-dimensional 0-1 row vector. When Pj(s∗i)>Pj(st), then xt=1, otherwise xt=0.
Ji is a 0-1 reachable matrix with the size of m×m of DM i. When DM i is reachable between states sp and sq, Ji(sp,sq)=1, otherwise Ji(sp,sq)=0. J+i is a 0-1 improved reachability matrix with the size of m×m of DM i. When Ji(sp,sq)=1 and Pi(sq)>Pi(sp), J+i(sp,sq)=1, otherwise J+i(sp,sq)=0. Jj and J+j are defined as Ji and J+i for DM j, respectively.
J+i(st,:) represents the st row of matrix J+i.sum(J+i(st,:)∘X) represents the sum of product J+i(st,:) and X corresponding elements multiplied.
Theorem 1 (Minimum value k): Let k0 be the minimum k value of DM j satisfying SEQ stability in state s∗i, then k0can be obtained by the following 0-1 programming model:
k0=min(m∑t=1xt)+1 |
{1−Jj(s∗i,st)⋅(1−xt)+sign(sum(J+i(st,:)∘X))>01−Jj(s∗i,st)⋅(1−xt)+sign(sum(J+i(st,:)∘X))≤2xt=0or1,t=1,2,⋯,mX=[x1,x2,⋯,xm] | (4) |
Proof: From expression i) 1−Jj(s∗i,st)⋅(1−xt)=0, it can be deduced that Jj(s∗i,st)=1 and xt=0 hold simultaneously.Jj(s∗i,st)=1 denotes that DM j can reach from state s∗i to the state st.xt=0 means that Pj(s∗i)<Pj(st). That is, DM j is improvement and reachable from state s∗i to state st. From expression ii) sign(sum(J+i(st,:)∘X))=0, it can be concluded that J+i(st,:)∘X=→0. That is, DM i starts from state st, and there is no improvement and reachable state sp which satisfying Pj(s∗i)>Pj(sp). According to Definition 5, if the expressions i) and ii) are both 0 at the same time, DM j is unstable in the state s∗i.Otherwise, it is stable. Because the values of expressions i) and ii) are only 0 and 1, if the sum of expressions i) and ii) is greater than 0 and less than or equal to 2, then DM j satisfies SEQ stability in state s∗i.
The element in the vector X equal to 1 indicates that the preference order value is less than Pj(s∗i). That is to say, k0=min(∑mt=1xt)+1 is the minimum k value under the constraint condition.
Given the preference order Pi of DM i, ideal conflict outcome s∗i and Pj(s∗i)=k0, the inverse problem model [29] can be used to find the preference order set Qj(s∗i) of DM j in the SEQ stable state s∗i. While, there is still a large number of preference orders in this set. It is impossible to see the amount of information implicit in it intuitively.
Shannon et al. proposed a concept of information entropy in 1948 [30]. It describes the amount of information, the greater the entropy, the higher degree of dispersion of variables. The distribution of preference value of each state in preference order set Qj(s∗i) is a discrete random variable. In view of the problem, there are so many elements in the set that the hidden information can not be displayed directly, the preference value distribution information entropy is introduced to describe the sensitivity of the ideal conflict outcome s∗i to the change of the preference value of each state.
Definition 8 (Preference value distribution information entropy): Given the preference order Pi of DM i and its ideal conflict outcome s∗i. k0 is the minimum value of DM j satisfying SEQ stability, and the corresponding preference order set is Qj(s∗i). The preference value of state st in Qj(s∗i) is 1, 2, ..., m, and the number is B1, B2,…,Bm which represents the number of values from 1 to m in Qj(s∗i), respectively. Its corresponding probability distribution is B1∑mh=1Bh, B2∑mh=1Bh,…,Bm∑mh=1Bh. Then the preference value distribution information entropy of state st is defined as follows.
Pv(st)=Bv∑mh=1Bh,v=1,2,⋯,m |
∑mv=1Pv(st)=1,0≤Pv(st)≤1 | (5) |
H(st)=∑mv=1Pv(st)⋅log21Pv(st),t=1,2,⋯,m | (6) |
The preference value distribution information entropy H(st) describes the influence of the change about the preference value on the state st to the stability of the conflict when the ideal conflict outcome s∗i is stable.
Property 1: The value range of preference value distribution information entropy H(st) is [0,log2m].
Proof: the process of detailed proof is obvious according to Definition 8.
The preference value distribution information entropy is derived from the concept of information entropy, so it has the typical characteristics of information entropy, which leads to Theorem 2.
Theorem 2: The larger H(st) of state st∈S is, the more even probability distribution of the preference value of this state in set Qj(s∗i) which taking 1, 2, ..., m is, and the smaller the change of preference value of DM j in state st influences the stability of state s∗i. Conversely, the more uneven the distribution of the preference value of the state st in the set Qj(s∗i), the greater influence of its change to the stability of DM j in the state s∗i.
Theorem 2 describes that the greater the preference value distribution information entropy in a certain state, the more even the preference value distribution of this state. Then, the change of preference value of this state has less influence on DM j maintaining SEQ stability in state s∗i; Otherwise, if the preference value distribution information entropy of a certain state is smaller in all states, it means that the value of this state is more sensitive to the stability of state s∗i to keep SEQ stability. By calculating the preference value distribution information entropy of all states, we can clearly get the sensitive state of DM j that DM i needs to pay attention to if he wants to maintain his ideal conflict outcome s∗i stable. That is to say, DM i can obtain the real preference information of DM j temporarily maintaining stable in his own ideal conflict outcome s∗i, and then make appropriate coping strategies.
In summary, the process of obtaining preference perception of conflict opponents is shown in Figure 2.
In recent years, China's economic growth has made rapid progress. While, at the same time, the problem of environmental pollution has become increasingly serious. A series of conflicts have broken out frequently. In June 2011, an incident of illegal transfer and dumping of chromium residues which caused environmental pollution occurred in Qujing County, Yunnan Province [31]. In August, the incident was disclosed by the media and aroused widespread national concern. On August 16, the environmental protection department of Yunnan Province asked Luliang Chemical Industry Co., Ltd. (hereinafter referred to as "Luliang chemical") to stop production for rectification. During this period, it must complete the harmless treatment of all chromium slag stored in the old chromium slag storehouse and in the factory. Besides, the production shall not be resumed without acceptance. If Luliang chemical wants to resume production, it needs to apply to the local environmental protection department for the resumption of production of chromium slag production line. After being approved by the provincial environmental protection department, the chromium slag production line shall be inspected according to the production conditions and relevant requirements. To pass the acceptance check, Luliang chemical must complete two tasks. The first is to complete the disposal of new chromium residue and contaminated soil. The second is to build and put the detoxification production line into operation to ensure "zero" pollution discharge or discharge below a certain limit. However, Luliang chemical believes that these requirements have seriously affected the economic interests of the company. They hope that the local government can modify the environmental protection plan according to local conditions. But the local government insisted not to modify the environmental protection plan because of the fear of the national document on chromium slag pollution control. Luliang chemical adopted a strategy of delay. The two sides are in a stalemate.
In response to the conflict, two DMs are extracted: the local government (LG) and Luliang chemical (LC). The options adopted by the two DMs are as follows.
The Local Government (LG):
1) Modify: Modify the original environmental protection plan so that Luliang chemical can accept the remediation plan.
Luliang Chemical (LC):
2) Delay: The strategy of delay has been adopted throughout the process. Neither effective rectification nor effective treatment measures are taken for local pollution.
3) Accept: Accept the environmental protection plan. Carry out equipment rectification and deal with local pollution actively and effectively.
This conflict event has a total of 8 states, as shown in Table 1, which are represented by s1, s2, ⋯, and s8, respectively. In Table 1, "Y" indicates that the DM chooses the strategy, and "N" indicates that the DM abandons the strategy. The graph model of this conflict is shown in Figure 3. In Figure 3, the dots indicate 8 feasible states, and the arrow direction of the arcs indicates the transfer from the initial state to its reachable state.
DM | options | feasible states | |||||||
s1 | s2 | s3 | s4 | s5 | s6 | s7 | s8 | ||
LG | Modify | N | N | N | N | Y | Y | Y | Y |
LC | Delay | N | Y | N | Y | N | Y | N | Y |
Accept | N | N | Y | Y | N | N | Y | Y |
In this conflict, Luliang chemical's preference perception is analyzed from the perspective of the local government, and vice versa. As a management agency, the local government is obviously very clear about its own preferences. It most hopes that Luliang chemical will accept the remediation plan, which means that s3, s7, s4, s8 are better than s2, s6, s1, s5. Under the premise that Luliang chemical accepts the remediation plan, it tends not to delay, that is, s3, s7 are better than s4, s8. However, under the premise that Luliang chemical does not accept it. It tends to delay for a while, that is, s2, s6 are better than s1, s5. Finally, under Luliang chemical's choice of an equivalent plan, the local government hopes not to modify the environmental protection plan. That is, s3 is better than s7, s4isbetterthans8, s2isbetterthans6, and s1isbetterthans5. In summary, the preference order of the local government is P1=[2,4,8,6,1,3,7,5].
Local government must consider local interests while making decisions. Its ideal conflict outcome is s3. However, as a company, its opponent Luliang chemical is pursuing short-term profits. Its preference will change with the attitude of the local government. According to Theorem 1, we can find k0=4. Let P2=[x1,x2,x3,…,x8], it can be seen from Figure 3 that the reachable states of LuLiang Chemical from state s3 are s1, s2, and s4. Under the premise of x3=4, the following 8 sets of inequalities are obtained according to the inverse problem model [29].
{x1<4x2<4x4<4or{x1<4x2<4x4>4min((R+1P2)(s4)<4or{x1<4x2>4x4<4min((R+1P2)(s2)<4 |
or{x1<4x2>4x4>4min((R+1P2)(s2)<4min((R+1P2)(s4)<4or{x1>4x2<4x4<4min((R+1P2)(s1)<4or{x1>4x2<4x4>4min((R+1P2)(s1)<4min((R+1P2)(s4)<4, |
{x1>4x2>4x4<4min((R+1P2)(s1)<4min((R+1P2)(s2)<4or{x1>4x2>4x4>4min((R+11P2)(s1)<4min((R+1P2)(s2)<4min((R+1P2)(s4)<4. |
The results are as follows.
{x1<4x2<4x4<4or{x1<4x2<4x4>4x8<4or{x1<4x2>4x4<4x6<4or{x1<4x2>4x4>4x6<4x8<4 |
or{x1>4x2<4x4<4x5<4or{x1>4x2<4x4>4x6<4x8<4or{x1>4x2>4x4<4x5<4x6<4or{x1>4x2>4x4>4x5<4x6<4x8<4. |
The number of elements in the preference order set Q2(s3) that respectively satisfy these 8 sets of conditions is 8×P33×P44. These preference orders can be listed one by one through permutation and combination.
According to the Eq (5) in Definition 8, we can obtain DM j's probability distribution of preference value of each state in the Q2(s3). Q2(s3) is a preference order set which satisfies the SEQ stable in the state s3 for DM j. As shown in Table 2.
Feasible states | Probability distribution of preference value | |||||||
1 | 2 | 3 | 4 | 5 | 6 | 7 | 8 | |
s1 | 1/6 | 1/6 | 1/6 | 0 | 1/8 | 1/8 | 1/8 | 1/8 |
s2 | 1/6 | 1/6 | 1/6 | 0 | 1/8 | 1/8 | 1/8 | 1/8 |
s3 | 0 | 0 | 0 | 1 | 0 | 0 | 0 | 0 |
s4 | 1/6 | 1/6 | 1/6 | 0 | 1/8 | 1/8 | 1/8 | 1/8 |
s5 | 1/8 | 1/8 | 1/8 | 0 | 5/32 | 5/32 | 5/32 | 5/32 |
s6 | 5/24 | 5/24 | 5/24 | 0 | 3/32 | 3/32 | 3/32 | 3/32 |
s7 | 0 | 0 | 0 | 0 | 1/4 | 1/4 | 1/4 | 1/4 |
s8 | 1/6 | 1/6 | 1/6 | 0 | 1/8 | 1/8 | 1/8 | 1/8 |
The row of each state in Table 2 represents the probability that Luliang chemical satisfies the preference value distribution probability of SEQ stable in this state in ideal conflict outcome s3. The column of each value indicates the probability of the value distribution of the preference value in each state when the Lulinag chemical satisfies the stability of the ideal conflict outcome s3. For example, the value 1/4 corresponding to row s7 and column 5 in Table 2 indicates that the probability that Luliang chemical satisfies SEQ stable in state s7 and takes preference value of 5 in order to maintain the ideal conflict outcome s3 is 1/4. Since the data in Table 2 represents a probability distribution, the sum of the data in each row and column in this Table is 1.
Using the data in Table 2, the preference value distribution information entropy of that satisfies the stability of SEQ can be obtained, respectively, according to the Eq (6) in Definition 8, as shown in Table 3.
stability | information entropy | |||||||
H(s1) | H(s2) | H(s3) | H(s4) | H(s5) | H(s6) | H(s7) | H(s8) | |
SEQ | 2.79 | 2.79 | 0 | 2.79 | 2.80 | 2.69 | 2.00 | 2.79 |
From the preference value distribution information entropy of each state in Table 3, it can be seen that for SEQ stability, H(s3)<H(s7)<H(s6)=H(s1)=H(s2)=H(s4)=H(s8)<H(s5). According to this result and Theorem 2, the following conclusions can be concluded. If the Local government wants Luliang chemical to meet the requirement of SEQ stability in its ideal conflict outcome s5, the state that needs close attention is not the one-step stable Nash stability s1, s2, s4, but the non-one-step reachable state s7. Feedback to the real conflict, it is found that the meaning of state s7 is that the local government agrees to modify the original environmental protection plan to meet Luliang chemical's blind pursuit of interests. In the face of huge commercial interests, Luliang chemical will choose to accept the attitude of no delay and actively carry out incomplete rectification of the pollution caused. At the same time, considering the local economic interests and fluke mentality, the Local government felt that there would not be a major conflict event. Therefore, the Local government took an attitude of turning a blind eye to the incident. It has not supervised Luliang chemical in accordance with the official national pollution control documents strictly. This state has been maintained until the conflict was reported on a large scale by the media. Until the conflict broke out, the environmental protection department of Yunnan Province strongly intervened, preferring to let Luliang chemical not only give up the right to operate in the local area, but also to clean up the local chromium slag pollution thoroughly. At the same time, taking effective measures to completely eliminate the environmental pollution caused by the dumping and stacking of chromium slag, and accepting the third-party agency entrusted by Yunnan Environmental Protection Department to assess according to law. Meanwhile, Luliang chemical also needs to compensate for the environmental loss of 10 million yuan caused by chromium slag pollution, which is used for the treatment of chromium slag pollution and environmental protection of Nanpanjiang River and surrounding ecology. Finally, the conflict was successfully solved [31]. This conclusion also confirms the rationality of the preference perception method in this paper.
Based on the cognition of the rational human being and the consideration of problem solving, all DMs in environmental governance conflicts want to maximize their own interests. At the same time, they do not want to aggravate the conflict process. However, the preference of the DMs involved in the environmental governance conflict is uncertain due to the contradictory nature and complexity of the DMs' interest demands. To achieve their own ideal resolution, the DMs in the conflict require a clear understanding of their strengths and weaknesses. Based on GMCR, this paper constructs a method for predicting the preferences of participants in environmental governance conflicts. This method can perform pre-predict and post-analysis on conflicts between two DMs. In fact, the purpose of studying conflicts is to focus on the reasonable and effective resolution of conflicts, not to aggravate the conflict process. For the ongoing conflict, through the method proposed in this paper, we can make each DM have a clear understanding of their strengths and weaknesses in the conflict, and can predict in advance the impact of taking a certain action on the trend of the conflict. This method can provide a theoretical basis for the high-level strategic choice of one DM to the environmental governance conflict, so that they can better grasp the future trend of the conflict.
The preference perception model developed in this paper applies to the conflicts between two DMs under strict preference order. While, environmental governance involves the interests of many stakeholders, sometimes the preference information of DMs may be partially known or not strictly ordered preference. For example, through the collection and analysis of historical behavior data of opponents, the preference information of opponents can be obtained. Therefore, in the case that the preference of DMs to the environmental governance conflict is partially known or not strictly ordered, it is of great research value to obtain the preference perception of other DMs who make the outcome stable. Then, coping strategies can be made in advance. Finally, creative strategies can be put forward to coordinate the interests of all DMs in environmental governance to resolve the actual conflict.
The research described in this paper was substantially supported by Grant (No. 19BGL235) from the National Social Science Fund of China.
All authors declare no conflicts of interest in this paper.
[1] |
O. Bodin, M. M. Garcia, G. Robins, Reconciling conflict and cooperation in environmental governance: a social network perspective, Annu. Rev. Environ. Resour., 45 (2020), 471–495. https://doi.org/10.1146/annurev-environ-011020-064352 doi: 10.1146/annurev-environ-011020-064352
![]() |
[2] |
Y. Li, V. Homburg, M. Jong, J. Koppenjan, Government responses to environmental conflicts in urban China: The case of the Panyu waste incineration power plant in Guangzhou, J. Cleaner Prod., 134 (2016), 354–361. https://doi.org/10.1016/j.jclepro.2015.10.123 doi: 10.1016/j.jclepro.2015.10.123
![]() |
[3] |
Q. Han, Y. Zhu, G. Y. Ke, H. Lin, A two-stage decision framework for resolving brownfield conflicts, Int. J. Environ. Res. Public Health, 16 (2019), 1039. https://doi.org/10.3390/ijerph16061039 doi: 10.3390/ijerph16061039
![]() |
[4] |
M. Wang, G. Liao, Y. Li, The relationship between environmental regulation, pollution and corporate environmental responsibility, Int. J. Environ. Res. Public Health, 18 (2021), 8018. https://doi.org/10.3390/ijerph18158018 doi: 10.3390/ijerph18158018
![]() |
[5] |
J. Rockström, W. Steffen, K. Noone, A. Persson, F. S. Chapin, E. F. Lambin, et al., A safe operating space for humanity, Nature, 461 (2009), 472–475. https://doi.org/10.1038/461472a doi: 10.1038/461472a
![]() |
[6] |
A. A. Nebasifu, N. M. Atong, Paradoxical gaps in resilient environmental governance, Environ. Rev., 28 (2019), 109–114. https://doi.org/10.1139/er-2019-0028 doi: 10.1139/er-2019-0028
![]() |
[7] |
Q. Xu, S. Dhaundiyal, C. Guan, Structural conflict under the new green dilemma: Inequalities in development of renewable energy for emerging economies, J. Environ. Manage., 273 (2020), 111117. https://doi.org/10.1016/j.jenvman.2020.111117 doi: 10.1016/j.jenvman.2020.111117
![]() |
[8] |
K. W. Hipel, L. Fang, The graph model for conflict resolution and decision support, IEEE Trans. Syst. Man Cybern. Syst., 51 (2021), 131–141. https://doi.org/10.1109/TSMC.2020.3041462 doi: 10.1109/TSMC.2020.3041462
![]() |
[9] |
H. Raiffa, The art and science of negotiation, Econ. J., 93 (1983), 672–673. https://doi.org/10.2307/2232420 doi: 10.2307/2232420
![]() |
[10] |
T. Bolognesi, S. Nahrath, Environmental governance dynamics: Some micro foundations of macro failures, Ecol. Econ., 170 (2020), 106555. https://doi.org/10.1016/j.ecolecon.2019.106555 doi: 10.1016/j.ecolecon.2019.106555
![]() |
[11] |
Y. Han, H. Xu, L. Fang, K. W. Hipel, An integer programming approach to solving the inverse graph model for conflict resolution with two decision makers, Group Decis. Negotiation, 31 (2022), 23–48. https://doi.org/10.1007/s10726-021-09755-w doi: 10.1007/s10726-021-09755-w
![]() |
[12] |
X. Li, Y. Wang, C. Li, Evolution of environmental regulation strategy among local governments and its impact on regional ecological efficiency in china, Environ. Eng. Manage. J., 16 (2017), 2425–2433. https://doi.org/10.30638/eemj.2017.250 doi: 10.30638/eemj.2017.250
![]() |
[13] |
J. Vince, B. D. Hardesty, Plastic pollution challenges in marine and coastal environments: From local to global governance, Restor. Ecol., 25 (2017), 123–128. https://doi.org/10.1111/rec.12388 doi: 10.1111/rec.12388
![]() |
[14] |
K. W. Hipel, D. M. Kilgour, R. A. Kinsara, Strategic investigations of water conflicts in the Middle East, Group Decis. Negotiation, 23 (2014), 355–376. https://doi.org/10.1007/s10726-012-9325-3 doi: 10.1007/s10726-012-9325-3
![]() |
[15] |
Y. Song, D. Huang, P. Xiao, Robustness analysis of equilibrium outcomes based on information-gap of hypergame preference perception, Syst. Eng. Electron., 35 (2013), 362–365. https://doi.org/10.3969/j.issn.1001-506X.2013.02.21 doi: 10.3969/j.issn.1001-506X.2013.02.21
![]() |
[16] |
Y. T. Matbouli, D. M. Kilgour, K. W. Hipel, Robustness of equilibria in the graph model for conflict resolution, J. Syst. Sci. Syst. Eng., 24 (2015), 450–465. https://doi.org/10.1007/s11518-015-5291-z doi: 10.1007/s11518-015-5291-z
![]() |
[17] |
L. C. Rêgo, A. M. Santos, Probabilistic preferences in the graph model for conflict resolution, IEEE Trans. Syst. Man Cybern. Syst., 45 (2015), 595–608. https://doi.org/10.1109/TSMC.2014.2379626 doi: 10.1109/TSMC.2014.2379626
![]() |
[18] |
S. G. B. Walker, K. W. Hipel, T. Inohara, Attitudes and preferences: Approaches to representing decision maker desires, Appl. Math. Comput., 218 (2012), 6637–6647. https://doi.org/10.1016/j.amc.2011.11.102 doi: 10.1016/j.amc.2011.11.102
![]() |
[19] |
M. Bristow, L. Fang, K. W. Hipel, From values to ordinal preferences for strategic governance, IEEE Trans. Syst. Man Cybern. Syst., 44 (2014), 1364–1383. https://doi.org/10.1109/TSMC.2014.2308154 doi: 10.1109/TSMC.2014.2308154
![]() |
[20] |
S. T. Asah, D. N. Bengston, K. Wendt, K. C. Nelson, Diagnostic reframing of intractable environmental problems: Case of a contested multiparty public land-use conflict, J. Environ. Manage., 108 (2012), 108–119. https://doi.org/10.1016/j.jenvman.2012.04.041 doi: 10.1016/j.jenvman.2012.04.041
![]() |
[21] |
X. Wang, X. Li, H. Qiao, Analysis of the crisis response strategies of prosecution-related letters and visits based on game theory, Manage. Rev., 27 (2015), 199–206. https://doi.org/10.14120/j.cnki.cn11-5057/f.2015.07.020 doi: 10.14120/j.cnki.cn11-5057/f.2015.07.020
![]() |
[22] |
J. Zhao, H. Xu, K. W. Hipel, B. Yang, Theory and implementation of sensitivity analyses based on their algebraic representation in the graph model, J. Syst. Sci. Syst. Eng., 28 (2019), 580–601. https://doi.org/10.1007/s11518-019-5412-1 doi: 10.1007/s11518-019-5412-1
![]() |
[23] |
F. Harinck, C. Dreu, A.Vianen, The impact of conflict issues on fixed-pie perceptions, problem solving, and integrative outcomes in negotiation, Organ. Behav. Hum. Decis. Processes, 81 (2000), 329–358. https://doi.org/10.1006/obhd.1999.2873 doi: 10.1006/obhd.1999.2873
![]() |
[24] |
H. Sakakibara, N. Okada, D. Nakase, The application of robustness analysis to the conflict with incomplete information, IEEE Trans. Syst. Man Cybern. C: Appli. Rev., 32 (2002), 14–23. https://doi.org/10.1109/TSMCC.2002.1009122 doi: 10.1109/TSMCC.2002.1009122
![]() |
[25] | H. Xu, K. W. Hipel, D. M. Kilgour, L. Fang, Conflict Resolution Using the Graph Model: Strategic Interactions in Competition and Cooperation, Springer, 2018. https://doi.org/10.1007/978-3-319-77670-5 |
[26] |
R. A. Kinsara, D. M. Kilgour, K. W. Hipel, Inverse approach to the graph model for conflict resolution, IEEE Trans. Syst. Man Cybern. Syst., 45 (2015), 734–742. https://doi.org/10.1109/TSMC.2014.2376473 doi: 10.1109/TSMC.2014.2376473
![]() |
[27] |
E. A. Treml, P. I. J. Fidelman, S. Kininmonth, J. A. Ekstrom, O Bodin, Analyzing the (mis)fit between the institutional and ecological networks of the Indo-West Pacific, Global Environ. Change, 31 (2015), 263–271. https://doi.org/10.1016/j.gloenvcha.2015.01.012 doi: 10.1016/j.gloenvcha.2015.01.012
![]() |
[28] |
S. Kininmonth, A. Bergsten, O. Bodin, Closing the collaborative gap: Aligning social and ecological connectivity for better management of interconnected wetlands, AMBIO, 44 (2015), 138–148. https://doi.org/10.1007/s13280-014-0605-9 doi: 10.1007/s13280-014-0605-9
![]() |
[29] | J. Zhao, H. Xu, Research on inverse problem based on the graph model for ordinal preference, Oper. Res. Manage. Sci., 27 (2018), 143–149. |
[30] |
C. E. Shannon, A mathematical theory of communication, Bell Syst. Tech. J., 27 (1948), 379–423. https://doi.org/10.1002/j.1538-7305.1948.tb01338.x doi: 10.1002/j.1538-7305.1948.tb01338.x
![]() |
[31] |
N. Guo, Y. Tian, The practical obstacles and improvement measures of environment public interest litigation in China–taking example of chromium pollution incident in Qujing, Yunnan, Environ. Pollut. Control, 35 (2013), 96–99. https://doi.org/10.15985/j.cnki.1001-3865.2013.01.003 doi: 10.15985/j.cnki.1001-3865.2013.01.003
![]() |
DM | options | feasible states | |||||||
s1 | s2 | s3 | s4 | s5 | s6 | s7 | s8 | ||
LG | Modify | N | N | N | N | Y | Y | Y | Y |
LC | Delay | N | Y | N | Y | N | Y | N | Y |
Accept | N | N | Y | Y | N | N | Y | Y |
Feasible states | Probability distribution of preference value | |||||||
1 | 2 | 3 | 4 | 5 | 6 | 7 | 8 | |
s1 | 1/6 | 1/6 | 1/6 | 0 | 1/8 | 1/8 | 1/8 | 1/8 |
s2 | 1/6 | 1/6 | 1/6 | 0 | 1/8 | 1/8 | 1/8 | 1/8 |
s3 | 0 | 0 | 0 | 1 | 0 | 0 | 0 | 0 |
s4 | 1/6 | 1/6 | 1/6 | 0 | 1/8 | 1/8 | 1/8 | 1/8 |
s5 | 1/8 | 1/8 | 1/8 | 0 | 5/32 | 5/32 | 5/32 | 5/32 |
s6 | 5/24 | 5/24 | 5/24 | 0 | 3/32 | 3/32 | 3/32 | 3/32 |
s7 | 0 | 0 | 0 | 0 | 1/4 | 1/4 | 1/4 | 1/4 |
s8 | 1/6 | 1/6 | 1/6 | 0 | 1/8 | 1/8 | 1/8 | 1/8 |
stability | information entropy | |||||||
H(s1) | H(s2) | H(s3) | H(s4) | H(s5) | H(s6) | H(s7) | H(s8) | |
SEQ | 2.79 | 2.79 | 0 | 2.79 | 2.80 | 2.69 | 2.00 | 2.79 |
DM | options | feasible states | |||||||
s1 | s2 | s3 | s4 | s5 | s6 | s7 | s8 | ||
LG | Modify | N | N | N | N | Y | Y | Y | Y |
LC | Delay | N | Y | N | Y | N | Y | N | Y |
Accept | N | N | Y | Y | N | N | Y | Y |
Feasible states | Probability distribution of preference value | |||||||
1 | 2 | 3 | 4 | 5 | 6 | 7 | 8 | |
s1 | 1/6 | 1/6 | 1/6 | 0 | 1/8 | 1/8 | 1/8 | 1/8 |
s2 | 1/6 | 1/6 | 1/6 | 0 | 1/8 | 1/8 | 1/8 | 1/8 |
s3 | 0 | 0 | 0 | 1 | 0 | 0 | 0 | 0 |
s4 | 1/6 | 1/6 | 1/6 | 0 | 1/8 | 1/8 | 1/8 | 1/8 |
s5 | 1/8 | 1/8 | 1/8 | 0 | 5/32 | 5/32 | 5/32 | 5/32 |
s6 | 5/24 | 5/24 | 5/24 | 0 | 3/32 | 3/32 | 3/32 | 3/32 |
s7 | 0 | 0 | 0 | 0 | 1/4 | 1/4 | 1/4 | 1/4 |
s8 | 1/6 | 1/6 | 1/6 | 0 | 1/8 | 1/8 | 1/8 | 1/8 |
stability | information entropy | |||||||
H(s1) | H(s2) | H(s3) | H(s4) | H(s5) | H(s6) | H(s7) | H(s8) | |
SEQ | 2.79 | 2.79 | 0 | 2.79 | 2.80 | 2.69 | 2.00 | 2.79 |