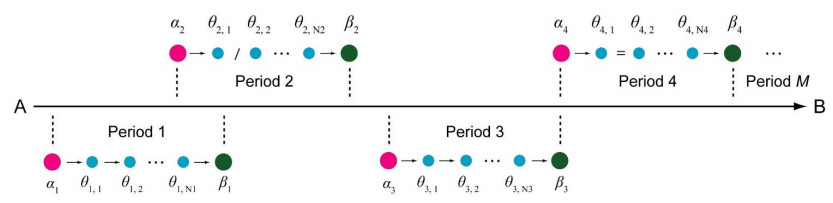
Radiocarbon ages must be calibrated due to the remarkable fluctuations of the atmospheric radiocarbon level. The traditional method (e.g., Calib) does not make use of any constraint such as the temporal/stratigraphical ordering of the ages, thereby resulting in one or several large age ranges. Bayesian age modeling is advantageous over the traditional method in several aspects. First, it can provide precise age estimates by applying some constraints known a priori. Second, it may provide a timing of an archaeological feature or a geological event that is unable to be dated directly. Although several Bayesian age modeling frameworks have been developed, inexperienced users may need not only a more user-friendly environment for data entry and definition of their project-specific problem, but also a powerful post-processing tool for analyzing and visualizing the results. Here a hierarchical Bayesian model with a minimum level of structural complexity is presented. It provides users with a flexible and powerful framework to incorporate radiocarbon ages into a sequence along a one-dimensional continuum so that it best reveals their temporal order, thereby yielding a more precise timing. The accompanying Matlab software package not only complements the existing MatCal package designed to calibrate radiocarbon ages individually, but also serves as an alternative to the online tools of Bayesian radiocarbon age modeling such as OxCal and BCal.
Citation: Shiyong Yu. MatCalib: a Matlab software package for Bayesian modeling of radiocarbon ages subject to temporal order constraints[J]. AIMS Geosciences, 2022, 8(1): 16-32. doi: 10.3934/geosci.2022002
[1] | Jama Mohamed, Dahir Abdi Ali, Abdimalik Ali Warsame, Mukhtar Jibril Abdi, Eid Ibrahim Daud, Mohamed Mohamoud Abdilleh . Bayesian extreme value modelling of annual maximum monthly rainfall in Somalia from 1901 to 2022. AIMS Geosciences, 2024, 10(3): 598-622. doi: 10.3934/geosci.2024031 |
[2] | Ian G Enting . Response function analysis of carbon dioxide and climate using the Padé-Laplace technique. AIMS Geosciences, 2022, 8(3): 346-365. doi: 10.3934/geosci.2022020 |
[3] | Ian Enting . Estimation and inversion across the spectrum of carbon cycle modeling. AIMS Geosciences, 2018, 4(2): 126-143. doi: 10.3934/geosci.2018.2.126 |
[4] | Brian E. Bunker, Jason A. Tullis, Jackson D. Cothren, Jesse Casana, Mohamed H. Aly . Object-based Dimensionality Reduction in Land Surface Phenology Classification. AIMS Geosciences, 2016, 2(4): 302-328. doi: 10.3934/geosci.2016.4.302 |
[5] | Paolo Dell'Aversana . Reinforcement learning in optimization problems. Applications to geophysical data inversion. AIMS Geosciences, 2022, 8(3): 488-502. doi: 10.3934/geosci.2022027 |
[6] | Diana I Bakalova, Ekaterina E Dimitrova . Optimistic expectations and life satisfaction as antecedents of emigration attitudes among Bulgarian Millennials and Zoomers. AIMS Geosciences, 2023, 9(2): 285-310. doi: 10.3934/geosci.2023016 |
[7] | Ray Kenny . Stable isotope ratios and speleothem chronology from a high-elevation alpine cave, southern San Juan Mountains, Colorado (USA): Evidence for substantial deglaciation as early as 13.5 ka. AIMS Geosciences, 2019, 5(1): 41-65. doi: 10.3934/geosci.2019.1.41 |
[8] | Eric Ariel L. Salas, Geoffrey M. Henebry . Canopy Height Estimation by Characterizing Waveform LiDAR Geometry Based on Shape-Distance Metric. AIMS Geosciences, 2016, 2(4): 366-390. doi: 10.3934/geosci.2016.4.366 |
[9] | J.M. Weygand . The temporal and spatial development of dB/dt for substorms. AIMS Geosciences, 2021, 7(1): 74-94. doi: 10.3934/geosci.2021004 |
[10] | Mike Long, Jean Sebastien L’Heureux, Bjørn Kristian Fiskvik Bache, Alf Kristian Lund, Svein Hove, Karl Gunnar Sødal, Helene Alexandra Amundsen, Steinar Nordal, Alberto Montafia . Site characterisation and some examples from large scale testing at the Klett quick clay research site. AIMS Geosciences, 2019, 5(3): 344-389. doi: 10.3934/geosci.2019.3.344 |
Radiocarbon ages must be calibrated due to the remarkable fluctuations of the atmospheric radiocarbon level. The traditional method (e.g., Calib) does not make use of any constraint such as the temporal/stratigraphical ordering of the ages, thereby resulting in one or several large age ranges. Bayesian age modeling is advantageous over the traditional method in several aspects. First, it can provide precise age estimates by applying some constraints known a priori. Second, it may provide a timing of an archaeological feature or a geological event that is unable to be dated directly. Although several Bayesian age modeling frameworks have been developed, inexperienced users may need not only a more user-friendly environment for data entry and definition of their project-specific problem, but also a powerful post-processing tool for analyzing and visualizing the results. Here a hierarchical Bayesian model with a minimum level of structural complexity is presented. It provides users with a flexible and powerful framework to incorporate radiocarbon ages into a sequence along a one-dimensional continuum so that it best reveals their temporal order, thereby yielding a more precise timing. The accompanying Matlab software package not only complements the existing MatCal package designed to calibrate radiocarbon ages individually, but also serves as an alternative to the online tools of Bayesian radiocarbon age modeling such as OxCal and BCal.
Radiocarbon (14C) dating represents a key chronological method that has been widely used for determining the age of geological and archaeological samples of the last ca. 55,000 years [1]. The physical basis of this method is that organisms assimilate 14C, directly or indirectly, from the atmosphere during their life span. However, their death cuts off the linkage to the contemporaneous global carbon cycle, which leaves the remainder 14C atoms inside their bodies to decay without replenishment with a half-life of ca. 5730 years. Therefore, by the law of radioactive decay, the radiocarbon age of a sample can be determined uniquely by measuring the residual 14C radioactivity [2], providing that the atmospheric 14C level is assumed to remain constant over time. However, owing to the complex global carbon cycle and the changes in the intensity of the solar and earth's magnetic field [3], the atmospheric 14C level fluctuated remarkably [4], exerting a profound impact on the radiocarbon dating method [5]. Therefore, the laboratory radiocarbon ages must be calibrated using a calibration curve [6], which is a key step for converting radiocarbon ages to calendar ages'.
Due to the non-monotonic nature of the calibration curves, the map from the radiocarbon year to the calendar year is not one to one, thereby leading to non-unique solutions usually expressed in terms of a probability density function with one or several highest posterior density (HPD) regions [7,8]. It appears that uncertainty is a nature of the calibrated age, which cannot be eliminated regardless of how small the laboratory error is [5]. Sometimes the uncertainty of the calibrated age could be extremely large if the radiocarbon age hits a plateau in the calibration curve [9,10]. Many attempts have been made to reduce the uncertainty of radiocarbon age calibration. Bayesian methods represent a promising approach to the solution of this problem [11,12,13], which takes advantage of the stratigraphical context or temporal order of the radiocarbon ages as a constraint [14]. Specifically, if the radiocarbon ages are assumed to come from a depositional sequence, they must follow a chronological order [15,16]. By applying the constraint of temporal order on the calibration, the uncertainty of the calibrated age can be greatly narrowed down [8].
Bayesian methods have been increasingly used for modeling a suite of 14C ages subject to temporal order constraints since 1990s. Several Bayesian frameworks have been developed and in use. Of particular note are the standalone package DateLab [17] and the online OxCal [11] and BCal [18] tools. DateLab provides a simple graphical user interface, while OxCal and BCal allow registered users to access through a worldwide web (www) browser for data entry and workflow setup. However, further analyses of the modeled ages require the random samples from the original Markov Chain Monte Carlo (MCMC) output. For example, if two ages need to be compared, the best way is to calculate the difference between their posterior ages and expresses it in terms of a probability density function with the HPD regions. Also, inexperienced users may need not only a more flexible and user-friendly environment for data entry and definition of their project-specific problems, but also a powerful post-processing tool for analyzing and visualizing the results. Towards this end, a hierarchical Bayesian framework for 14C age modeling subject to temporal order constraints is described and a Matlab package is presented here. The package is an extension to the previous version [19] by adding several new features such as the overlapping boundary relationship that deal with the coexistence and subsequent replacement of one period by another.
Considering a chronological sequence consisting of M periods, where "period" is defined as a stage of deposition or human occupation characterized by a distinct geological or archaeological feature. Similar to the "phase" in OxCal, each period contains a group of at least one radiocarbon age. For the ith period of the chronological sequence, where i=1,2,⋯,M denoting the number of the lowest (oldest) to the uppermost (most recent) period, Let ri=[ri,1,ri,2,⋯,ri,Ni], σσi=[σi,1,σi,2,⋯,σi,Ni], θθi=[θi,1,θi,2,⋯,θi,Ni], αi, and βi denote the Ni 14C ages, the associated one standard deviation of the Ni 14C ages, the corresponding calendar ages of the Ni 14C ages, and the calendar age of the early and late boundary of the ith period, respectively (Figure 1). Note that the division of periods in the sequence is subjective, but mainly depends on the sedimentary facies in geology or tool assemblage in archaeology or the scientific questions to be answered. Here a hierarchical Bayesian framework of 14C age modeling with a minimum level of structural complexity is described (Figure 2), within which θi, αi, and βi, where i=1,2,⋯,M, are treated as model parameters, which will be inferred using the laboratory radiocarbon ages. Due to lack of knowledge about these parameters, vague (noninformative) priors are preferred to use. For the sake of mathematical expression, the "BP" scale is used for model description, while the algorithms were implemented in both "BP" and "BC/AD" scales. The conversion from the "BP" to the "BCE|CE" scale is BCE|CE = 1950 – BP.
For the Ni radiocarbon ages in the ith period, where i=1,2,⋯,M, the corresponding calendar ages, say θi, are assumed to follow the uniform distribution supported on the interval of [αi,βi].
f(θθi|αi,βi)=1(αi−βi)NiI(θθi|αi,βi), | (1) |
where I(θθi|αi,βi) is an indicator function describing the temporal order of the calendar ages in the ith period. Here, three types of temporal order are considered: (1) "unordered", which means that the ages are not necessarily to be chronologically ordered in a period. An example is that ages were obtained from different archaeological sites of the same cultural period. As such, the indicator function can be defined as:
I(θθi|αi,βi)={1,αi>all(θθi)>βi0,otherwise, | (2) |
(2) "coeval", which means that the ages are assumed to be the same in a period. An example is that ages were obtained from the same depth of a stratigraphical unit or the same archaeological feature of a cultural period and thus their calendar ages must be the same. In this case, the indicator function can be defined as:
I(θθi|αi,βi)={1,αi>θi,1=θi,2=⋯=θi,Ni>βi0,otherwise, | (3) |
and (3) "ordered", which means that the ages follow a chronological order. An example is that ages were obtained from different depths of a stratigraphical unit. Therefore, the indicator function can be expressed as:
I(θθi|αi,βi)={1,αi>θi,1>θi,2>⋯>θi,Ni>βi0,otherwise, | (4) |
where " > " denotes "older than" and "=" denotes "same as".
The calendar age of the early and late boundaries of the ith period, say αi and βi, where i=1,2,⋯,M, is assumed to follow the uniform distribution supported on the interval [A, B], where A and B are two floating parameters defining the beginning and the end of the chronological sequence in calendar years BP, respectively. Therefore, for the first and last periods (i.e., i = 1 or M), the prior probability density function of the calendar ages of the early and late boundaries, say αi and βi, respectively, can be written as:
f(αi,βi|A,B)=1(A−B)2I(αi,βi|A,B), | (5) |
where I(αi,βi|A,B) is an indicator function describing the temporal order of the calendar ages of the early and late boundaries. It can be simply defined as:
I(αi,βi|A,B)={1,A>αi>βi>B0,otherwise. | (6) |
However, for the internal periods, the prior probability density function of the calendar ages of the early and late boundaries should be treated differently according to the relationship with the neighboring periods. Without loss of generality, for the ith internal period, where i=2,⋯,M−1, the prior probability density function of the early and late boundaries, say αi and βi, respectively, can be written as:
f(αi,βi|α1,βM)=1(α1−βM)2I(αi,βi|αi−1,βi−1,α1,βM), | (7) |
where I(αi,βi|αi−1,βi−1,α1,βM) is an indicator function describing the relationship of two neighboring periods. This mathematical framework is essentially the same as what Nicholls and Jones [14] proposed. Here three cases are considered: (1) "overlapping", which means the imbrication of two neighboring periods. An example is the initial coexistence of two archaeological cultures and subsequent replacement of one culture by another. Therefore, the indicator function can be expressed as:
I(αi,βi|αi−1,βi−1,α1,βM)={1,α1>αi−1>αi>βi−1>βi>βM0,otherwise, | (8) |
(2) "contiguous", which means a sudden transition from one period to another. An example is the rapid change of the depositional environment or the archaeological culture. As such, the indicator function can be defined as:
I(αi,βi|αi−1,βi−1,α1,βM)={1,α1>αi−1>βi−1=αi>βi>βM0,otherwise, | (9) |
and (3) "disjoint", which means that there is an interruption between two periods. An example is the existence of a depositional hiatus between two stratigraphical units or a gap of human occupation between two archaeological periods. As such, the indicator function can be written as:
I(αi,βi|αi−1,βi−1,α1,βM)={1,α1>αi−1>βi−1>αi>βi>βM0,otherwise, | (10) |
where " > " denotes "older than" and "=" denotes "same as".
The normal distribution is usually used for modeling 14C ages from the calibration curve [20,21]. However, the traditional normal distribution is less robust to outlying data. To better accommodate outliers in the 14C ages, we adopt the variance-multiplier normal distribution [22]. Let µ and ϵ be the mean and one standard deviation of a radiocarbon calibration curve, respectively. For the jth calendar age in the ith period, say θi,j, where i=1,2,⋯,M and j=1,2,⋯,Ni, the corresponding true 14C age is assumed to be normally distributed around the calibration curve mean, μμ(θi,j), confined by an additive variance of the laboratory 14C age and the calibration curve multiplied by a positive number. Hence, the true 14C age can be modeled as:
ˆri,j∼N(μ(θi,j),δω2i,j), | (11) |
where ω2i,j=σ2i,j+ϵϵ2(θi,j) is the sum of the laboratory 14C age variance (i.e., σ2i,j) and the calibration curve variance (i.e., ϵϵ2(θi,j)) and δ is a positive number. Let δ be a random variable with an improper prior probability density function defined as:
f(δ)=1δ, | (12) |
where δ∈(0,+∞). As such, for the ith period, where i=1,2,⋯,M, the likelihood function can be expressed as:
L(ri|θθi,σσi,δ)=∏Nij=11√2πδωi,jexp{−[ri,j−μμ(θi,j)]22δω2i,j}. | (13) |
An integrated likelihood can be obtained by integrating out hyperparameter δ (Supplementary), yielding:
L(ri|θθi,σσi)=Γ(Ni2)(∑Nij=1[ri,j−μμ(θi,j)]22ω2i,j)−Ni2, | (14) |
where Γ (·) denotes the gamma function.
Making use of Bayes' theorem and the integrated likelihood function (Eq. 14), for the ith period, where i=1,2,⋯,M, the joint posterior probability density function of parameters θi, αi, and βi, can be expressed as:
p(θθi,αi,βi|ri,σσi,A,B)∝L(ri|θθi,σσi)×f(θθi|αi,βi)×f(αi,βi|α1,βM)×f(α1,βM|A,B) |
=Γ(Ni2)(∑Nij=1[ri,j−μμ(θi,j)]22ω2i,j)−Ni2×1(αi−βi)NiI(θθi|αi,βi)×1(α1−βM)2I(αi,βi|αi−1,βi−1,α1,βM)×1(A−B)2I(α1,βM|A,B). | (15) |
Note that the prior indicator functions of the calendar ages and the boundaries have different expressions according to the ordering of the ages and the relationship of the boundaries as given above.
The structure of the above equation (Eq. 15) is so complex that we are unable to derive an analytical expression of the marginal posterior probability distribution function for each parameter. Therefore, this model framework can be implemented using the MCMC method. The Metropolis-approximated Gibbs sampler is used to generate a Markov Chain for the parameters one at a time, and a Metropolis-Hastings step is used to update each parameter [23]. Specifically, for the ith period of the sequence, where i=1,2,⋯,M, we choose to update the parameters in the order θi → αi → βi. Given the current state of the calendar ages of the 14C ages, say θi, and the early and late boundaries, say αi and βi, respectively, we first update θi using a uniform random walk process on the interval [αi, βi] such that:
θθ∗i|αi,βi=θθi+ρ×u, | (16) |
where u denotes a vector of Ni independent random numbers drawn from a uniform distribution on [–1, 1], and ρ is the step size of the random walk. Then, we update the calendar age of the early boundary of the ith period of the sequence, say αi, where i=1,2,⋯,M, using the triangular distribution. For the first period, the calendar age of the early boundary, say α1, can be updated according to:
α∗1|α1,A,θθ∗1∼T(max(θθ∗1),α1,A), | (17) |
where T (·) denotes the triangular distribution with three parameters describing the lower bound, the peak, and the upper bound, respectively. While for other periods (i.e., i > 1), special treatments are required, depending on the relationship of two neighboring periods. Three cases are considered accordingly. For the "overlapping" boundaries, the calendar age of the early boundary can be updated according to:
α∗i|αi,θθ∗i−1,θθ∗i∼T(max(θθ∗i),αi,min(θθ∗i−1(θθ∗i−1>αi))), | (18) |
while for the "contiguous" boundaries, the calendar age of the early boundary can be updated according to:
α∗i|αi,θθ∗i−1,θθ∗i∼T(max(θθ∗i),αi,min(θθ∗i−1)), | (19) |
and for the "disjoint" boundary, the calendar age of the early boundary can be updated according to:
α∗i|αi,βi−1,θθ∗i∼T(max(θθ∗i),αi,βi−1), | (20) |
Finally, we update the calendar age of the late boundary of the ith period, say βi, where i=1,2,⋯,M, using the triangular distribution too. Similarly, for the first M − 1 periods, three cases are considered. For the "overlapping" boundaries, the calendar age of the late boundary can be updated according to:
β∗i|βi,θθ∗i,θθ∗i+1∼T(max(θθ∗i+1(θθ∗i+1<βi)),βi,min(θθ∗i)); | (21) |
while for the "contiguous" boundaries, the calendar age of the late boundary, say βi, can be updated by simply setting:
β∗i=α∗i+1; | (22) |
and for the "disjoint" boundaries, the calendar age of the late boundary can be updated according to:
β∗i|βi,α∗i+1,θθ∗i∼T(α∗i+1,βi,min(θθ∗i)). | (23) |
For the last period (i.e., i = M), the calendar of the late boundary, say βM, can be updated according to:
β∗M|βM,B,θθ∗M∼T(B,βM,min(θθ∗M)). | (24) |
The acceptance or rejection of the proposed move of the chains is determined using the Metropolis-Hastings algorithm [24]. The state-of-the-art IntCal20 [25], SHCal20 [26], and Marine20 [27] calibration datasets are also provided for modeling of the 14C ages. For the sake of computing, all of the laboratory and the calibration curve radiocarbon ages are converted to the F14C space during the modeling process [20]. The convergence of the chains is monitored using the method proposed by Gelman [28]. Several chains are run parallelly with different starting points for each set of simulations, and the ratio of the between-sequence to with-sequence variance in terms of the potential scale reduction factor, ˆR is calculated. If ˆR is below the critical value of 1.1, the chains can be regarded as convergent. Once the chains for a model parameter converge, they are mixed to allow the estimate of the empirical probability density function that mimics the posterior probability distribution of this parameter. We implemented the above procedure in the Matlab® environment, yielding a software package named as MatCalib v2.0. The code is available on Mendeley Data [29] and also accessible in the Supplementary.
Running the software package is quite straightforward. A tab delimited spread sheet template is provided, which allow users to enter their data, including laboratory codes, depths, 14C ages and errors, reservoir ages and errors if any, specify the calibration curve (e.g., IntCal13 or IntCal20) for each age, and define the temporal ordering of the ages (e.g., "ordered", "unordered", or "coeval") in each period. The only file users need to modify is MatCalib_main.m, which provides a textual interface enabling users to read their data, define their project-specific problem (e.g., boundary relationships), specify parameters for MCMC simulations, and run the model to obtain, analyze, and visualize the results. In the Matlab environment, users need to open the main program MatCalib_main, give the nearest years that the modeled calendar ages are to be rounded to, choose the time scale in which the modeled calendar ages are to be reported, and specify the parameters for Monte Carlo simulations such as the number of chains to be run for convergence diagnoses as well as the length, burn-in period, and thinning interval of the chains. Then, MCMC simulations are conducted using the function age_modeling and the convergence of the chains is tested using the function convergence.
Upon completion of the MCMC simulations, the empirical probability density function, as well as the 68.2% and 95.4% HPD regions of the modeled calendar ages of the 14C ages and the early and late boundaries of each period, are estimated using the method of Lougheed and Obrochta [30], and the results are saved automatically to files for subsequent analyses. Users can plot the results either against sample numbers using the function plot_ages, or against depths using the function plot_age_depth if depth information is provided. Users also can compare any two ages by calculating their posterior difference and calculate the pooled mean of several ages using the functions age_difference and pooled_mean, respectively. The results are also given in terms of the empirical probability density function as well as the 68.2% and 95.4% HPD regions, and they can be plotted using the functions plot_difference and plot_pooled_mean, respectively.
It is noteworthy that the MCMC method is simulation-based, which requires a large number of iterations to produce reliable (i.e., converged and well mixed) results. Therefore, the model may yield slightly different results at each time of a run. Another caveat is that models may be so inconsistent with the data that no possible solutions will be yielded, particularly in the case of the presence of extreme outliers. Therefore, users should choose and remove the outliers before running the model to produce valid results. In other cases, the solution space may be very fragmentary, providing that the MCMC solutions will be different with each different starting position. Keeping these properties of the MCMC method in mind, users must check the reproducibility by making multiple runs of the model with a different number of iterations, burn-in period, thinning size, and initial value (automatically reset for each run using the function initialization).
Some of the functionalities of this software package are demonstrated using two datasets of 14C ages. One is obtained from the Neolithic archaeological sites on the Shandong Peninsula in North China, which is used to determine the timing of the Longshan and Yueshi cultures in this area. The other is retrieved from a sedimentary core on the southeastern Swedish Baltic coast, which is used to establish an age-depth relationship and to quantify the sedimentary hiatus induced by an erosional event during the transition from a lacustrine to a lagoonal environment. In both cases, a set of simulations with three chains are run for 20,000 iterations for each parameter. Every 20th iteration is kept after a burn-in period of 1000 iterations to reduce autocorrelation.
The dataset used in this study consists of nine 14C ages from sites belonging to the Longshan cultural period and 10 ages belonging to the Yueshi cultural period [31]. These ages are put together into a chronological sequence with two periods corresponding to the Longshan (Period 1) and Yueshi (Period 2), respectively. The latest Longshan site was dated to 3570 ± 80 14C yr BP and the earliest Yueshi site was dated to 3760 ± 145 14C yr BP, implying that these two cultures may have coexisted for a while [32]. Therefore, overlapping boundaries are used in the age modeling. As the 14C ages in each cultural period come from individual sites and their stratigraphical relationship is unknown, the temporal order of the ages is set to "unordered". The probability distributions of the modeled calendar ages are presented in Figure 3. The 95.4% HPD regions of calendar age of the boundaries are listed in Table 1 for the sake of comparison with those obtained from OxCal.
Software | Longshan Period (95.4% HPD region) | Yueshi Period (95.4% HPD region) | |||
Start | End | Start | End | ||
OxCal | 3040–2344 | 2332–1602 | 2552–1891 | 1693–1159 | |
MatCalib | 2885–2750 | 2005–1870 | 2490–2335 | 1480–1300 |
The Longshan culture, colloquially referred to as the black pottery culture, is a late-Neolithic culture in the middle and lower Yellow River areas of northern China from about 3000 to 1900 BCE. The culture flourished dramatically and expanded to the Shandong Peninsula at about 2885–2750 BCE as defined by the 95.4% HPD region (Table 1). It then decreased and vanished around 2005–1870 BC (95.4% HPD region). The Yueshi culture rose locally at about 2490–2335 BC (95.4% HPD region). It then replaced the Longshan culture and gradually evolved into the Bronze Age at about 1480–1300 BC (95.4% HPD region). These timings lie in the 95.4% age ranges obtained from OxCal (Table 1), suggesting that MatCalib can yield age estimates much firmer than OxCal. As defined by the age difference between the early boundary of Period 2 (i.e., Yueshi period) and the late boundary of Period 1 (i.e., Longshan period) (Figure 3), these two cultures may have coexisted for about 350–590 years (95.4% HPD region) on the Shandong Peninsula (Figure 4). Again, OxCal yields a relatively large overlap (280–740 years, 95% HPD region) of these two cultures.
Previous work about the 14C dating of a sediment core from the Hunnemara ancient lake (14°53' E, 56°10' N) on the southeastern Swedish Baltic coast revealed a depositional hiatus across the transition from the lacustrine to the lagoonal facies [33], which may have resulted from erosion during the rapid sea-level rise about 7600 years ago [34]. The lake is developed within a closed basin with an outflow threshold around 3.0 m as1. It has been drained and converted to agricultural land and a meadow by local farmers in the 19th century and is now partly used for a garbage dump. An 8.5-m-long core was taken near the center of the lake basin. A total of 10 14C ages on bulk sediments were obtained, which are incorporated into a chronological sequence consisting of two periods representing the lacustrine (Period 1) and lagoonal (Period 2) environments, respectively. Period 1 comprises three 14C ages, whereas Period 2 contains seven 14C ages subject to a local reservoir age offset of −108 ± 24 years. Therefore, the Marine20 calibration curve is applied on these ages [27]. As the ages are obtained from different depths along the core, the law of superposition was strictly enforced by using a temporal order constraint (e.g., "ordered") on the ages in both periods. The hiatus lies at 575 cm, which is marked by a non-depositional surface separating the two periods. Therefore, a "disjoint" relationship of the internal boundaries is applied.
The modeled calendar ages of the radiocarbon ages are plotted against their depths (Figure 5). The age-depth relationship reveals variable sedimentation rates within the lake basin. The results suggest that the lacustrine environment (Period 1) prevailed from 11,745–11,545 BP (95.4% HPD region) to 8530–8145 BP (95.4% HPD region), while the lagoonal environment (Period 2) occurred in the basin at about 7410–7040 BP (95.4% HPD region) as a result of rapid sea-level rise [34] and terminated at about 3090–2925 BP (95.4% HPD region). As defined by the posterior age difference of the late boundary of Period 1 and the early boundary of Period 2, the sedimentary hiatus is estimated to be 870–1355 years (95.4% HPD region) (Figure 6).
Bayesian methods are widely used for modeling 14C ages. It represents a flexible yet robust approach to integrating prior knowledge about the ages and their temporal or stratigraphical context, thereby yielding a more precise timing of the geological or archaeological events than the traditional methods [8]. Although some key components of the Bayesian methods for 14C age modeling have already been well explained [8,14,21], a formal treatment of several other outstanding issues (e.g., the relationship of neighboring boundaries) is lacking. Here a complete and detailed description of the analytical framework is presented along with a Matlab package for software implementation. Therefore, it can be used not only as an introductory guild to, but also a tutorial on Bayesian 14C age modeling.
There exist several software packages designed to perform Bayesian 14C age modeling [11,17,18]. Most of which shares some common features. For example, the law of superposition is the backbone of all Bayesian methods, which has been strictly enforced to observe the constraint of temporal order. Yet the model presented here differs from these tools in several aspects. First is the treatment of the relationship between two neighboring periods. As illustrated by the first example, coexistence of two cultures appears to be a common phenomenon in archaeology [32]. Similar to OxCal, an overlapping relationship is considered in this model, while this functionality was not implemented in other tools. Second is the application of different calibration curves on 14C ages of different types. This is particularly necessary if ages were obtained from different depositional environments (e.g., marine versus terrestrial). As illustrated by the second example, ages in the first period are obtained from a terrestrial setting, whereas those in the second period are retrieved from a marine environment subject to the reservoir effect [35]. As such, a local reservoir age and the marine calibration curve should be applied on these ages. Other minor differences of this model from the existing tools include the use of the non-Gaussian likelihood function to accommodate outlying data, the implementation of convergence diagnosis of the Markov Chains, and the employment of a triangular distribution to update the boundaries during the simulations.
The accompanying Matlab package (i.e., MatCalib 2.0) contains a wide range of functionality for Bayesian statistical analyses of 14C ages, including the inference of the calendar age of the individual 14C ages and the boundary of the periods. Random samples of these parameters generated from the MCMC simulations are saved automatically to a data file for future use without running the model again. Based on these data, the empirical probability density functions of these parameters are built up first and then the HPD regions and the median-probability age are calculated. Further analyses such as pooled mean of ages, timing, and duration of an event can be conducted and visualized using the high-quality graphical output (e.g., Figures 3–6). In future, the software will be improved to enable the modeling of multi-source ages, for example, calendar ages with errors and/or tie points for events without errors. For these age types, a calibration curve is no longer needed. If depth information is available, an age-depth relationship may be established through polynomial regression (e.g., CLAM [36]), Brownian bridge (e.g., OxCal [37]), or interpolation (e.g., Undatable [38]) on the stored random samples at known depths, instead of employing a stochastic depositional model such as Bchron [39] and Bacon [40].
The traditional method of 14C age calibration maps individual 14C ages to the calibration curve to obtain the calendar age. However, owing to the large fluctuations of the atmospheric 14C level, the resulting calibrated age is not a point estimate; rather, it is usually treated as a random variable expressed in terms of a probability distribution with one or several HPD regions. Most importantly, the probability distribution of the calibrated age could be extremely broad if the radiocarbon age hits a radiocarbon plateau in the calibration curve, thereby yielding several large HPD regions. The large uncertainty in the calibrated age hinders the precise timing of geological and/or archaeological events. Conceptually different from the traditional method, Bayesian model provides such an analytical framework that integrate prior knowledge about the ages and their temporal order as a constraint, which in turn can significantly narrow down the uncertainty of the calibrated ages.
The hierarchical Bayesian model presented in this paper provides a flexible approach to the precise timing of archaeological and/or geological events by making use of a suite of 14C ages and their stratigraphical context (temporal order). Implemented in the Matlab environment, the accompanying software package is not only an extension to the existing MatCal package that is only able to calibrate individual 14C ages [30], but also an alternative or complement to the online calibration tools such as BCal [18] and OxCal [11]. Also, this model can be used as a building block for age-depth modeling. If the depth information of the 14C ages is available, a probabilistic expression of the age-depth relationship can be readily obtained through either bootstrap regression or Brownian bridge transformation.
This work was supported by the National Science Foundation of China (grant number 41971102). My gratitude is due to the three anonymous reviewers for their insightful comments and constructive suggestions that greatly improved the manuscript.
The author declares no conflict of interest.
1. Derivation of the integrated likelihood function.
2. Matlab code for implementing Bayesian radiocarbon age modeling.
[1] |
Bronk Ramsey C (2008) Radiocarbon dating: revolutions in understanding. Archaeometry 50: 249-275. https://doi.org/10.1111/j.1475-4754.2008.00394.x doi: 10.1111/j.1475-4754.2008.00394.x
![]() |
[2] | Libby WF (1961) Radiocarbon dating. Science 133: 621-629. |
[3] |
Heaton T, Bard E, Bronk Ramsey C, et al. (2021) Radiocarbon: A key tracer for studying Earth's dynamo, climate system, carbon cycle, and Sun. Science 374: eabd7096. https://doi.org/10.1126/science.abd7096 doi: 10.1126/science.abd7096
![]() |
[4] |
Siegenthaler U, Heimann M, Oeschger H (1980) 14C variations caused by changes in the global carbon cycle. Radiocarbon 22: 177-191. https://doi.org/10.1017/S0033822200009449 doi: 10.1017/S0033822200009449
![]() |
[5] |
Guilderson TP, Reimer PJ, Brown TA (2005) The boon and bane of radiocarbon dating. Science 307: 362-364. https://doi.org/10.1126/science.1104164 doi: 10.1126/science.1104164
![]() |
[6] |
Clark RM (1975) A calibration curve for radiocarbon dates. Antiquity 49: 251-266. https://doi.org/10.1017/S0003598X00070277 doi: 10.1017/S0003598X00070277
![]() |
[7] |
Bronk Ramsey C (1995) Radiocarbon calibration and analysis of stratigraphy: the OxCal program. Radiocarbon 37: 425-430. https://doi.org/10.1017/S0033822200030903 doi: 10.1017/S0033822200030903
![]() |
[8] |
Buck CE, Kenworthy JB, Litton CD, et al. (1991) Combining archaeological and radiocarbon information: a Bayesian approach to calibration. Antiquity 65: 808-821. https://doi.org/10.1017/S0003598X00080534 doi: 10.1017/S0003598X00080534
![]() |
[9] |
Yu SY, Chen X, Fang Z, et al. (2021) Towards a precise timing of groundwater use in the lower Yellow River area during the late Bronze Age: Bayesian inference from the radiocarbon ages of ancient water wells at the Liang'ercun site, North China. Quat Geochronol 66: 101214. https://doi.org/10.1016/j.quageo.2021.101214 doi: 10.1016/j.quageo.2021.101214
![]() |
[10] |
Gómez-Paccard M, Rivero-Montero M, Chauvin A, et al. (2019) Revisiting the chronology of the Early Iron Age in the north-eastern Iberian Peninsula. Archaeol Anthropol Sci 11: 4755-4767. https://doi.org/10.1007/s12520-019-00812-9 doi: 10.1007/s12520-019-00812-9
![]() |
[11] | Bronk Ramsey C (2001) Development of the radiocarbon program OxCal. Radiocarbon 43: 355-363. |
[12] |
Buck CE, Meson B (2015) On being a good Bayesian. World Archaeol 47: 567-584. https://doi.org/10.1080/00438243.2015.1053977 doi: 10.1080/00438243.2015.1053977
![]() |
[13] |
Heaton TJ, Blackwell PG, Buck CE (2009) A Bayesian approach to the estimation of radiocarbon calibration curves: the IntCal09 methodology. Radiocarbon 51: 1151-1164. https://doi.org/10.1017/S0033822200034214 doi: 10.1017/S0033822200034214
![]() |
[14] |
Nicholls G, Jones M (2001) Radiocarbon dating with temporal order constraints. J R Stat Soc 50: 503-521. https://doi.org/10.1111/1467-9876.00250 doi: 10.1111/1467-9876.00250
![]() |
[15] |
Guntau M (1989) Concepts of natural law and time in the history of geology. Earth Sci Hist 8: 106-110. https://doi.org/10.17704/eshi.8.2.02w88w234323x503 doi: 10.17704/eshi.8.2.02w88w234323x503
![]() |
[16] | Harris EC (1979) The laws of archaeological stratigraphy. World Archaeol 11: 111-117. |
[17] |
Jones M, Nicholls G (2002) New radiocarbon calibration software. Radiocarbon 44: 663-674. https://doi.org/10.1017/S0033822200032112 doi: 10.1017/S0033822200032112
![]() |
[18] |
Buck CE, Christen JA, James GN (1999) BCal: an on-line Bayesian radiocarbon calibration tool. Internet Archaeol 7: 1192-1201. https://doi.org/10.11141/ia.7.1 doi: 10.11141/ia.7.1
![]() |
[19] | Yu SY (2021) Bayesian radiocarbon age modeling. Mendeley Data, V1. https://doi.org/10.17632/sfdwkyh848.1 |
[20] |
Reimer P, Baillie M, Bard E, et al. (2004) IntCal04 terrestrial radiocarbon age calibration, 0-26 cal kyr BP. Radiocarbon 46: 1029-1058. https://doi.org/10.1017/S0033822200032999 doi: 10.1017/S0033822200032999
![]() |
[21] |
Bronk Ramsey C (2009) Bayesian analysis of radiocarbon dates. Radiocarbon 51: 337-360. https://doi.org/10.1017/S0033822200033865 doi: 10.1017/S0033822200033865
![]() |
[22] |
Christen JA, Pérez ES (2009) A new robust statistical model for radiocarbon data. Radiocarbon 51: 1047-1059. https://doi.org/10.1017/S003382220003410X doi: 10.1017/S003382220003410X
![]() |
[23] |
Gilks WR, Best NG, Tan KK (1995) Adaptive rejection Metropolis sampling within Gibbs sampling. J R Stat Soc 44: 455-472. https://doi.org/10.2307/2986138 doi: 10.2307/2986138
![]() |
[24] |
Chib S, Greenberg E (1995) Understanding the Metropolis-Hastings algorithm. Am Stat 49: 327-335. https://doi.org/10.2307/2684568 doi: 10.2307/2684568
![]() |
[25] |
Reimer PJ, Austin WE, Bard E, et al. (2020) The IntCal20 Northern Hemisphere radiocarbon age calibration curve (0-55 cal kBP). Radiocarbon 62: 725-757. https://doi.org/10.1017/RDC.2020.41 doi: 10.1017/RDC.2020.41
![]() |
[26] |
Hogg AG, Heaton TJ, Hua Q, et al. (2020) SHCal20 Southern Hemisphere calibration, 0-55,000 years cal BP. Radiocarbon 62: 759-778. https://doi.org/10.1017/RDC.2020.59 doi: 10.1017/RDC.2020.59
![]() |
[27] |
Heaton TJ, Köhler P, Butzin M, et al. (2020) Marine20—the marine radiocarbon age calibration curve (0-55,000 cal BP). Radiocarbon 62: 779-820. https://doi.org/10.1017/RDC.2020.68 doi: 10.1017/RDC.2020.68
![]() |
[28] | Gelman A, Inference and monitoring convergence, In: Gilks WR, Richarson S, Spiegelhalter DJ, editors. Markov Chain Monte Carlo in Practice, New York: Chapman and Hall/CRC, 1995. https://doi.org/10.1201/b14835 |
[29] | Yu SY (2021) MatCalib: A Matlab software package for Bayesian calibration of radiocarbon ages subject to temporal order constraints. Mendeley Data, V1. https://doi.org/10.17632/rx478cbpm5.1 |
[30] | Lougheed BC, Obrochta SP (2016) MatCal: Open source Bayesian 14C age calibration in MatLab. J Open Res Softw 4: p.e42. http://doi.org/10.5334/jors.130 |
[31] | The Institute of Archaeology (1992) Radiocarbon Dates in Chinese Archaeology (1965-1991), Beijing: Cultural Relics Publishing House, 488. |
[32] |
Long T, Wagner M, Tarasov PE (2017) A Bayesian analysis of radiocarbon dates from prehistoric sites in the Haidai Region, East China, for evaluation of the archaeological chronology. J Archaeol Sci Rep 12: 81-90. https://doi.org/10.1016/j.jasrep.2017.01.024 doi: 10.1016/j.jasrep.2017.01.024
![]() |
[33] |
Yu SY, Berglund BE, Sandgren P, et al. (2005) Holocene palaeoecology along the Blekinge coast, SE Sweden, and implications for climate and sea-level changes. Holocene 15: 278-292. https://doi.org/10.1191/0959683605hl792rp doi: 10.1191/0959683605hl792rp
![]() |
[34] |
Yu SY, Berglund B, Sandgren P, et al. (2007) Evidence for a rapid sea-level rise 7600 yr ago. Geology 35: 891-894. https://doi.org/10.1130/G23859A.1 doi: 10.1130/G23859A.1
![]() |
[35] |
Alves EQ, Macario K, Ascough P, et al. (2018) The worldwide marine radiocarbon reservoir effect: definitions, mechanisms, and prospects. Rev Geophys 56: 278-305. https://doi.org/10.1002/2017RG000588 doi: 10.1002/2017RG000588
![]() |
[36] |
Blaauw M (2010) Methods and code for 'classical' age-modelling of radiocarbon sequences. Quat Geochronol 5: 512-518. https://doi.org/10.1016/j.quageo.2010.01.002 doi: 10.1016/j.quageo.2010.01.002
![]() |
[37] |
Bronk Ramsey C (2008) Deposition models for chronological records. Quat Sci Rev 27: 42-60. https://doi.org/10.1016/j.quascirev.2007.01.019 doi: 10.1016/j.quascirev.2007.01.019
![]() |
[38] |
Lougheed BC, Obrochta S (2019) A papid, deterministic age-depth modeling routine for geological sequences with inherent depth uncertainty. Paleoceanography Paleoclimatology 34: 122-133. https://doi.org/10.1029/2018PA003457 doi: 10.1029/2018PA003457
![]() |
[39] |
Haslett J, Parnell A (2008) A simple monotone process with application to radiocarbon-dated depth chronologies. J R Stat Soc 57: 399-418. https://doi.org/10.1111/j.1467-9876.2008.00623.x doi: 10.1111/j.1467-9876.2008.00623.x
![]() |
[40] |
Blaauw M, Christen JA (2011) Flexible paleoclimate age-depth models using an autoregressive gamma process. Bayesian Anal 6: 457-474. https://doi.org/10.1214/11-BA618 doi: 10.1214/11-BA618
![]() |
![]() |
![]() |
![]() |
![]() |
1. | Shi-Yong Yu, Wen-Jia Li, Liang Zhou, Xuefeng Yu, Qiang Zhang, Zhixiong Shen, Human disturbances dominated the unprecedentedly high frequency of Yellow River flood over the last millennium, 2023, 9, 2375-2548, 10.1126/sciadv.adf8576 | |
2. | Shi-Yong Yu, Cheng Zhu, Inverse modeling of lichen growth curves and implications for lichenometric dating, 2022, 69, 18711014, 101257, 10.1016/j.quageo.2022.101257 | |
3. | Shi-Yong Yu, Integrating age modeling into a hierarchical Bayesian framework for inferring the pattern and rate of past sea-level changes from uncertainty-prone proxy data, 2024, 85, 18711014, 101617, 10.1016/j.quageo.2024.101617 |
Software | Longshan Period (95.4% HPD region) | Yueshi Period (95.4% HPD region) | |||
Start | End | Start | End | ||
OxCal | 3040–2344 | 2332–1602 | 2552–1891 | 1693–1159 | |
MatCalib | 2885–2750 | 2005–1870 | 2490–2335 | 1480–1300 |