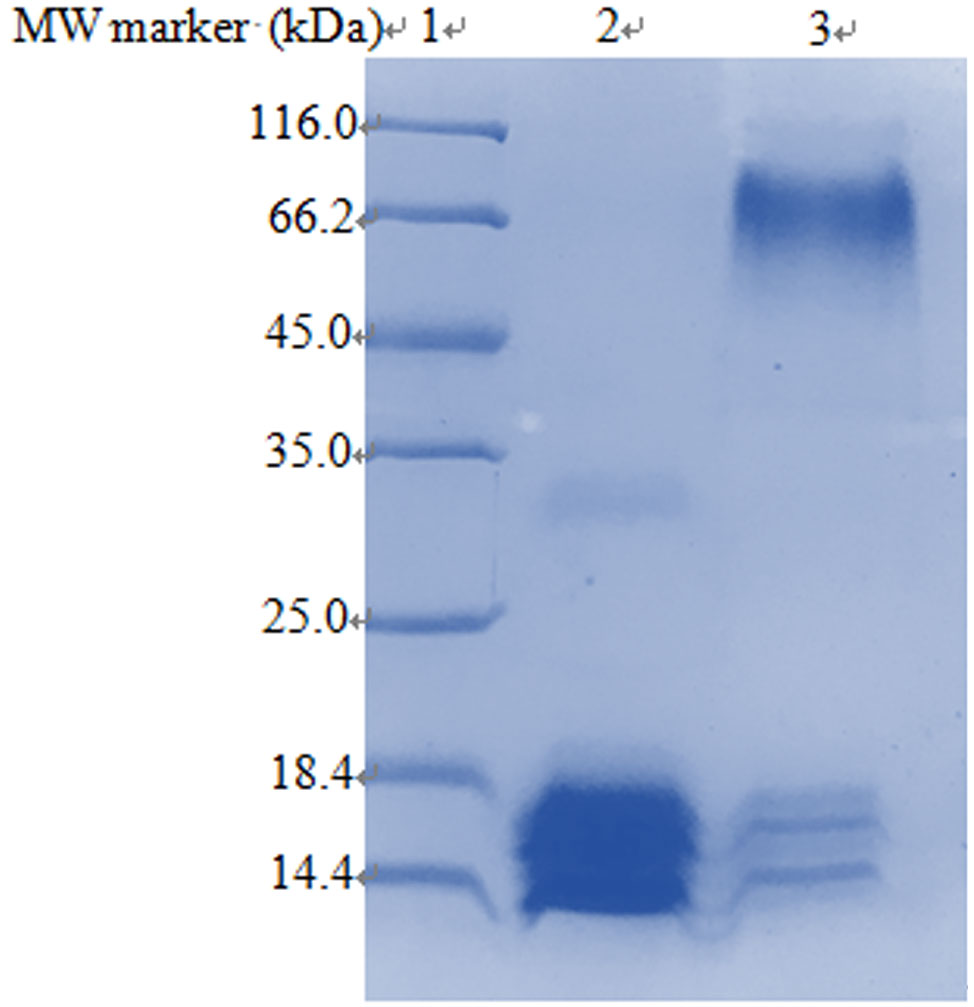
This paper proposes a probabilistic graphical model that integrates interpretive structural modeling (ISM) and Bayesian belief network (BBN) approaches to predict cone penetration test (CPT)-based soil liquefaction potential. In this study, an ISM approach was employed to identify relationships between influence factors, whereas BBN approach was used to describe the quantitative strength of their relationships using conditional and marginal probabilities. The proposed model combines major causes, such as soil, seismic and site conditions, of seismic soil liquefaction at once. To demonstrate the application of the propose framework, the paper elaborates on each phase of the BBN framework, which is then validated with historical empirical data. In context of the rate of successful prediction of liquefaction and non-liquefaction events, the proposed probabilistic graphical model is proven to be more effective, compared to logistic regression, support vector machine, random forest and naive Bayes methods. This research also interprets sensitivity analysis and the most probable explanation of seismic soil liquefaction appertaining to engineering perspective.
Citation: Mahmood Ahmad, Feezan Ahmad, Jiandong Huang, Muhammad Junaid Iqbal, Muhammad Safdar, Nima Pirhadi. Probabilistic evaluation of CPT-based seismic soil liquefaction potential: towards the integration of interpretive structural modeling and bayesian belief network[J]. Mathematical Biosciences and Engineering, 2021, 18(6): 9233-9252. doi: 10.3934/mbe.2021454
[1] | Zhuang Wang, Renting Liu, Jie Xu, Yusheng Fu . FedSC: A federated learning algorithm based on client-side clustering. Electronic Research Archive, 2023, 31(9): 5226-5249. doi: 10.3934/era.2023266 |
[2] | Xiaoyu Jiang, Ruichun Gu, Huan Zhan . Research on incentive mechanisms for anti-heterogeneous federated learning based on reputation and contribution. Electronic Research Archive, 2024, 32(3): 1731-1748. doi: 10.3934/era.2024079 |
[3] | Zhiyong Qian, Wangsen Xiao, Shulan Hu . The generalization ability of logistic regression with Markov sampling. Electronic Research Archive, 2023, 31(9): 5250-5266. doi: 10.3934/era.2023267 |
[4] | Shuangjie Yuan, Jun Zhang, Yujia Lin, Lu Yang . Hybrid self-supervised monocular visual odometry system based on spatio-temporal features. Electronic Research Archive, 2024, 32(5): 3543-3568. doi: 10.3934/era.2024163 |
[5] | Wenya Shi, Xinpeng Yan, Zhan Huan . Faster free pseudoinverse greedy block Kaczmarz method for image recovery. Electronic Research Archive, 2024, 32(6): 3973-3988. doi: 10.3934/era.2024178 |
[6] | Fang Wang, Weiguo Li, Wendi Bao, Li Liu . Greedy randomized and maximal weighted residual Kaczmarz methods with oblique projection. Electronic Research Archive, 2022, 30(4): 1158-1186. doi: 10.3934/era.2022062 |
[7] | Shiqi Zou, Xiaoping Shi, Shenmin Song . MOEA with adaptive operator based on reinforcement learning for weapon target assignment. Electronic Research Archive, 2024, 32(3): 1498-1532. doi: 10.3934/era.2024069 |
[8] | Hui Li, Rongrong Gong, Pengfei Hou, Libao Xing, Dongbao Jia, Haining Li . Online learning resources recommendation model based on improved NSGA-Ⅱ algorithm. Electronic Research Archive, 2023, 31(5): 3030-3049. doi: 10.3934/era.2023153 |
[9] | Jing Chen, Weiyu Ye, Shaowei Kang . Learning user preferences from Multi-Contextual Sequence influences for next POI recommendation. Electronic Research Archive, 2024, 32(1): 486-504. doi: 10.3934/era.2024024 |
[10] | Bangna Li, Qingqing Zhang, Xingshi He . Multi-label feature selection via constraint mapping space regularization. Electronic Research Archive, 2024, 32(4): 2598-2620. doi: 10.3934/era.2024118 |
This paper proposes a probabilistic graphical model that integrates interpretive structural modeling (ISM) and Bayesian belief network (BBN) approaches to predict cone penetration test (CPT)-based soil liquefaction potential. In this study, an ISM approach was employed to identify relationships between influence factors, whereas BBN approach was used to describe the quantitative strength of their relationships using conditional and marginal probabilities. The proposed model combines major causes, such as soil, seismic and site conditions, of seismic soil liquefaction at once. To demonstrate the application of the propose framework, the paper elaborates on each phase of the BBN framework, which is then validated with historical empirical data. In context of the rate of successful prediction of liquefaction and non-liquefaction events, the proposed probabilistic graphical model is proven to be more effective, compared to logistic regression, support vector machine, random forest and naive Bayes methods. This research also interprets sensitivity analysis and the most probable explanation of seismic soil liquefaction appertaining to engineering perspective.
Streptavidin is a ∼60 kDa homotetramer that is resistant to heat, Tm ≥ 75 °C [1], proteolysis [2] and chemical denaturants [3]. The protein binds four molecules of biotin with the highest affinity. The binding affinity of biotin to streptavidin and avidin is one of the highest reported for a non-covalent interaction to date, with a KD ∼10−14 M [4]. Stability of the streptavidin tetramer is significantly enhanced upon biotin binding, with Tm increasing from 75 °C to 112 °C [1]. The reaction model is simple, with a single transition state and association rate constant assumed to be near diffusion-limited [5]. Energetic contributions to this tight-binding complex include van der Waals forces and hydrophobic interactions at the biotin binding pocket, hydrogen bond network that extends from the biotin binding pocket and the transition of the binding loop, residues 45 to 52 [6]. Perturbation of hydrophobic side chains at the biotin binding pocket or deletion of the binding loop results in a massive loss in binding affinity, by as much as six orders of magnitude [7],[8]. Amino acid residues, N23, S27, Y43, S45 and D128, that form hydrogen bonds with the ureido atoms of biotin have been shown to significantly contribute to the complex stability as loss of these hydrogen bonds results in a significant decrease in the free energy of binding and up to a 300-fold decrease in the binding affinity [6],[8],[9]. An interface mutation, N54A, prevents dimerization of the streptavidin monomers even upon ligand binding and reduces the binding affinity to KD ∼ 10−7 M [5],[10].
The streptavidin-biotin complex has been exploited in various biological, medical, chemical and material science applications [2],[5],[11]. Molecular recognition of biotin and streptavidin has been studied using radio-labelling [12],[13], equilibrium dialysis [14],[15], fluorescence [3], enzyme-linked immunosorbent assays [7], surface plasmon resonance [16], atomic force microscopy [17],[18], molecular dynamics simulations [19]; stopped-flow kinetics [5], micro-calorimeter [20], conventional isothermal titration calorimetry (ITC) [6] and ligand-displacement ITC [15]. However, none of these techniques is without drawbacks. For example, immobilised proteins lose activity over time thus, limiting the sensitivity of the techniques. On the other hand, the use of labelling agents introduces artefacts that interfere with the signal whilst the use of computational modelling tools is limited by the failure to capture the extent of binding interactions.
An ITC has an advantage over these techniques due to its ability to directly measure the thermodynamic parameters of interaction in solution without immobilisation, labelling, modification and with no molecular weight restrictions [21],[22]. ITC is a sensitive and robust biophysical technique that measures the direct heat released or absorbed upon ligand-receptor binding. The heat absorbed or generated as a result of the interaction is measured as power changes needed to maintain isothermal conditions between the reference and the sample cell. From a single ITC assay, we can instantly obtain the binding constant (KD), binding enthalpy (ΔH), entropy (ΔS) and stoichiometry (n), from which Gibb's free energy (ΔG) can be calculated [15],[23],[24]. These thermodynamic parameters can be extrapolated to help define the nature and mechanism of binding, i.e. enthalpically-favoured interactions tend to be driven by polar and van der Waals interactions whereas, hydrophobic interactions tend to be entropically-favoured [25],[26]. Despite the accuracy of ITC in measuring affinities of chemical and biochemical interactions, the conventional ITC is limited by its large sample volume and concentration requirements, and the inability to reliably interrogate molecular interactions of tight ligand-receptor binding, Ka > 109 M−1 [21],[27]. The current study set out to investigate the binding thermodynamic parameters, and temperature-dependence thereof, of biotin to the bacterium Streptomyces avidinii-derived streptavidin protein using an Affinity ITC instrument from TA Instruments.
Streptavidin was purchased from Sigma-Aldrich (catalogue number 85878-5 mg). Lyophilized streptavidin was reconstituted according to manufacturers' instructions in phosphate-buffered saline (PBS) buffer, pH 7.8, without further purification prior to use. HPLC quality grade biotin was purchased as a lyophilized powder from Sigma-Aldrich (catalogue number B4501-1 g). The working stock solution of biotin was prepared by dissolving the lyophilized powder in dimethyl sulfoxide (DMSO) before diluting to a final concentration of 0.1 M in PBS buffer, pH 7.8.
Sodium dodecyl sulphate-polyacrylamide (SDS–PAGE) gel electrophoresis analysis was performed as previously described [28], using a 12 % gel to assess protein purity and integrity. The protein sample was boiled for 5 minutes before loading and electrophoresis were performed at 80 V for 60 min. The gel was stained then destained to visualise protein bands. An aliquot of the protein solution was used to estimate streptavidin concentration using the Beer-Lambert law, and an extinction coefficient of 41 820 M−1 cm−1 (at 280 nm) [9],[29]. Absorbance spectra of the protein were recorded using a Chirascan (Applied PhotoPhysics, UK).
Final streptavidin concentration of 40 µM was loaded into the Affinity ITC (TA Instruments) sample cell while 750 µM of biotin was used in the titration syringe. Total volumes of 350 µl and 250 µl were loaded into the sample cell and titration syringe, respectively. Titrations were performed by injecting 5 µl of the ligand into the protein at 200 seconds intervals and stirring at 75 rpm. Thermodynamics of the binding of biotin to streptavidin were measured at 9 different temperatures, 2 °C, 6 °C, 10 °C, 15 °C, 20 °C, 25 °C, 30 °C, 35 °C and 40 °C. Raw ITC data were integrated using the NanoAnalyze software (TA Instruments) and presented as thermograms. The data were fitted using an independent model to yield equilibrium thermodynamic parameters using NanoAnalyze software (TA Instruments).
Upon reconstitution of streptavidin into PBS buffer, purity and the size of the protein were confirmed using a 12% SDS-PAGE gel (Figure 1). No further purification of the protein was carried out. Unbound core-streptavidin presented as monomers of ∼16 kDa whereas, the biotin-bound protein, > 66 kDa, proved to be resistant to strong denaturant, SDS, as well as the influence of boiling during sample preparations thus, maintaining its quaternary structure. The streptavidin concentration was calculated at 280 nm using an extinction coefficient of 41 820 M−1 cm−1 [9],[29]. To understand the forces that enhanced the high-affinity protein-ligand interactions of biotin and streptavidin, an ITC Affinity instrument (TA Instruments) was employed. Raw ITC data were integrated and an independent binding model was selected to fit the isotherms as represented in Figure 2. Assays were carried out at various temperatures, i.e., 2 °C, 6 °C, 10 °C, 15 °C, 20 °C, 25 °C, 30 °C, 35 °C and 40 °C, to determine the temperature-dependence of the binding enthalpy. Other thermodynamic parameters of binding were also determined (Table 1). A high affinity, with a dissociation constant (KD) of 10−9 M, is reported at lower temperatures (2 °C to 20 °C) relative to moderate affinity (KD = 10−4 M to 10−7 M) at higher temperatures (25 °C to 40 °C). The stoichiometry of binding or molecular binding ratio of a ligand per monomer of protein, n, was determined from the model fit (Figure 2, lower panel) as the midpoint of the transition. A stoichiometry closer to 1 (0.94–0.98) was reported at 15 °C to 25 °C, and increased from thereon, to 1.59–3.41 between 30 °C and 40 °C whilst, on the other hand, it ranged from 2.74 to 5.76 at lower temperatures (2 °C to 10 °C)
Temp (°C) | KD (M) | n | ΔG (kcal/mol) | ΔH (kcal/mol) | ΔS (cal/mol K) | TΔS (kcal/mol) |
2 | 1.00E-09 | 3.14 | −11.33 | 3.67 | 0.05 | 15.01 |
6 | 1.00E-09 | 5.76 | −11.49 | 2.98 | 0.05 | 14.47 |
10 | 1.00E-09 | 2.74 | −11.66 | 2.10 | 0.04 | 13.76 |
15 | 1.00E-09 | 0.94 | −11.86 | −2.57 | 0.03 | 9.29 |
20 | 1.00E-09 | 0.95 | −12.07 | −3.72 | 0.02 | 8.35 |
25 | 3.14E-07 | 0.98 | −8.87 | −5.63 | 0.01 | 3.24 |
30 | 1.79E-05 | 1.92 | −6.58 | −8.80 | −0.007 | −2.21 |
35 | 1.74E-04 | 1.59 | −5.30 | −23.90 | −0.06 | −18.60 |
40 | 2.88E-04 | 3.41 | −5.08 | −23.90 | −0.06 | −18.82 |
The change in heat capacity (ΔCp) was also measured from 2 °C to 30 °C to provide further thermodynamic insight into the streptavidin and biotin binding interaction (Figure 3). We report ΔCp value of −459.9 cal/mol K, calculated as the slope of temperature-dependence of binding enthalpy between 2 °C and 30 °C (Figure 3). This ΔCp is in agreement with that previously obtained and is consistent with a buried surface area in the streptavidin-biotin bound state [30]. Similar to protein unfolding, protein-ligand interactions are often accompanied by significant changes in heat capacity (ΔCp) of the system. The extent to which heat capacity changes are associated with the solvent-accessible surface area of the molecule thus, ΔCp may be employed to determine solvation state independent of other factors [31]. The binding mechanism of biotin to streptavidin was studied by determining the contribution of enthalpic and entropic energies to the overall affinity at various reaction temperatures. The complex formation proved to be affected by temperature changes (Figure 4). The reaction was entropically-driven between 15 °C and 25 °C but had unfavourable entropic contributions at higher temperatures tested (30 °C–40 °C). A tighter binding, indicated by an increasingly negative ΔG and lower KD values was observed at lower reaction temperatures, i.e., 2 °C to 20 °C.
Each of the four subunits of the streptavidin protein binds a single molecule of biotin with high specificity and forms the strongest non-covalent bond known in biochemistry. Upon ligand saturation, the thermal stability of streptavidin becomes significantly enhanced, with Tm increasing from 75 °C to 112 °C [1]. Structural stability of the streptavidin-biotin complex was tested in the current study using SDS-PAGE. When proteins are treated with a reducing agent and a detergent, i.e. β-mercaptoethanol and SDS, respectively, protein folding is hampered and proteins are linearised into polypeptides. The complex proved to be stable in the presence of both denaturing agents and at high temperature (Figure 1), as it remained intact following an SDS electrophoresis. Since streptavidin does not possess any cysteine residues thus, no disulphide bridges, its structural stability is derived from an extensive hydrogen bond network and salt bridges between the subunits [32]. When bound to biotin, the complex is held together by hydrogen bonds, van der Waals forces and hydrophobic interactions among nonpolar groups including several direct aromatic side-chain contacts [19],[33]. Additionally, the inter-subunit interactions are further enhanced by the interaction of Trp-120 of the adjacent dimer with biotin [1].
Thermodynamic parameters of binding inform us of various forces that drive molecular interactions. Herein, we used the Affinity ITC (TA Instruments) to determine the energetics of the streptavidin and biotin binding interactions well as how the forces involved in complex formation are affected by the reaction temperature. We report that the formation of this complex is a spontaneous binding process, indicated by a negative ΔG at all temperatures tested (Table 1 and Figure 4). It is also observed that ΔG becomes increasingly negative with tighter binding, KD ≥ 10−9 M, as observed between 2 °C and 20 °C (Table 1 and Figure 4). An accurate calculation of the free energy of binding relies on a thorough understanding of forces that stabilise the interaction and most importantly, the interaction of a molecule with the solvent. Aromatic side chains of the tryptophan residues in streptavidin (6 per monomer) are major contributors to the hydrophobic interactions and van der Waals forces responsible for the tight binding of biotin to streptavidin. Studies have been conducted to prove that conformational changes are induced upon biotin binding, resulting in decreased accessibility of tryptophan residues in streptavidin [1],[3],[7]. Based on the polarisation state of groups involved in binding, an entropy-driven binding interaction at a certain temperature may change to an enthalpy-driven process at another temperature [34]. At higher temperatures, 30–40 °C, we observed an enthalpically-driven interaction, relative to the entropically-driven interaction at lower temperatures, and attributed this to the possible conformational changes that occur upon complex formation and leading to decreased solvent accessibility of streptavidin tryptophan residues (Trp-79, Trp-92, Trp-108 and Trp-120) lining the biotin binding site. Other hydrophobic interactions that are implicated in the binding include Leu-25, Val-47 and Leu-110 which could also reduce the solvent accessibility of the neighboring tryptophan residues. Enthalpically favorable interactions at higher temperatures are also due to polar interactions established by Ser-45, Asn-23, Ser-27 and Tyr-43 in the biotin binding pocket.
The most diverse thermodynamic parameter of binding, ΔCp, is regarded as energy fluctuations during a binding process. This variable is defined by several factors including protein conformational entropy, hydrogen bonding and hydrophobic groups [34]. The negative ΔCp (Figure 3) alludes to the polar solvation of the interaction that corresponds to a decreasing ΔH with increasing reaction temperature. Our ΔCp ∼ −459.9 cal/mol K, calculated as the slope of ΔH vs temperature, between 2–30 °C, is consistent with ΔCp ∼ −461 cal/mol K reported by Swamy [30]. However, others have reported ΔCp of −345 cal/mol K [33] whilst a study of a Ser45Ala mutant yielded the least favourable ΔH, most favourable ΔS and ΔCp ∼ −223 cal/mol K as a result of perturbation of hydrogen bonding to the ureido nitrogen of biotin [6].
Using molecular dynamics simulations, Liu et al. [19], studied the cooperativity of streptavidin active site mutants and showed that enthalpic contributions were mainly from the alternations in solvation free energy. The unfavourable entropy observed in the current study (Table 1 and Figure 4) is suggestive of thermally-induced conformational changes occurring between 30 °C and 40 °C, resulting in moderate streptavidin affinity with KD 10−4 ≤ 10−5 M, towards biotin at the higher reaction temperatures. In a study by Qureshi et al. [9], looking at the effects of certain mutations on the binding affinities, they reported KD values ranging from 10−6 to 10−11 M. One of their mutants, TM1, yielded a monomeric streptavidin, with a KD ∼10−6 M. The other mutant had KD ∼10−8 M, and it existed in both monomeric and tetrameric forms. Our purchased core-streptavidin appears to exist in both forms too (Figure 1) thus, the discrepancy in KD values when compared to the values reported by Green [4].
Spectroscopic studies of the streptavidin-biotin complex formation by Green, [20], concluded that hydrophobic interactions made a large contribution to the free energy of binding. Contrary to other types of non-covalent reactions that result in a decrease in both ΔS and heat content [35],[36], this contribution is accompanied by a large increase in ΔS and only a small change in heat content. Similarly, our study reports on entropy-driven streptavidin-biotin interactions, i.e. hydrophobic in nature, at reaction temperatures between 15 °C and 25 °C.
The binding mechanism of biotin to streptavidin was further characterised by determining the molar binding ratio of ligand to the protein, i.e. stoichiometry (n). We report a stoichiometry of 0.94 to 0.98 between 15 °C and 25 °C (Table 1). These values are consistent with those determined at ambient temperature by both Yumura et al. [37], when studying functional mutations of core streptavidin (n = 0.8–1.2), and Hyre [33] from the streptavidin-biotin cooperative hydrogen bond interactions study. An increase in reaction temperature led to an almost two-fold increase or more in n, notably; 1.59 to 3.41 between 30 °C to 40 °C (Table 1). At lower reaction temperatures, significantly higher molar binding ratios were recorded, i.e. n ∼ 2.74 to 5.76 between 2 °C and 10 °C. Additionally, depending on the protein conformation, a various number of biotin molecules can bind in the binding pocket of streptavidin. The binding pocket closes as the flexible loop becomes immobilised following biotin-binding [9]. Therefore, at either lower or higher temperatures, the resultant structural conformation (monomer, dimer or tetramer) allows for one or more biotin molecules to bind as shown by Sano and Cantor 1990 [38]. It is thus possible that at these different temperatures the streptavidin assumes distinct conformation(s) (monomeric, dimeric or tetrameric) which invariably affect the biotin binding stoichiometry.
This work reports on how the reaction temperature influences the binding thermodynamics of the streptavidin-biotin interaction. We report that the streptavidin-biotin complex formation is a spontaneous binding process, demonstrated by a negative ΔG at all tested temperatures. Change in solvation state of streptavidin at higher temperatures results in decreased accessibility of tryptophan residues on the protein thus, moderate binding affinity, KD 10−4 ≤ 10−5 M. Furthermore, an enthalpy-driven interaction at higher temperatures, 30–40 °C, becomes entropy-driven at lower temperatures. We report a stoichiometry of 0.94 to 0.98 between 15 °C and 25 °C and ΔCp ∼ −459.9 cal/mol K.
[1] |
H. B. Seed, I. M. Idriss, Simplified procedure for evaluating soil liquefaction potential, J. Soil Mech. Found. Div., 97 (1971), 1249-1273. doi: 10.1061/JSFEAQ.0001662
![]() |
[2] | H. B. Seed, I. M. Idriss, Evaluation of liquefaction potential sand deposits based on observation of performance in previous earthquakes, in Proceedings of ASCE national convention (MO), 481-544. |
[3] |
P. K. Robertson, C. Wride, Evaluating cyclic liquefaction potential using the cone penetration test, Can. Geotech. J., 35 (1998), 442-459. doi: 10.1139/t98-017
![]() |
[4] |
T. L. Youd, I. M. Idriss, Liquefaction resistance of soils: summary report from the 1996 NCEER and 1998 NCEER/NSF workshops on evaluation of liquefaction resistance of soils, J. Geotech. Geoenviron., 127 (2001), 297-313. doi: 10.1061/(ASCE)1090-0241(2001)127:4(297)
![]() |
[5] |
C. H. Juang, H. Yuan, D. H. Lee, P. S. Lin, Simplified cone penetration test-based method for evaluating liquefaction resistance of soils, J. Geotech. Geoenviron., 129 (2003), 66-80. doi: 10.1061/(ASCE)1090-0241(2003)129:1(66)
![]() |
[6] |
R. Moss, R. B. Seed, R. E. Kayen, J. P. Stewart, A. Der Kiureghian, K. O. Cetin, CPT-based probabilistic and deterministic assessment of in situ seismic soil liquefaction potential, J. Geotech. Geoenviron., 132 (2006), 1032-1051. doi: 10.1061/(ASCE)1090-0241(2006)132:8(1032)
![]() |
[7] |
I. Idriss, R. Boulanger, Semi-empirical procedures for evaluating liquefaction potential during earthquakes, Soil Dyn. Earthquake Eng., 26 (2006), 115-130. doi: 10.1016/j.soildyn.2004.11.023
![]() |
[8] |
V. Kohestani, M. Hassanlourad, A. Ardakani, Evaluation of liquefaction potential based on CPT data using random forest, Nat. Hazards, 79 (2015), 1079-1089. doi: 10.1007/s11069-015-1893-5
![]() |
[9] |
X. Xue, X. Yang, Application of the adaptive neuro-fuzzy inference system for prediction of soil liquefaction, Nat. Hazards, 67 (2013), 901-917. doi: 10.1007/s11069-013-0615-0
![]() |
[10] |
P. Samui, Seismic liquefaction potential assessment by using relevance vector machine, Earthquake Eng. Eng. Vib., 6 (2007), 331-336. doi: 10.1007/s11803-007-0766-7
![]() |
[11] |
A. T. Goh, Neural-network modeling of CPT seismic liquefaction data, J. Geotech. Eng., 122 (1996), 70-73. doi: 10.1061/(ASCE)0733-9410(1996)122:1(70)
![]() |
[12] |
P. Samui, T. Sitharam, M. Contadakis, Machine learning modelling for predicting soil liquefaction susceptibility, Nat. Hazards Earth Syst. Sci., 11 (2011), 1-9. doi: 10.5194/nhess-11-1-2011
![]() |
[13] |
A. H. Gandomi, A. H. Alavi, A new multi-gene genetic programming approach to non-linear system modeling. Part Ⅱ: geotechnical and earthquake engineering problems, Neural Comput. Appl., 21 (2012), 189-201. doi: 10.1007/s00521-011-0735-y
![]() |
[14] |
P. K. Muduli, S. K. Das, CPT-based seismic liquefaction potential evaluation using multi-gene genetic programming approach, Indian Geotech. J., 44 (2014), 86-93. doi: 10.1007/s40098-013-0048-4
![]() |
[15] |
P. K. Muduli, S. K. Das, S. Bhattacharya, CPT-based probabilistic evaluation of seismic soil liquefaction potential using multi-gene genetic programming, Georisk: Assess. Manage. Risk Eng. Syst. Geohazards, 8 (2014), 14-28. doi: 10.1080/17499518.2013.845720
![]() |
[16] |
P. K. Muduli, S. K. Das, First-order reliability method for probabilistic evaluation of liquefaction potential of soil using genetic programming, Int. J. Geomech., 15 (2015), 04014052. doi: 10.1061/(ASCE)GM.1943-5622.0000377
![]() |
[17] |
A. T. Goh, S. Goh, Support vector machines: their use in geotechnical engineering as illustrated using seismic liquefaction data, Comput. Geotech., 34 (2007), 410-421. doi: 10.1016/j.compgeo.2007.06.001
![]() |
[18] |
T. Oommen, L. G. Baise, R. Vogel, Validation and application of empirical liquefaction models, J. Geotech. Geoenviron., 136 (2010), 1618-1633. doi: 10.1061/(ASCE)GT.1943-5606.0000395
![]() |
[19] |
M. Pal, Support vector machines-based modelling of seismic liquefaction potential, Int. J. Numer. Anal. Methods Geomech., 30 (2006), 983-996. doi: 10.1002/nag.509
![]() |
[20] | J. Pearl, Probabilistic reasoning in intelligent systems: Representation & reasoning, Morgan Kaufmann Publishers, San Mateo, 1988. |
[21] |
M. Ahmad, X. W. Tang, J. N. Qiu, W. J. Gu, F. Ahmad, A hybrid approach for evaluating CPT-based seismic soil liquefaction potential using Bayesian belief networks, J. Cent. South Univ., 27 (2020), 500-516. doi: 10.1007/s11771-020-4312-3
![]() |
[22] |
M. Ahmad, X. W. Tang, J. N. Qiu, F. Ahmad, W. J. Gu, A step forward towards a comprehensive framework for assessing liquefaction land damage vulnerability: Exploration from historical data, Front. Struct. Civ. Eng., 14 (2020), 1476-1491. doi: 10.1007/s11709-020-0670-z
![]() |
[23] |
M. Ahmad, X. W. Tang, J. N. Qiu, F. Ahmad, Evaluating Seismic Soil Liquefaction Potential Using Bayesian Belief Network and C4. 5 Decision Tree Approaches, Appl. Sci., 9 (2019), 4226. doi: 10.3390/app9204226
![]() |
[24] | A. P. Sage, Methodology for Large-Scale Systems, New York, 1977. |
[25] | J. N. Warfield, Developing interconnection matrices in structural modeling, IEEE Trans. Syst. Man Cybern., 4 (1974), 81-87. |
[26] |
V. Ravi, R. Shankar, Analysis of interactions among the barriers of reverse logistics, Technol. Forecast. Soc. Change., 72 (2005), 1011-1029. doi: 10.1016/j.techfore.2004.07.002
![]() |
[27] |
R. Shankar, R. Narain, A. Agarwal, An interpretive structural modeling of knowledge management in engineering industries, J. Adv. Manag. Res., 1 (2003), 28-40. doi: 10.1108/97279810380000356
![]() |
[28] |
T. Raj, R. Attri, Identification and modelling of barriers in the implementation of TQM, Int. J. Product. Qual., 8 (2011), 153-179. doi: 10.1504/IJPQM.2011.041844
![]() |
[29] |
M. Ahmad, X. W. Tang, J. N. Qiu, F. Ahmad, Evaluation of liquefaction-induced lateral displacement using Bayesian belief networks, Front. Struct. Civ. Eng., 15 (2021), 80-98, doi: 10.1007/s11709-021-0682-3
![]() |
[30] | M. Ahmad, X. W. Tang, F. Ahmad, M. Hadzima-Nyarko, A. Nawaz, A. Farooq, Elucidation of Seismic Soil Liquefaction Significant Factors. in Earthquakes-From Tectonics to Buildings, 2021. |
[31] |
K. Masmoudi, L. Abid, A. Masmoudi, Credit risk modeling using Bayesian network with a latent variable, Expert Syst. Appl., 127 (2019), 157-166. doi: 10.1016/j.eswa.2019.03.014
![]() |
[32] | A. Castelletti, R. Soncini-Sessa, Bayesian Networks and participatory modelling in water resource management, Environ. Model. Software, 22 (2007), 1075-1088. |
[33] |
A. Ghribi, A. Masmoudi, A compound poisson model for learning discrete Bayesian networks, Acta Math. Sci., 33 (2013), 1767-1784. doi: 10.1016/S0252-9602(13)60122-8
![]() |
[34] |
S. A. Joseph, B. J. Adams, B. McCabe, Methodology for Bayesian belief network development to facilitate compliance with water quality regulations, J. Infrastruct. Syst., 16 (2010), 58-65. doi: 10.1061/(ASCE)1076-0342(2010)16:1(58)
![]() |
[35] |
S. Nadkarni, P. P. Shenoy, A Bayesian network approach to making inferences in causal maps, Eur. J. Oper. Res., 128 (2001), 479-498. doi: 10.1016/S0377-2217(99)00368-9
![]() |
[36] |
G. Kabir, S. Tesfamariam, A. Francisque, R. Sadiq, Evaluating risk of water mains failure using a Bayesian belief network model, Eur. J. Oper. Res., 240 (2015), 220-234. doi: 10.1016/j.ejor.2014.06.033
![]() |
[37] | S. Tesfamariam, Z. Liu, Seismic risk analysis using Bayesian belief networks, in Handbook of seismic risk analysis and management of civil infrastructure systems, 2013,175-208. |
[38] | R. Boulanger, I. Idriss, CPT and SPT based liquefaction triggering procedures, 2014. |
[39] | C. Okoli, K. Schabram, A guide to conducting a systematic literature review of information systems research, Inf. Syst., 10 (2010), 1-51. |
[40] |
D. Tranfield, D. Denyer, P. Smart, Towards a methodology for developing evidence-informed management knowledge by means of systematic review, Br. J. Manage., 14 (2003), 207-222. doi: 10.1111/1467-8551.00375
![]() |
[41] |
M. Ahmad, X. W. Tang, J. N. Qiu, F. Ahmad, Interpretive Structural Modeling and MICMAC Analysis for Identifying and Benchmarking Significant Factors of Seismic Soil Liquefaction, Appl. Sci., 9 (2019), 233. doi: 10.3390/app9020233
![]() |
[42] |
L. Zhang, Predicting seismic liquefaction potential of sands by optimum seeking method, Soil Dyn. Earthquake Eng., 17 (1998), 219-226. doi: 10.1016/S0267-7261(98)00004-9
![]() |
[43] |
J. L. Hu, X. W. Tang, J. N. Qiu, A Bayesian network approach for predicting seismic liquefaction based on interpretive structural modeling, Georisk: Assess. Manage. Risk Eng. Syst. Geohazards, 9 (2015), 200-217. doi: 10.1080/17499518.2015.1076570
![]() |
[44] | D. W. Hosmer, S. Lemeshow, Applied Logistic Regression, 2nd edition, John Wiley & Sons, New York, 2000. |
[45] | V. N. Vapnik, The nature of statistical learning, Theory, 1995. |
[46] | L. Breiman, Random forests, Mach. Learn., 45 (2001), 5-32. |
[47] | G. John, P. Langley, Estimating Continuous Distributions in Bayesian Classifiers, in proceedings of the Eleventh Conference on Uncertainty in Artificial Intelligence, 1995. |
[48] |
S. K. Das, R. Mohanty, M. Mohanty, M. Mahamaya, Multi-objective feature selection (MOFS) algorithms for prediction of liquefaction susceptibility of soil based on in situ test methods, Nat. Hazards, 103 (2020), 2371-2393. doi: 10.1007/s11069-020-04089-3
![]() |
[49] | M. Kubat, S. Matwin, Addressing the curse of imbalanced training sets: one-sided selection, in Proceedings of the Fourteenth International Conference on Machine Learning, (1997), 179-186. |
[50] | B. Yuan, W. Liu, A measure oriented training scheme for imbalanced classification problems, in Proceedings of Pacific-Asia Conference on Knowledge Discovery and Data Mining, (2001), 293-303. |
[51] | Y. Sun, M. S. Kamel, Y. Wang, Boosting for learning multiple classes with imbalanced class distribution, in Proceedings of Sixth international conference on data mining (ICDM06), (2006), 592-602. |
1. | Shaoying Xu, Yan Sun, Xiaoyan Dong, Design of Gallic Acid–Glutamine Conjugate and Chemical Implications for Its Potency Against Alzheimer’s Amyloid-β Fibrillogenesis, 2022, 33, 1043-1802, 677, 10.1021/acs.bioconjchem.2c00073 | |
2. | Jing Han, Pengfei Ma, Imran Mahmood Khan, Yin Zhang, Zhouping Wang, Study of binding mechanism of aptamer to kanamycin and the development of fluorescent aptasensor in milk detection, 2023, 00399140, 124530, 10.1016/j.talanta.2023.124530 | |
3. | Tao Xiong, Yingchao Chen, Qiang Peng, Mingle Li, Sheng Lu, Xiaoqiang Chen, Jiangli Fan, Lei Wang, Xiaojun Peng, Pyrazolone-Protein Interaction Enables Long-Term Retention Staining and Facile Artificial Biorecognition on Cell Membranes, 2024, 146, 0002-7863, 24158, 10.1021/jacs.4c08987 | |
4. | Hongpeng Wang, Alexey Tarabarov, Qingqing Rao, Xing Wang, Yiyu Qi, Yongqi Wang, Zhuqian Xiao, Changjiang Lv, Jiayao Yang, Jun Huang, Shengxiang Yang, Nanobody immobilization on magnetic nanoparticles via monomeric streptavidin-biotin specific interaction for aflatoxin adsorption, 2024, 7, 2522-0128, 10.1007/s42114-024-00893-8 | |
5. | B Wu, L Li, D Wang, Y Xiong, W Lu, B-017 The Effect of Temperature on the Performance of Streptavidin-coated Paramagnetic Particles in Immunoassay Applications, 2023, 69, 0009-9147, 10.1093/clinchem/hvad097.360 | |
6. | Xiaotong Meng, Danny O'Hare, Sylvain Ladame, Surface immobilization strategies for the development of electrochemical nucleic acid sensors, 2023, 237, 09565663, 115440, 10.1016/j.bios.2023.115440 | |
7. | Woo-Ri Shin, Gna Ahn, Jin-Pyo Lee, In-Hwan Oh, Ji-Young Ahn, Yang-Hoon Kim, Soryong Chae, Recent advances in engineering aptamer-based sensing and recovery of heavy metals and rare earth elements for environmental sustainability, 2023, 472, 13858947, 144742, 10.1016/j.cej.2023.144742 | |
8. | Dipak Koirala, Forrest Dalbec, Jeremy May, Kailash Hamal, Peter B. Allen, I. Francis Cheng, Biosensing with Polymerase Chain Reaction-Stable DNA-Functionalized Magnetically Susceptible Carbon–Iron Microparticles, 2023, 95, 0003-2700, 16631, 10.1021/acs.analchem.3c02978 | |
9. | Mladen Simonović, Sanja Ostojić, Darko Micić, Petar Djurdjić, Thorsten Mix, Čeda Kuzmanović, Drago Jelovac, Biotin as a structural component in the detection of small model antigens in BLA-S-ELISA, 2024, 1478-6419, 1, 10.1080/14786419.2024.2318785 | |
10. | 李长青 Li Changqing, 陆道礼 Lu Daoli, 陈斌 Chen Bin, 基于连续光谱分析的滴定系统及其应用研究, 2024, 61, 1006-4125, 2330001, 10.3788/LOP240760 | |
11. | Qinglin Xia, Mo Zhou, Kai Jiao, Bin Li, Linjie Guo, Lihua Wang, Jiang Li, Recent Advances in DNA‐Templated Protein Patterning, 2025, 2366-9608, 10.1002/smtd.202401835 |
Temp (°C) | KD (M) | n | ΔG (kcal/mol) | ΔH (kcal/mol) | ΔS (cal/mol K) | TΔS (kcal/mol) |
2 | 1.00E-09 | 3.14 | −11.33 | 3.67 | 0.05 | 15.01 |
6 | 1.00E-09 | 5.76 | −11.49 | 2.98 | 0.05 | 14.47 |
10 | 1.00E-09 | 2.74 | −11.66 | 2.10 | 0.04 | 13.76 |
15 | 1.00E-09 | 0.94 | −11.86 | −2.57 | 0.03 | 9.29 |
20 | 1.00E-09 | 0.95 | −12.07 | −3.72 | 0.02 | 8.35 |
25 | 3.14E-07 | 0.98 | −8.87 | −5.63 | 0.01 | 3.24 |
30 | 1.79E-05 | 1.92 | −6.58 | −8.80 | −0.007 | −2.21 |
35 | 1.74E-04 | 1.59 | −5.30 | −23.90 | −0.06 | −18.60 |
40 | 2.88E-04 | 3.41 | −5.08 | −23.90 | −0.06 | −18.82 |
Temp (°C) | KD (M) | n | ΔG (kcal/mol) | ΔH (kcal/mol) | ΔS (cal/mol K) | TΔS (kcal/mol) |
2 | 1.00E-09 | 3.14 | −11.33 | 3.67 | 0.05 | 15.01 |
6 | 1.00E-09 | 5.76 | −11.49 | 2.98 | 0.05 | 14.47 |
10 | 1.00E-09 | 2.74 | −11.66 | 2.10 | 0.04 | 13.76 |
15 | 1.00E-09 | 0.94 | −11.86 | −2.57 | 0.03 | 9.29 |
20 | 1.00E-09 | 0.95 | −12.07 | −3.72 | 0.02 | 8.35 |
25 | 3.14E-07 | 0.98 | −8.87 | −5.63 | 0.01 | 3.24 |
30 | 1.79E-05 | 1.92 | −6.58 | −8.80 | −0.007 | −2.21 |
35 | 1.74E-04 | 1.59 | −5.30 | −23.90 | −0.06 | −18.60 |
40 | 2.88E-04 | 3.41 | −5.08 | −23.90 | −0.06 | −18.82 |