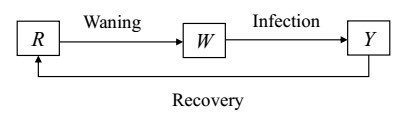
This paper deals with studying monotonicity analysis for discrete fractional operators with Mittag-Leffler in kernel. The ν−monotonicity definitions, namely ν−(strictly) increasing and ν−(strictly) decreasing, are presented as well. By examining the basic properties of the proposed discrete fractional operators together with ν−monotonicity definitions, we find that the investigated discrete fractional operators will be ν2−(strictly) increasing or ν2−(strictly) decreasing in certain domains of the time scale Na:={a,a+1,…}. Finally, the correctness of developed theories is verified by deriving mean value theorem in discrete fractional calculus.
Citation: Pshtiwan Othman Mohammed, Christopher S. Goodrich, Aram Bahroz Brzo, Dumitru Baleanu, Yasser S. Hamed. New classifications of monotonicity investigation for discrete operators with Mittag-Leffler kernel[J]. Mathematical Biosciences and Engineering, 2022, 19(4): 4062-4074. doi: 10.3934/mbe.2022186
[1] | Kento Okuwa, Hisashi Inaba, Toshikazu Kuniya . An age-structured epidemic model with boosting and waning of immune status. Mathematical Biosciences and Engineering, 2021, 18(5): 5707-5736. doi: 10.3934/mbe.2021289 |
[2] | Sarita Bugalia, Jai Prakash Tripathi, Hao Wang . Mathematical modeling of intervention and low medical resource availability with delays: Applications to COVID-19 outbreaks in Spain and Italy. Mathematical Biosciences and Engineering, 2021, 18(5): 5865-5920. doi: 10.3934/mbe.2021295 |
[3] | Mengshi Shu, Rui Fu, Wendi Wang . A bacteriophage model based on CRISPR/Cas immune system in a chemostat. Mathematical Biosciences and Engineering, 2017, 14(5&6): 1361-1377. doi: 10.3934/mbe.2017070 |
[4] | Yendry N. Arguedas, Mario Santana-Cibrian, Jorge X. Velasco-Hernández . Transmission dynamics of acute respiratory diseases in a population structured by age. Mathematical Biosciences and Engineering, 2019, 16(6): 7477-7493. doi: 10.3934/mbe.2019375 |
[5] | Jinhu Xu, Yicang Zhou . Bifurcation analysis of HIV-1 infection model with cell-to-cell transmission and immune response delay. Mathematical Biosciences and Engineering, 2016, 13(2): 343-367. doi: 10.3934/mbe.2015006 |
[6] | Sharmin Sultana, Gilberto González-Parra, Abraham J. Arenas . Dynamics of toxoplasmosis in the cat's population with an exposed stage and a time delay. Mathematical Biosciences and Engineering, 2022, 19(12): 12655-12676. doi: 10.3934/mbe.2022591 |
[7] | Sarafa A. Iyaniwura, Rabiu Musa, Jude D. Kong . A generalized distributed delay model of COVID-19: An endemic model with immunity waning. Mathematical Biosciences and Engineering, 2023, 20(3): 5379-5412. doi: 10.3934/mbe.2023249 |
[8] | Kai Zhang, Xinwei Wang, Hua Liu, Yunpeng Ji, Qiuwei Pan, Yumei Wei, Ming Ma . Mathematical analysis of a human papillomavirus transmission model with vaccination and screening. Mathematical Biosciences and Engineering, 2020, 17(5): 5449-5476. doi: 10.3934/mbe.2020294 |
[9] | Georgi Kapitanov, Christina Alvey, Katia Vogt-Geisse, Zhilan Feng . An age-structured model for the coupled dynamics of HIV and HSV-2. Mathematical Biosciences and Engineering, 2015, 12(4): 803-840. doi: 10.3934/mbe.2015.12.803 |
[10] | Mohammed Meziane, Ali Moussaoui, Vitaly Volpert . On a two-strain epidemic model involving delay equations. Mathematical Biosciences and Engineering, 2023, 20(12): 20683-20711. doi: 10.3934/mbe.2023915 |
This paper deals with studying monotonicity analysis for discrete fractional operators with Mittag-Leffler in kernel. The ν−monotonicity definitions, namely ν−(strictly) increasing and ν−(strictly) decreasing, are presented as well. By examining the basic properties of the proposed discrete fractional operators together with ν−monotonicity definitions, we find that the investigated discrete fractional operators will be ν2−(strictly) increasing or ν2−(strictly) decreasing in certain domains of the time scale Na:={a,a+1,…}. Finally, the correctness of developed theories is verified by deriving mean value theorem in discrete fractional calculus.
Sustained oscillations in the number of infections have been observed for some infectious diseases. A classic example is the highly regular biennial pattern in measles case numbers in England and Wales from 1950 to 1967 (depicted in Fine and Clarkson, 1982 [1]), which is driven by a combination of births and seasonality of transmission related to the opening of school terms.
Mathematically, sustained oscillations can be demonstrated by relatively simple models. Such models focus on a key mechanism such as seasonality in transmission (Aron and Schwartz, 1984 [2]), births and vaccinations (Earn et al., 2000 [3]), pathogen evolution through time scale separation of within and between season dynamics (Andreasen, 2003 [4]) or in combination with seasonality forcing (Dushoff et al., 2004 [5]), changes in human social behavior (d'Onofrio and Manfredi, 2009 [6]), importations of infectious individuals (Silva and Monteiro, 2014 [7]), heterogeneity in transmission due to chronological age (Kuniya and Inaba, 2023 [8] in this special issue) and change in behavior of healthy individuals due to social pressure (Baccili and Monteiro, 2023 [9]). These mechanisms can drive a surge in infections through three main methods: (i) replenishment of susceptible individuals, (ii) replenishment of infectious individuals and (iii) variation of the transmission parameter. Sustained oscillations are possible in these models for certain choices of parameter values.
We argue that waning immunity is also a mechanism that can, by itself, produce sustained oscillations in infection numbers. For diseases with waning immunity, previous infection or vaccination only confers a temporary period of full protection against reinfection. The duration of protection ranges from a few weeks or months for the Omicron variant of SARS-CoV-2 (Burkholz et al., 2023 [10] and Bobrovitz et al., 2023 [11]), to possibly a few years for pertussis (Wendelboe et al., 2005 [12]). As fully immune individuals wane, more individuals become susceptible to infection and more infections occur. Eventually, fewer susceptibles remain and the number of infections falls. When new individuals lose full immunity, replenishment of susceptibles occurs and the cycle repeats.
Here, we present a discrete-time waning immunity model (SIRWY model) that features the aforementioned loss of full immunity as a general population waning distribution, where the proportion of fully immune individuals who wane can be specified on a daily basis. In this Susceptible-Infectious-Immune-Waned-Infectious (SIRWY) model, S and I represent susceptible and infectious individuals without prior immunity, R represents fully immune individuals and W and Y represent waned (susceptible) and infectious individuals with prior immunity.
A special case of SIRWY is the Susceptible-Infectious-Recovered-Susceptible (SIRS) model with geometric distributions for waning and recovery. This is the discrete-time counterpart to the continuous-time classic SIRS model with exponential distributions (Hethcote, 1976 [13]):
dSdt=ωR−βSIdIdt=βSI−γIdRdt=γI−ωR. | (1.1) |
In these equations, ω is the waning rate, β is the transmission rate per infectious individual and γ is the recovery rate. For all parameter values, the number of infections either tends to zero or the endemic equilibrium via exponential decay or damped oscillations (see Hethcote, 1976 [13] or a different proof in §6 of this paper). A variation of the classic SIRS is the SIR1...RnS model with Erlang distribution (sum of exponentials), in which individuals wait in the additional immune sub-compartments before becoming susceptible. It has been shown that sustained oscillations are possible for the SIR1...RnS model when n≥3 (Hethcote et al., 1981 [14]). In this paper, we show that the discrete-time version of SIRS with geometric distributions can generate undamped oscillations, and the oscillatory regime disappears when discrete-time tends to continuous-time.
A different special case of SIRWY is one with homogeneous waning and recovery distributions. This model has fixed waning and recovery times, in which individuals spend exactly the same number of days fully immune and are infectious for exactly same number of days if infected. Due to this fixed delay in terms of time spent in the immune compartment, surges in susceptibles occur at fixed intervals. We show that sustained oscillations can be produced, similar to continuous-time models with delays (Hethcote et al., 1981 [14], Diekmann and Montijn, 1982 [15] and Kyrychko and Blyuss, 2005 [16]).
Besides having a general waning distribution, the full SIRWY model features another effect of waning immunity, the loss of partial immunity, which also contributes to oscillatory dynamics. After losing full immunity, individuals still have partial immunity that reduces their susceptibility to reinfection and potentially also their infectiousness if infected. However, partial immunity wanes over time and individuals become more susceptible and infectious. The accumulation of susceptibility and infectiousness in the population leads to a surge in infections. Similar to the loss of full immunity, this results in a cyclical pattern in infection numbers.
While waning immunity is a mechanism that can generate oscillations, it does not always do so as it depends on the choice of waning immunity effects (losses of full and partial immunity) and how exactly these effects are represented in the model (discrete/continuous time, population waning distribution, changes in susceptibility and infectiousness over time). As a general model which also encompasses different simpler sub-models, SIRWY allows an appropriate model to be chosen based on what is currently known about the infectiousness of the disease and the effects of waning immunity in the population, before scaling up to the full model when needed. Furthermore, for model implementation, discrete-time SIRWY is a system of difference equations, which makes numerical simulations easier to perform as compared with integro-differential equations in continuous-time.
The rest of this paper is structured as follows. We start by presenting the Susceptible-Infectious-Immune-Waned-Infectious (SIRWY) model in §2, before presenting a stability analysis about the disease-free and endemic equilibria in §3. We then proceed to recover two sub-models of the SIRWY model, one with fixed waning and recovery times in §4 and another with geometric distributions for waning and recovery in §5. For these sub-models, we perform bifurcation analysis, on top of the stability analyses about the disease-free and endemic equilibria. We then study the continuous-time exponential SIRS model (equation 1.1) in §6, before making the connection between this model and its discrete-time counterpart in §7 to show that the oscillatory regime disappears in continuous-time.
Since our goal is to understand how waning immunity shapes the long term dynamics of a disease, we consider a population comprising individuals with some degree of immunity because all individuals would have been infected at least once in the long run. Furthermore, as the focus is on waning immunity, other mechanisms that replenish susceptibles such as births are not included (similar to how the evolutionary model is constructed in Pease, 1987 [17]). Since we are also not considering pathogen evolution, this means that all properties of the disease related to infection, recovery and waning of immunity are unchanged over extended periods. Let Rt, Wt and Yt denote the proportions of the population who are in the fully immune, waned and infectious compartments respectively on day t. Here, we always discuss in terms of proportions of population in each compartment rather than exact numbers. For completeness, we give the full Susceptible-Infectious-Immune-Waned-Infectious (SIRWY) model in Appendix 12 but our focus is on the Immune-Waned-Infectious (RWY) model (Figure 1) for the rest of the paper.
Each compartment in the model (R, W and Y) is further divided into sub-compartments based on time since most recent recovery τ (first introduced by Kermack and McKendrick, 1932 [18]) with respect to day t. For example, Rt,τ denotes the proportion of population in the immune R compartment on day t and day τ since recovery. In this notation, we can deduce the day of recovery by subtracting the second subscript from the first subscript (day t−τ for Rt,τ).
The use of time since recovery τ allows us to track the total number of days an individual spends in the R, W and Y compartments. Time since recovery τ starts from day 0 when the individual first joins the R compartment and increases with the day as the individual progresses through the waned W and infectious Y compartments. The value of τ then resets to 0 when the individual rejoins the R compartment (see Figure 2 for an example). This formulation tracks a full RWY cycle (R→W→Y→R→⋯), not just the time spent in each compartment (Inaba, 2001 [19]).
We cover the entire immune spectrum as we have the fully immune R compartment and the waned W and infectious Y compartments with partial immunity based on each day τ since recovery. We do not have the fully susceptible S in the RWY model but it is present in the full SIRWY model. The R compartment represents full immunity against infection, unlike that in Kermack and McKendrick (1932) [18], where R individuals are partially susceptible to infection. Partial immunity provides a reduction in susceptibility ατ of waned W individuals and a reduction in infectiousness χτ of infectious Y individuals. Since the susceptibility of waned individuals depends on the time since recovery τ, there is no need to use the method of stages to partition susceptibles into a fixed number of sub-compartments S1, S2, ..., Sn with n different susceptibilities.
The RWY model, along with a summary of terms in Table 1, is described by the following system of difference equations,
Rt+1,0=∑¯θθ=0(ϕθ−ϕθ+1)yt−θRt+1,τ=ζτRt−(τ−1),0for τ∈{1,⋯,¯τ}Wt+1,1=(ζ0−ζ1)Rt,0Wt+1,τ=Wt,τ−1−yt+1,τ+(ζτ−1−ζτ)Rt−(τ−1),0for τ∈{2,⋯,¯τ+1}Wt+1,τ=Wt,τ−1−yt+1,τfor τ∈{¯τ+2,⋯,˜τ−1}Wt+1,˜τ=Wt,˜τ−1+Wt,˜τ−yt+1,˜τFt=∑¯θθ=0∑˜ττ=2ξθϕθyt−θ,τ(1−χτ)yt+1,τ=(1−ατ−1)Wt,τ−1Ftfor τ∈{2,⋯,˜τ−1}yt+1,˜τ=[(1−α˜τ−1)Wt,˜τ−1+(1−α˜τ)Wt,˜τ]Ft | (2.1) |
Symbol | Variable | Symbol | Index | Symbol | Parameter |
R | immune | θ | time since infection | ϕθ | fraction still infectious |
W | waned | ¯θ+1 | max infectious period | ξθ | infectiousness |
y | newly infected | τ | time since recovery | ζτ | fraction still immune |
Y | infectious | ¯τ+1 | max immune period | χτ | reduction in infectiousness |
F | force of infection | ˜τ | max value of τ | ατ | reduction in susceptibility |
and the conservation of individuals means that
Rt+Wt+Yt=1. | (2.2) |
Each compartment (R, W and Y) can be defined in terms of their corresponding sub-compartments (see Table 2).
Symbol | Variable | Composition |
Rt | immune | Rt=∑¯ττ=0Rt,τ or Rt=∑¯ττ=0ζτRt−τ,0 |
Wt | waned | Wt=∑˜ττ=1Wt,τ |
Yt | infectious | Yt=∑˜ττ=2Yt,τ or Yt=∑¯θθ=0ϕθyt−θ |
yt | newly infected | yt=∑˜ττ=2yt,τ |
In the RWY model (equation 2.1), the newly infected y is used instead of the infectious Y because the infectious Yt=∑¯θθ=0ϕθyt−θ can be expressed in terms of newly infected y at time t and earlier times, with ϕθ representing the fraction of yt−θ still infectious on day θ since infection (as similarly defined by Kermack and McKendrick, 1927 [20]). Here, ϕ0=1 because all infecteds would have just joined the infectious compartment the same day they became infected, which is day 0 since infection. By defining the maximum allowable infectious period to be ¯θ+1 days, the fraction still infectious ϕθ=0 for θ≥¯θ+1 and the infectious Yt is expressed in terms of newly infecteds yt−¯θ to yt.
The force of infection Ft has contributions from the fraction ϕθ of newly infecteds yt−θ,τ who are still infectious on day θ since infection. On day t, each of these individuals contributes a baseline infectiousness ξθ based on the time since infection θ, which is then reduced by a factor of χτ. Here, the τ in both χτ and yt−θ,τ refers to the number of days since recovery with respect to the day of infection t−θ and not with respect to day t. As the maximum infectious period is ¯θ+1 days, infectiousness ξθ=0 for θ≥¯θ+1.
Individuals who recover on day t+1 join the newly recovered Rt+1,0 immune compartment. Using the fraction still infectious ϕθ, we define the population recovery distribution ϕθ−ϕθ+1, which gives the proportion of newly infected yt−θ who recover on day θ+1 since infection.
We define the immune Rt in a similar way as the infectious Yt. Here, Rt=∑¯ττ=0ζτRt−τ,0, where ζτ represents the fraction of newly recovered Rt−τ,0 still immune on day τ since recovery. Again, ζ0 is equals to 1 because all individuals would have just joined the immune compartment the day they recover. By defining the maximum allowable immune period to be ¯τ+1 days, the fraction still immune ζτ=0 for τ≥¯τ+1 and the immune Rt only consists of newly recovereds Rt−¯τ,0 to Rt,0. Since individuals can only remain in the immune compartment for ¯τ+1 days and the immune compartment is the first compartment individuals join after recovery, we can express Rt=∑¯ττ=0Rt,τ in terms of its sub-compartments Rt,τ, with the final term being Rt,¯τ as Rt,τ=0 for τ≥¯τ+1. Using the fraction still immune ζτ, we define the population waning distribution ζτ−1−ζτ, which gives the proportion of newly recovered Rt−(τ−1),0 who wane on day τ since recovery.
The waned Wt and newly infected yt can also be expressed in terms of their sub-compartments. For the waned on day t, Wt,τ starts from Wt,1 rather than Wt,0 because individuals are assumed to spend at least one day in the immune compartment before moving to the waned compartment. By the same reasoning, yt,τ starts from yt,2 because individuals are assumed to spend at least another day in the waned compartment. By defining a maximum value of τ=˜τ, we have Wt=∑˜ττ=1Wt,τ and yt=∑˜ττ=2yt,τ, where the final terms Wt,˜τ and yt,˜τ comprise all waned and newly infecteds individuals respectively with τ≥˜τ. We may also define the infectious Yt=∑˜ττ=2Yt,τ, in terms of its sub-compartments Yt,τ, where Yt,˜τ comprises all infectious individuals with τ≥˜τ.
The waning of partial immunity is a decrease in protection, which is equivalent to decreases in the value of the reduction in susceptibility ατ and potentially also the reduction in infectiousness χτ as τ increases. As we are using a maximum value of τ=˜τ, the reduction in susceptibility ατ reaches a constant value α˜τ for τ≥˜τ and the reduction in infectiousness χτ a constant value χ˜τ for τ≥˜τ. Together with the population waning distribution ζτ−1−ζτ, these three features specify how the losses of full and partial immunity are modeled in the RWY model.
We conclude this section by remarking that we have intentionally made a linear approximation on how the newly infected yt depends on the force of infection Ft (Hernandez-Ceron et al., 2013 [21], Diekmann et al., 2021 [22]). The full description is the following:
yt+1,τ=(1−ατ−1)Wt,τ−1(1−e−Ft)for τ∈{2,⋯,˜τ−1}yt+1,˜τ=[(1−α˜τ−1)Wt,˜τ−1+(1−α˜τ)Wt,˜τ](1−e−Ft). | (2.3) |
This form, however, cannot be utilized easily as Ft is also a function of newly infected yt at earlier times. As long as the force of infection Ft is not too large, we can work with the linearized equation (2.1) to perform stability analysis. Equation 2.1 also allows for efficient numerical simulations to be performed via matrix-vector multiplications.
In this section, we derive the model equations (equation 2.1) at steady state. Letting time tend to infinity and using ∗ to denote the steady state, the immune R equations become
R∗,0=y∗R∗,τ=ζτy∗for τ∈{1,⋯,¯τ} | (3.1) |
after substituting ∑¯θθ=0(ϕθ−ϕθ+1)=1. Adding the equations in (3.1), the proportion of recovered individuals in the population at steady state is
R∗=y∗¯τ∑τ=0ζτ, | (3.2) |
where we have used ζ0=1. Performing a similar analysis on the waned equations, we get:
W∗,1=(ζ0−ζ1)y∗W∗,τ=W∗,τ−1−y∗,τ+(ζτ−1−ζτ)y∗for τ∈{2,⋯,¯τ+1}W∗,τ=W∗,τ−1−y∗,τfor τ∈{¯τ+2,⋯,˜τ−1}W∗,˜τ−1=y∗,˜τ, | (3.3) |
where we have substituted R∗,0=y∗. The waned equations can also expressed in terms of y∗ and its components:
W∗,1=(ζ0−ζ1)y∗W∗,τ=˜τ∑k=τ+1y∗,k−ζτy∗for τ∈{2,⋯,¯τ}W∗,τ=˜τ∑k=τ+1y∗,kfor τ∈{¯τ+1,⋯,˜τ−1}. | (3.4) |
Note that in the above analysis, we do not have an expression for W∗,˜τ yet. The steady state equations for the force of infection and newly infectious are:
F∗=∑¯θθ=0ξθϕθ∑˜ττ=2y∗,τ(1−χτ)y∗,τ=(1−ατ−1)W∗,τ−1F∗for τ∈{2,⋯,˜τ−1}y∗,˜τ=[(1−α˜τ−1)W∗,˜τ−1+(1−α˜τ)W∗,˜τ]F∗. | (3.5) |
Since Yt=∑¯θθ=0ϕθyt−θ, the infectious proportion of population at steady state is
Y∗=y∗¯θ∑θ=0ϕθ. | (3.6) |
The steady state equations above will be used to derive the disease-free and endemic equilibria in the sub-sections that follow.
We derive the disease-free equilibrium by setting the newly infected proportion of the population y∗,τ to be zero for all τ in the steady state equations 3.1, 3.4 and 3.5. By the conservation of individuals (equation 2.2), we find that in the disease-free equilibrium, all individuals end up in the waned W∗,˜τ compartment, with
y∗,τ=0for τ∈{2,⋯,˜τ}W∗,τ=0for τ∈{1,⋯,˜τ−1}W∗,˜τ=1. | (3.7) |
We now proceed to set up a vector system of the model for linear stability analysis about the disease-free equilibrium. Let xt+1=g(xt), where xt is a vector that is formed by the newly infected yt−¯τ−¯θ to yt and the waned Wt. Here, g is a function to be specified using the model equations (2.1 and 2.2).
Linearizing about the disease-free equilibrium and performing elementary column operations on J−λI (where J is the Jacobian for the model equations evaluated at the disease-free equilibrium), we obtain the following characteristic equation:
λ(¯τ+¯θ+2)(˜τ−1)−¯θ[λ¯θ+1−(1−α˜τ)(1−χ˜τ)¯θ∑k=0ξkϕkλ¯θ−k]=0. | (3.8) |
Theorems related to the zeroes of polynomial are discussed in Appendix B. Using Theorem B.6 and Lemma B.7, we find that the disease-free equilibrium is asymptotically stable if and only if R0<1, where the modified basic reproductive ratio is defined as R0=(1−α˜τ)(1−χ˜τ)∑¯θk=0ξkϕk. Note that R0<1 is the explicit threshold for all solutions to tend towards the disease-free equilibrium. Here, R0 is a modified basic reproductive ratio because α˜τ and χ˜τ are not necessary zero, in particular, waned W individuals at τ=˜τ may still be less susceptible to infection than susceptible S individuals.
There are two key observations. First, R0 depends on the reductions in infectiousness χτ and susceptibility ατ only when τ=˜τ and not earlier values of τ. This is because earlier values of τ are transient and hence not present in the steady disease-free equilibrium. The only value of τ that has a lasting effect is when τ takes the maximum value of ˜τ because τ remains at this value thereafter even as the day increases. Second, R0 is independent of population recovery distribution ϕθ−ϕθ+1 and population waning distribution ζτ−1−ζτ (see equation 2.1). Again, this is because recovery takes place for a maximum allowable period of ¯θ+1 days (infectious period) and waning for a maximum allowable period of ¯τ+1 days (immune period), which are transient periods that do not continue until τ=˜τ.
For the endemic equilibrium, an explicit form is difficult to obtain because the steady state equations (3.1, 3.4 and 3.5) are non-linear in y∗,τ. We can make progress in the special case where the reduction in susceptibility and infectiousness are independent of τ, namely, ατ=α and χτ=χ respectively. Then, the force of infection and the newly infected (equation 3.5) can be simplified to:
F∗=(1−χ)y∗∑¯θθ=0ξθϕθy∗,τ=R0y∗W∗,τ−1for τ∈{2,⋯,˜τ−1}y∗,˜τ=R0y∗(W∗,˜τ−1+W∗,˜τ), | (3.9) |
where we have substituted R0=(1−χ)(1−α)∑¯θθ=0ξθϕθ defined earlier. Summing up the y∗,τ equations, we get W∗=1R0. Substituting this equation along with equations 3.2 and 3.6 into R∗+W∗+Y∗=1, we obtain an expression for y∗:
y∗=R0−1R0(∑¯ττ=0ζτ+∑¯θθ=0ϕθ). | (3.10) |
The term in brackets is the sum of the fraction of newly recovered who remain immune and the fraction of newly infecteds who remain infectious throughout the entire immune and infectious period respectively. Clearly, y∗ exists (is greater than zero) if and only if R0 is greater than one. Now, W∗ and y∗ are completely defined in terms of parameter values. Using W∗=∑˜ττ=1W∗,τ, equation 3.4 and W∗=1R0, we can express W∗,˜τ in terms of y∗,τ:
W∗,˜τ=1R0+y∗¯τ∑τ=1ζτ−˜τ∑τ=2˜τ∑j=τy∗,j. | (3.11) |
This equation, along with equations 3.9 and 3.3, allow us to obtain a closed form for the endemic equilibrium:
y∗,τ=R0y2∗∑min(τ−2,¯τ)k=0(ζk−ζk+1)(1−R0y∗)τ−2−kfor τ∈{2,⋯,˜τ−1}y∗,˜τ=y∗∑¯τk=0(ζk−ζk+1)(1−R0y∗)˜τ−2−kW∗,τ=y∗∑min(τ−1,¯τ)k=0(ζk−ζk+1)(1−R0y∗)τ−1−kfor τ∈{1,⋯,˜τ−1}W∗,˜τ=1R0+y∗∑¯ττ=1ζτ−(˜τ−1)y∗∑¯τk=0(ζk−ζk+1)(1−R0y∗)˜τ−2−k−R0y2∗∑˜τ−1τ=2∑˜τ−1j=τ∑min(j−2,¯τ)k=0(ζk−ζk+1)(1−R0y∗)j−2−k. | (3.12) |
In this case, the endemic equilibrium is uniquely defined. Proceeding, we perform stability analysis for the simplest case (˜τ=3, ¯τ=1 and ¯θ=1). Here, R0=(1−χ)(1−α)(ξ0ϕ0+ξ1ϕ1). Equation 3.10 for y∗ simplifies to y∗=R0−1R0(2+ϕ1+ζ1) and the endemic equilibrium (equation 3.12) is
y∗,2=R0y2∗(ζ0−ζ1)y∗,3=y∗[(ζ0−ζ1)(1−R0y∗)+ζ1]W∗,1=y∗(ζ0−ζ1)W∗,2=y∗,3=y∗[(ζ0−ζ1)(1−R0y∗)+ζ1]W∗,3=1R0+y∗ζ1−2y∗[(ζ0−ζ1)(1−R0y∗)+ζ1]−R0y2∗(ζ0−ζ1). | (3.13) |
Performing elementary column operations on J−λI (where J is the Jacobian for the model equations evaluated at the endemic equilibrium), we get the following characteristic equation:
λ5(λ4+3∑s=0ksλs)=0, | (3.14) |
where
k3=(1−α)(1−χ)[−1R0σ0+y∗(σ0+σ1)]k2=(1−α)(1−χ)[−1R0σ1+y∗(σ0+σ1)]k1=[ϕ1+ζ1(1−ϕ1)]R0y∗k0=ζ1ϕ1R0y∗. | (3.15) |
Here, we have used the short-form σθ=ξθϕθ. Using Theorem B.6, we obtain sufficient conditions for the endemic equilibrium to be asymptotically stable. Depending on the sign of k2 and k3, there are four different cases to consider:
1. For k2<0 and k3>0, i.e., 1+2+ϕ1+ζ1σ0+σ1σ0<R0<1+2+ϕ1+ζ1σ0+σ1σ1,
the condition for stability is R0<1+2σ0(2+ϕ1+ζ1)(σ0+σ1)(ϕ1+ζ1).
2. For k2>0 and k3<0, i.e., 1+2+ϕ1+ζ1σ0+σ1σ1<R0<1+2+ϕ1+ζ1σ0+σ1σ0,
the condition for stability is R0<1+2σ1(2+ϕ1+ζ1)(σ0+σ1)(ϕ1+ζ1).
3. For k2>0 and k3>0, i.e., R0>1+2+ϕ1+ζ1σ0+σ1max(σ0,σ1),
the condition for stability is R0<3.
4. For k2<0 and k3<0, i.e., R0<1+2+ϕ1+ζ1σ0+σ1min(σ0,σ1),
the condition for stability is ϕ1+ζ1<2.
Using Lemma B.7, the necessary conditions for the endemic equilibrium to be asymptotically stable are:
1. R0<1+2+ϕ1+ζ1ζ1ϕ1
2. R0<1+2σ0(2+ϕ1+ζ1)(σ0+σ1)(ϕ1+ζ1−2ζ1ϕ1) if ζ1ϕ1<12(ϕ1+ζ1)
R0>1+2σ0(2+ϕ1+ζ1)(σ0+σ1)(ϕ1+ζ1−2ζ1ϕ1) if ζ1ϕ1>12(ϕ1+ζ1).
Unlike the case for the disease-free equilibrium, the sufficient and necessary conditions here do not together provide equivalence conditions for asymptotic stability. As such, we do not have explicit thresholds for the solutions to be in the endemic equilibrium regime. This is similar to models with varying delays in continuous-time, in which sufficient conditions for the endemic equilibrium regime are obtained (Stech and Williams, 1981 [23]) or numerical bifurcation analysis is performed (Blyuss and Kyrychko, 2010 [24]).
We consider the case where every individual follows exactly the same waning and recovery trajectory. Infected individuals join the immune compartment on day ¯θ+1 since infection and recovered individuals join the waned compartment on day ¯τ+1 since recovery. Similar to how classic SIRS is formulated, we consider the simplest model by dropping the dependence on time since infection θ and time since recovery τ, arriving at a constant value of infectiousness ξ, reduction in susceptibility α and reduction in infectiousness χ. The RWY model (equation 2.1) becomes:
Rt+1=Rt+yt−¯θ−yt−¯τ−¯θ−1Wt+1=Wt−yt+1+yt−¯τ−¯θ−1yt+1=βWt¯θ∑θ=0yt−θ, | (4.1) |
where β=ξ(1−χ)(1−α). Note that the proportions of the population in the sub-compartments yt+1,τ and Wt+1,τ are no longer needed to be considered individually as they have been summed up. In addition, from the RWY model (equation 2.1), we get Rt+1,0=yt−¯θ and Rt=∑¯θ+¯τ+1θ=¯θ+1yt−θ. Substituting the latter expression and Wt=1−Rt−Yt into the last equation of (4.1), we obtain a non-linear scalar equation:
yt+1=β¯θ∑θ=0yt−θ(1−¯θ+¯τ+1∑θ=0yt−θ). | (4.2) |
We linearize about the disease-free equilibrium by setting yt=ϵλt, where ϵ is small, to get the following characteristic equation:
λ¯θ+1−β¯θ∑s=0λs=0. | (4.3) |
By Theorem B.6 and Lemma B.7, the disease-free equilibrium is asymptotically stable if and only if R0<1, where R0=β(¯θ+1). The endemic equilibrium can be found using equation (4.2) to be y∗=R0−1R0(¯θ+¯τ+2), which is uniquely defined. Linearizing about the endemic equilibrium, we get the following characteristic equation:
λt+1=2¯θ+¯τ+3−β(¯θ+1)2(¯θ+1)(¯θ+¯τ+2)¯θ∑θ=0λt−θ−β(¯θ+1)−1¯θ+¯τ+2¯θ+¯τ+1∑θ=¯θ+1λt−θ. | (4.4) |
Applying Theorem B.6, the sufficient conditions for asymptotic stability are:
1<R0<3and¯θ>¯τ. | (4.5) |
The second condition requires the infectious period to be greater than the immune period for stability. This agrees with what other continuous-time models have found (Diekmann and Montijn, 1982 [15], Hethcote et al. (1981) [14] and Stech and Williams (1981) [23]): a long immune period can lead to instability of the endemic equilibrium. On the other hand, applying Lemma B.7, the necessary conditions for asymptotic stability are given by:
1<R0<¯θ+¯τ+3. | (4.6) |
Note that ¯θ+¯τ+3 is the minimum amount of time an individual spends going through the R (¯τ+1 days), W (1 day) and Y (¯θ+1 days) compartments before returning to the R compartment.
To obtain explicit thresholds, we consider two special cases. Case 1: If the immune period is one day (¯τ=0), then using Theorem B.5, the endemic equilibrium is asymptotically stable if and only if equation (4.6) holds. Case 2: If the infectious period is one day (¯θ=0), then we use the Determinantal Criterion directly (Theorem B.3) for ¯τ=1, 2 and 3 to get 1<R0<52, 1<R0<5−2√2 and 1<R0<19−5√54 respectively.
We perform bifurcation analysis for these two cases. For case 1 (¯τ=0), at the critical value R0=¯θ+3, the endemic equilibrium loses stability. There are two sub-cases.
1. For ¯θ odd, setting ¯θ=2n+1, the characteristic polynomial is λ2n+3+2n+12n+2∑2n+2j=1λj+1. We apply Theorem (B.8) with a=2n+12n+2, and find that all eigenvalues lie on the unit circle. One of the eigenvalues is −1 and there are (n+1) complex conjugage pairs. A flip (also known as period doubling) and Neimark-Sacker bifurcation occur concurrently.
2. For ¯θ even, setting ¯θ=2n, the characteristic polynomial is λ2n+2+2n2n+1∑2n+1j=1λj+1. Again, applying Theorem (B.8) with a=2n2n+1, all eigenvalues lie on the unit circle and there are (n+1) complex conjugate pairs. A Neimark-Sacker bifurcation occurs.
(a) If ¯θ=0, the eigenvalues are ±i, which leads to a 1:4 (also known as period 4) resonance, for which the constants b and d in the complex normal form can be computed using equations (C.11) and (C.12) to be 94−9i8 and −9i8 respectively.
For case 2 (¯θ=0), for ¯τ=1 to 3, at the critical value of R0, we have a pair of complex conjugate eigenvalues with modulus 1, leading to a Neimark-Sacker bifurcation. There is no strong resonance for these eigenvalues found. The invariant expression d can be computed using equation (C.9) and they are all negative, which means that a stable closed invariant curve bifurcates from the fixed point for R0 greater than the critical value.
Our analysis above for the RWY sub-model with fixed recovery and waning times implies that there are three dynamic regimes - (i) disease-free equilibrium, (ii) endemic equilibrium and (iii) oscillatory solutions due to flip or Neimark-Sacker bifurcations.
We now consider geometric distributions for both recovery and waning. At the individual level, on each day, an infectious individual has a probability γ of recovering and a recovered individual has a probability ω of joining the waned compartment. At the population level, the fraction still infectious is ϕθ=(1−γ)θ and the fraction still immune is ζτ=(1−ω)τ. The R equations can thus be expressed as:
Rt+1=∞∑τ=0(1−ω)τ+1Rt−τ,0+γ∞∑θ=0(1−γ)θyt−θ. | (5.1) |
Note that both the immune period and the infectious period are infinite days long. Furthermore, if we assume that the infectiousness ξ, reduction in susceptibility α and reduction in infectiousness χ are constants, then the RWY model (using equations 2.1, 2.2 and 5.1) becomes:
Rt+1=(1−ω)Rt+γYtWt+1=Wt+ωRt−βWtYtYt+1=(1−γ)Yt+βWtYt, | (5.2) |
where the transmission parameter β=ξ(1−χ)(1−α). Using the property of geometric distributions, we note that the mean number of days an individual stays in the infectious and immune compartments is 1γ and 1ω respectively. Such a model with geometric distributions is often described as 'memoryless' because the probabilities that an infectious individual remains infectious and an immune individual remains immune on a particular day do not depend on the number of days they have already spent in the infectious and immune compartments respectively.
Substituting Rt=1−Yt−Wt and Wt=1βYt[Yt+1−(1−γ)Yt] into the second equation of (5.2), we obtain a scalar nonlinear difference equation:
Yt+1=[(1−ω)YtYt−1−βYt+ω(1−γ+β)+β(1−γ−ω)Yt−1]Yt. | (5.3) |
We linearize about the disease-free equilibrium by letting Yt=ϵλt, where ϵ is small, and obtain the characteristic equation ω[λ−(1−γ+β)]=0. The disease-free equilibrium is asymptotically stable if and only if R0<1, where R0=βγ.
From equation (5.3), the endemic equilibrium can be found to be Y∗=ω(β−γ)β(ω+γ), which is uniquely defined. Linearizing about the endemic equilibrium, we get the characteristic equation λ2+p1λ+p0=0, where p1=−2+ω+ω(β−γ)ω+γ and p0=1−ω+ω(β−γ)(ω+γ−1ω+γ). Using the Determinantal Criterion for order 2 (equation B.2), the endemic equilibrium is asymptotically stable if and only if
1<R0<1+q, | (5.4) |
where
q={γ+ωγ(γ+ω−1)if0<ω<1and4−4ω+ω24−ω<γ<12(2−ω)(γ+ω)γω(2−γ−ω)if0<ω<1and0<γ<4−4ω+ω24−ω. | (5.5) |
At the critical value R∗0=1+2(2−ω)(γ+ω)γω(2−γ−ω), the eigenvalues are −1 and −2+(γ+ω)(−3+ω)−2+γ+ω. The latter eigenvalue cannot take the value 1 or −1 under the constraints for γ and ω. Hence, the Jacobian J has a simple critical eigenvalue −1 and a flip bifurcation occurs. The invariant formula b in the normal form of a flip bifurcation (equation C.6) can be calculated using the formula (equation C.7) to be
b=(−2+γ+ω)3[γ(−1+ω)+(−2+ω)ω][γ2ω+2(−2+ω)ω+γ(−4+ω2)]2[γ(−4+ω)+(−2+ω)2]3(−2+ω)2ω2(γ+ω). | (5.6) |
Under the constraints for γ and ω, b is always greater than 0, which means that a stable period two cycle bifurcates from the fixed point for R0>R∗0.
On the other hand, at the critical value R∗0=1+γ+ωγ(γ+ω−1), the eigenvalues are
−2−γ(−2+ω)+2ω−ω2±i√ω[γ2(4−ω)−(−2+ω)2ω+2γ(−2+4ω−ω2)]2(−1+γ+ω), | (5.7) |
which have modulus one under the constraints for γ and ω. Thus, a Neimark-Sacker bifurcation occurs at the critical value R∗0.
Like the RWY sub-model with fixed recovery and waning times, our analysis here for the sub-model with geometric distributions in discrete-time implies that there are three dynamic regimes - (i) the disease-free equilibrium, (ii) the endemic equilibrium and (iii) oscillatory solutions due to flip or Neimark-Sacker bifurcation.
We take the limit as time step tends to zero for the discrete-time sub-model with geometric distributions (equation 5.2), to get the continuous-time RWY model with exponential distributions:
dRdt=−ωR+γYdWdt=ωR−βWYdYdt=−γY+βWY, | (6.1) |
where β is the transmission rate per infectious individual, ω is the waning rate and γ is the recovery rate. We present the following analysis in the same manner as earlier sections of the paper, and refer the reader to Hethcote, 1976 [13] for an alternative derivation of these results.
The disease-free equilibrium is given by: R∗=0, W∗=1 and Y∗=0. It is asymptotically stable if and only if R0<1, where R0=βγ.
The endemic equilibrium is given by: R∗=γ(β−γ)β(ω+γ), W∗=γβ and Y∗=ω(β−γ)β(ω+γ), which is uniquely defined. Note that the expression for the endemic equilibrium in continuous-time is the same as that in discrete-time. The eigenvalues of the Jacobian matrix are
λ±=12[−ˇω±√ˇω2−4ωγ(R0−1)], | (6.2) |
where ˇω=ω(ωγ+R0ωγ+1). The endemic equilibrium is asymptotically stable if and only if both eigenvalues are negative, which occurs if and only if R0>1. Hence, the RWY sub-model with exponential distributions in continuous-time only has two dynamic regimes: the disease-free equilibrium and the endemic equilibrium. No oscillatory solution is possible.
In this section, we seek to understand how the dynamic regimes change when we transit from discrete-time to continuous-time. The discrete-time sub-model with geometric distributions with time step h is:
Rt+1=(1−hω)Rt+hγYtWt+1=Wt+hωRt−hβWtYtYt+1=(1−hγ)Yt+hβWtYt, | (7.1) |
which is almost identical to the discrete-time sub-model with geometric distributions (equation 5.2), except that each of the parameters ω, β and γ is scaled by h. By taking into account this factor h in previous results for the discrete-time model with a time step of one day, we see that as before, R0=βγ and the disease-free equilibrium is asymptotically stable if and only if R0<1. The endemic equilibrium also remains the same: R∗=γ(β−γ)β(ω+γ), W∗=γβ and Y∗=ω(β−γ)β(ω+γ). It is asymptotically stable if and only if 1<R0<1+qh, where
qh={γ+ωγ(hγ+hω−1)if0<ω<1hand4−4hω+h2ω2h(4−hω)<γ<1h2(2−hω)(γ+ω)hγω(2−hγ−hω)if0<ω<1hand0<γ<4−4hω+h2ω2h(4−hω). | (7.2) |
In the limit as h tends to zero, the top case in equation 7.2 collapses and qh tends to infinity. Hence, the endemic equilibrium is asymptotically stable if and only if R0>1 and the last regime with flip/Neimark-Sacker bifurcation in discrete-time disappears (see Figure 3). The number of dynamic regimes decreases from three to two and oscillatory solutions are no longer possible.
We have intentionally chosen to focus on waning immunity to improve our understanding of the underlying mechanism behind the recent waves of COVID-19 infections in this ongoing pandemic. The emergence of new variants has often been invoked as the reason causing waves of surges in case numbers. However, our work shows that the waning of prior immunity, whether that immunity is from infection or vaccine, is sufficient on its own to drive oscillations. Hence, at least in theory, pathogen evolution may be purely consequential rather the cause of these waves.
In practice, COVID-19 waves are unlikely to be driven by a single mechanism. The effects of phylodynamics (Grenfell et al., 2004 [25]) are complex and there is likely a feedback loop between waning immunity dynamics and pathogen evolution. However, we argue that for diseases with evidence of waning immunity, the loss of immunity should be considered to be a candidate for the main mechanism driving oscillatory dynamics. This is particularly true for diseases with a short temporary period of strong protection like COVID-19, where parsimonious waning immunity models can be used to explain the key trends of observed oscillations in case numbers.
From the public health point of view, policy makers may want to know whether surges in case numbers will happen in the long term for endemic diseases with waning immunity. Surges in case numbers are particularly problematic if the disease has severe health outcomes, especially when healthcare capacity is a major constraint. Models can help by distinguishing between two model outcomes for endemic diseases – the endemic equilibrium and sustained oscillations. The former does not lead to long term surges in case numbers but the latter does. If waning immunity plays a role in sustaining oscillations, then understanding the population infection dynamics can help policy makers decide the timing of booster campaigns to reduce the size of future case surges.
We thank the three anonymous reviewers for their useful feedback, which helped to improve this manuscript.
Desmond Z. Lai is supported by the Trinity Hall Research Studentship and Cambridge International & Department of Applied Mathematics and Theoretical Physics (DAMTP) Scholarship. Julia R. Gog is supported by the JUNIPER partnership (MRC grant no MR/X018598/1).
The authors declare they have not used Artificial Intelligence (AI) tools in the creation of this article.
All authors declare that there is no conflict of interest.
The SIRWY model (see Figure 4) is an extension to the RWY model as it takes into account individuals in the population who do not have prior immunity to the disease. These individuals belong to either the susceptible (S) or infectious (I) compartment. The proportion of population that is infectious at time t is denoted by It=∑¯θθ=0ˇϕθit−θ, which is defined in an analogous manner as its counterpart Yt with prior immunity. Table 3 gives a list of the variables, indices and parameters used in SIRWY model, comparing terms with/without prior immunity whenever possible. The SIRWY model is described by the following system of difference equations,
Ft=ˇft+ft=∑¯θθ=0ˇξθˇϕθit−θ+∑¯θθ=0∑˜ττ=2ξθϕθyt−θ,τ(1−χτ)St+1=St−it+1it+1=StFtRt+1,0=∑¯θθ=0(ˇϕθ−ˇϕθ+1)it−θ+∑¯θθ=0(ϕθ−ϕθ+1)yt−θRt+1,τ=ζτRt−(τ−1),0for τ∈{1,⋯,¯τ}Wt+1,1=(ζ0−ζ1)Rt,0Wt+1,τ=Wt,τ−1−yt+1,τ+(ζτ−1−ζτ)Rt−(τ−1),0for τ∈{2,⋯,¯τ+1}Wt+1,τ=Wt,τ−1−yt+1,τfor τ∈{¯τ+2,⋯,˜τ−1}Wt+1,˜τ=Wt,˜τ−1+Wt,˜τ−yt+1,˜τyt+1,τ=(1−ατ−1)Wt,τ−1Ftfor τ∈{2,⋯,˜τ−1}yt+1,˜τ=[(1−α˜τ−1)Wt,˜τ−1+(1−α˜τ)Wt,˜τ]Ft | (A.1) |
Without | With | Terms without/with prior immunity | Symbol | Other terms |
ˇf | f | force of infection | F | total force of infection |
S | W | susceptible | R | immune |
i | y | newly infected | θ | time since infection |
I | Y | infectious | ¯θ+1 | max infectious period |
ˇϕθ | ϕθ | fraction still infectious | τ | time since recovery |
ˇξθ | ξθ | infectiousness | ¯τ+1 | max immune period |
˜τ | max value of τ | |||
ζτ | fraction still immune | |||
χτ | reduction in infectiousness | |||
ατ | reduction in susceptibility |
and the conservation of individuals means that
St+It+Rt+Wt+Yt=1. | (A.2) |
The I, R, W and Y compartments can be defined in terms of their corresponding sub-compartments (see Table 4). Similar to the RWY model, the SIRWY model is specified using the proportion of newly infecteds (i and y) rather than the proportion of infectious individuals (I and Y).
Symbol | Variable | Composition |
It | infectious (without prior immunity) | It=∑¯θθ=0ˇϕθit−θ |
Rt | immune | Rt=∑¯ττ=0Rt,τ or Rt=∑¯ττ=0ζτRt−τ,0 |
Wt | waned | Wt=∑˜ττ=1Wt,τ |
Yt | infectious (with prior immunity) | Yt=∑˜ττ=2Yt,τ or Yt=∑¯θθ=0ϕθyt−θ |
yt | newly infected (with prior immunity) | yt=∑˜ττ=2yt,τ |
For a kth order difference equation, we define the following characteristic polynomial:
p(λ)=λk+pk−1λk−1+⋯+p1λ+p0, | (B.1) |
where pi are real numbers in our case.
Definition B.1 (Inners of a matrix). (Jury, 1971 [26], Jury, 1974 [27] and Elaydi, 2005 [28]) The inners of a matrix B=(bij) are the matrix itself and all the matrices obtained by sequentially removing the first and last rows and the first and last columns. For example, other than the matrix itself, the inners of the following matrices are indicated by boxes:
![]() |
Definition B.2 (Postive Innerwise). (Jury, 1971 [26], Jury, 1974 [27] and Elaydi, 2005 [28]) A matrix B is positive innerwise if the determinants of all of its inners are positive.
Theorem B.3 (Determinantal Criterion, a special case of Schur-Cohn Criterion). (Jury, 1964 [29], Jury, 1971 [26], Jury, 1974 [27] and Elaydi, 2005 [28]) The zeroes of the characteristic polynomial (equation B.1) lie inside the unit disc if and only if the following hold:
(i) p(1)>0
(ii) (−1)kp(−1)>0
(iii) The (k−1) by (k−1) matrices
B±k−1=(100⋯000pk−110⋯000pk−2pk−11⋯000⋮⋮⋮⋮⋮⋮⋮p4p5p6⋯100p3p4p5⋯pk−110p2p3p4⋯pk−2pk−11)±(000⋯00p0000⋯0p0p1000⋯p0p1p2⋮⋮⋮⋮⋮⋮⋮00p0⋯pk−6pk−5pk−40p0p1⋯pk−5pk−4pk−3p0p1p2⋯pk−4pk−3pk−2) |
are positive innerwise.
Corollary B.4. (Eladyi, 2005 [28]) The three criteria in the Determinantal Criterion have an explicit form for lower orders.
For k=2,
|p1|<1+p0<2. | (B.2) |
For k=3,
|p2+p0|<1+p1and|p1−p2p0|<1−p20. | (B.3) |
In the event that equation B.1 is of the following form with only 2 parameters:
p(λ)=λk+ak−1∑i=iλi+b, | (B.4) |
we have an explicit form for the necessary and sufficient conditions for asymptotic stability.
Theorem B.5. (Tomášek, 2015 [30]) Let a and b be real non-zero constants and k≥2. The zeroes of the characteristic polynomial (equation B.4) lie inside the unit disc if and only if
(i) b>a−1
(ii) b<1
(iii) b>(1−k)a−1.
Theorem B.6 (Sufficient condition for stability). (Eladyi, 2005 [28]) The zeroes of the characteristic polynomial (equation B.1) lie inside the unit disc if
k−1∑i=0|pi|<1. | (B.5) |
Lemma B.7 (Necessary conditions for stability). (Bistritz, 1984 [31]) If the zeroes of the characteristic polynomial (equation B.1) lie inside the unit disc, then the following hold:
(i) |p0|<1
(ii) p(1)>0
(iii) (−1)kp(−1)>0.
Theorem B.8. (Botta et al., 2014 [32]) The zeroes of the polynomial p(λ)=λk+a(λk−1+⋯+λ)+1, a∈R, of degree k>1 lie on the unit circle if and only if
(i) −2k−1≤a≤2 if k is even
(ii) −2k−1≤a≤2+2k−1 if k is odd.
We first start from the following discrete time dynamical system (map) given by
x↦f(x,ω),x∈Rn,ω∈R1. | (C.1) |
To perform a bifurcation analysis on the fixed point x∗ at the critical parameter value ω∗, we do a coordinate shift, placing the fixed point at the origin. Now, in the new coordinates, after renaming and keeping the same variable x, we assume that this system can be written in the form
˜x=Jx+F(x),x∈Rn, | (C.2) |
where J is the Jacobian matrix and F(x) is a smooth function of order O(||x||2) with the following Taylor expansion
F(x)=12K(x,x)+16L(x,x,x)+O(||x||4). | (C.3) |
Here, K(x,y) and L(x,y,z) are defined by
Ki(x,y)=n∑j,k=1∂2Fi(s)∂sj∂sk|s=0xjyk | (C.4) |
and
Li(x,y,z)=n∑j,k,l=1∂3Fi(s)∂sj∂sk∂sl|s=0xjykzl | (C.5) |
for i=1,⋯,n. This presentation and subsequent results in this section are attributed to Kuznetsov, 2004 [33].
Here, assume that the Jacobian J has a simple eigenvalue −1 on the unit circle. The map (equation C.2), when restricted to the center manifold, can be transformed to the normal form
˜y=−y+by3+O(y4), | (C.6) |
where b gives the direction of bifurcation of the period-two cycle and can be computed using the following invariant formula:
b=16⟨p,L(q,q,q)⟩−12⟨p,K(q,(J−I)−1K(q,q))⟩. | (C.7) |
In this case, q is the real eigenvector satisfying Jq=−q while p is the real adjoint eigenvector satisfying JTp=−p. The eigenvectors p and q have been normalized such that ⟨p,q⟩=1. Functions K(x,y) and L(x,y,z) are as defined in equation C.4 and C.5 respectively.
If b>0, then a stable period-two cycle bifurcates from the fixed point for parameter values ω larger than the critical parameter value ω∗. When b<0, then the cycle of period-two is unstable. The case of b=0 means that the bifurcation is more degenerate than the normal form.
Assuming that the Jacobian J has a simple pair of eigenvalues on the unit circle e±iθ0, where θ0∈(0,π) and these are the only eigenvalues with modulus 1. The absence of strong resonances means that eikθ0≠1 for k∈{1,2,3,4}. Then, the map (equation C.2) can be restricted to the center manifold and transformed to the complex normal form
˜z=eiθ0z(1+d1|z|2)+O(|z|4), | (C.8) |
where d=Re d1 gives the direction of the closed invariant curve and can be computed using the following invariant expression
d=12Re{e−iθ0[⟨p,L(q,q,¯q)⟩+2⟨p,K(q,(I−J)−1K(q,¯q))⟩+⟨p,K(¯q,(e2iθ0I−J)−1K(q,q))⟩]}. | (C.9) |
Here, q is the complex eigenvector satisfying Jq=eiθ0q and J¯q=e−iθ0¯q while p is the complex adjoint eigenvector satisfying JTp=e−iθ0p and JT¯p=eiθ0¯p. The scalar product ⟨p,q⟩=¯pTq is the standard one in Cn; p and q have been normalized such that ⟨p,q⟩=1. Functions K(x,y) and L(x,y,z) are as defined in equation C.4 and C.5 respectively.
If d<0, then a stable closed invariant curve bifurcates from the fixed point for parameter values ω larger than the critical parameter value ω∗. When d>0, then the closed invariant curve is unstable. The case of d=0 means that the bifurcation is more degenerate than the normal form.
In this case, Jacobian J has eigenvalues e±iπ2=±i and the map (equation C.2) can be transformed to the following complex normal form
˜z=iz+bz|z|2+d¯z3+O(|z|4), | (C.10) |
where
b=12⟨p,L(q,q,¯q)+2K(q,(I−J)−1K(q,¯q))−K(¯q,(I+J)−1K(q,q))⟩ | (C.11) |
and
d=16⟨p,L(¯q,¯q,¯q)−3K(¯q,(I+J)−1K(¯q,¯q))⟩. | (C.12) |
As before, the terms K, L, p and q are as defined in the previous section where there is an absence of strong resonances.
[1] | C. Goodrich, A. C. Peterson, Discrete Fractional Calculus, Springer, New York, 2015. |
[2] |
T. Abdeljawad, Different type kernel h–fractional differences and their fractional h–sums, Chaos Solitons Fractals, 116 (2018), 146–156. https://doi.org/10.1016/j.chaos.2018.09.022 doi: 10.1016/j.chaos.2018.09.022
![]() |
[3] |
T. Abdeljawad, F. Jarad, A. Atangana, P. O. Mohammed, On a new type of fractional difference operators on h-step isolated time scales, J. Fractional Calculus Nonlinear Syst., 1 (2021), 46–74. https://doi.org/10.48185/jfcns.v1i1.148 doi: 10.48185/jfcns.v1i1.148
![]() |
[4] |
P. O. Mohammed, T. Abdeljawad, Discrete generalized fractional operators defined using h-discrete Mittag-Leffler kernels and applications to AB fractional difference systems, Math. Meth. Appl. Sci., 2020 (2020), 1–26, https://doi.org/10.1002/mma.7083 doi: 10.1002/mma.7083
![]() |
[5] |
T. Abdeljawad, D. Baleanu, Discrete fractional differences with nonsingular discrete Mittag-Leffler kernels, Adv. Differ. Equation, 2016 (2016), 232. https://doi.org/10.1186/s13662-016-0949-5 doi: 10.1186/s13662-016-0949-5
![]() |
[6] |
T. Abdeljawad, F. Madjidi, Lyapunov-type inequalities for fractional difference operators with discrete Mittag-Leffler kernel of order 2<α<5/2, Eur. Phys. J. Spec. Top., 226 (2017), 3355–3368. https://doi.org/10.1140/epjst/e2018-00004-2 doi: 10.1140/epjst/e2018-00004-2
![]() |
[7] |
T. Abdeljawad, Q. M. Al-Mdallal, Q. M. Hajji, Arbitrary order fractional difference operators with discrete exponential kernels and applications, Discrete Dyn. Nat. Soc., 2017 (2017). https://doi.org/10.1155/2017/4149320 doi: 10.1155/2017/4149320
![]() |
[8] |
M. Yavuz, Characterizations of two different fractional operators without singular kernel, Math. Model. Nat. Phenom., 14 (2019), 302. https://doi.org/10.1051/mmnp/2018070 doi: 10.1051/mmnp/2018070
![]() |
[9] |
A. Keten, M. Yavuz, D. Baleanu, Nonlocal cauchy problem via a fractional operator involving power kernel in Banach spaces, Fractal Fractional, 3 (2019), 27. https://doi.org/10.3390/fractalfract3020027 doi: 10.3390/fractalfract3020027
![]() |
[10] |
F. M. Atici, M. Atici, M. Belcher, D. Marshall, A new approach for modeling with discrete fractional equations, Fundam. Inf., 151 (2017), 313–324. https://doi.org/10.3233/FI-2017-1494 doi: 10.3233/FI-2017-1494
![]() |
[11] |
F. M. Atici, M. Atici, N. Nguyen, T. Zhoroev, G. Koch, A study on discrete and discrete fractional pharmacokinetics-pharmacodynamics models for tumor growth and anti-cancer effects, Comput. Math. Biophys., 7 (2019), 10–24. https://doi.org/10.1515/cmb-2019-0002 doi: 10.1515/cmb-2019-0002
![]() |
[12] |
F. M. Atici, S. Sengul, Modeling with fractional difference equations, J. Math. Anal. Appl. 369 (2010), 1–9. https://doi.org/10.1016/j.jmaa.2010.02.009 doi: 10.1016/j.jmaa.2010.02.009
![]() |
[13] |
Z. Wang, B. Shiri, D. Baleanu, Discrete fractional watermark technique, Front. Inf. Technol. Electron. Eng., 21 (2020), 880–883. https://doi.org/10.1631/FITEE.2000133 doi: 10.1631/FITEE.2000133
![]() |
[14] |
G. Wu, D. Baleanu, Y. Bai, Discrete fractional masks and their applications to image enhancement, Handb. Fractional Calculus Appl., 8 (2019), 261–270. https://doi.org/10.1515/9783110571929 doi: 10.1515/9783110571929
![]() |
[15] |
T. Abdeljawad, Q. M. Al-Mdallal, Discrete Mittag-Leffler kernel type fractional difference initial value problems and Gronwall's inequality, J. Comput. Appl. Math., 339 (2018), 218–230. https://doi.org/10.1016/j.cam.2017.10.021 doi: 10.1016/j.cam.2017.10.021
![]() |
[16] |
A. Khan, H. M. Alshehri, T. Abdeljawad, Q. M. Al-Mdallal, H. Khan, Stability analysis of fractional nabla difference COVID-19 model, Results Phys., 22 (2021), 103888. https://doi.org/10.1016/j.rinp.2021.103888 doi: 10.1016/j.rinp.2021.103888
![]() |
[17] |
A. Shaikh, K. S. Nisar, V. Jadhav, S. K.Elagan, M. Zakarya, Dynamical behaviour of HIV/AIDS model using fractional derivative with Mittag-Leffler kernel, Alexandria Eng. J., 61 (2022), 2601–2610. https://doi.org/10.1016/j.aej.2021.08.030 doi: 10.1016/j.aej.2021.08.030
![]() |
[18] |
C. Ravichandran, K. Logeswari, S. K. Panda, K. S. Nisar, On new approach of fractional derivative by Mittag-Leffler kernel to neutral integro-differential systems with impulsive conditions, Chaos Solitons Fractals, 139 (2020), 110012. https://doi.org/10.1016/j.chaos.2020.110012 doi: 10.1016/j.chaos.2020.110012
![]() |
[19] |
H. Dong, Y.Gao, Existence and uniqueness of bounded stable solutions to the Peierls-Nabarro model for curved dislocations, Calculus Variations Partial Differ. Equation, 60 (2021), 62. https://doi.org/10.1007/s00526-021-01939-1 doi: 10.1007/s00526-021-01939-1
![]() |
[20] | Y. Gao, J. G. Liu, Z. Liu, Existence and rigidity of the vectorial Peierls-Nabarro model for dislocations in high dimensions, Nonlinearity, 34 (2021), 7778. |
[21] |
P. O. Mohammed, O. Almutairi, R. P. Agarwal, Y. S. Hamed, On convexity, monotonicity and positivity analysis for discrete fractional operators defined using exponential kernels, Fractal Fractional, 6 (2022), 55. https://doi.org/10.3390/fractalfract6020055 doi: 10.3390/fractalfract6020055
![]() |
[22] |
R. Dahal, C. S. Goodrich, A monotonicity result for discrete fractional difference operators, Arch. Math. (Basel), 102 (2014), 293–299. https://doi.org/10.1007/s00013-014-0620-x doi: 10.1007/s00013-014-0620-x
![]() |
[23] |
T. Abdeljawad, D. Baleanu, Monotonicity analysis of a nabla discrete fractional operator with discrete Mittag-Leffler kernel, Chaos Solitons Fractals, 116 (2017), 1–5. https://doi.org/10.1016/j.chaos.2017.04.006 doi: 10.1016/j.chaos.2017.04.006
![]() |
[24] |
I. Suwan, T. Abdeljawad, F. Jarad, Monotonicity analysis for nabla h-discrete fractional Atangana-Baleanu differences, Chaos Solitons Fractals, 117 (2018), 50–59. https://doi.org/10.1016/j.chaos.2018.10.010 doi: 10.1016/j.chaos.2018.10.010
![]() |
[25] | T. Abdeljawad, B. Abdallaa, Monotonicity results for delta and nabla Caputo and Riemann fractional differences via dual identities, preprint, arXiv: 1601.05510. |
[26] |
C. S. Goodrich, J. M. Jonnalagadda, An analysis of polynomial sequences and their application to discrete fractional operators, J. Differ. Equations Appl., 27 (2021), 1081–1102. https://doi.org/10.1080/10236198.2021.1965132 doi: 10.1080/10236198.2021.1965132
![]() |
[27] |
P. O. Mohammed, T. Abdeljawad, F. K. Hamasalh, On Riemann-Liouville and Caputo fractional forward difference monotonicity analysis, Mathematics, 9(2021), 1303. https://doi.org/10.3390/math9111303 doi: 10.3390/math9111303
![]() |
[28] |
P. O. Mohammed, F. K. Hamasalh, T. Abdeljawad, Difference monotonicity analysis on discrete fractional operators with discrete generalized Mittag-Leffler kernels, Adv. Differ. Equation, 2021, 2021, 213. https://doi.org/10.1186/s13662-021-03372-2 doi: 10.1186/s13662-021-03372-2
![]() |
[29] |
J. Bravo, C. Lizama, S. Rueda, Second and third order forward difference operator: what is in between?, Rev. R. Acad. Cienc. Exactas Fís. Nat. Ser. A Mat. RACSAM, 115 (2021), 1–20. https://doi.org/10.1007/s13398-021-01015-5 doi: 10.1007/s13398-021-01015-5
![]() |
[30] |
C. S. Goodrich, C. Lizama, A transference principle for nonlocal operators using a convolutional approach: fractional monotonicity and convexity, Israel J. Math., 236 (2020), 533–589. https://doi.org/10.1007/s11856-020-1991-2 doi: 10.1007/s11856-020-1991-2
![]() |
[31] |
C. S. Goodrich, C. Lizama, Positivity, monotonicity, and convexity for convolution operators, Discrete Contin. Dyn. Syst., 40 (2020), 4961–4983. https://doi.org/10.3934/dcds.2020207 doi: 10.3934/dcds.2020207
![]() |
[32] |
C. S. Goodrich, B. Lyons, Positivity and monotonicity results for triple sequential fractional differences via convolution, Analysis, 40 (2020), 89–103. https://doi.org/10.1515/anly-2019-0050 doi: 10.1515/anly-2019-0050
![]() |
[33] |
C. S. Goodrich, B. Lyons, M. T. Velcsov, Analytical and numerical monotonicity results for discrete fractional sequential differences with negative lower bound, Commun. Pure Appl. Anal., 20 (2021), 339–358. https://doi.org/10.3934/cpaa.2020269 doi: 10.3934/cpaa.2020269
![]() |
[34] |
C. S. Goodrich, J. M. Jonnalagadda, B. Lyons, Convexity, monotonicity, and positivity results for sequential fractional nabla difference operators with discrete exponential kernels, Math. Meth. Appl. Sci., 44 (2021), https://doi.org/10.1002/mma.7247 doi: 10.1002/mma.7247
![]() |
[35] |
C. S. Goodrich, M. Muellner, An analysis of the sharpness of monotonicity results via homotopy for sequential fractional operators, Appl. Math. Lett., 98 (2019), 446–452. https://doi.org/10.1016/j.aml.2019.07.003 doi: 10.1016/j.aml.2019.07.003
![]() |
[36] | F. M. Atici, M. Uyanik, Analysis of discrete fractional operators, Appl. Anal. Discrete Math., 9 2015,139–149. https://doi.org/10.2298/AADM150218007A |
1. | Min-Jia Hsieh, Ping-Hsing Tsai, Pin-Hsuan Chiang, Zih-Kai Kao, Zi-Qing Zhuang, Ai-Ru Hsieh, Hsiang-Ling Ho, Shih-Hwa Chiou, Kung-Hao Liang, Yu-Chun Chen, Genomic insights into mRNA COVID-19 vaccines efficacy: Linking genetic polymorphisms to waning immunity, 2024, 20, 2164-5515, 10.1080/21645515.2024.2399382 |
Symbol | Variable | Symbol | Index | Symbol | Parameter |
R | immune | θ | time since infection | ϕθ | fraction still infectious |
W | waned | ¯θ+1 | max infectious period | ξθ | infectiousness |
y | newly infected | τ | time since recovery | ζτ | fraction still immune |
Y | infectious | ¯τ+1 | max immune period | χτ | reduction in infectiousness |
F | force of infection | ˜τ | max value of τ | ατ | reduction in susceptibility |
Symbol | Variable | Composition |
Rt | immune | Rt=∑¯ττ=0Rt,τ or Rt=∑¯ττ=0ζτRt−τ,0 |
Wt | waned | Wt=∑˜ττ=1Wt,τ |
Yt | infectious | Yt=∑˜ττ=2Yt,τ or Yt=∑¯θθ=0ϕθyt−θ |
yt | newly infected | yt=∑˜ττ=2yt,τ |
Without | With | Terms without/with prior immunity | Symbol | Other terms |
ˇf | f | force of infection | F | total force of infection |
S | W | susceptible | R | immune |
i | y | newly infected | θ | time since infection |
I | Y | infectious | ¯θ+1 | max infectious period |
ˇϕθ | ϕθ | fraction still infectious | τ | time since recovery |
ˇξθ | ξθ | infectiousness | ¯τ+1 | max immune period |
˜τ | max value of τ | |||
ζτ | fraction still immune | |||
χτ | reduction in infectiousness | |||
ατ | reduction in susceptibility |
Symbol | Variable | Composition |
It | infectious (without prior immunity) | It=∑¯θθ=0ˇϕθit−θ |
Rt | immune | Rt=∑¯ττ=0Rt,τ or Rt=∑¯ττ=0ζτRt−τ,0 |
Wt | waned | Wt=∑˜ττ=1Wt,τ |
Yt | infectious (with prior immunity) | Yt=∑˜ττ=2Yt,τ or Yt=∑¯θθ=0ϕθyt−θ |
yt | newly infected (with prior immunity) | yt=∑˜ττ=2yt,τ |
Symbol | Variable | Symbol | Index | Symbol | Parameter |
R | immune | θ | time since infection | ϕθ | fraction still infectious |
W | waned | ¯θ+1 | max infectious period | ξθ | infectiousness |
y | newly infected | τ | time since recovery | ζτ | fraction still immune |
Y | infectious | ¯τ+1 | max immune period | χτ | reduction in infectiousness |
F | force of infection | ˜τ | max value of τ | ατ | reduction in susceptibility |
Symbol | Variable | Composition |
Rt | immune | Rt=∑¯ττ=0Rt,τ or Rt=∑¯ττ=0ζτRt−τ,0 |
Wt | waned | Wt=∑˜ττ=1Wt,τ |
Yt | infectious | Yt=∑˜ττ=2Yt,τ or Yt=∑¯θθ=0ϕθyt−θ |
yt | newly infected | yt=∑˜ττ=2yt,τ |
Without | With | Terms without/with prior immunity | Symbol | Other terms |
ˇf | f | force of infection | F | total force of infection |
S | W | susceptible | R | immune |
i | y | newly infected | θ | time since infection |
I | Y | infectious | ¯θ+1 | max infectious period |
ˇϕθ | ϕθ | fraction still infectious | τ | time since recovery |
ˇξθ | ξθ | infectiousness | ¯τ+1 | max immune period |
˜τ | max value of τ | |||
ζτ | fraction still immune | |||
χτ | reduction in infectiousness | |||
ατ | reduction in susceptibility |
Symbol | Variable | Composition |
It | infectious (without prior immunity) | It=∑¯θθ=0ˇϕθit−θ |
Rt | immune | Rt=∑¯ττ=0Rt,τ or Rt=∑¯ττ=0ζτRt−τ,0 |
Wt | waned | Wt=∑˜ττ=1Wt,τ |
Yt | infectious (with prior immunity) | Yt=∑˜ττ=2Yt,τ or Yt=∑¯θθ=0ϕθyt−θ |
yt | newly infected (with prior immunity) | yt=∑˜ττ=2yt,τ |