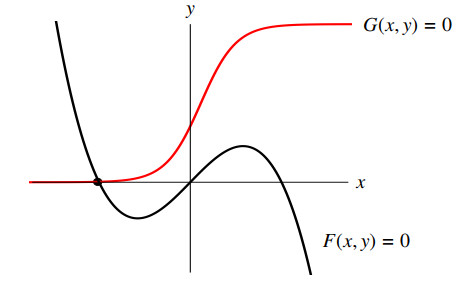
Citation: Hwayeon Ryu, Sue Ann Campbell. Stability, bifurcation and phase-locking of time-delayed excitatory-inhibitory neural networks[J]. Mathematical Biosciences and Engineering, 2020, 17(6): 7931-7957. doi: 10.3934/mbe.2020403
[1] | Yuan Ma, Yunxian Dai . Stability and Hopf bifurcation analysis of a fractional-order ring-hub structure neural network with delays under parameters delay feedback control. Mathematical Biosciences and Engineering, 2023, 20(11): 20093-20115. doi: 10.3934/mbe.2023890 |
[2] | Wenlong Wang, Chunrui Zhang . Bifurcation of a feed forward neural network with delay and application in image contrast enhancement. Mathematical Biosciences and Engineering, 2020, 17(1): 387-403. doi: 10.3934/mbe.2020021 |
[3] | Sarita Bugalia, Jai Prakash Tripathi, Hao Wang . Mathematical modeling of intervention and low medical resource availability with delays: Applications to COVID-19 outbreaks in Spain and Italy. Mathematical Biosciences and Engineering, 2021, 18(5): 5865-5920. doi: 10.3934/mbe.2021295 |
[4] | LanJiang Luo, Haihong Liu, Fang Yan . Dynamic behavior of P53-Mdm2-Wip1 gene regulatory network under the influence of time delay and noise. Mathematical Biosciences and Engineering, 2023, 20(2): 2321-2347. doi: 10.3934/mbe.2023109 |
[5] | Juenu Yang, Fang Yan, Haihong Liu . Dynamic behavior of the p53-Mdm2 core module under the action of drug Nutlin and dual delays. Mathematical Biosciences and Engineering, 2021, 18(4): 3448-3468. doi: 10.3934/mbe.2021173 |
[6] | P. E. Greenwood, L. M. Ward . Rapidly forming, slowly evolving, spatial patterns from quasi-cycle Mexican Hat coupling. Mathematical Biosciences and Engineering, 2019, 16(6): 6769-6793. doi: 10.3934/mbe.2019338 |
[7] | Ranjit Kumar Upadhyay, Swati Mishra, Yueping Dong, Yasuhiro Takeuchi . Exploring the dynamics of a tritrophic food chain model with multiple gestation periods. Mathematical Biosciences and Engineering, 2019, 16(5): 4660-4691. doi: 10.3934/mbe.2019234 |
[8] | Zhichao Jiang, Xiaohua Bi, Tongqian Zhang, B.G. Sampath Aruna Pradeep . Global Hopf bifurcation of a delayed phytoplankton-zooplankton system considering toxin producing effect and delay dependent coefficient. Mathematical Biosciences and Engineering, 2019, 16(5): 3807-3829. doi: 10.3934/mbe.2019188 |
[9] | Shunyi Li . Hopf bifurcation, stability switches and chaos in a prey-predator system with three stage structure and two time delays. Mathematical Biosciences and Engineering, 2019, 16(6): 6934-6961. doi: 10.3934/mbe.2019348 |
[10] | Shishi Wang, Yuting Ding, Hongfan Lu, Silin Gong . Stability and bifurcation analysis of $ SIQR $ for the COVID-19 epidemic model with time delay. Mathematical Biosciences and Engineering, 2021, 18(5): 5505-5524. doi: 10.3934/mbe.2021278 |
Neuronal networks in the brain involve two fundamental types of neurons: excitatory neurons tend to promote the firing of action potentials in other neurons, while inhibitory neurons do the opposite [1,2,3]. The presence of both types of cells is thought to be important for the formation of network oscillation patterns such as bursting and clustering [4,5,6,7,8,9].
Different network architectures can be found in different brain areas. Global inhibition, where the excitatory cells are sparsely connected but receive a common inhibitory input due to highly connected inhibitory cells, has been implicated in the formation of bursting oscillations in the thalamus [7,10,11]. Networks where reciprocal excitatory and inhibitory connections dominate are thought to be important in rhythm generation in the hippocampus [6,9]. However, for networks in the cortex, coupling between excitatory cells is considered to be important [12]. Large scale networks in the cortex typically have local excitatory-inhibitory circuits with long range excitatory connections [13,14].
Whether excitatory or inhibitory, synaptic coupling represents the communication of electrical information from one neuron to another. Inherent in this process are time delays. Conduction delay results from the time it takes for the electrical information to travel along the axon of one neuron to the synapse with another neuron. Synaptic delay results from processed and chemical reactions that occur at the synapse itself. We refer to the total effect of these two delays as the coupling delay.
Numerical simulation studies of biophysical neural network models indicate that time delays can be important in the formation of network behavior [6,15,16,17,18,19,20]. To understand the mechanisms behind such behavior, mathematical analysis is needed. Several different approaches to this problem can be taken, depending on the context. Model networks of neurons that are intrinsically oscillatory can be studied using a phase model, phase resetting curve or a Poincaré map approach [21,22,23,24,25], but other approaches exist [26,27]. Continuum models have been useful for studying delay induced wave-propagation in large scale networks [28,29,30]. Stability and bifurcation analysis has proved useful for studying networks of excitable neurons [27,31,32,33,34,35,36,37,38,39,40,41]. Relaxation oscillators, either inherently oscillatory or excitable, can be studied via geometric singular perturbation theory [42,43,44,45,46,47]. However, with a few exceptions [44,45,46,47] the work above focuses on networks where all the neurons are of a single type (either excitatory or inhibitory) or the coupling is diffusive or sigmoidal rather than synaptic. We note that there is an extensive literature on the effects of time delay on stability and synchronization in artificial neural networks, which we do not attempt to review here. For recent examples see [48,49,50,51].
In our previous study [47], we considered a network of excitable, relaxation oscillator neurons, previously studied in [7,10], where two distinct populations, one excitatory and one inhibitory, are coupled with time-delayed synapses. The excitatory population is uncoupled, while the inhibitory population is tightly coupled without time delay. Based on a geometric singular perturbation analysis for relaxation oscillators, our results showed delays play a key role in producing network rhythms where the excitatory cells are synchronized, that is, phase-locked with zero phase difference. The analysis helped to explain how coupling delays in either excitatory or inhibitory synapses contribute to generating these rhythms and determine the phase relationship between the excitatory and inhibitory cells. Despite the richness of these results, our work was restricted to networks of neurons modeled as relaxation oscillators. Also, further analysis on other types of network behaviors, such as clustering, is needed to obtain a more complete understanding of how different population rhythms arise as a result of the interaction between coupling delays, intrinsic properties of each cell and network architecture.
To extend previous work while relaxing the model limitations mentioned above, we study a general network of excitable, non-relaxation oscillators. In this network, there are two distinct populations, each of which includes a pair of excitatory and inhibitory neurons. To relax our previous assumption where the excitatory cells were uncoupled, we couple them through time-delayed synapses while leaving inhibitory cells coupled only with their respective excitatory ones. This allows the interaction among excitatory cells, which may result in the emergence of different network behaviors such as clustering. Based on the extended model, our goal is to provide the existence and stability conditions of differing population behaviors in terms of intrinsic properties of cells, the size of coupling delays, and strengths.
Our paper is structured as follows: Section 2 presents the models for a single cell and for the coupled network which will be considered in our study. Section 3 describes our analysis results including linear stability analysis. Section 4 presents supplementary numerical simulations along with numerical bifurcation analysis results using XPPAUT [52] and DDE-BIFTOOL [53]. We conclude with a discussion in Section 5.
We first describe the model equations corresponding to an uncoupled, single cell. There are two types: one for inhibitory cells and one for excitatory cells. Then we introduce the synaptic coupling between the cells, coupling delays, and network architecture to be considered.
The generalized equations for this model are as follows:
x′=F(x,y), | (2.1) |
y′=G(x,y), | (2.2) |
where ′=ddt, x∈R, and y∈Rn. Here x represents the voltage or activity of the neuron and y represents the gating or recovery variables. To simplify the analysis, we consider n=1 in our study (see [10] for an example with n>1). We assume that the x-nullcline, F(x,y)=0, is a cubic- shaped function, with left, middle, right branches, and F>0 (F<0) below (above) the x-nullcline curve. In addition, the y-nullcline is assumed to be a monotone increasing function of x that intersects with x-nullcline at the unique equilibrium point, and G>0 (G<0) below (above) the y-nullcline curve. This model framework includes several in the literature [54,55,56], and often higher dimensional models can be reduced to two dimensional models in this form [3].
Intersections of the nullclines determine equilibrium points of the system. Depending on the location of the equilibrium point (either left or middle branch) along the x-nullcline, the system is either excitable or oscillatory, respectively. For the excitable system, as can be seen in Figure 1, the equilibrium point is stable so no periodic solutions arise. In this situation, the equilibrium point is typically called the resting equilibrium point as the neurons are not active. However, if a sufficient amount of input, such as applied current, is applied to the excitable system, the equilibrium point can occur in the middle branch of the x-nullcline, resulting in an oscillatory system. A detailed discussion on oscillatory system behaviors with varying applied currents is provided in Appendix B. Since many cells in the brain are active only when stimulated [1,2,3] our model cells are assumed to be excitable not oscillatory.
We consider networks with the architecture as shown in Figure 2. This is motivated by many brain regions, where there are local connections (within a region) between inhibitory and excitatory cells but global connections (between different region) are primarily between excitatory cells. This is the case, for example in the cortex, where inhibitory connections are primarily confined within a column, and even within a layer of a column, while the connections between columns or to different regions are excitatory [13,14]. As a simple model of this, we consider a system with two excitatory and two inhibitory cells, where each E-I pair represents a different population. Thus there are no time delays in the connections between excitatory and inhibitory cells, but there are time delays in the connections between excitatory cells. We consider only two cells of each type to simplify the analysis. We will discuss how our work might be extended to larger networks in Section 5.
The equations corresponding to each Ei for i=1,2 in the network are
x′i=FE(xi,yi)−gEIs(xi+2(t))(xi−xEI)−gEEs(x3−i(t−τ3−i))(xi−xEE), | (2.3) |
y′i=GE(xi,yi), | (2.4) |
where FE,GE correspond to F,G in Eqs (2.1) and (2.2), and gEI>0 represents the maximal conductance of the inhibitory synapse, which can be viewed as coupling strength from the I-cell to its connected E-cell in the same population. The function s determines the synaptic coupling. It is a sigmoidal function which takes values in [0,1]. Since the I-cell sends inhibition to the E-cells, xEI, the reversal potential for the synaptic connection, is set so that xi−xEI>0, for the physiological range of values for xi. Thus we will assume xi is less than the x-value of the resting equilbrium point for the uncoupled cell. We denote xinh≡xEI. In a similar way, gEE>0 represents the maximal conductance of the excitatory synapse from the E-cell in the different population. xEE represents the reversal potential for the excitatory connection, which is set so that xi−xEE<0, for most of the physiological range of values for xi. Thus we will assume that xEE is a small positive value. Finally, τ3−i for i=1,2 denotes the delay in the excitatory synapse from E3−i to Ei cells in different populations. Note that these are the only coupling delays in the network to be considered.
The model equations for Ij−2 for j=3,4 are similarly given by
x′j=FI(xj,yj)−gIEs(xj−2(t))(xj−xIE), | (2.5) |
y′j=GI(xj,yj), | (2.6) |
where gIE denotes the maximal conductance of the excitatory synapse from E to I within the same population. For analysis simplicity, we assume gEI=gIE<gEE but we could consider a different case for these strengths. The reversal potential for the excitatory synapse, denoted by xIE, is chosen so that xj−xIE<0 for most of the physiological range of values for xj. We assume xexc≡xEE=xIE but a different combination could be also considered for more complex network connections. It is assumed that there is no coupling delay in the synaptic connection from E to I within the same population.
Note that we do not incorporate chemical kinetics for synapses into our model. However, τ1 and τ2 include the effect of delays due to the chemical kinetics, as well as other factors. In addition, intrinsic properties of E- and I-cells, respectively, are identical in the system, in other words, FE and GE are the same for both E-cells, while FI and GI are the same for both I-cells.
Recall that an excitable cell stays at its stable equilibrium point if there is no an external synaptic input applied to the cell. The effect of this input depends on the type of coupling. For example, since xi−xinh>0, inhibitory coupling decreases x′i in Eq (2.3) while excitatory coupling increases x′j in Eq (2.5), so long as xj−xexc<0.
Equations (2.3)–(2.6) is a system of delay differential equations which can be written in vector form as
x′=F(x,x(t−τ1),x(t−τ2)), | (2.7) |
where x=(x1,y1,x2,y2)T. Let τmax=max(τ1,τ2). Then appropriate initial conditions are
x(t)=ϕ(t),−τmax≤t≤0, | (2.8) |
where ϕ is a continuous function from [−τmax,0] to R4. The initial value problem in the systems (2.7) and (2.8) is guaranteed to have a unique solution if F is a C1 function [57]. Thus we assume F satisfies this condition.
Let E∗=(x∗1,y∗1,x∗2,y∗2,x∗3,y∗3,x∗4,y∗4) be an equilibrium point of Eqs (2.3)–(2.6). We assume that it is symmetric, i.e., in the form E∗=(x∗E,y∗E,x∗E,y∗E,x∗I,y∗I,x∗I,y∗I). Plugging E∗ into the system yields that
0=FE(x∗E,y∗E)−gEIs(x∗I)(x∗E−xinh)−gEEs(x∗E)(x∗E−xexc), | (3.1) |
0=GE(x∗E,y∗E), | (3.2) |
0=FI(x∗I,y∗I)−gIEs(x∗E)(x∗I−xexc), | (3.3) |
0=GI(x∗I,y∗I). | (3.4) |
If there is no coupling, gEE=gEI=gIE=0, then there is an equilibrium point where each neuron is at its resting equilibrium point: xR=(xRE,yRE,xRE,yRE,xRI,yRI,xRI,yRI) where xRE,yRE,xRI,yRI satisfy
0=FE(xRE,yRE),0=GE(xRE,yRE),0=FI(xRI,yRI),0=GI(xRI,yRI). |
Typically the synapses are not active when the neuron is at rest, thus we will assume that s(xRE),s(xRI)=O(δ) where 0<δ≪1. A simple perturbation argument then shows that when there is coupling in the network with gEE,gIE,gEI=O(1) (or smaller) the resting equilibrium point persists and is given by xR+O(δ).
Other equilibrium points may also exist. To see this, consider a simplified situation when there is no inhibition, that is, gEI=0. Then x∗E,y∗E satisfy
0=FE(x∗E,y∗E)−gEEs(x∗E)(x∗E−xexc), | (3.5) |
0=GE(x∗E,y∗E), | (3.6) |
while the equations for x∗I,y∗I are unchanged, see Eqs (3.3) and (3.4). We can visualize the values of x∗E and y∗E via intersections of the nullclines as shown in Figure 3. The effect of the coupling term IEE≡gEEs(xE)(xE−xexc) can be understood as follows. Recall that gEE>0 and s(xE)≥0 for all xE. Thus IEE=0 if xE=xexc, negative if xE<xexc and positive otherwise. This can be seen in Figure 3a: the blue curve (gEE>0) intersects the black curve (gEE=0) at xexc, lies above it for x<xexc and below it for x>xexc. However, if xE is sufficiently small then s(xE)≈0. Thus the black and blue curves are virtually identical for xE sufficiently small. Since we assume s(xRE) is small, the resting equilibrium point is almost unchanged by gEE, but if gEE is large enough, other equilibrium points may exist as shown by the multiple intersection points of the blue and red curves in Figure 3a. The effect of the excitatory coupling on the inhibitory cell, IIE≡gIEs(x∗E)(xI−xexc), is similar but simpler. If x∗E is small then s(x∗E)≈0 and (x∗I,y∗I)≈(xRI,yRI). If x∗E is large enough then IIE will shift the x-nullcline up yielding moving (x∗I,y∗I) up and to the right. See Figure 3b.
If there is no coupling between the E-cells, gEE=0 but gEI,gIE>0, the only equilibrium point is the resting equilibrium point. This follows from the fact that for any xI, CEI=gIEs(xI)(xE−xinh)=0 if xE=xinh, is postive for xE>xinh and negative for xE<xinh. Thus there can only be one equilibrium value for the E-cell and this satisfies x∗E<xRE. This means that s(x∗E)≈0, which in turn implies x∗I≈xRI.
If all couplings are present, the situation will be similar to the case with gEI=0. The only difference is that to obtain the extra equilibrium points, gEE may need to be larger to overcome the inhibition.
To conduct the linear stability analysis, we first write xi(t)=x∗i+μi(t) and yi(t)=y∗i+ηi(t) for i=1,2 and analogously, xj(t),yj(t) for j=3,4. Linearizing Eqs (2.3)–(2.6) about E∗ yields for i=1,2, j=3,4,
μ′i(t)=(a1−c1−d1)μi(t)+a2ηi(t)−c2μi+2(t)−d2μ3−i(t−τ3−i),η′i(t)=(b1μi(t)+b2ηi(t)),μ′j(t)=(a3−c3)μj(t)+a4ηj(t)−c4μj−2(t),η′j(t)=(b3μj(t)+b4ηj(t)), | (3.7) |
where [a1a2b1b2]=[∂FE∂x∂FE∂y∂GE∂x∂GE∂y]|(x∗E,y∗E)=[∗<0>0<0],[a3a4b3b4]=[∂FI∂x∂FI∂y∂GI∂x∂GI∂y]|(x∗I,y∗I)=[∗<0>0<0],
[c1c2c3c4]=[gEIs(x∗I)gEIs′(x∗I)(x∗E−xinh)gIEs(x∗E)gIEs′(x∗E)(x∗I−xexc)]=[>0>0>0<0], |
[d1d2]=gEE[s(x∗E)s′(x∗E)(x∗E−xexc)]=[>0<0]. |
The signs for most coefficients are fixed as indicated based on our assumptions about the forms of the nonlinear functions. However, as can be seen from Figure 3, if gEE>0 the signs of a1 and a3 will depend on the value of x∗E and x∗I. For the resting equilibrium point a1<0,a3<0 always. Further, if s′(xRE),s′(xRI)=O(δ) and gEE,gIE,gEI=O(1) then cj,dj=O(δ). Then, a simple perturbation argument shows that the stability of the resting equilibrium point is unaffected by the coupling.
The characteristic equation for this linear DDE is obtained by considering solutions of the form
(μ1(t)η1(t)μ2(t)η2(t)μ3(t)η3(t)μ4(t)η4(t))=eλt(v1v2v3v4v5v6v7v8), |
where λ=β+iω∈C for β,ω∈R. Such solutions will be nontrivial if and only if
det[(λ−Π1)−a2d2e−λτ20c2000−b1(λ−b2)000000d2e−λτ10(λ−Π1)−a200c2000−b1(λ−b2)0000c4000(λ−Π2)−a4000000−b3(λ−b4)0000c4000(λ−Π2)−a4000000−b3(λ−b4)]=0, | (3.8) |
where Π1=a1−c1−d1 and Π2=a3−c3.
Expanding the determinant, we get the characteristic equation for the above 8-dimensional system:
Δ(λ)={[λ2−(Π1+b2)λ+(Π1b2−a2b1)][λ2−(Π2+b4)λ+(Π2b4−a4b3)]−c2c4(λ−b2)(λ−b4)}2 −e−λ(τ1+τ2){d2(λ−b2)[λ2−(Π2+b4)λ+(Π2b4−a4b3)]}2=0. | (3.9) |
Let τ=(τ1+τ2)2 and c=c2c4<0. Then the characteristic equation (3.9) could be simplified as follows:
Δ(λ)=Δ+(λ)Δ−(λ)=0,Δ±(λ)≡[f1(λ)f2(λ)−c(λ−b2)(λ−b4)±e−λτd2(λ−b2)f2(λ)], | (3.10) |
where f1(λ)=λ2−(Π1+b2)λ+(Π1b2−a2b1) and f2(λ)=λ2−(Π2+b4)λ+(Π2b4−a4b3). Since the coefficients of all terms in Δ+(λ) and Δ+(λ) are real, the roots of these functions come in complex conjugate pairs. Thus we may analyze the roots of the full characteristic equation by studying the roots of each factor separately.
We first consider the single neuron case where there is no coupling within the two E-I pairs as well as between two the E cells, that is, gEE=gEI=gIE=0. This implies ci=dj=0 for i=1,2,3,4 and j=1,2 in Eq (3.10). Thus we have
Δ(λ)=[f1(λ)f2(λ)]2=[λ2−(a1+b2)λ+(a1b2−a2b1)]2[λ2−(a3+b4)λ+(a3b4−a4b3)]2=0. |
Note that the discriminant of the quadratic function, f1(λ), is (a1−b2)2+4a2b1 whose sign depends on the specific values of the parameters. As discussed above, the only equilibrium point in this case is the resting equilibrium point, thus a1<0,a3<0. It follows that (a1+b2)<0 and (a1b2−a2b1)>0, which implies that if there are two real roots they are both negative. Even for complex roots, the first condition implies that the real part of complex conjugate roots will be negative. Similarly, the roots of f2(λ) all have negative real part. Thus, the equilibrium point E∗ is stable, which confirms that the neuron is unable to fire or oscillate without any synaptic coupling, representing an excitable system.
Next we consider the coupled system but with a E-I coupling only to investigate how the stability of equilibrium point changes in response to the presence of inhibition from the I cells. This situation corresponds to gEE=0,gEI>0,gIE>0, thus we set dj=0 while keeping nonzero ci in Eq (3.10), which gives
Δ(λ)=[f1(λ)f2(λ)−c(λ−b2)(λ−b4)]2=0. |
From the discussion in the previous section, the only equilibrium point in this case is the resting equilibrium point, thus a1<0,a3<0.
I. λ=0 case: Plugging λ=0 to the above equation results in
Δ(0)=[f1(0)f2(0)−c2b2b4)]2=0. |
Since f1(0)=((a1−c1)b2−a2b1)>0,f2(0)=((a3−c3)b4−a4b3)>0 and b2b4>0,Δ(0)≠0 for any c<0. Thus, the characteristic equation cannot have a zero eigenvalue.
II. λ=±iω case: Without loss of generality, we shall consider λ=iω only as the other case (λ=−iω) will be similar. Plugging λ=iω into Eq (3.10) implies:
Δ+(iω)=Δ−(iω)=(ω2+E1iω−F1)(ω2+E2iω−F2)+c(ω2+E3iω−F3)=0, | (3.11) |
where E1=(Π1+b2),F1=(Π1b2−a2b1),E2=(Π2+b4),F2=(Π2b4−a4b3),E3=(b2+b4),F3=2b2b4. Given all the specified signs in the system (3.7) from Section 3.2, we know that Ei<0,Fi>0 for all i=1,2,3.
Separating Eq (3.11) into real and imaginary parts to rewrite in the form of Δ±(iω)=ℜ±(iω)+iℑ±(iω) gives:
ℜ±(iω)=ω4−(F1+F2+E1E2−c)ω2+(F1F2−cF3),ℑ±(iω)=ω((E1+E2)ω2−(E1F2+E2F1−cE3)). |
To satisfy Δ±(iω)=0 for a nonzero ω∈R, both ℜ±(iω)=0 and ℑ±(iω)=0 should be satisfied. Solving for ℑ±(iω)=0 yields
ω2=(E1F2+E2F1−cE3)/(E1+E2). | (3.12) |
Since both the numerator and denominator in Eq (3.12) are negative, such ω2 should exist. Also, because (F1+F2+E1E2−c)>0,(F1F2−cF3)>0 in ℜ±(iω), there are two positive roots for ω2. Using the quadratic formula, the two solutions could be obtained, however, none of which is the same as ω2 found in Eq (3.12). This implies that there is no ω, for which ℜ±(iω)=ℑ±(iω)=0, i.e., there are no pure imaginary eigenvalues for Eq (3.10). Thus, the equilibrium point E∗ is asymptotically stable if no coupling between E-E is present despite the synaptic coupling between E-I. This result is consistent with our previous study [47], in which no oscillatory solution was found in the case of no delay in the E-I coupling.
Now we study the impact of excitatory coupling on the solution behaviors by considering the fully coupled system with delay. Note that the corresponding characteristic equation with nonzero dj and τ is given in Eq (3.10).
I. λ=0 case: To check the existence of a zero eigenvalue, we plug in λ=0 in Eq (3.10) to obtain
Δ±(0)=f1(0)f2(0)−cb2b4∓b2d2f2(0)=[(Π2b4−a4b3)((Π1∓d2)b2−a2b1)−cb2b4]=0. | (3.13) |
Assume that a1<0 and a3<0. Since Π2b4−a4b3>0,(Π1+d2)b2−a2b1>0 and c,b2,b4<0, Δ−(0)≠0 since d2<0. However, there may exist d∗≡d2=[(Π2b4−a4b3)(Π1b2−a2b1)−cb2b4]/[b2(Π2b4−a4b3)]<0 such that Δ+(0)=0, because the numerator is positive but the denominator is negative. Thus, there may be a zero eigenvalue, indicating that it is possible for the system to have multiple equilibrium solutions. This may also imply that the presence of excitatory synaptic coupling plays an important role in exhibiting other types of solutions in addition to the resting equilibrium solution.
Note that the existence of zero eigenvalue is valid even if there is no coupling delay (i.e., τ=0) between E-E cells because e−λτ=1 for λ=0 in Eq (3.10). In addition, we could consider an even simpler case where the coupling between E-I is additionally removed by setting ci=0 for all i in Eq (3.13). Then, the four-cell system reduces to a pair of coupled E-E cells only as two I cells are completely decoupled from this pair. Based on the same analysis, it follows that a zero eigenvalue can still exist with no coupling between the E and I cells. This result supports previous modeling studies [32,33,34,35,58], in which qualitatively different equilibrium solutions in neural networks were observed regardless of the presence of coupling delay.
II. λ=±iω case: In addition, if we want to check whether the equilibrium loses its stability as the value of τ increases and also to find the critical delay value at which the delay-induced bifurcation occurs, let us consider the case of λ=±iω.
First plugging λ=iω in Eq (3.10), we get
Δ±(iω)=f2(iω)[f1(iω)±e−iτωd2(iω−b2)]−c(iω−b2)(iω−b4)=f2(iω)[f1(iω)±(cos(τω)−isin(τω))d2(iω−b2)]−c(iω−b2)(iω−b4). | (3.14) |
Separating Eq (3.14) into real and imaginary parts, i.e., Δ±(iω)=ℜ±(iω)+iℑ±(iω), and setting each part equal zero:
ℜ±(iω)=±d2(A(ω)cos(τω)+B(ω)sin(τω))+HR(ω)=0,ℑ±(iω)=±d2(B(ω)cos(τω)−A(ω)sin(τω))+HI(ω)=0, | (3.15) |
where
A(ω)=((b2+b4)+Π2)ω2−b2(a3b4−a4b3−b4c3)≡A1ω−A2,B(ω)=−ω3+((a3b4−a4b3−b4c3)−b2(c3−a3−b4))ω≡−ω3+B2ω,HR(ω)=−ω4+(b2b4+[(Π1+Π2)(b2+b4)−a2b1−a4b3]+Π1Π2−c)ω2−[(a3b4−a4b3−b4c3)(b2Π1−a2b1)−b2b4c)]≡−ω4+C2ω2−C3,HI(ω)=−[b2+b4+Π1+Π2]ω3+[(((Π1+Π2)b4−a4b3)b2−a2b1b4)+Π1(Π2(b2+b4)−a4b3)−a2b1Π2−c(b2+b4)]ω≡−D1ω3+D2ω. | (3.16) |
For Δ+(iω), we first gather all the terms of cos(τω) and sin(τω) by rearranging the above two equations in Eq (3.15):
d2(A(ω)cos(τω)+B(ω)sin(τω))=−HR(ω),d2(B(ω)cos(τω)−A(ω)sin(τω))=−HI(ω). |
After algebraic manipulation, we can isolate cos(τω) and sin(τω) in terms of all other parameters.
d2(A(ω)2+B(ω)2)cos(τω)=−(A(ω)HR(ω)+B(ω)HI(ω))≡−C(ω), | (3.17) |
d2(A(ω)2+B(ω)2)sin(τω)=−(B(ω)HR(ω)−A(ω)HI(ω))≡−S(ω). | (3.18) |
To eliminate τ, square the equations in Eqs (3.17) and (3.18), add and simplify to give
HR(ω)2+HI(ω)2−d22(A(ω)2+B(ω)2)=0. | (3.19) |
This is a degree-eight function in ω with coefficients that depend on all the parameters except τ. Dividing Eq (3.18) by Eq (3.17) results in tan(τω)=S(ω)/C(ω). However, this loses information about the signs of cos(τω) and sin(τω) that are in Eqs (3.17)–(3.18). Thus we introduce y=arctan(u) as the branch of the arctangent function with range (−π2,π2). Note that this corresponds to cos(y)>0 and that the function arctan(u)+π corresponds to cos(y)<0. The other branches of the arctangent function are obtained from these two by adding multiple of 2π. As can be seen from Eq (3.17), since d2<0 the sign of cos(τω) is determined by C(ω), and thus we define
τ=τk+(ω)≡1ω{arctan(S(ω)C(ω))+2kπ,C(ω)>0,arctan(S(ω)C(ω))+(2k+1)π,C(ω)<0, | (3.20) |
where k=0,1,….
For Δ−(iω) the only difference is that d2 is replaced by −d2 in Eqs (3.17) and (3.18). Thus, in this case the signs of cos(τω) and C(ω) are the opposite. Hence we have
τ=τk−(ω)≡1ω{arctan(S(ω)C(ω))+2kπ,C(ω)<0,arctan(S(ω)C(ω))+(2k+1)π,C(ω)>0, | (3.21) |
where k=0,1,…. Note that we do not take k<0 as these branches yield τ<0.
Let us finally consider a simpler case of no delay, as similarly discussed in zero eigenvalue section. One can do similar analysis and derive the same result in Eq (3.19) by setting τ=0 in Eqs (3.14) and (3.15) with the functions defined in the expressions of (3.16). It can be easily shown that, for appropriate parameter values, there may exist pure imaginary eigenvalues for the characteristic equation associated with the coupled system with no delay.
To complete this section, we consider the form of the eigenvectors associated with the characteristic equation (3.10). First we rewrite the eigenvalue-eigenvector equation corresponding to the linearization system (3.7) in a slightly different form, which corresponds to reordering the variables as (μ1,η1,μ3,η3,μ2,η2,μ4,η4). Then solutions of the system (3.7) of the form veλt satisfy
M(λ,τ)v=0, | (3.22) |
where
M=(λI−Ad2e−λτ1Bd2e−λτ2BλI−A), |
with I the 4×4 identity matrix and
A=(a1−c1−d1a2−c20b1b200−c40a3−c3a400b3b4),B=(1000000000000000). |
Nontrivial solutions will correspond to values of λ that satisfy the characteristic equation (3.10). Let λ be such a value and v the corresponding solution of Eq (3.22). Define the 8×8 invertible matrix
P(λ)=(I00e(τ1−τ2)λ/2I). |
Then Eq (3.22) implies
0=P−1M(λ)PP−1v=ˆM(λ)ˆv, | (3.23) |
where ˆv=P−1v,
ˆM(λ)=(λI−Ad2e−λτBd2e−λτBλI−A), |
and τ=(τ1+τ2)/2. The matrix ˆM is exactly in the form considered by [27]. Following their approach, let ξ∈R4. Then there is nontrivial vector
ˆv±=(ξ±ξ) |
that satisfies Eq (3.23) if and only if there is a nontrivial ξ that satisfies
(λI−A±d2e−λτB)ξ=0. | (3.24) |
This latter is true if
Δ±(λ)=det(λI−A±d2e−λτB)=0. | (3.25) |
It follows that if λ is root of Δ±(λ) and ξ is a nontrivial vector satisfying Eq (3.24) then the vector
v±=P(λ)(ξ±ξ)=(ξ±e(τ1−τ2)λ/2ξ) |
satisfies Eq (3.22).
For example, suppose that τ1=τ2. If λ=±iω is a root of Δ+(λ) in Eq (3.25) then the corresponding eigenvector will be (ξ,ξ)T, which will yield a periodic solution of the system (3.7) with μ1(t)=μ2(t), μ3(t)=μ4(t) and similarly for νj. However, if λ=±iω is a root of Δ−(λ) then the corresponding eigenvector will be (ξ,−ξ)T. The complex form of the solution will satisfy ei(ω(t+π/ω))v=−ei(ωt)v. Thus the corresponding periodic solution of the system (3.7) will satisfy μ1(t+T/2)=μ2(t),μ3(t+T/2)=μ4(t) where T=2π/ω, and similarly for ηj. So the E-cells are phase-locked with phase difference of T/2, as are the I-cells. A similar analysis if τ1≠τ2 shows that the corresponding solution will be phase locked with phase difference τ1−τ22, if λ=±iω are roots of Δ+(λ), and with phase difference T+τ1−τ22 if λ=±iω are roots of Δ−(λ).
To further our study, we considered the following specific choice for the functions in Eqs (2.1) and (2.2):
F(x,y)=μ(3x−x3)−y+Iapp,G(x,y)=ϵ(γ(1+tanh(β(x−δ)))−y). | (4.1) |
This model is inspired by that of [56]. It has a cubic nonlinearity as for the FitzHugh-Nagumo model [59,60], but with a nonlinearity in the equation for the "recovery" variable which is similar to that for a gating variable in a conductance-based model. We choose the same F and G for both excitatory and inhibitory cells.
The parameters were chosen as follows
μ=0.4,γ=1.75,δ=0.2,ϵ=0.5. | (4.2) |
A bifurcation study of this model shows that with these parameters the parameter β could be used to switch the model from a class 2 (β=1.5) to a class 1 (β=2.0) oscillator, with Iapp used as the parameter. Details can be found in Appendix B. For both values of β the cell is excitable for Iapp small enough, exhibits stable spiking behavior (has a stable limit cycle) for a middle range of Iapp values and has a "high amplitude" stable equilibrium point for Iapp large enough. Typical spiking solutions are show in Appendix B, where it can be seen that that x varies in the range [−2,1] for these solutions.
For our studies of coupled cells, we set Iapp=0 in all cells, so that all cells are excitable and the only input comes from the synapses with other cells. The synaptic coupling function is
s(x)=1/(1+exp(k(θ−u))), |
with k=5 and θ=0.1. The synaptic reversal potentials are xEE=xIE=0.5, and xEI=−2. With this choice xE−xEI>0 for all x on a typical spiking solution while xE/I−0.5<0 for most points of the typical spiking solution. Thus these choices give similar input to the cells as standard values in conductance based models. The coupling strengths were varied as shown below.
To begin, we studied the case when there is no delay in the coupling. That is, we considered the system given by Eqs (2.3)–(2.6), with τ1=τ2=0, FE=FI=F and GE=GI=G where F,G are given by Eq (4.1). We used XPPAUT [52] to carryout numerical bifurcation analysis of the model when the excitatory coupling strength is varied. As shown in Figure 4, there is one equilibrium point that appears to always exist and be asymptotically stable, at least for the range of gEE we considered. This equilibrium point corresponds to all cells being at their resting equilibrium point. In other trials we considered gEE∈[0,700] and this equilibrium point still persisted. Note that, for our parameter values, s(xRE),s(xRI)≈0.0001, and s′(xRE),s′(xRI)≈0.0006. This confirms the analysis of Section 3.1: if the coupling strengths are O(1) with respect to the size of s(xRE),s(xRI),s′(xRE),s′(xRI) the resting equilibrium point exists and is asymptotically stable. At a critical value of the excitatory coupling, gEE, a pair of unstable equilibrium points of the form (x∗E,y∗E,x∗I,y∗I), is created in a saddle-node (fold) bifurcation. For larger gEE, one of these equilibria is stabilized in a Hopf bifurcation. The other appears to always be unstable. The Hopf bifurcation is subcritical and gives rises to an unstable periodic orbit which is destroyed in a homoclinic bifurcation. Varying the other conductances gEI and gIE or the value of β did not affect the structure of the diagram, just the sizes of the equilibrium points and the region of existence of the periodic orbit. See Figure 5. In this figure we see a threshold for g=gEI=gIE. If g<1 the saddle node and Hopf bifurcation occur as the same value as when g=0. Thus the connections between the E and I cells have little effect on the dynamics of the system. Above this threshold the inhibition of the E cells by the I cells has an effect: larger gEE values are needed to cause the saddle node and Hopf bifurations. In these diagrams we kept gEI=gIE, however, varying these independently showed the similar behavior. There are thresholds for these parameters such that both must be above their threshold for inhibition to have an effect.
Next we considered the coupled system with delay, given by Eqs (2.3)–(2.6), with FE=FI=F and GE=GI=G given by Eq (4.1) and the parameters as described above. Using Maple, we numerically solved for the equilibrium points as a function of gEE. This yielded the same curves of equilibrium points as in Figure 4a, which is to be expected since the equilibrium points do not depend on the size of the delays. Next we evaluated the Jacobian of the linearization at the equilibrium points and plotted the curves corresponding to Eqs (3.20) and (3.21) as a function of gEE for two different values of g=gIE=gEI. These are shown in Figure 6. Note that these curves are defined in terms of the mean delay τ=τ1+τ22. The blue curves correspond to the highest equilibrium point in Figure 4a, with the thin curves corresponding to the Δ+ factor in Eq (3.10) having a pair of pure imaginary eigenvalues and the thick to the Δ− factor. The cyan curves correspond the middle equilibrium point. For all parameter values we considered, the middle equilibrium point was always unstable, thus these curves will not affect the observable dynamics. As noted above, for our choice of parameter values we expect the low (resting) equilibrium point to be asymptotically stable for gEE in the range we consider. The black vertical line corresponds to the characteristic equation having a zero root. This value is independent of the delay and corresponds to the saddle-node bifurcation points in Figure 4.
The high equilibrium point is asymptotically stable in the region to the right of all the blue curves. This follows from the fact that for τ=0 the high equilibrium point is stable to the right of the intersection point of the lowest thin blue curve with the gEE axis. This point corresponds to the Hopf bifurcation at gEE=gHEE∼7.18 in Figure 4a and at gEE=gHEE∼8.9 in Figure 4b. Consider Figure 6a. For any fixed gEE>gHEE as τ is increased the high equilibrium point will lose stability at the first thick blue curve. If gEE is large enough then no curve is crossed for any τ>0 and the equilibrium point remains stable. If gEE<gHEE then the high equilibrium point will gain stability at the first thin blue curve then lose it at the first thick blue curve. The effect of inhibition can be seen by comparing Figure 6a, b. While qualitatively, the figures are similar, there are some quantitative differences. From a purely geometric perspective, larger gEI and gIE shift Figure 6a to the right, stretch it horizontally and compress it vertically. The biological implication is that if the E-I coupling is stronger, then stronger E-E coupling is needed to create the nonresting equilibrium points (as seen for zero delay in Figure 4). However, smaller delays are needed to induce the Hopf bifurcations.
We expect that the high equilibrium point will undergo a Hopf bifurcation at τ values corresponding to the blue curves. The relative phases of the two cell populations on the resulting periodic orbits will be determined by the eigenvector structure. For example, if τ1=τ2 then the eigenvector corresponding to a pair of pure imaginary roots of Δ+(λ) will be of the form (ξ,ξ)T. Thus the periodic orbits emanating from Hopf bifurcations along the thin blue curves will be of in-phase type: xE1(t)=xE2(t), xI1(t)=xI2(t), yE1(t)=yE2(t) and yI1(t)=yI2(t). We refer to these as synchronized solutions since the corresponding variables of each cell type are synchronized. In general there will be phase-locking with non-zero phase difference between different variables of a given cell type (e.g., xE1 vs. yE1) and between the same variables of different cell types (e.g., xE1 vs. xI1). Along the thick blue curves, the roots correspond to Δ−(λ) and the corresponding eigenvector is of the form (ξ,−ξ)T. Thus the corresponding periodic orbits will be of anti-phase type that is xE1(t)=xE2(t+T/2) and xI1(t)=xI2(t+T/2) and similarly for the y variables. This follows from symmetric Hopf bifurcation theory [27,61,62].
Finally, we note that the intersections of the blue lines correspond to points where the high equilibrium point has two pairs of pure imaginary eigenvalues, while the intersection points of the black line with the blue and cyan curves corresponds to points where there is an equilibrium point with one zero eigenvalue and a pair of pure imaginary eigenvalues. These correspond to Hopf-Hopf bifurcations and saddle-node(fold)/Hopf bifurcations, respectively [63,64]. While such codimension two bifurcations are relatively rare in ordinary differential equation models, they are not unusual in delay differential equation models [27,33,34,35,65].
To verify the predictions about Hopf bifurcations and periodic solutions, we use the numerical continuation software DDE-BIFTOOL [53]. In addition to locating Hopf bifurcation points, this software can numerically compute the coefficient of the normal form to determine the criticality of the Hopf bifurcation. Applying the DDE-BIFTOOL to Eqs (2.3)–(2.6), with the functions in Eq (4.1), the parameter values in the expressions (4.2) and τ1=τ2≡τ, we confirmed the curves in Figure 6 are indeed curves of Hopf bifurcation. The bifurcations of the high equilibrium point were found to be always subcritical while those of the middle equilibrium point were supercritical. The periodic orbits produced by the bifurcation of the middle equilibrium point are unstable since the equilibrium point is unstable before the bifurcation.
We also used DDE-BIFTOOL to follow branches of periodic solutions emanating from the Hopf bifurcation points. Figure 7a shows branches of periodic solutions aring from various branches of Hopf bifurcations at gEE≈7.2 using τ1=τ2≡τ as the bifurcation parameter. Note that, the Hopf bifurcations are subcritical and produce unstable periodic orbits which coexist with the stable equilibrium point. However, in almost all cases, these branches undergo a saddle node of periodic orbits giving rise to stable periodic orbits. The far left curve in Figure 7a starts from the Hopf point on lowest thick blue curve in Figure 6a at τ≈1.5. The far right curve in Figure 7a (which remains unstable) starts from the same thick blue curve but at τ≈2.7. The other curve of periodic solutions starts from the Hopf point on the thin blue curve at τ≈3.2. Figure 7b shows the form of the periodic solutions for xE1 and xE2 at particular points on the branches. Other points on the same branch produced different shapes of periodic orbits, but the phase relationship between xE1 and xE2 remains the same. Note that the phase relationships correspond with the predictions of our analysis, namely, the periodic solutions arising from the thick blue curves give rise to anti-phase periodic solutions and those arising from the thin blue curves to in-phase (synchronized) solutions.
Figure 8 verifies this from a different perspective. It shows two branches of periodic solutions with gEE as the bifurcation parameter, arising from to two different Hopf branches. The upper branch of periodic solutions in Figure 8a corresponds to thin blue Hopf curve in Figure 6a which passes through τ=4.1864 when gEE≈7.23. The corresponding periodic solution in Figure 8b is of in-phase type as predicted by our analysis. The lower branch of periodic solutions in Figure 8a corresponds to the thick blue Hopf curve in Figure 6a which passes through τ=1.9184 when gEE≈7.23. The corresponding periodic solution in Figure 8b is of anti-phase type as predicted.
Finally, we confirmed the predictions of our analysis using numerical simulations of the model in XPPAUT [52]. Some examples are show in Figure 9. Here we fixed gEE=7.2>gHEE. In all simulations the initial conditions were
xE1=−1,xE2=−1.2,xI1=−1,xI2=−1.1,yEj=yIj=0, −max(τ1,τ2)≤t≤0, |
which leads to the low (resting) equilibrium point which is asymptotically stable. A brief stimulation, using the applied current Iapp is applied (for details see the caption of Figure 9) and the solution switches to another asymptotically stable solution. For τ sufficiently small the high equilibrium point is asymptotically stable (not shown). At τ∼1.3 (on the thick blue line) the high equilibrium point loses stability. If τ1=τ2, the resulting periodic orbit is predicted to be of anti-phase type. Figure 9a confirms this. As τ is increased further the thick blue line is crossed again (which restabilizes the equilibrium point) and then the think blue line is crossed. This latter results in a Hopf bifurcation leading to a periodic orbit which is predicted to be of in-phase (synchronized) type for the case τ1=τ2. Figure 9b confirms this. Note that both periodic orbits are large amplitude which is consistent Figures 7 and 8. If τ1≠τ2 the Hopf bifurcations will still occur at the values of (τ1+τ2)/2 indicated by Figure 6, however, the phase relationship of the resulting periodic solution will be different. This is illustrated in Figure 9c, d where solutions are shown which have with the same parameters and value of τ1+τ2 as in Figure 9a, b, but τ1≠τ2. In all cases, the solution can be switched from the oscillatory solution back to the resting equilibrium point by a transient stimulus to the inhibitory cells (not shown).
In this paper, we study a model for a network of excitable neurons with excitatory and inhibitory synaptic coupling to investigate the role of coupling delays in the existence and stability of different types of oscillatory network behavior. Unlike many neural network models, a single, uncoupled neuron in our neural system is assumed to have zero applied current so that the synaptic coupling between neurons is the main factor to give rise to network behavior. We argue that the resting equilibrium point, i.e., the equilibrium where all the neurons are at their uncoupled rest state, should persist and remain asymptotically stable in the presence of coupling, but that sufficiently large excitatory coupling can give rise to new equilibria. We then use linear stability analysis of these equilibria to show that bifurcations are only expected when there is coupling between the excitatory neurons. We show that the stability only depends on the average of the delays between the excitatory neurons and give explicit expressions for values of the average delay where the equilibria lose stability via a pair of pure imaginary eigenvalues. We show that these expressions come in two types, which correspond to two factors in the characteristic equation. We then analyze the eigenvector structure to predict the type of oscillatory patterns that would emerge in the corresponding delay-induced Hopf bifurcations. To our knowledge, this is the first article to carry out stability and bifurcation analyses on such a system.
We apply our analytical results to an example model inspired by that of Terman and Wang [56]. We solve for the equilibria of the model and show that the resting point always persists, while for sufficiently large excitatory coupling a saddle node bifurcation occurs giving rise to two other equilibria. We find curves of potential Hopf bifurcation of these equilibria in the parameter space of the average delay and excitatory coupling strength, and show the two types described above alternate as the delay increases.
We supplement and verify our analytical results using numerical simulations and numerical bifurcation analysis. Using DDE-BIFTOOL [53] we verify that the curves of pure imaginary eigenvalues are indeed Hopf bifurcations. For the case of equal delays, the two types of Hopf bifurcation give rise to periodic solutions where corresponding cells are in-phase (synchronized) and anti-phase. We find sets of parameter values where both types of periodic solutions exist and are asymptotically stable. Using numerical simulations in XPPAUT [52], we show that if the delays are not equal, but the average delay remains the same, the oscillation patterns are transformed as predicted by the eigenvector analysis.
This study extends our previous work [47] in that the neural networks considered herein include two distinct pairs of excitatory and inhibitory neurons, which are coupled through excitatory synapses. Previously, we considered the global inhibitory network where an excitatory population is uncoupled but connected to a global inhibitory neuron only. Thus, our study exhibits other types of network behaviors such as solutions where the excitatory cells are in anti-phase, in addition to synchronization among excitatory neurons. Here, we also consider a general network of non-relaxation oscillators unlike our previous study. Moreover, we extend our previous work by conducting numerical bifurcation analysis based on DDE-BIFTOOL, in which we show the coupling delay plays a significant role in generating various types of periodic solutions as a result of Hopf bifurcations.
An important finding in the current work is that the resting equilibrium point is unaffected by the coupling. This is due to the fact that the synaptic coupling is effectively zero when the neuron is at rest, which is a common feature of biophysical models of neurons. Thus all the delay-induced bifurcations involve nontrivial equilibria which are induced by the excitatory coupling and the resultant stable periodic solutions coexist with the stable resting equilibrium. This is in contrast to studies of neural networks with delayed diffusive or sigmoidal coupling where the resting/trivial equilibrium point undergoes the delay-induced bifurcations and becomes unstable [27,31,32,33,34,35,36,37,38,39].
Our results about Hopf bifurcation leading to in-phase and anti-phase periodic solutions, for the case of symmetric delays in the E-E connections, mirror those found in many systems of two coupled identical oscillators [31,33,34,36,37,39,40,41]. Here we have shown that these results persist in models with synaptic coupling and even if the resting (trivial) equilibrium remains asymptotically stable. Further, we have shown how these results may be extended to the case of non-symmetric delays.
Our results have interesting implications for how rhythms may arise in biological neural networks. Rhythms are associated with frequencies that are observed at the network level as opposed to the individual neuron level. One way that these can arise is through phase-locked oscillatory solutions [6,66]. For example, in our small network in-phase (synchronized) oscillations give rise to a network frequency equal to the frequency of the individual neurons, while anti-phase oscillations give rise to a network frequency twice that of the individual neurons. Several studies have shown that rhythms can arise in networks of oscillatory neurons [6,66], however, these rhythms are generally always present unless a parameter changes. In our model, due to the bistability between the resting equilibrium and the oscillatory solutions, the network can transition between a non-rhythmic state and a rhythmic state, simply through a transient input. Further, we observe parameter ranges where there is tristability between the resting equilibrium point and two different oscillatory patterns (in-phase and anti-phase). In this parameter regime the system can transition from the non-rhythmic activity to two different rhythmic states, depending on the input.
Model limitations and future directions. Despite the richness of our analytical and numerical results, our study is based on the small-size network with four, two excitatory and two inhibitory, neurons only resulting in two excitatory-inhibitory pairs. The results for two neurons with symmetric delays have been extended to larger networks with circulant coupling in [27]. We expect that we can use a similar approach to extend our results for non-symmetric delays to these types of larger networks.
In addition, there is only one location where coupling delays are assumed to arise, which is in synapses between excitatory cells in different populations. Though our study considers asymmetric delay case among excitatory neurons but additional delays between coupled excitatory and inhibitory cells are needed to obtain a more complete understanding of a realistic neural network. We could also include delays within a population to better represent the synaptic delay between neurons. Further, in some brain networks there exist long-range connections from excitatory cells in one population to inhibitory cells in the other [6,14]. If such connections were added to our model, it would be necessary to include the corresponding coupling delay. Thus, our future study would extend the current model to investigate the impact of delays between different types of neurons on the observed network behaviors in this study.
Our work here focused on models with one "activity" variable and one "gating" variable. However, as shown in [27] the linear stability analysis we use could be applied to more general conductance-based models with multiple gating variables.
Finally, real neurons are not likely to be perfectly symmetric. In other studies without delay, it has been shown that some results for symmetric systems persist, but new phenomena can also occur [67,68]. Thus it would be interesting to include the effects of heterogeneity in neural parameters in a future study.
SAC acknowledges the support of the Natural Sciences and Engineering Research Council of Canada.
The authors declare that they have no known competing financial interests or personal relationships that could have appeared to influence the work reported in this paper.
In this paper we consider a single cell model inspired by that of Terman and Wang [56]. The model is based on the FitzHugh-Nagumo [59,60] model but with a nonlinearity in equation for the "recovery" variable which is similar to that for a gating variable in a conductance-based model. The equations for this model are as follows
x′=μ(3x−x3)−y+Iapp,y′=ϵ(γ(1+tanh(β(x−δ)))−y). |
This nonlinearity for the recovery variable means that the model may act either as a class Ⅰ and class Ⅱ oscillator, depending on the parameter values.
For the parameters used in this study, that is, given in the expressions (4.2), the parameter β can be used to switch the model from a class 2 to a class 1 oscillator, with Iapp used as the bifurcation parameter. This can be seen in Figure 10, which shows a one parameter bifurcation diagram of the system when Iapp is used as the bifurcation parameter.
When β=1.5 there is a unique equilibrium point which goes loses stability in a subcritical Hopf bifurcation. A stable periodic orbit is created by a saddle node of periodic orbits. When β=2.0 there are up to three equilibrium points and a stable periodic orbit is created in a saddle node on an invariant circle bifurcation. In both cases the cell is excitable for Iapp small enough, exhibits stable spiking behavior (has a stable limit cycle) for a mid range of Iapp values and has a "high amplitude" stable equilibrium point for Iapp large enough. Typical spiking solutions are shown in Figure 11. Note that the x varies in the range [−2,1] for these solutions.
[1] | P. Dayan, L. Abbott, Theoretical Neuroscience, MIT Press, Cambridge, MA, 2001. |
[2] | G. Ermentrout, D. Terman, Mathematical Foundations of Neuroscience, Springer, New York, NY, 2010. |
[3] | E. M. Izhikevich, Dynamical Systems in Neuroscience, MIT Press, Cambridge, MA, 2007. |
[4] | N. Brunel, X. J. Wang, What determines the frequency of fast network oscillations with irregular neural discharges? I. synaptic dynamics and excitation-inhibition balance, J. Neurophysiol., 90 (2003), 415-430. |
[5] |
G. B. Ermentrout, N. Kopell, Fine structure of neural spiking and synchronization in the presence of conduction delays, Proc. Natl. Acad. Sci., 95 (1998), 1259-1264. doi: 10.1073/pnas.95.3.1259
![]() |
[6] |
N. Kopell, G. Ermentrout, M. Whittington, R. Traub, Gamma rhythms and beta rhythms have different synchronization properties, Proc. Natl. Acad. Sci., 97 (2000), 1867-1872. doi: 10.1073/pnas.97.4.1867
![]() |
[7] |
J. E. Rubin, D. Terman, Analysis of clustered firing patterns in synaptically coupled networks of oscillators, J. Math. Biol., 41 (2000), 513-545. doi: 10.1007/s002850000065
![]() |
[8] |
B. Pfeuty, G. Mato, D. Golomb, D. Hansel, The combined effects of inhibitory and electrical synapses in synchrony, Neural Comput., 17 (2005), 633-670. doi: 10.1162/0899766053019917
![]() |
[9] |
M. A. Whittington, R. Traub, N. Kopell, B. Ermentrout, E. Buhl, Inhibition-based rhythms: experimental and mathematical observations on network dynamics, Int. J. Psychophysiol., 38 (2000), 315-336. doi: 10.1016/S0167-8760(00)00173-2
![]() |
[10] |
J. E. Rubin, D. Terman, Geometric analysis of population rhythms in synaptically coupled neuronal networks, Neural Comput., 12 (2000), 597-645. doi: 10.1162/089976600300015727
![]() |
[11] | J. E. Rubin, D. Terman, Geometric singular perturbation analysis of neuronal dynamics, Handbook Dyn. Syst., 2 (2002), 93-146. |
[12] |
C. D. Acker, N. Kopell, J. A. White, Synchronization of strongly coupled excitatory neurons: relating network behavior to biophysics, J. Comput. Neurosci., 15 (2003), 71-90. doi: 10.1023/A:1024474819512
![]() |
[13] | P. J. Hellyer, B. Jachs, C. Clopath, R. Leech, Local inhibitory plasticity tunes macroscopic brain dynamics and allows the emergence of functional brain networks, Neuroimage, 24 (2016), 85-95. |
[14] |
E. M. Izhikevich, G. M. Edelman, Large-scale model of mammalian thalamocortical systems, Proc. Natl. Acad. Sci., 105 (2008), 3593-3598. doi: 10.1073/pnas.0707563105
![]() |
[15] |
M. Bazhenov, N. F. Rulkov, I. Timofeev, Effect of synaptic connectivity on long-range synchronization of fast cortical oscillations, J. Neurophysiol., 100 (2008), 1562-1575. doi: 10.1152/jn.90613.2008
![]() |
[16] |
A. Ghosh, Y. Rho, A. McIntosh, R. Kötter, V. Jirsa, Cortical network dynamics with time delays reveals functional connectivity in the resting brain, Cognitive Neurodyn., 2 (2008), 115. doi: 10.1007/s11571-008-9044-2
![]() |
[17] |
D. Guo, Q. Wang, M. Perc, Complex synchronous behavior in interneuronal networks with delayed inhibitory and fast electrical synapses, Phys. Rev. E, 85 (2012), 061905. doi: 10.1103/PhysRevE.85.061905
![]() |
[18] |
T. Pérez, G. C. Garcia, V. M. Eguiluz, R. Vicente, G. Pipa, C. Mirasso, Effect of the topology and delayed interactions in neuronal networks synchronization, PLoS ONE, 6 (2011), e19900. doi: 10.1371/journal.pone.0019900
![]() |
[19] | X. Sun, L. Guofang, Fast regular firings induced by intra- and inter-time delays in two clustered neuronal networks, Chaos, 28 (2017), 106310. |
[20] |
Q. Wang, Q. Lu, G. Chen, Synchronization transition induced by synaptic delay in coupled fastspiking neurons, Int. J. Bifurcat. Chaos, 18 (2008), 1189-1198. doi: 10.1142/S0218127408020914
![]() |
[21] |
S. Crook, G. Ermentrout, M. Vanier, J. Bower, The role of axonal delay in synchronization of networks of coupled cortical oscillators, J. Comput. Neurosci., 4 (1997), 161-172. doi: 10.1023/A:1008843412952
![]() |
[22] |
P. Bressloff, S. Coombes, Symmetry and phase-locking in a ring of pulse-coupled oscillators with distributed delays, Physica D, 126 (1999), 99-122. doi: 10.1016/S0167-2789(98)00264-4
![]() |
[23] |
P. Bressloff, S. Coombes, Travelling waves in chains of pulse-coupled integrate-and-fire oscillators with distributed delays, Physica D, 130 (1999), 232-254. doi: 10.1016/S0167-2789(99)00013-5
![]() |
[24] |
T. W. Ko, G. B. Ermentrout, Effects of axonal time delay on synchronization and wave formation in sparsely coupled neuronal oscillators, Phys. Rev. E, 76 (2007), 056206. doi: 10.1103/PhysRevE.76.056206
![]() |
[25] |
S. A. Campbell, Z. Wang, Phase models and clustering in networks of oscillators with delayed coupling, Physica D, 363 (2018), 44-55. doi: 10.1016/j.physd.2017.09.004
![]() |
[26] |
G. Orosz, Decomposition of nonlinear delayed networks around cluster states with applications to neurodynamics, SIAM J. Appl. Dyn. Syst., 13 (2014), 1353-1386. doi: 10.1137/130915637
![]() |
[27] |
Z. Wang, S. A. Campbell, Symmetry, Hopf bifurcation, and the emergence of cluster solutions in time delayed neural networks, Chaos, 27 (2017), 114316. doi: 10.1063/1.5006921
![]() |
[28] |
D. Golomb, G. B. Ermentrout, Continuous and lurching traveling pulses in neuronal networks with delay and spatially decaying connectivity, Proc. Natl. Acad. Sci., 96 (1999), 13480-13485. doi: 10.1073/pnas.96.23.13480
![]() |
[29] |
D. Golomb, G. B. Ermentrout, Effects of delay on the type and velocity of travelling pulses in neuronal networks with spatially decaying connectivity, Network: Comput. Neural Syst., 11 (2000), 221-246. doi: 10.1088/0954-898X_11_3_304
![]() |
[30] |
A. Roxin, N. Brunel, D. Hansel, Role of delays in shaping spatiotemporal dynamics of neuronal activity in large networks, Phys. Rev. Lett.s, 94 (2005), 238103. doi: 10.1103/PhysRevLett.94.238103
![]() |
[31] | M. Dahlem, G. Hiller, A. Panchuk, E. Schöll, Dynamics of delay-coupled excitable neural systems, Int. J. Bifurc. Chaos, 29 (2009), 745-753. |
[32] |
N. Burić, I. Grozdanović, N. Vasović, Type I vs type II excitable systems with delayed coupling, Chaos Solitons Fractals, 23 (2005), 1221-1233. doi: 10.1016/j.chaos.2004.06.033
![]() |
[33] | N. Burić, D. Todorović, Dynamics of Fitzhugh-Nagumo excitable systems with delayed coupling, Phys. Rev. E, 67 (2003), 066-222. |
[34] |
N. Burić, D. Todorović, Bifurcations due to small time-lag in coupled excitable systems, Int. J. Bifurc. Chaos, 15 (2005), 1775-1785. doi: 10.1142/S0218127405012831
![]() |
[35] | S. A. Campbell, R. Edwards, P. van den Dreissche, Delayed coupling between two neural network loops, SIAM J. Appl. Math., 65 (2004), 316-335. |
[36] |
E. Schöll, G. Hiller, P. Hövel, M. A. Dahlem, Time-delay feedback in neurosystems, Phil. Trans. Roy. Soc. A, 367 (2009), 1079-10956. doi: 10.1098/rsta.2008.0258
![]() |
[37] |
C. U. Choe, T. Dahms, P. Hovel, E. Schöll, Controlling synchrony by delay coupling in networks: From in-phase to splay and cluster states, Phys. Rev. E, 81 (2010), 025205. doi: 10.1103/PhysRevE.81.025205
![]() |
[38] |
T. Dahms, J. Lehnert, E. Scholl, Cluster and group synchronization in delay-coupled networks, Phys. Rev. E, 86 (2012), 016202. doi: 10.1103/PhysRevE.86.016202
![]() |
[39] |
J. Lehnert, T. Dahms, P. Hovel, E. Schöll, Loss of synchronization in complex neuronal networks with delay, EPL (Europhys. Lett.), 96 (2011), 60013. doi: 10.1209/0295-5075/96/60013
![]() |
[40] |
Y. Song, J. Xu, Inphase and antiphase synchronization in a delay-coupled system with applications to a delay-coupled Fitzhugh-Nagumo system, IEEE Trans. Neural. Netw. Learn. Syst., 23 (2012), 1659-1670. doi: 10.1109/TNNLS.2012.2209459
![]() |
[41] | A. Panchuk, D. Rosin, P. Hovel, E. Schöll, Synchronization of coupled neural oscillators with heterogeneous delays, Int. J. Bifurc. Chaos, 23. |
[42] |
S. R. Campbell, D. Wang, Relaxation oscillators with time delay coupling, Physica D, 111 (1998), 151-178. doi: 10.1016/S0167-2789(97)80010-3
![]() |
[43] |
J. J. Fox, C. Jayaprakash, D. Wang, S. R. Campbell, Synchronization in relaxation oscillator networks with conduction delays, Neural Comput., 13 (2001), 1003-1021. doi: 10.1162/08997660151134307
![]() |
[44] | A. Bose, S. Kunec, Synchrony and frequency regulation by synaptic delay in networks of selfinhibiting neurons, Neurocomputing, 38 (2001), 505-513. |
[45] |
S. Kunec, A. Bose, Role of synaptic delay in organizing the behavior of networks of self-inhibiting neurons, Phys. Rev. E, 63 (2001), 021908. doi: 10.1103/PhysRevE.63.021908
![]() |
[46] |
S. Kunec, A. Bose, High-frequency, depressing inhibition facilitates synchronization in globally inhibitory networks, Network: Comput. Neural Syst., 14 (2003), 647-672. doi: 10.1088/0954-898X_14_4_303
![]() |
[47] | H. Ryu, S. A. Campbell, Geometric analysis of synchronization in neuronal networks with global inhibition and coupling delays, Phil. Trans. R. Soc. A, 337 (2019), 20180129. |
[48] | X. Ji, J. Lu, J. Lou, J. Qiu, K. Shi, A unified criterion for global exponential stability of quaternionvalued neural networks with hybrid impulses, Int. J. Robust Nonlinear Control, 2020. |
[49] | B. Sun, Y. Cao, Z. Guo, Z. Yan, S. Wen, Synchronization of discrete-time recurrent neural networks with time-varying delays via quantized sliding mode control, Appl. Math. Comput., 375 (2020), 125093. |
[50] |
Y. Tian, Z. Wang, Stability analysis for delayed neural networks based on the augmented Lyapunov-Krasovskii functional with delay-product-type and multiple integral terms, Neurocomputing, 410 (2020), 295-303. doi: 10.1016/j.neucom.2020.05.045
![]() |
[51] |
J. Xiao, Z. Zeng, A. Wu, S. Wen, Fixed-time synchronization of delayed Cohen-Grossberg neural networks based on a novel sliding mode, Neural Networks, 128 (2020), 1-12. doi: 10.1016/j.neunet.2020.04.020
![]() |
[52] | E. Ermentrout, Simulating, Analyzing, and Animating Dynamical Systems: a Guide to XPPAUT for Researchers and Students, SIAM, 2002. |
[53] | K. Engelborghs, T. Luzyanina, G. Samaey, DDE-BIFTOOL v. 2.00: a Matlab Package for Bifurcation Analysis of Delay Differential Equations, Technical Report TW-330, Department of Computer Science, K.U. Leuven, Leuven, Belgium, 2001. |
[54] |
C. Morris, H. Lecar, Voltage oscillations in the barnacle giant muscle fibre, Biophysical J., 35 (1981), 193-213. doi: 10.1016/S0006-3495(81)84782-0
![]() |
[55] | J. Rinzel, Excitation dynamics: insights from simplified membrane models, Fed. Proc, 44 (1985), 2944-2946. |
[56] |
D. Terman, D. Wang, Global competition and local cooperation in a network of neural oscillators, Physica D, 81 (1995), 148-176. doi: 10.1016/0167-2789(94)00205-5
![]() |
[57] | J. Hale, S. Verduyn Lunel, Introduction to Functional Differential Equations, Springer Verlag, New York, 1993. |
[58] | Z. Wang, Clustering of Networks with Time Delayed All-to-all Coupling, Ph.D thesis, University of Waterloo, 2017, |
[59] |
R. Fitzhugh, Impulses and physiological states in theoretical models of nerve membrane, Biophysical J., 1 (1961), 445-466. doi: 10.1016/S0006-3495(61)86902-6
![]() |
[60] |
J. Nagumo, S. Arimoto, S. Yoshizawa, An active pulse transmission line simulating nerve axon, Proceeding IRE, 50 (1962), 2061-2070. doi: 10.1109/JRPROC.1962.288235
![]() |
[61] | M. Golubitsky, I. Stewart, D. G. Scherffer, Singularities and Groups in Bifurcation Theory, Springer-Verlag, New York, 1988. |
[62] |
J. Wu, Symmetric functional-differential equations and neural networks with memory, Trans. Amer. Math. Soc., 350 (1998), 4799-4838. doi: 10.1090/S0002-9947-98-02083-2
![]() |
[63] | J. Guckenheimer, P. Holmes, Nonlinear Oscillations, Dynamical Systems and Bifurcations of Vector Fields, Springer-Verlag, New York, 1983. |
[64] | Y. A. Kuznetsov, Elements of Applied Bifurcation Theory, Springer Science & Business Media, 2013. |
[65] |
J. Bélair, S. A. Campbell, Stability and bifurcations of equilibria in a multiple-delayed differential equation, SIAM J. Appl. Math., 54 (1994), 1402-1424. doi: 10.1137/S0036139993248853
![]() |
[66] |
Z. Kilpatrick, B. Ermentrout, Sparse gamma rhythms arising through clustering in adapting neuronal networks, PLoS Comput. Biol., 7 (2011), e1002281. doi: 10.1371/journal.pcbi.1002281
![]() |
[67] |
S. A. Campbell, M. Waite, Multistability in coupled Fitzhugh-Nagumo oscillators, Nonlinear Anal., 47 (2001), 1093-1104. doi: 10.1016/S0362-546X(01)00249-8
![]() |
[68] |
F. K. Skinner, H. Bazzazi, S. A. Campbell, Two-cell to N-cell heterogeneous, inhibitory networks: precise linking of multistable and coherent properties, J. Comput. Neurosci., 18 (2005), 343-352. doi: 10.1007/s10827-005-0331-1
![]() |
1. | L. Chen, S.A. Campbell, Hysteresis bifurcation and application to delayed FitzHugh-Nagumo neural systems, 2021, 500, 0022247X, 125151, 10.1016/j.jmaa.2021.125151 | |
2. | I. S. Proskurkin, V. K. Vanag, Random Decision-Making in Networks of Pulse-Coupled Spike Oscillators, 2022, 83, 0005-1179, 935, 10.1134/S0005117922060108 | |
3. | R. Loula, L. H. A. Monteiro, Monoamine neurotransmitters and mood swings: a dynamical systems approach, 2022, 19, 1551-0018, 4075, 10.3934/mbe.2022187 | |
4. | Vladimir K. Vanag, Plasticity in networks of active chemical cells with pulse coupling, 2022, 32, 1054-1500, 123108, 10.1063/5.0110190 | |
5. | Vladimir K. Vanag, Ivan S. Proskurkin, Implementation of Hebb's rules in a network of excitable chemical cells coupled by pulses, 2023, 25, 1463-9076, 17420, 10.1039/D3CP01238G | |
6. | I. Pinder, M. R. Nelson, J. J. Crofts, Bifurcations and synchrony in a ring of delayed Wilson–Cowan oscillators, 2023, 479, 1364-5021, 10.1098/rspa.2023.0313 | |
7. | Isam Al-Darabsah, Sue Ann Campbell, Bootan Rahman, Distributed Delay and Desynchronization in a Neural Mass Model, 2024, 23, 1536-0040, 3013, 10.1137/23M1618028 | |
8. | Gaurav Dar, High-order synchronization in identical neurons with asymmetric pulse coupling, 2025, 111, 2470-0045, 10.1103/PhysRevE.111.034202 |