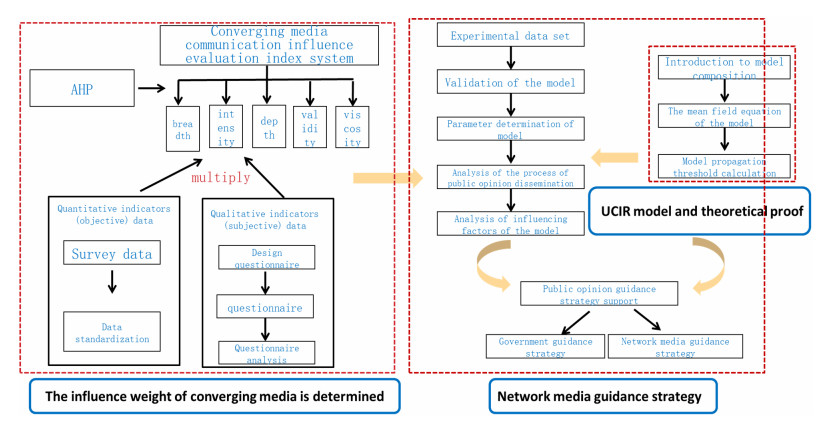
In the 5G era, media convergence and technological updates lead to tremendous changes in the dissemination of public opinion information. The guidance of public opinion in the converged media environment is a new research topic. Users participate in the discussion of different media, and flow in and out from different media, which gradually generate a new complex dynamics model of the dissemination of public opinion information. An index system for evaluating the influence of converged media communication that combines the advantages of traditional media and new media is constructed. We use AHP to determine the index weights in the evaluation system and conduct consistency tests. The final weight of each media is determined through the combination of subjective and objective methods, which provides data supports for later determining the participation of various media in the dissemination process of public opinion information. Based on the SIR model, a UCIR (unknown-contact-infected-recovered) dynamic model is established. The simulation results show that the root mean square error (RMSE) of the UCIR model is 31.6% lower than that of the SIR model. Finally, by fixing the key parameters α, β, θ, ε, p, q in the UCIR model, and changing only one of them, we studied the effect of the transition probability between different states on the process of public opinion information transmission, and then proposed corresponding guidance. In addition, from the two perspectives of network media and government, the countermeasures and suggestions for the guidance of public opinion are proposed. We try to solve the problems of humanities and social sciences with the method of natural science. These research results can provide theoretical and methodological support for people to understand the law of public opinion information transmission and establish a guiding mechanism for public opinion information transmission.
Citation: Jinbao Song, Xiaoya Zhu. Research on public opinion guidance of converging media based on AHP and transmission dynamics[J]. Mathematical Biosciences and Engineering, 2021, 18(5): 6857-6886. doi: 10.3934/mbe.2021341
[1] | Fulian Yin, Jinxia Wang, Xinyi Jiang, Yanjing Huang, Qianyi Yang, Jianhong Wu . Modeling and analyzing an opinion network dynamics considering the environmental factor. Mathematical Biosciences and Engineering, 2023, 20(9): 16866-16885. doi: 10.3934/mbe.2023752 |
[2] | Wei Hong, Yiting Gu, Linhai Wu, Xujin Pu . Impact of online public opinion regarding the Japanese nuclear wastewater incident on stock market based on the SOR model. Mathematical Biosciences and Engineering, 2023, 20(5): 9305-9326. doi: 10.3934/mbe.2023408 |
[3] | Qiao Xiang, Tianhong Huang, Qin Zhang, Yufeng Li, Amr Tolba, Isack Bulugu . A novel sentiment analysis method based on multi-scale deep learning. Mathematical Biosciences and Engineering, 2023, 20(5): 8766-8781. doi: 10.3934/mbe.2023385 |
[4] | Elodie Yedomonhan, Chénangnon Frédéric Tovissodé, Romain Glèlè Kakaï . Modeling the effects of Prophylactic behaviors on the spread of SARS-CoV-2 in West Africa. Mathematical Biosciences and Engineering, 2023, 20(7): 12955-12989. doi: 10.3934/mbe.2023578 |
[5] | Fulian Yin, Xueying Shao, Jianhong Wu . Nearcasting forwarding behaviors and information propagation in Chinese Sina-Microblog. Mathematical Biosciences and Engineering, 2019, 16(5): 5380-5394. doi: 10.3934/mbe.2019268 |
[6] | Fulian Yin, Xinyi Tang, Tongyu Liang, Yanjing Huang, Jianhong Wu . External intervention model with direct and indirect propagation behaviors on social media platforms. Mathematical Biosciences and Engineering, 2022, 19(11): 11380-11398. doi: 10.3934/mbe.2022530 |
[7] | Fulian Yin, Jiahui Lv, Xiaojian Zhang, Xinyu Xia, Jianhong Wu . COVID-19 information propagation dynamics in the Chinese Sina-microblog. Mathematical Biosciences and Engineering, 2020, 17(3): 2676-2692. doi: 10.3934/mbe.2020146 |
[8] | Wei Liu, Yi Huang, Yue Sun, Changlong Yu . Research on design elements of household medical products for rhinitis based on AHP. Mathematical Biosciences and Engineering, 2023, 20(5): 9003-9017. doi: 10.3934/mbe.2023395 |
[9] | Arvind Kumar Misra, Rajanish Kumar Rai, Yasuhiro Takeuchi . Modeling the control of infectious diseases: Effects of TV and social media advertisements. Mathematical Biosciences and Engineering, 2018, 15(6): 1315-1343. doi: 10.3934/mbe.2018061 |
[10] | Dongmei Li, Bing Chai, Weihua Liu, Panpan Wen, Ruixue Zhang . Qualitative analysis of a class of SISM epidemic model influenced by media publicity. Mathematical Biosciences and Engineering, 2020, 17(5): 5727-5751. doi: 10.3934/mbe.2020308 |
In the 5G era, media convergence and technological updates lead to tremendous changes in the dissemination of public opinion information. The guidance of public opinion in the converged media environment is a new research topic. Users participate in the discussion of different media, and flow in and out from different media, which gradually generate a new complex dynamics model of the dissemination of public opinion information. An index system for evaluating the influence of converged media communication that combines the advantages of traditional media and new media is constructed. We use AHP to determine the index weights in the evaluation system and conduct consistency tests. The final weight of each media is determined through the combination of subjective and objective methods, which provides data supports for later determining the participation of various media in the dissemination process of public opinion information. Based on the SIR model, a UCIR (unknown-contact-infected-recovered) dynamic model is established. The simulation results show that the root mean square error (RMSE) of the UCIR model is 31.6% lower than that of the SIR model. Finally, by fixing the key parameters α, β, θ, ε, p, q in the UCIR model, and changing only one of them, we studied the effect of the transition probability between different states on the process of public opinion information transmission, and then proposed corresponding guidance. In addition, from the two perspectives of network media and government, the countermeasures and suggestions for the guidance of public opinion are proposed. We try to solve the problems of humanities and social sciences with the method of natural science. These research results can provide theoretical and methodological support for people to understand the law of public opinion information transmission and establish a guiding mechanism for public opinion information transmission.
It is interesting and definitely worth investigating to solve the problems of humanities and social sciences with the method of natural science. The gradual formation of the converged media environment has a great influence on the transmission and guidance of public opinion. Most of the existing researches on public opinion guidance are focused on single media, while there are few researches on converged media. Especially, the cross-influence of different media on public opinion guidance, which makes the work of public opinion guidance more complicated. The research on public opinion guidance of converging media based on AHP and transmission dynamics is worthy to solve. The classic transmission dynamics model is extended to the transmission environment of the converged media. It is of great significance to study the transmission law of public opinion and control the transmission of public opinion in the converged media environment. Based on the above analysis, the research on public opinion guidance of converging media based on AHP and transmission dynamics is worthy to solve.
The advancement of Internet technology has changed the way people communicate and share information: various social software and portals are emerging one after another, such as Facebook, Twitter, Instagram commonly used in foreign countries, and WeChat, Weibo, short video frequently used in China. These media platforms have become an important part of people's lives. People have gradually changed from being a passive receiver of public opinion to a creator of public opinion in the traditional media era, and they actively understand and participate in the discussion of public opinion events. In this way, people with similar opinions can easily gather together.
The current development of information technology has made the boundary between traditional media and new media more and more blurred. Therefore, the convergence model of traditional media and new media is constantly being explored. In the environment of converged media, the transmission of information has become more rapid, and the media's reporting of public opinion has become more comprehensive and hierarchical, which is also more conducive to the transmission of public opinion. Therefore, correct public opinion transmission guidance is needed. This paper uses the science and engineering method to solve the humanities and social science problems. The current research hotspot is to solve the core problems through the construction of the model, and provide corresponding public opinion guidance strategies.
The research foundation of this paper has been extended to a communication environment such as converged media, and the urgent need to promote effective government intervention in the transmission of public opinion is considered. A public opinion transmission guidance research model (UCIR) based on the converged media is proposed, and the model is closer to actual transmission. In this paper, the balance and stability of the model have been proved, and the process of public opinion transmission has been analyzed. Finally, countermeasures and suggestions for public opinion guidance are given from the perspectives of online media and the government.
Foreign research on converged media started earlier, and the research results are more mature than domestic. For the research on the concept of converged media, Niehoff believes that converged media is the development trend of multiple media platforms showing multi-functional integration [1]. Andrew defines converged media as a strategic, operational, and cultural alliance between audio, video, text and other media forms [2]. Gordon summed up the converged media as the integration of ownership, strategy, structure, information collection, and news expression [3].
The earliest domestic research on integrated media was in 2002, a little later than foreign research. For the research on the concept of converging media, domestic scholars often adopt the concept of media convergence, which is directly translated from Media Convergence. Although domestic scholars have some understanding of the concept of converging media. In essence, converging media means combining the advantages of traditional media and new media. In the research on converged media and public opinion, Liao Feng studied the development status and characteristics of converged media in his article, and based on the occurrence mechanism of public emergencies, strategies and suggestions to effectively guide public opinion were put forward [4].
Many scholars have found that the process of information transmission is very similar to the development process of infectious diseases. Therefore, the establishment of information transmission models based on infectious disease models has become one of the focuses of domestic and foreign scholars. The earliest SIR model was proposed by Kermack and McKendrick in 1927 when they were studying the Black Death epidemic in London [5]. The SIR model divides the population into three states: Susceptible refers to those who are not infected but are easily infected after contact with an infected person. Infective refers to people who have been infected and can be transmitted to S state. Removed refers to people who have recovered from illness and have immunity. After that, scholars improved the SIR model according to different research environments, and derived multiple infectious disease models such as the SI (Susceptible-Infective) model and the SIS (Susceptible-Infective-Susceptible) model.
In recent studies, most scholars have focused on improving the dynamic model of information transmission. In 2012, Zhao [6] et al. considered the feature that users would forget information in their proposed SIHR rumor propagation model. In 2015, Ding [7] et al. improved the traditional SIR model to the SCIR model, adding a new node state-contact state C. Lin [8] et al. studied the SEIR network public opinion propagation model with population dynamics and saturated growth rate. In 2016, You [9] et al. introduced foreign users into the SIR model to better predict the dissemination trend of public opinion. Based on the SIR model, Liu [10] et al. proposed the SHIR model with the hesitation state. In 2018, Rui [11] et al. established the SPIR model and introduced a new state—the potential propagation state (PS). Only when all the infected nodes around are no longer transmissioning information, the nodes in this state will be converted to an unknown state. Ebadizadeh [12] et al. established the ISRC model that can block information transmission, where C is defined as the state of controlling information transmission. Zan [13] studied the propagation characteristics of dual opinion information in the network in the DSIR model. In 2019, Kabir [14] introduced node sensitivity in the SIR model. Each state in the model is again divided into a sensitive state and an insensitive state. Taking the S state as an example, it is divided into an unknown sensitive state SA and an unknown insensitive state SU.
Based on the mean field theory and the influence factors of public opinion, many studies have proposed further improved models [15,16,17,18,19,20,21,22,23]. Yu [24] et al. analyzed the difference between the SEIR model including the latent state E and the SIR model, and the different phenomena of the two models in the information diffusion process when the parameters are changed.
In the actual public opinion transmission process, users may not transmission the public opinion immediately after learning the public opinion. Similarly, users may directly refuse to understand public opinion. Based on this situation, a UCIR (unknown-contact-infected-recovered) dynamic model is established. In the UCIR model, the contact status is introduced to describe the hesitation process of the information transmission process. At the same time, the status conversion of (ⅰ) unknown persons who directly refuse to understand a certain public opinion topic and become immunized, and (ⅱ) some immunized persons are affected by other related derivative public opinion topics and then return to contact status are proposed. Each state increases the probability of entering and leaving, enhancing the dynamics of the UCIR model.
The Analytic Hierarchy Process (AHP) is a comprehensive evaluation method that combines quantitative and qualitative methods to calculate index weight. Its principle [25] is to decompose the problem into different factors according to the understanding of the problem and the goal to be achieved. And according to the relationship between different factors, they are combined at different levels to form a multi-level analysis structure model [26]. Radovanovic [27] presents a decision support model when choosing the most efficient rectification procedure of the optical sight of the long-range rifle. Bobar [28] presents methods of ranking and evaluation of the effectiveness of Social Media (SM). The methodology presented is based on multicircular decision-making using the Fuzzy Analytical Hierarchical Process (fuzzy AHP)-Z number model-Fuzzy Multi-Attributive Border Approximation Area Comparison (fuzzy MABAC), which eliminates the traditional intuitive ratings of PR services. Lyu [29] incorporates the original analytic hierarchy process (AHP) and triangular fuzzy number-based AHP (TFN-AHP) into a geographic information system (GIS) to assess the inundation risk of the metro system in Shenzhen. Duleba [30] originally constructed the methodology of Parsimonious Analytic Hierarchy Process (PAHP) to unburden the evaluators of an AHP survey from the numerous pairwise comparisons caused by the several alternatives in decision problems. Ohta [31] presents Hesitant FAHP (HFAHP) and Intuitionistic FAHP (IFAHP). Choi [32] proposes a method of weight adjustment as a modification of AHP. Lin [33] proposes a heuristic method (Bayesian cosine maximization method (BCCM)) to rank the alternatives in the Analytic Hierarchy Process (AHP) synthesis. Leal [34] presents in detail a simplified method for the application of the analytic hierarchy method (AHP) that aims to calculate the priorities of each alternative against a set of criteria with only n−1 comparisons of n alternatives for each criterion (instead of (n(2)-n)/2 comparisons. Deng [35] proposed a method called D-AHP (AHP method extended by D numbers preference relation) to study the MCDM. Zhang [36] presents an improved OWA-Fuzzy AHP decision model for multi-attribute decision making problem. Abastante [37] presents a new parsimonious AHP methodology: Assigning priorities to many objects by comparing pairwise few reference objects. Chen [38] proposed diversified AHP-tree approach for multiple-criteria supplier selection. Li [39] proposed an improved analytic hierarchy process-back propagation (AHP-BP) neural network algorithm.
The evaluation index is generally an objective evaluation of the influence of media users, media communication and information dissemination. The establishment of the evaluation index system should not only cover the characteristics of all aspects of media influence, but also initially reflect the interrelationship of various indicators of media influence. When the evaluation index system is established, it is necessary to ensure that the established indexes do not overlap as much as possible or the index direction is clear. According to the above related requirements, the following evaluation indicators have been established:
The transmission breadth refers to the degree of media information sharing, the scope of the content covered by the media information, and the area and population that can be affected. The following secondary indicators are included:
● Number of visitors: the number of visitors during the evaluation period.
● Number of information released: the number of information released during the evaluation period.
● Content coverage: the scope of the published content type.
● User coverage: the number of users that the content can reach.
The transmission intensity is used to measure the degree of interaction between people in the process of information transmission. The higher the degree of interaction, the greater the influence of the media. The following secondary indicators are included:
● Number of comments: the number of comments during the evaluation period.
● Number of reposts: the number of reposts during the evaluation period.
● Number of likes: the number of likes during the evaluation period.
The transmission depth refers to users' deep impressions of the media. The following secondary indicators are included:
● Cognitive channel: the information channel that recognizes the media.
● Quality cognition: the user's subjective evaluation of the quality of the content released by the media platform.
● Functional cognition: Under normal circumstances, whether the media can achieve the functions required by the user.
The transmission validity refers to the degree of user satisfaction with the various services provided by the media. The following secondary indicators are included:
● Platform satisfaction: easy access through different devices.
● Content satisfaction: the published content is fresh and diverse.
● Service satisfaction: Media platforms can not only communicate with others, but also satisfy self-expression.
Transmission viscosity refers to the obvious preference behavior of users. The following secondary indicators are included:
● User viscosity: how often users use the media and how long each time they use it.
● Recommendation ratio: the likelihood of users recommending to others and their recommendation behavior.
● Intention to use: reasons for users to use media.
The relevant indicators are decomposed into several levels from top to bottom according to different attributes, as shown in Figure 2.
The level of importance | Meaning |
1 | Equally important |
3 | Slightly important |
5 | Obviously important |
7 | Very important |
9 | Extremely important |
2, 4, 6, 8 | ………… |
In this round of analytic hierarchy process, a total of 10 experts who are responsible for relevant operations on various media platforms were invited to score the indicator system. The first-level and second-level indicators of the 10 questionnaires were compared in pairs according to the scores of experts. The judgment matrix was constructed, and a total of 60 judgment matrices were obtained. The index weight is calculated by the characteristic root method and checked for consistency. The following is the calculation process of an expert's first-level indicator questionnaire result data as an example:
Step 1: The construction of the judgment matrix:
A=[11/2433217551/41/711/21/31/31/52111/31/5311] |
Step 2: The calculation of the product of elements in each row:
Mi=n∏j=1aij(j=1,2Λ⋯,n) | (1) |
The results: M1 = 18, M2 = 350, M3 = 1/168, M4 = 2/15, M5 = 1/5.
Step 3: The calculation of the nth root of Mi in each row:
¯Wi=n√Mi(i=1,2Λ⋯,n) | (2) |
¯W1=1.783,¯W2=3.227,¯W3=0.359,¯W4=0.668,¯W5=0.725 |
Step 4: Normalize W to calculate the index weight:
Wi=¯Win∑i=1¯Wi | (3) |
W1 = 0.264, W2 = 0.477, W3 = 0.053, W4 = 0.099, W5 = 0.107
Step 5: The calculation of the consistency index CI:
CI=λmax−nn−1=0.018 | (4) |
Step 6: The calculation of the test coefficient CR:
CR=CIRI=0.016 | (5) |
Generally speaking, if CR < 0.1, the judgment matrix is considered to have satisfactory consistency. The matrix CR calculated in this paper is less than 0.1, so it is proved that the matrix passes the consistency test.
According to the above method steps, 60 judgment matrices are calculated, and the consistency test is performed through the analytic hierarchy process. The results are shown in Table 2.
First-level indicator | The weight of first-level indicators | Secondary indicators | The weight of secondary indicators | Combination weight |
Transmission breadth | 0.158 | Number of visitors | 0.178 | 0.028 |
Number of information released | 0.295 | 0.047 | ||
Content coverage | 0.273 | 0.043 | ||
User coverage | 0.254 | 0.04 | ||
Transmission intensity | 0.35 | Number of comments | 0.231 | 0.081 |
Number of reposts | 0.546 | 0.191 | ||
Number of likes | 0.223 | 0.078 | ||
Transmission depth | 0.162 | Cognitive channel | 0.268 | 0.043 |
Quality cognition | 0.521 | 0.085 | ||
Functional cognition | 0.211 | 0.034 | ||
Transmission validity | 0.172 | Platform satisfaction | 0.198 | 0.034 |
Content satisfaction | 0.546 | 0.094 | ||
Service satisfaction | 0.256 | 0.044 | ||
Transmission viscosity | 0.158 | User viscosity | 0.182 | 0.028 |
Recommendation ratio | 0.541 | 0.085 | ||
Intention to use | 0.277 | 0.045 |
The final media weight evaluation system includes two parts: quantitative indicators and qualitative indicators. Quantitative indicators are quantified based on intuitive data, which can evaluate the influence of media influence on the transmission and intensity of the media, such as the number of visitors, the number of comments, the number of reposts and so on. Qualitative indicators are still in the form of questionnaires, divided into five levels. 1-disagree, 2-little disagree, 3-generally agree, 4-relatively agree, 5-strongly agree. Each level represents 20, 40, 60, 80, and 100 points to quantify the index. The rest of the data is obtained through web crawlers, APIs and market research data. In addition, it needs to be clarified that because traditional media such as TV and radio may have slightly different evaluation dimensions from new media, the traditional media data here comes from related clients and websites. The final result obtained by combining the index score with the determined index weight is shown in Table 3.
Indicator | Short video | TV | radio | newspapers | ||
Number of visitors | 1.37 | 2.8 | 0.97 | 0.6 | 0.09 | 0.04 |
Number of information released | 2.44 | 4.7 | 1.97 | 0.43 | 0.18 | 0.08 |
Content coverage | 1.26 | 2.63 | 1.34 | 3.82 | 3.8 | 4.23 |
User coverage | 3.33 | 4 | 3.06 | 3.61 | 3.78 | 2.94 |
Transmission breadth | 8.4 | 14.13 | 7.34 | 8.46 | 7.85 | 7.29 |
Number of comments | 5.86 | 8.1 | 3.58 | 0.35 | 0.37 | 0.67 |
Number of reposts | 9.51 | 19.1 | 14.39 | 1.05 | 1.95 | 1.03 |
Number of likes | 5.61 | 7.8 | 4.6 | 0.54 | 0.75 | 0.79 |
Transmission intensity | 20.98 | 35 | 22.57 | 1.94 | 3.07 | 2.49 |
Cognitive channel | 3.18 | 3.53 | 2.93 | 3.01 | 2.28 | 2.16 |
Quality cognition | 6.34 | 6.06 | 4.87 | 5.84 | 4.69 | 4.69 |
Functional cognition | 2.28 | 2.79 | 1.93 | 1.95 | 1.56 | 1.49 |
Transmission depth | 11.8 | 12.38 | 9.73 | 10.8 | 8.53 | 8.34 |
Platform satisfaction | 2.44 | 2.68 | 1.98 | 1.82 | 1.64 | 1.34 |
Content satisfaction | 7.42 | 6.71 | 6.55 | 6.25 | 5.08 | 5.03 |
Service satisfaction | 2.93 | 3.71 | 2.59 | 1.9 | 1.9 | 1.52 |
Transmission validity | 12.79 | 13.1 | 11.12 | 9.97 | 8.62 | 7.89 |
User viscosity | 1.68 | 2.42 | 1.27 | 1.3 | 1.01 | 0.85 |
Recommendation ratio | 5.38 | 7.31 | 4.23 | 4.64 | 3.86 | 3.86 |
Intention to use | 2.85 | 3.87 | 1.97 | 2.43 | 1.9 | 1.73 |
Transmission viscosity | 9.91 | 13.6 | 7.47 | 8.37 | 6.77 | 6.44 |
Final score | 61.6 | 85.42 | 56.3 | 37.59 | 33.28 | 30.96 |
Therefore, the final media influence weight is obtained as shown in Figure 3.
The UCIR model proposed in this paper is an upgrade of the classic transmission dynamics model. In the UCIR model, the status conversion of (ⅰ) unknown persons who directly refuse to understand a certain public opinion topic and become immunized, and (ⅱ) some immunized persons are affected by other related derivative public opinion topics and then return to contact status are proposed. This can make the model more complete and the dissemination mechanism of public opinion can be explained better. On this basis, the system dynamics differential equation is constructed. In the case of comprehensively considering the public opinion transmission process, users are divided into the following four forms:
The total number of people N is assumed to be unchanged, and the entire population is divided into four types:
● U (Unknown): Refers to individuals who currently do not know the public opinion. After understanding the public opinion, they can either become contacts and consider whether to transmission or become directly immunized.
● C (Contact): Refers to hesitating individuals who have known public opinion but have not transmission it yet. They can either directly transmission to become communicators or become immunized without transmissioning.
● I (Infective): Refers to individuals who know public opinion and immediately transmission it.
● R (Recovered): Refers to individuals who know public opinion but are not interested in transmissioning. The influence of related derivative public opinion is considered, and the immunized may become a contact again.
Unknown, Contact, Infective, and Recovered are represented by U(t), C(t), I(t), and R(t). α is the contact rate, β is the transmission rate, θ is the transmission immunity rate, ε is the direct immunity rate, p is the direct shielding rate, and q is the derived transmission rate. The relationship between them is shown in the following Figure 4.
According to the above figure, the dynamic differential equation of the model is as follows:
dU(t)dt=−αU(t)I(t)−pU(t)I(t) | (6) |
dC(t)dt=αU(t)I(t)−βC(t)−εC(t)+qR(t) | (7) |
dI(t)dt=βC(t)−θI(t) | (8) |
dR(t)dt=pU(t)I(t)+εC(t)+θI(t)−qR(t) | (9) |
In the study of infectious diseases, the reproducible number is a key indicator to measure whether the disease will break out during the transmission of the infectious disease. Similarly, in the transmission of public opinion, the reproducible number can also be used to measure whether the transmission of public opinion is likely to explode.
Let X(t) = (C(t), I(t), R(t), U(t))T, then the differential equation of the model can be expressed as:
dxdt=F(x)−V(x) | (10) |
˜F(x)=[αUI000],˜V(x)=[βC+εC−qR−βC+θI−pU−εC−θI+qRαUI+pU] |
Calculated:
F=[0α00000000000000],V=[β+ε0−q0−βθ00−ε−θq−p0α0α+p] |
The reproducible number R0 can be obtained by the spectral radius of the reproducible matrix FV–1:
R0=ρ(FV−1)=αβεθq(β+q−ε) | (11) |
In this article, the discussion on the related topics of #Qingdao epidemic# in 2020 was selected. The amount of discussion on this topic in each media with typical characteristics is given in detail in the table. The first time the data was collected in this article is the date of the first occurrence of the event, October 11, 2020, and the data sampling frequency is 1 day. Specific data are shown in Table 4.
10.11 | 10.12 | 10.13 | 10.14 | 10.15 | 10.16 | 10.17 | 10.18 | 10.19 | |
2064 | 60175 | 18731 | 17197 | 12442 | 9560 | 3788 | 2617 | 2019 | |
25 | 4200 | 4037 | 5481 | 3937 | 6575 | 3231 | 2423 | 3541 | |
Short video | 48 | 1314 | 793 | 611 | 248 | 997 | 400 | 322 | 169 |
Newspapers | 0 | 7 | 45 | 47 | 40 | 161 | 104 | 8 | 43 |
TV | 720 | 485 | 338 | 369 | 770 | 341 | 253 | 354 | 166 |
Radio | 500 | 743 | 345 | 248 | 555 | 366 | 322 | 237 | 172 |
10.20 | 10.21 | 10.22 | 10.23 | 10.24 | 10.25 | 10.26 | 10.27 | 10.28 | |
1007 | 630 | 395 | 215 | 240 | 224 | 250 | 279 | 232 | |
2041 | 1549 | 1045 | 709 | 428 | 294 | 475 | 572 | 650 | |
Short Video | 97 | 26 | 16 | 5 | 8 | 10 | 9 | 14 | 3 |
Newspapers | 100 | 15 | 10 | 4 | 3 | 2 | 7 | 3 | 2 |
TV | 126 | 50 | 21 | 13 | 15 | 14 | 48 | 14 | 41 |
Radio | 124 | 79 | 56 | 58 | 30 | 25 | 22 | 37 | 25 |
10.29 | 10.30 | 10.31 | 11.1 | 11.2 | 11.3 | 11.4 | 11.5 | 11.6 | |
390 | 333 | 175 | 108 | 84 | 100 | 75 | 33 | 43 | |
508 | 569 | 446 | 621 | 386 | 292 | 214 | 116 | 88 | |
Short video | 14 | 15 | 1 | 2 | 3 | 2 | 4 | 3 | 3 |
Newspapers | 5 | 10 | 1 | 1 | 4 | 16 | 3 | 2 | 1 |
TV | 44 | 30 | 43 | 24 | 16 | 11 | 7 | 5 | 2 |
Radio | 31 | 27 | 32 | 33 | 27 | 7 | 4 | 2 | 1 |
According to the calculated influence weight of each media in the second subsection, the amount of discussion on this topic under the converged media can be calculated as shown in Table 5.
10.11 | 10.12 | 10.13 | 10.14 | 10.15 | 10.16 | 10.17 | 10.18 | 10.19 | |
Converged media | 558 | 13576 | 5097 | 5156 | 3781 | 4028 | 1808 | 1323 | 1467 |
10.20 | 10.21 | 10.22 | 10.23 | 10.24 | 10.25 | 10.26 | 10.27 | 10.28 | |
Converged media | 830 | 236 | 168 | 202 | 130 | 106 | 77 | 41 | 34 |
10.29 | 10.30 | 10.31 | 11.1 | 11.2 | 11.3 | 11.4 | 11.5 | 11.6 | |
Converged media | 231 | 236 | 168 | 202 | 130 | 106 | 77 | 41 | 34 |
SIR | UCIR | |
Converged media | 271.39 | 185.72 |
Since there will be a certain error between the estimated value of the model and the actual data, in order to accurately verify the effectiveness of the UCIR model. In this paper, the error between the estimated value of the model and the actual data is calculated through the root mean square error (RMSE). The smaller the error, the better the fit of the curve and the greater the effectiveness of the model. The calculation formula of root mean square error (RMSE) is shown in formula 12.
RMSE=√1NN∑t=1(observed−predicted) | (12) |
RMSE represents the root mean square error; N represents the number of data; observed represents the actual data value collected; predicted represents the data value estimated by the model. The value of I estimated by the classic SIR model was compared with the actual data on the topic of #Qingdao epidemic# in 2020. As shown in Figure 4, it can be seen that this model has a large fitting error. At the same time, the value of I estimated by the UCIR model was compared with the actual data. As shown in Figure 5, it is obvious that this model greatly reduces the curve fitting error. It can better fit the actual data of public opinion events.
The table lists the root-mean-square error of the estimated values of the SIR model and the UCIR model compared with the actual data on the related topics of #Qingdao epidemic# in 2020. Compared with the SIR model, the RMSE of the UCIR model is reduced by 31.6%.
A single communication channel can no longer accurately describe the transmission process of public opinion, and the transmission of public opinion in converged media has more research value. The research on the transmission of public opinion in the converged media is an indispensable link in the work of public opinion transmission. For example, in real life, people can not only obtain public opinion through traditional media such as TV and radio, but also discuss and communicate with friends on new media platforms such as Weibo and WeChat. This is the most common manifestation of interaction between traditional media and new media. Traditional media and new media are slightly different in information acquisition, information can be obtained more in new media, but generally the acceptance of such information is not high, Although the information obtained in traditional media is limited, the acceptance of information is relatively high. In recent years, the transmission of public opinion in traditional media and new media has gradually shown a characteristic that "the trend of public opinion transmission is expanded by new media, while mainstream public opinion transmission is led by traditional media".
In this paper, Matlab2017a is used for experimental verification of the model, and the horizontal axis is set as the time step, and the vertical axis is the change in the proportion of each state. Analyze the law of the public opinion transmission.
It can be seen from Figure 7. that the number of individuals in the four states of the converged media changes over time. As time increases, the number of individuals in the unknown (U) gradually decreases, roughly at t = 18, the unknown (U) will tend to a stable value and no longer change. The individual number of contacts (C) gradually increases with the increase of time. At about t = 12, the individual number of contacts reaches its peak, then begins to decline and eventually tends to zero. The change process of Infective (I) is roughly the same as that of contacts. It also increases first and then decreases. At about t = 13, the number of Infective reaches a peak, then begins to decline and eventually tends to zero. The change in the number of individuals in the recovered (R) is just the opposite of the unknown. As time increases, the number of individuals in the recovered (R) gradually increases, reaching its peak at about t = 18, and then stabilizing at the value. When the entire process of transmission of public opinion is over, the four states of unknown, contact, Infective and recovered all tend to stabilize and remain unchanged.
In the actual transmission of public opinion, the public opinion transmission will be affected by many factors. The influencing factors in this article mainly include: contact rate α, transmission rate β, transmission immunity rate θ, direct immunity rate ε, direct shielding rate p, and derivative transmission rate q. This section mainly studies these six parameters, and the specific experimental results and analysis will be introduced in detail.
Therefore, through the remaining parameters are fixed, only one of them is changed to study the important factors that affecting the transmission of public opinion. Matlab2017a is used to simulate and analyze that the model proposed in this article. The total number of users N = 20000, at t = 0, set S(0) = N−1/N, E(0) = 0, I(0) = 10/N, R(0) = 0. The main parameters are: α = 3.6; β = 2.3; θ = 1.9; p = 0.4; ε = 0.1; q = 0.5.
It can be seen from Figure 8. that with the increase of the contact rate α, the two curves of the C(t) and I(t) increase at a faster rate, which means that the speed of the outbreak of public opinion is accelerated, the time to reach the peak is earlier, and the corresponding peak value is also increased. This shows that the number of users who have converted to C(t) after browsing the public opinion under the converged media has increased, the speed of the transmission of public opinion has been accelerated, and the scope of the transmission of influence has been increased. In the actual public opinion transmission process, whether users are willing to understand a public opinion, the most important factor is the appeal of the content publisher and his credibility. Therefore, if the transmission of public opinion is to be promoted, factors such as the word-of-mouth of the content publisher and the attributes of users deserve to be paid attention to.
It can be seen from Figure 10. that as the transmission rate β increases, the peak of I(t) gradually exceeds E(t), and the time to reach the peak of I(t) is continuously shortened, which means that the greater the transmission rate, the more individuals who are interested in the public opinion and are willing to spread it after being exposed to the public opinion, and the greater the amount of transmission. At the same time, it also shows that the faster the transmission of public opinion, the wider the coverage of public opinion. Therefore, if the transmission of public opinion wants to be suppressed, such as the transmission of rumors needs to be controlled by the government, the method of monitoring important nodes to strictly control the transmission rate can be selected. At the same time, the government can also suppress the transmission of rumors by publishing clarifying information. On the contrary, if the transmission of public opinion wants to be promoted, the transmission rate can be increased through online media, and the public opinion can be forwarded to the greatest extent to expand its scope of influence.
It can be seen from Figure 12. that as the transmission immunity rate θ increases, the peak of I(t) gradually decreases, and the time to reach the peak of I(t) is continuously extended, which means that the greater the transmission immunity rate θ, the more individuals in the I(t) will lose their vitality and can no longer affect individuals in other states to spread. The smaller the amount of transmission, the smaller the coverage of public opinion in the process of transmission, and the transmission of public opinion will also be reduced. Therefore, the transmission of public opinion can be suppressed by enhancing the transmission immunity rate, such as clarifying that the information is quickly released by the government to accelerate the speed of the transformation of I(t) to R(t), and then the transmission of the entire public opinion is controlled.
It can be seen from Figure 14. that the increase of ε indicates that the appeal of the public opinion is insufficient or the content users are not interested, and the more individuals will turn directly from C(t) to R(t). Therefore, the lower the peak of the I(t) curve, the lower the final spread of information. This shows that when users are not interested in the content of public opinion, the number of users of I(t) will be greatly reduced, which will have a significant impact on the transmission of public opinion. Therefore, if the transmission of public opinion is to be promoted, it is necessary to reduce the direct immunity rate and pay more attention to users' points of interest. The content should be edited in the direction that users are interested in, so as to increase the amount of information transmission.
It can be seen from Figure 16. that as the value of p increases, the rising speed of the R(t) curve is accelerated. It shows that with the increase of the direct shielding rate p, the proportion of U(t) individuals in the network gradually decreases, and the proportion of R(t) individuals gradually increases. The spread value of public opinion is reduced, and the effective spread time of public opinion is shortened. Therefore, if the transmission of public opinion is to be strengthened, it is necessary to ensure that the intuitive content such as the title, form, and category of the public opinion published by the platform can attract users, increase users' attention to the public opinion, and reduce the possibility of users directly rejecting the transmission.
It can be seen from Figure 19. that as the derivative transmission rate q increases, the peak of the curve of I(t) increases, the time to stay at the peak becomes longer, and the time for the individual I(t) to return to the initial state becomes later. Even if q is larger, C(t) and I(t) will always exist in the system and reach a certain balance. This shows that as q increases, the effective transmission time of public opinion becomes longer, and its influence also increases. In the process of transmission of public opinion, the stronger the derivation of public opinion, the greater the scope of influence of the transmission. Therefore, if the transmission of public opinion wants to be promoted, the methods of topical information transmission can be used to increase the derivative transmission rate of public opinion, and increase the effective transmission time. In this way, the spread of public opinion is strengthened. Conversely, if the transmission of public opinion wants to be suppressed, the government can stop the transmission of public opinion in time through external intervention and other means, so as to reduce the topic's derivative transmission rate, and reduce the amount of public opinion transmission and the scope of influence.
With the support of the experimental analysis results, the different stages of the public opinion transmission process can be adjusted by the characteristics of each parameter. The guidance of public opinion transmission is realized in order to provide better public opinion transmission strategies. In the initial stage of public opinion transmission, the reproducible number R0 of public opinion transmission is calculated to determine the possibility of public opinion transmission, which is convenient for guiding the transmission of public opinion. Next, suggestions for public opinion guidance are given.
For positive public opinion information, it is expected to transmission as wide as possible. A platform with a higher network density as the source of public opinion transmission can be selected to increase the contact rate α, and the basis for increasing the effect of public opinion transmission is provided. As the party that needs to promote the transmission of public opinion, the transmission rate β can be increased and the direct shielding rate p, the direct immunity rate ε, and the transmission immunity rate θ can be reduced by making the content more sophisticated and more eye-catching. A large amount of information related to the topic of public opinion is released by opinion leaders, and users' discussions are stimulated to increase the derivative transmission rate q. The high-profile transmission is maintained, so that more users can see the public opinion information and actively participate in the transmission. In the end, the scope of public opinion information transmission will be expanded.
When negative public opinion is transmission to a certain scale, we hope to control the transmission of negative public opinion within a certain range and restrain its ability to transmission. The contact rate can be reduced by inhibiting key users from acquiring information. Through external intervention, such as rumor information is clarified and accurate content released by the government to reduce the transmission rate β, increase the direct blocking rate p, the direct immunization rate ε, and the transmission immunity rate θ. At the same time, the false content is corrected, so that users who contact the information can directly read the correct content, the user's derivative transmission rate q of the wrong content is reduced, and the transmissioning scope of the information is reduced.
In this paper, the proportion of each media in the converged media, the existence value of the contact state in the converged media and the derivative characteristics of topical information are comprehensively considered, and the UCIR model of public opinion transmission based on the SIR model is constructed. The feasibility and effectiveness of the model are verified through experiments and comparative analysis, and the law of public opinion transmission that is more in line with the actual situation is studied. On this basis, through the adjustment of each parameter value in the UCIR model, the influence of its changes on the node density is analyzed, so as to find out the law of public opinion transmission. This provides a reference basis for the spread of positive information to be increased and strategies to effectively control the spread of rumors are formulated. It is interesting and definitely worth investigating to solve the problems of humanities and social sciences with the method of natural science. This paper establishes a data-driven public opinion guidance method for converging media. This paper provides scientific data method support for public opinion management of converging media.
Although this paper has made some achievements in the study of the communication of public opinion information, there are still many shortcomings and many problems to be further studied. The future outlook is as follows: the parameter estimation in the model in this paper is based on an initial value to find the parameter local optimal solution that minimizes the error between the model estimate value and the actual data. The best approach is to keep repeating the selection until the objective function has the smallest stable state. In this case, the parameter estimate is the best match with the actual data. In the future, the repeated selection method can be considered as an algorithm for better parameter optimization.
This research was supported by the Research Project of the Beijing Chaoyang District Bureau of Science, Technology and Information Technology (No. CYXC2012) and the Fundamental Research Fund of the Central University (No. CUC2019T008).
We declare that we have no financial and personal relationships with other people or organizations that can inappropriately influence our work, there is no professional or other personal interest of any nature or kind in any product, service or company.
[1] | B. P. Niehoff, R. H. Moorman, Justice as a mediator of the relationship between methods of monitoring and organizational citizenship behavior, Acade. Manage. J., 36 (1993), 527-556. |
[2] | A. Nachison, Good business or good journalism, Lessons from the bleeding edge, World Editors' Forum, 2001. |
[3] | R. Gordon, Implications of convergence, in Digital journalism: Emerging media and the changing horizons of journalism, 2003. |
[4] | F. Liao, Research on the spread mode of emergencies in overseas chinese hometown and the guiding strategies of public opinion in the hometown of overseas chinese in the media era, J. Lishui Univ., 38 (2016), 58-64. |
[5] | W. O. Kermack, A. G. McKendrick, A contribution to the mathematical theory of epidemics, Proc. R. Soc. London, Ser. A, 115 (1927), 1095-1934. |
[6] |
L. Zhao, J. Wang, Y. Chen, Q. Wang, J. Cheng, H. Cui, SIHR rumor spreading model in social networks, Phys. A, 391 (2012), 2444-2453. doi: 10.1016/j.physa.2011.12.008
![]() |
[7] | X. Ding, Research on propagation model of public opinion topics based on SCIR in microblogging, Comput. Eng. Appl., 51 (2015), 20-26. |
[8] | X. Lin, Y. Zhuang, L. Sun, Research on SEIR network public opinion dissemination model with saturated contact rate, J. Inf., 34 (2015), 150-155. |
[9] | X. You, Q. Liu, Research on microblog information dissemination prediction based on infectious disease model, Comput. Appl. Software, 33 (2016), 53-56. |
[10] |
Y. Liu, S. M. Diao, Y. X. Zhu, Q. Liu, SHIR competitive information diffusion model for online social media, Phys. A, 461 (2016), 543-553. doi: 10.1016/j.physa.2016.06.080
![]() |
[11] |
X. Rui, F. Meng, Z. Wang, G. Yuan, C. Du, SPIR: The potential transmissioners involved SIR model for information diffusion in social networks, Phys. A, 506 (2018), 254-269. doi: 10.1016/j.physa.2018.04.062
![]() |
[12] | H. A. Ebadizadeh, H. Haghbayan, Dynamics of rumor spreading, Ann. Optim. Theory Pract., 1 (2018), 45-54. |
[13] |
Y. Zan, DSIR double-rumors spreading model in complex networks, Chaos Solitons Fractals, 110 (2018), 191-202. doi: 10.1016/j.chaos.2018.03.021
![]() |
[14] |
K. M. A. Kabir, K. Kuga, J. Tanimoto, Analysis of SIR epidemic model with information spreading of awareness, Chaos Solitons Fractals, 119 (2019), 118-125. doi: 10.1016/j.chaos.2018.12.017
![]() |
[15] |
R. Xiao, T. Yu, A multi-agent simulation approach to rumor spread in virtual commnunity based on social network, Intell. Autom. Soft Comput., 17 (2011), 859-869. doi: 10.1080/10798587.2011.10643194
![]() |
[16] |
Y. Zan, J. Wu, P. Li, Q. Yu, SICR rumor spreading model in complex networks: Counterattack and self-resistance, Phys. A, 405 (2014), 159-170. doi: 10.1016/j.physa.2014.03.021
![]() |
[17] |
J. Zhou, Z. Liu, B Li, Influence of network structure on rumor propagation, Phys. Lett. A, 368 (2007), 458-463. doi: 10.1016/j.physleta.2007.01.094
![]() |
[18] | Z. Chen, Z. Xu, A delayed diffusive influenza model with two-strain and two vaccinations, Appl. Math. Comput., 349 (2019), 439-453. |
[19] |
R. Y. Tian, X. F. Zhang, Y. J. Liu, SSIC model: A multi-layer model for intervention of online rumors transmissioning, Phys. A, 427 (2015), 181-191. doi: 10.1016/j.physa.2015.02.008
![]() |
[20] |
Q. Liu, T. Li, M. Sun, The analysis of an SEIR rumor propagation model on heterogeneous network, Phys. A, 469 (2017), 372-380. doi: 10.1016/j.physa.2016.11.067
![]() |
[21] |
C. Wan, T. Li, Z. Sun, Global stability of a SEIR rumor transmissioning model with demographics on scale-free networks, Adv. Differ. Equation, 2017 (2017), 1-15. doi: 10.1186/s13662-016-1057-2
![]() |
[22] | Y. Zhang, C. Chen, A rumor spreading model considering latent state, Proceedings of the Eighth International Conference on Management Science and Engineering Management, (2014), 155-162. |
[23] | L. Zhang, C. Su, Y. Jin, M. Goh, Z. Wu, Cross-network dissemination model of public opinion in coupled networks, Inf. Sci., 451 (2018), 240-252. |
[24] |
Y. A. Kuznetsov, C. Piccardi, Bifurcation analysis of periodic SEIR and SIR epidemic models, Math. Biol., 32 (1994), 109-121. doi: 10.1007/BF00163027
![]() |
[25] | J. M. Pan, Z. Chen, X. J. Zhao, The Construction of Evaluation Index System of Network Public Opinion Based on Large Data of Mobile Internet-take Hunan as an example, J. Hunan Ind. Polytech., 15 (2015), 21-24. |
[26] | D. Du, Q. Pang, Y. Wu, Modern Comprehensive Evaluation Method and Case Selection, Beijing: Tsinghua University Press, (2008), 21-32. |
[27] |
M. Radovanovic, A. Ranđelović, Ž. Jokić, Application of hybrid model fuzzy AHP-VIKOR in selection of the most efficient procedure for rectification of the optical sight of the long-range rifle, Decis. Making Appl. Manage. Eng., 3 (2020), 131-148. doi: 10.31181/dmame2003131r
![]() |
[28] |
Z. Bobar, D. Božanić, K. Djurić, D. Pamučar, Ranking and Assessment of the Efficiency of Social Media using the Fuzzy AHP-Z Number Model-Fuzzy MABAC, Acta Polytech. Hung., 17 (2020), 43-70. doi: 10.12700/APH.17.3.2020.3.3
![]() |
[29] |
H. M. Lyu, W. H. Zhou, S. L. Shen, A. N. Zhou, Inundation risk assessment of metro system using AHP and TFN-AHP in Shenzhen, Sustainable Cities Soc., 56 (2020), 102103. doi: 10.1016/j.scs.2020.102103
![]() |
[30] | S. Duleba, Introduction and comparative analysis of the multi-level parsimonious AHP methodology in a public transport development decision problem, J. Oper. Res. Soc., 2020 (2020), 1-14. |
[31] |
R. Ohta, V. A. P. Salomon, M. B. Silva, Classical, fuzzy, hesitant fuzzy and intuitionistic fuzzy analytic hierarchy processes applied to industrial maintenance management, J. Intell. Fuzzy Syst., 38 (2020), 601-608. doi: 10.3233/JIFS-179433
![]() |
[32] | M. C. Choi, Evaluation of analytic hierarchy process method and development of a weight modified model, Manage. Inf. Syst. Rev., 39 (2020), 145-162. |
[33] | C. Lin, G. Kou, A heuristic method to rank the alternatives in the AHP synthesis, Appl. Soft Comput., 100 (2020), 106916. |
[34] |
J. E. Leal, AHP-express: A simplified version of the analytical hierarchy process method, MethodsX, 7 (2020), 100748. doi: 10.1016/j.mex.2019.11.021
![]() |
[35] |
X. Deng, Y. Deng, D-AHP method with different credibility of information, Soft Comput., 23 (2019), 683-691. doi: 10.1007/s00500-017-2993-9
![]() |
[36] |
P Zhang, Q Liu, B Kang, An improved OWA-Fuzzy AHP decision model for multi-attribute decision making problem, J. Intell. Fuzzy Syst., 40 (2021), 9655-9668. doi: 10.3233/JIFS-202168
![]() |
[37] |
F. Abastante, S. Corrente, S. Greco, A. Ishizaka, I. M. Lami, A new parsimonious AHP methodology: Assigning priorities to many objects by comparing pairwise few reference objects, Expert Syst. Appl., 127 (2019), 109-120. doi: 10.1016/j.eswa.2019.02.036
![]() |
[38] | T. Chen, A diversified AHP-tree approach for multiple-criteria supplier selection, Comput. Manage. Sci., 2021 (2021), 1-23. |
[39] |
W. Li, G. Xu, Q. Xing, M. Lyu, Application of improved AHP-BP neural network in CSR performance evaluation model, Wireless Pers. Commun., 111 (2020), 2215-2230. doi: 10.1007/s11277-019-06981-z
![]() |
1. | Sicheng Huang, Zibo Lin, Xinqi Lin, Lin Li, Feng Ruan, Wenhua Mei, Sidong Chen, Dragan Pamucar, Establishment of a no-notice drill mode evaluation system for public health emergencies, 2022, 17, 1932-6203, e0266093, 10.1371/journal.pone.0266093 | |
2. | Guangyu Mu, Zehan Liao, Jiaxue Li, Nini Qin, Ziye Yang, Nebojsa Bacanin, IPSO-LSTM hybrid model for predicting online public opinion trends in emergencies, 2023, 18, 1932-6203, e0292677, 10.1371/journal.pone.0292677 | |
3. | Shunli Zhang, Evaluation and guidance of university network public opinion environment based on fuzzy evaluation method, 2024, 24, 14727978, 2763, 10.3233/JCM-247511 | |
4. | Hongying Fei, Jinyin Zhu, Analysis of the Influence of Online Public Opinion on Corporate Brand Value: An Efficient Way to Avoid Unexpected Shocks from the Internet, 2024, 12, 2079-8954, 337, 10.3390/systems12090337 | |
5. | Hua Wang, Ling Luo, Tao Liu, Applying the DEMATEL−ANP Fuzzy Comprehensive Model to Evaluate Public Opinion Events, 2023, 13, 2076-3417, 5737, 10.3390/app13095737 |
The level of importance | Meaning |
1 | Equally important |
3 | Slightly important |
5 | Obviously important |
7 | Very important |
9 | Extremely important |
2, 4, 6, 8 | ………… |
First-level indicator | The weight of first-level indicators | Secondary indicators | The weight of secondary indicators | Combination weight |
Transmission breadth | 0.158 | Number of visitors | 0.178 | 0.028 |
Number of information released | 0.295 | 0.047 | ||
Content coverage | 0.273 | 0.043 | ||
User coverage | 0.254 | 0.04 | ||
Transmission intensity | 0.35 | Number of comments | 0.231 | 0.081 |
Number of reposts | 0.546 | 0.191 | ||
Number of likes | 0.223 | 0.078 | ||
Transmission depth | 0.162 | Cognitive channel | 0.268 | 0.043 |
Quality cognition | 0.521 | 0.085 | ||
Functional cognition | 0.211 | 0.034 | ||
Transmission validity | 0.172 | Platform satisfaction | 0.198 | 0.034 |
Content satisfaction | 0.546 | 0.094 | ||
Service satisfaction | 0.256 | 0.044 | ||
Transmission viscosity | 0.158 | User viscosity | 0.182 | 0.028 |
Recommendation ratio | 0.541 | 0.085 | ||
Intention to use | 0.277 | 0.045 |
Indicator | Short video | TV | radio | newspapers | ||
Number of visitors | 1.37 | 2.8 | 0.97 | 0.6 | 0.09 | 0.04 |
Number of information released | 2.44 | 4.7 | 1.97 | 0.43 | 0.18 | 0.08 |
Content coverage | 1.26 | 2.63 | 1.34 | 3.82 | 3.8 | 4.23 |
User coverage | 3.33 | 4 | 3.06 | 3.61 | 3.78 | 2.94 |
Transmission breadth | 8.4 | 14.13 | 7.34 | 8.46 | 7.85 | 7.29 |
Number of comments | 5.86 | 8.1 | 3.58 | 0.35 | 0.37 | 0.67 |
Number of reposts | 9.51 | 19.1 | 14.39 | 1.05 | 1.95 | 1.03 |
Number of likes | 5.61 | 7.8 | 4.6 | 0.54 | 0.75 | 0.79 |
Transmission intensity | 20.98 | 35 | 22.57 | 1.94 | 3.07 | 2.49 |
Cognitive channel | 3.18 | 3.53 | 2.93 | 3.01 | 2.28 | 2.16 |
Quality cognition | 6.34 | 6.06 | 4.87 | 5.84 | 4.69 | 4.69 |
Functional cognition | 2.28 | 2.79 | 1.93 | 1.95 | 1.56 | 1.49 |
Transmission depth | 11.8 | 12.38 | 9.73 | 10.8 | 8.53 | 8.34 |
Platform satisfaction | 2.44 | 2.68 | 1.98 | 1.82 | 1.64 | 1.34 |
Content satisfaction | 7.42 | 6.71 | 6.55 | 6.25 | 5.08 | 5.03 |
Service satisfaction | 2.93 | 3.71 | 2.59 | 1.9 | 1.9 | 1.52 |
Transmission validity | 12.79 | 13.1 | 11.12 | 9.97 | 8.62 | 7.89 |
User viscosity | 1.68 | 2.42 | 1.27 | 1.3 | 1.01 | 0.85 |
Recommendation ratio | 5.38 | 7.31 | 4.23 | 4.64 | 3.86 | 3.86 |
Intention to use | 2.85 | 3.87 | 1.97 | 2.43 | 1.9 | 1.73 |
Transmission viscosity | 9.91 | 13.6 | 7.47 | 8.37 | 6.77 | 6.44 |
Final score | 61.6 | 85.42 | 56.3 | 37.59 | 33.28 | 30.96 |
10.11 | 10.12 | 10.13 | 10.14 | 10.15 | 10.16 | 10.17 | 10.18 | 10.19 | |
2064 | 60175 | 18731 | 17197 | 12442 | 9560 | 3788 | 2617 | 2019 | |
25 | 4200 | 4037 | 5481 | 3937 | 6575 | 3231 | 2423 | 3541 | |
Short video | 48 | 1314 | 793 | 611 | 248 | 997 | 400 | 322 | 169 |
Newspapers | 0 | 7 | 45 | 47 | 40 | 161 | 104 | 8 | 43 |
TV | 720 | 485 | 338 | 369 | 770 | 341 | 253 | 354 | 166 |
Radio | 500 | 743 | 345 | 248 | 555 | 366 | 322 | 237 | 172 |
10.20 | 10.21 | 10.22 | 10.23 | 10.24 | 10.25 | 10.26 | 10.27 | 10.28 | |
1007 | 630 | 395 | 215 | 240 | 224 | 250 | 279 | 232 | |
2041 | 1549 | 1045 | 709 | 428 | 294 | 475 | 572 | 650 | |
Short Video | 97 | 26 | 16 | 5 | 8 | 10 | 9 | 14 | 3 |
Newspapers | 100 | 15 | 10 | 4 | 3 | 2 | 7 | 3 | 2 |
TV | 126 | 50 | 21 | 13 | 15 | 14 | 48 | 14 | 41 |
Radio | 124 | 79 | 56 | 58 | 30 | 25 | 22 | 37 | 25 |
10.29 | 10.30 | 10.31 | 11.1 | 11.2 | 11.3 | 11.4 | 11.5 | 11.6 | |
390 | 333 | 175 | 108 | 84 | 100 | 75 | 33 | 43 | |
508 | 569 | 446 | 621 | 386 | 292 | 214 | 116 | 88 | |
Short video | 14 | 15 | 1 | 2 | 3 | 2 | 4 | 3 | 3 |
Newspapers | 5 | 10 | 1 | 1 | 4 | 16 | 3 | 2 | 1 |
TV | 44 | 30 | 43 | 24 | 16 | 11 | 7 | 5 | 2 |
Radio | 31 | 27 | 32 | 33 | 27 | 7 | 4 | 2 | 1 |
10.11 | 10.12 | 10.13 | 10.14 | 10.15 | 10.16 | 10.17 | 10.18 | 10.19 | |
Converged media | 558 | 13576 | 5097 | 5156 | 3781 | 4028 | 1808 | 1323 | 1467 |
10.20 | 10.21 | 10.22 | 10.23 | 10.24 | 10.25 | 10.26 | 10.27 | 10.28 | |
Converged media | 830 | 236 | 168 | 202 | 130 | 106 | 77 | 41 | 34 |
10.29 | 10.30 | 10.31 | 11.1 | 11.2 | 11.3 | 11.4 | 11.5 | 11.6 | |
Converged media | 231 | 236 | 168 | 202 | 130 | 106 | 77 | 41 | 34 |
SIR | UCIR | |
Converged media | 271.39 | 185.72 |
The level of importance | Meaning |
1 | Equally important |
3 | Slightly important |
5 | Obviously important |
7 | Very important |
9 | Extremely important |
2, 4, 6, 8 | ………… |
First-level indicator | The weight of first-level indicators | Secondary indicators | The weight of secondary indicators | Combination weight |
Transmission breadth | 0.158 | Number of visitors | 0.178 | 0.028 |
Number of information released | 0.295 | 0.047 | ||
Content coverage | 0.273 | 0.043 | ||
User coverage | 0.254 | 0.04 | ||
Transmission intensity | 0.35 | Number of comments | 0.231 | 0.081 |
Number of reposts | 0.546 | 0.191 | ||
Number of likes | 0.223 | 0.078 | ||
Transmission depth | 0.162 | Cognitive channel | 0.268 | 0.043 |
Quality cognition | 0.521 | 0.085 | ||
Functional cognition | 0.211 | 0.034 | ||
Transmission validity | 0.172 | Platform satisfaction | 0.198 | 0.034 |
Content satisfaction | 0.546 | 0.094 | ||
Service satisfaction | 0.256 | 0.044 | ||
Transmission viscosity | 0.158 | User viscosity | 0.182 | 0.028 |
Recommendation ratio | 0.541 | 0.085 | ||
Intention to use | 0.277 | 0.045 |
Indicator | Short video | TV | radio | newspapers | ||
Number of visitors | 1.37 | 2.8 | 0.97 | 0.6 | 0.09 | 0.04 |
Number of information released | 2.44 | 4.7 | 1.97 | 0.43 | 0.18 | 0.08 |
Content coverage | 1.26 | 2.63 | 1.34 | 3.82 | 3.8 | 4.23 |
User coverage | 3.33 | 4 | 3.06 | 3.61 | 3.78 | 2.94 |
Transmission breadth | 8.4 | 14.13 | 7.34 | 8.46 | 7.85 | 7.29 |
Number of comments | 5.86 | 8.1 | 3.58 | 0.35 | 0.37 | 0.67 |
Number of reposts | 9.51 | 19.1 | 14.39 | 1.05 | 1.95 | 1.03 |
Number of likes | 5.61 | 7.8 | 4.6 | 0.54 | 0.75 | 0.79 |
Transmission intensity | 20.98 | 35 | 22.57 | 1.94 | 3.07 | 2.49 |
Cognitive channel | 3.18 | 3.53 | 2.93 | 3.01 | 2.28 | 2.16 |
Quality cognition | 6.34 | 6.06 | 4.87 | 5.84 | 4.69 | 4.69 |
Functional cognition | 2.28 | 2.79 | 1.93 | 1.95 | 1.56 | 1.49 |
Transmission depth | 11.8 | 12.38 | 9.73 | 10.8 | 8.53 | 8.34 |
Platform satisfaction | 2.44 | 2.68 | 1.98 | 1.82 | 1.64 | 1.34 |
Content satisfaction | 7.42 | 6.71 | 6.55 | 6.25 | 5.08 | 5.03 |
Service satisfaction | 2.93 | 3.71 | 2.59 | 1.9 | 1.9 | 1.52 |
Transmission validity | 12.79 | 13.1 | 11.12 | 9.97 | 8.62 | 7.89 |
User viscosity | 1.68 | 2.42 | 1.27 | 1.3 | 1.01 | 0.85 |
Recommendation ratio | 5.38 | 7.31 | 4.23 | 4.64 | 3.86 | 3.86 |
Intention to use | 2.85 | 3.87 | 1.97 | 2.43 | 1.9 | 1.73 |
Transmission viscosity | 9.91 | 13.6 | 7.47 | 8.37 | 6.77 | 6.44 |
Final score | 61.6 | 85.42 | 56.3 | 37.59 | 33.28 | 30.96 |
10.11 | 10.12 | 10.13 | 10.14 | 10.15 | 10.16 | 10.17 | 10.18 | 10.19 | |
2064 | 60175 | 18731 | 17197 | 12442 | 9560 | 3788 | 2617 | 2019 | |
25 | 4200 | 4037 | 5481 | 3937 | 6575 | 3231 | 2423 | 3541 | |
Short video | 48 | 1314 | 793 | 611 | 248 | 997 | 400 | 322 | 169 |
Newspapers | 0 | 7 | 45 | 47 | 40 | 161 | 104 | 8 | 43 |
TV | 720 | 485 | 338 | 369 | 770 | 341 | 253 | 354 | 166 |
Radio | 500 | 743 | 345 | 248 | 555 | 366 | 322 | 237 | 172 |
10.20 | 10.21 | 10.22 | 10.23 | 10.24 | 10.25 | 10.26 | 10.27 | 10.28 | |
1007 | 630 | 395 | 215 | 240 | 224 | 250 | 279 | 232 | |
2041 | 1549 | 1045 | 709 | 428 | 294 | 475 | 572 | 650 | |
Short Video | 97 | 26 | 16 | 5 | 8 | 10 | 9 | 14 | 3 |
Newspapers | 100 | 15 | 10 | 4 | 3 | 2 | 7 | 3 | 2 |
TV | 126 | 50 | 21 | 13 | 15 | 14 | 48 | 14 | 41 |
Radio | 124 | 79 | 56 | 58 | 30 | 25 | 22 | 37 | 25 |
10.29 | 10.30 | 10.31 | 11.1 | 11.2 | 11.3 | 11.4 | 11.5 | 11.6 | |
390 | 333 | 175 | 108 | 84 | 100 | 75 | 33 | 43 | |
508 | 569 | 446 | 621 | 386 | 292 | 214 | 116 | 88 | |
Short video | 14 | 15 | 1 | 2 | 3 | 2 | 4 | 3 | 3 |
Newspapers | 5 | 10 | 1 | 1 | 4 | 16 | 3 | 2 | 1 |
TV | 44 | 30 | 43 | 24 | 16 | 11 | 7 | 5 | 2 |
Radio | 31 | 27 | 32 | 33 | 27 | 7 | 4 | 2 | 1 |
10.11 | 10.12 | 10.13 | 10.14 | 10.15 | 10.16 | 10.17 | 10.18 | 10.19 | |
Converged media | 558 | 13576 | 5097 | 5156 | 3781 | 4028 | 1808 | 1323 | 1467 |
10.20 | 10.21 | 10.22 | 10.23 | 10.24 | 10.25 | 10.26 | 10.27 | 10.28 | |
Converged media | 830 | 236 | 168 | 202 | 130 | 106 | 77 | 41 | 34 |
10.29 | 10.30 | 10.31 | 11.1 | 11.2 | 11.3 | 11.4 | 11.5 | 11.6 | |
Converged media | 231 | 236 | 168 | 202 | 130 | 106 | 77 | 41 | 34 |
SIR | UCIR | |
Converged media | 271.39 | 185.72 |