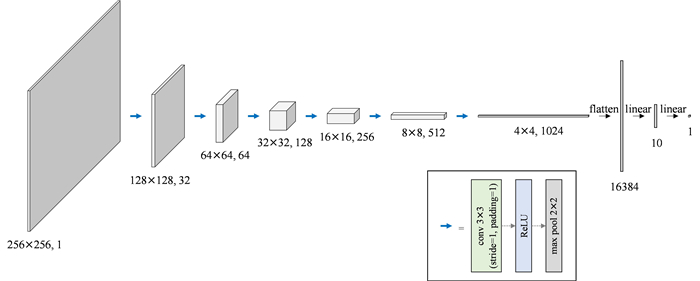
In this study, the tensile properties of tempered martensite steel were analyzed using a combination of an experimental approach and deep learning. The martensite steels were tempered in two stages, and fine and coarse cementite particles were mixed through two-stage tempering. The samples were heated to 923 and 973 K and held isothermally for 30, 45, and 60 min. They were then cooled to 723, 773, and 823 K; held isothermally for 30, 45, and 60 min; and furnace-cooled to room temperature (296 ± 2 K). The combination of low tempering temperature and short holding time in the first stage resulted in high tensile strength. When the tempering temperature at the first stage was 923 K, the combination of low tempering temperature and long holding time at the second stage resulted in high total elongation. This means that decreasing the number of coarse cementite particles and increasing the number of fine cementite particles improve the strength–ductility balance. Using the results obtained by the experimental approach, an image-based regression model was constructed that can accurately suggest the relationship between the microstructure and tensile properties of tempered martensite steel. We succeeded in developing image-based regression models with high accuracy using a convolutional neural network (CNN). Moreover, gradient-weighted class activation mapping (Grad-CAM) suggested that fine cementite particles and coarse and spheroidal cementite particles are the dominant factors for tensile strength and total elongation, respectively.
Citation: Kengo Sawai, Keiya Sugiura, Toshio Ogawa, Ta-Te Chen, Fei Sun, Yoshitaka Adachi. Analysis of tensile properties in tempered martensite steels with different cementite particle size distributions[J]. AIMS Materials Science, 2024, 11(5): 1056-1064. doi: 10.3934/matersci.2024050
[1] | Toshio Ogawa, Hiroyuki Dannoshita, Yoshitaka Adachi . Evaluation of tensile properties of ferrite single-phase low-carbon steel with different initial microstructures. AIMS Materials Science, 2019, 6(5): 798-805. doi: 10.3934/matersci.2019.5.798 |
[2] | Pavan Hiremath, Gowrishankar M. C., Manjunath Shettar, Sathyashankara Sharma, Jayashree P. K., Suhas Kowshik . Influence of normalizing post carburizing treatment on microstructure, mechanical properties and fracture behavior of low alloy gear steels. AIMS Materials Science, 2021, 8(5): 836-851. doi: 10.3934/matersci.2021051 |
[3] | Vinda Puspasari, Satrio Herbirowo, Alvin Muhammad Habieb, Dedi Pria Utama, Rahadian Roberto, Bintang Adjiantoro . Effect of sub-zero treatments on hardness and corrosion properties of low-alloy nickel steel. AIMS Materials Science, 2023, 10(1): 55-69. doi: 10.3934/matersci.2023004 |
[4] | Ivan Jandrlić, Stoja Rešković, Dušan Ćurčija, Ladislav Lazić, Tin Brlić . Modeling of stress distribution on the basis of the measured values of strain and temperature changes. AIMS Materials Science, 2019, 6(4): 601-609. doi: 10.3934/matersci.2019.4.601 |
[5] | Ayad Ramadan . Shear crack control for a reinforced concrete T-beam using coupled stochastic-multi-objective optimization methods. AIMS Materials Science, 2023, 10(6): 1077-1089. doi: 10.3934/matersci.2023057 |
[6] | Paul Kah, Pavel Layus, Benoit Ndiwe . Submerged arc welding process peculiarities in application for Arctic structures. AIMS Materials Science, 2022, 9(3): 498-511. doi: 10.3934/matersci.2022029 |
[7] | Mochamad Asrofi, Mochammad Agus Vian Hidayatulloh, Gaguk Jatisukamto, Hary Sutjahjono, Rahma Rei Sakura . The effect of temperature and volume fraction of mahoni (Swietenia mahogani) wood charcoal on SS400 steel using pack carburizing method: Study of hardness and microstructure characteristics. AIMS Materials Science, 2020, 7(3): 354-363. doi: 10.3934/matersci.2020.3.354 |
[8] | Bauyrzhan Rakhadilov, Lyaila Bayatanova, Sherzod Kurbanbekov, Ravil Sulyubayev, Nurdaulet Shektibayev, Nurbol Berdimuratov . Investigation on the effect of technological parameters of electrolyte-plasma cementation method on phase structure and mechanical properties of structural steel 20X. AIMS Materials Science, 2023, 10(5): 934-947. doi: 10.3934/matersci.2023050 |
[9] | Mohamed E. Meshaly, Maged A. Youssef, Ahmed A. Elansary . Seismic performance of ductile corrosion-free reinforced concrete frames. AIMS Materials Science, 2022, 9(5): 750-769. doi: 10.3934/matersci.2022046 |
[10] | Alexandre Lavrov, Elvia Anabela Chavez Panduro, Kamila Gawel, Malin Torsæter . Electrophoresis-induced structural changes at cement-steel interface. AIMS Materials Science, 2018, 5(3): 414-421. doi: 10.3934/matersci.2018.3.414 |
In this study, the tensile properties of tempered martensite steel were analyzed using a combination of an experimental approach and deep learning. The martensite steels were tempered in two stages, and fine and coarse cementite particles were mixed through two-stage tempering. The samples were heated to 923 and 973 K and held isothermally for 30, 45, and 60 min. They were then cooled to 723, 773, and 823 K; held isothermally for 30, 45, and 60 min; and furnace-cooled to room temperature (296 ± 2 K). The combination of low tempering temperature and short holding time in the first stage resulted in high tensile strength. When the tempering temperature at the first stage was 923 K, the combination of low tempering temperature and long holding time at the second stage resulted in high total elongation. This means that decreasing the number of coarse cementite particles and increasing the number of fine cementite particles improve the strength–ductility balance. Using the results obtained by the experimental approach, an image-based regression model was constructed that can accurately suggest the relationship between the microstructure and tensile properties of tempered martensite steel. We succeeded in developing image-based regression models with high accuracy using a convolutional neural network (CNN). Moreover, gradient-weighted class activation mapping (Grad-CAM) suggested that fine cementite particles and coarse and spheroidal cementite particles are the dominant factors for tensile strength and total elongation, respectively.
Rare metals are often added to steels to improve their material properties. Alternatively, the use of large amounts of rare metals is essential to generate renewable energy for realizing a carbon-neutral society [1]. Therefore, minimizing the amount of rare metals used in steels is important to realize a carbon-neutral society. However, many rare metals must be used to develop next-generation steels with high strength and excellent ductility [2,3]. Therefore, it is necessary to achieve the conflicting social needs to improve the materials properties of steels and reducing the amount of rare metals. This means that the material properties of pure iron and carbon steels without alloying elements need to be improved.
The material properties of pure iron and carbon steels were improved without alloying elements from various perspectives [4,5,6]. In particular, numerous studies have been conducted on the control of tempered martensite microstructure [6,7,8,9]. For instance, Liu et al. [6] investigated the mechanical properties of carbon steel with different ferrite grain sizes and cementite particle distribution by tempering martensite. According to a previous report [6], the mechanical properties of high- carbon (above 0.55 mass%C) steel depend on the spacing between cementite particles. Additionally, we demonstrated that the strength–ductility balance can be improved by dispersing both small and large cementite particles in tempered martensite high-carbon steel without alloying elements [9]. However, the cementite particle size distribution has not been optimized sufficiently because of the limited heating patterns in a previous study. This means that there is still a potential to improve the tensile properties of tempered martensite steels without alloying elements. If the mechanical properties can be further improved by optimizing the tempered martensite microstructure, then steel materials without alloying elements can be expected.
In addition to the experimental approaches, the development of materials research using deep learning has been remarkable in recent years [10,11,12]. For instance, we developed a properties-to-microstructure-to-processing inverse analysis model [10]. Moreover, the developed model showed that a microstructure with Widmanstӓtten ferrite, bainite, and martensite is beneficial to obtaining good mechanical properties. In another report, it has been demonstrated that image regression analysis is useful for linking the microstructure and tensile properties of steel [11]. Therefore, deep learning is also likely to be useful for understanding the relationship between microstructure and tensile properties of tempered martensite steels without alloying elements. In order to perform inverse analysis in the future, it is meaningful to construct a regression model with high accuracy that suggests the relationship between microstructure and tensile properties of tempered martensite steel without alloying elements.
This study has two main purposes. First, the tempering conditions were optimized to improve the mechanical properties of tempered martensite steel without alloying elements using an experimental approach. Second, using the results obtained by the experimental approach, an image-based regression model was constructed that can accurately suggest the relationship between the microstructure and tensile properties of tempered martensite steel without alloying elements.
In this study, eutectoid steel (Fe-0.8C-0.013Al-0.0005N (mass%)) was used as the test sample. Samples were pre-annealed at 1273 K for 5 min and then water-quenched to room temperature (296 ± 2 K). The pre-annealed samples were tempered in two stages, and fine and coarse cementite particles were mixed through two-stage tempering. The pre-annealed samples were heated to 923 and 973 K and held isothermally for 30, 45, and 60 min. They were then cooled to 723, 773, and 823 K; held isothermally for 30, 45, and 60 min; and furnace-cooled to room temperature.
A tensile test was performed on the tempered samples at room temperature using a universal testing machine (AG-Xplus, Shimadzu, Kyoto, Japan) at a strain rate of 4.17 × 10−4 s−1. The strain was estimated by measuring the length in the gauge region using an optical camera. Microstructural observations were conducted using scanning electron microscopy (SEM).
Based on those experimental results, regression models were constructed using a convolutional neural network (CNN) [13]. Figure 1 shows the architecture of the CNN-based image regression model used in this study. The network consists of complex layers of neurons in a multilayer nonlinear structure and automatically predicts material properties without completely losing positional information in the microstructural images. A total of 108 microstructural images were used as input data, and the values of tensile strength and total elongation were used as output data. Ten images were randomly cropped from each microstructural image and used for the CNN-based image regression model. Among the 1080 images, 972 and 108 images were used for training and testing, respectively. The features in this study have no materials science meaning such as cementite particle fraction, size, and size distribution. The CNN merely extracts image features by convolution and downsampling. In addition, gradient-weighted class activation mapping (Grad-CAM) [14] was used to visualize the area in the microstructural image on which the model focused to determine the values of tensile strength and total elongation.
Figure 2 shows the relationship between the tensile strength and elongation of each sample. The dashed line corresponds to the strength–ductility balance index. As shown in Figure 2a, the tensile strength increased and the total elongation decreased with decreasing tempering temperature in the first stage. Furthermore, the tensile strength–total elongation balance improved with decreasing holding time in the first stage. These results suggest that the combination of low tempering temperature and short holding time at the first stage results in high tensile strength. When the tempering at the first stage were conducted at 923 K for various periods, the total elongation increased with decreasing tempering temperature at second stage (Figure 2b). Moreover, the tensile strength–total elongation balance was improved with increasing holding time at the second stage (Figure 2c). These results indicate that the combination of low tempering temperature and long holding time at the second stage results in high total elongation. In addition, a decrease in the holding time at the first stage and an increase in the holding time at the second stage can improve the strength–ductility balance. This implies that decreasing the number of coarse cementite particles and increasing the number of fine cementite particles improve the strength–ductility balance. In our previous report [9], although we found that the strength–ductility balance can be improved by dispersing both small and large cementite particles, the cementite particle size distribution is not optimized. In this study, we succeeded in experimentally demonstrating the cementite particle size distribution that further improves the strength–ductility balance.
Microstructures of samples A and B (indicated in Figure 2c) are shown in Figure 3. The tensile strength and total elongation of samples A and B are 617 and 579 MPa and 25.7% and 22.1%, respectively. This means that the strength–ductility balance of sample A is better than sample B. In the case of the steel used in this study, the equilibrium volume fraction of cementite particles is estimated to be approximately 12.2%, and the volume fraction of cementite particles was approximately 12% in all the samples. Therefore, the effect of the cementite particle volume fraction on the tensile properties can be neglected. Additionally, our previous study [9] evaluated the effect of various factors (dislocation density, ferrite grain size, texture, and features of cementite particles) on tensile properties of tempered martensite steels. As a result, we revealed that the cementite particle size distribution had the greatest influence on the tensile properties. Therefore, we focus on the cementite particles size distribution in this study. In the case of sample A, coarse and fine cementite particles were observed, and areas with high density of fine cementite particles were confirmed. In contrast, coarse and spheroidal cementite particles were mainly observed in sample B, although fine cementite particles were also confirmed.
These results also suggest that decreasing the number of coarse cementite particles and increasing the number of fine cementite particles improve the strength–ductility balance.
Figure 4 shows the accuracy of the tensile strength and total elongation regression models obtained using CNN. The coefficients of determination of the tensile strength (Figure 4a) and total elongation (Figure 4b) models were 0.951 and 0.894, respectively. Therefore, it can be inferred that the regression models were constructed with high accuracy. On the basis of the constructed models, this study attempted to visualize the dominant factors for tensile strength and total elongation using Grad-CAM. Figure 5 shows the original SEM images and important areas in the microstructural images indicated by the tensile strength and total elongation models. The areas that contribute to the increases in tensile strength (Figure 5a) and total elongation (Figure 5b) are highlighted in red. The tensile strength and total elongation of the sample with the microstructure shown in Figure 5a are 617 MPa and 25.7%, respectively. In addition, the tensile strength and total elongation of the sample with the microstructure shown in Figure 5b are 518 MPa and 32.5%, respectively. For tensile strength (Figure 5a), fine cementite particles and areas with high density of fine cementite particles are highlighted. The Ashby-Orowan equation can be used to evaluate the precipitation strengthening effect of small cementite particles [9,15]:
σy=0.538Gbf0.52Rln(Rb) | (1) |
where σy is the increment related to the precipitation strengthening contribution, b is the Burgers vector, G is the elastic modulus of the steel, and f and R are the volume fraction and mean radius of the cementite particles, respectively. According to this formula, the smaller the cementite particles, the more pronounced the precipitation strengthening effect. Thus, the validity of the results obtained using Grad-CAM was proved. For total elongation (Figure 5b), coarse and spheroidal cementite particles were mainly highlighted. We reported that voids nucleate preferentially at the interface between the matrix and coarse cementite particles, but the nucleated voids are difficult to coalesce because the spacing between large cementite particles [9]. Therefore, the existence of coarse cementite particles can improve local elongation. Moreover, according to Eq 1, the larger the cementite particles, the more weakened the precipitation strengthening effect. This indicates that coarse cementite particles contribute to the improvement of total elongation. This theory is consistent with the results obtained using Grad-CAM.
We demonstrated that the strength–ductility balance can be improved by dispersing both small and large cementite particles [9]. Experimental results obtained in this study indicated that decreasing the number of coarse cementite particles and increasing the number of fine cementite particles further improve the strength–ductility balance. In addition, we succeeded in developing image-based regression models with high accuracy using CNN in this study. Finally, inverse analysis should be performed to propose the optimal cementite particle size distribution that maximizes the strength–ductility balance of tempered martensite steel. Maximization of the strength–ductility balance of tempered martensite steel with optimal cementite particle size distribution will be carried out using the constructed regression models in the future.
In this study, the tensile properties of tempered martensite steel were analyzed using a combination of an experimental approach and deep learning. The following results were obtained:
(1) The combination of low tempering temperature and short holding time in the first stage resulted in high tensile strength.
(2) When the tempering temperature at the first stage was 923 K, the combination of low tempering temperature and long holding time at the second stage resulted in high total elongation.
(3) We succeeded in developing image-based regression models with high accuracy using CNN. Moreover, Grad-CAM suggested that fine cementite particles and coarse and spheroidal cementite particles are the dominant factors affecting tensile strength and total elongation, respectively.
The authors declare they have not used Artificial Intelligence (AI) tools in the creation of this article.
This study was partly supported by a research grant from the SUZUKI Foundation, Japan.
Conceptualization: T.O. and Y.A.; Data curation: K.S. (Kengo Sawai), K.S. (Keiya Sugiura) and T.O.; Formal analysis: K.S. (Kengo Sawai), K.S. (Keiya Sugiura) and T.O.; Funding acquisition: T.O.; Investigation: K.S. (Kengo Sawai) and K.S. (Keiya Sugiura); Methodology: K.S. (Kengo Sawai), K.S. (Keiya Sugiura), T.O. and Y.A.; Project administration: T.O. and Y.A.; Resources: T.O. and Y.A.; Software: K.S. (Kengo Sawai) and K.S. (Keiya Sugiura); Supervision: T.O. and Y.A.; Validation: K.S. (Kengo Sawai), K.S. (Keiya Sugiura), T.-T.C., F.S., T.O. and Y.A.; Visualization: K.S. (Kengo Sawai), K.S. (Keiya Sugiura) and T.O.; Writing—original draft preparation: T.O.; Writing—review and editing: K.S. (Kengo Sawai), K.S. (Keiya Sugiura), T.-T.C., F.S. and Y.A. All authors have read and agreed to the published version of the manuscript.
The authors declare no conflict of interest.
[1] |
Nansai K, Watari T (2022) Innovative changes in material use for transition to a carbon-neutral society. Mater Cycles Waste Manag Res 33: 17–24. https://doi.org/10.3985/mcwmr.33.17 doi: 10.3985/mcwmr.33.17
![]() |
[2] |
Tsuchiyama T, Sakamoto T, Tanaka S, et al. (2020) Control of core-shell type second phase formed via interrupted quenching and intercritical annealing in a medium manganese steel. ISIJ Int 60: 2954–2962. https://doi.org/10.2355/isijinternational.ISIJINT-2020-164 doi: 10.2355/isijinternational.ISIJINT-2020-164
![]() |
[3] |
Kim JH, Kwon MH, Lee JS, et al. (2021) Influence of isothermal treatment prior to initial quenching of Q & P process on microstructure and mechanical properties of medium Mn steel. ISIJ Int 61: 518–526. https://doi.org/10.2355/isijinternational.ISIJINT-2019-733 doi: 10.2355/isijinternational.ISIJINT-2019-733
![]() |
[4] |
Okai D, Yae M, Yamamoto A, et al. (2017) EBSD observation of pure iron with near-cube orientation fabricated by cold rolling and annealing. Mater Trans 58: 838–841. https://doi.org/10.2320/matertrans.M2016443 doi: 10.2320/matertrans.M2016443
![]() |
[5] |
Okai D, Yamamoto A, DoiT, et al. (2021) Characteristics of cube orientation for pure iron tape fabricated by cold rolling and annealing. Mater Sci Forum 1016: 1830–1834. https://doi.org/10.4028/www.scientific.net/msf.1016.1830 doi: 10.4028/www.scientific.net/msf.1016.1830
![]() |
[6] | Liu CT, Gurland J (1968) The strengthening mechanism in spheroidized carbon steel. Trans TMS-AIME 242: 1535–1542. |
[7] |
Ning J, Feng Y, Wang M, et al. (2017) Dependence of tensile properties on microstructural features of bimodal-sized ferrite/cementite steels. J Iron Steel Res Int 24: 67–76. https://doi.org/10.1016/S1006-706X(17)30010-9 doi: 10.1016/S1006-706X(17)30010-9
![]() |
[8] |
Tsuchida N, Ueji R, Gong W, et al. (2023) Stress partitioning between bcc and cementite phases discussed from phase stress and dislocation density in martensite steels. Scr Mater 222: 115002. https://doi.org/10.1016/j.scriptamat.2022.115002 doi: 10.1016/j.scriptamat.2022.115002
![]() |
[9] |
Hayakawa K, Ogawa T, He L, et al. (2024) Improvement in the strength–ductility balance of tempered martensite steel by controlling cementite particle size distribution. J Mater Eng Perform 33: 6675–6685. https://doi.org/10.1007/s11665-023-08428-w doi: 10.1007/s11665-023-08428-w
![]() |
[10] |
Wang Z, Ogawa T, Adachi Y (2019) Properties-to-microstructure-to-processing inverse analysis for steels via machine learning. ISIJ Int 59: 1691–1694. https://doi.org/10.2355/isijinternational.ISIJINT-2019-089 doi: 10.2355/isijinternational.ISIJINT-2019-089
![]() |
[11] |
Sawai K, Chen TT, Sun F, et al. (2024) Image regression analysis for linking the microstructure and property of steel. Results Mater 21: 100526. https://doi.org/10.1016/j.rinma.2023.100526 doi: 10.1016/j.rinma.2023.100526
![]() |
[12] |
Noguchi S, Wang H, Inoue J (2022) Identification of microstructures critically affecting material properties using machine learning framework based on met-allurgists' thinking process. Sci Rep 12: 14238. https://doi.org/10.1038/s41598-022-17614-0 doi: 10.1038/s41598-022-17614-0
![]() |
[13] |
Krizhevsky A, Sutskever I, Hinton GE (2017) ImageNet classification with deep convolutional neural networks. Commun ACM 60: 84–90. https://doi.org/10.1145/3065386 doi: 10.1145/3065386
![]() |
[14] |
Selvaraju RR, Cogswell M, Das A, et al. (2020) Grad-CAM: Visual explanations from deep networks via gradient-based localization. Int J Comput Vision 128: 336–359. https://doi.org/10.1007/s11263-019-01228-7 doi: 10.1007/s11263-019-01228-7
![]() |
[15] |
Liang JW, Shen YF, Misra RDK, et al. (2021) High strength-superplasticity combination of ultrafine-grained ferritic steel: the significant role of nanoscale carbides. J Mater Sci Technol 83: 131–144. https://doi.org/10.1016/j.jmst.2020.11.078 doi: 10.1016/j.jmst.2020.11.078
![]() |