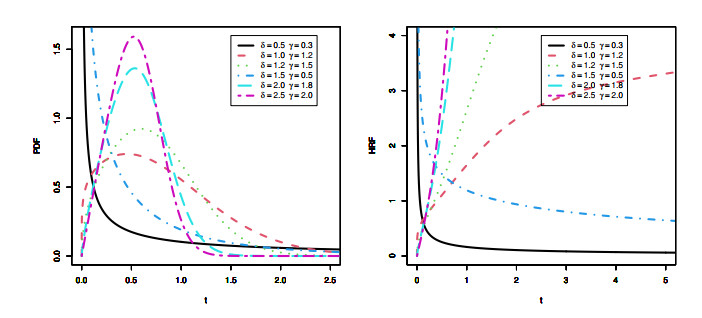
This study provided a significant contribution to developing an adaptable trigonometric extension of the power half-logistic distribution. To be more specific, we created an innovative two-parameter lifetime model called the sine power half-logistic distribution (SPHLD) by using features from the sine-generated family of distributions. The novel distribution could be more effective in modeling lifetime phenomena when asymmetric data was presented, which was the primary motivating factor. The SPHLD's density function plots showed that the distribution adopted several asymmetric shape configurations. Furthermore, the SPHLD's hazard rate plots displayed both monotonic increases and decreases. The quantile function, moments, incomplete moment, and stress-strength reliability were among the statistical characteristics of the SPHLD that were computed. Statistical inference using sixteen distinct classical estimating techniques was utilized to estimate the SPHLD parameters. A simulation study was done to evaluate the consistency of the different estimates and determine the best estimating approach based on some accuracy measures. Analyses of real data revealed that the SPHLD performed better than a number of alternative distributions.
Citation: Amal S. Hassan, Najwan Alsadat, Mohammed Elgarhy, Laxmi Prasad Sapkota, Oluwafemi Samson Balogun, Ahmed M. Gemeay. A novel asymmetric form of the power half-logistic distribution with statistical inference and real data analysis[J]. Electronic Research Archive, 2025, 33(2): 791-825. doi: 10.3934/era.2025036
[1] | Mahmoud El-Morshedy, Mohamed S. Eliwa, Mohamed El-Dawoody, Hend S. Shahen . A weighted hybrid discrete probability model: Mathematical framework, statistical analysis, estimation techniques, simulation-based ranking, and goodness-of-fit evaluation for over-dispersed data. Electronic Research Archive, 2025, 33(4): 2061-2091. doi: 10.3934/era.2025091 |
[2] | Weishang Gao, Qin Gao, Lijie Sun, Yue Chen . Design of a novel multimodal optimization algorithm and its application in logistics optimization. Electronic Research Archive, 2024, 32(3): 1946-1972. doi: 10.3934/era.2024089 |
[3] | Shaban Khidr, Salomon Sambou . $ L^p $-theory for the $ {\partial }\overline{\partial} $-equation and isomorphisms results. Electronic Research Archive, 2025, 33(1): 68-86. doi: 10.3934/era.2025004 |
[4] | Shuai Chang, Jinrui Guan . A study on the estimator for the extreme value index of heavy-tailed distribution generated from moment statistic. Electronic Research Archive, 2025, 33(4): 2295-2311. doi: 10.3934/era.2025101 |
[5] | Hanan H. Sakr, Mohamed S. Mohamed . On residual cumulative generalized exponential entropy and its application in human health. Electronic Research Archive, 2025, 33(3): 1633-1666. doi: 10.3934/era.2025077 |
[6] | Caiwen Chen, Tianxiu Lu, Ping Gao . Chaotic performance and circuitry implement of piecewise logistic-like mapping. Electronic Research Archive, 2025, 33(1): 102-120. doi: 10.3934/era.2025006 |
[7] | Xuerui Li, Lican Kang, Yanyan Liu, Yuanshan Wu . Distributed Bayesian posterior voting strategy for massive data. Electronic Research Archive, 2022, 30(5): 1936-1953. doi: 10.3934/era.2022098 |
[8] | Sadia Anwar, Showkat Ahmad Lone, Aysha Khan, Salmeh Almutlak . Stress-strength reliability estimation for the inverted exponentiated Rayleigh distribution under unified progressive hybrid censoring with application. Electronic Research Archive, 2023, 31(7): 4011-4033. doi: 10.3934/era.2023204 |
[9] | Zhensheng Zhou, Lin Wang, Xue Zou, Fei Wang, Zaijun Zhang, Xiaobo Yan . The first hitting time analysis of evolutionary algorithms based on renewal process. Electronic Research Archive, 2024, 32(5): 2994-3015. doi: 10.3934/era.2024137 |
[10] | Xin Gao, Hao Liu, Zhou Fang, Yang Zhang . Microscopic mechanism of subgrade vibration compaction based on discrete element method. Electronic Research Archive, 2023, 31(11): 7061-7077. doi: 10.3934/era.2023358 |
This study provided a significant contribution to developing an adaptable trigonometric extension of the power half-logistic distribution. To be more specific, we created an innovative two-parameter lifetime model called the sine power half-logistic distribution (SPHLD) by using features from the sine-generated family of distributions. The novel distribution could be more effective in modeling lifetime phenomena when asymmetric data was presented, which was the primary motivating factor. The SPHLD's density function plots showed that the distribution adopted several asymmetric shape configurations. Furthermore, the SPHLD's hazard rate plots displayed both monotonic increases and decreases. The quantile function, moments, incomplete moment, and stress-strength reliability were among the statistical characteristics of the SPHLD that were computed. Statistical inference using sixteen distinct classical estimating techniques was utilized to estimate the SPHLD parameters. A simulation study was done to evaluate the consistency of the different estimates and determine the best estimating approach based on some accuracy measures. Analyses of real data revealed that the SPHLD performed better than a number of alternative distributions.
The features and interrelationships of distribution functions are important for representing naturally occurring events. The conventional distributions, however, have not been able to adequately describe complex data due to the dynamic and ever-increasing complexity of current datasets. Aware of these limitations, scientists have focused their attention on improving the effectiveness and suitability of these distributions in an attempt to find better matches for the variety of real-world information. There are several approaches for defining statistical distributions. Different approaches are available for defining failure rate and density functions to create distributions with more desired and modifiable qualities. One of these approaches is the development of generated families of the probability distributions. Notable has helped to create new distribution families that aim to solve the shortcomings of their earlier versions. Recently, a variety of academics have developed an interest in the families of distributions that have been generated, such as the Marshall Olkin-G proposed by Ref. [1], beta-G presented by Ref. [2], transformed- transformer (T-X) family proposed by Ref. [3], Topp-Leone-G prepared by Ref. [4], new Topp Leone-G prepared by Ref. [5], transmuted odd Fréchet-G created by Ref. [6], modified T-X family prepared by Ref. [7], truncated power Lomax-G proposed by Ref. [8], odd Lindley-G, and power Lindley-G discussed, respectively, by [9,10]. For more recently generated families, the reader can refer to [11,12,13,14].
Nowadays, there has been a lot of interest in the families represented by "trigonometric transformations" due to their versatility and ability for modeling a variety of real-world datasets. The sine-G (S-G) family of distributions, which was first presented by Ref. [15], is the first trigonometric family. The cumulative distribution function (CDF) of the S-G family is as follows:
G(t;ζ)=sin(12πF(t;ζ)),t∈R, | (1.1) |
where F(t;ζ) is the baseline CDF of a continuous distribution and ζ is a parameter vector. The following is the probability density function (PDF) associated with CDF (1.1):
g(t;ζ)=12πf(t;ζ)cos(12πF(t;ζ)),t∈R, | (1.2) |
where f(t;ζ) is the PDF corresponding to F(t;ζ). As indicated in Ref. [16], the S-G family offers several benefits, such as the following, (ⅰ) It is straightforward; (ⅱ) the CDF G(t;ζ) and the CDF F(t;ζ) have the same number of parameters that is, no extra parameter is used therefore, there is no risk of over-parameterization; (ⅲ) the trigonometric function enables G(t;ζ) to expand F(t;ζ)'s flexibility, resulting in the creation of new flexible models.
One popular lifespan model that was created by Ref. [17] is the half logistic distribution (HLD). It has attracted significant interest due to its simplicity, tractable mathematical features, and capacity to accommodate survival data. Basically, the HLD is characterized by the survival function (SF) provided by
S(y)=21+eδy;y,δ>0, |
where δ>0, is the scale parameter. Recently, a number of HLD generalizations and extensions have been proposed in an effort to increase or use some of its features. Among them are the inverse HLD [18], generalized HLD [19], exponentiated half logistic-G [20], McDonald-HLD [21], type Ⅱ half logistic-G [22], power- HLD [23], Kumaraswamy-HLD [24], Marshall-Olkin HLD [25], type Ⅰ half logistic Lindley distribution [26], transmuted-HLD [27], type Ⅱ half logistic Weibull distribution [28], half-logistic generalized Weibull distribution [29], type Ⅰ half logistic Burr X-G [30], modified-HLD [31], unit exponentiated-HLD [32], and, for more extended forms, refer to [33,34,35,36].
The main focus here is on a more flexible model, the power-HLD (PHLD) with an extra shape parameter compared to HLD. The CDF and PDF, with scale parameter δ>0 and shape parameter γ>0 of the PHLD, are provided by:
F(t;ζ)=1−21+eδtγ;t>0, | (1.3) |
and
f(t;ζ)=2δγtγ−1eδtγ(1+eδtγ)2;t>0, | (1.4) |
where ζ=(δ,γ).
The requirement for flexible and efficient probability distributions is fundamental in statistics and probability theory. The fact that no one distribution fits every dataset makes academics constantly look for ways to improve the accuracy and flexibility of current models. In light of this, a new two-parameter distribution called the sine-PHLD (SPHLD) was created, which is based on the PHLD. The SPHLD is intended to efficiently simulate datasets with a range of asymmetrical shapes. The following motivates us to recommend the SPHLD.
1) To enhance the versatility of the traditional PHLD in the modeling of different phenomena. The SPHLD can be used to model skewed data from a range of sources, including agricultural and survival times. Additionally, SPHLD performs better than other distributions that are available in the literature, according to our evaluation of its performance on actual data.
2) A few SPHLD features are identified, including the quantile function (QF), moments (both full and incomplete), and stress-strength (SS) reliability.
3) To evaluate the SPHLD parameters' behavior, sixteen estimation strategies are advised. The approaches that have been suggested include percentiles (PC), Kolmogorov, maximum product spacing (MPS), ordinary least squares (OLS), Anderson-Darling (AD), Cramér-von Mises (CVM), left tail AD (LTAD), minimum spacing square distance (MSSD), maximum likelihood (ML), weighted LS (WLS), minimum spacing absolute-log distance (MSALD), right-tail AD (RTAD), AD left tail second order (ADSO), minimum spacing Linex distance (MSLND), minimum spacing absolute distance (MSAD), and minimum spacing square log distance (MSSLD).
4) A comprehensive simulation study is conducted to evaluate the behaviors of different estimators because it is challenging to compare them theoretically. Tables and graphs were included to demonstrate how these methods worked for different parameter values and sample sizes.
5) In comparison to other current models, the SPHLD's adaptability makes it a competitive model for fitting real data. This study illustrates its efficacy by contrasting it with a number of well-known statistical models, such as the exponentiated generalized standard HLD, exponentiated HLD, Poisson generalized HLD, Kumaraswamy HLD, Type-Ⅱ half logistic Weibull distribution, and PHLD. Two practical applications from the fields of agriculture and medical science further demonstrate the SPHLD's superiority.
This article has the following setup: A novel model extending the PHLD is presented in Section 2. The essential properties of the SPHLD distribution are obtained in Section 3. Many methods for estimating model parameters are covered in Section 4. Section 5 uses Monte Carlo simulations to conduct a numerical analysis. Two real datasets are quantitatively examined in Section 6, and the results are presented in Section 7.
In this section, a relatively new flexible distribution model known as the SPHLD is created by setting CDF (1.3) into CDF (1.1). This allows us to get the CDF, which may be represented as
F(t;ζ)=sin[12π(1−21+eδtγ)]=cos(π1+eδtγ);t,δ,γ>0, | (2.1) |
where ζ=(δ,γ) is the set of parameters. The PDF of the SPHLD associated with (2.1) is given by:
f(t;ζ)=δγπtγ−1eδtγ(1+eδtγ)2sin(π1+eδtγ);t,δ,γ>0. | (2.2) |
For γ=1, the PDF (2.2) reduces to the sine HLD (SHLD) as a new sub-model. The SF and hazard rate function (HRF) of the SPHLD are as below:
S(t;ζ)=1−cos(π1+eδtγ), | (2.3) |
and
h(t;ζ)=δγπtγ−1eδtγ(1+eδtγ)2sin(π1+eδtγ)[1−cos(π1+eδtγ)]−1. |
The SPHLD can accurately represent the positively skewed, reverse J-shaped, and unimodal data (left panel in Figure 1) with varying failure rates and decreasing, increasing, and reverse J-shaped failure data (right panel in Figure 1) structures. Figure 2 shows the 3D plots of the PDF and HRF for the SPHLD at δ = 1.5.
Several statistical features, including SS reliability, inverse moments, QF, and central moments, have been covered in the subsections that follow.
The QF of the SPHLD is determined by inverting (2.1) as follows:
Q(p)=[1δln(πcos−1(p)−1)]1/γ;0<p<1 | (3.1) |
Specifically, by setting p = 0.25, 0.5, and 0.75, respectively, in (3.1), the first quartile, or Q1, the second quartile, or Q2, and the third quartile, or Q3, are achieved. Some numerical values of the proposed model quantiles are presented in Table 1.
Parameters | Measures | ||||||||
δ | γ | Q(0.1) | Q(0.25) | Q(0.35) | Q(0.5) | Q(0.6) | Q(0.75) | Q(0.85) | Q(0.95) |
0.15 | 0.5 | 0.724887 | 4.68121 | 9.54363 | 21.3535 | 33.6724 | 64.8572 | 105.342 | 212.237 |
1.0 | 0.851403 | 2.16361 | 3.08928 | 4.62098 | 5.80279 | 8.0534 | 10.2636 | 14.5684 | |
3.0 | 0.947789 | 1.29338 | 1.45642 | 1.66563 | 1.79699 | 2.00444 | 2.1732 | 2.44233 | |
5.0 | 0.968338 | 1.16691 | 1.25306 | 1.35815 | 1.42144 | 1.51773 | 1.59316 | 1.70876 | |
7.0 | 0.977281 | 1.11656 | 1.17484 | 1.24441 | 1.28556 | 1.34718 | 1.39467 | 1.46623 | |
10 | 0.984042 | 1.08023 | 1.1194 | 1.1654 | 1.19224 | 1.23196 | 1.26221 | 1.3072 | |
0.6 | 0.5 | 0.0453054 | 0.292575 | 0.596477 | 1.33459 | 2.10452 | 4.05357 | 6.58386 | 13.2648 |
1.0 | 0.212851 | 0.540902 | 0.772319 | 1.15525 | 1.4507 | 2.01335 | 2.5659 | 3.64209 | |
3.0 | 0.59707 | 0.814779 | 0.917485 | 1.04928 | 1.13203 | 1.26272 | 1.36903 | 1.53857 | |
5.0 | 0.733863 | 0.884349 | 0.949641 | 1.02928 | 1.07725 | 1.15023 | 1.20739 | 1.295 | |
7.0 | 0.801698 | 0.915955 | 0.963765 | 1.02083 | 1.05459 | 1.10514 | 1.1441 | 1.2028 | |
10 | 0.856658 | 0.940398 | 0.974495 | 1.01454 | 1.03791 | 1.07249 | 1.09881 | 1.13798 | |
0.9 | 0.5 | 0.0201357 | 0.130033 | 0.265101 | 0.593152 | 0.935344 | 1.80159 | 2.92616 | 5.89547 |
1.0 | 0.1419 | 0.360602 | 0.514879 | 0.770164 | 0.967132 | 1.34223 | 1.7106 | 2.42806 | |
3.0 | 0.521588 | 0.711775 | 0.801497 | 0.916631 | 0.988922 | 1.10309 | 1.19596 | 1.34406 | |
5.0 | 0.676701 | 0.815465 | 0.875671 | 0.94911 | 0.993338 | 1.06063 | 1.11335 | 1.19413 | |
7.0 | 0.75658 | 0.864407 | 0.909526 | 0.96338 | 0.995237 | 1.04294 | 1.07971 | 1.13511 | |
10 | 0.822618 | 0.903031 | 0.935773 | 0.974223 | 0.996664 | 1.02987 | 1.05515 | 1.09276 | |
1.5 | 0.5 | 0.00724887 | 0.0468121 | 0.0954363 | 0.213535 | 0.336724 | 0.648572 | 1.05342 | 2.12237 |
1.0 | 0.0851403 | 0.216361 | 0.308928 | 0.462098 | 0.580279 | 0.80534 | 1.02636 | 1.45684 | |
3.0 | 0.439925 | 0.600334 | 0.676009 | 0.773116 | 0.834089 | 0.930379 | 1.00871 | 1.13363 | |
5.0 | 0.61098 | 0.736268 | 0.790626 | 0.856933 | 0.896866 | 0.957626 | 1.00522 | 1.07816 | |
7.0 | 0.703335 | 0.803573 | 0.845517 | 0.895581 | 0.925196 | 0.969546 | 1.00372 | 1.05522 | |
10 | 0.781652 | 0.85806 | 0.889172 | 0.925707 | 0.94703 | 0.978584 | 1.00261 | 1.03834 | |
2.5 | 0.5 | 0.00260959 | 0.0168523 | 0.0343571 | 0.0768725 | 0.121221 | 0.233486 | 0.37923 | 0.764053 |
1.0 | 0.0510842 | 0.129817 | 0.185357 | 0.277259 | 0.348167 | 0.483204 | 0.615817 | 0.874101 | |
3.0 | 0.371047 | 0.506341 | 0.570168 | 0.652071 | 0.703498 | 0.784712 | 0.85078 | 0.956138 | |
5.0 | 0.551642 | 0.664762 | 0.713841 | 0.773708 | 0.809762 | 0.864622 | 0.907591 | 0.973447 | |
7.0 | 0.653837 | 0.747021 | 0.786013 | 0.832554 | 0.860085 | 0.901313 | 0.933086 | 0.980961 | |
10 | 0.742726 | 0.815329 | 0.844891 | 0.879607 | 0.899868 | 0.92985 | 0.952676 | 0.986634 | |
4.5 | 0.5 | 0.00080543 | 0.00520134 | 0.010604 | 0.0237261 | 0.0374137 | 0.0720635 | 0.117046 | 0.235819 |
1.0 | 0.0283801 | 0.0721203 | 0.102976 | 0.154033 | 0.193426 | 0.268447 | 0.34212 | 0.485612 | |
3.0 | 0.305027 | 0.416248 | 0.468718 | 0.536049 | 0.578325 | 0.645088 | 0.699401 | 0.786013 | |
5.0 | 0.490459 | 0.591033 | 0.634669 | 0.687896 | 0.719951 | 0.768726 | 0.80693 | 0.865482 | |
7.0 | 0.601176 | 0.686855 | 0.722707 | 0.765499 | 0.790813 | 0.828721 | 0.857935 | 0.901954 | |
10 | 0.700328 | 0.768787 | 0.796661 | 0.829395 | 0.848499 | 0.87677 | 0.898293 | 0.930313 |
Studying the random variable's moments can help in understanding a number of its distribution characteristics. We'll provide the nth moment of the recommended SPHLD below.
E(Tn)=∫∞0tnδγπtγ−1eδtγ(1+eδtγ)2sin(π1+eδtγ)dt. | (3.2) |
The following is the series of the sine function:
sin(t)=∞∑j=0(−1)jt2j+1(2j+1)!. | (3.3) |
Using expansion (3.3) in (3.2) gives
E(Tn)=∞∑j=0(−1)jπ2j+2(2j+1)!∫∞0δγtn+γ−1e−2(j+1)δtγ(1+e−δtγ)2j+3dt. | (3.4) |
The following is the binomial expansion
(1+y)−(a+1)=∞∑i=0(−1)i(a+ii)yi. | (3.5) |
Employing (3.5) in (3.4), we have
E(Tn)=∞∑j,i=0ηi,jδ−(n/γ)(2(j+1)+i)(n/γ)+1Γ(nγ+1), |
where ηi,j=(−1)j+iπ2j+2(2j+1)!(2j+2+ii), Γ(.) is the gamma function (GaF).
Furthermore, using moments around the origin, we may compute the nth central moment of T based on the complete moments as follows:
μn=E[(T−E(T))n]=n∑k=0(−1)k(nk)(E(T))k(E(T))n−k. |
Next, we get skewness coefficient SK=μ3/μ1.52 and kurtosis coefficient KU=μ4/μ22. They are essential for determining whether the SPHLD is symmetrical or asymmetric. In conclusion, Figure 3 offers 3D plots of mean, variance, skewness, and kurtosis for the SPHLD.
The nth incomplete moment (IM) of the SPHLD is obtained by using PDF (2.2) as follows:
ϑn(z)=∫z0tnδγπtγ−1eδtγ(1+eδtγ)2sin(π1+eδtγ)dt. |
Using the expansions (3.2) and (3.4), we have
ϑn(z)=∞∑j,i=0ηi,jz∫0δγtn+γ−1e−(2(j+1)+i)δtγdt=∞∑j,i=0ηi,jδ−(n/γ)((2(j+1)+i))(n/γ)+1Γ(nγ+1,(2(j+1)+i)δzγ), |
where Γ(.,x) is the incomplete GaF. A valuable tool in science, engineering, economics, and demography, the IM is used to estimate Lorenz and Bonferroni curves. These values may be mathematically defined as L(z)=ϑ1(z)/E(T) and B(z)=ϑ1(z)/pE(T). Additionally, it is employed in determining the mean waiting time, say MW(z)=z−ϑ1(z)/F(z;ζ), and the mean residual life, say MR(z)=[1−ϑ1(z)]/S(z;ζ)−z.
The life of a component with random strength T1under random stress T2 is described by the SS model. When the component is subjected to stress greater than its strength, it fails instantly, and when T1>T2, it works properly. Suppose that T1∼SPHLD(δ1,γ) and T2∼SPHLD(δ2,γ), then the SS reliability is given by
β=P(T2<T1)=∫∞0δ1γπtγ−1eδ1tγ(1+eδ1tγ)2sin(π1+eδ1tγ)cos(π1+eδ2tγ)dt, | (3.6) |
since
cos(t)=∞∑j2=0(−1)j2t2j22j2!. | (3.7) |
Then, using expansions (3.3) and (3.7) in (3.6) provide
β=∞∑j1,j2=0Dj1,j2∫∞0δ1γtγ−1e−2(j1+1)δ1tγ(1+e−δ1tγ)2j1+3e−2δ2j2tγ(1+e−δ2tγ)2j2dt, | (3.8) |
where Dj1,j2=(−1)j1+j2π2j1+2j2+2(2j1+1)!2j2!. Again, using the expansion (3.5) two times in (3.8), we obtain
β=∞∑j1,j2,i1,i2=0Dj1,j2Ki1,i2∫∞0δ1γtγ−1e−{δ1[2(j1+1)+i1]+δ2(i2+2j2)}tγdt, | (3.9) |
where Ki1,i2=(−1)i1+i2(2j1+2+i1i1)(2j2−1+i2i2). Hence, (3.9) has the following expression
β=∞∑j1,j2,i1,i2=0Dj1,j2Ki1,i2δ1[δ1[2(j1+1)+i1]+δ2(i2+2j2)]. |
To estimate the SPHLD parameters, we investigate various classical estimation techniques in this section. The proposed methods are AD, MSALD, MPS, ML, Kolmogorov, LTAD, MSAD, CVM, OLS, MSSD, PC, WLS, MSLD, RTAD, ADSO, and MSSLD. In order to enhance the straightforward nature of the estimation techniques, we have incorporated a more comprehensive explanation of the derivation process for every equation.
The SPHLD parameters are estimated in this subsection using the ML technique. Let t1,t2,…,tn be an n observed sample from PDF (2.2). The log-likelihood function is as below:
logℓ∝nlog(δγ)+n∑i=1(γ−1)logti+n∑i=1δtγi−2n∑i=1log(1+eδtγi)+n∑i=1log[sin(π1+eδtγ)]. |
The log-likelihood function can be maximized to determine the ML estimators (MLEs) ˆδ1 and ˆγ1 of the parameters δ and γ. Alternatively, the following differential equations can be solved about of δ and γ
∂logℓ∂δ=nδ+n∑i=1tγi−2n∑i=1tγi1+e−δtγi−n∑i=1πtγi(1+e−δtγi)2cot(π1+eδtγ)=0, | (4.1) |
and
∂logℓ∂γ=nγ+n∑i=1logti+n∑i=1δtγilogti−2n∑i=1δtγilogti(1+e−δtγi)−n∑i=1cot(π1+eδtγ)δπtγilogti(1+e−δtγi)2=0. | (4.2) |
Since the aforementioned Eqs (4.1) and (4.2) have no explicit solutions, in order to determine the MLEs of the SPHLD parameters, nonlinear numerical techniques must be used.
A particular class of minimal distance estimators is the AD estimators. Here, four different estimators for the SPHLD parameters based on the AD approach are determined. These estimators are AD estimators (ADEs), RTAD estimators (RTADEs), ADSO estimators (ADSOEs), and LTAD estimators (LTADEs).
Suppose that t(1),t(2),…,t(n) are the order statistics of a random sample drawn from the SPHLD. Minimizing the following function with respect to the SPHLD parameters, the ADEs ˆδ2 and ˆγ2 of δ and γ are determined
A1(ζ)=−n−1nn∑b=1(2b−1){logF(t(b)|ζ)+log(S(t(n−b+1)|ζ))}, |
where F(.) is the CDF (2.1) and S(.) is the SF (2.3). Solving the following nonlinear equations will also yield the ADEs:
n∑b=1(2b−1){υζ(t(b)|ζ)F(t(b)|ζ)−υζ(t(n−b+1)|ζ)S(t(n−b+1)|ζ)}=0,ζ=(δ,γ), |
where
υδ(t(b)|ζ)=∂F(t(b)|ζ)∂δ=πtγ(b)eδtγ(b)(1+eδtγ(b))2sin(π1+eδtγ(b)), | (4.3) |
and
υγ(t(b)|ζ)=∂F(t(b)|ζ)∂γ=πtγ(b)logt(b)eδtγ(b)(1+eδtγ(b))2sin(π1+eδtγ(b)). | (4.4) |
The RTADEs ˆδ3 and ˆγ3 of parameters δ and γ are determined by minimizing the following function with respect to parameters,
A2(ζ)=n2−2n∑b=1F(t(b)|δ,γ)−1nn∑=1(2b−1)logS(t(n+1−b)|δ,γ)=0, | (4.5) |
where F(.) is the CDF (2.1) and S(.) is the SF (2.3). As an alternative to (4.5), the RTADEs can additionally be obtained by solving the following nonlinear equations:
−2n∑b=1υζ(t(b)|δ,γ)+1nn∑=1(2b−1)S(t(n+1−b)|δ,γ)=0,ζ=(δ,γ), |
where, υζ(t(b)|δ,γ) and ζ=(δ,γ) are given in (4.3) and (4.4).
Next, the ADSOE ˆδ4 and ˆγ4 of parameters δ and γ may be obtained by minimizing the function shown below:
A3(ζ)=2n∑b=1logF(t(b)|δ,γ)+1nn∑b=12b−1F(t(b)|δ,γ), | (4.6) |
where F(.) is the CDF (2.1). The ADSOEs can also be produced by solving the following nonlinear equations as an alternative to Eq (4.6):
2n∑b=1υζ(t(b)|δ,γ)F(t(b)|δ,γ)−1nn∑b=1(2b−1)υζ(t(b)|δ,γ)[F(t(b)|δ,γ)]2=0,ζ=(δ,γ), |
where, υζ(t(b)|δ,γ) and ζ=(δ,γ) are given in (4.3) and (4.4).
The LTADE ˆδ5 and ˆγ5 of parameters δ and γ are determined, respectively, by minimizing the following function:
A4(ζ)=−3n2+2n∑b=1F(t(b)|ζ)−1nn∑b=1(2b−1)logF(t(b)|ζ). | (4.7) |
The following nonlinear equations can be quantitatively solved in place of (4.7) to get ˆδ5 and ˆγ5 respectively:
2n∑b=1F(t(b)|ζ)υζ(t(b)|δ,γ)−1nn∑b=1(2b−1)F(t(b)|ζ)υζ(t(b)|δ,γ)=0,ζ=(δ,γ), |
where, υζ(t(b)|δ,γ) and ζ=(δ,γ) are given in (4.3) and (4.4).
In place of MLE for estimating the unknown parameters of continuous univariate distributions, [37,38] presented the MPS approach. Ranneby [39] demonstrated that in some circumstances, the MPSE asymptotically has the same properties as the MLE and that the MSP method produces consistent estimates while the ML method does not. Let t(1),t(2),…,t(n) be the order statistics of a random sample drawn from the SPHLD. Subsequently, the uniform spacing is established by
ωb(t;δ,γ)=F(t(b)|δ,γ)−F(t(b−1)|δ,γ),b=1,2,...,n+1, |
where F(t(0)|δ,γ)=0,F(t(n+1)|δ,γ)=1, and n+1∑b=1ωb(t;δ,γ)=1. Hence, the following function is maximized with respect to δ and γ to obtain the MPS estimators (MPSEs) of the SPHLD.
D∙(ζ)=1n+1n+1∑b=1log[ωb(t;δ,γ)]. | (4.8) |
It is also possible for assessing the MPSEs ˆδ6 and ˆγ6 of parameters δ and γ by solving the following nonlinear equation.
1n+1n+1∑b=1υζ(t(b)|ζ)−υζ(t(b−1)|ζ)ωb(t;ζ)=0, |
where, υζ(t(b)|ζ) and ζ=(δ,γ) are given in (4.3) and (4.4).
On minimizing the subsequent functions to δ and γ, the OLS estimators (OLSEs) and WLS estimators (WLSEs) of the unknown parameters δ and γ of the SPHLD distribution are obtained
ls∙=n∑b=1(F(t(b)|ζ)−bn+1)2ws∙=n∑b=1(n+1)2(n+2)b(n−b+1)(F(t(b)|ζ)−bn+1)2}, | (4.9) |
where F(.) is the CDF (2.1). In an equivalent manner to (4.9), the OLSEs ˆδ7 and ˆγ7 and WLSEs ˆδ8 and ˆγ8 of unknown parameters δ and γ may be acquired by working out the following equations, with respect to δ and γ :
n∑b=1(F(t(b)|ζ)−bn+1)υζ(t(b)|ζ)=0,n∑b=1(n+1)2(n+2)b(n−b+1)(F(t(b)|ζ)−bn+1)υζ(t(b)|ζ)=0, |
where F(.) is the CDF (2.1), and υζ(t(b)|δ,γ) and ζ=(δ,γ) are given in (4.3) and (4.4).
Here, the MSSD estimators (MSSDEs), MSLND estimators (MSLNDEs), MSALD estimators (MSALDEs), MSAD estimators (MSADEs), and MSSLD estimators (MSSLDEs) are obtained.
The next function is minimized to obtain the MSSDEs ˆδ9 and ˆγ9 of the SPHLD parameters δ and γ :
V1(ζ)=n+1∑b=1(ωb(t;ζ)−1n+1)2, |
where ωb(t;ζ)=F(t(b)|δ,γ)−F(t(b−1)|δ,γ). The MSSDEs ˆδ9 and ˆγ9 are generated by solving the following nonlinear equations:
n+1∑b=1(ωb(t;ζ)−1n+1)[υζ(t(b)|ζ)−υζ(t(b−1)|ζ)]=0, |
where υζ(.|ζ) and ζ=(δ,γ) are given in (4.3) and (4.4).
The function that follows is minimized to provide the MSLNDEs ˆδ10 and ˆγ10 of the SPHLD parameters δ and γ
V2(ζ)=n+1∑b=1(eωb−1n+1−(ωb−1n+1)−1)2. | (4.10) |
The following nonlinear equation can be solved as an alternative to (4.10), in order to find the MSLNDEs ˆδ10 and ˆγ10
n+1∑b=1(eωb−1n+1−(ωb−1n+1)−1)(eωb−1n+1−1)[υζ(t(b)|δ,γ)−υζ(t(b−1)|δ,γ)]=0,ζ=(δ,γ), |
where, υζ(.|ζ) and ζ=(δ,γ) are given in (4.3) and (4.4).
Minimizing the function below yields the MSALDEs ˆδ11 and ˆγ11 of δ and γ
V3(ζ)=n+1∑b=1|logωb(t;ζ)−log1n+1|. |
The MSALDEs ˆδ11 and ˆγ11 are determined by solving the following nonlinear equations:
n+1∑b=1logωb(t;ζ)−log1n+1|logωb(t;ζ)−log1n+1|[υζ(t(b)|ζ)−υζ(t(b−1)|ζ)]1ωb(t;ζ)=0, |
where υζ(.|ζ) and ζ=(δ,γ) are given in (4.3) and (4.4).
The MSADEs ˆδ12 and ˆγ12 of the SPHLD parameters δ and γ are produced by minimizing the following function:
V4(ζ)=n+1∑b=1|ωb(t;ζ)−1n+1|. |
After minimizing the function shown below, the MSADEs ˆδ12 and ˆγ12 are established
n+1∑b=1ωb(t;ζ)−1n+1|ωb(t;ζ)−1n+1|[υζ(t(b)|ζ)−υζ(t(b−1)|ζ)]=0, |
where υζ(.|ζ) and ζ=(δ,γ) are given in (4.3) and (4.4).
The MSSLDEs ˆδ13 and ˆγ13 of parameters δ and γ are produced by minimizing the following function:
V5(ζ)=n+1∑b=1(logωb(t;ζ)−log1n+1)2. | (4.11) |
As opposed to using (4.11), the following nonlinear equation must be solved in order to derive the MSSLDEs ˆδ13 and ˆγ13 :
n+1∑b=1(logωb(t;ζ)−log1n+1)[vζ(t(b)∣zeta)−vζ(t(b−1)∣ζ)]1ωb(t;ζ)=0, |
where υζ(.|ζ) and ζ=(δ,γ) are given in (4.3) and (4.4).
In comparison to other estimators of the same kind, the CVM estimators (CVMEs) are less biased and fall within the category of minimal distance estimators. Finding the difference between the estimated and empirical CDFs allows one to generate these estimators. The function below can be minimized with regard to δ and γ to get the CVMEs ˆδ14 and ˆγ14 of δ and γ
C(ζ)=112n+n∑b=1{F(t(b)|ζ)−2b−12n}2. | (4.12) |
The following nonlinear equation might be solved in place of (4.12), yielding ˆδ14 and ˆγ14
n∑b=1{F(t(b)|ζ)−2b−12n}υζ(t(b)|ζ)=0, |
where υζ(.|δ,γ) and ζ=(δ,γ) are given in (4.3) and (4.4).
In this subsection, the Kolmogorov estimators (KEs) and the PC estimators (PCEs) of the SPHLD parameters are obtained.
The estimation of the SPHLD parameters δ and γ is done using the Kolmogorov method. The KEs ˆδ15 and ˆγ15 are obtained after minimizing the following function:
H(ζ)=Max1≤b≤nn∑b=1[bn−F(t(b)|ζ),F(t(b)|ζ)−b−1n]2, |
where F(.) is the CDF (2.1).
Next, by equating the sample and population percentile values, the PCEs ˆδ16 and ˆγ16 of the unknown parameters δ and γ are produced. The following function can be minimized in relation to δ and γ to get the ˆδ16 and ˆγ16
P=n∑b=1[z(b)−F−1(pb)]2,pb=bn+1. |
Using a significant quantity of simulated data, this section compares the performance of several estimate methods for estimating the parameters of the proposed model. In our simulation, we used the suggested model quantity function to create random datasets for a variety of sample sizes (n=25,75,150,200,250, and 400). In this part, we will investigate the performance and behavior of our model estimators. Furthermore, we will examine the performance of various estimating strategies using a range of metrics, such as average of bias (|Bias(ζ)|=1L∑Li=1|ˆζ−ζ|), mean squared errors (MSE=1L∑Li=1(ˆζ−ζ)2), mean relative errors (MRE=1L∑Li=1|ˆζ−ζ|/ζ), average absolute difference (Dabs=1nL∑Li=1∑nj=1|F(tij|ζ)−F(tij|ˆζ)|), maximum absolute difference (Dmax=1L∑Li=1maxj|F(tij|ζ)−F(tij|ˆζ)|), and average squared absolute error (ASAE=1n∑ni=1|ti−^ti|tn−t1), where the observations ti are in ascending order and ζ=(δ,γ).
Tables 6–10 illustrate the results of simulating the specified model parameters using 16 estimating approaches. Figures 4 through 9 visually show the data from Table 6. It is critical to note that all of the parameter estimates for the proposed distribution are quite trustworthy and reasonably near to their real values. As n increases, all anticipated metrics for each scenario under consideration decline. All of the estimating approaches are quite effective at approximating the recommended model parameters. Table 11 shows that the MPS estimate has the lowest overall score, equal to 67.0 for the criteria included in our investigation, followed by the ML estimate as the second-best method for our investigation with a score of 69.0. Table 11 displays the total rankings for all estimating procedures.
Model | Parameter | SE | Parameter | SE | Parameter | SE |
SPHLD(δ,γ) | 3.7870 | 0.4370 | 2.2162 | 0.1836 | – | – |
EGSHLD(α,β) | 7.0611 | 0.5863 | 3.3169 | 0.4593 | – | – |
EHLD(λ,θ) | 4.7771 | 0.3748 | 2.7077 | 0.3918 | – | – |
PGHLD(α,β,δ) | 0.5901 | 0.0916 | 2.6059 | 0.2187 | 133.3208 | 3.0465 |
KHLD(α,β,θ) | 2.5987 | 0.2161 | 141.1152 | 2.1935 | 0.5731 | 0.0893 |
HLWD(β,θ,δ) | 9.2668 | 0.0013 | 7.7567 | 0.0075 | 0.2865 | 0.0236 |
PHLD(δ,γ) | 6.3117 | 0.7676 | 2.2754 | 0.1877 | – | – |
Models | -2logL | AIC | BIC | CAIC | HQIC | KS | p(KS) | CVM | p(CVM) | AD | p(AD) |
SPHLD | -50.0009 | -46.0009 | -40.6552 | -45.8877 | -43.8338 | 0.0635 | 0.7812 | 0.1027 | 0.5727 | 0.8752 | 0.4295 |
EGSHLD | -18.5522 | -14.5522 | -9.2065 | -14.439 | -12.3851 | 0.1367 | 0.0367 | 0.678 | 0.0142 | 3.9479 | 0.0093 |
EHLD | -20.661 | -16.661 | -11.3154 | -16.5478 | -14.494 | 0.1291 | 0.0566 | 0.6068 | 0.0213 | 3.6361 | 0.0132 |
PGHLD | -41.7841 | -35.7841 | -27.7656 | -35.5556 | -32.5335 | 0.0868 | 0.3958 | 0.2084 | 0.2519 | 1.5726 | 0.1601 |
KHLD | -42.0805 | -36.0805 | -28.062 | -35.8519 | -32.8299 | 0.0865 | 0.4001 | 0.2067 | 0.255 | 1.5561 | 0.1637 |
HLWD | -50.7479 | -44.7479 | -36.7294 | -44.5193 | -41.4973 | 0.083 | 0.4532 | 0.1787 | 0.3131 | 1.088 | 0.3141 |
PHLD | -48.4568 | -44.4568 | -39.1112 | -44.3436 | -42.2898 | 0.0647 | 0.7624 | 0.1082 | 0.5469 | 0.9613 | 0.378 |
Model | parameter | SE | parameter | SE | parameter | SE |
SPHLD(δ,γ) | 0.0134 | 0.0048 | 1.0754 | 0.0806 | – | – |
EGSHLD(α,β) | 0.0271 | 0.0029 | 1.4176 | 0.1774 | – | – |
EHLD(λ,θ) | 0.0330 | 0.0030 | 1.1827 | 0.1406 | – | – |
PGHLD(α,β,δ) | 0.0029 | 6.0E-04 | 1.3095 | 0.0959 | 31.6416 | 3.0606 |
KHLD(α,β,θ) | 1.7763 | 2.0E-04 | 0.1171 | 0.0106 | 0.2100 | 1.0E-04 |
HLWD(β,θ,δ) | 3.2E-05 | 0.0000 | 2.3012 | 0.0925 | 0.5459 | 0.0382 |
PHLD(δ,γ) | 0.0188 | 0.0068 | 1.1120 | 0.0833 | – | – |
Models | -2logL | AIC | BIC | CAIC | HQIC | KS | p(KS) | CVM | p(CVM) | AD | p(AD) |
SPHLD | 1158.5700 | 1162.5700 | 1168.1620 | 1162.4700 | 1164.8410 | 0.0460 | 0.9603 | 0.0697 | 0.7543 | 0.5285 | 0.7176 |
EGSHLD | 1161.6180 | 1165.6180 | 1171.2090 | 1165.7180 | 1167.8890 | 0.0848 | 0.3489 | 0.0784 | 0.7023 | 0.5126 | 0.7337 |
EHLD | 1158.7411 | 1162.7411 | 1168.4002 | 1162.5110 | 1164.9820 | 0.0569 | 0.8286 | 0.0519 | 0.8652 | 0.4096 | 0.8389 |
PGHLD | 1158.6990 | 1164.1990 | 1172.5860 | 1164.4010 | 1167.6050 | 0.0606 | 0.7658 | 0.0522 | 0.8638 | 0.3987 | 0.8498 |
KHLD | 1163.9130 | 1169.9130 | 1178.3000 | 1170.1140 | 1173.3190 | 0.1032 | 0.1521 | 0.1349 | 0.4395 | 0.7670 | 0.5051 |
HLWD | 1158.8090 | 1163.0090 | 1171.3960 | 1163.2110 | 1166.4150 | 0.0538 | 0.8752 | 0.0634 | 0.7934 | 0.4578 | 0.7898 |
PHLD | 1158.6310 | 1162.6310 | 1168.8022 | 1162.5310 | 1164.8702 | 0.0492 | 0.9311 | 0.0597 | 0.8170 | 0.4685 | 0.7788 |
n | Est. | MLE | ADE | CVME | MPSE | OLSE | PCE | RTADE | WLSE | LTADE | MSADE | MSALDE | ADSOE | KE | MSSD | MSSLD | MSLND |
20 | BIAS(ˆδ) | 0.50997{10} | 0.47624{8} | 0.55618{14} | 0.4227{1} | 0.52808{11} | 0.45642{4} | 0.48106{9} | 0.4697{6} | 0.55874{15} | 0.47011{7} | 0.45026{3} | 0.69895{16} | 0.4578{5} | 0.54062{12} | 0.43213{2} | 0.55229{13} |
BIAS(ˆγ) | 0.65945{6} | 0.6582{5} | 0.7497{13} | 0.62205{2} | 0.73052{12} | 0.62897{3} | 0.70944{10} | 0.67628{8} | 0.7155{11} | 0.65291{4} | 0.70363{9} | 0.76909{14} | 0.55328{1} | 0.82507{15} | 0.66467{7} | 0.87293{16} | |
MSE(ˆδ) | 0.48274{13} | 0.3941{8} | 0.58214{15} | 0.27484{1} | 0.46439{12} | 0.33067{4} | 0.40226{9} | 0.37185{7} | 0.53257{14} | 0.3537{5} | 0.3227{3} | 0.7722{16} | 0.36868{6} | 0.4295{10} | 0.31669{2} | 0.44799{11} | |
MSE(ˆγ) | 0.75419{9} | 0.70861{5} | 0.97653{14} | 0.58115{2} | 0.87688{12} | 0.59901{3} | 0.84103{11} | 0.73695{7} | 0.79691{10} | 0.7111{6} | 0.73851{8} | 0.91542{13} | 0.53207{1} | 0.99521{15} | 0.69597{4} | 1.09317{16} | |
MRE(ˆδ) | 0.20399{10} | 0.1905{8} | 0.22247{14} | 0.16908{1} | 0.21123{11} | 0.18257{4} | 0.19243{9} | 0.18788{6} | 0.2235{15} | 0.18804{7} | 0.1801{3} | 0.27958{16} | 0.18312{5} | 0.21625{12} | 0.17285{2} | 0.22092{13} | |
MRE(ˆγ) | 0.16486{6} | 0.16455{5} | 0.18742{13} | 0.15551{2} | 0.18263{12} | 0.15724{3} | 0.17736{10} | 0.16907{8} | 0.17887{11} | 0.16323{4} | 0.17591{9} | 0.19227{14} | 0.13832{1} | 0.20627{15} | 0.16617{7} | 0.21823{16} | |
Dabs | 0.05542{1} | 0.05852{6} | 0.05875{7} | 0.05789{4} | 0.06077{10} | 0.05774{3} | 0.05704{2} | 0.05827{5} | 0.05946{9} | 0.06363{13} | 0.06702{14} | 0.06358{12} | 0.05891{8} | 0.06948{15} | 0.06084{11} | 0.0712{16} | |
Dmax | 0.09015{1} | 0.09333{7} | 0.09632{9} | 0.0904{2} | 0.09725{11} | 0.09107{3} | 0.09261{5} | 0.0929{6} | 0.09717{10} | 0.10099{12} | 0.10555{13} | 0.10614{14} | 0.09162{4} | 0.1109{15} | 0.09595{8} | 0.11392{16} | |
ASAE | 0.04585{1} | 0.04191{7} | 0.04337{9} | 0.04308{2} | 0.04118{11} | 0.04172{3} | 0.04508{5} | 0.04062{6} | 0.04319{10} | 0.05482{12} | 0.05271{13} | 0.05203{14} | 0.04583{4} | 0.05973{15} | 0.04937{8} | 0.06062{16} | |
∑Ranks | 66{7} | 56{6} | 106{13} | 20{1} | 93{11} | 30{2} | 73{9} | 54{4.5} | 101{12} | 72{8} | 75{10} | 127{15} | 40{3} | 124{14} | 54{4.5} | 133{16} | |
70 | BIAS(ˆδ) | 0.23302{3} | 0.2412{4} | 0.30468{12} | 0.22791{1} | 0.27786{11} | 0.23246{2} | 0.26005{8} | 0.25407{7} | 0.32622{15} | 0.27753{10} | 0.24555{5} | 0.49886{16} | 0.26772{9} | 0.31504{14} | 0.24582{6} | 0.30999{13} |
BIAS(ˆγ) | 0.3245{1} | 0.32947{2} | 0.39565{12} | 0.33268{3} | 0.38705{11} | 0.34282{5} | 0.37162{7} | 0.36078{6} | 0.40446{13} | 0.3758{8} | 0.38459{10} | 0.54412{16} | 0.33343{4} | 0.45321{14} | 0.38433{9} | 0.4608{15} | |
MSE(ˆδ) | 0.09308{3} | 0.09963{5} | 0.17211{14} | 0.08165{1} | 0.131{11} | 0.0835{2} | 0.12498{8} | 0.11594{7} | 0.19003{15} | 0.12754{10} | 0.09977{6} | 0.41958{16} | 0.12736{9} | 0.15069{13} | 0.09772{4} | 0.14683{12} | |
MSE(ˆγ) | 0.17324{2} | 0.17331{3} | 0.25132{12} | 0.16753{1} | 0.23172{10} | 0.18154{4} | 0.23363{11} | 0.20815{6} | 0.26128{13} | 0.22809{9} | 0.22537{7} | 0.4488{16} | 0.20041{5} | 0.32895{15} | 0.22666{8} | 0.32661{14} | |
MRE(ˆδ) | 0.09321{3} | 0.09648{4} | 0.12187{12} | 0.09117{1} | 0.11114{11} | 0.09299{2} | 0.10402{8} | 0.10163{7} | 0.13049{15} | 0.11101{10} | 0.09822{5} | 0.19954{16} | 0.10709{9} | 0.12602{14} | 0.09833{6} | 0.12399{13} | |
MRE(ˆγ) | 0.08113{1} | 0.08237{2} | 0.09891{12} | 0.08317{3} | 0.09676{11} | 0.0857{5} | 0.0929{7} | 0.0902{6} | 0.10112{13} | 0.09395{8} | 0.09615{10} | 0.13603{16} | 0.08336{4} | 0.1133{14} | 0.09608{9} | 0.1152{15} | |
Dabs | 0.0291{1} | 0.03155{7} | 0.03136{6} | 0.03121{5} | 0.03271{10} | 0.03079{2} | 0.03097{3} | 0.03118{4} | 0.03217{9} | 0.03687{13} | 0.0343{11} | 0.039{14} | 0.03186{8} | 0.03963{15} | 0.03489{12} | 0.03977{16} | |
Dmax | 0.04706{1} | 0.05079{5} | 0.05236{8} | 0.04993{3} | 0.05362{9} | 0.04926{2} | 0.05056{4} | 0.05084{6} | 0.05385{10} | 0.05874{13} | 0.0553{11} | 0.06884{16} | 0.05146{7} | 0.06406{14} | 0.05617{12} | 0.0649{15} | |
ASAE | 0.02068{1} | 0.0194{5} | 0.02022{8} | 0.02038{3} | 0.0197{9} | 0.01966{2} | 0.02055{4} | 0.01914{6} | 0.02024{10} | 0.0263{13} | 0.0254{11} | 0.02805{16} | 0.02126{7} | 0.02941{14} | 0.02373{12} | 0.02958{15} | |
∑Ranks | 24{1} | 34{4} | 93{11} | 25{2} | 88{10} | 27{3} | 64{6} | 50{5} | 109{13} | 94{12} | 77{8.5} | 140{16} | 65{7} | 128{14} | 77{8.5} | 129{15} | |
150 | BIAS(ˆδ) | 0.15673{2} | 0.15991{3} | 0.18883{10} | 0.15145{1} | 0.18886{11} | 0.16135{4} | 0.1763{8} | 0.16792{6} | 0.22306{15} | 0.19224{12} | 0.17614{7} | 0.37121{16} | 0.17811{9} | 0.21099{13} | 0.16783{5} | 0.21401{14} |
BIAS(ˆγ) | 0.21048{1} | 0.23683{4} | 0.26398{12} | 0.22694{2} | 0.25585{10} | 0.23069{3} | 0.25039{8} | 0.24322{6} | 0.28777{13} | 0.26282{11} | 0.25477{9} | 0.39249{16} | 0.23897{5} | 0.32228{15} | 0.24795{7} | 0.31651{14} | |
MSE(ˆδ) | 0.03876{2} | 0.0421{4} | 0.05973{11} | 0.03526{1} | 0.06179{12} | 0.03983{3} | 0.05052{8} | 0.04654{5} | 0.0843{15} | 0.05917{10} | 0.04776{7} | 0.22463{16} | 0.05493{9} | 0.07{13} | 0.04684{6} | 0.07287{14} | |
MSE(ˆγ) | 0.07143{1} | 0.08939{4} | 0.10989{11} | 0.07985{2} | 0.10559{10} | 0.08356{3} | 0.10142{8} | 0.09184{5} | 0.1303{13} | 0.11125{12} | 0.10348{9} | 0.24262{16} | 0.0989{7} | 0.15864{15} | 0.09643{6} | 0.15711{14} | |
MRE(ˆδ) | 0.06269{2} | 0.06396{3} | 0.07553{10} | 0.06058{1} | 0.07554{11} | 0.06454{4} | 0.07052{8} | 0.06717{6} | 0.08923{15} | 0.07689{12} | 0.07046{7} | 0.14849{16} | 0.07124{9} | 0.0844{13} | 0.06713{5} | 0.0856{14} | |
MRE(ˆγ) | 0.05262{1} | 0.05921{4} | 0.066{12} | 0.05674{2} | 0.06396{10} | 0.05767{3} | 0.0626{8} | 0.06081{6} | 0.07194{13} | 0.06571{11} | 0.06369{9} | 0.09812{16} | 0.05974{5} | 0.08057{15} | 0.06199{7} | 0.07913{14} | |
Dabs | 0.02054{1} | 0.02072{2} | 0.02154{6.5} | 0.0209{3} | 0.02214{8} | 0.02154{6.5} | 0.0215{5} | 0.02124{4} | 0.02222{10} | 0.02553{13} | 0.02417{12} | 0.02878{15} | 0.02217{9} | 0.029{16} | 0.02327{11} | 0.02835{14} | |
Dmax | 0.03296{1} | 0.03367{3} | 0.03572{7} | 0.03363{2} | 0.03616{9} | 0.03467{5} | 0.03506{6} | 0.03443{4} | 0.03725{10} | 0.04088{13} | 0.03877{12} | 0.0513{16} | 0.03581{8} | 0.04664{15} | 0.03742{11} | 0.04585{14} | |
ASAE | 0.01285{1} | 0.0121{3} | 0.01253{7} | 0.01291{2} | 0.0126{9} | 0.0126{5} | 0.01309{6} | 0.01218{4} | 0.01276{10} | 0.01719{13} | 0.01618{12} | 0.01927{16} | 0.0134{8} | 0.01885{15} | 0.01518{11} | 0.01927{14} | |
∑Ranks | 18{1} | 28{3} | 82.5{9} | 22{2} | 86{11} | 35.5{4} | 68{6} | 44{5} | 110{13} | 107{12} | 84{10} | 143{16} | 71{8} | 129{15} | 69{7} | 127{14} | |
200 | BIAS(ˆδ) | 0.13265{2} | 0.13595{3} | 0.1673{11} | 0.13118{1} | 0.16857{12} | 0.13838{5} | 0.13802{4} | 0.14025{6} | 0.18959{15} | 0.16663{10} | 0.15183{8} | 0.33625{16} | 0.16201{9} | 0.18672{14} | 0.14916{7} | 0.1847{13} |
BIAS(ˆγ) | 0.1819{1} | 0.2009{4} | 0.23223{11} | 0.19663{2} | 0.23062{10} | 0.19721{3} | 0.21404{7} | 0.20405{5} | 0.24252{13} | 0.23595{12} | 0.22556{9} | 0.35139{16} | 0.21768{8} | 0.26822{14} | 0.21092{6} | 0.28227{15} | |
MSE(ˆδ) | 0.02876{2} | 0.03111{5} | 0.04706{12} | 0.02608{1} | 0.04487{11} | 0.02986{3} | 0.02999{4} | 0.03198{6} | 0.05815{15} | 0.04388{9} | 0.03648{8} | 0.17779{16} | 0.04457{10} | 0.0543{14} | 0.03468{7} | 0.05337{13} | |
MSE(ˆγ) | 0.05319{1} | 0.06643{5} | 0.0866{11} | 0.05773{2} | 0.08181{9} | 0.06042{3} | 0.07294{7} | 0.0649{4} | 0.09347{13} | 0.0891{12} | 0.07835{8} | 0.18831{16} | 0.08335{10} | 0.10843{14} | 0.07018{6} | 0.12237{15} | |
MRE(ˆδ) | 0.05306{2} | 0.05438{3} | 0.06692{11} | 0.05247{1} | 0.06743{12} | 0.05535{5} | 0.05521{4} | 0.0561{6} | 0.07583{15} | 0.06665{10} | 0.06073{8} | 0.1345{16} | 0.0648{9} | 0.07469{14} | 0.05966{7} | 0.07388{13} | |
MRE(ˆγ) | 0.04548{1} | 0.05022{4} | 0.05806{11} | 0.04916{2} | 0.05765{10} | 0.0493{3} | 0.05351{7} | 0.05101{5} | 0.06063{13} | 0.05899{12} | 0.05639{9} | 0.08785{16} | 0.05442{8} | 0.06705{14} | 0.05273{6} | 0.07057{15} | |
Dabs | 0.01756{1} | 0.01875{6} | 0.01966{9} | 0.01806{2} | 0.01886{7} | 0.01851{3} | 0.01865{5} | 0.01857{4} | 0.02{10} | 0.02234{13} | 0.02071{12} | 0.02553{16} | 0.01939{8} | 0.02374{14} | 0.02001{11} | 0.02467{15} | |
Dmax | 0.02827{1} | 0.03015{5} | 0.03226{10} | 0.02899{2} | 0.03112{7} | 0.02971{3} | 0.03023{6} | 0.02997{4} | 0.03328{11} | 0.03587{13} | 0.03331{12} | 0.04625{16} | 0.03149{8} | 0.03869{14} | 0.03217{9} | 0.03998{15} | |
ASAE | 0.01079{1} | 0.01033{5} | 0.01064{10} | 0.01096{2} | 0.01058{7} | 0.0105{3} | 0.01128{6} | 0.01037{4} | 0.01071{11} | 0.01466{13} | 0.0135{12} | 0.01705{16} | 0.01127{8} | 0.01565{14} | 0.01282{9} | 0.01625{15} | |
∑Ranks | 18{1} | 36{4} | 91{11} | 21{2} | 82{9} | 31{3} | 54{6} | 42{5} | 111{13} | 104{12} | 86{10} | 144{16} | 79{8} | 126{14} | 70{7} | 129{15} | |
300 | BIAS(ˆδ) | 0.10974{2} | 0.11844{5} | 0.13179{9} | 0.10665{1} | 0.13783{12} | 0.1132{3} | 0.11493{4} | 0.11974{6} | 0.14628{13} | 0.13581{11} | 0.12478{8} | 0.2783{16} | 0.1341{10} | 0.15588{15} | 0.12086{7} | 0.14797{14} |
BIAS(ˆγ) | 0.14742{1} | 0.16142{3} | 0.18156{10} | 0.16198{4} | 0.1938{12} | 0.1533{2} | 0.16496{5} | 0.17827{7} | 0.1921{11} | 0.19653{13} | 0.17965{8} | 0.28618{16} | 0.18104{9} | 0.22094{15} | 0.17758{6} | 0.2143{14} | |
MSE(ˆδ) | 0.01875{2} | 0.02203{5} | 0.02817{9} | 0.01818{1} | 0.03043{12} | 0.02021{3} | 0.02064{4} | 0.02295{7} | 0.03499{13} | 0.02881{11} | 0.02412{8} | 0.12932{16} | 0.0286{10} | 0.03896{15} | 0.02229{6} | 0.03584{14} | |
MSE(ˆγ) | 0.03488{1} | 0.04131{4} | 0.05192{9} | 0.04036{3} | 0.05898{12} | 0.03722{2} | 0.04372{5} | 0.04954{7} | 0.05812{11} | 0.06262{13} | 0.05007{8} | 0.13548{16} | 0.05346{10} | 0.07594{15} | 0.04802{6} | 0.07302{14} | |
MRE(ˆδ) | 0.0439{2} | 0.04737{5} | 0.05272{9} | 0.04266{1} | 0.05513{12} | 0.04528{3} | 0.04597{4} | 0.0479{6} | 0.05851{13} | 0.05433{11} | 0.04991{8} | 0.11132{16} | 0.05364{10} | 0.06235{15} | 0.04834{7} | 0.05919{14} | |
MRE(ˆγ) | 0.03685{1} | 0.04036{3} | 0.04539{10} | 0.04049{4} | 0.04845{12} | 0.03832{2} | 0.04124{5} | 0.04457{7} | 0.04802{11} | 0.04913{13} | 0.04491{8} | 0.07155{16} | 0.04526{9} | 0.05524{15} | 0.04439{6} | 0.05357{14} | |
Dabs | 0.01362{1} | 0.01495{6} | 0.01533{8} | 0.01478{5} | 0.01539{9} | 0.01426{2} | 0.01476{4} | 0.01472{3} | 0.01529{7} | 0.01826{13} | 0.01734{12} | 0.02195{16} | 0.01583{10} | 0.0199{14} | 0.0161{11} | 0.02005{15} | |
Dmax | 0.02211{1} | 0.02424{6} | 0.02529{7} | 0.02366{3} | 0.02549{8} | 0.02312{2} | 0.02388{4} | 0.02416{5} | 0.0255{9} | 0.02944{13} | 0.02777{12} | 0.03943{16} | 0.0259{10} | 0.03232{15} | 0.02613{11} | 0.0323{14} | |
ASAE | 0.00851{1} | 0.00811{6} | 0.00842{7} | 0.00842{3} | 0.00824{8} | 0.00839{2} | 0.00871{4} | 0.00814{5} | 0.00844{9} | 0.01156{13} | 0.0109{12} | 0.01371{16} | 0.00883{10} | 0.01255{15} | 0.01019{11} | 0.01271{14} | |
∑Ranks | 19{1} | 38{4} | 77{8} | 27{3} | 92{11} | 23{2} | 44{5} | 50{6} | 95{12} | 111{13} | 84{9} | 144{16} | 88{10} | 133{15} | 71{7} | 128{14} | |
450 | BIAS(ˆδ) | 0.08895{2} | 0.09072{3} | 0.11445{12} | 0.08675{1} | 0.10738{10} | 0.09073{4} | 0.09415{5} | 0.0949{6} | 0.12204{13} | 0.11394{11} | 0.10417{8} | 0.22716{16} | 0.10475{9} | 0.12807{15} | 0.09963{7} | 0.12233{14} |
BIAS(ˆγ) | 0.1248{1} | 0.13118{4} | 0.15604{12} | 0.12656{2} | 0.15209{10} | 0.13038{3} | 0.15018{9} | 0.13814{5} | 0.15294{11} | 0.16044{13} | 0.14765{8} | 0.24681{16} | 0.14338{6} | 0.17673{15} | 0.14537{7} | 0.17526{14} | |
MSE(ˆδ) | 0.01257{2} | 0.01291{4} | 0.02057{11} | 0.01176{1} | 0.01849{10} | 0.01269{3} | 0.01407{5} | 0.01462{6} | 0.02287{13} | 0.02088{12} | 0.01688{8} | 0.08455{16} | 0.0176{9} | 0.02511{15} | 0.01571{7} | 0.02359{14} | |
MSE(ˆγ) | 0.0249{1} | 0.02715{4} | 0.03896{12} | 0.02562{2} | 0.03687{10} | 0.02638{3} | 0.03486{9} | 0.03042{5} | 0.03691{11} | 0.04153{13} | 0.03326{7} | 0.09873{16} | 0.03448{8} | 0.04877{15} | 0.03238{6} | 0.04832{14} | |
MRE(ˆδ) | 0.03558{2} | 0.03629{3.5} | 0.04578{12} | 0.0347{1} | 0.04295{10} | 0.03629{3.5} | 0.03766{5} | 0.03796{6} | 0.04881{13} | 0.04558{11} | 0.04167{8} | 0.09086{16} | 0.0419{9} | 0.05123{15} | 0.03985{7} | 0.04893{14} | |
MRE(ˆγ) | 0.0312{1} | 0.0328{4} | 0.03901{12} | 0.03164{2} | 0.03802{10} | 0.0326{3} | 0.03755{9} | 0.03453{5} | 0.03823{11} | 0.04011{13} | 0.03691{8} | 0.0617{16} | 0.03584{6} | 0.04418{15} | 0.03634{7} | 0.04381{14} | |
Dabs | 0.01206{3} | 0.0118{1} | 0.01283{9} | 0.01195{2} | 0.01252{6} | 0.0122{4} | 0.01285{10} | 0.01236{5} | 0.01279{8} | 0.0151{13} | 0.01376{12} | 0.01756{16} | 0.01274{7} | 0.01662{15} | 0.01351{11} | 0.01591{14} | |
Dmax | 0.0194{3} | 0.01913{1} | 0.02112{9} | 0.01918{2} | 0.02062{6} | 0.01963{4} | 0.0208{8} | 0.02007{5} | 0.02128{10} | 0.02436{13} | 0.02228{12} | 0.03163{16} | 0.02078{7} | 0.02684{15} | 0.02176{11} | 0.02572{14} | |
ASAE | 0.0068{3} | 0.00657{1} | 0.00666{9} | 0.0068{2} | 0.00659{6} | 0.00666{4} | 0.00675{8} | 0.00645{5} | 0.00672{10} | 0.00925{13} | 0.00841{12} | 0.01085{16} | 0.00715{7} | 0.01005{15} | 0.00797{11} | 0.01017{14} | |
∑Ranks | 24{2} | 26.5{3} | 94{11} | 21{1} | 75{9} | 31.5{4} | 67{6} | 44{5} | 96{12} | 112{13} | 83{10} | 144{16} | 71{7} | 134{15} | 74{8} | 127{14} |
n | Est. | MLE | ADE | CVME | MPSE | OLSE | PCE | RTADE | WLSE | LTADE | MSADE | MSALDE | ADSOE | KE | MSSD | MSSLD | MSLND |
20 | BIAS(ˆδ) | 0.13569{4} | 0.13611{5} | 0.14304{12} | 0.11914{1} | 0.13443{3} | 0.23732{16} | 0.13881{8} | 0.13078{2} | 0.145{13} | 0.14272{11} | 0.13867{7} | 0.15516{15} | 0.14722{14} | 0.13911{9} | 0.13978{10} | 0.13755{6} |
BIAS(ˆγ) | 0.04852{2} | 0.04836{1} | 0.06184{11} | 0.04882{3} | 0.05532{8} | 0.10105{16} | 0.05227{6} | 0.05221{5} | 0.05666{9} | 0.0622{12} | 0.05323{7} | 0.0738{15} | 0.05983{10} | 0.06465{13} | 0.04883{4} | 0.06543{14} | |
MSE(ˆδ) | 0.02924{3} | 0.03072{6.5} | 0.03339{12} | 0.02293{1} | 0.02941{4} | 0.08556{16} | 0.03083{9} | 0.02791{2} | 0.03622{14} | 0.03338{11} | 0.03296{10} | 0.04423{15} | 0.0359{13} | 0.03072{6.5} | 0.03037{5} | 0.03074{8} | |
MSE(ˆγ) | 0.00409{4} | 0.0038{3} | 0.00673{14} | 0.00366{1} | 0.00511{8} | 0.01506{16} | 0.00465{7} | 0.00459{6} | 0.00576{9} | 0.00621{12} | 0.00433{5} | 0.00882{15} | 0.00612{10} | 0.00618{11} | 0.00373{2} | 0.00633{13} | |
MRE(ˆδ) | 0.16961{4} | 0.17014{5} | 0.17881{12} | 0.14892{1} | 0.16804{3} | 0.29665{16} | 0.17352{8} | 0.16347{2} | 0.18125{13} | 0.1784{11} | 0.17334{7} | 0.19395{15} | 0.18402{14} | 0.17389{9} | 0.17473{10} | 0.17193{6} | |
MRE(ˆγ) | 0.16174{2} | 0.1612{1} | 0.20613{11} | 0.16273{3} | 0.18441{8} | 0.33685{16} | 0.17424{6} | 0.17403{5} | 0.18886{9} | 0.20735{12} | 0.17743{7} | 0.24601{15} | 0.19943{10} | 0.21551{13} | 0.16277{4} | 0.21812{14} | |
Dabs | 0.05531{1} | 0.05792{3} | 0.06031{6} | 0.05725{2} | 0.06112{9} | 0.10551{16} | 0.05954{5} | 0.05815{4} | 0.06079{8} | 0.06665{12} | 0.06419{11} | 0.06793{13} | 0.06068{7} | 0.07137{15} | 0.06132{10} | 0.07046{14} | |
Dmax | 0.09073{2} | 0.09264{3} | 0.10036{8} | 0.0902{1} | 0.09881{7} | 0.17373{16} | 0.09574{5} | 0.09359{4} | 0.1012{9} | 0.10726{12} | 0.10174{11} | 0.11822{15} | 0.10136{10} | 0.11505{14} | 0.09648{6} | 0.11428{13} | |
ASAE | 0.04603{2} | 0.04811{3} | 0.05374{8} | 0.04434{1} | 0.06565{7} | 0.0267{16} | 0.0424{5} | 0.04964{4} | 0.08063{9} | 0.04583{12} | 0.04342{11} | 0.42444{15} | 0.05812{10} | 0.0862{14} | 0.05103{6} | 0.08934{13} | |
∑Ranks | 28{2} | 34.5{3} | 96{9} | 17{1} | 62{7} | 129{15} | 56{5} | 38{4} | 97{10} | 98{11} | 68{8} | 134{16} | 99{12} | 104.5{14} | 60{6} | 103{13} | |
70 | BIAS(ˆδ) | 0.07296{5} | 0.06964{1} | 0.07359{7} | 0.07124{3} | 0.07313{6} | 0.18982{16} | 0.07141{4} | 0.06974{2} | 0.07362{8} | 0.0874{13} | 0.0797{11} | 0.08887{15} | 0.07713{10} | 0.08667{12} | 0.0757{9} | 0.08745{14} |
BIAS(ˆγ) | 0.02459{1} | 0.02612{5} | 0.03127{11} | 0.02602{4} | 0.0292{8} | 0.0696{16} | 0.02598{3} | 0.02544{2} | 0.02942{9} | 0.03295{12} | 0.02869{7} | 0.04133{15} | 0.03019{10} | 0.03481{14} | 0.02684{6} | 0.03435{13} | |
MSE(ˆδ) | 0.00838{5} | 0.00771{1.5} | 0.00865{7} | 0.00801{3} | 0.0085{6} | 0.0611{16} | 0.00817{4} | 0.00771{1.5} | 0.00869{8} | 0.01225{12} | 0.01009{11} | 0.01295{15} | 0.00958{10} | 0.01229{13} | 0.00905{9} | 0.01253{14} | |
MSE(ˆγ) | 0.00099{1} | 0.00108{4} | 0.00163{11} | 0.00104{3} | 0.00135{8} | 0.00721{16} | 0.00111{5} | 0.00103{2} | 0.00144{9} | 0.00171{12} | 0.00125{7} | 0.00265{15} | 0.00151{10} | 0.00187{14} | 0.00114{6} | 0.0018{13} | |
MRE(ˆδ) | 0.09119{5} | 0.08705{1} | 0.09199{7} | 0.08905{3} | 0.09141{6} | 0.23728{16} | 0.08926{4} | 0.08718{2} | 0.09203{8} | 0.10925{13} | 0.09963{11} | 0.11109{15} | 0.09642{10} | 0.10834{12} | 0.09462{9} | 0.10931{14} | |
MRE(ˆγ) | 0.08196{1} | 0.08707{5} | 0.10422{11} | 0.08674{4} | 0.09732{8} | 0.232{16} | 0.08659{3} | 0.0848{2} | 0.09806{9} | 0.10984{12} | 0.09562{7} | 0.13777{15} | 0.10063{10} | 0.11604{14} | 0.08946{6} | 0.1145{13} | |
Dabs | 0.03066{4} | 0.03051{2} | 0.0325{7} | 0.03188{5} | 0.03278{8} | 0.07892{16} | 0.03045{1} | 0.03058{3} | 0.03232{6} | 0.03856{12} | 0.03556{11} | 0.04174{15} | 0.0333{10} | 0.04018{13} | 0.03322{9} | 0.04055{14} | |
Dmax | 0.04975{3.5} | 0.04967{2} | 0.05402{8} | 0.05105{5} | 0.05348{7} | 0.12657{16} | 0.04963{1} | 0.04975{3.5} | 0.05403{9} | 0.06225{12} | 0.0567{11} | 0.07376{15} | 0.0551{10} | 0.06547{14} | 0.05338{6} | 0.06543{13} | |
ASAE | 0.0162{3.5} | 0.01797{2} | 0.02094{8} | 0.01643{5} | 0.02195{7} | 0.01337{16} | 0.01615{1} | 0.01862{3.5} | 0.02546{9} | 0.01928{12} | 0.01803{11} | 0.09751{15} | 0.02182{10} | 0.02609{14} | 0.01813{6} | 0.02645{13} | |
∑Ranks | 28.5{4} | 26.5{2} | 79{8.5} | 34{5} | 69{7} | 129{15} | 27{3} | 26{1} | 79{8.5} | 107{12} | 82{10} | 136{16} | 91{11} | 120{13} | 67{6} | 123{14} | |
150 | BIAS(ˆδ) | 0.04684{1} | 0.04829{3} | 0.04887{4.5} | 0.04806{2} | 0.04987{7} | 0.17968{16} | 0.04887{4.5} | 0.04975{6} | 0.05064{10} | 0.05928{12} | 0.05586{11} | 0.06197{13} | 0.05002{8} | 0.06334{14} | 0.05037{9} | 0.06384{15} |
BIAS(ˆγ) | 0.01666{1} | 0.01759{4} | 0.02009{10} | 0.01747{2} | 0.02006{9} | 0.06159{16} | 0.01832{5} | 0.0185{6} | 0.02003{8} | 0.0217{12} | 0.0192{7} | 0.02988{15} | 0.02063{11} | 0.02394{13} | 0.01753{3} | 0.02409{14} | |
MSE(ˆδ) | 0.00343{1} | 0.00367{3} | 0.00382{5} | 0.00363{2} | 0.00389{7} | 0.05875{16} | 0.00372{4} | 0.00387{6} | 0.00409{10} | 0.00543{12} | 0.00503{11} | 0.0063{14} | 0.00394{8} | 0.00615{13} | 0.00404{9} | 0.00634{15} | |
MSE(ˆγ) | 0.00045{1} | 0.00048{4} | 0.00064{10} | 0.00046{2} | 0.00062{8.5} | 0.00575{16} | 0.00054{5.5} | 0.00054{5.5} | 0.00062{8.5} | 0.00073{12} | 0.00056{7} | 0.0014{15} | 7e−04{11} | 9e−04{14} | 0.00047{3} | 0.00088{13} | |
MRE(ˆδ) | 0.05855{1} | 0.06037{3} | 0.06108{4.5} | 0.06008{2} | 0.06234{7} | 0.2246{16} | 0.06108{4.5} | 0.06219{6} | 0.0633{10} | 0.0741{12} | 0.06982{11} | 0.07746{13} | 0.06253{8} | 0.07917{14} | 0.06296{9} | 0.0798{15} | |
MRE(ˆγ) | 0.05553{1} | 0.05865{4} | 0.06697{10} | 0.05823{2} | 0.06687{9} | 0.2053{16} | 0.06107{5} | 0.06166{6} | 0.06675{8} | 0.07234{12} | 0.06399{7} | 0.09959{15} | 0.06876{11} | 0.07981{13} | 0.05845{3} | 0.08029{14} | |
Dabs | 0.02014{1} | 0.02095{2} | 0.02155{5} | 0.02132{3} | 0.02237{8} | 0.07261{16} | 0.02134{4} | 0.02169{6} | 0.02256{10} | 0.02615{12} | 0.02437{11} | 0.02995{15} | 0.02244{9} | 0.0284{13} | 0.02199{7} | 0.02844{14} | |
Dmax | 0.03246{1} | 0.03388{2} | 0.03559{7} | 0.03417{3} | 0.03664{8} | 0.11597{16} | 0.03474{4} | 0.03524{5} | 0.0373{10} | 0.04235{12} | 0.0391{11} | 0.05332{15} | 0.03699{9} | 0.04607{13} | 0.03549{6} | 0.04632{14} | |
ASAE | 0.00893{1} | 0.01018{2} | 0.01121{7} | 0.00853{3} | 0.01162{8} | 0.00883{16} | 0.009{4} | 0.00981{5} | 0.01321{10} | 0.01058{12} | 0.00981{11} | 0.03661{15} | 0.01211{9} | 0.01329{13} | 0.00993{6} | 0.01331{14} | |
∑Ranks | 11{1} | 33{3} | 66{7} | 19{2} | 74.5{8} | 128{14.5} | 40.5{4} | 52.5{5} | 87.5{10} | 104{12} | 81{9} | 129{16} | 91{11} | 123{13} | 56{6} | 128{14.5} | |
200 | BIAS(ˆδ) | 0.04341{8} | 0.03894{1} | 0.04242{5} | 0.04287{7} | 0.04155{3} | 0.16861{16} | 0.04223{4} | 0.04141{2} | 0.0428{6} | 0.0525{12} | 0.04708{10} | 0.05372{15} | 0.04369{9} | 0.05334{14} | 0.04767{11} | 0.05288{13} |
BIAS(ˆγ) | 0.01466{1} | 0.0152{3} | 0.01737{9} | 0.01482{2} | 0.01744{10} | 0.05522{16} | 0.01611{5} | 0.01546{4} | 0.01751{11} | 0.019{12} | 0.01669{7} | 0.026{15} | 0.01733{8} | 0.02049{13} | 0.01646{6} | 0.02067{14} | |
MSE(ˆδ) | 0.00301{8} | 0.00239{1} | 0.00281{4} | 0.0029{6} | 0.00279{3} | 0.05237{16} | 0.00282{5} | 0.00268{2} | 0.00291{7} | 0.00451{13} | 0.00352{10} | 0.00452{14} | 0.00307{9} | 0.00453{15} | 0.00356{11} | 0.00449{12} | |
MSE(ˆγ) | 0.00035{2} | 0.00038{3} | 0.00047{8} | 0.00034{1} | 0.00048{9} | 0.00465{16} | 4e−04{5} | 0.00039{4} | 0.00049{10.5} | 0.00056{12} | 0.00044{7} | 0.00111{15} | 0.00049{10.5} | 0.00064{13} | 0.00042{6} | 0.00067{14} | |
MRE(ˆδ) | 0.05427{8} | 0.04867{1} | 0.05302{5} | 0.05358{7} | 0.05194{3} | 0.21077{16} | 0.05278{4} | 0.05176{2} | 0.05349{6} | 0.06562{12} | 0.05885{10} | 0.06715{15} | 0.05462{9} | 0.06668{14} | 0.05959{11} | 0.06611{13} | |
MRE(ˆγ) | 0.04887{1} | 0.05067{3} | 0.05789{9} | 0.04941{2} | 0.05813{10} | 0.18407{16} | 0.05369{5} | 0.05154{4} | 0.05836{11} | 0.06333{12} | 0.05564{7} | 0.08668{15} | 0.05776{8} | 0.0683{13} | 0.05488{6} | 0.0689{14} | |
Dabs | 0.01861{4} | 0.01734{1} | 0.01893{7} | 0.01868{5} | 0.0188{6} | 0.06765{16} | 0.01843{3} | 0.01809{2} | 0.01907{8} | 0.02302{12} | 0.02074{11} | 0.02596{15} | 0.01938{9} | 0.0239{13} | 0.02062{10} | 0.02397{14} | |
Dmax | 0.02971{3} | 0.02823{1} | 0.03122{7} | 0.02995{4} | 0.03106{6} | 0.10653{16} | 0.02997{5} | 0.02958{2} | 0.0319{8} | 0.03705{12} | 0.03334{11} | 0.04661{15} | 0.03204{9} | 0.03867{13} | 0.03292{10} | 0.03921{14} | |
ASAE | 0.00707{3} | 0.00822{1} | 0.00916{7} | 0.00728{4} | 0.00916{6} | 0.00736{16} | 0.00708{5} | 0.00804{2} | 0.01016{8} | 0.00844{12} | 0.00794{11} | 0.02823{15} | 0.0094{9} | 0.01004{13} | 0.00783{10} | 0.0105{14} | |
∑Ranks | 36{3} | 22{1} | 64{7} | 37{4} | 59{6} | 131{15} | 49{5} | 29{2} | 80.5{10} | 105{12} | 78{9} | 134{16} | 82.5{11} | 120{13} | 75{8} | 122{14} | |
300 | BIAS(ˆδ) | 0.03359{1} | 0.03426{4} | 0.03522{5} | 0.03414{3} | 0.0339{2} | 0.15982{16} | 0.03524{6} | 0.03559{7} | 0.03562{8} | 0.04236{12} | 0.03961{11} | 0.04313{13} | 0.03677{9} | 0.04397{14} | 0.03893{10} | 0.04451{15} |
BIAS(ˆγ) | 0.01137{1} | 0.01228{3} | 0.01405{9} | 0.01219{2} | 0.01354{7} | 0.05028{16} | 0.01315{5} | 0.01242{4} | 0.01412{10} | 0.01532{12} | 0.01366{8} | 0.02121{15} | 0.01461{11} | 0.01687{14} | 0.01337{6} | 0.01621{13} | |
MSE(ˆδ) | 0.00174{1} | 0.00183{3} | 0.00192{5} | 0.00187{4} | 0.0018{2} | 0.04814{16} | 0.00198{7} | 0.00197{6} | 0.00199{8} | 0.00292{12} | 0.00245{11} | 0.003{13} | 0.00214{9} | 0.00314{14} | 0.00231{10} | 0.00318{15} | |
MSE(ˆγ) | 0.00021{1} | 0.00023{2.5} | 0.00032{9.5} | 0.00023{2.5} | 0.00029{8} | 0.00397{16} | 0.00027{5} | 0.00025{4} | 0.00032{9.5} | 0.00036{12} | 0.00028{6.5} | 0.00074{15} | 0.00033{11} | 0.00045{14} | 0.00028{6.5} | 0.00043{13} | |
MRE(ˆδ) | 0.04199{1} | 0.04282{4} | 0.04403{5} | 0.04268{3} | 0.04238{2} | 0.19977{16} | 0.04404{6} | 0.04449{7} | 0.04453{8} | 0.05295{12} | 0.04952{11} | 0.05391{13} | 0.04597{9} | ||||
MRE() | |||||||||||||||||
ASAE | |||||||||||||||||
450 | BIAS() | ||||||||||||||||
BIAS() | |||||||||||||||||
MSE() | |||||||||||||||||
MSE() | |||||||||||||||||
MRE() | |||||||||||||||||
MRE() | |||||||||||||||||
ASAE | |||||||||||||||||
n | Est. | MLE | ADE | CVME | MPSE | OLSE | PCE | RTADE | WLSE | LTADE | MSADE | MSALDE | ADSOE | KE | MSSD | MSSLD | MSLND |
20 | BIAS() | ||||||||||||||||
BIAS() | |||||||||||||||||
MSE() | |||||||||||||||||
MSE() | |||||||||||||||||
MRE() | |||||||||||||||||
MRE() | |||||||||||||||||
ASAE | |||||||||||||||||
70 | BIAS() | ||||||||||||||||
BIAS() | |||||||||||||||||
MSE() | |||||||||||||||||
MSE() | |||||||||||||||||
MRE() | |||||||||||||||||
MRE() | |||||||||||||||||
ASAE | |||||||||||||||||
150 | BIAS() | ||||||||||||||||
BIAS() | |||||||||||||||||
MSE() | |||||||||||||||||
MSE() | |||||||||||||||||
MRE() | |||||||||||||||||
MRE() | |||||||||||||||||
ASAE | |||||||||||||||||
200 | BIAS() | ||||||||||||||||
BIAS() | |||||||||||||||||
MSE() | |||||||||||||||||
MSE() | |||||||||||||||||
MRE() | |||||||||||||||||
MRE() | |||||||||||||||||
ASAE | |||||||||||||||||
300 | BIAS() | ||||||||||||||||
BIAS() | |||||||||||||||||
MSE() | |||||||||||||||||
MSE() | |||||||||||||||||
MRE() | |||||||||||||||||
MRE() | |||||||||||||||||
ASAE | |||||||||||||||||
450 | BIAS() | ||||||||||||||||
BIAS() | |||||||||||||||||
MSE() | |||||||||||||||||
MSE() | |||||||||||||||||
MRE() | |||||||||||||||||
MRE() | |||||||||||||||||
ASAE | |||||||||||||||||
n | Est. | MLE | ADE | CVME | MPSE | OLSE | PCE | RTADE | WLSE | LTADE | MSADE | MSALDE | ADSOE | KE | MSSD | MSSLD | MSLND |
20 | BIAS() | ||||||||||||||||
BIAS() | |||||||||||||||||
MSE() | |||||||||||||||||
MSE() | |||||||||||||||||
MRE() | |||||||||||||||||
MRE() | |||||||||||||||||
ASAE | |||||||||||||||||
70 | BIAS() | ||||||||||||||||
BIAS() | |||||||||||||||||
MSE() | |||||||||||||||||
MSE() | |||||||||||||||||
MRE() | |||||||||||||||||
MRE() | |||||||||||||||||
ASAE | |||||||||||||||||
150 | BIAS() | ||||||||||||||||
BIAS() | |||||||||||||||||
MSE() | |||||||||||||||||
MSE() | |||||||||||||||||
MRE() | |||||||||||||||||
MRE() | |||||||||||||||||
ASAE | |||||||||||||||||
200 | BIAS() | ||||||||||||||||
BIAS() | |||||||||||||||||
MSE() | |||||||||||||||||
MSE() | |||||||||||||||||
MRE() | |||||||||||||||||
MRE() | |||||||||||||||||
ASAE | |||||||||||||||||
300 | BIAS() | ||||||||||||||||
BIAS() | |||||||||||||||||
MSE() | |||||||||||||||||
MSE() | |||||||||||||||||
MRE() | |||||||||||||||||
MRE() | |||||||||||||||||
ASAE | |||||||||||||||||
450 | BIAS() | ||||||||||||||||
BIAS() | |||||||||||||||||
MSE() | |||||||||||||||||
MSE() | |||||||||||||||||
MRE() | |||||||||||||||||
MRE() | |||||||||||||||||
ASAE | |||||||||||||||||
n | Est. | MLE | ADE | CVME | MPSE | OLSE | PCE | RTADE | WLSE | LTADE | MSADE | MSALDE | ADSOE | KE | MSSD | MSSLD | MSLND |
20 | BIAS() | ||||||||||||||||
BIAS() | |||||||||||||||||
MSE() | |||||||||||||||||
MSE() | |||||||||||||||||
MRE() | |||||||||||||||||
MRE() | |||||||||||||||||
ASAE | |||||||||||||||||
70 | BIAS() | ||||||||||||||||
BIAS() | |||||||||||||||||
MSE() | |||||||||||||||||
MSE() | |||||||||||||||||
MRE() | |||||||||||||||||
MRE() | |||||||||||||||||
ASAE | |||||||||||||||||
150 | BIAS() | ||||||||||||||||
BIAS() | |||||||||||||||||
MSE() | |||||||||||||||||
MSE() | |||||||||||||||||
MRE() | |||||||||||||||||
MRE() | |||||||||||||||||
ASAE | |||||||||||||||||
200 | BIAS() | ||||||||||||||||
BIAS() | |||||||||||||||||
MSE() | |||||||||||||||||
MSE() | |||||||||||||||||
MRE() | |||||||||||||||||
MRE() | |||||||||||||||||
ASAE | |||||||||||||||||
300 | BIAS() | ||||||||||||||||
BIAS() | |||||||||||||||||
MSE() | |||||||||||||||||
MSE() | |||||||||||||||||
MRE() | |||||||||||||||||
MRE() | |||||||||||||||||
ASAE | |||||||||||||||||
450 | BIAS() | ||||||||||||||||
BIAS() | |||||||||||||||||
MSE() | |||||||||||||||||
MSE() | |||||||||||||||||
MRE() | |||||||||||||||||
MRE() | |||||||||||||||||
ASAE | |||||||||||||||||
Parameter | MLE | ADE | CVME | MPSE | OLSE | PCE | RTADE | WLSE | LTADE | MSADE | MSALDE | ADSOE | KE | MSSD | MSSLD | MSLND | |
20 | 7.0 | 6.0 | 13.0 | 1.0 | 11.0 | 2.0 | 9.0 | 4.5 | 12.0 | 8.0 | 10.0 | 15.0 | 3.0 | 14.0 | 4.5 | 16.0 | |
70 | 1.0 | 4.0 | 11.0 | 2.0 | 10.0 | 3.0 | 6.0 | 5.0 | 13.0 | 12.0 | 8.5 | 16.0 | 7.0 | 14.0 | 8.5 | 15.0 | |
150 | 1.0 | 3.0 | 9.0 | 2.0 | 11.0 | 4.0 | 6.0 | 5.0 | 13.0 | 12.0 | 10.0 | 16.0 | 8.0 | 15.0 | 7.0 | 14.0 | |
200 | 1.0 | 4.0 | 11.0 | 2.0 | 9.0 | 3.0 | 6.0 | 5.0 | 13.0 | 12.0 | 10.0 | 16.0 | 8.0 | 14.0 | 7.0 | 15.0 | |
300 | 1.0 | 4.0 | 8.0 | 3.0 | 11.0 | 2.0 | 5.0 | 6.0 | 12.0 | 13.0 | 9.0 | 16.0 | 10.0 | 15.0 | 7.0 | 14.0 | |
450 | 2.0 | 3.0 | 11.0 | 1.0 | 9.0 | 4.0 | 6.0 | 5.0 | 12.0 | 13.0 | 10.0 | 16.0 | 7.0 | 15.0 | 8.0 | 14.0 | |
20 | 2.0 | 3.0 | 9.0 | 1.0 | 7.0 | 15.0 | 5.0 | 4.0 | 10.0 | 11.0 | 8.0 | 16.0 | 12.0 | 14.0 | 6.0 | 13.0 | |
70 | 4.0 | 2.0 | 8.5 | 5.0 | 7.0 | 15.0 | 3.0 | 1.0 | 8.5 | 12.0 | 10.0 | 16.0 | 11.0 | 13.0 | 6.0 | 14.0 | |
150 | 1.0 | 3.0 | 7.0 | 2.0 | 8.0 | 14.5 | 4.0 | 5.0 | 10.0 | 12.0 | 9.0 | 16.0 | 11.0 | 13.0 | 6.0 | 14.5 | |
200 | 3.0 | 1.0 | 7.0 | 4.0 | 6.0 | 15.0 | 5.0 | 2.0 | 10.0 | 12.0 | 9.0 | 16.0 | 11.0 | 13.0 | 8.0 | 14.0 | |
300 | 1.0 | 3.0 | 7.0 | 2.0 | 6.0 | 16.0 | 4.0 | 5.0 | 9.0 | 12.0 | 10.0 | 15.0 | 11.0 | 14.0 | 8.0 | 13.0 | |
450 | 3.0 | 2.0 | 6.0 | 1.0 | 7.0 | 16.0 | 5.0 | 4.0 | 11.0 | 12.0 | 10.0 | 15.0 | 8.0 | 13.0 | 9.0 | 14.0 | |
20 | 3.0 | 1.5 | 12.0 | 5.0 | 8.5 | 4.0 | 8.5 | 6.0 | 10.0 | 11.0 | 13.0 | 14.0 | 1.5 | 15.0 | 7.0 | 16.0 | |
70 | 1.0 | 4.0 | 10.0 | 5.5 | 8.0 | 5.5 | 7.0 | 2.0 | 11.0 | 13.0 | 12.0 | 14.0 | 3.0 | 15.0 | 9.0 | 16.0 | |
150 | 1.0 | 5.0 | 10.0 | 2.0 | 8.0 | 4.0 | 7.0 | 6.0 | 9.0 | 14.0 | 12.0 | 13.0 | 3.0 | 15.0 | 11.0 | 16.0 | |
200 | 1.0 | 5.0 | 10.0 | 3.0 | 8.0 | 6.0 | 9.0 | 4.0 | 7.0 | 14.0 | 11.0 | 13.0 | 2.0 | 15.0 | 12.0 | 16.0 | |
300 | 3.0 | 5.0 | 9.5 | 1.0 | 8.0 | 6.0 | 7.0 | 2.0 | 9.5 | 14.0 | 13.0 | 12.0 | 4.0 | 15.0 | 11.0 | 16.0 | |
450 | 2.0 | 6.0 | 11.0 | 1.0 | 7.5 | 3.0 | 9.0 | 4.0 | 7.5 | 14.0 | 13.0 | 12.0 | 5.0 | 16.0 | 10.0 | 15.0 | |
20 | 7.0 | 4.0 | 12.0 | 1.0 | 11.0 | 9.5 | 6.0 | 5.0 | 13.0 | 9.5 | 8.0 | 15.0 | 2.0 | 14.0 | 3.0 | 16.0 | |
70 | 2.0 | 7.0 | 10.0 | 1.0 | 8.5 | 11.0 | 5.5 | 4.0 | 13.0 | 12.0 | 8.5 | 16.0 | 3.0 | 14.0 | 5.5 | 15.0 | |
150 | 1.0 | 5.0 | 10.0 | 2.0 | 8.5 | 11.0 | 7.0 | 3.0 | 13.0 | 12.0 | 8.5 | 16.0 | 4.0 | 15.0 | 6.0 | 14.0 | |
200 | 1.0 | 3.0 | 10.0 | 2.0 | 8.0 | 12.0 | 5.0 | 7.0 | 11.0 | 13.0 | 9.0 | 16.0 | 4.0 | 14.0 | 6.0 | 15.0 | |
300 | 1.0 | 5.0 | 10.0 | 2.0 | 8.0 | 11.0 | 3.0 | 4.0 | 12.0 | 13.0 | 9.0 | 16.0 | 6.0 | 15.0 | 7.0 | 14.0 | |
450 | 1.0 | 5.0 | 10.0 | 2.0 | 8.0 | 12.0 | 4.0 | 3.0 | 11.0 | 13.0 | 9.0 | 16.0 | 6.0 | 15.0 | 7.0 | 14.0 | |
20 | 4.5 | 3.0 | 9.0 | 2.0 | 12.0 | 10.0 | 8.0 | 4.5 | 13.0 | 7.0 | 11.0 | 14.0 | 1.0 | 15.0 | 6.0 | 16.0 | |
70 | 3.0 | 4.0 | 11.0 | 2.0 | 10.0 | 15.0 | 5.0 | 6.5 | 12.0 | 8.0 | 9.0 | 16.0 | 1.0 | 14.0 | 6.5 | 13.0 | |
150 | 3.0 | 5.0 | 10.0 | 1.0 | 9.0 | 15.0 | 6.0 | 4.0 | 12.0 | 11.0 | 8.0 | 16.0 | 2.0 | 13.0 | 7.0 | 14.0 | |
200 | 3.0 | 5.0 | 11.0 | 2.0 | 8.0 | 15.0 | 6.0 | 4.0 | 12.0 | 10.0 | 9.0 | 16.0 | 1.0 | 14.0 | 7.0 | 13.0 | |
300 | 2.5 | 4.0 | 10.0 | 2.5 | 8.0 | 15.0 | 6.0 | 5.0 | 12.0 | 11.0 | 9.0 | 16.0 | 1.0 | 13.0 | 7.0 | 14.0 | |
450 | 2.0 | 5.0 | 10.0 | 4.0 | 9.0 | 15.0 | 6.0 | 3.0 | 12.0 | 11.0 | 8.0 | 16.0 | 1.0 | 14.0 | 7.0 | 13.0 | |
Ranks | 69.0 | 119.5 | 293.0 | 67.0 | 258.0 | 289.5 | 179.0 | 128.5 | 333.5 | 351.5 | 293.5 | 456.0 | 157.5 | 428.0 | 220.0 | 436.5 | |
Overall Rank | 2 | 3 | 10 | 1 | 8 | 9 | 6 | 4 | 12 | 13 | 11 | 16 | 5 | 14 | 7 | 15 |
To demonstrate the effectiveness of the proposed model, we have utilized two real-world datasets, one from agriculture and the other from medical sciences. The subsequent evaluation, application, and analysis of our model are detailed below. For the purpose of comparing our model, we selected several existing models that share similar characteristics and use HLD base function. The models chosen for this comparison include:
● The exponentiated generalized standard HLD (EGSHLD), developed by Cordeiro et al. [40].
● The exponentiated HLD, proposed by Seo and Kang [41].
● The Poisson generalized HLD (PGHLD), introduced by Muhammad and Liu [42].
● The Kumaraswamy HLD (KHLD), formulated by Usman et al. [43].
● The Type-Ⅱ half logistic Weibull distribution (HLWD), created by Hassan et al. [28].
● The PHLD, developed by Krishnarani [23].
These models were selected for their relevance and compatibility with the HLD base function framework, providing a robust basis for comparison with our proposed model.
First data:
This dataset discusses the total milk production in the first birth of 107 cows from the SINDI race [44]. These cows are property of the Carnauba farm, which belongs to the Agropecuaria Manoel Dantas Ltda (AMDA), located in Taperoa City, Paraiba (Brazil).
0.4365, 0.4260, 0.5140, 0.6907, 0.7471, 0.2605, 0.6196, 0.8781, 0.4990, 0.6058, 0.6891, 0.5770, 0.5394, 0.1479, 0.2356, 0.6012, 0.1525, 0.5483, 0.6927, 0.7261, 0.3323, 0.0671, 0.2361, 0.4800, 0.5707, 0.7131, 0.5853, 0.6768, 0.5350, 0.4151, 0.6789, 0.4576, 0.3259, 0.2303, 0.7687, 0.4371, 0.3383, 0.6114, 0.3480, 0.4564, 0.7804, 0.3406, 0.4823, 0.5912, 0.5744, 0.5481, 0.1131, 0.7290, 0.0168, 0.5529, 0.4530, 0.3891, 0.4752, 0.3134, 0.3175, 0.1167, 0.6750, 0.5113, 0.5447, 0.4143, 0.5627, 0.5150, 0.0776, 0.3945, 0.4553, 0.4470, 0.5285, 0.5232, 0.6465, 0.0650, 0.8492, 0.8147, 0.3627, 0.3906, 0.4438, 0.4612, 0.3188, 0.2160, 0.6707, 0.6220, 0.5629, 0.4675, 0.6844, 0.3413, 0.4332, 0.0854, 0.3821, 0.4694, 0.3635, 0.4111, 0.5349, 0.3751, 0.1546, 0.4517, 0.2681, 0.4049, 0.5553, 0.5878, 0.4741, 0.3598, 0.7629, 0.5941, 0.6174, 0.6860, 0.0609, 0.6488, 0.2747
We applied basic exploratory data analysis techniques to examine the dataset under study, with the results displayed in Figure 10. The left side shows a box plot, while the right side presents a total-time-on-test (TTT) plot. From the box plot, we observed that the data is approximately normally distributed. To understand the nature of the hazard function, we used the TTT plot as described by Aarset [45]. The TTT plot reveals a concave curve, indicating that the hazard function is increasing.
Second data:
The data presented by [46], characterized by right-skewness, represents the survival times of 121 breast cancer patients treated at a tertiary care center between 1929 and 1938.
"0.3, 0.3, 4.0, 5.0, 5.6, 6.2, 6.3, 6.6, 6.8, 7.4, 7.5, 8.4, 8.4, 10.3, 11.0, 11.8, 12.2, 12.3, 13.5, 14.4, 14.4, 14.8, 15.5, 15.7, 16.2, 16.3, 16.5, 16.8, 17.2, 17.3, 17.5, 17.9, 19.8, 20.4, 20.9, 21.0, 21.0, 21.1, 23.0, 23.4, 23.6, 24.0, 24.0, 27.9, 28.2, 29.1, 30.0, 31.0, 31.0, 32.0, 35.0, 35.0, 37.0, 37.0, 37.0, 38.0, 38.0, 38.0, 39.0, 39.0, 40.0, 40.0, 40.0, 41.0, 41.0, 41.0, 42.0, 43.0, 43.0, 43.0, 44.0, 45.0, 45.0, 46.0, 46.0, 47.0, 48.0, 49.0, 51.0, 51.0, 51.0, 52.0, 54.0, 55.0, 56.0, 57.0, 58.0, 59.0, 60.0, 60.0, 60.0, 61.0, 62.0, 65.0, 65.0, 67.0, 67.0, 68.0, 69.0, 78.0, 80.0, 83.0, 88.0, 89.0, 90.0, 93.0, 96.0,103.0,105.0,109.0,109.0,111.0,115.0,117.0,125.0,126.0,127.0,129.0,129.0,139.0,154.0"
Similarly, the results are presented in Figure 14 for the second dataset. A box plot is displayed on the left, and on the right, a TTT plot is shown. The box plot indicates that the data is right-skewed along with some outliers. The TTT plot reveals a concave curve, indicating that the hazard function is increasing.
The parameters of the proposed distribution are estimated using the ML method, implemented through the 'maxLik' package as described by Henningsen and Toomet [47]. This estimation is performed using R programming software [48], with further details available in Lambert [49]. The aforementioned candidate distributions and the SPHLD also fit the studied datasets. The MLEs and their corresponding standard errors (SEs) are calculated, with the results presented in Tables 2 and 4, respectively. We have also displayed the profile log-likelihood plots for both parameters of the proposed model in Figures 11 and 15, respectively, for both datasets. These plots indicate that the parameter estimation through the ML method is unique and consistent. PP plots of the models under comparison of the first and second datasets are presented in Figures 13 and 17.
Model Selection
To determine the best model among the candidates, we used several standard criteria: log-likelihood , Akaike's information criterion (AIC), Bayesian information criterion (BIC), corrected AIC (CAIC), Hannan-Quinn information criterion (HQIC) and goodness-of-fit test statistics, including the Kolmogorov-Smirnov (KS) test, AD test, and CVM test. The corresponding p-values for these tests are denoted as , , and , respectively. The best model is identified by the smallest values of , AIC, BIC, CAIC, HQIC, KS, AD, and CVM, and the largest p-values, as recommended by Burnham and Anderson [50].
The results in Tables 3 and 5 show that the proposed SPHLD outperforms the other candidate models. This conclusion is further supported by the graphical representations of the fitted PDF and CDF shown in Figures 12 and 16, where the SPHLD provides the best fit for the data.
Model Validation
We calculated the KS distances between the fitted distribution function and the empirical distribution function for both datasets to evaluate the model's validity. The KS distances are 0.0635 and 0.0460, with corresponding p-values of 0.7812 and 0.9603, respectively. These calculations used the parameters estimated by the ML method. The results indicate that the SPHLD fits the data very well (see other metrics AD and CVM also in Tables 3 and 5).
This work provides a significant contribution to the creation of a flexible trigonometric extension of the PHLD. In particular, we use properties from the sine-generated family of distributions to create a novel two-parameter lifespan model, called the SPHLD. The primary reason behind this is that asymmetric datasets may make the new distribution more useful for simulating lifespan phenomena. The distribution takes on a number of asymmetric form configurations, as seen by the density function graphs of the SPHLD. In addition, the hazard rate graphs of the SPHLD showed both monotonic rises and declines. Statistical properties of the SPHLD that are computed include the quantile function, moments, central moments, incomplete moments, and stress-strength reliability. The SPHLD parameters are estimated using several different classical estimating methods. To assess the consistency of the various estimates and identify the most accurate estimating strategy, a simulation study was conducted based on a few accuracy metrics. The SPHLD outperforms several other distributions, according to analyses of actual data. Simulation analysis consistently demonstrated that the maximum product spacing exhibited the highest accuracy among the selected estimation techniques, followed by the maximum likelihood method, making it the preferred method for parameter estimation. The current study's primary limitation is its only focus on traditional estimating techniques for model parameters, which depend on complete sample data. Future studies can examine both Bayesian and traditional parameter estimation methods, with an emphasis on how they could be used in ranked set sampling.
The authors declare they have not used Artificial Intelligence (AI) tools in the creation of this article.
This research is supported by researchers Supporting Project number (RSPD2025R548), King Saud University, Riyadh, Saudi Arabia.
The authors declare there are no conflicts of interest.
[1] |
A. Marshall, I. Olkin, A new method for adding a parameter to a family of distributions with applications to the exponential and Weibull families, Biometrika, 84 (1997), 641–652. https://doi.org/10.1093/biomet/84.3.641 doi: 10.1093/biomet/84.3.641
![]() |
[2] |
N. Eugene, C. Lee, F. Famoye, Beta-normal distribution and its applications, Commun. Stat.-Theory Methods, 31 (2002), 497–512. https://doi.org/10.1081/STA-120003130 doi: 10.1081/STA-120003130
![]() |
[3] |
A. Alzaatreh, F. Famoye, C. Lee, A new method for generating families of continuous distributions, Metron, 71 (2013), 63–79. https://doi.org/10.1007/s40300-013-0007-y doi: 10.1007/s40300-013-0007-y
![]() |
[4] |
A. Al-Shomrani, O. Arif, A. Shawky, S. Hanif, M. Q. Shahbaz, Topp-Leone family of distributions: Some properties and application, Pak. J. Stat. Oper. Res., 12 (2016), 443–451. https://doi.org/10.18187/pjsor.v12i3.1458 doi: 10.18187/pjsor.v12i3.1458
![]() |
[5] |
A. S. Hassan, E. A. El-Sherpieny, S. A. El-Taweel, New Topp Leone-G family with mathematical properties and applications, J. Phys.: Conf. Ser., 12 (2021). https://doi:10.1088/1742-6596/1860/1/012011. doi: 10.1088/1742-6596/1860/1/012011
![]() |
[6] |
M. A. Badr, I. Elbatal, F. Jamal, C. Chesneau, M. Elgarhy, The transmuted odd Fréchet-G family of distributions: Theory and applications, Mathematics, 8 (2020), 958. https://doi.org/10.3390/math8060958 doi: 10.3390/math8060958
![]() |
[7] |
M. Aslam, Z. Asghar, Z. Hussain, S. F. Shah, A modified T-X family of distributions: Classical and Bayesian analysis, J. Taibah Univ. Sci., 14 (2020), 254–264. https://doi.org/10.1080/16583655.2020.1732642 doi: 10.1080/16583655.2020.1732642
![]() |
[8] |
A. S. Hassan, M. A. H. Sabry, A. M. Elsehtery, A new probability distribution family arising from truncated power Lomax distribution with application to Weibull model, Pak. J. Stat. Oper. Res., 16 (2020), 661–674. https://doi.org/10.1007/s12015-020-09979-4 doi: 10.1007/s12015-020-09979-4
![]() |
[9] |
F. S. Gomes-Silva, A. Percontini, E. de Brito, M. W. Ramos, R. Venâncio, G. M. Cordeiro, The odd Lindley-G family of distributions, Austrian J. Stat., 46 (2017), 65–87. https://doi.org/10.17713/ajs.v46i1.222 doi: 10.17713/ajs.v46i1.222
![]() |
[10] |
A. S. Hassan, S. G. Nassar, Power Lindley-G family of distributions, Ann. Data Sci., 6 (2019), 189–210. https://doi.org/10.1007/s40745-018-0159-y doi: 10.1007/s40745-018-0159-y
![]() |
[11] |
I. Elbatal, N. Alotaibi, E. M. Almetwally, S. A. Alyami, M. Elgarhy, On odd perks-G class of distributions: properties, regression model, discretization, Bayesian and non-Bayesian estimation, and applications, Symmetry, 14 (2022), 883. https://doi.org/10.3390/sym14050883 doi: 10.3390/sym14050883
![]() |
[12] |
A. Z. Afify, G. M. Cordeiro, N. A. Ibrahim, J. M. Elgarhy, M. A. Nasir, The Marshall-Olkin odd Burr Ⅲ-G family: Theory, estimation, and engineering applications, IEEE Access, 9 (2021), 4376–4387. https://doi.org/10.1109/ACCESS.2020.3044156 doi: 10.1109/ACCESS.2020.3044156
![]() |
[13] |
C. Chesneau, T. E. Achi, Modified odd Weibull family of distributions: Properties and applications, J. Indian Soc. Probab. Stat., 21 (2020), 259–286. https://doi.org/10.1007/s41096-020-00075-x doi: 10.1007/s41096-020-00075-x
![]() |
[14] | J. T. Eghwerido, D. J. Ikwuoche, O. D. AdubisiM, Inverse odd Weibull generated family of distribution, Pak. J. Stat. Oper. Res., 16 (2020), 617—633. |
[15] | D. Kumar, U. Singh, S. K. Singh, A new distribution using sine function- its application to bladder cancer patients data, J. Stat. Appl. Prob., 4 (2015), 417–427. |
[16] | Z. Mahmood, C. Chesneau, M.H. Tahir, A new sine-G family of distributions: properties and applications, Bull. Comput. Appl. Math., 7 (2019), 53–81. |
[17] | N. Balakrishnan, Order statistics from the half logistic distribution. J. Stat. Comput. Simul., 20 (1985), 287–309. |
[18] | R. S. Rao, P. L. Mamidi, R. R. Kantam, Modified maximum likelihood estimation: Inverse half logistic distribution, J. Math., 5 (2016), 11–19. |
[19] | H. Torabi, F. L. Bagheri, Estimation of parameters for an extended generalized half logistic distribution based on complete and censored data, J. Iranian Stat. Soc., 9 (2010), 171–195. |
[20] |
G. M. Cordeiro, M. Alizadeh, E. M. M. Ortega, The exponentiated half logistic family of distributions: properties and applications, J. Probab. Stat., 2014 (2014), 864396. https://doi.org/10.1155/2014/864396 doi: 10.1155/2014/864396
![]() |
[21] |
J. Oliveira, J. Santos, C. Xavier, D. Trindade, G. M. Cordeiro, The McDonald half-logistic distribution: theory and practice, Commun. Stat. Theory Methods, 45 (2016), 2005–2022. https://doi.org/10.1080/03610926.2013.873131 doi: 10.1080/03610926.2013.873131
![]() |
[22] |
A. H. Hassan, M. Elgarhy, M. Shakil, Type Ⅱ half logistic family of distributions with applications, Pak. J. Stat. Oper. Res., 13 (2017), 245–264. https://doi.org/10.18187/pjsor.v13i2.1560 doi: 10.18187/pjsor.v13i2.1560
![]() |
[23] | S. D. Krishnarani, On a power transformation of half-logistic distribution, J. Probab. Stat., 20 (2016), 1–10. |
[24] |
R. M. Usman, A. M. Haq, J. Talib, Kumaraswamy half-logistic distribution: properties and applications, J. Stat. Appl. Probab., 6 (2017), 597–609. https://doi.org/10.18576/jsap/060315 doi: 10.18576/jsap/060315
![]() |
[25] |
D. Yegen, G. Ozel, Marshall-Olkin half logistic distribution with theory and applications, Alphanum. J., 6 (2018), 408–416. https://doi.org/10.17093/alphanumeric.409992 doi: 10.17093/alphanumeric.409992
![]() |
[26] |
M. Elgarhy, A. S. Hassan, S. Fayomi, Maximum likelihood and Bayesian estimation for two-parameter type Ⅰ half logistic Lindley distribution, J. Comput. Theor. Nanosci., 15 (2018), 1–9. https://doi.org/10.1166/jctn.2018.7600 doi: 10.1166/jctn.2018.7600
![]() |
[27] | A. F. Samuel, O. A. Kehinde, A study on transmuted half logistic distribution: Properties and application, Int. J. Stat. Distribut. Appl., 5 (2019), 54–59. |
[28] |
A. S. Hassan, M. Elgarhy, M. A. Haq, S. Alrajhi, On type Ⅱ half logistic Weibull distribution with applications, Math. Theory Model., 19(2019), 49–63. https://doi.org/10.21608/esju.2019.268726 doi: 10.21608/esju.2019.268726
![]() |
[29] |
M. Anwar, A. Bibi, The half-logistic generalized Weibull distribution, J. Probab. Stat., (2018), 8767826. https://doi.org/10.1155/2018/8767826 doi: 10.1155/2018/8767826
![]() |
[30] |
A. Algarni, A. M. Almarashi, I. Elbatal, A. S. Hassan, E. M. Almetwally, c Daghistani, et al., Type Ⅰ half logistic Burr X-G family: Properties, Bayesian, and non-Bayesian estimation under censored samples and applications to COVID-19 data, Math. Probl. Eng., 2021, (2021), 5461130. https://doi.org/10.1155/2021/5461130 doi: 10.1155/2021/5461130
![]() |
[31] |
G. S. Mohammad, A new two-parameter modified half-logistic distribution: Properties and Applications, Stat. Optim. Inf. Comput., 10 (2022), 589–605. https://doi.org/10.19139/soic-2310-5070-1210 doi: 10.19139/soic-2310-5070-1210
![]() |
[32] |
A. S. Hassan, A. Fayomi, A. Algarni, E. M. Almetwally, Bayesian and non-Bayesian inference for unit-exponentiated half-logistic distribution with data analysis, Appl. Sci., 12 (2022), 11253. https://doi.org/10.3390/app122111253 doi: 10.3390/app122111253
![]() |
[33] |
H. Majid, An extended type Ⅰ half-logistic family of distributions: Properties, applications and different method of estimations, Math. Slovaca, 72 (2022), 745–764. https://doi.org/10.1515/ms-2022-0051 doi: 10.1515/ms-2022-0051
![]() |
[34] |
R. M. I. Arshad, M. H. Tahir, C. Chesneau, S. Khan, F. Jamal, The gamma power half-logistic distribution: theory and applications, Sao Paulo J. Math. Sci., 17 (2023), 1142–1169. https://doi.org/10.1007/s40863-022-00331-x doi: 10.1007/s40863-022-00331-x
![]() |
[35] |
S. M. Alghamdi, M. Shrahili, A. S. Hassan, A. M. Gemeay, I. Elbatal, M. Elgarhy, Statistical inference of the half logistic modified Kies exponential model with modeling to engineering data, Symmetry, 15 (2023), 586. https://doi.org/10.3390/sym15030586 doi: 10.3390/sym15030586
![]() |
[36] | O. D. Adubisi, C. E. Adubisi, Novel distribution for modeling uncensored and censored survival time data and regression model, Reliab. Theory Appl., 3 (2023), 808–824. |
[37] | R. C. H. Cheng, N. A. K. Amin, Maximum product-of-spacings estimation with applications to the log-normal distribution, University of Wales IST, Math Report, (1979), 79–103. |
[38] | R. C. H. Cheng, N. A. K. Amin, Estimating parameters in continuous univariate distributions with a shifted origin, J. R. Stat. Soc.: Ser. B (Methodol.) 45 (1983), 394–403. https://doi.org/10.1111/j.2517-6161.1983.tb01268.x |
[39] | B. Ranneby, The maximum spacing method: An estimation method related to the maximum likelihood method, Scand. J. Stat., 11 (1984), 93–112. |
[40] |
G. M. Cordeiro, T. A. de Andrade, M. Bourguignon, F. G. Silva, The exponentiated generalized standardized half-logistic distribution, Int. J. Stat. Probab., 6 (2017), 24–42. https://doi.org/10.5539/ijsp.v6n3p24 doi: 10.5539/ijsp.v6n3p24
![]() |
[41] | J. I. Seo, S. B. Kang, Notes on the exponentiated half logistic distribution, Appl. Math. Model., 39 (2015), 6491–6500. |
[42] |
M. Muhammad, L. Liu, A new extension of the generalized half logistic distribution with applications to real data, Entropy, 21 (2019), 339. https://doi.org/10.3390/e21040339 doi: 10.3390/e21040339
![]() |
[43] |
R. M. Usman, M. Haq, J. Talib, Kumaraswamy half-logistic distribution: properties and applications, J. Stat. Appl. Probab., 6 (2017), 597–609. https://doi.org/10.18576/jsap/060315 doi: 10.18576/jsap/060315
![]() |
[44] |
G. M. Cordeiro, R. B. dos Santos, The beta power distribution. Brazil J. Probab. Stat., 26 (2012), 88–112. https://doi.org/10.1214/10-BJPS124 doi: 10.1214/10-BJPS124
![]() |
[45] |
M. V. Aarset, How to identify a bathtub hazard rate, IEEE Trans. Reliab., 36 (1987), 106–108. https://doi.org/10.1109/TR.1987.5222310 doi: 10.1109/TR.1987.5222310
![]() |
[46] |
E. T. Lee, Statistical methods for survival data analysis, IEEE Trans. Reliab., 35 (1986), 123–123. https://doi.org/10.1109/TR.1986.4335370 doi: 10.1109/TR.1986.4335370
![]() |
[47] |
A. Henningsen, O. Toomet, maxLik: A package for maximum likelihood estimation in R, Comput. Stat., 26 (2011), 443–458. https://doi.org/10.1007/s00180-010-0217-1 doi: 10.1007/s00180-010-0217-1
![]() |
[48] | R Core Team, R: A language and environment for statistical computing, Foundation for Statistical Computing, Vienna, Austria, 2013. |
[49] | B. Lambert, A student's guide to Bayesian statistics, in A Student's Guide to Bayesian Statistics, (2018), 1–520. |
[50] | K. P. Burnham, D. R. Anderson, Model selection and multimodel inference: a practical information-theoretic approach, Springer, 2002. |
Parameters | Measures | ||||||||
0.15 | 0.5 | 0.724887 | 4.68121 | 9.54363 | 21.3535 | 33.6724 | 64.8572 | 105.342 | 212.237 |
1.0 | 0.851403 | 2.16361 | 3.08928 | 4.62098 | 5.80279 | 8.0534 | 10.2636 | 14.5684 | |
3.0 | 0.947789 | 1.29338 | 1.45642 | 1.66563 | 1.79699 | 2.00444 | 2.1732 | 2.44233 | |
5.0 | 0.968338 | 1.16691 | 1.25306 | 1.35815 | 1.42144 | 1.51773 | 1.59316 | 1.70876 | |
7.0 | 0.977281 | 1.11656 | 1.17484 | 1.24441 | 1.28556 | 1.34718 | 1.39467 | 1.46623 | |
10 | 0.984042 | 1.08023 | 1.1194 | 1.1654 | 1.19224 | 1.23196 | 1.26221 | 1.3072 | |
0.6 | 0.5 | 0.0453054 | 0.292575 | 0.596477 | 1.33459 | 2.10452 | 4.05357 | 6.58386 | 13.2648 |
1.0 | 0.212851 | 0.540902 | 0.772319 | 1.15525 | 1.4507 | 2.01335 | 2.5659 | 3.64209 | |
3.0 | 0.59707 | 0.814779 | 0.917485 | 1.04928 | 1.13203 | 1.26272 | 1.36903 | 1.53857 | |
5.0 | 0.733863 | 0.884349 | 0.949641 | 1.02928 | 1.07725 | 1.15023 | 1.20739 | 1.295 | |
7.0 | 0.801698 | 0.915955 | 0.963765 | 1.02083 | 1.05459 | 1.10514 | 1.1441 | 1.2028 | |
10 | 0.856658 | 0.940398 | 0.974495 | 1.01454 | 1.03791 | 1.07249 | 1.09881 | 1.13798 | |
0.9 | 0.5 | 0.0201357 | 0.130033 | 0.265101 | 0.593152 | 0.935344 | 1.80159 | 2.92616 | 5.89547 |
1.0 | 0.1419 | 0.360602 | 0.514879 | 0.770164 | 0.967132 | 1.34223 | 1.7106 | 2.42806 | |
3.0 | 0.521588 | 0.711775 | 0.801497 | 0.916631 | 0.988922 | 1.10309 | 1.19596 | 1.34406 | |
5.0 | 0.676701 | 0.815465 | 0.875671 | 0.94911 | 0.993338 | 1.06063 | 1.11335 | 1.19413 | |
7.0 | 0.75658 | 0.864407 | 0.909526 | 0.96338 | 0.995237 | 1.04294 | 1.07971 | 1.13511 | |
10 | 0.822618 | 0.903031 | 0.935773 | 0.974223 | 0.996664 | 1.02987 | 1.05515 | 1.09276 | |
1.5 | 0.5 | 0.00724887 | 0.0468121 | 0.0954363 | 0.213535 | 0.336724 | 0.648572 | 1.05342 | 2.12237 |
1.0 | 0.0851403 | 0.216361 | 0.308928 | 0.462098 | 0.580279 | 0.80534 | 1.02636 | 1.45684 | |
3.0 | 0.439925 | 0.600334 | 0.676009 | 0.773116 | 0.834089 | 0.930379 | 1.00871 | 1.13363 | |
5.0 | 0.61098 | 0.736268 | 0.790626 | 0.856933 | 0.896866 | 0.957626 | 1.00522 | 1.07816 | |
7.0 | 0.703335 | 0.803573 | 0.845517 | 0.895581 | 0.925196 | 0.969546 | 1.00372 | 1.05522 | |
10 | 0.781652 | 0.85806 | 0.889172 | 0.925707 | 0.94703 | 0.978584 | 1.00261 | 1.03834 | |
2.5 | 0.5 | 0.00260959 | 0.0168523 | 0.0343571 | 0.0768725 | 0.121221 | 0.233486 | 0.37923 | 0.764053 |
1.0 | 0.0510842 | 0.129817 | 0.185357 | 0.277259 | 0.348167 | 0.483204 | 0.615817 | 0.874101 | |
3.0 | 0.371047 | 0.506341 | 0.570168 | 0.652071 | 0.703498 | 0.784712 | 0.85078 | 0.956138 | |
5.0 | 0.551642 | 0.664762 | 0.713841 | 0.773708 | 0.809762 | 0.864622 | 0.907591 | 0.973447 | |
7.0 | 0.653837 | 0.747021 | 0.786013 | 0.832554 | 0.860085 | 0.901313 | 0.933086 | 0.980961 | |
10 | 0.742726 | 0.815329 | 0.844891 | 0.879607 | 0.899868 | 0.92985 | 0.952676 | 0.986634 | |
4.5 | 0.5 | 0.00080543 | 0.00520134 | 0.010604 | 0.0237261 | 0.0374137 | 0.0720635 | 0.117046 | 0.235819 |
1.0 | 0.0283801 | 0.0721203 | 0.102976 | 0.154033 | 0.193426 | 0.268447 | 0.34212 | 0.485612 | |
3.0 | 0.305027 | 0.416248 | 0.468718 | 0.536049 | 0.578325 | 0.645088 | 0.699401 | 0.786013 | |
5.0 | 0.490459 | 0.591033 | 0.634669 | 0.687896 | 0.719951 | 0.768726 | 0.80693 | 0.865482 | |
7.0 | 0.601176 | 0.686855 | 0.722707 | 0.765499 | 0.790813 | 0.828721 | 0.857935 | 0.901954 | |
10 | 0.700328 | 0.768787 | 0.796661 | 0.829395 | 0.848499 | 0.87677 | 0.898293 | 0.930313 |
Model | Parameter | SE | Parameter | SE | Parameter | SE |
SPHLD() | 3.7870 | 0.4370 | 2.2162 | 0.1836 | – | – |
EGSHLD() | 7.0611 | 0.5863 | 3.3169 | 0.4593 | – | – |
EHLD() | 4.7771 | 0.3748 | 2.7077 | 0.3918 | – | – |
PGHLD() | 0.5901 | 0.0916 | 2.6059 | 0.2187 | 133.3208 | 3.0465 |
KHLD() | 2.5987 | 0.2161 | 141.1152 | 2.1935 | 0.5731 | 0.0893 |
HLWD() | 9.2668 | 0.0013 | 7.7567 | 0.0075 | 0.2865 | 0.0236 |
PHLD() | 6.3117 | 0.7676 | 2.2754 | 0.1877 | – | – |
Models | -2logL | AIC | BIC | CAIC | HQIC | KS | p(KS) | CVM | p(CVM) | AD | p(AD) |
SPHLD | -50.0009 | -46.0009 | -40.6552 | -45.8877 | -43.8338 | 0.0635 | 0.7812 | 0.1027 | 0.5727 | 0.8752 | 0.4295 |
EGSHLD | -18.5522 | -14.5522 | -9.2065 | -14.439 | -12.3851 | 0.1367 | 0.0367 | 0.678 | 0.0142 | 3.9479 | 0.0093 |
EHLD | -20.661 | -16.661 | -11.3154 | -16.5478 | -14.494 | 0.1291 | 0.0566 | 0.6068 | 0.0213 | 3.6361 | 0.0132 |
PGHLD | -41.7841 | -35.7841 | -27.7656 | -35.5556 | -32.5335 | 0.0868 | 0.3958 | 0.2084 | 0.2519 | 1.5726 | 0.1601 |
KHLD | -42.0805 | -36.0805 | -28.062 | -35.8519 | -32.8299 | 0.0865 | 0.4001 | 0.2067 | 0.255 | 1.5561 | 0.1637 |
HLWD | -50.7479 | -44.7479 | -36.7294 | -44.5193 | -41.4973 | 0.083 | 0.4532 | 0.1787 | 0.3131 | 1.088 | 0.3141 |
PHLD | -48.4568 | -44.4568 | -39.1112 | -44.3436 | -42.2898 | 0.0647 | 0.7624 | 0.1082 | 0.5469 | 0.9613 | 0.378 |
Model | parameter | SE | parameter | SE | parameter | SE |
SPHLD() | 0.0134 | 0.0048 | 1.0754 | 0.0806 | – | – |
EGSHLD() | 0.0271 | 0.0029 | 1.4176 | 0.1774 | – | – |
EHLD() | 0.0330 | 0.0030 | 1.1827 | 0.1406 | – | – |
PGHLD() | 0.0029 | 6.0E-04 | 1.3095 | 0.0959 | 31.6416 | 3.0606 |
KHLD() | 1.7763 | 2.0E-04 | 0.1171 | 0.0106 | 0.2100 | 1.0E-04 |
HLWD() | 3.2E-05 | 0.0000 | 2.3012 | 0.0925 | 0.5459 | 0.0382 |
PHLD() | 0.0188 | 0.0068 | 1.1120 | 0.0833 | – | – |
Models | -2logL | AIC | BIC | CAIC | HQIC | KS | p(KS) | CVM | p(CVM) | AD | p(AD) |
SPHLD | 1158.5700 | 1162.5700 | 1168.1620 | 1162.4700 | 1164.8410 | 0.0460 | 0.9603 | 0.0697 | 0.7543 | 0.5285 | 0.7176 |
EGSHLD | 1161.6180 | 1165.6180 | 1171.2090 | 1165.7180 | 1167.8890 | 0.0848 | 0.3489 | 0.0784 | 0.7023 | 0.5126 | 0.7337 |
EHLD | 1158.7411 | 1162.7411 | 1168.4002 | 1162.5110 | 1164.9820 | 0.0569 | 0.8286 | 0.0519 | 0.8652 | 0.4096 | 0.8389 |
PGHLD | 1158.6990 | 1164.1990 | 1172.5860 | 1164.4010 | 1167.6050 | 0.0606 | 0.7658 | 0.0522 | 0.8638 | 0.3987 | 0.8498 |
KHLD | 1163.9130 | 1169.9130 | 1178.3000 | 1170.1140 | 1173.3190 | 0.1032 | 0.1521 | 0.1349 | 0.4395 | 0.7670 | 0.5051 |
HLWD | 1158.8090 | 1163.0090 | 1171.3960 | 1163.2110 | 1166.4150 | 0.0538 | 0.8752 | 0.0634 | 0.7934 | 0.4578 | 0.7898 |
PHLD | 1158.6310 | 1162.6310 | 1168.8022 | 1162.5310 | 1164.8702 | 0.0492 | 0.9311 | 0.0597 | 0.8170 | 0.4685 | 0.7788 |
n | Est. | MLE | ADE | CVME | MPSE | OLSE | PCE | RTADE | WLSE | LTADE | MSADE | MSALDE | ADSOE | KE | MSSD | MSSLD | MSLND |
20 | BIAS() | ||||||||||||||||
BIAS() | |||||||||||||||||
MSE() | |||||||||||||||||
MSE() | |||||||||||||||||
MRE() | |||||||||||||||||
MRE() | |||||||||||||||||
ASAE | |||||||||||||||||
70 | BIAS() | ||||||||||||||||
BIAS() | |||||||||||||||||
MSE() | |||||||||||||||||
MSE() | |||||||||||||||||
MRE() | |||||||||||||||||
MRE() | |||||||||||||||||
ASAE | |||||||||||||||||
150 | BIAS() | ||||||||||||||||
BIAS() | |||||||||||||||||
MSE() | |||||||||||||||||
MSE() | |||||||||||||||||
MRE() | |||||||||||||||||
MRE() | |||||||||||||||||
ASAE | |||||||||||||||||
200 | BIAS() | ||||||||||||||||
BIAS() | |||||||||||||||||
MSE() | |||||||||||||||||
MSE() | |||||||||||||||||
MRE() | |||||||||||||||||
MRE() | |||||||||||||||||
ASAE | |||||||||||||||||
300 | BIAS() | ||||||||||||||||
BIAS() | |||||||||||||||||
MSE() | |||||||||||||||||
MSE() | |||||||||||||||||
MRE() | |||||||||||||||||
MRE() | |||||||||||||||||
ASAE | |||||||||||||||||
450 | BIAS() | ||||||||||||||||
BIAS() | |||||||||||||||||
MSE() | |||||||||||||||||
MSE() | |||||||||||||||||
MRE() | |||||||||||||||||
MRE() | |||||||||||||||||
ASAE | |||||||||||||||||
n | Est. | MLE | ADE | CVME | MPSE | OLSE | PCE | RTADE | WLSE | LTADE | MSADE | MSALDE | ADSOE | KE | MSSD | MSSLD | MSLND |
20 | BIAS() | ||||||||||||||||
BIAS() | |||||||||||||||||
MSE() | |||||||||||||||||
MSE() | |||||||||||||||||
MRE() | |||||||||||||||||
MRE() | |||||||||||||||||
ASAE | |||||||||||||||||
70 | BIAS() | ||||||||||||||||
BIAS() | |||||||||||||||||
MSE() | |||||||||||||||||
MSE() | |||||||||||||||||
MRE() | |||||||||||||||||
MRE() | |||||||||||||||||
ASAE | |||||||||||||||||
150 | BIAS() | ||||||||||||||||
BIAS() | |||||||||||||||||
MSE() | |||||||||||||||||
MSE() | |||||||||||||||||
MRE() | |||||||||||||||||
MRE() | |||||||||||||||||
ASAE | |||||||||||||||||
200 | BIAS() | ||||||||||||||||
BIAS() | |||||||||||||||||
MSE() | |||||||||||||||||
MSE() | |||||||||||||||||
MRE() | |||||||||||||||||
MRE() | |||||||||||||||||
ASAE | |||||||||||||||||
300 | BIAS() | ||||||||||||||||
BIAS() | |||||||||||||||||
MSE() | |||||||||||||||||
MSE() | |||||||||||||||||
MRE() | |||||||||||||||||
MRE() | |||||||||||||||||
ASAE | |||||||||||||||||
450 | BIAS() | ||||||||||||||||
BIAS() | |||||||||||||||||
MSE() | |||||||||||||||||
MSE() | |||||||||||||||||
MRE() | |||||||||||||||||
MRE() | |||||||||||||||||
ASAE | |||||||||||||||||
n | Est. | MLE | ADE | CVME | MPSE | OLSE | PCE | RTADE | WLSE | LTADE | MSADE | MSALDE | ADSOE | KE | MSSD | MSSLD | MSLND |
20 | BIAS() | ||||||||||||||||
BIAS() | |||||||||||||||||
MSE() | |||||||||||||||||
MSE() | |||||||||||||||||
MRE() | |||||||||||||||||
MRE() | |||||||||||||||||
ASAE | |||||||||||||||||
70 | BIAS() | ||||||||||||||||
BIAS() | |||||||||||||||||
MSE() | |||||||||||||||||
MSE() | |||||||||||||||||
MRE() | |||||||||||||||||
MRE() | |||||||||||||||||
ASAE | |||||||||||||||||
150 | BIAS() | ||||||||||||||||
BIAS() | |||||||||||||||||
MSE() | |||||||||||||||||
MSE() | |||||||||||||||||
MRE() | |||||||||||||||||
MRE() | |||||||||||||||||
ASAE | |||||||||||||||||
200 | BIAS() | ||||||||||||||||
BIAS() | |||||||||||||||||
MSE() | |||||||||||||||||
MSE() | |||||||||||||||||
MRE() | |||||||||||||||||
MRE() | |||||||||||||||||
ASAE | |||||||||||||||||
300 | BIAS() | ||||||||||||||||
BIAS() | |||||||||||||||||
MSE() | |||||||||||||||||
MSE() | |||||||||||||||||
MRE() | |||||||||||||||||
MRE() | |||||||||||||||||
ASAE | |||||||||||||||||
450 | BIAS() | ||||||||||||||||
BIAS() | |||||||||||||||||
MSE() | |||||||||||||||||
MSE() | |||||||||||||||||
MRE() | |||||||||||||||||
MRE() | |||||||||||||||||
ASAE | |||||||||||||||||
n | Est. | MLE | ADE | CVME | MPSE | OLSE | PCE | RTADE | WLSE | LTADE | MSADE | MSALDE | ADSOE | KE | MSSD | MSSLD | MSLND |
20 | BIAS() | ||||||||||||||||
BIAS() | |||||||||||||||||
MSE() | |||||||||||||||||
MSE() | |||||||||||||||||
MRE() | |||||||||||||||||
MRE() | |||||||||||||||||
ASAE | |||||||||||||||||
70 | BIAS() | ||||||||||||||||
BIAS() | |||||||||||||||||
MSE() | |||||||||||||||||
MSE() | |||||||||||||||||
MRE() | |||||||||||||||||
MRE() | |||||||||||||||||
ASAE | |||||||||||||||||
150 | BIAS() | ||||||||||||||||
BIAS() | |||||||||||||||||
MSE() | |||||||||||||||||
MSE() | |||||||||||||||||
MRE() | |||||||||||||||||
MRE() | |||||||||||||||||
ASAE | |||||||||||||||||
200 | BIAS() | ||||||||||||||||
BIAS() | |||||||||||||||||
MSE() | |||||||||||||||||
MSE() | |||||||||||||||||
MRE() | |||||||||||||||||
MRE() | |||||||||||||||||
ASAE | |||||||||||||||||
300 | BIAS() | ||||||||||||||||
BIAS() | |||||||||||||||||
MSE() | |||||||||||||||||
MSE() | |||||||||||||||||
MRE() | |||||||||||||||||
MRE() | |||||||||||||||||
ASAE | |||||||||||||||||
450 | BIAS() | ||||||||||||||||
BIAS() | |||||||||||||||||
MSE() | |||||||||||||||||
MSE() | |||||||||||||||||
MRE() | |||||||||||||||||
MRE() | |||||||||||||||||
ASAE | |||||||||||||||||
n | Est. | MLE | ADE | CVME | MPSE | OLSE | PCE | RTADE | WLSE | LTADE | MSADE | MSALDE | ADSOE | KE | MSSD | MSSLD | MSLND |
20 | BIAS() | ||||||||||||||||
BIAS() | |||||||||||||||||
MSE() | |||||||||||||||||
MSE() | |||||||||||||||||
MRE() | |||||||||||||||||
MRE() | |||||||||||||||||
ASAE | |||||||||||||||||
70 | BIAS() | ||||||||||||||||
BIAS() | |||||||||||||||||
MSE() | |||||||||||||||||
MSE() | |||||||||||||||||
MRE() | |||||||||||||||||
MRE() | |||||||||||||||||
ASAE | |||||||||||||||||
150 | BIAS() | ||||||||||||||||
BIAS() | |||||||||||||||||
MSE() | |||||||||||||||||
MSE() | |||||||||||||||||
MRE() | |||||||||||||||||
MRE() | |||||||||||||||||
ASAE | |||||||||||||||||
200 | BIAS() | ||||||||||||||||
BIAS() | |||||||||||||||||
MSE() | |||||||||||||||||
MSE() | |||||||||||||||||
MRE() | |||||||||||||||||
MRE() | |||||||||||||||||
ASAE | |||||||||||||||||
300 | BIAS() | ||||||||||||||||
BIAS() | |||||||||||||||||
MSE() | |||||||||||||||||
MSE() | |||||||||||||||||
MRE() | |||||||||||||||||
MRE() | |||||||||||||||||
ASAE | |||||||||||||||||
450 | BIAS() | ||||||||||||||||
BIAS() | |||||||||||||||||
MSE() | |||||||||||||||||
MSE() | |||||||||||||||||
MRE() | |||||||||||||||||
MRE() | |||||||||||||||||
ASAE | |||||||||||||||||
Parameter | MLE | ADE | CVME | MPSE | OLSE | PCE | RTADE | WLSE | LTADE | MSADE | MSALDE | ADSOE | KE | MSSD | MSSLD | MSLND | |
20 | 7.0 | 6.0 | 13.0 | 1.0 | 11.0 | 2.0 | 9.0 | 4.5 | 12.0 | 8.0 | 10.0 | 15.0 | 3.0 | 14.0 | 4.5 | 16.0 | |
70 | 1.0 | 4.0 | 11.0 | 2.0 | 10.0 | 3.0 | 6.0 | 5.0 | 13.0 | 12.0 | 8.5 | 16.0 | 7.0 | 14.0 | 8.5 | 15.0 | |
150 | 1.0 | 3.0 | 9.0 | 2.0 | 11.0 | 4.0 | 6.0 | 5.0 | 13.0 | 12.0 | 10.0 | 16.0 | 8.0 | 15.0 | 7.0 | 14.0 | |
200 | 1.0 | 4.0 | 11.0 | 2.0 | 9.0 | 3.0 | 6.0 | 5.0 | 13.0 | 12.0 | 10.0 | 16.0 | 8.0 | 14.0 | 7.0 | 15.0 | |
300 | 1.0 | 4.0 | 8.0 | 3.0 | 11.0 | 2.0 | 5.0 | 6.0 | 12.0 | 13.0 | 9.0 | 16.0 | 10.0 | 15.0 | 7.0 | 14.0 | |
450 | 2.0 | 3.0 | 11.0 | 1.0 | 9.0 | 4.0 | 6.0 | 5.0 | 12.0 | 13.0 | 10.0 | 16.0 | 7.0 | 15.0 | 8.0 | 14.0 | |
20 | 2.0 | 3.0 | 9.0 | 1.0 | 7.0 | 15.0 | 5.0 | 4.0 | 10.0 | 11.0 | 8.0 | 16.0 | 12.0 | 14.0 | 6.0 | 13.0 | |
70 | 4.0 | 2.0 | 8.5 | 5.0 | 7.0 | 15.0 | 3.0 | 1.0 | 8.5 | 12.0 | 10.0 | 16.0 | 11.0 | 13.0 | 6.0 | 14.0 | |
150 | 1.0 | 3.0 | 7.0 | 2.0 | 8.0 | 14.5 | 4.0 | 5.0 | 10.0 | 12.0 | 9.0 | 16.0 | 11.0 | 13.0 | 6.0 | 14.5 | |
200 | 3.0 | 1.0 | 7.0 | 4.0 | 6.0 | 15.0 | 5.0 | 2.0 | 10.0 | 12.0 | 9.0 | 16.0 | 11.0 | 13.0 | 8.0 | 14.0 | |
300 | 1.0 | 3.0 | 7.0 | 2.0 | 6.0 | 16.0 | 4.0 | 5.0 | 9.0 | 12.0 | 10.0 | 15.0 | 11.0 | 14.0 | 8.0 | 13.0 | |
450 | 3.0 | 2.0 | 6.0 | 1.0 | 7.0 | 16.0 | 5.0 | 4.0 | 11.0 | 12.0 | 10.0 | 15.0 | 8.0 | 13.0 | 9.0 | 14.0 | |
20 | 3.0 | 1.5 | 12.0 | 5.0 | 8.5 | 4.0 | 8.5 | 6.0 | 10.0 | 11.0 | 13.0 | 14.0 | 1.5 | 15.0 | 7.0 | 16.0 | |
70 | 1.0 | 4.0 | 10.0 | 5.5 | 8.0 | 5.5 | 7.0 | 2.0 | 11.0 | 13.0 | 12.0 | 14.0 | 3.0 | 15.0 | 9.0 | 16.0 | |
150 | 1.0 | 5.0 | 10.0 | 2.0 | 8.0 | 4.0 | 7.0 | 6.0 | 9.0 | 14.0 | 12.0 | 13.0 | 3.0 | 15.0 | 11.0 | 16.0 | |
200 | 1.0 | 5.0 | 10.0 | 3.0 | 8.0 | 6.0 | 9.0 | 4.0 | 7.0 | 14.0 | 11.0 | 13.0 | 2.0 | 15.0 | 12.0 | 16.0 | |
300 | 3.0 | 5.0 | 9.5 | 1.0 | 8.0 | 6.0 | 7.0 | 2.0 | 9.5 | 14.0 | 13.0 | 12.0 | 4.0 | 15.0 | 11.0 | 16.0 | |
450 | 2.0 | 6.0 | 11.0 | 1.0 | 7.5 | 3.0 | 9.0 | 4.0 | 7.5 | 14.0 | 13.0 | 12.0 | 5.0 | 16.0 | 10.0 | 15.0 | |
20 | 7.0 | 4.0 | 12.0 | 1.0 | 11.0 | 9.5 | 6.0 | 5.0 | 13.0 | 9.5 | 8.0 | 15.0 | 2.0 | 14.0 | 3.0 | 16.0 | |
70 | 2.0 | 7.0 | 10.0 | 1.0 | 8.5 | 11.0 | 5.5 | 4.0 | 13.0 | 12.0 | 8.5 | 16.0 | 3.0 | 14.0 | 5.5 | 15.0 | |
150 | 1.0 | 5.0 | 10.0 | 2.0 | 8.5 | 11.0 | 7.0 | 3.0 | 13.0 | 12.0 | 8.5 | 16.0 | 4.0 | 15.0 | 6.0 | 14.0 | |
200 | 1.0 | 3.0 | 10.0 | 2.0 | 8.0 | 12.0 | 5.0 | 7.0 | 11.0 | 13.0 | 9.0 | 16.0 | 4.0 | 14.0 | 6.0 | 15.0 | |
300 | 1.0 | 5.0 | 10.0 | 2.0 | 8.0 | 11.0 | 3.0 | 4.0 | 12.0 | 13.0 | 9.0 | 16.0 | 6.0 | 15.0 | 7.0 | 14.0 | |
450 | 1.0 | 5.0 | 10.0 | 2.0 | 8.0 | 12.0 | 4.0 | 3.0 | 11.0 | 13.0 | 9.0 | 16.0 | 6.0 | 15.0 | 7.0 | 14.0 | |
20 | 4.5 | 3.0 | 9.0 | 2.0 | 12.0 | 10.0 | 8.0 | 4.5 | 13.0 | 7.0 | 11.0 | 14.0 | 1.0 | 15.0 | 6.0 | 16.0 | |
70 | 3.0 | 4.0 | 11.0 | 2.0 | 10.0 | 15.0 | 5.0 | 6.5 | 12.0 | 8.0 | 9.0 | 16.0 | 1.0 | 14.0 | 6.5 | 13.0 | |
150 | 3.0 | 5.0 | 10.0 | 1.0 | 9.0 | 15.0 | 6.0 | 4.0 | 12.0 | 11.0 | 8.0 | 16.0 | 2.0 | 13.0 | 7.0 | 14.0 | |
200 | 3.0 | 5.0 | 11.0 | 2.0 | 8.0 | 15.0 | 6.0 | 4.0 | 12.0 | 10.0 | 9.0 | 16.0 | 1.0 | 14.0 | 7.0 | 13.0 | |
300 | 2.5 | 4.0 | 10.0 | 2.5 | 8.0 | 15.0 | 6.0 | 5.0 | 12.0 | 11.0 | 9.0 | 16.0 | 1.0 | 13.0 | 7.0 | 14.0 | |
450 | 2.0 | 5.0 | 10.0 | 4.0 | 9.0 | 15.0 | 6.0 | 3.0 | 12.0 | 11.0 | 8.0 | 16.0 | 1.0 | 14.0 | 7.0 | 13.0 | |
Ranks | 69.0 | 119.5 | 293.0 | 67.0 | 258.0 | 289.5 | 179.0 | 128.5 | 333.5 | 351.5 | 293.5 | 456.0 | 157.5 | 428.0 | 220.0 | 436.5 | |
Overall Rank | 2 | 3 | 10 | 1 | 8 | 9 | 6 | 4 | 12 | 13 | 11 | 16 | 5 | 14 | 7 | 15 |
Parameters | Measures | ||||||||
0.15 | 0.5 | 0.724887 | 4.68121 | 9.54363 | 21.3535 | 33.6724 | 64.8572 | 105.342 | 212.237 |
1.0 | 0.851403 | 2.16361 | 3.08928 | 4.62098 | 5.80279 | 8.0534 | 10.2636 | 14.5684 | |
3.0 | 0.947789 | 1.29338 | 1.45642 | 1.66563 | 1.79699 | 2.00444 | 2.1732 | 2.44233 | |
5.0 | 0.968338 | 1.16691 | 1.25306 | 1.35815 | 1.42144 | 1.51773 | 1.59316 | 1.70876 | |
7.0 | 0.977281 | 1.11656 | 1.17484 | 1.24441 | 1.28556 | 1.34718 | 1.39467 | 1.46623 | |
10 | 0.984042 | 1.08023 | 1.1194 | 1.1654 | 1.19224 | 1.23196 | 1.26221 | 1.3072 | |
0.6 | 0.5 | 0.0453054 | 0.292575 | 0.596477 | 1.33459 | 2.10452 | 4.05357 | 6.58386 | 13.2648 |
1.0 | 0.212851 | 0.540902 | 0.772319 | 1.15525 | 1.4507 | 2.01335 | 2.5659 | 3.64209 | |
3.0 | 0.59707 | 0.814779 | 0.917485 | 1.04928 | 1.13203 | 1.26272 | 1.36903 | 1.53857 | |
5.0 | 0.733863 | 0.884349 | 0.949641 | 1.02928 | 1.07725 | 1.15023 | 1.20739 | 1.295 | |
7.0 | 0.801698 | 0.915955 | 0.963765 | 1.02083 | 1.05459 | 1.10514 | 1.1441 | 1.2028 | |
10 | 0.856658 | 0.940398 | 0.974495 | 1.01454 | 1.03791 | 1.07249 | 1.09881 | 1.13798 | |
0.9 | 0.5 | 0.0201357 | 0.130033 | 0.265101 | 0.593152 | 0.935344 | 1.80159 | 2.92616 | 5.89547 |
1.0 | 0.1419 | 0.360602 | 0.514879 | 0.770164 | 0.967132 | 1.34223 | 1.7106 | 2.42806 | |
3.0 | 0.521588 | 0.711775 | 0.801497 | 0.916631 | 0.988922 | 1.10309 | 1.19596 | 1.34406 | |
5.0 | 0.676701 | 0.815465 | 0.875671 | 0.94911 | 0.993338 | 1.06063 | 1.11335 | 1.19413 | |
7.0 | 0.75658 | 0.864407 | 0.909526 | 0.96338 | 0.995237 | 1.04294 | 1.07971 | 1.13511 | |
10 | 0.822618 | 0.903031 | 0.935773 | 0.974223 | 0.996664 | 1.02987 | 1.05515 | 1.09276 | |
1.5 | 0.5 | 0.00724887 | 0.0468121 | 0.0954363 | 0.213535 | 0.336724 | 0.648572 | 1.05342 | 2.12237 |
1.0 | 0.0851403 | 0.216361 | 0.308928 | 0.462098 | 0.580279 | 0.80534 | 1.02636 | 1.45684 | |
3.0 | 0.439925 | 0.600334 | 0.676009 | 0.773116 | 0.834089 | 0.930379 | 1.00871 | 1.13363 | |
5.0 | 0.61098 | 0.736268 | 0.790626 | 0.856933 | 0.896866 | 0.957626 | 1.00522 | 1.07816 | |
7.0 | 0.703335 | 0.803573 | 0.845517 | 0.895581 | 0.925196 | 0.969546 | 1.00372 | 1.05522 | |
10 | 0.781652 | 0.85806 | 0.889172 | 0.925707 | 0.94703 | 0.978584 | 1.00261 | 1.03834 | |
2.5 | 0.5 | 0.00260959 | 0.0168523 | 0.0343571 | 0.0768725 | 0.121221 | 0.233486 | 0.37923 | 0.764053 |
1.0 | 0.0510842 | 0.129817 | 0.185357 | 0.277259 | 0.348167 | 0.483204 | 0.615817 | 0.874101 | |
3.0 | 0.371047 | 0.506341 | 0.570168 | 0.652071 | 0.703498 | 0.784712 | 0.85078 | 0.956138 | |
5.0 | 0.551642 | 0.664762 | 0.713841 | 0.773708 | 0.809762 | 0.864622 | 0.907591 | 0.973447 | |
7.0 | 0.653837 | 0.747021 | 0.786013 | 0.832554 | 0.860085 | 0.901313 | 0.933086 | 0.980961 | |
10 | 0.742726 | 0.815329 | 0.844891 | 0.879607 | 0.899868 | 0.92985 | 0.952676 | 0.986634 | |
4.5 | 0.5 | 0.00080543 | 0.00520134 | 0.010604 | 0.0237261 | 0.0374137 | 0.0720635 | 0.117046 | 0.235819 |
1.0 | 0.0283801 | 0.0721203 | 0.102976 | 0.154033 | 0.193426 | 0.268447 | 0.34212 | 0.485612 | |
3.0 | 0.305027 | 0.416248 | 0.468718 | 0.536049 | 0.578325 | 0.645088 | 0.699401 | 0.786013 | |
5.0 | 0.490459 | 0.591033 | 0.634669 | 0.687896 | 0.719951 | 0.768726 | 0.80693 | 0.865482 | |
7.0 | 0.601176 | 0.686855 | 0.722707 | 0.765499 | 0.790813 | 0.828721 | 0.857935 | 0.901954 | |
10 | 0.700328 | 0.768787 | 0.796661 | 0.829395 | 0.848499 | 0.87677 | 0.898293 | 0.930313 |
Model | Parameter | SE | Parameter | SE | Parameter | SE |
SPHLD() | 3.7870 | 0.4370 | 2.2162 | 0.1836 | – | – |
EGSHLD() | 7.0611 | 0.5863 | 3.3169 | 0.4593 | – | – |
EHLD() | 4.7771 | 0.3748 | 2.7077 | 0.3918 | – | – |
PGHLD() | 0.5901 | 0.0916 | 2.6059 | 0.2187 | 133.3208 | 3.0465 |
KHLD() | 2.5987 | 0.2161 | 141.1152 | 2.1935 | 0.5731 | 0.0893 |
HLWD() | 9.2668 | 0.0013 | 7.7567 | 0.0075 | 0.2865 | 0.0236 |
PHLD() | 6.3117 | 0.7676 | 2.2754 | 0.1877 | – | – |
Models | -2logL | AIC | BIC | CAIC | HQIC | KS | p(KS) | CVM | p(CVM) | AD | p(AD) |
SPHLD | -50.0009 | -46.0009 | -40.6552 | -45.8877 | -43.8338 | 0.0635 | 0.7812 | 0.1027 | 0.5727 | 0.8752 | 0.4295 |
EGSHLD | -18.5522 | -14.5522 | -9.2065 | -14.439 | -12.3851 | 0.1367 | 0.0367 | 0.678 | 0.0142 | 3.9479 | 0.0093 |
EHLD | -20.661 | -16.661 | -11.3154 | -16.5478 | -14.494 | 0.1291 | 0.0566 | 0.6068 | 0.0213 | 3.6361 | 0.0132 |
PGHLD | -41.7841 | -35.7841 | -27.7656 | -35.5556 | -32.5335 | 0.0868 | 0.3958 | 0.2084 | 0.2519 | 1.5726 | 0.1601 |
KHLD | -42.0805 | -36.0805 | -28.062 | -35.8519 | -32.8299 | 0.0865 | 0.4001 | 0.2067 | 0.255 | 1.5561 | 0.1637 |
HLWD | -50.7479 | -44.7479 | -36.7294 | -44.5193 | -41.4973 | 0.083 | 0.4532 | 0.1787 | 0.3131 | 1.088 | 0.3141 |
PHLD | -48.4568 | -44.4568 | -39.1112 | -44.3436 | -42.2898 | 0.0647 | 0.7624 | 0.1082 | 0.5469 | 0.9613 | 0.378 |
Model | parameter | SE | parameter | SE | parameter | SE |
SPHLD() | 0.0134 | 0.0048 | 1.0754 | 0.0806 | – | – |
EGSHLD() | 0.0271 | 0.0029 | 1.4176 | 0.1774 | – | – |
EHLD() | 0.0330 | 0.0030 | 1.1827 | 0.1406 | – | – |
PGHLD() | 0.0029 | 6.0E-04 | 1.3095 | 0.0959 | 31.6416 | 3.0606 |
KHLD() | 1.7763 | 2.0E-04 | 0.1171 | 0.0106 | 0.2100 | 1.0E-04 |
HLWD() | 3.2E-05 | 0.0000 | 2.3012 | 0.0925 | 0.5459 | 0.0382 |
PHLD() | 0.0188 | 0.0068 | 1.1120 | 0.0833 | – | – |
Models | -2logL | AIC | BIC | CAIC | HQIC | KS | p(KS) | CVM | p(CVM) | AD | p(AD) |
SPHLD | 1158.5700 | 1162.5700 | 1168.1620 | 1162.4700 | 1164.8410 | 0.0460 | 0.9603 | 0.0697 | 0.7543 | 0.5285 | 0.7176 |
EGSHLD | 1161.6180 | 1165.6180 | 1171.2090 | 1165.7180 | 1167.8890 | 0.0848 | 0.3489 | 0.0784 | 0.7023 | 0.5126 | 0.7337 |
EHLD | 1158.7411 | 1162.7411 | 1168.4002 | 1162.5110 | 1164.9820 | 0.0569 | 0.8286 | 0.0519 | 0.8652 | 0.4096 | 0.8389 |
PGHLD | 1158.6990 | 1164.1990 | 1172.5860 | 1164.4010 | 1167.6050 | 0.0606 | 0.7658 | 0.0522 | 0.8638 | 0.3987 | 0.8498 |
KHLD | 1163.9130 | 1169.9130 | 1178.3000 | 1170.1140 | 1173.3190 | 0.1032 | 0.1521 | 0.1349 | 0.4395 | 0.7670 | 0.5051 |
HLWD | 1158.8090 | 1163.0090 | 1171.3960 | 1163.2110 | 1166.4150 | 0.0538 | 0.8752 | 0.0634 | 0.7934 | 0.4578 | 0.7898 |
PHLD | 1158.6310 | 1162.6310 | 1168.8022 | 1162.5310 | 1164.8702 | 0.0492 | 0.9311 | 0.0597 | 0.8170 | 0.4685 | 0.7788 |
n | Est. | MLE | ADE | CVME | MPSE | OLSE | PCE | RTADE | WLSE | LTADE | MSADE | MSALDE | ADSOE | KE | MSSD | MSSLD | MSLND |
20 | BIAS() | ||||||||||||||||
BIAS() | |||||||||||||||||
MSE() | |||||||||||||||||
MSE() | |||||||||||||||||
MRE() | |||||||||||||||||
MRE() | |||||||||||||||||
ASAE | |||||||||||||||||
70 | BIAS() | ||||||||||||||||
BIAS() | |||||||||||||||||
MSE() | |||||||||||||||||
MSE() | |||||||||||||||||
MRE() | |||||||||||||||||
MRE() | |||||||||||||||||
ASAE | |||||||||||||||||
150 | BIAS() | ||||||||||||||||
BIAS() | |||||||||||||||||
MSE() | |||||||||||||||||
MSE() | |||||||||||||||||
MRE() | |||||||||||||||||
MRE() | |||||||||||||||||
ASAE | |||||||||||||||||
200 | BIAS() | ||||||||||||||||
BIAS() | |||||||||||||||||
MSE() | |||||||||||||||||
MSE() | |||||||||||||||||
MRE() | |||||||||||||||||
MRE() | |||||||||||||||||
ASAE | |||||||||||||||||
300 | BIAS() | ||||||||||||||||
BIAS() | |||||||||||||||||
MSE() | |||||||||||||||||
MSE() | |||||||||||||||||
MRE() | |||||||||||||||||
MRE() | |||||||||||||||||
ASAE | |||||||||||||||||
450 | BIAS() | ||||||||||||||||
BIAS() | |||||||||||||||||
MSE() | |||||||||||||||||
MSE() | |||||||||||||||||
MRE() | |||||||||||||||||
MRE() | |||||||||||||||||
ASAE | |||||||||||||||||
n | Est. | MLE | ADE | CVME | MPSE | OLSE | PCE | RTADE | WLSE | LTADE | MSADE | MSALDE | ADSOE | KE | MSSD | MSSLD | MSLND |
20 | BIAS() | ||||||||||||||||
BIAS() | |||||||||||||||||
MSE() | |||||||||||||||||
MSE() | |||||||||||||||||
MRE() | |||||||||||||||||
MRE() | |||||||||||||||||
ASAE | |||||||||||||||||
70 | BIAS() | ||||||||||||||||
BIAS() | |||||||||||||||||
MSE() | |||||||||||||||||
MSE() | |||||||||||||||||
MRE() | |||||||||||||||||
MRE() | |||||||||||||||||
ASAE | |||||||||||||||||
150 | BIAS() | ||||||||||||||||
BIAS() | |||||||||||||||||
MSE() | |||||||||||||||||
MSE() | |||||||||||||||||
MRE() | |||||||||||||||||
MRE() | |||||||||||||||||
ASAE | |||||||||||||||||
200 | BIAS() | ||||||||||||||||
BIAS() | |||||||||||||||||
MSE() | |||||||||||||||||
MSE() | |||||||||||||||||
MRE() | |||||||||||||||||
MRE() | |||||||||||||||||
ASAE | |||||||||||||||||
300 | BIAS() | ||||||||||||||||
BIAS() | |||||||||||||||||
MSE() | |||||||||||||||||
MSE() | |||||||||||||||||
MRE() | |||||||||||||||||
MRE() | |||||||||||||||||
ASAE | |||||||||||||||||
450 | BIAS() | ||||||||||||||||
BIAS() | |||||||||||||||||
MSE() | |||||||||||||||||
MSE() | |||||||||||||||||
MRE() | |||||||||||||||||
MRE() | |||||||||||||||||
ASAE | |||||||||||||||||
n | Est. | MLE | ADE | CVME | MPSE | OLSE | PCE | RTADE | WLSE | LTADE | MSADE | MSALDE | ADSOE | KE | MSSD | MSSLD | MSLND |
20 | BIAS() | ||||||||||||||||
BIAS() | |||||||||||||||||
MSE() | |||||||||||||||||
MSE() | |||||||||||||||||
MRE() | |||||||||||||||||
MRE() | |||||||||||||||||
ASAE | |||||||||||||||||
70 | BIAS() | ||||||||||||||||
BIAS() | |||||||||||||||||
MSE() | |||||||||||||||||
MSE() | |||||||||||||||||
MRE() | |||||||||||||||||
MRE() | |||||||||||||||||
ASAE | |||||||||||||||||
150 | BIAS() | ||||||||||||||||
BIAS() | |||||||||||||||||
MSE() | |||||||||||||||||
MSE() | |||||||||||||||||
MRE() | |||||||||||||||||
MRE() | |||||||||||||||||
ASAE | |||||||||||||||||
200 | BIAS() | ||||||||||||||||
BIAS() | |||||||||||||||||
MSE() | |||||||||||||||||
MSE() | |||||||||||||||||
MRE() | |||||||||||||||||
MRE() | |||||||||||||||||
ASAE | |||||||||||||||||
300 | BIAS() | ||||||||||||||||
BIAS() | |||||||||||||||||
MSE() | |||||||||||||||||
MSE() | |||||||||||||||||
MRE() | |||||||||||||||||
MRE() | |||||||||||||||||
ASAE | |||||||||||||||||
450 | BIAS() | ||||||||||||||||
BIAS() | |||||||||||||||||
MSE() | |||||||||||||||||
MSE() | |||||||||||||||||
MRE() | |||||||||||||||||
MRE() | |||||||||||||||||
ASAE | |||||||||||||||||
n | Est. | MLE | ADE | CVME | MPSE | OLSE | PCE | RTADE | WLSE | LTADE | MSADE | MSALDE | ADSOE | KE | MSSD | MSSLD | MSLND |
20 | BIAS() | ||||||||||||||||
BIAS() | |||||||||||||||||
MSE() | |||||||||||||||||
MSE() | |||||||||||||||||
MRE() | |||||||||||||||||
MRE() | |||||||||||||||||
ASAE | |||||||||||||||||
70 | BIAS() | ||||||||||||||||
BIAS() | |||||||||||||||||
MSE() | |||||||||||||||||
MSE() | |||||||||||||||||
MRE() | |||||||||||||||||
MRE() | |||||||||||||||||
ASAE | |||||||||||||||||
150 | BIAS() | ||||||||||||||||
BIAS() | |||||||||||||||||
MSE() | |||||||||||||||||
MSE() | |||||||||||||||||
MRE() | |||||||||||||||||
MRE() | |||||||||||||||||
ASAE | |||||||||||||||||
200 | BIAS() | ||||||||||||||||
BIAS() | |||||||||||||||||
MSE() | |||||||||||||||||
MSE() | |||||||||||||||||
MRE() | |||||||||||||||||
MRE() | |||||||||||||||||
ASAE | |||||||||||||||||
300 | BIAS() | ||||||||||||||||
BIAS() | |||||||||||||||||
MSE() | |||||||||||||||||
MSE() | |||||||||||||||||
MRE() | |||||||||||||||||
MRE() | |||||||||||||||||
ASAE | |||||||||||||||||
450 | BIAS() | ||||||||||||||||
BIAS() | |||||||||||||||||
MSE() | |||||||||||||||||
MSE() | |||||||||||||||||
MRE() | |||||||||||||||||
MRE() | |||||||||||||||||
ASAE | |||||||||||||||||
n | Est. | MLE | ADE | CVME | MPSE | OLSE | PCE | RTADE | WLSE | LTADE | MSADE | MSALDE | ADSOE | KE | MSSD | MSSLD | MSLND |
20 | BIAS() | ||||||||||||||||
BIAS() | |||||||||||||||||
MSE() | |||||||||||||||||
MSE() | |||||||||||||||||
MRE() | |||||||||||||||||
MRE() | |||||||||||||||||
ASAE | |||||||||||||||||
70 | BIAS() | ||||||||||||||||
BIAS() | |||||||||||||||||
MSE() | |||||||||||||||||
MSE() | |||||||||||||||||
MRE() | |||||||||||||||||
MRE() | |||||||||||||||||
ASAE | |||||||||||||||||
150 | BIAS() | ||||||||||||||||
BIAS() | |||||||||||||||||
MSE() | |||||||||||||||||
MSE() | |||||||||||||||||
MRE() | |||||||||||||||||
MRE() | |||||||||||||||||
ASAE | |||||||||||||||||
200 | BIAS() | ||||||||||||||||
BIAS() | |||||||||||||||||
MSE() | |||||||||||||||||
MSE() | |||||||||||||||||
MRE() | |||||||||||||||||
MRE() | |||||||||||||||||
ASAE | |||||||||||||||||
300 | BIAS() | ||||||||||||||||
BIAS() | |||||||||||||||||
MSE() | |||||||||||||||||
MSE() | |||||||||||||||||
MRE() | |||||||||||||||||
MRE() | |||||||||||||||||
ASAE | |||||||||||||||||
450 | BIAS() | ||||||||||||||||
BIAS() | |||||||||||||||||
MSE() | |||||||||||||||||
MSE() | |||||||||||||||||
MRE() | |||||||||||||||||
MRE() | |||||||||||||||||
ASAE | |||||||||||||||||
Parameter | MLE | ADE | CVME | MPSE | OLSE | PCE | RTADE | WLSE | LTADE | MSADE | MSALDE | ADSOE | KE | MSSD | MSSLD | MSLND | |
20 | 7.0 | 6.0 | 13.0 | 1.0 | 11.0 | 2.0 | 9.0 | 4.5 | 12.0 | 8.0 | 10.0 | 15.0 | 3.0 | 14.0 | 4.5 | 16.0 | |
70 | 1.0 | 4.0 | 11.0 | 2.0 | 10.0 | 3.0 | 6.0 | 5.0 | 13.0 | 12.0 | 8.5 | 16.0 | 7.0 | 14.0 | 8.5 | 15.0 | |
150 | 1.0 | 3.0 | 9.0 | 2.0 | 11.0 | 4.0 | 6.0 | 5.0 | 13.0 | 12.0 | 10.0 | 16.0 | 8.0 | 15.0 | 7.0 | 14.0 | |
200 | 1.0 | 4.0 | 11.0 | 2.0 | 9.0 | 3.0 | 6.0 | 5.0 | 13.0 | 12.0 | 10.0 | 16.0 | 8.0 | 14.0 | 7.0 | 15.0 | |
300 | 1.0 | 4.0 | 8.0 | 3.0 | 11.0 | 2.0 | 5.0 | 6.0 | 12.0 | 13.0 | 9.0 | 16.0 | 10.0 | 15.0 | 7.0 | 14.0 | |
450 | 2.0 | 3.0 | 11.0 | 1.0 | 9.0 | 4.0 | 6.0 | 5.0 | 12.0 | 13.0 | 10.0 | 16.0 | 7.0 | 15.0 | 8.0 | 14.0 | |
20 | 2.0 | 3.0 | 9.0 | 1.0 | 7.0 | 15.0 | 5.0 | 4.0 | 10.0 | 11.0 | 8.0 | 16.0 | 12.0 | 14.0 | 6.0 | 13.0 | |
70 | 4.0 | 2.0 | 8.5 | 5.0 | 7.0 | 15.0 | 3.0 | 1.0 | 8.5 | 12.0 | 10.0 | 16.0 | 11.0 | 13.0 | 6.0 | 14.0 | |
150 | 1.0 | 3.0 | 7.0 | 2.0 | 8.0 | 14.5 | 4.0 | 5.0 | 10.0 | 12.0 | 9.0 | 16.0 | 11.0 | 13.0 | 6.0 | 14.5 | |
200 | 3.0 | 1.0 | 7.0 | 4.0 | 6.0 | 15.0 | 5.0 | 2.0 | 10.0 | 12.0 | 9.0 | 16.0 | 11.0 | 13.0 | 8.0 | 14.0 | |
300 | 1.0 | 3.0 | 7.0 | 2.0 | 6.0 | 16.0 | 4.0 | 5.0 | 9.0 | 12.0 | 10.0 | 15.0 | 11.0 | 14.0 | 8.0 | 13.0 | |
450 | 3.0 | 2.0 | 6.0 | 1.0 | 7.0 | 16.0 | 5.0 | 4.0 | 11.0 | 12.0 | 10.0 | 15.0 | 8.0 | 13.0 | 9.0 | 14.0 | |
20 | 3.0 | 1.5 | 12.0 | 5.0 | 8.5 | 4.0 | 8.5 | 6.0 | 10.0 | 11.0 | 13.0 | 14.0 | 1.5 | 15.0 | 7.0 | 16.0 | |
70 | 1.0 | 4.0 | 10.0 | 5.5 | 8.0 | 5.5 | 7.0 | 2.0 | 11.0 | 13.0 | 12.0 | 14.0 | 3.0 | 15.0 | 9.0 | 16.0 | |
150 | 1.0 | 5.0 | 10.0 | 2.0 | 8.0 | 4.0 | 7.0 | 6.0 | 9.0 | 14.0 | 12.0 | 13.0 | 3.0 | 15.0 | 11.0 | 16.0 | |
200 | 1.0 | 5.0 | 10.0 | 3.0 | 8.0 | 6.0 | 9.0 | 4.0 | 7.0 | 14.0 | 11.0 | 13.0 | 2.0 | 15.0 | 12.0 | 16.0 | |
300 | 3.0 | 5.0 | 9.5 | 1.0 | 8.0 | 6.0 | 7.0 | 2.0 | 9.5 | 14.0 | 13.0 | 12.0 | 4.0 | 15.0 | 11.0 | 16.0 | |
450 | 2.0 | 6.0 | 11.0 | 1.0 | 7.5 | 3.0 | 9.0 | 4.0 | 7.5 | 14.0 | 13.0 | 12.0 | 5.0 | 16.0 | 10.0 | 15.0 | |
20 | 7.0 | 4.0 | 12.0 | 1.0 | 11.0 | 9.5 | 6.0 | 5.0 | 13.0 | 9.5 | 8.0 | 15.0 | 2.0 | 14.0 | 3.0 | 16.0 | |
70 | 2.0 | 7.0 | 10.0 | 1.0 | 8.5 | 11.0 | 5.5 | 4.0 | 13.0 | 12.0 | 8.5 | 16.0 | 3.0 | 14.0 | 5.5 | 15.0 | |
150 | 1.0 | 5.0 | 10.0 | 2.0 | 8.5 | 11.0 | 7.0 | 3.0 | 13.0 | 12.0 | 8.5 | 16.0 | 4.0 | 15.0 | 6.0 | 14.0 | |
200 | 1.0 | 3.0 | 10.0 | 2.0 | 8.0 | 12.0 | 5.0 | 7.0 | 11.0 | 13.0 | 9.0 | 16.0 | 4.0 | 14.0 | 6.0 | 15.0 | |
300 | 1.0 | 5.0 | 10.0 | 2.0 | 8.0 | 11.0 | 3.0 | 4.0 | 12.0 | 13.0 | 9.0 | 16.0 | 6.0 | 15.0 | 7.0 | 14.0 | |
450 | 1.0 | 5.0 | 10.0 | 2.0 | 8.0 | 12.0 | 4.0 | 3.0 | 11.0 | 13.0 | 9.0 | 16.0 | 6.0 | 15.0 | 7.0 | 14.0 | |
20 | 4.5 | 3.0 | 9.0 | 2.0 | 12.0 | 10.0 | 8.0 | 4.5 | 13.0 | 7.0 | 11.0 | 14.0 | 1.0 | 15.0 | 6.0 | 16.0 | |
70 | 3.0 | 4.0 | 11.0 | 2.0 | 10.0 | 15.0 | 5.0 | 6.5 | 12.0 | 8.0 | 9.0 | 16.0 | 1.0 | 14.0 | 6.5 | 13.0 | |
150 | 3.0 | 5.0 | 10.0 | 1.0 | 9.0 | 15.0 | 6.0 | 4.0 | 12.0 | 11.0 | 8.0 | 16.0 | 2.0 | 13.0 | 7.0 | 14.0 | |
200 | 3.0 | 5.0 | 11.0 | 2.0 | 8.0 | 15.0 | 6.0 | 4.0 | 12.0 | 10.0 | 9.0 | 16.0 | 1.0 | 14.0 | 7.0 | 13.0 | |
300 | 2.5 | 4.0 | 10.0 | 2.5 | 8.0 | 15.0 | 6.0 | 5.0 | 12.0 | 11.0 | 9.0 | 16.0 | 1.0 | 13.0 | 7.0 | 14.0 | |
450 | 2.0 | 5.0 | 10.0 | 4.0 | 9.0 | 15.0 | 6.0 | 3.0 | 12.0 | 11.0 | 8.0 | 16.0 | 1.0 | 14.0 | 7.0 | 13.0 | |
Ranks | 69.0 | 119.5 | 293.0 | 67.0 | 258.0 | 289.5 | 179.0 | 128.5 | 333.5 | 351.5 | 293.5 | 456.0 | 157.5 | 428.0 | 220.0 | 436.5 | |
Overall Rank | 2 | 3 | 10 | 1 | 8 | 9 | 6 | 4 | 12 | 13 | 11 | 16 | 5 | 14 | 7 | 15 |