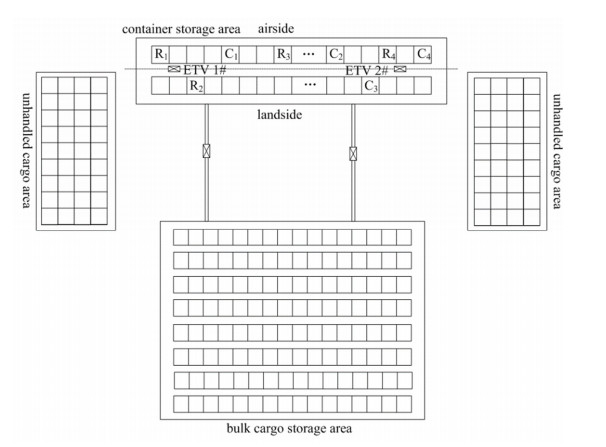
The problem of the onset of Marangoni bio-thermal convection is investigated for a horizontal layer of fluid containing motile gyrotactic microorganisms. The fluid layer is assumed to rest on a rigid surface with fixed temperature and the top boundary of the layer is assumed to be a free non deformable surface. The resulting equations of the problem constitute an eigenvalue problem which is solved using the Chebyshev tau numerical method. The critical values of the thermal Marangoni number are calculated for several values of the bioconvection Péclet number, bioconvection Marangoni number, bioconvection Lewis number and gyrotaxis number. The results of this study showed that the existence of gyrotactic microorganisms increases the critical thermal Marangoni numbers. Moreover, the critical eigenvalues obtained were real-valued indicating that the mode of instability is via a stationary mode, however oscillatory mode is possible for some ranges of the parameters values.
Citation: Latifa I. Khayyat, Abdullah A. Abdullah. The onset of Marangoni bio-thermal convection in a layer of fluid containing gyrotactic microorganisms[J]. AIMS Mathematics, 2021, 6(12): 13552-13565. doi: 10.3934/math.2021787
[1] | Zhenao Yu, Peng Duan, Leilei Meng, Yuyan Han, Fan Ye . Multi-objective path planning for mobile robot with an improved artificial bee colony algorithm. Mathematical Biosciences and Engineering, 2023, 20(2): 2501-2529. doi: 10.3934/mbe.2023117 |
[2] | Mingming Zhang, Huiyuan Jin, Ying Yang . ECG classification efficient modeling with artificial bee colony optimization data augmentation and attention mechanism. Mathematical Biosciences and Engineering, 2024, 21(3): 4626-4647. doi: 10.3934/mbe.2024203 |
[3] | Xiangyang Ren, Xinxin Jiang, Liyuan Ren, Lu Meng . A multi-center joint distribution optimization model considering carbon emissions and customer satisfaction. Mathematical Biosciences and Engineering, 2023, 20(1): 683-706. doi: 10.3934/mbe.2023031 |
[4] | Shaofeng Yan, Guohui Zhang, Jinghe Sun, Wenqiang Zhang . An improved ant colony optimization for solving the flexible job shop scheduling problem with multiple time constraints. Mathematical Biosciences and Engineering, 2023, 20(4): 7519-7547. doi: 10.3934/mbe.2023325 |
[5] | Tian Xue, Liu Li, Liu Shuang, Du Zhiping, Pang Ming . Path planning of mobile robot based on improved ant colony algorithm for logistics. Mathematical Biosciences and Engineering, 2021, 18(4): 3034-3045. doi: 10.3934/mbe.2021152 |
[6] | Jun Chen, Gloria DeGrandi-Hoffman, Vardayani Ratti, Yun Kang . Review on mathematical modeling of honeybee population dynamics. Mathematical Biosciences and Engineering, 2021, 18(6): 9606-9650. doi: 10.3934/mbe.2021471 |
[7] | Mehrdad Ahmadi Kamarposhti, Ilhami Colak, Kei Eguchi . Optimal energy management of distributed generation in micro-grids using artificial bee colony algorithm. Mathematical Biosciences and Engineering, 2021, 18(6): 7402-7418. doi: 10.3934/mbe.2021366 |
[8] | Xue Li, Lei Wang . Application of improved ant colony optimization in mobile robot trajectory planning. Mathematical Biosciences and Engineering, 2020, 17(6): 6756-6774. doi: 10.3934/mbe.2020352 |
[9] | Huawei Jiang, Shulong Zhang, Tao Guo, Zhen Yang, Like Zhao, Yan Zhou, Dexiang Zhou . Improved whale swarm algorithm for solving material emergency dispatching problem with changing road conditions. Mathematical Biosciences and Engineering, 2023, 20(8): 14414-14437. doi: 10.3934/mbe.2023645 |
[10] | Chikun Gong, Yuhang Yang, Lipeng Yuan, Jiaxin Wang . An improved ant colony algorithm for integrating global path planning and local obstacle avoidance for mobile robot in dynamic environment. Mathematical Biosciences and Engineering, 2022, 19(12): 12405-12426. doi: 10.3934/mbe.2022579 |
The problem of the onset of Marangoni bio-thermal convection is investigated for a horizontal layer of fluid containing motile gyrotactic microorganisms. The fluid layer is assumed to rest on a rigid surface with fixed temperature and the top boundary of the layer is assumed to be a free non deformable surface. The resulting equations of the problem constitute an eigenvalue problem which is solved using the Chebyshev tau numerical method. The critical values of the thermal Marangoni number are calculated for several values of the bioconvection Péclet number, bioconvection Marangoni number, bioconvection Lewis number and gyrotaxis number. The results of this study showed that the existence of gyrotactic microorganisms increases the critical thermal Marangoni numbers. Moreover, the critical eigenvalues obtained were real-valued indicating that the mode of instability is via a stationary mode, however oscillatory mode is possible for some ranges of the parameters values.
The economy of China is expanding rapidly with the increasing demand for air freight services. Since 2008, China's air cargo and postal transport volume maintains sustained growth, from 4.076 million tons in 2008 to 8.632 million tons in 2020 [1], meanwhile the products needed to be transported become more diversified, a large amount of high value-added goods including fresh goods, seasonal or precious commodities appear. In other words, the situations of multi batch, small batch, fast transportation are more prominent, and higher real-time requirement is presented compared with other logistics industries.
In order to improve the efficiency of air freight transport system, an efficient automated multi-dimensional warehouse with ETVs needs to be established, and as the basis of the system, a proper scheduling strategy considering the assignment of cargo locations as well as the sequence of inbound/outbound tasks should be designed.
From limited references in the past few years, the relevant research mainly focus on deciding the cargoes' transportation sequence. Guo [2] and Qiu et al. [3] studied the inbound and outbound cargoes scheduling problem with single ETV and solved it with particle swarm optimization (PSO) algorithm. Different from the above single ETV scheduling problem, Lei [4] discussed the task scheduling problem with dual ETVs considering collision avoidance and introduced expert system to improve the operation efficiency. Ding [5] proposed task chain generation algorithm with improved shared fitness strategy and applied it to assign two ETVs to different cargo areas.
The works mentioned above developed different scheduling models with considering picking sequence and ETV routing, but they neglected to discuss the problem of assignment of input and output ports (I/O ports) if the air freight station has several entrances and exits. On the other hand, previous scheduling problems have been solved with different intelligent algorithms, but a more efficient algorithm needs to be designed for improving the efficiency and accuracy as the problems become more complex. In our research, ABC algorithm which possesses strong global optimization ability and few parameters [6,7,8,9] is first introduced to solve the scheduling problem in air freight transport system.
Actually, as one of the most recently defined bio-inspired algorithms, ABC is motivated by the swarm intelligence behaviors of gregarious colony, and it has been proved to be useful in solving various engineering problems, such as civil engineering design [10], aerospace industry [11], software testing [12], logistics warehouse management [13], manufacturing production [14], communication problem [15] and so on. However, it often suffers from the problems of poor exploitation and slow convergence, so developing new search mechanisms is crucial for complex optimization problems. Recently, other metaheuristic algorithms were introduced and combined with traditional ABC to improve its performance. Ghanem et al. [16] incorporated monarch butterfly optimization into ABC. The firework explosion search mechanism was introduced to explore the potential food sources of ABC in [17]. Gaidhane et al. [18] mixed ABC with grey wolf optimizer to balance the exploration and exploitation capabilities. Liang et al. [19] introduced differential operator in employed bee phase and revised the selection probability to be a step function. Moreover, another improving idea was to replace the single search strategy in original ABC with two or more search strategies. Xu et al. [20] introduced differential evolution strategy in employed bee phase to accelerate its convergence and adopted the global best position to guide the following updating processes in onlooker bee phase which could enhance the local search ability. Literature [21] proposed two search strategies with the help of the information of the best-so-far solution and the mean of two random solutions. In [22], three search strategies with different characteristics were employed to construct a strategy candidate pool, and the Parzen window method was applied to select the best candidate individuals. Two new search strategies were adopted based on the k-neighborhood of best solution in [23] which didn't need to calculate the selection probability. Different from the above literatures, from the perspective of improving the search mechanism, Zhang et al. [24] proposed a new search mechanism named full-dimensional ABC algorithm (fdABC), it was executed with all dimensions of each solution thus the search area could be expanded and the probability of obtaining the optimal solution could be improved. Although the proposed algorithm possessed better optimization performance especially for high-dimensional optimization problems, the time cost increased substantially because more dimensions needed to be updated compared with traditional ABC. In this paper, improvement on the search mechanisms is introduced and applied to solve the scheduling problem in air freight station.
The main features that distinguish this study from previous studies are presented below. a) For solving the tasks scheduling problem in airport container storage area with several I/O ports, the model considering the assignment of I/O ports, as well as the optimization of picking sequence and ETVs' travelling route is first established. The proposed modeling method could be applied to all scheduling problems of storage systems in fast moving consumer goods industry, e-commerce industry and logistics industry; b) In order to balance the abilities of exploration and exploitation of ABC algorithm, two improvements on search mechanisms are proposed. Different from original ABC that only one dimension is randomly updated, improvement is realized by saving the most valuable dimension of current solution and guiding the direction of subsequent exploration. Related improvement could be adopted to solve all optimization and scheduling problems in engineering fields.
The rest of this paper is organized as follows: Section 2 introduces the scheduling model with dual ETVs in the freight station of Luoyang Beijiao Airport. Then ABC and IMABC algorithms are proposed in Section 3, and their optimization performance is verified on benchmark functions. In Section 4, the improved algorithms are applied to the dual ETVs scheduling problem and their effectiveness is proved. Finally, the above work is summarized.
The air freight station in Luoyang Beijiao Airport consists of three parts, which are container storage area, bulk cargo storage area, and unhandled cargo area respectively as shown in Figure 1.
As the core of the whole system, the container storage area is used for handling the containerized cargoes, which are unloaded from aircraft in the airside or inbounded from the bulk cargo storage area in the landside. It is a three-dimensional warehouse with two rows of shelves and 16 I/O ports, each row has eight layers and 60 columns, the total slots are 60×8×2=960. Two ETVs are employed for handling cargoes between the 14 I/O ports in airside and the two I/O ports in landside, and each ETV is responsible for half of the shelf and I/O ports.
The operational process of the two ETVs is depicted as Figure 2 (some columns and I/O ports are omitted). ETVs start from R1 and R3 respectively, the 1# ETV picks up cargo at entrance R2, and stores it at I1. And then, it retrieves outbound cargo from O2 and delivers it to the exit C1. The same actions are executed with 2# ETV from R3 to C2.
Obviously, in order to improve the efficiency of cargo transportation in the container storage area, the sequence of inbound/outbound tasks as well as the corresponding I/O ports need to be scheduled, meanwhile the actions of ETVs should be optimized to obtain the minimum running time.
For solving the scheduling problem, the objective function should be established firstly based on the notations defined in Table 1.
Indices | Description |
x | The horizontal direction |
y | The vertical direction |
c | The number of columns between two slots in the shelf |
l | The number of layers between two slots in the shelf |
i | Task number, i= 1, 2, ..., n |
j | Number of ETV, j= 1, 2 |
Parameters | Description |
Vx | the horizontal travelling speeds of ETV, Vx=120(m/min) |
Vy | the vertical travelling speeds of ETV, Vy=20(m/min) |
ax | the horizontal acceleration of ETV, ax=0.5(m/s2) |
ay | the vertical acceleration of ETV, ay=0.3(m/s2) |
Txa | the time needed to travel horizontally from static to the maximum speed and immediately decreases to 0, Txa=2×Vxax=8(s) |
Tya | the time needed to lift vertically from static to the maximum speed and immediately decreases to 0, Tya=2×Vyay=2.2(s) |
Dx | the total travelling distance needed to travel from static to the maximum speed or from maximum speed to 0, Dx=14×ax×Txa2=8(m) |
Dy | the total lifting distance needed to travel from static to the maximum speed or from maximum speed to 0, Dy=14×ay×Tya2=0.36(m) |
w | width of the storage location, w=3.75(m) |
h | height of the storage location, h=3.75(m) |
δ | the execution time for ETV to load or unload cargoes, δ= 25(s) |
Variables | Description |
Txc | the time needed to move between two positions whose interval is c in horizontal direction |
Tyl | the time taken to move between two positions whose interval is l in vertical direction |
Hio | for the i-th task, the running time needed for travelling from the current position to the nearest I/O port |
Hi1 | The time of the i-th task for travelling from the current position to the scheduled target. |
M1 | working area of 1# ETV, 1≤M1≤30 |
M2 | working area of 2# ETV, 31≤M2≤60 |
fitj | the total time of the j-th ETV needed to finish the scheduled task |
If there are several inbound and outbound tasks in the storage area, the objective of optimized problem which is named as fitness function is to minimize the total execution time Hmax between two ETVs under the constraints in Eq (2).
Minimize Hmax=max{fit1,fit2} | (1) |
s.t.{|M1−M2|≥4set=set1∪set2,set1≠set2 | (2) |
fitj=∑ni=1(Hi0+Hi1)+2nδ | (3) |
The first constraint in Eq (2) ensures that the columns between two ETVs at any time are no less than four, which could avoid collision with each other. As the task set is divided into two equal parts and assigned to two ETVs, the second constraint avoids repeated allocation. The time fitj in Eq (3) is defined as the summation of execution time Hio and Hi1 of n tasks assigned to the specific ETV, it is the total time cost for picking up, releasing as well as transporting all assigned cargoes. Here the execution time Hio and Hi1 corresponding to different tasks can be obtained from Table 2 (Only the values corresponding to the first five layers and six columns are listed), which are calculated from Eqs (4) and (5). The first row and first column of Table 2 respectively represent the number of difference of rows and columns between the start position and destination in the shelf.
{Txc=2∗√w∗cax,w∗c≤Dx;Txc=Txa+w∗c−DxVx,w∗c>Dx. | (4) |
{Tyl=2∗√h∗lay,h∗l≤Dy;Tyl=Tya+h∗l−DyVy,h∗l>Dy. | (5) |
1 | 2 | 3 | 4 | 5 | 6 | |
1 | 0 | 5.47 | 7.74 | 9.62 | 11.50 | 13.37 |
2 | 11.62 | 11.62 | 11.62 | 11.62 | 11.62 | 13.37 |
3 | 22.87 | 22.87 | 22.87 | 22.87 | 22.87 | 22.87 |
4 | 34.12 | 34.12 | 34.12 | 34.12 | 34.12 | 34.12 |
5 | 45.37 | 45.37 | 45.37 | 45.37 | 45.37 | 45.37 |
For solving the above nonlinear scheduling problem, an effective optimization algorithm should be introduced.
ABC is an optimization algorithm based on the intelligent foraging behavior of honeybee swarm, where the bee colony consists of three groups: employed bees, onlooker bees and scout bees. The position of a food source represents an optimal solution of the specific problem, and its quality could be evaluated through the fitness value of the corresponding solution [9,25].
The algorithm starts iterative optimization from employed bee phase, it executes a crossover and mutation process with one randomly chosen companion, and the new solution x'mk is updated based on xmk as shown in Eq (6). Then the fitness value of each solution fitnessm could be calculated, and the onlooker bee randomly chooses to exploit or not around corresponding employed bee with the probability Pm defined as Eq (7). If the current mth solution to be exploited cannot improve for several iterations, it will be abandoned, and a scout bee corresponding to a new randomly produced solution will replace it.
x'mk=xmk+rand(−1,1)∗(xmk−xnk) | (6) |
Pm=fitnessm∑SNm=1fitnessm | (7) |
where m, n represent the indices of specific solution in the population, m,n∈{1,2,…,N}m≠n. k is the dimension of the population, k∈{1,2,…,D}.rand(−1,1) is a random number between [-1, 1]. SN is the number of food sources as well as the number of employed bees.
In ABC algorithm, for each solution, only one dimension is randomly selected and updated according to Eq (6) in employed bee phase and onlooker bee phase. In this case, the updated dimension may be different in each iteration and the optimal dimension obtained in the previous iteration is likely to be omitted in the following iterations. Thus, the search toward the possible optimal solutions is unable to be continued, the optimization accuracy and the convergence speed will be affected.
In [23], full dimensional search strategy (fdABC) is introduced to traverse all dimensions of the solution and select the optimal dimension for further exploration, therefore the search could be extended and the possibility of obtaining optimal solution will be improved, but the optimization time increases inevitably. Another improvement called random multi-dimensional artificial bee colony algorithm (RmdABC) is mentioned in [26], the key improvement of the strategy is to randomly select several different dimensions from {1,2,…,D} for one solution, and execute the updated process with Eq (6) in the employed bee and onlooker bee phases. Obviously, it randomly traverses any several dimensions of the solution in each iteration, and fewer dimensions are updated compared with fdABC, as the result, its time complexity could be greatly improved.
In order to further balance the abilities of exploration and exploitation, IMABC strategy is proposed where more valuable dimensions of solution will be picked out and saved in the external set which is used to guide the subsequent exploration. The operations of IMABC are shown as Figure 3 and outlined as follows:
1) In the first iteration, all dimensions of the solution are searched, and new solutions are generated with Eq (6) in employed bee and onlooker bee phases.
2) The fitness values of the generated solutions need to be compared with the optimal one, and if the new solution is superior to the old one, the solution in that dimension could be recognized as having the potential to be optimized, thus the optimal solution should be substituted with the generated one, the valuable dimension will be recorded in the external set and the flag is set to be one.
3) In employed bee and onlooker bee phases, after updating all dimensions of the solution, the value of flag will be checked. If the flag sign is equal to one, it means there is at least one dimension has been updated. If flag sign is zero, it demonstrates that the exploration is failed, and the number of iteration trial should increase by 1.
if sign==1then trial=0; |
else trial=trial+1 |
When the whole cycle is greater than or equal to the value of MaxCycle, if trial < Limit, the above updating operations based on the stored optimal dimensions should be performed iteratively. If trial > Limit, the value of trial resets back to zero and a new random solution will be generated to replace the old one.
Obviously, more dimensions will be explored in IMABC, it could cover more solution space and the ability of exploration could be improved compared with ABC algorithm. Meanwhile, with the help of the stored optimal dimension searched so far, the speed towards the global optimal solution could be accelerated and the ability of exploitation will be enhanced compared with fdABC and RmdABC. Therefore, the performance of the exploration and exploitation could be balanced.
The pseudo-code of IMABC is given below.
Improved Multi-dimensional ABC | |
01 | Initialization, set the maximum cycle number, the swarm size, the number of dimensions, the value of limit |
02 | for iter = 1 to MaxCycle //Employed bee phase |
03 | for m = 1 to Number of food sources |
04 | flag = 0; |
05 | if flag(m) = = 0, do |
06 | flag(m) = D, Dim(m, :) = (1:D); |
07 | end if |
08 | Temp = 0; //temp is used to temporarily store the number of currently mineable dimensions //greed strategy on multi-dimension |
09 | for j = 1 to flag(m) |
10 | Neighborhood search of employed bees with Eq (6); |
11 | Evaluate the fitness value fitness(Solm) with Eq (7); |
12 | if fitness(Solm) < fitness(Foodm), do |
13 | Foodm = Solm; Dim(m, temp) = Param2Change; sign = 1; temp = temp + 1; |
14 | end if |
15 | end for |
16 | flag(m) = temp; |
17 | if sign = 1 do |
18 | trial = 0; |
19 | else |
20 | trial = trial + 1; |
21 | end if |
22 | end for // onlooker bee phase |
23 | Calculate the probability probm if the onlooker bees choose to exploit around the specific source |
24 | for m = 1 to Food Number |
25 | sign = 0; |
26 | if rand < probm, do |
27 | Sign = 0; |
28 | if flag(m) = = 0, do |
29 | flag(m) = D, Dim(m, :) = (1:D); |
30 | end if |
31 | Temp = 0; // temp is used to temporarily store the number of currently mineable dimensions |
32 | for j = 1 to flag(m) |
33 | Neighborhood search of onlooker bees with Eq (6); |
34 | Evaluate the fitness values of the generated solutions by Eq (7); |
35 | if fitness(Solm) < fitness(Foodm), do |
36 | Foodm = Solm; sign = 1; temp = temp + 1; Dim(m, temp) = Param2Change; |
37 | end if |
38 | end for |
39 | flag(m) = temp; |
40 | if sign = 1, do |
41 | trial = 0; |
42 | else |
43 | trial = trial + 1; |
44 | end if |
45 | end if |
46 | end for |
//scout bee phase | |
47 | if trial > limit, do |
48 | trial = 0; |
49 | randomly generate a new solution |
50 | end if |
51 | end for |
In order to evaluate the performance of the proposed IMABC algorithm, nine CEC 2017 benchmark functions as listed in Table 3 are employed, where f1(x), f2(x) and f6(x) are unimodal functions, and the others are multi-modal functions. All simulations are executed on an Intel Core i7-8750H CPU with 8G RAM, the population size is 200, the dimension of solution is set to 60, 80 and 100 respectively, the number of maximum iterations is set as being 1000, and the limit used in scout bee phase is taken 100. Independent experiments are run 20 trials, the indices including the mean and standard deviation (Mean ± std dev) which reflect the quality of solution and the stability of algorithm, the average running time (Aver-R), the shortest running time (Best-R) and fitness value of the optimal solution (Best-F) are selected to evaluate the optimization performance of different algorithms.
Function expression | Searching space | Minimum value | Modality |
f1(x)=x21+106∑Di=2x2i | [−100,100] | 0 | Unimodal |
f2(x)=∑Di=1|xi|i+1 | [−100,100] | 0 | Unimodal |
f3(x)=∑D−1i=1(100(xi2−xi+1)2+(xi−1)2) | [−100,100] | 0 | Multi-modal |
f4(x)=−20exp(−0.2√1n∑ni=1x2i)−exp(1n∑ni=1cos(2πxi))+20+e | [−5,5] | 0 | Multi-modal |
f5(x)=∑ni=1[x2i−10cos(2πxi)+10] | [−500,500] | 0 | Multi-modal |
f6(x)=∑ni=1(|xi+0.5|)2 | [−100,100] | 0 | Unimodal |
f7(x)=∑Di=1(x2i−10cos(2πxi)+10) | [−5.12,5.12] | 0 | Multi-modal |
f8(x)=sin2(πw1)+∑D−1i=1(wi−1)2[1+10sin2(πwi+1)]+(wD−1)2[1+sin2(2πwD)] wi=1+xi−14,∀i=1,…,D |
[−10,10] | 0 | Multi-modal |
f9(x)=−20exp(−0.2√1D∑Di=1x2i)−exp(1D∑Di=1cos(2πxi))+20+e | [−32.768,32.768] | 0 | Multi-modal |
Tables 4–6 illustrate the optimization results with four different algorithms in different dimensions, where the best results among the four indices are highlighted in bold font. As can be seen from the tables, all algorithms could solve the nonlinear problems within limited time, the average running time Aver-R and the shortest running time Best-R increase from 60 dimensions to 100 dimensions because more dimensions need to be updated for all algorithms.
Aver-R(s) | Mean ± std dev | Best-F | Best-R(s) | ||
f1(x) | ABC | 111.849 | 2.320e+5 ± 4.515e+4 | 1.709e+5 | 111.4955 |
fdABC | 253.316 | 2.929e-252 ± 0 | 1.416e-252 | 251.577 | |
RmdABC | 188.172 | 1.654e-2 ± 1.433e-2 | 1.49e-4 | 179.199 | |
IMABC | 29.213 | 3.469e-159 ± 5.567e-159 | 1.6314e-160 | 28.702 | |
f2(x) | ABC | 121.258 | 3.024e+41 ± 6.658e+41 | 1.593e+36 | 120.909 |
fdABC | 676.282 | 8.680e-255 ± 0 | 7.047e-258 | 672.294 | |
RmdABC | 401.083 | 2.091e-42 ± 4.015e-42 | 6.660e-46 | 395.373 | |
IMABC | 229.23419 | 0 ± 0 | 0 | 383.842 | |
f3(x) | ABC | 116.400 | 5490.448 ± 4756.626 | 4884.468 | 115.536 |
fdABC | 284.881 | 2.33e-3 ± 1.35e-3 | 1.27e-4 | 279.176 | |
RmdABC | 212.479 | 1.1359 ± 0.955 | 0.323 | 208.025 | |
IMABC | 79.286 | 0.0018 ± 0.0020 | 4.004e-05 | 78.222 | |
f4(x) | ABC | 122.561 | 0.0294 ± 0.0017 | 0.0266 | 121.327 |
fdABC | 266.4800 | 6.271e-14 ± 3.432e-15 | 5.773e-14 | 272.640 | |
RmdABC | 203.594 | 4.250e-06 ± 3.376e-06 | 1.808e-07 | 200.635 | |
IMABC | 58.415 | 6.306e-14 ± 3.837e-15 | 5.773e-14 | 55.179 | |
f5(x) | ABC | 121.356 | 198.642 ± 12.773 | 179.002 | 121.051 |
fdABC | 250.787 | 0 ± 0 | 0 | 245.425 | |
RmdABC | 190.324 | 4.145e-06 ± 5.252e-06 | 2.271e-07 | 189.681 | |
IMABC | 52.091 | 0 ± 0 | 0 | 51.353288 | |
f6(x) | ABC | 120.309 | 0.232 ± 0.029 | 0.177 | 119.949 |
fdABC | 224.032 | 0 ± 0 | 0 | 218.470 | |
RmdABC | 178.819 | 1.262e-08 ± 1.059e-08 | 4.285e-10 | 178.200 | |
IMABC | 29.427 | 0 ± 0 | 0 | 28.468 | |
f7(x) | ABC | 123.764 | 4.449e-09 ± 3.849e-09 | 5.566e-10 | 122.544 |
fdABC | 255.694 | 0 ± 0 | 0 | 254.511 | |
RmdABC | 192.567 | 4.210e-08 ± 3.580e-08 | 4.677e-10 | 190.664 | |
IMABC | 84.560 | 0 ± 0 | 0 | 82.977 | |
f8(x) | ABC | 127.611 | 1.354e-05 ± 1.623e-05 | 2.079e-07 | 126.884 |
fdABC | 256.347 | 0 ± 0 | 0 | 254.650 | |
RmdABC | 194.270 | 8.781e-11 ± 2.043e-10 | 6.019e-15 | 192.641 | |
IMABC | 90.816 | 0 ± 0 | 0 | 88.339 | |
f9(x) | ABC | 125.083 | 1.694 ± 0.0995 | 1.518 | 123.144 |
fdABC | 221.099 | 6.484e-14 ± 2.901e-15 | 5.773e-14 | 220.187 | |
RmdABC | 195.0828 | 1.738 ± 3.070 | 1.224e-05 | 188.5410 | |
IMABC | 35.2991 | 0.058 ± 0.183 | 5.773e-14 | 34.9160 |
Aver-R(s) | Mean ± std dev | Best-F | Best-R(s) | ||
f1(x) | ABC | 186.356 | 7.443e+7 ± 9.252e+6 | 6.612e+7 | 185.785 |
fdABC | 349.896 | 9.500e-252 ± 0 | 1.073e-251 | 341.500 | |
RmdABC | 254.843 | 1.830 ± 2.791 | 0.187 | 242.967 | |
IMABC | 37.905 | 2.141e-150 ± 2.160e-150 | 1.580e-151 | 37.526 | |
f2(x) | ABC | 161.169 | 7.666e+82 ± 2.424e+83 | 9.065e+73 | 157.306 |
fdABC | 1092.133 | 5.377e-250 ± 0 | 9.849e-253 | 1083.415 | |
RmdABC | 641.927 | 1.236e-18 ± 3.277e-18 | 4.733e-22 | 629.479 | |
IMABC | 385.096 | 0 ± 0 | 0 | 383.842 | |
f3(x) | ABC | 153.868 | 65508.192 ± 5912.818 | 53885.588 | 152.849 |
fdABC | 408.311 | 0.00517 ± 0.00572 | 0.000291 | 393.921 | |
RmdABC | 297.845 | 2.626 ± 1.258 | 1.1049 | 287.474 | |
IMABC | 111.143 | 0.00295 ± 0.00379 | 2.251e-05 | 100.158 | |
f4(x) | ABC | 155.879 | 0.152 ± 0.00873 | 0.140 | 155.490 |
fdABC | 381.999 | 8.757e-14 ± 5.605e-15 | 7.905e-14 | 368.461 | |
RmdABC | 279.508 | 2.649e-05 ± 1.28648e-05 | 9.587e-06 | 275.084 | |
IMABC | 79.558 | 8.900e-14 ± 3.669e-15 | 8.615e-14 | 77.818 | |
f5(x) | ABC | 155.747 | 759.215 ± 50.780 | 687.486 | 153.785 |
fdABC | 351.982 | 0 ± 0 | 0 | 346.306 | |
RmdABC | 275.060 | 0.0184 ± 0.0096 | 0.0056 | 270.149 | |
IMABC | 74.003 | 0 ± 0 | 0 | 72.661 | |
f6(x) | ABC | 151.843 | 8.142 ± 0.982 | 6.349 | 151.444 |
fdABC | 300.048 | 0 ± 0 | 0 | 297.340 | |
RmdABC | 537.590 | 6.049e-07 ± 6.005e-07 | 2.1807e-08 | 373.611 | |
IMABC | 56.487 | 0 ± 0 | 0 | 53.586 | |
f7(x) | ABC | 162.425 | 6.674e-08 ± 8.736e-08 | 1.244e-09 | 161.965 |
fdABC | 344.317 | 0 ± 0 | 0 | 341.703 | |
RmdABC | 266.554 | 3.471e-07 ± 5.419e-07 | 1.397e-08 | 260.925 | |
IMABC | 112.593 | 0 ± 0 | 0 | 111.564 | |
f8(x) | ABC | 165.271 | 2.556e-05 ± 2.445e-05 | 9.431e-08 | 164.439 |
fdABC | 344.205 | 0 ± 0 | 0 | 343.570 | |
RmdABC | 260.079 | 2.892e-10 ± 3.535e-10 | 1.326e-14 | 258.259 | |
IMABC | 121.254 | 0 ± 0 | 0 | 119.521 | |
f9(x) | ABC | 162.977 | 3.614 ± 0.087 | 3.503 | 161.545 |
fdABC | 306.928 | 8.971e-14 ± 3.745e-15 | 8.615e-14 | 301.461 | |
RmdABC | 273.895 | 2.0297e-04 ± 1.3504e-04 | 9.416e-05 | 261.859 | |
IMABC | 56.5127 | 0.086 ± 0.272 | 7.905e-14 | 54.828 |
Aver-R(s) | Mean ± std dev | Best-F | Best-R(s) | ||
f1(x) | ABC | 223.196 | 3.787e+8 ± 3.315e+7 | 3.416e+8 | 222.774 |
fdABC | 425.692 | 2.044e-251 ± 0 | 1.091e-251 | 420.020 | |
RmdABC | 303.243 | 12.037 ± 13.399 | 0.405 | 300.169 | |
IMABC | 47.675 | 2.788e-144 ± 1.955e-144 | 6.548e-145 | 47.180 | |
f2(x) | ABC | 199.808 | 2.279e+120 ± 4.714e+120 | 7.215e+111 | 197.343 |
fdABC | 1664.371 | 1.671e-245 ± 0 | 6.863e-248 | 1619.398 | |
RmdABC | 920.823 | 3.470e-06 ± 9.807e-06 | 4.151e-09 | 911.149 | |
IMABC | 583.836 | 0 ± 0 | 0 | 579.155 | |
f3(x) | ABC | 189.997 | 5.919e+3 ± 4.890e+3 | 4.346e+5 | 186.093 |
fdABC | 538.976 | 0.00884 ± 0.00966 | 0.001 | 504.637 | |
RmdABC | 351.792 | 6.042937 ± 4.06785 | 1.430 | 337.071 | |
IMABC | 149.570532 | 0.00414 ± 0.00539 | 7.879e-05 | 128.478 | |
f4(x) | ABC | 192.425 | 0.585 ± 0.057 | 0.476 | 191.377 |
fdABC | 733.001 | 1.1493e-13 ± 3.910e-15 | 1.110e-13 | 721.068 | |
RmdABC | 376.785 | 0.006227 ± 0.00221 | 0.003694 | 372.387 | |
IMABC | 100.566 | 1.167e-13 ± 6.311e-15 | 1.110e-13 | 98.099 | |
f5(x) | ABC | 193.990 | 152.642 ± 8.074 | 193.287 | 193.2873 |
fdABC | 458.470157 | 0 ± 0 | 0 | 452.713643 | |
RmdABC | 574.398 | 7.97e-4 ± 8.87e-4 | 8.599e-05 | 573.802 | |
IMABC | 93.270 | 0 ± 0 | 0 | 92.877 | |
f6(x) | ABC | 189.754 | 78.395 ± 9.899 | 63.6545 | 187.726 |
fdABC | 637.867 | 0 ± 0 | 0 | 622.008 | |
RmdABC | 289.625 | 1.379e-07 ± 1.368e-07 | 1.838e-08 | 287.601 | |
IMABC | 49.458 | 0 ± 0 | 0 | 48.919 | |
f7(x) | ABC | 202.594 | 2.755e-08 ± 2.477e-08 | 6.526e-11 | 202.314 |
fdABC | 441.625 | 0 ± 0 | 0 | 433.375 | |
RmdABC | 338.374 | 1.000e-06 ± 1.085e-06 | 8.739e-10 | 328.849 | |
IMABC | 152.650 | 0 ± 0 | 0 | 148.707 | |
f8(x) | ABC | 205.3121 | 4.432e-05 ± 3.308e-05 | 2.5522e-06 | 204.430 |
fdABC | 444.093 | 0 ± 0 | 0 | 440.009 | |
RmdABC | 332.326 | 2.141e-08 ± 4.487e-08 | 2.021e-11 | 330.175 | |
IMABC | 152.809 | 0 ± 0 | 0 | 150.659 | |
f9(x) | ABC | 203.890 | 5.293 ± 0.181 | 4.981 | 203.639 |
fdABC | 406.710 | 1.1564e-13 ± 6.050e-15 | 1.039e-13 | 402.956 | |
RmdABC | 342.476 | 4.945e-04 ± 3.758e-04 | 2.069e-4 | 338.625 | |
IMABC | 77.486 | 0.012 ± 0.036 | 1.039e-13 | 72.511 |
For functions f3(x), f5(x), f6(x), f7(x) and f8(x), the values of all four indices in each dimension corresponding to IMABC are smallest, which means IMABC possesses the highest optimization accuracy, the fastest convergence speed as well as the best stability when solves the above functions. Another improved algorithm RmdABC gets smaller fitness values and Mean ± std dev values, but it takes longer time compared with the one of ABC. On the other hand, it improves the optimization efficiency of fdABC at the expense of a worse solution. In other words, RmdABC balances the exploration and exploitation abilities of fdABC and ABC.
In addition, IMABC obtains the lowest values of Mean ± std dev and Best-F, but it takes longer running time to obtain optimal solution than ABC algorithm for f2(x), which means that the stability and global search ability of IMABC are the best, while its convergence performance is better than fdABC and RmdABC because it updates less dimensions. Besides that, as the results of optimizing functions f1(x), f4(x) and f9(x), fdABC obtains the best fitness value as well as best stability among all algorithms as it executes full-dimensional search and IMABC takes much less time to get the optimal solution.
The curves of fitness values for functions f3(x) under different dimensions are depicted as Figures 4–6, and the same conclusions as mentioned above could be obtained.
From the analysis above, it can be seen that the proposed IMABC algorithm is able to produce better solutions with higher stability compared with ABC, and costs shorter computational time than other improved algorithms. RmdABC which is deduced from fdABC could reduce its time cost, but the quality of solution becomes poor because it updates less dimensions. Therefore, the proposed IMABC and RmdABC algorithms can balance exploration and exploitation abilities of ABC and fdABC.
The performance of proposed algorithms has been evaluated by solving complex mathematical problems above, and they will be applied to solve the scheduling problem in the container storage area of Luoyang Beijiao Airport in this section.
There are 16 entrances and exits in the container storage area of the airport station, the entrance coordinates are R1 (1-1-5), R2 (1-1-15), R3 (2-1-20), R4 (1-1-25), R5 (1-1-30), R6 (1-1-35), R7 (1-1-40), R8 (1-1-50) and R9 (1-1-60), and the exit coordinates are C1 (1-1-8), C2 (1-1-18), C3 (1-1-28), C4 (1-1-38), C5 (2-1-48), C6 (1-1-53) and C7 (1-1-58), where R3, C5 are I/O ports at the landside, and the rest are I/O ports at the airside. The first value in the bracket represents the row number of the shelf, the second value indicates the number of layer and the third value is the number of column. Table 7 depicts the assigned positions and current positions of 60 tasks needed to be scheduled, where the first 30 ones are input tasks, and the last 30 ones are output tasks.
Assigned position of inbound task | Assigned position of inbound task | Current position of outbound task | Current position of outbound task | ||||
1 | I(1-5-10) | 16 | I(1-8-44) | 31 | O(1-3-10) | 46 | O(2-8-10) |
2 | I(2-3-14) | 17 | I(2-8-32) | 32 | O(1-5-55) | 47 | O(1-3-32) |
3 | I(1-3-23) | 18 | I(2-3-54) | 33 | O(1-5-25) | 48 | O(1-4-50) |
4 | I(1-5-26) | 19 | I(1-3-40) | 34 | O(2-4-8) | 49 | O(2-3-38) |
5 | I(1-5-30) | 20 | I(1-4-60) | 35 | O(2-2-18) | 50 | O(2-1-58) |
6 | I(1-2-18) | 21 | I(1-3-20) | 36 | O(2-1-16) | 51 | O(1-5-24) |
7 | I(1-5-24) | 22 | I(2-2-43) | 37 | O(2-3-51) | 52 | O(1-4-30) |
8 | I(1-4-40) | 23 | I(2-4-50) | 38 | O(1-5-6) | 53 | O(2-6-40) |
9 | I(1-5-40) | 24 | I(1-6-10) | 39 | O(2-5-3) | 54 | O(2-4-35) |
10 | I(1-5-35) | 25 | I(2-7-20) | 40 | O(1-6-12) | 55 | O(2-8-51) |
11 | I(2-5-23) | 26 | I(1-6-15) | 41 | O(2-6-13) | 56 | O(2-2-30) |
12 | I(1-7-43) | 27 | I(2-8-30) | 42 | O(2-7-49) | 57 | O(1-2-60) |
13 | I(1-3-48) | 28 | I(2-2-45) | 43 | O(1-7-57) | 58 | O(1-3-26) |
14 | I(1-8-50) | 29 | I(1-7-58) | 44 | O(1-5-25) | 59 | O(1-6-35) |
15 | I(1-6-21) | 30 | I(1-4-9) | 45 | O(2-6-18) | 60 | O(2-8-45) |
For this constrained optimization problem as shown in Eqs (1) and (2), the solution is the sequence of inbound and outbound tasks with dual ETVs, which corresponds to the minimum total time cost as Eq (1). In other words, it is a discrete optimization problem, integer encoding scheme mentioned in [27] is introduced and random numbers between -10 and 10 are assigned to each dimension of the optimized solutions, after sorting them in ascending order based on their values, the corresponding scheduling scheme as well as the optimal solution could be obtained. Furthermore, the constraint conditions as Eq (2) should be checked for each obtained scheduling scheme in the iterative optimization procedure. If the constraints corresponding to the generated solution are satisfied, the solution will be saved and used for further exploration, otherwise a new solution needs to be introduced.
Comparative studies among four algorithms, including ABC, fdABC, RmdABC and IMABC, are executed for the above scheduling problem. The swarm size of all algorithms is set to 200 with 60 dimensions, the maximum local search time is 50, the stopping criterion is set to 1000 generations. Initial populations are generated through uniformly random sampling from the search space. Each algorithm is independently tested 20 times. The experiments are performed with an Intel Core i7-8750H CPU and 8GB of RAM.
Table 8 presents the optimization results (fitness value which is the scheduling time corresponding to the optimal solution) in the first ten trials. It can be seen that all proposed algorithms are able to produce high-quality solutions for scheduling problem, the performance of IMABC is better than RmdABC and ABC.
1 | 2 | 3 | 4 | 5 | 6 | 7 | 8 | 9 | 10 | |
ABC | 2783.30 | 2784.07 | 2793.17 | 2793.17 | 2801.13 | 2801.13 | 2789.08 | 2793.07 | 2793.17 | 2783.30 |
fdABC | 2632.56 | 2632.56 | 2631.32 | 2631.08 | 2632.56 | 2632.56 | 2632.56 | 2632.56 | 2632.56 | 2632.56 |
RmdABC | 2669.17 | 2664.82 | 2675.17 | 2654.29 | 2667.66 | 2660.38 | 2643.60 | 2646.47 | 2653.82 | 2653.82 |
IMABC | 2634.83 | 2634.20 | 2641.65 | 2639.56 | 2625.64 | 2630.45 | 2627.47 | 2624.59 | 2634.13 | 2629.54 |
Table 9 lists four important indices, they are the average time needed to execute the sequence corresponding to optimal solution in 20 trials (Avg), the fitness value of the best solution (Min), the fitness value of the worst solution (Max) and the corresponding running time (CPU time). It is clear that the last two proposed algorithms possess better performance as the first three indices decrease by 6% at most compared with ABC, but the time needed to obtain the optimal solution is longer. It means RmdABC and IMABC can keep the balance between the optimization accuracy and convergence speed compared with ABC. Moreover, because fdABC covers more dimensions than other mentioned algorithms, the fitness values are better than RmdABC, but the running time is 1.97 and 2.35 times of RmdABC and IMABC.
Min(s) | Max(s) | Avg(s) | CPU time (s) | |
ABC | 2783.299 | 2,801.132 | 2791.459 | 22,016.33881 |
fdABC | 2631.078 | 2632.564 | 2632.291 | 114,872.57987 |
RmdABC | 2643.596 | 2675.170 | 2658.920 | 58,699.41842 |
IMABC | 2624.589 | 2641.653 | 2632.203 | 48,795.48948 |
For the two proposed algorithms, the fitness value obtained by IMABC was a 1% reduction with respect to RmdABC and the searching time with IMABC is 83% of RmABC, obviously the optimization ability of IMABC is better than RmABC.
The conclusion also can be obtained from Figure 7 which depicts the average fitness values of the optimization problem, the curve slope of fitness values corresponding to the three improved algorithms is relatively steeper as compared with ABC, which proves their convergence. And the fitness values corresponding to IMABC converges at about 100 iterations, thus its ability of convergence could be proved.
Figure 8 and Table 10 show the corresponding trajectories of two ETVs and the sequence of task set optimized with IMABC algorithm respectively. It is clear that the dual ETVs could execute the scheduling tasks successfully without conflicting with each other.
Inbound tasks | Outbound tasks | ||
1 | 1, 24, 30 | 1 | 31, 34, 38, 39, 40, 41, 46, 60 |
2 | 2, 26 | 2 | 35, 36, 45 |
3 | 6, 11, 15, 21, 25 | 3 | 33, 44, 47, 51, 52, 56, 58 |
4 | 3, 4, 7 | 4 | 49, 53, 54, 59 |
5 | 5, 17, 27 | 5 | 42, 48, 55 |
6 | 10 | 6 | 32, 37, 43 |
7 | 8, 9, 12, 16, 19, 22, 28 | 7 | 50, 57 |
8 | 13, 14, 18, 23 | ||
9 | 20, 29 |
From the above results, the following conclusions could be obtained:
1) IMABC are valid for solving the complex scheduling problem and they can improve the searching ability of traditional ABC algorithm.
2) fdABC possesses the excellent exploration ability, IMABC could keep the balance between the optimization accuracy and convergence speed compared with ABC and fdABC under this scheduling background.
3) For IMABC, with the help of the current optimal dimensions, the convergence speed could be improved compared with fdABC and RmdABC.
In this paper, in order to improve the efficiency in the container storage area of airport cargo terminal, a study on scheduling of cargoes sequence and the action of ETV with ABC algorithms was performed. The dual ETVs scheduling model was established which considered the assignment of I/O ports as well as the trajectory of two ETVs with the constraint of avoiding collisions, and improved IMABC algorithm was proposed to solve this scheduling problem more effective. The computational experiments were carried out and the results proved the proposed algorithm could effectively avoid conflicts and generate optimal scheduling sequences.
ABC and corresponding improved algorithms have been proved to be effective in solving the scheduling problem, but the time cost for obtaining the optimal solution is high because of the iterative calculations. Improving its efficiency with appropriate methods, such as parallelization, can make the algorithm more useful especially in the scheduling problems. In addition, how to choose appropriate control parameters for different optimization issues is a problem in ABC and improved algorithms as with other metaheuristic algorithms. This problem has not been investigated sufficiently in the literature. Therefore, designing a general principle for tuning the control parameters of ABC can be addressed as a searching subject in future studies.
This work was supported by International joint research center for logistics management and engineering in Zhongyuan University of Technology, as well as the High-end foreign expert program of Ministry of Science and Technology, grant number G2021026006L; the Training Program for Young Teachers in Universities of Henan Province, grant number 2020GGJS137; the NSFC-Zhejiang Joint Fund for the Integration of Industrialization and Informatization, grant number U1709215; the Zhejiang Province Key R & D projects, grant number No. 2019C03104; the Key Scientific Research Projects of Henan Province, grant number 22A413011; Henan Province Science and Technology R & D projects, grant number 202102210135, 212102310547 and 212102210080; National Nature Science Foundation of China, grant number U1813201, 72101033 and 71831001; Beijing Natural Science Foundation Project grant number KZ202210037046; Canal Plan-Youth Top-notch Talent Project of Beijing Tongzhou District, grant number YHQN2017014.
The authors declare there are no conflicts of interest.
[1] |
J. Platt, Bioconvection patterns in cultures of free-swimming organisms, Science, 133 (1961), 1766–1767. doi: 10.1126/science.133.3466.1766
![]() |
[2] |
S. Childress, M. Levandowsky, E. Spiegel, Pattern formation in a suspension of swimming micro-organisms: Equations and stability theory, J. Fluid Mech., 69 (1975), 591–613. doi: 10.1017/S0022112075001577
![]() |
[3] |
N. Hill, T. Pedley, J. Kessler, Growth of bioconvection patterns in a suspension of gyrotactic microorganisms in a layer of finite depth, J. Fluid Mech., 208 (1989), 509–543. doi: 10.1017/S0022112089002922
![]() |
[4] |
S. Ghorai, N. Hill, Development and stability of gyrotactic plumes in bio-thermal convection, J Fluid Mech., 400 (1999), 1–31. doi: 10.1017/S0022112099006473
![]() |
[5] |
T. Pedley, J. Kessler, Hydrodynamic phenomena of in suspensions of swimming micro-organisms, Annu. Rev. Fluid Mech., 24 (1992), 313–358. doi: 10.1146/annurev.fl.24.010192.001525
![]() |
[6] |
A. Kuznetsov, A. Avramenko, Effect of small particles on the stability of bioconvection in a suspension of gyrotactic microorganisms in a fluid layer of finite depth, Int. Commun. Heat Mass, 31 (2004), 1–10. doi: 10.1016/S0735-1933(03)00196-9
![]() |
[7] |
P. Geng, A. Kuznetsov, Effect of small solid particles on the development of bioconvection plumes, Int. Commun. Heat Mass, 31 (2004), 629–638. doi: 10.1016/S0735-1933(04)00050-8
![]() |
[8] |
A. Kuznetsov, The onset of bioconvection in a suspension of gyrotactic microorganisms in a fluid layer of finite depth heated from below, Int. Commun. Heat Mass, 32 (2005), 574–582. doi: 10.1016/j.icheatmasstransfer.2004.10.021
![]() |
[9] |
D. Nield, A. Kuznetsov, The onset of bio-thermal convection in a suspension of gyrotactic microorganisms in a fluid layer: oscillatory convection, Int. J. Therm. Sci., 45 (2006), 990–997. doi: 10.1016/j.ijthermalsci.2006.01.007
![]() |
[10] |
A. Avramenko, A. Kuznetsov, The onset of bio-thermal convection in a suspension of gyrotactic microorganisms in a fluid layer with an inclined temperature gradient, Int. J. Numer. Method. H., 20 (2010), 111–129. doi: 10.1108/09615531011008154
![]() |
[11] |
A. Kuznetsov, The onset of nanofluid bioconvection in a suspension containing both nanoparticles and gyrotactic microorganisms, Int. Commun. Heat Mass, 37 (2010), 1421–1425. doi: 10.1016/j.icheatmasstransfer.2010.08.015
![]() |
[12] |
A. Kuznetsov, Non-oscillatory and oscillatory nanofluid bio-thermal convection in a horizontal layer of finite depth, Eur. J. Mech. B-Fluid., 30 (2011), 156–165. doi: 10.1016/j.euromechflu.2010.10.007
![]() |
[13] | A. Kuznetsov, Bio-thermal convection induced by two different species of microorganisms, Int. J. Numer. Method. H., 8 (2011), 548–553. |
[14] |
A. Kuznetsov, The onset of bio-thermal convection induced by a combined effect of gyrotactic and oxytactic microorganisms, Int. J. Numer. Method. H., 23 (2013), 979–1000. doi: 10.1108/HFF-09-2011-0178
![]() |
[15] |
S. Saini, Y. Sharma, Analysis of onset of bio-thermal convection in a fluid containing gravitactic microorganisms by the energy method, Chinese J. Phys., 56 (2018), 2031–2038. doi: 10.1016/j.cjph.2018.09.001
![]() |
[16] |
M. Zhao, S. Wang, H. Wang, U. Mahabaleshwar, Darcy-Brinkman bio-thermal convection in a suspension of gyrotactic microorganisms in a porous medium, Neural Comput. Appl., 31 (2019), 1061–1067. doi: 10.1007/s00521-017-3137-y
![]() |
[17] |
J. Pearson, On convection cells induced by surface tension, J. Fluid Mech., 4 (1958), 489–500. doi: 10.1017/S0022112058000616
![]() |
[18] |
D. Nield, Surface tension and buoyancy effects in cellular convection, J. Fluid Mech., 19 (1964), 341–352. doi: 10.1017/S0022112064000763
![]() |
[19] |
M. Takashima, Surface tension driven instability in a horizontal liquid layer with a deformable free surface I. Stationary convection, J. Phys. Soc. Jpn., 50 (1981), 2745–2750. doi: 10.1143/JPSJ.50.2745
![]() |
[20] | R. Benguria, M. Depassier, On the linear stability theory of Benard-Marangoni convection, Phys. Fluids A, 1 (1989), 1123–1127. |
[21] |
S. Wilson, The effect of a uniform magnetic field on the onset of steady Benard-Mrangoni convection in a layer of conducting fluid, J. Eng. Math., 27 (1993), 161–188. doi: 10.1007/BF00127480
![]() |
[22] |
I. Shivakumara, M. Venkatachalappa, S. Summa, Exact analysis of Marangoni convection with throughflow, Acta Mech., 136 (1999), 109–117. doi: 10.1007/BF01292301
![]() |
[23] |
I. Hashim, N. Arfin, Oscillatory Marangoni convection in a conducting fluid layer with a deformable free surface in the presence of a vertical magnetic field, Acta Mech., 164 (2003), 199–215. doi: 10.1007/s00707-003-0008-7
![]() |
[24] |
I. Shivakumara, C. Nanjundappa, K. Chavaraddi, Darcy-Benard-Marangoni convection in porous media, Int. J. Heat Mass Tran., 52 (2009), 2815–2823. doi: 10.1016/j.ijheatmasstransfer.2008.09.038
![]() |
[25] |
A. Abdullah, K. Lindsay, Marangoni convection in a layer of nanofluid, Int. J. Heat Mass Tran., 104 (2017), 693–702. doi: 10.1016/j.ijheatmasstransfer.2016.08.099
![]() |
[26] |
A. Abdullah, N. Alraiqab, K. Lindsay, Modelling the stability of Marangoni convection in a layer of nanofluid, Int. J. Therm. Sci., 151 (2020), 106228. doi: 10.1016/j.ijthermalsci.2019.106228
![]() |
[27] |
T. Pedley, J. Kessler, The orientation of spheroidal microorganisms swimming in a flow field, Proc. R. Soc. Lond. B, 231 (1987), 47–70. doi: 10.1098/rspb.1987.0035
![]() |
1. | Zeki Yetgin, Uğur Ercan, Honey formation optimization with single component for numerical function optimization: HFO-1, 2023, 35, 0941-0643, 24897, 10.1007/s00521-023-08984-1 | |
2. | Shih-Cheng Horng, Shieh-Shing Lin, Advanced golden jackal optimization for solving the constrained integer stochastic optimization problems, 2024, 217, 03784754, 188, 10.1016/j.matcom.2023.10.021 | |
3. | Shih-Cheng Horng, Shieh-Shing Lin, Chih-Yueh Chen, 2024, Optimal Allocation of Container Freight Station, 979-8-3503-5988-6, 1, 10.1109/ICSSE61472.2024.10608877 | |
4. | Leticia Amador-Angulo, Oscar Castillo, 2023, Chapter 68, 978-3-031-27408-4, 746, 10.1007/978-3-031-27409-1_68 |
Indices | Description |
x | The horizontal direction |
y | The vertical direction |
c | The number of columns between two slots in the shelf |
l | The number of layers between two slots in the shelf |
i | Task number, i= 1, 2, ..., n |
j | Number of ETV, j= 1, 2 |
Parameters | Description |
Vx | the horizontal travelling speeds of ETV, Vx=120(m/min) |
Vy | the vertical travelling speeds of ETV, Vy=20(m/min) |
ax | the horizontal acceleration of ETV, ax=0.5(m/s2) |
ay | the vertical acceleration of ETV, ay=0.3(m/s2) |
Txa | the time needed to travel horizontally from static to the maximum speed and immediately decreases to 0, Txa=2×Vxax=8(s) |
Tya | the time needed to lift vertically from static to the maximum speed and immediately decreases to 0, Tya=2×Vyay=2.2(s) |
Dx | the total travelling distance needed to travel from static to the maximum speed or from maximum speed to 0, Dx=14×ax×Txa2=8(m) |
Dy | the total lifting distance needed to travel from static to the maximum speed or from maximum speed to 0, Dy=14×ay×Tya2=0.36(m) |
w | width of the storage location, w=3.75(m) |
h | height of the storage location, h=3.75(m) |
δ | the execution time for ETV to load or unload cargoes, δ= 25(s) |
Variables | Description |
Txc | the time needed to move between two positions whose interval is c in horizontal direction |
Tyl | the time taken to move between two positions whose interval is l in vertical direction |
Hio | for the i-th task, the running time needed for travelling from the current position to the nearest I/O port |
Hi1 | The time of the i-th task for travelling from the current position to the scheduled target. |
M1 | working area of 1# ETV, 1≤M1≤30 |
M2 | working area of 2# ETV, 31≤M2≤60 |
fitj | the total time of the j-th ETV needed to finish the scheduled task |
1 | 2 | 3 | 4 | 5 | 6 | |
1 | 0 | 5.47 | 7.74 | 9.62 | 11.50 | 13.37 |
2 | 11.62 | 11.62 | 11.62 | 11.62 | 11.62 | 13.37 |
3 | 22.87 | 22.87 | 22.87 | 22.87 | 22.87 | 22.87 |
4 | 34.12 | 34.12 | 34.12 | 34.12 | 34.12 | 34.12 |
5 | 45.37 | 45.37 | 45.37 | 45.37 | 45.37 | 45.37 |
Function expression | Searching space | Minimum value | Modality |
f1(x)=x21+106∑Di=2x2i | [−100,100] | 0 | Unimodal |
f2(x)=∑Di=1|xi|i+1 | [−100,100] | 0 | Unimodal |
f3(x)=∑D−1i=1(100(xi2−xi+1)2+(xi−1)2) | [−100,100] | 0 | Multi-modal |
f4(x)=−20exp(−0.2√1n∑ni=1x2i)−exp(1n∑ni=1cos(2πxi))+20+e | [−5,5] | 0 | Multi-modal |
f5(x)=∑ni=1[x2i−10cos(2πxi)+10] | [−500,500] | 0 | Multi-modal |
f6(x)=∑ni=1(|xi+0.5|)2 | [−100,100] | 0 | Unimodal |
f7(x)=∑Di=1(x2i−10cos(2πxi)+10) | [−5.12,5.12] | 0 | Multi-modal |
f8(x)=sin2(πw1)+∑D−1i=1(wi−1)2[1+10sin2(πwi+1)]+(wD−1)2[1+sin2(2πwD)] wi=1+xi−14,∀i=1,…,D |
[−10,10] | 0 | Multi-modal |
f9(x)=−20exp(−0.2√1D∑Di=1x2i)−exp(1D∑Di=1cos(2πxi))+20+e | [−32.768,32.768] | 0 | Multi-modal |
Aver-R(s) | Mean ± std dev | Best-F | Best-R(s) | ||
f1(x) | ABC | 111.849 | 2.320e+5 ± 4.515e+4 | 1.709e+5 | 111.4955 |
fdABC | 253.316 | 2.929e-252 ± 0 | 1.416e-252 | 251.577 | |
RmdABC | 188.172 | 1.654e-2 ± 1.433e-2 | 1.49e-4 | 179.199 | |
IMABC | 29.213 | 3.469e-159 ± 5.567e-159 | 1.6314e-160 | 28.702 | |
f2(x) | ABC | 121.258 | 3.024e+41 ± 6.658e+41 | 1.593e+36 | 120.909 |
fdABC | 676.282 | 8.680e-255 ± 0 | 7.047e-258 | 672.294 | |
RmdABC | 401.083 | 2.091e-42 ± 4.015e-42 | 6.660e-46 | 395.373 | |
IMABC | 229.23419 | 0 ± 0 | 0 | 383.842 | |
f3(x) | ABC | 116.400 | 5490.448 ± 4756.626 | 4884.468 | 115.536 |
fdABC | 284.881 | 2.33e-3 ± 1.35e-3 | 1.27e-4 | 279.176 | |
RmdABC | 212.479 | 1.1359 ± 0.955 | 0.323 | 208.025 | |
IMABC | 79.286 | 0.0018 ± 0.0020 | 4.004e-05 | 78.222 | |
f4(x) | ABC | 122.561 | 0.0294 ± 0.0017 | 0.0266 | 121.327 |
fdABC | 266.4800 | 6.271e-14 ± 3.432e-15 | 5.773e-14 | 272.640 | |
RmdABC | 203.594 | 4.250e-06 ± 3.376e-06 | 1.808e-07 | 200.635 | |
IMABC | 58.415 | 6.306e-14 ± 3.837e-15 | 5.773e-14 | 55.179 | |
f5(x) | ABC | 121.356 | 198.642 ± 12.773 | 179.002 | 121.051 |
fdABC | 250.787 | 0 ± 0 | 0 | 245.425 | |
RmdABC | 190.324 | 4.145e-06 ± 5.252e-06 | 2.271e-07 | 189.681 | |
IMABC | 52.091 | 0 ± 0 | 0 | 51.353288 | |
f6(x) | ABC | 120.309 | 0.232 ± 0.029 | 0.177 | 119.949 |
fdABC | 224.032 | 0 ± 0 | 0 | 218.470 | |
RmdABC | 178.819 | 1.262e-08 ± 1.059e-08 | 4.285e-10 | 178.200 | |
IMABC | 29.427 | 0 ± 0 | 0 | 28.468 | |
f7(x) | ABC | 123.764 | 4.449e-09 ± 3.849e-09 | 5.566e-10 | 122.544 |
fdABC | 255.694 | 0 ± 0 | 0 | 254.511 | |
RmdABC | 192.567 | 4.210e-08 ± 3.580e-08 | 4.677e-10 | 190.664 | |
IMABC | 84.560 | 0 ± 0 | 0 | 82.977 | |
f8(x) | ABC | 127.611 | 1.354e-05 ± 1.623e-05 | 2.079e-07 | 126.884 |
fdABC | 256.347 | 0 ± 0 | 0 | 254.650 | |
RmdABC | 194.270 | 8.781e-11 ± 2.043e-10 | 6.019e-15 | 192.641 | |
IMABC | 90.816 | 0 ± 0 | 0 | 88.339 | |
f9(x) | ABC | 125.083 | 1.694 ± 0.0995 | 1.518 | 123.144 |
fdABC | 221.099 | 6.484e-14 ± 2.901e-15 | 5.773e-14 | 220.187 | |
RmdABC | 195.0828 | 1.738 ± 3.070 | 1.224e-05 | 188.5410 | |
IMABC | 35.2991 | 0.058 ± 0.183 | 5.773e-14 | 34.9160 |
Aver-R(s) | Mean ± std dev | Best-F | Best-R(s) | ||
f1(x) | ABC | 186.356 | 7.443e+7 ± 9.252e+6 | 6.612e+7 | 185.785 |
fdABC | 349.896 | 9.500e-252 ± 0 | 1.073e-251 | 341.500 | |
RmdABC | 254.843 | 1.830 ± 2.791 | 0.187 | 242.967 | |
IMABC | 37.905 | 2.141e-150 ± 2.160e-150 | 1.580e-151 | 37.526 | |
f2(x) | ABC | 161.169 | 7.666e+82 ± 2.424e+83 | 9.065e+73 | 157.306 |
fdABC | 1092.133 | 5.377e-250 ± 0 | 9.849e-253 | 1083.415 | |
RmdABC | 641.927 | 1.236e-18 ± 3.277e-18 | 4.733e-22 | 629.479 | |
IMABC | 385.096 | 0 ± 0 | 0 | 383.842 | |
f3(x) | ABC | 153.868 | 65508.192 ± 5912.818 | 53885.588 | 152.849 |
fdABC | 408.311 | 0.00517 ± 0.00572 | 0.000291 | 393.921 | |
RmdABC | 297.845 | 2.626 ± 1.258 | 1.1049 | 287.474 | |
IMABC | 111.143 | 0.00295 ± 0.00379 | 2.251e-05 | 100.158 | |
f4(x) | ABC | 155.879 | 0.152 ± 0.00873 | 0.140 | 155.490 |
fdABC | 381.999 | 8.757e-14 ± 5.605e-15 | 7.905e-14 | 368.461 | |
RmdABC | 279.508 | 2.649e-05 ± 1.28648e-05 | 9.587e-06 | 275.084 | |
IMABC | 79.558 | 8.900e-14 ± 3.669e-15 | 8.615e-14 | 77.818 | |
f5(x) | ABC | 155.747 | 759.215 ± 50.780 | 687.486 | 153.785 |
fdABC | 351.982 | 0 ± 0 | 0 | 346.306 | |
RmdABC | 275.060 | 0.0184 ± 0.0096 | 0.0056 | 270.149 | |
IMABC | 74.003 | 0 ± 0 | 0 | 72.661 | |
f6(x) | ABC | 151.843 | 8.142 ± 0.982 | 6.349 | 151.444 |
fdABC | 300.048 | 0 ± 0 | 0 | 297.340 | |
RmdABC | 537.590 | 6.049e-07 ± 6.005e-07 | 2.1807e-08 | 373.611 | |
IMABC | 56.487 | 0 ± 0 | 0 | 53.586 | |
f7(x) | ABC | 162.425 | 6.674e-08 ± 8.736e-08 | 1.244e-09 | 161.965 |
fdABC | 344.317 | 0 ± 0 | 0 | 341.703 | |
RmdABC | 266.554 | 3.471e-07 ± 5.419e-07 | 1.397e-08 | 260.925 | |
IMABC | 112.593 | 0 ± 0 | 0 | 111.564 | |
f8(x) | ABC | 165.271 | 2.556e-05 ± 2.445e-05 | 9.431e-08 | 164.439 |
fdABC | 344.205 | 0 ± 0 | 0 | 343.570 | |
RmdABC | 260.079 | 2.892e-10 ± 3.535e-10 | 1.326e-14 | 258.259 | |
IMABC | 121.254 | 0 ± 0 | 0 | 119.521 | |
f9(x) | ABC | 162.977 | 3.614 ± 0.087 | 3.503 | 161.545 |
fdABC | 306.928 | 8.971e-14 ± 3.745e-15 | 8.615e-14 | 301.461 | |
RmdABC | 273.895 | 2.0297e-04 ± 1.3504e-04 | 9.416e-05 | 261.859 | |
IMABC | 56.5127 | 0.086 ± 0.272 | 7.905e-14 | 54.828 |
Aver-R(s) | Mean ± std dev | Best-F | Best-R(s) | ||
f1(x) | ABC | 223.196 | 3.787e+8 ± 3.315e+7 | 3.416e+8 | 222.774 |
fdABC | 425.692 | 2.044e-251 ± 0 | 1.091e-251 | 420.020 | |
RmdABC | 303.243 | 12.037 ± 13.399 | 0.405 | 300.169 | |
IMABC | 47.675 | 2.788e-144 ± 1.955e-144 | 6.548e-145 | 47.180 | |
f2(x) | ABC | 199.808 | 2.279e+120 ± 4.714e+120 | 7.215e+111 | 197.343 |
fdABC | 1664.371 | 1.671e-245 ± 0 | 6.863e-248 | 1619.398 | |
RmdABC | 920.823 | 3.470e-06 ± 9.807e-06 | 4.151e-09 | 911.149 | |
IMABC | 583.836 | 0 ± 0 | 0 | 579.155 | |
f3(x) | ABC | 189.997 | 5.919e+3 ± 4.890e+3 | 4.346e+5 | 186.093 |
fdABC | 538.976 | 0.00884 ± 0.00966 | 0.001 | 504.637 | |
RmdABC | 351.792 | 6.042937 ± 4.06785 | 1.430 | 337.071 | |
IMABC | 149.570532 | 0.00414 ± 0.00539 | 7.879e-05 | 128.478 | |
f4(x) | ABC | 192.425 | 0.585 ± 0.057 | 0.476 | 191.377 |
fdABC | 733.001 | 1.1493e-13 ± 3.910e-15 | 1.110e-13 | 721.068 | |
RmdABC | 376.785 | 0.006227 ± 0.00221 | 0.003694 | 372.387 | |
IMABC | 100.566 | 1.167e-13 ± 6.311e-15 | 1.110e-13 | 98.099 | |
f5(x) | ABC | 193.990 | 152.642 ± 8.074 | 193.287 | 193.2873 |
fdABC | 458.470157 | 0 ± 0 | 0 | 452.713643 | |
RmdABC | 574.398 | 7.97e-4 ± 8.87e-4 | 8.599e-05 | 573.802 | |
IMABC | 93.270 | 0 ± 0 | 0 | 92.877 | |
f6(x) | ABC | 189.754 | 78.395 ± 9.899 | 63.6545 | 187.726 |
fdABC | 637.867 | 0 ± 0 | 0 | 622.008 | |
RmdABC | 289.625 | 1.379e-07 ± 1.368e-07 | 1.838e-08 | 287.601 | |
IMABC | 49.458 | 0 ± 0 | 0 | 48.919 | |
f7(x) | ABC | 202.594 | 2.755e-08 ± 2.477e-08 | 6.526e-11 | 202.314 |
fdABC | 441.625 | 0 ± 0 | 0 | 433.375 | |
RmdABC | 338.374 | 1.000e-06 ± 1.085e-06 | 8.739e-10 | 328.849 | |
IMABC | 152.650 | 0 ± 0 | 0 | 148.707 | |
f8(x) | ABC | 205.3121 | 4.432e-05 ± 3.308e-05 | 2.5522e-06 | 204.430 |
fdABC | 444.093 | 0 ± 0 | 0 | 440.009 | |
RmdABC | 332.326 | 2.141e-08 ± 4.487e-08 | 2.021e-11 | 330.175 | |
IMABC | 152.809 | 0 ± 0 | 0 | 150.659 | |
f9(x) | ABC | 203.890 | 5.293 ± 0.181 | 4.981 | 203.639 |
fdABC | 406.710 | 1.1564e-13 ± 6.050e-15 | 1.039e-13 | 402.956 | |
RmdABC | 342.476 | 4.945e-04 ± 3.758e-04 | 2.069e-4 | 338.625 | |
IMABC | 77.486 | 0.012 ± 0.036 | 1.039e-13 | 72.511 |
Assigned position of inbound task | Assigned position of inbound task | Current position of outbound task | Current position of outbound task | ||||
1 | I(1-5-10) | 16 | I(1-8-44) | 31 | O(1-3-10) | 46 | O(2-8-10) |
2 | I(2-3-14) | 17 | I(2-8-32) | 32 | O(1-5-55) | 47 | O(1-3-32) |
3 | I(1-3-23) | 18 | I(2-3-54) | 33 | O(1-5-25) | 48 | O(1-4-50) |
4 | I(1-5-26) | 19 | I(1-3-40) | 34 | O(2-4-8) | 49 | O(2-3-38) |
5 | I(1-5-30) | 20 | I(1-4-60) | 35 | O(2-2-18) | 50 | O(2-1-58) |
6 | I(1-2-18) | 21 | I(1-3-20) | 36 | O(2-1-16) | 51 | O(1-5-24) |
7 | I(1-5-24) | 22 | I(2-2-43) | 37 | O(2-3-51) | 52 | O(1-4-30) |
8 | I(1-4-40) | 23 | I(2-4-50) | 38 | O(1-5-6) | 53 | O(2-6-40) |
9 | I(1-5-40) | 24 | I(1-6-10) | 39 | O(2-5-3) | 54 | O(2-4-35) |
10 | I(1-5-35) | 25 | I(2-7-20) | 40 | O(1-6-12) | 55 | O(2-8-51) |
11 | I(2-5-23) | 26 | I(1-6-15) | 41 | O(2-6-13) | 56 | O(2-2-30) |
12 | I(1-7-43) | 27 | I(2-8-30) | 42 | O(2-7-49) | 57 | O(1-2-60) |
13 | I(1-3-48) | 28 | I(2-2-45) | 43 | O(1-7-57) | 58 | O(1-3-26) |
14 | I(1-8-50) | 29 | I(1-7-58) | 44 | O(1-5-25) | 59 | O(1-6-35) |
15 | I(1-6-21) | 30 | I(1-4-9) | 45 | O(2-6-18) | 60 | O(2-8-45) |
1 | 2 | 3 | 4 | 5 | 6 | 7 | 8 | 9 | 10 | |
ABC | 2783.30 | 2784.07 | 2793.17 | 2793.17 | 2801.13 | 2801.13 | 2789.08 | 2793.07 | 2793.17 | 2783.30 |
fdABC | 2632.56 | 2632.56 | 2631.32 | 2631.08 | 2632.56 | 2632.56 | 2632.56 | 2632.56 | 2632.56 | 2632.56 |
RmdABC | 2669.17 | 2664.82 | 2675.17 | 2654.29 | 2667.66 | 2660.38 | 2643.60 | 2646.47 | 2653.82 | 2653.82 |
IMABC | 2634.83 | 2634.20 | 2641.65 | 2639.56 | 2625.64 | 2630.45 | 2627.47 | 2624.59 | 2634.13 | 2629.54 |
Min(s) | Max(s) | Avg(s) | CPU time (s) | |
ABC | 2783.299 | 2,801.132 | 2791.459 | 22,016.33881 |
fdABC | 2631.078 | 2632.564 | 2632.291 | 114,872.57987 |
RmdABC | 2643.596 | 2675.170 | 2658.920 | 58,699.41842 |
IMABC | 2624.589 | 2641.653 | 2632.203 | 48,795.48948 |
Inbound tasks | Outbound tasks | ||
1 | 1, 24, 30 | 1 | 31, 34, 38, 39, 40, 41, 46, 60 |
2 | 2, 26 | 2 | 35, 36, 45 |
3 | 6, 11, 15, 21, 25 | 3 | 33, 44, 47, 51, 52, 56, 58 |
4 | 3, 4, 7 | 4 | 49, 53, 54, 59 |
5 | 5, 17, 27 | 5 | 42, 48, 55 |
6 | 10 | 6 | 32, 37, 43 |
7 | 8, 9, 12, 16, 19, 22, 28 | 7 | 50, 57 |
8 | 13, 14, 18, 23 | ||
9 | 20, 29 |
Indices | Description |
x | The horizontal direction |
y | The vertical direction |
c | The number of columns between two slots in the shelf |
l | The number of layers between two slots in the shelf |
i | Task number, i= 1, 2, ..., n |
j | Number of ETV, j= 1, 2 |
Parameters | Description |
Vx | the horizontal travelling speeds of ETV, Vx=120(m/min) |
Vy | the vertical travelling speeds of ETV, Vy=20(m/min) |
ax | the horizontal acceleration of ETV, ax=0.5(m/s2) |
ay | the vertical acceleration of ETV, ay=0.3(m/s2) |
Txa | the time needed to travel horizontally from static to the maximum speed and immediately decreases to 0, Txa=2×Vxax=8(s) |
Tya | the time needed to lift vertically from static to the maximum speed and immediately decreases to 0, Tya=2×Vyay=2.2(s) |
Dx | the total travelling distance needed to travel from static to the maximum speed or from maximum speed to 0, Dx=14×ax×Txa2=8(m) |
Dy | the total lifting distance needed to travel from static to the maximum speed or from maximum speed to 0, Dy=14×ay×Tya2=0.36(m) |
w | width of the storage location, w=3.75(m) |
h | height of the storage location, h=3.75(m) |
δ | the execution time for ETV to load or unload cargoes, δ= 25(s) |
Variables | Description |
Txc | the time needed to move between two positions whose interval is c in horizontal direction |
Tyl | the time taken to move between two positions whose interval is l in vertical direction |
Hio | for the i-th task, the running time needed for travelling from the current position to the nearest I/O port |
Hi1 | The time of the i-th task for travelling from the current position to the scheduled target. |
M1 | working area of 1# ETV, 1≤M1≤30 |
M2 | working area of 2# ETV, 31≤M2≤60 |
fitj | the total time of the j-th ETV needed to finish the scheduled task |
1 | 2 | 3 | 4 | 5 | 6 | |
1 | 0 | 5.47 | 7.74 | 9.62 | 11.50 | 13.37 |
2 | 11.62 | 11.62 | 11.62 | 11.62 | 11.62 | 13.37 |
3 | 22.87 | 22.87 | 22.87 | 22.87 | 22.87 | 22.87 |
4 | 34.12 | 34.12 | 34.12 | 34.12 | 34.12 | 34.12 |
5 | 45.37 | 45.37 | 45.37 | 45.37 | 45.37 | 45.37 |
Function expression | Searching space | Minimum value | Modality |
f1(x)=x21+106∑Di=2x2i | [−100,100] | 0 | Unimodal |
f2(x)=∑Di=1|xi|i+1 | [−100,100] | 0 | Unimodal |
f3(x)=∑D−1i=1(100(xi2−xi+1)2+(xi−1)2) | [−100,100] | 0 | Multi-modal |
f4(x)=−20exp(−0.2√1n∑ni=1x2i)−exp(1n∑ni=1cos(2πxi))+20+e | [−5,5] | 0 | Multi-modal |
f5(x)=∑ni=1[x2i−10cos(2πxi)+10] | [−500,500] | 0 | Multi-modal |
f6(x)=∑ni=1(|xi+0.5|)2 | [−100,100] | 0 | Unimodal |
f7(x)=∑Di=1(x2i−10cos(2πxi)+10) | [−5.12,5.12] | 0 | Multi-modal |
f8(x)=sin2(πw1)+∑D−1i=1(wi−1)2[1+10sin2(πwi+1)]+(wD−1)2[1+sin2(2πwD)] wi=1+xi−14,∀i=1,…,D |
[−10,10] | 0 | Multi-modal |
f9(x)=−20exp(−0.2√1D∑Di=1x2i)−exp(1D∑Di=1cos(2πxi))+20+e | [−32.768,32.768] | 0 | Multi-modal |
Aver-R(s) | Mean ± std dev | Best-F | Best-R(s) | ||
f1(x) | ABC | 111.849 | 2.320e+5 ± 4.515e+4 | 1.709e+5 | 111.4955 |
fdABC | 253.316 | 2.929e-252 ± 0 | 1.416e-252 | 251.577 | |
RmdABC | 188.172 | 1.654e-2 ± 1.433e-2 | 1.49e-4 | 179.199 | |
IMABC | 29.213 | 3.469e-159 ± 5.567e-159 | 1.6314e-160 | 28.702 | |
f2(x) | ABC | 121.258 | 3.024e+41 ± 6.658e+41 | 1.593e+36 | 120.909 |
fdABC | 676.282 | 8.680e-255 ± 0 | 7.047e-258 | 672.294 | |
RmdABC | 401.083 | 2.091e-42 ± 4.015e-42 | 6.660e-46 | 395.373 | |
IMABC | 229.23419 | 0 ± 0 | 0 | 383.842 | |
f3(x) | ABC | 116.400 | 5490.448 ± 4756.626 | 4884.468 | 115.536 |
fdABC | 284.881 | 2.33e-3 ± 1.35e-3 | 1.27e-4 | 279.176 | |
RmdABC | 212.479 | 1.1359 ± 0.955 | 0.323 | 208.025 | |
IMABC | 79.286 | 0.0018 ± 0.0020 | 4.004e-05 | 78.222 | |
f4(x) | ABC | 122.561 | 0.0294 ± 0.0017 | 0.0266 | 121.327 |
fdABC | 266.4800 | 6.271e-14 ± 3.432e-15 | 5.773e-14 | 272.640 | |
RmdABC | 203.594 | 4.250e-06 ± 3.376e-06 | 1.808e-07 | 200.635 | |
IMABC | 58.415 | 6.306e-14 ± 3.837e-15 | 5.773e-14 | 55.179 | |
f5(x) | ABC | 121.356 | 198.642 ± 12.773 | 179.002 | 121.051 |
fdABC | 250.787 | 0 ± 0 | 0 | 245.425 | |
RmdABC | 190.324 | 4.145e-06 ± 5.252e-06 | 2.271e-07 | 189.681 | |
IMABC | 52.091 | 0 ± 0 | 0 | 51.353288 | |
f6(x) | ABC | 120.309 | 0.232 ± 0.029 | 0.177 | 119.949 |
fdABC | 224.032 | 0 ± 0 | 0 | 218.470 | |
RmdABC | 178.819 | 1.262e-08 ± 1.059e-08 | 4.285e-10 | 178.200 | |
IMABC | 29.427 | 0 ± 0 | 0 | 28.468 | |
f7(x) | ABC | 123.764 | 4.449e-09 ± 3.849e-09 | 5.566e-10 | 122.544 |
fdABC | 255.694 | 0 ± 0 | 0 | 254.511 | |
RmdABC | 192.567 | 4.210e-08 ± 3.580e-08 | 4.677e-10 | 190.664 | |
IMABC | 84.560 | 0 ± 0 | 0 | 82.977 | |
f8(x) | ABC | 127.611 | 1.354e-05 ± 1.623e-05 | 2.079e-07 | 126.884 |
fdABC | 256.347 | 0 ± 0 | 0 | 254.650 | |
RmdABC | 194.270 | 8.781e-11 ± 2.043e-10 | 6.019e-15 | 192.641 | |
IMABC | 90.816 | 0 ± 0 | 0 | 88.339 | |
f9(x) | ABC | 125.083 | 1.694 ± 0.0995 | 1.518 | 123.144 |
fdABC | 221.099 | 6.484e-14 ± 2.901e-15 | 5.773e-14 | 220.187 | |
RmdABC | 195.0828 | 1.738 ± 3.070 | 1.224e-05 | 188.5410 | |
IMABC | 35.2991 | 0.058 ± 0.183 | 5.773e-14 | 34.9160 |
Aver-R(s) | Mean ± std dev | Best-F | Best-R(s) | ||
f1(x) | ABC | 186.356 | 7.443e+7 ± 9.252e+6 | 6.612e+7 | 185.785 |
fdABC | 349.896 | 9.500e-252 ± 0 | 1.073e-251 | 341.500 | |
RmdABC | 254.843 | 1.830 ± 2.791 | 0.187 | 242.967 | |
IMABC | 37.905 | 2.141e-150 ± 2.160e-150 | 1.580e-151 | 37.526 | |
f2(x) | ABC | 161.169 | 7.666e+82 ± 2.424e+83 | 9.065e+73 | 157.306 |
fdABC | 1092.133 | 5.377e-250 ± 0 | 9.849e-253 | 1083.415 | |
RmdABC | 641.927 | 1.236e-18 ± 3.277e-18 | 4.733e-22 | 629.479 | |
IMABC | 385.096 | 0 ± 0 | 0 | 383.842 | |
f3(x) | ABC | 153.868 | 65508.192 ± 5912.818 | 53885.588 | 152.849 |
fdABC | 408.311 | 0.00517 ± 0.00572 | 0.000291 | 393.921 | |
RmdABC | 297.845 | 2.626 ± 1.258 | 1.1049 | 287.474 | |
IMABC | 111.143 | 0.00295 ± 0.00379 | 2.251e-05 | 100.158 | |
f4(x) | ABC | 155.879 | 0.152 ± 0.00873 | 0.140 | 155.490 |
fdABC | 381.999 | 8.757e-14 ± 5.605e-15 | 7.905e-14 | 368.461 | |
RmdABC | 279.508 | 2.649e-05 ± 1.28648e-05 | 9.587e-06 | 275.084 | |
IMABC | 79.558 | 8.900e-14 ± 3.669e-15 | 8.615e-14 | 77.818 | |
f5(x) | ABC | 155.747 | 759.215 ± 50.780 | 687.486 | 153.785 |
fdABC | 351.982 | 0 ± 0 | 0 | 346.306 | |
RmdABC | 275.060 | 0.0184 ± 0.0096 | 0.0056 | 270.149 | |
IMABC | 74.003 | 0 ± 0 | 0 | 72.661 | |
f6(x) | ABC | 151.843 | 8.142 ± 0.982 | 6.349 | 151.444 |
fdABC | 300.048 | 0 ± 0 | 0 | 297.340 | |
RmdABC | 537.590 | 6.049e-07 ± 6.005e-07 | 2.1807e-08 | 373.611 | |
IMABC | 56.487 | 0 ± 0 | 0 | 53.586 | |
f7(x) | ABC | 162.425 | 6.674e-08 ± 8.736e-08 | 1.244e-09 | 161.965 |
fdABC | 344.317 | 0 ± 0 | 0 | 341.703 | |
RmdABC | 266.554 | 3.471e-07 ± 5.419e-07 | 1.397e-08 | 260.925 | |
IMABC | 112.593 | 0 ± 0 | 0 | 111.564 | |
f8(x) | ABC | 165.271 | 2.556e-05 ± 2.445e-05 | 9.431e-08 | 164.439 |
fdABC | 344.205 | 0 ± 0 | 0 | 343.570 | |
RmdABC | 260.079 | 2.892e-10 ± 3.535e-10 | 1.326e-14 | 258.259 | |
IMABC | 121.254 | 0 ± 0 | 0 | 119.521 | |
f9(x) | ABC | 162.977 | 3.614 ± 0.087 | 3.503 | 161.545 |
fdABC | 306.928 | 8.971e-14 ± 3.745e-15 | 8.615e-14 | 301.461 | |
RmdABC | 273.895 | 2.0297e-04 ± 1.3504e-04 | 9.416e-05 | 261.859 | |
IMABC | 56.5127 | 0.086 ± 0.272 | 7.905e-14 | 54.828 |
Aver-R(s) | Mean ± std dev | Best-F | Best-R(s) | ||
f1(x) | ABC | 223.196 | 3.787e+8 ± 3.315e+7 | 3.416e+8 | 222.774 |
fdABC | 425.692 | 2.044e-251 ± 0 | 1.091e-251 | 420.020 | |
RmdABC | 303.243 | 12.037 ± 13.399 | 0.405 | 300.169 | |
IMABC | 47.675 | 2.788e-144 ± 1.955e-144 | 6.548e-145 | 47.180 | |
f2(x) | ABC | 199.808 | 2.279e+120 ± 4.714e+120 | 7.215e+111 | 197.343 |
fdABC | 1664.371 | 1.671e-245 ± 0 | 6.863e-248 | 1619.398 | |
RmdABC | 920.823 | 3.470e-06 ± 9.807e-06 | 4.151e-09 | 911.149 | |
IMABC | 583.836 | 0 ± 0 | 0 | 579.155 | |
f3(x) | ABC | 189.997 | 5.919e+3 ± 4.890e+3 | 4.346e+5 | 186.093 |
fdABC | 538.976 | 0.00884 ± 0.00966 | 0.001 | 504.637 | |
RmdABC | 351.792 | 6.042937 ± 4.06785 | 1.430 | 337.071 | |
IMABC | 149.570532 | 0.00414 ± 0.00539 | 7.879e-05 | 128.478 | |
f4(x) | ABC | 192.425 | 0.585 ± 0.057 | 0.476 | 191.377 |
fdABC | 733.001 | 1.1493e-13 ± 3.910e-15 | 1.110e-13 | 721.068 | |
RmdABC | 376.785 | 0.006227 ± 0.00221 | 0.003694 | 372.387 | |
IMABC | 100.566 | 1.167e-13 ± 6.311e-15 | 1.110e-13 | 98.099 | |
f5(x) | ABC | 193.990 | 152.642 ± 8.074 | 193.287 | 193.2873 |
fdABC | 458.470157 | 0 ± 0 | 0 | 452.713643 | |
RmdABC | 574.398 | 7.97e-4 ± 8.87e-4 | 8.599e-05 | 573.802 | |
IMABC | 93.270 | 0 ± 0 | 0 | 92.877 | |
f6(x) | ABC | 189.754 | 78.395 ± 9.899 | 63.6545 | 187.726 |
fdABC | 637.867 | 0 ± 0 | 0 | 622.008 | |
RmdABC | 289.625 | 1.379e-07 ± 1.368e-07 | 1.838e-08 | 287.601 | |
IMABC | 49.458 | 0 ± 0 | 0 | 48.919 | |
f7(x) | ABC | 202.594 | 2.755e-08 ± 2.477e-08 | 6.526e-11 | 202.314 |
fdABC | 441.625 | 0 ± 0 | 0 | 433.375 | |
RmdABC | 338.374 | 1.000e-06 ± 1.085e-06 | 8.739e-10 | 328.849 | |
IMABC | 152.650 | 0 ± 0 | 0 | 148.707 | |
f8(x) | ABC | 205.3121 | 4.432e-05 ± 3.308e-05 | 2.5522e-06 | 204.430 |
fdABC | 444.093 | 0 ± 0 | 0 | 440.009 | |
RmdABC | 332.326 | 2.141e-08 ± 4.487e-08 | 2.021e-11 | 330.175 | |
IMABC | 152.809 | 0 ± 0 | 0 | 150.659 | |
f9(x) | ABC | 203.890 | 5.293 ± 0.181 | 4.981 | 203.639 |
fdABC | 406.710 | 1.1564e-13 ± 6.050e-15 | 1.039e-13 | 402.956 | |
RmdABC | 342.476 | 4.945e-04 ± 3.758e-04 | 2.069e-4 | 338.625 | |
IMABC | 77.486 | 0.012 ± 0.036 | 1.039e-13 | 72.511 |
Assigned position of inbound task | Assigned position of inbound task | Current position of outbound task | Current position of outbound task | ||||
1 | I(1-5-10) | 16 | I(1-8-44) | 31 | O(1-3-10) | 46 | O(2-8-10) |
2 | I(2-3-14) | 17 | I(2-8-32) | 32 | O(1-5-55) | 47 | O(1-3-32) |
3 | I(1-3-23) | 18 | I(2-3-54) | 33 | O(1-5-25) | 48 | O(1-4-50) |
4 | I(1-5-26) | 19 | I(1-3-40) | 34 | O(2-4-8) | 49 | O(2-3-38) |
5 | I(1-5-30) | 20 | I(1-4-60) | 35 | O(2-2-18) | 50 | O(2-1-58) |
6 | I(1-2-18) | 21 | I(1-3-20) | 36 | O(2-1-16) | 51 | O(1-5-24) |
7 | I(1-5-24) | 22 | I(2-2-43) | 37 | O(2-3-51) | 52 | O(1-4-30) |
8 | I(1-4-40) | 23 | I(2-4-50) | 38 | O(1-5-6) | 53 | O(2-6-40) |
9 | I(1-5-40) | 24 | I(1-6-10) | 39 | O(2-5-3) | 54 | O(2-4-35) |
10 | I(1-5-35) | 25 | I(2-7-20) | 40 | O(1-6-12) | 55 | O(2-8-51) |
11 | I(2-5-23) | 26 | I(1-6-15) | 41 | O(2-6-13) | 56 | O(2-2-30) |
12 | I(1-7-43) | 27 | I(2-8-30) | 42 | O(2-7-49) | 57 | O(1-2-60) |
13 | I(1-3-48) | 28 | I(2-2-45) | 43 | O(1-7-57) | 58 | O(1-3-26) |
14 | I(1-8-50) | 29 | I(1-7-58) | 44 | O(1-5-25) | 59 | O(1-6-35) |
15 | I(1-6-21) | 30 | I(1-4-9) | 45 | O(2-6-18) | 60 | O(2-8-45) |
1 | 2 | 3 | 4 | 5 | 6 | 7 | 8 | 9 | 10 | |
ABC | 2783.30 | 2784.07 | 2793.17 | 2793.17 | 2801.13 | 2801.13 | 2789.08 | 2793.07 | 2793.17 | 2783.30 |
fdABC | 2632.56 | 2632.56 | 2631.32 | 2631.08 | 2632.56 | 2632.56 | 2632.56 | 2632.56 | 2632.56 | 2632.56 |
RmdABC | 2669.17 | 2664.82 | 2675.17 | 2654.29 | 2667.66 | 2660.38 | 2643.60 | 2646.47 | 2653.82 | 2653.82 |
IMABC | 2634.83 | 2634.20 | 2641.65 | 2639.56 | 2625.64 | 2630.45 | 2627.47 | 2624.59 | 2634.13 | 2629.54 |
Min(s) | Max(s) | Avg(s) | CPU time (s) | |
ABC | 2783.299 | 2,801.132 | 2791.459 | 22,016.33881 |
fdABC | 2631.078 | 2632.564 | 2632.291 | 114,872.57987 |
RmdABC | 2643.596 | 2675.170 | 2658.920 | 58,699.41842 |
IMABC | 2624.589 | 2641.653 | 2632.203 | 48,795.48948 |
Inbound tasks | Outbound tasks | ||
1 | 1, 24, 30 | 1 | 31, 34, 38, 39, 40, 41, 46, 60 |
2 | 2, 26 | 2 | 35, 36, 45 |
3 | 6, 11, 15, 21, 25 | 3 | 33, 44, 47, 51, 52, 56, 58 |
4 | 3, 4, 7 | 4 | 49, 53, 54, 59 |
5 | 5, 17, 27 | 5 | 42, 48, 55 |
6 | 10 | 6 | 32, 37, 43 |
7 | 8, 9, 12, 16, 19, 22, 28 | 7 | 50, 57 |
8 | 13, 14, 18, 23 | ||
9 | 20, 29 |