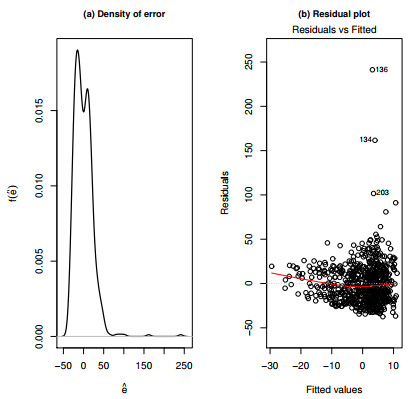
Breast cancer has a very high incidence rate worldwide, and effective screening and early diagnosis are particularly important. In this paper, two improved You Only Look Once version 8 (YOLOv8) models, the YOLOv8-GHOST and YOLOv8-P2 models, are proposed to address the difficulty of distinguishing lesions from normal tissues in mammography images. The YOLOv8-GHOST model incorporates GHOSTConv and C3GHOST modules into the original YOLOv8 model to capture richer feature information while using only 57% of the number of parameters required by the original model. The YOLOv8-P2 algorithm significantly reduces the number of necessary parameters by streamlining the number of channels in the feature map. This paper proposes the YOLOv8-GHOST-P2 model by combining the above two improvements. Experiments conducted on the MIAS and DDSM datasets show that the new models achieved significantly improved computational efficiency while maintaining high detection accuracy. Compared with the traditional YOLOv8 method, the three new models improved and achieved F1 scores of 98.38%, 98.8%, and 98.57%, while the number of parameters reduced by 42.9%, 46.64%, and 2.8%. These improvements provide a more efficient and accurate tool for clinical breast cancer screening and lay the foundation for subsequent studies. Future work will explore the potential applications of the developed models to other medical image analysis tasks.
Citation: Yihua Lan, Yingjie Lv, Jiashu Xu, Yingqi Zhang, Yanhong Zhang. Breast mass lesion area detection method based on an improved YOLOv8 model[J]. Electronic Research Archive, 2024, 32(10): 5846-5867. doi: 10.3934/era.2024270
[1] | Kayode Oshinubi, Firas Ibrahim, Mustapha Rachdi, Jacques Demongeot . Functional data analysis: Application to daily observation of COVID-19 prevalence in France. AIMS Mathematics, 2022, 7(4): 5347-5385. doi: 10.3934/math.2022298 |
[2] | Ahmed Sedky Eldeeb, Muhammad Ahsan-ul-Haq, Mohamed S. Eliwa . A discrete Ramos-Louzada distribution for asymmetric and over-dispersed data with leptokurtic-shaped: Properties and various estimation techniques with inference. AIMS Mathematics, 2022, 7(2): 1726-1741. doi: 10.3934/math.2022099 |
[3] | Fatimah A Almulhim, Torkia Merouan, Mohammed B. Alamari, Boubaker Mechab . Local linear estimation for the censored functional regression. AIMS Mathematics, 2024, 9(6): 13980-13997. doi: 10.3934/math.2024679 |
[4] | Yanting Xiao, Yifan Shi . Robust estimation for varying-coefficient partially nonlinear model with nonignorable missing response. AIMS Mathematics, 2023, 8(12): 29849-29871. doi: 10.3934/math.20231526 |
[5] | Oussama Bouanani, Salim Bouzebda . Limit theorems for local polynomial estimation of regression for functional dependent data. AIMS Mathematics, 2024, 9(9): 23651-23691. doi: 10.3934/math.20241150 |
[6] | Lijie Zhou, Liucang Wu, Bin Yang . Estimation and diagnostic for single-index partially functional linear regression model with p-order autoregressive skew-normal errors. AIMS Mathematics, 2025, 10(3): 7022-7066. doi: 10.3934/math.2025321 |
[7] | Alanazi Talal Abdulrahman, Khudhayr A. Rashedi, Tariq S. Alshammari, Eslam Hussam, Amirah Saeed Alharthi, Ramlah H Albayyat . A new extension of the Rayleigh distribution: Methodology, classical, and Bayes estimation, with application to industrial data. AIMS Mathematics, 2025, 10(2): 3710-3733. doi: 10.3934/math.2025172 |
[8] | Amal S. Hassan, Najwan Alsadat, Oluwafemi Samson Balogun, Baria A. Helmy . Bayesian and non-Bayesian estimation of some entropy measures for a Weibull distribution. AIMS Mathematics, 2024, 9(11): 32646-32673. doi: 10.3934/math.20241563 |
[9] | Liqi Xia, Xiuli Wang, Peixin Zhao, Yunquan Song . Empirical likelihood for varying coefficient partially nonlinear model with missing responses. AIMS Mathematics, 2021, 6(7): 7125-7152. doi: 10.3934/math.2021418 |
[10] | Said Attaoui, Billal Bentata, Salim Bouzebda, Ali Laksaci . The strong consistency and asymptotic normality of the kernel estimator type in functional single index model in presence of censored data. AIMS Mathematics, 2024, 9(3): 7340-7371. doi: 10.3934/math.2024356 |
Breast cancer has a very high incidence rate worldwide, and effective screening and early diagnosis are particularly important. In this paper, two improved You Only Look Once version 8 (YOLOv8) models, the YOLOv8-GHOST and YOLOv8-P2 models, are proposed to address the difficulty of distinguishing lesions from normal tissues in mammography images. The YOLOv8-GHOST model incorporates GHOSTConv and C3GHOST modules into the original YOLOv8 model to capture richer feature information while using only 57% of the number of parameters required by the original model. The YOLOv8-P2 algorithm significantly reduces the number of necessary parameters by streamlining the number of channels in the feature map. This paper proposes the YOLOv8-GHOST-P2 model by combining the above two improvements. Experiments conducted on the MIAS and DDSM datasets show that the new models achieved significantly improved computational efficiency while maintaining high detection accuracy. Compared with the traditional YOLOv8 method, the three new models improved and achieved F1 scores of 98.38%, 98.8%, and 98.57%, while the number of parameters reduced by 42.9%, 46.64%, and 2.8%. These improvements provide a more efficient and accurate tool for clinical breast cancer screening and lay the foundation for subsequent studies. Future work will explore the potential applications of the developed models to other medical image analysis tasks.
Functional data analysis (FDA) (e.g., [1]) has drawn considerable attention over recent years, owing to a great deal of flexibilities and universal applications in handling high-dimensional data sets. A fundamental and important tool for FDA is functional linear models.
There are a lot of researches in literature on the inference of functional linear models and their extensions, see, among others, [2,3,4] for earlier works, and [5,6,7,8,9,10] for recent works. As is well known, in the estimation of regression models, the choice of loss function is essential to obtain a highly efficient and robust estimator. Most of earlier works employed the square loss function and obtained ordinary least squares (OLS) estimators. In recent years, many other loss functions have been considered in the estimation of functional linear models and their extensions. Kato [6], Tang and Cheng [11] studied the quantile regression (QR) with functional linear models and partial functional linear models, respectively. Yu et al. [12] proposed a robust exponential squared loss estimation procedure (ESL) and established the asymptotic properties of the proposed estimators. Cai et al. [13] introduced a new robust estimation procedure by employing a modified Huber function, whose tail function is replaced by the exponential squared loss (H-ESL) in the partial functional linear model.
It is well known that the square loss function pays attention to reflect the distributional features of the entire distribution, whereas QR, ESL and H-ESL methods focus on bounding the influence of outliers when the data are heavy-tailed, respectively. Thus, developing a method, which can both reflect distributional features and bound outliers effectively, is highly desirable in data analysis. We note that, in the context of principal component analysis (PCA), Lim and Oh [14] proposed a new approach using a weighted linear combination of asymmetric Huber loss functions to demonstrate the distributional features of data as well as keep robust to outliers. The asymmetric Huber loss functions are defined as
ρτ(u)={(τ−1)(u+0.5c∗)foru<−c∗0.5(1−τ)u2/c∗for−c∗≤u<00.5τu2/c∗for0≤u<c∗τ(u−0.5c∗)forc∗≤u, | (1.1) |
with c∗=1.345, and τ∈(0,1) being a parameter to control the degree of skewness. The function ρτ(⋅) is equivalent to the Huber loss function (see, [15]) when τ is equal to 0.5 and is most exactly the same as the quantile loss function when c∗ is small enough.
Motivated by the appealing characteristics of the asymmetric Huber functions, in this paper, we first investigate a new asymmetric Huber regression (AHR) estimation procedure to analyse skewed data for the partial functional linear model, based on the functional principal component analysis. To improve the estimation accuracy for single AHR estimation, we develop a weighted composite asymmetric Huber regression (WCAHR) estimation by combining the strength across multiple asymmetric Huber regression models. A practical algorithm for WCAHR estimators based on pseudo data is developed to implement the estimation method. The asymptotic properties of the proposed estimators are also derived. Extensive simulations are carried out to show the superiority of the proposed estimators.
Finally, we apply the proposed methods to two data sets. In the first example, we analyze the electricity data. Figure 1 presents the estimated density of the residuals and the residual diagnostic plot obtained by fitting the model (4.1) in Section 4.1 via the OLS method. The distribution of the residuals is skewed, bimodal, and there are some outliers in the dataset. Given that the WCAHR can effectively manage such data, we use the proposed method to conduct an analysis to this data set. Another example in Section 4 considers the Tecator data set. Similarly, Figure 2 presents the density of the residuals and the residual diagnostic plot obtained by fitting the model (4.2) in Section 4.2 via the OLS method, which demonstrates that the distribution of the residuals is skewed and far from normality. Undoubtedly, WCAHR regression is applicable to analyzing this data set on account of its appealing features.
To our knowledge, it is the first to discuss the asymmetric Huber regression problems under functional models framework. The proposed WCAHR method possesses advantages that include the robustness to outliers as well as reflecting the relationships between potential explanatory variables and the entire distribution of response. It retains the advantages in analysing skewed data and the obtained estimators rely on the shape of the entire distribution rather than merely on the data nearby a specific quantile level or skewness level of the asymmetric Huber loss, thereby avoiding the limitations of these methods. These advantages are revealed by both theoretical conclusions and numerical results. The relevant algorithm is data-adaptive, and capable of reflecting the distributional features of the data without prior information, and is robust to outliers.
The rest of this paper is organized as follows. In Section 2, we formally describe the estimation procedures, and develop a new algorithm. We also establish the asymptotic behaviors of the proposed estimators as well as a list of technical assumptions needed in the theoretical research. In Section 3, the finite performances of the proposed estimators are evaluated through simulations. Section 4 illustrates the use of the proposed methods in the analyses of electricity data and Tecator data. Brief conclusions on the proposed methods are made in Section 5. All technique proofs are provided in Section A.
Let Y be a real value random variable, Z=(Z1,⋯,Zp)T be a p dimensional random vector with zero mean and finite second moment. Let {X(t):t∈T} be a zero mean, second-order stochastic process with sample paths in L2(T), which consists of square integrable functions with inner product ⟨x,y⟩=∫Tx(t)y(t)dt and norm ‖x‖=⟨x,x⟩1/2, respectively, here T is a bounded closed interval. Without loss of generality, we suppose T=[0,1] throughout the paper. The dependence between Y and (X,Z) is expressed by the partial functional linear regression as following,
Y=ZTα+∫10β(t)X(t)dt+e. | (2.1) |
Here, random error e is assumed to be independent of Z and X, α=(α1,⋯,αp)T is an unknown p-dimensional parameter vector, and the slope function β(⋅) is an unknown square integrable function on [0,1].
Let (Zi,Xi(⋅),Yi),i=1,⋯,n, be independent observations generated by model (2.1) and let ei=Yi−ZTiα−∫10β(t)Xi(t)dt,i=1,⋯,n. The covariance and empirical covariance functions for X(⋅) are defined as cX(t,s)=Cov(X(t),X(s)), ˆcX(t,s)=1n∑ni=1Xi(t)Xi(s) respectively. Based on the Mercer's Theorem, cX and ˆcX can be represented as following,
cX(t,s)=∞∑i=1λivi(t)vi(s),ˆcX(t,s)=∞∑i=1ˆλiˆvi(t)ˆvi(s), |
where λ1>λ2>⋅⋅⋅>0andˆλ1≥ˆλ2≥⋯≥ˆλn+1=⋅⋅⋅=0 are each the ordered eigenvalue sequences of the covariance operator CX and its estimator ˆCX with kernels cX and ˆcX, which are defined by CXf(s)=∫10cX(t,s)f(t)dt and ˆCXf(s)=∫10ˆcX(t,s)f(t)dt with CX being assumed strictly positive, and {vi(⋅)} and {ˆvi(⋅)} are the corresponding orthonormal eigenfunction sequences. Besides, (ˆvi(⋅),ˆλi) is treated as an estimator of (vi(⋅),λi).
Similarly, we can define cYX(⋅)=Cov(Y,X(⋅)), cZ=Var(Z)=E[ZZT], cZY=Cov(Z,Y), cZX(⋅)=Cov(Z,X(⋅))=(cZ1X(⋅),⋯,cZpX(⋅))T. And the corresponding empirical counterparts defined below can be used as their estimators,
ˆcYX=1nn∑i=1YiXi,ˆcZ=1nn∑i=1ZiZTi,ˆcZY=1nn∑i=1ZiYi,ˆcZX=1nn∑i=1ZiXi. |
By the Karhunen-Loève representation, Xi(t) and β(t) can be expanded into
β(t)=∞∑j=1γjvj(t),Xi(t)=∞∑j=1ξijvj(t),i=1,⋯,n, | (2.2) |
here γj=⟨β(⋅),vj(⋅)⟩=∫10β(t)vj(t)dt, and ξij=⟨Xi(⋅),vj(⋅)⟩.
Owing to the orthogonality of {v1(⋅),...,vm(⋅)} and Eq (2.2), Model (2.1) can be transformed into
Yi=ZTiα+m∑j=1γjξij+˜ei=ZTiα+UTiγ+˜ei,i=1,⋯,n, |
where Ui=(ξi1,...,ξim)T, γ=(γ1,...,γm)T, ˜ei=∑∞j=m+1γjξij+ei, and the tuning parameter m may increase with the sample size n.
Replacing vj(⋅) with its estimator ˆvj(⋅), the τth AHR estimators ˉα and ˉβ(t)=∑mj=1ˉγjˆvj(t) can be obtained by minimizing the loss function over α, γ and bτ as follows:
(ˉb,ˉα,ˉγ)≜argmin(bτ,α,γ)n∑i=1ρτ(Yi−bτ−ZTiα−ˆUTiγ), |
where the asymmetric Huber loss function ρτ(u) is defined in (1.1), and ˆUi=(ˆξi1,⋯,ˆξim)T with ˆξij=⟨Xi(⋅),ˆvj(⋅)⟩. Here the true value of bτ is defined as the solution that minimizes the loss function E{ρτ(e−θ)} over θ∈R, and we call it the τth number of e with respect to (1.1).
Remark 1. In model (2.1), we suppose the intercept term is zero. In fact, if there is an intercept, we then may absorb it into the distribution of e. Thus, the main impact of model (2.1) is finding the contribution of the predictors to the response, and the zero mean assumption for e is not needed.
Noting that the regression coefficients are the same across different skewness asymmetric Huber regression models, and being inspirited by [14] and [16], we combine the strength across multiple asymmetric Huber regression models and propose a WCAHR method. Specifically, the WCAHR estimators ˆα and ˆβ(t)=∑mj=1ˆγjˆvj(t) can be obtained by minimizing the following loss function with respect to (b,α,γ):
Qn(b,α,γ)≜n∑i=1K∑k=1wkρτk(Yi−bk−ZTiα−ˆUTiγ), |
where {τk}Kk=1 are predetermined levels over (0, 1), bk=bτk for brevity, b=(b1,⋯,bK)T, and the weights w1,…,wK, which control the contribution of each loss function, are positive constants satisfying ∑kwk=1.
Remark 2. Generally speaking, one can choose the equidistant levels as τk=k/(K+1) for k=1,2,…,K for a given K, similar to what often has been done in composite quantile regression. Although one can also apply data-adaptive methods, such as cross validation, to select K, we do not pursue this topic here. As for the weights, we consider two choices. The first is using the equal weights w1=⋯=wK=1/K. The obtained estimators are called composite asymmetric Huber regression (CAHR) estimators. As the second choice in this study, to preferably describe the distribution information of the data, we consider a K-dimensional weight vector (w1,⋯,wK)=(f(b01),⋯,f(b0K))/∑Kk=1f(b0k), where b0=(b01,⋯,b0K) is the true value vector of b, and f(⋅) is the density function of the random error. In practice, we estimate f(⋅) by kernel density estimation method.
Denote S={(Yi,Zi,Xi(⋅)):1≤i≤n}, and given a new copy of (Z,X), namely the predictor variables (Zn+1,Xn+1(⋅)), once we gain the estimated α and β(t), the mean squared prediction error (MSPE) can be obtained, take asymmetric Huber regression for example,
MSPEAHR=E[{(ˉb+ZTn+1ˉα+∫10ˉβ(t)Xn+1(t)dt)−(bτ+ZTn+1α0+∫10β0(t)Xn+1(t)dt)}2|S], |
where α0 and β0(t) are the true values of α and β(t), respectively. The MSPEs of CAHR and WCAHR have analoguos definitions, and denoted by MSPECAHR and MSPEWCAHR, respectively.
Note that the minimization problems for AHR and CAHR estimators are special cases of WCAHR method. Here, we just present the practical algorithm for WCAHR based on pseudo data. A similar argument can be found in [14] to implement the data-adaptive principal component analysis. The algorithm is simply described as following.
Step 1. Given initial estimators ˆα(0) and ˆγ(0) for α0 and γ0, respectively.
Step 2. Iterate, until convergence, following these three steps for L=0,1,…
(a) Compute the residuals as ˆe(L)i=Yi−ZTiˆα(L)−ˆUTiˆγ(L). (b) Calculate the empirical pseudo data vector G(L)=(G(L)1,⋯,G(L)n)T in the element-wise way, G(L)i=ZTiˆα(L)+ˆUTiˆγ(L)+∑Kk=1wkψτk(ˆe(L)i−ˆb(L)k), for given weights (w1,⋯,wK) and ˆb(L)k=argminμ∑ni=1ρτk(ˆe(L)i−μ) at each k. Here ψτk(u)=ρ′τk(u)=(τk−1)I(u<−c∗)+(1−τk)c∗uI(−c∗≤u<0)+τkc∗uI(0≤u<c∗)+τkI(u≥c∗). (c) Obtain next iterative estimates ˆα(L+1) and ˆγ(L+1) by using the OLS method for response variable ˜Yi=G(L)i and covariates Zi,ˆUi.
In this section, we will establish the asymptotic properties of the estimators defined in the previous section. We shall first present some notations, suppose γ0=(γ01,...,γ0m)T is the true values of γ, F(⋅) is the cumulative distribution function of the random error. In addition, the notation ‖⋅‖ represents the L2 norm of a function or the Euclidean norm of a vector, and an∼bn indicates that an/bn is bounded away from zero and infinity as n→∞. For simplicity, in this paper, C represents a generic positive constant whose value may change from line to line. Next, to obtain the asymptotic properties, some technical assumptions are listed as follows.
C1. The random process X(⋅) and score ξj=⟨X(⋅),vj(⋅)⟩ satisfy the following condition: E‖X(⋅)‖4<∞ and E[ξ4j]≤Cλ2j, j≥1.
C2. The eigenvalues of CX and the score coefficients fulfil the conditions below:
(a) There exist constants C and a>1 such that C−1j−a≤λj≤Cj−a,λj−λj+1≥Cj−a−1, j≥1;
(b) There exist constants C and b>a/2+1 such that |γj|≤Cj−b, j≥1.
C3. The random vector Z satisfies E‖Z‖4<∞.
C4. There is some constant C such that ∣⟨cZlX,vj⟩∣≤Cj−(a+b),l=1,⋯,p, j≥1.
C5. Let ηil=Zil−⟨gl,Xi⟩ with gl=∞∑j=1λ−1j⟨cZlX,vj⟩vj, and ηi=(ηi1,⋯,ηip)T, then η1,⋯,ηn are i.i.d random vectors. We further assume that E[ηi|Xi]=0,E[ηiηiT|Xi]a.s.=Σ is a constant positive definite matrix.
C6. bτ is the unique solution of E[ρ′τ(e−bτ)]=0, and hτ(bτ)=(1−τ)f(bτ−c∗)+1−τc∗(F(bτ)−F(bτ−c∗))+τc∗(F(bτ+c∗)−F(bτ))+τf(bτ+c∗) is continuous at bτ. Furthermore, we suppose that hτ(bτ)>0.
C6'. b0k is the unique solution of E[ρ′τk(ei−b0k)]=0, hτk(b0k)=(1−τk)f(b0k−c∗)+1−τkc∗(F(b0k)−F(b0k−c∗))+τkc∗(F(b0k+c∗)−F(b0k))+τkf(b0k+c∗) is continuous at b0k, k=1,⋯,K. Furthermore, there exist some positive constants C1,C2 such that 0<C1≤min.
C1 is the commonly used condition for establishing the consistency of the the empirical covariance operator of in functional linear model and partial functional regression model. For example, it has been adopted in [3,17,18] (mean regression), [6,11] (quantile regression), [12,13] (robust estimation procedure), among others. C2(a) is used to identify the slope function via preventing the spacings between eigenvalues being too small, and C2(b) ensures the sufficiently smooth of slope function . C3–C5 are needed to deal with the vector-type covariate in the model () (see [19]). More concretely, C3 is for the asymptotic behaviors of and . C4 is used to ensure the effect of truncation on the estimator of be sufficiently small. C5 is a commonly used condition in the literature on partial functional regression models (see for example, [4,20,21]). The assumptions on and are slightly strong, and are used to fix the identifiability of and simplify the proof of the theorems. It is easy to see that is the projection of onto , and , , even without the assumptions. The facts can be used to obtain similar results to the following theorems with more complicated technics (see, for example, [6]) and more conditions to ensure the identifiability. Other type conditions on can be found in [11,22]. C6 and C6 are specific to the AHR and WCAHR (CAHR) cases respectively, which are primarily used to ensure the asymptotic behaviors of our estimators.
The following theorems discuss the convergence rate of the estimated , the asymptotic normality of the estimated and the convergence rate of the mean squared prediction error. To obtain this, we further assume is independent of in this paper.
The next theorem establishes the large sample properties of the AHR estimators.
Theorem 1. Suppose that the Conditions C1–C6 are satisfied, and the tuning parameter , then
where represents convergence in distribution, and with .
The asymptotic properties of the proposed WCAHR estimators are presented in the following theorem.
Theorem 2. Under the Conditions C1–C5 and C6, if the tuning parameter is taken as , then
where and , here with .
Remark 3. The results illustrate that the slope function estimator has the same convergence rate as the estimators in [6] and [4], which are optimal in the minimax sense. Note that it is similar to quantile regression that no moment condition on error term is needed here. In addition, we notice that the rate attained in predicting is faster than the rate attained in estimating . Trace its root and use as an example, it is for the integral operator providing additional smoothness in computing from .
Remark 4. If all s are equal, then Theorem 2.2 reduces to the asymptotic properties of the CAHR estimators. Taking , it is easy to see that there is a weight vector such that . Note that the right hand side of the inequality is just the asymptotic variance given in Theorem 1.
In this section, a Monte Carlo simulation is used to investigate the finite sample properties of the proposed estimation approaches. The data sets used in the simulation are generated from the following model,
where the slope function , and , here , and s are mutually independent normal random variables with mean 0 and variance . The true values of parameters are set as , and with for .
Five different distributions for are considered as follows: (a) standard normal distribution ; (b) positively skewed normal distribution ; (c) positively skewed -distribution ; (d) mixture of normals , which produces a distribution with outliers of response; (e) bimodal distribution () with . The multiplier can be generated from either of the following two models:
(A) (homoscedastic) ;
(B) (heteroscedastic) , where , and .
Implementing the proposed estimation method requires the predetermined levels over (0, 1), i.e., . Similar to the setting in [14], we take , and choose the equidistant levels , . In addition, for the WCAHR estimator, we employ the adaptive weights given in Remark 2.
For comparison, we also calculate the OLS estimator, the least absolute deviation (LAD) estimator, the ESL estimator, the H-ESL estimator, the Huber estimator (which corresponds to the case of AHR estimator at ), the CAHR estimator, and the AHR estimators at and . In this study, the sample size is set as 200 or 400.
To implement these methods, we need to choose the tuning parameter . In this paper, is selected by the cumulative percentage of total variability (CPV) method, that is,
where equals . Other criterion, such as AIC, BIC, can be employed.
For each setting and different methods, bias (Bias), standard deviation (Sd) of the estimated and , and the mean squared error (MSE) of the estimated with , as well as the mean integrated squared error (MISE) of the estimated over S = 500 repetitions are summarized, where with s being 100 equally spaced grids in [0, 1], here , are the estimates of and from the th sampling, .
Table 1 presents the results in the homoscedastic case. From Table 1, we can see the following facts: (a) The Sd, MSE and MISE decrease as the sample size increases from to . (b) The proposed estimators are almost unbiased, which further illustrates the consistency combining with the fact (a). (c) The proposed adaptively weighted estimator performs similarly to the OLS estimator under the normal error, and is comparable to the corresponding H-ESL estimator for the mixture of normal distributions, but is significantly better than the other estimators considered when the distribution of model error is skewed or bimodal, and still enjoys the favoured being robust to outliers. This demonstrates that the proposed WCAHR estimator can well adapt to different error distributions, thus is more useful in practice.
Errors | Method | MISE | MSE() | |||||
Bias | Sd | Bias | Sd | |||||
200 | OLS | 0.2690 | 0.0229 | 0.0048 | 0.1074 | 0.0096 | 0.1059 | |
LAD | 0.3294 | 0.0354 | 0.0099 | 0.1320 | 0.0115 | 0.1333 | ||
ESL | 0.3434 | 0.0394 | 0.0009 | 0.1405 | 0.0013 | 0.1403 | ||
H-ESL | 0.2824 | 0.0259 | 0.0055 | 0.1141 | 0.0059 | 0.1133 | ||
AHR(0.25) | 0.3294 | 0.0786 | 0.0077 | 0.2014 | 0.0105 | 0.1948 | ||
Huber | 0.2758 | 0.0234 | 0.0063 | 0.1090 | 0.0102 | 0.1067 | ||
AHR(0.75) | 0.5251 | 0.0754 | 0.0092 | 0.1894 | 0.0150 | 0.1981 | ||
CAHR | 0.2689 | 0.0233 | 0.0051 | 0.1095 | 0.0091 | 0.1060 | ||
WCAHR | 0.2693 | 0.0230 | 0.0055 | 0.1080 | 0.0101 | 0.1058 | ||
400 | OLS | 0.1004 | 0.0105 | 0.0011 | 0.0710 | 0.0015 | 0.0738 | |
LAD | 0.1304 | 0.0163 | 0.0027 | 0.0880 | 0.0045 | 0.0924 | ||
ESL | 0.1349 | 0.0175 | 0.0010 | 0.0918 | 0.0008 | 0.0955 | ||
H-ESL | 0.1031 | 0.0113 | 0.0011 | 0.0727 | 0.0010 | 0.0773 | ||
AHR(0.25) | 0.1304 | 0.0358 | 0.0048 | 0.1313 | 0.0013 | 0.1361 | ||
Huber | 0.1048 | 0.0107 | 0.0018 | 0.0720 | 0.0027 | 0.0742 | ||
AHR(0.75) | 0.2337 | 0.0405 | 0.0043 | 0.1454 | 0.0019 | 0.1390 | ||
CAHR | 0.1006 | 0.0107 | 0.0014 | 0.0721 | 0.0020 | 0.0743 | ||
WCAHR | 0.1005 | 0.0105 | 0.0014 | 0.0709 | 0.0019 | 0.0736 | ||
200 | OLS | 0.2929 | 0.0241 | 0.0037 | 0.1127 | 0.0012 | 0.1065 | |
LAD | 0.2452 | 0.0137 | 0.0001 | 0.0844 | 0.0007 | 0.0813 | ||
ESL | 0.3665 | 0.0387 | 0.0031 | 0.1412 | 0.0023 | 0.1368 | ||
H-ESL | 0.3377 | 0.0305 | 0.0009 | 0.1244 | 0.0028 | 0.1225 | ||
AHR(0.25) | 0.2260 | 0.0086 | 0.0012 | 0.0652 | 0.0022 | 0.0655 | ||
Huber | 0.1998 | 0.0098 | 0.0011 | 0.0698 | 0.0007 | 0.0702 | ||
AHR(0.75) | 0.2314 | 0.0172 | 0.0038 | 0.0949 | 0.0001 | 0.0903 | ||
CAHR | 0.2122 | 0.0099 | 0.0007 | 0.0717 | 0.0010 | 0.0691 | ||
WCAHR | 0.1884 | 0.0086 | 0.0002 | 0.0659 | 0.0017 | 0.0652 | ||
400 | OLS | 0.0994 | 0.0122 | 0.0024 | 0.0794 | 0.0052 | 0.0769 | |
LAD | 0.0718 | 0.0065 | 0.0004 | 0.0569 | 0.0001 | 0.0568 | ||
ESL | 0.1372 | 0.0193 | 0.0012 | 0.1003 | 0.0031 | 0.0962 | ||
H-ESL | 0.1022 | 0.0139 | 0.0043 | 0.0832 | 0.0056 | 0.0832 | ||
AHR(0.25) | 0.0796 | 0.0037 | 0.0028 | 0.0418 | 0.0010 | 0.0437 | ||
Huber | 0.0688 | 0.0045 | 0.0005 | 0.0468 | 0.0003 | 0.0483 | ||
AHR(0.75) | 0.0912 | 0.0082 | 0.0010 | 0.0627 | 0.0019 | 0.0652 | ||
CAHR | 0.0712 | 0.0043 | 0.0007 | 0.0454 | 0.0009 | 0.0471 | ||
WCAHR | 0.0567 | 0.0035 | 0.0009 | 0.0418 | 0.0018 | 0.0424 | ||
200 | OLS | 0.4781 | 0.0695 | 0.0047 | 0.1815 | 0.0185 | 0.1902 | |
LAD | 0.2461 | 0.0215 | 0.0057 | 0.1052 | 0.0094 | 0.1015 | ||
ESL | 0.3793 | 0.0451 | 0.0023 | 0.1538 | 0.0062 | 0.1462 | ||
H-ESL | 0.3682 | 0.0443 | 0.0059 | 0.1533 | 0.0078 | 0.1437 | ||
AHR(0.25) | 0.2541 | 0.0203 | 0.0014 | 0.0987 | 0.0039 | 0.1025 | ||
Huber | 0.2829 | 0.0284 | 0.0082 | 0.1204 | 0.0013 | 0.1175 | ||
AHR(0.75) | 1.8541 | 0.3745 | 0.0128 | 0.4566 | 0.0243 | 0.4065 | ||
CAHR | 0.3606 | 0.0286 | 0.0037 | 0.1188 | 0.0001 | 0.1202 | ||
WCAHR | 0.2205 | 0.0169 | 0.0018 | 0.0920 | 0.0089 | 0.0915 | ||
400 | OLS | 0.2310 | 0.0325 | 0.0021 | 0.1296 | 0.0008 | 0.1252 | |
LAD | 0.1004 | 0.0109 | 0.0006 | 0.0742 | 0.0001 | 0.0735 | ||
ESL | 0.1563 | 0.0212 | 0.0053 | 0.1002 | 0.0027 | 0.1054 | ||
H-ESL | 0.1516 | 0.0178 | 0.0045 | 0.0917 | 0.0011 | 0.0966 | ||
AHR(0.25) | 0.1000 | 0.0088 | 0.0028 | 0.0671 | 0.0004 | 0.0659 | ||
Huber | 0.1108 | 0.0116 | 0.0019 | 0.0781 | 0.0021 | 0.0743 | ||
AHR(0.75) | 1.5565 | 0.3644 | 0.0416 | 0.4269 | 0.0216 | 0.4242 | ||
CAHR | 0.1496 | 0.0153 | 0.0016 | 0.0873 | 0.0007 | 0.0874 | ||
WCAHR | 0.0838 | 0.0076 | 0.0015 | 0.0616 | 0.0000 | 0.0618 | ||
200 | OLS | 0.8806 | 0.1464 | 0.0134 | 0.2675 | 0.0016 | 0.2732 | |
LAD | 0.3783 | 0.0358 | 0.0005 | 0.1320 | 0.0013 | 0.1355 | ||
ESL | 0.3719 | 0.0363 | 0.0025 | 0.1331 | 0.0044 | 0.1361 | ||
H-ESL | 0.3101 | 0.0280 | 0.0018 | 0.1175 | 0.0028 | 0.1189 | ||
AHR(0.25) | 0.3297 | 0.1148 | 0.0120 | 0.2346 | 0.0105 | 0.2439 | ||
Huber | 0.3685 | 0.0499 | 0.0042 | 0.1613 | 0.0071 | 0.1543 | ||
AHR(0.75) | 0.7037 | 0.1060 | 0.0078 | 0.2321 | 0.0033 | 0.2281 | ||
CAHR | 0.7857 | 0.1035 | 0.0031 | 0.2292 | 0.0035 | 0.2257 | ||
WCAHR | 0.3252 | 0.0340 | 0.0046 | 0.1289 | 0.0033 | 0.1316 | ||
400 | OLS | 0.3822 | 0.0715 | 0.0063 | 0.1887 | 0.0099 | 0.1892 | |
LAD | 0.1307 | 0.0190 | 0.0055 | 0.0983 | 0.0060 | 0.0965 | ||
ELS | 0.1268 | 0.0180 | 0.0032 | 0.0963 | 0.0043 | 0.0932 | ||
H-ESL | 0.1032 | 0.0129 | 0.0048 | 0.0807 | 0.0067 | 0.0793 | ||
AHR(0.25) | 0.1491 | 0.0604 | 0.0052 | 0.1731 | 0.0055 | 0.1742 | ||
Huber | 0.1332 | 0.0156 | 0.0007 | 0.0880 | 0.0033 | 0.0887 | ||
AHR(0.75) | 0.3391 | 0.0505 | 0.0049 | 0.1597 | 0.0040 | 0.1581 | ||
CAHR | 0.3435 | 0.0419 | -0.0030 | 0.1442 | 0.0074 | 0.1449 | ||
WCAHR | 0.1127 | 0.0157 | 0.0055 | 0.0894 | 0.0077 | 0.0872 | ||
200 | OLS | 0.4317 | 0.0560 | 0.0001 | 0.1690 | 0.0018 | 0.1657 | |
LAD | 0.8634 | 0.1201 | 0.0040 | 0.2438 | 0.0008 | 0.2463 | ||
ESL | 2.2921 | 0.4163 | 0.0148 | 0.4541 | 0.0002 | 0.4582 | ||
H-ESL | 0.4417 | 0.0558 | 0.0004 | 0.1687 | 0.0017 | 0.1653 | ||
AHR(0.25) | 0.9240 | 0.3169 | 0.0258 | 0.3973 | 0.0352 | 0.3964 | ||
Huber | 0.5694 | 0.0776 | 0.0056 | 0.2018 | 0.0129 | 0.1914 | ||
AHR(0.75) | 1.8171 | 0.3150 | 0.0110 | 0.4044 | 0.0107 | 0.3889 | ||
CAHR | 0.5191 | 0.0546 | 0.0075 | 0.1652 | 0.0116 | 0.1647 | ||
WCAHR | 0.4215 | 0.0552 | 0.0016 | 0.1679 | 0.0016 | 0.1642 | ||
400 | OLS | 0.1861 | 0.0296 | 0.0032 | 0.1170 | 0.0017 | 0.1262 | |
LAD | 0.4069 | 0.0670 | 0.0068 | 0.1765 | 0.0061 | 0.1891 | ||
ESL | 1.5317 | 0.2511 | 0.0185 | 0.3481 | 0.0033 | 0.3600 | ||
H-ESL | 0.1860 | 0.0298 | 0.0032 | 0.1175 | 0.0021 | 0.1265 | ||
AHR(0.25) | 0.4420 | 0.1620 | 0.0081 | 0.2835 | 0.0025 | 0.2855 | ||
Huber | 0.2369 | 0.0454 | 0.0023 | 0.1483 | 0.0082 | 0.1529 | ||
AHR(0.75) | 0.8504 | 0.1523 | 0.0142 | 0.2774 | 0.0021 | 0.2742 | ||
CAHR | 0.2047 | 0.0296 | 0.0025 | 0.1195 | 0.0014 | 0.1239 | ||
WCAHR | 0.1825 | 0.0291 | 0.0033 | 0.1162 | 0.0019 | 0.1249 |
Table 2 presents the results in the more challenged heteroscedastic case, which violates the condition in this paper. The proposed WCAHR estimator outperforms the other estimators considered for the normal, skewed normal and skewed error distributions, and is comparable to the corresponding H-ESL estimator for the mixture of normal distribution and bimodal distribution. This further illustrates that the proposed WCAHR estimator may be more applicable. Although the simulation results show the appealing performance for the considered heteroscedastic errors, general theoretical results are still challenging, and more conditions on the conditional moments of may be helpful.
Errors | Method | MISE | MSE() | |||||
Bias | Sd | Bias | Sd | |||||
200 | OLS | 0.2560 | 0.0237 | 0.0049 | 0.1105 | 0.0052 | 0.1069 | |
LAD | 0.2988 | 0.0300 | 0.0054 | 0.1228 | 0.0009 | 0.1222 | ||
ELS | 0.2990 | 0.0316 | 0.0041 | 0.1272 | 0.0021 | 0.1240 | ||
H-ESL | 0.2655 | 0.0258 | 0.0060 | 0.1163 | 0.0052 | 0.1104 | ||
AHR(0.25) | 0.2988 | 0.1065 | 0.0860 | 0.2178 | 0.0965 | 0.2058 | ||
Huber | 0.2531 | 0.0234 | 0.0050 | 0.1099 | 0.0045 | 0.1062 | ||
AHR(0.75) | 0.5960 | 0.0911 | 0.0958 | 0.1880 | 0.0836 | 0.1990 | ||
CAHR | 0.2562 | 0.0236 | 0.0055 | 0.1099 | 0.0051 | 0.1070 | ||
WCAHR | 0.2519 | 0.0227 | 0.0051 | 0.1081 | 0.0045 | 0.1047 | ||
400 | OLS | 0.1056 | 0.0119 | 0.0029 | 0.0767 | 0.0002 | 0.0777 | |
LAD | 0.1269 | 0.0153 | 0.0027 | 0.0865 | 0.0008 | 0.0882 | ||
ELS | 0.1257 | 0.0157 | 0.0026 | 0.0884 | 0.0019 | 0.0888 | ||
H-ESL | 0.1061 | 0.0116 | 0.0036 | 0.0760 | 0.0018 | 0.0762 | ||
AHR(0.25) | 0.1269 | 0.0588 | 0.1020 | 0.1418 | 0.0889 | 0.1427 | ||
Huber | 0.1060 | 0.0117 | 0.0032 | 0.0764 | 0.0001 | 0.0766 | ||
AHR(0.75) | 0.2695 | 0.0581 | 0.0990 | 0.1428 | 0.0859 | 0.1434 | ||
CAHR | 0.1064 | 0.0120 | 0.0023 | 0.0769 | 0.0005 | 0.0777 | ||
WCAHR | 0.1046 | 0.0115 | 0.0030 | 0.0754 | 0.0002 | 0.0762 | ||
200 | OLS | 0.3164 | 0.0357 | 0.0862 | 0.1070 | 0.0748 | 0.1057 | |
LAD | 0.2417 | 0.0209 | 0.0695 | 0.0789 | 0.0586 | 0.0803 | ||
ELS | 0.3578 | 0.0306 | 0.0140 | 0.1234 | 0.0119 | 0.1228 | ||
H-ESL | 0.3390 | 0.0316 | 0.0308 | 0.1211 | 0.0304 | 0.1228 | ||
AHR(0.25) | 0.2417 | 0.0139 | 0.0555 | 0.0651 | 0.0485 | 0.0650 | ||
Huber | 0.2344 | 0.0209 | 0.0780 | 0.0711 | 0.0684 | 0.0713 | ||
AHR(0.75) | 0.2809 | 0.0427 | 0.1122 | 0.1026 | 0.0969 | 0.1010 | ||
CAHR | 0.2454 | 0.0211 | 0.0774 | 0.0725 | 0.0684 | 0.0718 | ||
WCAHR | 0.2155 | 0.0174 | 0.0727 | 0.0644 | 0.0623 | 0.0642 | ||
400 | OLS | 0.1180 | 0.0242 | 0.0859 | 0.0754 | 0.0776 | 0.0718 | |
LAD | 0.0776 | 0.0149 | 0.0683 | 0.0573 | 0.0622 | 0.0553 | ||
ELS | 0.1267 | 0.0143 | 0.0180 | 0.0841 | 0.0001 | 0.0833 | ||
H-ESL | 0.1199 | 0.0179 | 0.0527 | 0.0831 | 0.0393 | 0.0819 | ||
AHR(0.25) | 0.0776 | 0.0096 | 0.0532 | 0.0478 | 0.0493 | 0.0453 | ||
Huber | 0.0763 | 0.0163 | 0.0753 | 0.0511 | 0.0740 | 0.0500 | ||
AHR(0.75) | 0.1060 | 0.0330 | 0.1043 | 0.0689 | 0.1117 | 0.0700 | ||
CAHR | 0.0813 | 0.0158 | 0.0732 | 0.0491 | 0.0755 | 0.0483 | ||
WCAHR | 0.0672 | 0.0130 | 0.0678 | 0.0451 | 0.0668 | 0.0441 | ||
200 | OLS | 0.5695 | 0.0947 | 0.1071 | 0.1861 | 0.1158 | 0.1876 | |
LAD | 0.3044 | 0.0287 | 0.0704 | 0.0970 | 0.0738 | 0.0941 | ||
ELS | 0.4205 | 0.0400 | 0.0035 | 0.1404 | 0.0162 | 0.1415 | ||
H-ESL | 0.3927 | 0.0405 | 0.0125 | 0.1409 | 0.0038 | 0.1431 | ||
AHR(0.25) | 0.3044 | 0.0219 | 0.0477 | 0.0944 | 0.0466 | 0.0925 | ||
Huber | 0.3450 | 0.0355 | 0.0764 | 0.1077 | 0.0784 | 0.1093 | ||
AHR(0.75) | 2.6347 | 0.5711 | 0.1826 | 0.5139 | 0.1919 | 0.4867 | ||
CAHR | 0.4548 | 0.0423 | 0.0776 | 0.1227 | 0.0826 | 0.1200 | ||
WCAHR | 0.2863 | 0.0246 | 0.0667 | 0.0868 | 0.0703 | 0.0875 | ||
400 | OLS | 0.2663 | 0.0577 | 0.1027 | 0.1286 | 0.1196 | 0.1278 | |
LAD | 0.1092 | 0.0199 | 0.0732 | 0.0694 | 0.0738 | 0.0657 | ||
ELS | 0.1423 | 0.0190 | 0.0083 | 0.0973 | 0.0090 | 0.0968 | ||
H-ESL | 0.1429 | 0.0202 | 0.0184 | 0.0971 | 0.0177 | 0.1003 | ||
AHR(0.25) | 0.1092 | 0.0148 | 0.0456 | 0.0725 | 0.0507 | 0.0698 | ||
Huber | 0.1447 | 0.0237 | 0.0777 | 0.0762 | 0.0784 | 0.0755 | ||
AHR(0.75) | 2.4856 | 0.6166 | 0.2100 | 0.5037 | 0.1899 | 0.5317 | ||
CAHR | 0.1858 | 0.0264 | 0.0726 | 0.0833 | 0.0831 | 0.0852 | ||
WCAHR | 0.0932 | 0.0160 | 0.0645 | 0.0596 | 0.0694 | 0.0592 | ||
200 | OLS | 0.9780 | 0.1653 | 0.0191 | 0.2838 | 0.0090 | 0.2904 | |
LAD | 0.3672 | 0.0409 | 0.0111 | 0.1436 | 0.0065 | 0.1420 | ||
ELS | 0.3644 | 0.0390 | 0.0092 | 0.1407 | 0.0091 | 0.1381 | ||
H-ESL | 0.3186 | 0.0321 | 0.0072 | 0.1260 | 0.0047 | 0.1271 | ||
AHR(0.25) | 0.3672 | 0.1579 | 0.1021 | 0.2647 | 0.0797 | 0.2665 | ||
Huber | 0.3700 | 0.0407 | 0.0134 | 0.1412 | 0.0106 | 0.1431 | ||
AHR(0.75) | 0.7890 | 0.1350 | 0.0802 | 0.2419 | 0.0878 | 0.2498 | ||
CAHR | 0.8570 | 0.0989 | 0.0114 | 0.2229 | 0.0077 | 0.2214 | ||
WCAHR | 0.3324 | 0.0352 | 0.0109 | 0.1326 | 0.0071 | 0.1322 | ||
400 | OLS | 0.4215 | 0.0781 | 0.0126 | 0.2002 | 0.0081 | 0.1944 | |
LAD | 0.1244 | 0.0189 | 0.0000 | 0.0980 | 0.0028 | 0.0964 | ||
ELS | 0.1247 | 0.0173 | 0.0004 | 0.0940 | 0.0028 | 0.0921 | ||
H-ESL | 0.1073 | 0.0131 | 0.0010 | 0.0808 | 0.0019 | 0.0808 | ||
AHR(0.25) | 0.1244 | 0.0759 | 0.0849 | 0.1701 | 0.0953 | 0.1752 | ||
Huber | 0.1222 | 0.0147 | 0.0054 | 0.0861 | 0.0011 | 0.0854 | ||
AHR(0.75) | 0.3546 | 0.0735 | 0.1014 | 0.1642 | 0.0908 | 0.1673 | ||
CAHR | 0.3496 | 0.0531 | 0.0116 | 0.1647 | 0.0069 | 0.1604 | ||
WCAHR | 0.1114 | 0.0148 | 0.0038 | 0.0860 | 0.0001 | 0.0857 | ||
200 | OLS | 0.4259 | 0.0604 | 0.0118 | 0.1733 | 0.0020 | 0.1738 | |
LAD | 0.7261 | 0.1323 | 0.0248 | 0.2573 | 0.0096 | 0.2558 | ||
ELS | 1.9087 | 0.3505 | 0.0283 | 0.4120 | 0.0078 | 0.4241 | ||
H-ESL | 0.4337 | 0.0614 | 0.0118 | 0.1758 | 0.0031 | 0.1743 | ||
AHR(0.25) | 0.7261 | 0.5567 | 0.1960 | 0.5100 | 0.1893 | 0.4716 | ||
Huber | 0.4839 | 0.0763 | 0.0180 | 0.1974 | 0.0064 | 0.1924 | ||
AHR(0.75) | 2.4130 | 0.4776 | 0.1823 | 0.4534 | 0.1885 | 0.4509 | ||
CAHR | 0.6745 | 0.1187 | 0.0223 | 0.2449 | 0.0087 | 0.2411 | ||
WCAHR | 0.4361 | 0.0642 | 0.0096 | 0.1797 | 0.0068 | 0.1783 | ||
400 | OLS | 0.1934 | 0.0295 | 0.0087 | 0.1251 | 0.0113 | 0.1169 | |
LAD | 0.3626 | 0.0578 | 0.0145 | 0.1715 | 0.0169 | 0.1670 | ||
ELS | 1.0701 | 0.1718 | 0.0200 | 0.2893 | 0.0187 | 0.2955 | ||
H-ESL | 0.1948 | 0.0299 | 0.0098 | 0.1256 | 0.0113 | 0.1178 | ||
AHR(0.25) | 0.3626 | 0.3363 | 0.2023 | 0.3440 | 0.2239 | 0.3562 | ||
Huber | 0.2316 | 0.0383 | 0.0093 | 0.1438 | 0.0130 | 0.1320 | ||
AHR(0.75) | 1.2586 | 0.3302 | 0.2280 | 0.3504 | 0.1848 | 0.3484 | ||
CAHR | 0.3292 | 0.0506 | 0.0163 | 0.1645 | 0.0174 | 0.1516 | ||
WCAHR | 0.2058 | 0.0313 | 0.0119 | 0.1298 | 0.0092 | 0.1193 |
In order to detect the effect of the level choice to the performance of the WCAHR estimators, especially for the skewed error distributions, we also change in the simulation the number of levels over (0, 0.5) given the total level number . Specifically, for the given and different values of , we set and Table 3 presents the estimation results. We find from the results that the choice of the levels does not destroy the performance of the WCAHR estimators, although less number of levels over (0, 0.5) leads to slightly larger MSE and MISE for the positively skewed error distributions. In addition, the MISEs and MSEs decrease as increases, and stabilize eventually. This may motivate that one can take more levels appropriately over (0, 0.5) in dealing with the positively skewed error distributions.
Errors | MISE | MSE() | ||||||
Bias | Sd | Bias | Sd | |||||
200 | 4 | 0.2321 | 0.0088 | 0.0034 | 0.0663 | 0.0044 | 0.0665 | |
6 | 0.2295 | 0.0084 | 0.0036 | 0.0646 | 0.0042 | 0.0645 | ||
8 | 0.2279 | 0.0081 | 0.0038 | 0.0635 | 0.0042 | 0.0633 | ||
10 | 0.2269 | 0.0079 | 0.0040 | 0.0629 | 0.0041 | 0.0626 | ||
12 | 0.2264 | 0.0078 | 0.0041 | 0.0627 | 0.0041 | 0.0621 | ||
14 | 0.2262 | 0.0078 | 0.0043 | 0.0627 | 0.0041 | 0.0620 | ||
400 | 4 | 0.0626 | 0.0039 | 0.0071 | 0.0450 | -0.0054 | 0.0425 | |
6 | 0.0611 | 0.0037 | 0.0068 | 0.0440 | 0.0050 | 0.0415 | ||
8 | 0.0601 | 0.0036 | 0.0065 | 0.0434 | 0.0046 | 0.0410 | ||
10 | 0.0595 | 0.0036 | 0.0063 | 0.0432 | 0.0043 | 0.0409 | ||
12 | 0.0591 | 0.0036 | 0.0060 | 0.0432 | 0.0039 | 0.0409 | ||
14 | 0.0589 | 0.0036 | 0.0059 | 0.0433 | 0.0038 | 0.0411 | ||
200 | 4 | 0.2445 | 0.0178 | 0.0027 | 0.0961 | 0.0023 | 0.0927 | |
6 | 0.2388 | 0.0169 | 0.0025 | 0.0938 | 0.0024 | 0.0900 | ||
8 | 0.2349 | 0.0163 | 0.0025 | 0.0923 | 0.0023 | 0.0880 | ||
10 | 0.2321 | 0.0158 | 0.0025 | 0.0911 | 0.0023 | 0.0866 | ||
12 | 0.2301 | 0.0155 | 0.0024 | 0.0903 | 0.0022 | 0.0856 | ||
14 | 0.2287 | 0.0153 | 0.0023 | 0.0898 | 0.0022 | 0.0849 | ||
400 | 4 | 0.0925 | 0.0090 | 0.0079 | 0.0678 | 0.0076 | 0.0652 | |
6 | 0.0899 | 0.0085 | 0.0078 | 0.0659 | 0.0076 | 0.0637 | ||
8 | 0.0880 | 0.0082 | 0.0076 | 0.0646 | 0.0076 | 0.0626 | ||
10 | 0.0867 | 0.0080 | 0.0076 | 0.0636 | 0.0076 | 0.0619 | ||
12 | 0.0857 | 0.0078 | 0.0075 | 0.0629 | 0.0077 | 0.0615 | ||
14 | 0.0850 | 0.0077 | 0.0074 | 0.0623 | 0.0077 | 0.0611 |
In this section, we use the proposed estimation methods to the Electricity data and the Tecator data set, and the competing methods mentioned in Section 3. In the applications, all the observations are centralized before the regression analysis.
The data set consists of the daily average hourly electricity spot prices of the German electricity market (), the hourly values of Germany's wind power infeed (), the precipitation height () and the sunshine duration (). Here we only consider the working days span from January 1, 2006 to September 30, 2008. The hourly values of Germany's wind power infeed curves are shown in the left panel of Figure 3. The data set can be obtained from the online supplements of Liebl [23]. Now we adopt the following partial functional linear regression model to fit the data:
(4.1) |
Firstly, the OLS method is applied to fit model (4.1). Then Shapiro-Wilk test is applied to test the normality of the residuals and the -value is less than . In addition, we also give the estimated density of the residuals and the residual diagnostic plot (see Figure 1). Both the test and plot clearly indicate that follows a skewed distribution with outliers. Notice that the density of the residuals is similar to the error distribution discussed in Section 3, and the simulation results illustrate that the proposed method can be applied and provide reliable inference for this kind of data.
To evaluate the predictions obtained with different estimation methods, we randomly divide data set into a training sample of size 478 subjects and a testing sample with the remaining 160 subjects (indexed by ). The data are split for times, respectively. We use the median quadratic error of prediction () defined below as the criterion to evaluate the performances:
The left 3 columns of Table 4 present the MEDQEPs of different methods mentioned above. According to the results of calculation, the WCAHR method is uniformly superior to the other estimators.
Electricity prices | Tecator | |||||
Methods | N=100 | N=200 | N=400 | N=100 | N=200 | N=400 |
OLS | 0.4269 | 0.4132 | 0.4153 | 2.782410 | 2.718210 | 2.725710 |
LAD | 0.4094 | 0.4005 | 0.4068 | 2.938810 | 2.861110 | 2.825310 |
ESL | 0.5751 | 0.5626 | 0.5578 | 2.726810 | 2.670110 | 2.614210 |
H-ESL | 0.4104 | 0.4026 | 0.4064 | 2.739510 | 2.733610 | 2.647610 |
AHR(0.25) | 0.4052 | 0.3985 | 0.4032 | 2.905610 | 2.765810 | 2.775310 |
Huber | 0.4077 | 0.4027 | 0.4074 | 2.763610 | 2.649110 | 2.627810 |
AHR(0.75) | 0.4296 | 0.4198 | 0.4280 | 2.740910 | 2.649410 | 2.606310 |
CAHR | 0.4103 | 0.4019 | 0.4089 | 2.844210 | 2.810610 | 2.745710 |
WCAHR | 0.4030 | 0.3967 | 0.4008 | 2.723610 | 2.615210 | 2.579910 |
Table 5 (the first 2 columns) presents the estimates of the parametric part by the estimation methods based on the whole data set. According to the results, both the precipitation height and the sunshine duration have negative effects on the daily average hourly electricity spot prices. In addition, Figure 4(a) plots the estimated slope function obtained by the WCAHR method, the estimates for obtained by other methods mentioned above exhibit similar patterns and thus omitted here. From the figure, we can see the prices have a larger (in the absolute value) linkage with the wind power infeed in the daytime, which reflects the economic phenomena of price sensitivity, and more specifically, the market is active during the daytime and thus there is more correlation between the prices and the wind power infeed in the daytime. Secondly, the Germany's wind power infeed has a negative effect on the daily average hourly electricity spot prices, which reflects supply-demand balance, that is, more wind infeed creates the oversupply of electricity and thus reduces the price.
Electricity prices | Tecator | |||
OLS | 0.5983 | 0.4672 | 1.1056 | 0.6894 |
LAD | 0.7095 | 0.9438 | 1.0828 | 0.7611 |
ESL | 0.5125 | 0.4629 | 1.0894 | 0.7455 |
H-ESL | 0.6007 | 0.7212 | 1.0983 | 0.7367 |
AHR(0.25) | 0.5618 | 0.4725 | 1.1122 | 0.7026 |
Huber | 0.5799 | 0.4416 | 1.0981 | 0.7235 |
AHR(0.75) | 0.5812 | 0.4302 | 1.0854 | 0.7576 |
CAHR | 0.5394 | 0.4582 | 1.0990 | 0.7274 |
WCAHR | 0.5924 | 0.6182 | 1.0964 | 0.7270 |
The Tecator data set consists of 215 meat samples. For each sample, moisture, fat and protein are recorded in percent, and a 100-channel spectrum of absorbances is measured by the spectrometer. The data set is available from the R package fda.usc (see [24]). The right panel of Figure 3 shows the spectral trajectories. In this paper, the objective is to investigate the effects of the spectral trajectories , water content and protein content on the fat content by fitting the following model:
(4.2) |
The density of the residuals and the residual diagnostic plot in Figure 2 illustrate the error follows a skewed distribution with outliers. Similarly, to assess the prediction accuracy, the 215 meat samples are randomized into training set with 180 subjects and testing set with 35 subjects. We also randomly split the data set for times and use MEDQEP as criterion to evaluate the finite sample performances of different estimation procedures. The comparison results are shown in the right 3 columns of Table 2, from which we know the proposed method performs better than the competing estimation procedures in view of prediction accuracy.
The estimated coefficients , using various methods based on the whole data set are also shown in the last 2 columns of Table 3. Both the protein content and water content have negative effects on the fat content. Next, the right panel of Figure 4 demonstrates the estimated slope function curves based on the WCAHR method. It is obvious that the spectrum curve of absorbance has negative impact on the quantity of fatty. In addition, the estimated slope functions by other methods mentioned above exhibit similar patterns and thus omitted here.
In this paper, we study the WCAHR estimation in the partial functional linear regression model. We use the functional principal component basis to approximate the functions, and obtain the estimators of the unknown parameter vector and the slope function through minimizing the weighted asymmetric Huber loss function. The asymptotic normality of the estimated parameter vector and the convergence rate of the estimated slope function are presented.
The proposed approach is designed for automatically reflecting distributional features as well as bounding outliers effectively without requiring prior information of the data. Simulation results show that the proposed method is almost as efficient as OLS when the error follows a normal distribution, while keeps robust to outliers and still works well when the error follows skewed or bimodal distributions. That is to say, the method is adaptive to the distribution of the error in the regression model. The analyses of two examples further illustrate that the utility of the proposed methods in modelling and forecasting.
The novelty of the method is that it focuses on the extraction of major features as well as shielding the estimator from outliers. The proposed WCAHR estimation procedure can be extended to more general situations, including dependent functional data, sparse modeling, partially observed functional data, and high dimension setting. In addition, we still need to try objective way to select .
Authors are highly grateful to anonymous referees and editor in chief for their valuable comments and suggestions for improving this paper. This research was supported by National Natural Science Foundation of China (Grant No. 11771032 and No. 11971045), Natural Science Foundation of Beijing (Grant No. 1202001), and Natural Science Foundation of Shanxi Province, China (Grant No. 20210302124530).
The authors declare that they have no competing interests.
We just prove Theorem 2. The Theorem 1 is a special case of Theorem 2.
Proof of Theorem 2:
Let , , , , , , , , , , , , , , , .
Next, we will show that, for any given , there exists a sufficiently large constant , such that
(A.1) |
This means that there is a local minimizer , and in the ball such that , , with a probability at least .
First, by (see, [4]), one has
For , using Conditions C1, C2, and the Hölder inequality, one can obtain
As for , due to one has To sum up, we have .
Now consider . Due to
by Conditions C1, C2, C4, and the Hölder inequality, we obtain
In addition, noting that
together with the above inequality, one has
(A.2) |
Recall that and denote . Then can be transformed into
where
Consider . According to (A.2), we have
(A.3) |
The proof of Theorem 3.1 in [6] indicates that with , which leads to
(A.4) |
Observe that then by Conditions C1–C3, C5, and . Hence,
(A.5) |
Similarly, Then, together with formulas (A.3)–(A.5), we have
As for , due to the continuity of , we have
then
from which we get
For the term , it is easy to show that
and
Combining with the equation (A.4), we can obtain
From the results about and , it is easy to obtain that is dominated by the positive quadratic term . Hence, Eq (A.1) is established, and there exists local minimizer such that
(A.6) |
Now we consider the convergence rate of . Since
and based on the basic Condition C2, we have
(A.7) |
Note that
Based on the Condition C2, Eq (A.7) and the orthogonality of , as well as , we can obtain
(A.8) |
These lead to
Next, we turn to the asymptotic normality of . Note that attains the minimal value at with probability tending to 1 as tends to infinity. Then, we have the following score equations
(A.9) |
(A.10) |
Further, we can write (A.9) as , with
By simple calculations, we have
Through calculating the mean and variance directly, we can obtain . Then, Eq (A.9) can be written as
(A.11) |
Similarly, Eq (A.10) can be changed into
Now let Then, above equation allows . Substituting it into (A.11), we obtain
Noting that
then, it is easy to conclude that
Note that
Then we have
(A.12) |
It is easy to see that . And based on Lemma 1 in [8] and the Condition we can obtain .
Through some simple calculations, one has
(A.13) |
where with and . Then, according to Eqs (A.12) and (A.13), the Slutsky's theorem, and the properties of multivariate normal distributions, we can obtain
Lastly, we prove the third conclusion of Theorem 2. By the definition of , we have
Firstly, according to the previous proof process, we have . As for , based on triangle inequality and inequality,
By Eq (A.8), by. As for , it is easy to know , then Next, by (A.8), we have . By (A.6), we know and . Then, we have
The proof of Theorem 2 is complete.
[1] |
X. Pei, R. Zhou, Current status and future of Chinese medicine diagnosis and treatment of breast cancer (in Chinese), Beijing Tradit. Chin. Med., 42 (2023), 704–707. https://doi.org/10.16025/j.1674-1307.2023.07.001 doi: 10.16025/j.1674-1307.2023.07.001
![]() |
[2] | E. Mahoro, M. A. Akhloufi, Breast masses detection on mammograms using recent one-shot deep object detectors, in 2023 5th International Conference on Bio-engineering for Smart Technologies (BioSMART), IEEE, (2023), 1–4. https://doi.org/10.1109/BioSMART58455.2023.10162036 |
[3] | A. Intasam, Y. Promworn, A. Juhong, S. Thanasitthichai, S. Khwayotha, T. Jiranantanakorn, et al., Optimizing the hyperparameter tuning of yolov5 for breast cancer detection, in 2023 9th International Conference on Engineering, Applied Sciences, and Technology (ICEAST), IEEE, (2023), 184–187. https://doi.org/10.1109/ICEAST58324.2023.10157611 |
[4] | X. Gao, B. Braden, S. Taylor, W. Pang, Towards real-time detection of squamous pre-cancers from oesophageal endoscopic videos, in 2019 18th IEEE International Conference On Machine Learning And Applications (ICMLA), IEEE, (2019), 1606–1612. https://doi.org/10.1109/ICMLA.2019.00264 |
[5] |
Y. Yang, J. Wang, Research on breast cancer pathological image classification method based on wavelet transform and YOLOv8, J. X-Ray Sci. Technol., 32 (2024), 677–687. https://doi.org/10.3233/XST-230296 doi: 10.3233/XST-230296
![]() |
[6] |
M. A. Al-Antari, S. Han, T. Kim, Evaluation of deep learning detection and classification towards computer-aided diagnosis of breast lesions in digital X-ray mammograms, Comput. Methods Programs Biomed., 196 (2020), 105584. https://doi.org/10.1016/j.cmpb.2020.105584 doi: 10.1016/j.cmpb.2020.105584
![]() |
[7] | M. A. Al-masni, M. A. Al-antari, J. M. Park, G. Gi, T. Y. Kim, P. Rivera, et al., Detection and classification of the breast abnormalities in digital mammograms via regional convolutional neural network, in 2017 39th Annual International Conference of the IEEE Engineering in Medicine and Biology Society (EMBC), IEEE, (2017), 1230–1233. https://doi.org/10.1109/EMBC.2017.8037053 |
[8] | R. K. Kassahun, M. Molinara, A. Bria, C. Marrocco, F Tortorella, Breast mass detection and classification using transfer learning on OPTIMAM dataset through RadImageNet weights, in International Conference on Image Analysis and Processing, Springer, (2024), 71–82. https://doi.org/10.1007/978-3-031-51026-7_7 |
[9] | F. Touazi, D. Gaceb, M. Chirane, S. Hrzallah, Two-stage approach for semantic image segmentation of breast cancer: Deep learning and mass detection in mammographic images, in 6th International Workshop on Informatics & Data-Driven Medicine (IDDM), CEUR Workshop Proceedings, (2023), 62–76. https://doi.org/10.1109/iciprob54042.2022.9798724 |
[10] | W. Ouyang, P. Luo, X. Zeng, S. Qiu, Y. Tian, H. Li, et al., Deepid-net: Multi-stage and deformable deep convolutional neural networks for object detection, preprint, arXiv: 1409.3505. |
[11] | C. Szegedy, W. Liu, Y. Jia, P. Sermanet, S. Reed, D. Anguelov, et al., Going deeper with convolutions, in 2015 IEEE Conference on Computer Vision and Pattern Recognition (CVPR), IEEE, (2015), 1–9. https://doi.org/10.1109/CVPR.2015.7298594 |
[12] | S. Cheng, G. Shang, L. Zhang, Handwritten digit recognition based on improved VGG16 network, in Tenth International Conference on Graphics and Image Processing (ICGIP 2018), (2019), 110693B. https://doi.org/10.1117/12.2524281 |
[13] | B. Zoph, Q. V. Le, Neural architecture search with reinforcement learning, preprint, arXiv: 1611.01578. |
[14] | D. Cai, X. Sun, N. Zhou, X. Han, J. Yao, Efficient mitosis detection in breast cancer histology images by RCNN, in 2019 IEEE 16th International Symposium on Biomedical Imaging (ISBI 2019), IEEE, (2019), 919–922. https://doi.org/10.1109/ISBI.2019.8759461 |
[15] |
S. Ren, K. He, R. Girshick, J. Sun, Faster R-CNN: Towards real-time object detection with region proposal networks, IEEE Trans. Pattern Anal. Mach. Intell., 39 (2016), 1137–1149. https://doi.org/10.1109/TPAMI.2016.2577031 doi: 10.1109/TPAMI.2016.2577031
![]() |
[16] |
T. Mahmood, M. Arsalan, M. Owais, M. B. Lee, K. R. Park, Artificial intelligence-based mitosis detection in breast cancer histopathology images using faster R-CNN and deep CNNs, J. Clin. Med., 9 (2020), 749. https://doi.org/10.3390/jcm9030749 doi: 10.3390/jcm9030749
![]() |
[17] | J. Redmon, S. Divvala, R. Girshick, A. Farhadi, You only look once: Unified, real-time object detection, preprint, arXiv: 1506.02640. |
[18] | S. R. Sindhu, J. George, S. Skaria, V. V. Varun, Using YOLO based deep learning network for real time detection and localization of lung nodules from low dose CT scans, in Medical Imaging 2018: Computer-Aided Diagnosis, SPIE, 10575 (2018), 347–355. https://doi.org/10.1117/12.2293699 |
[19] |
H. M. Ünver, E. Ayan, Skin lesion segmentation in dermoscopic images with combination of YOLO and grabcut algorithm, Diagnostics, 9 (2019), 72. https://doi.org/10.3390/diagnostics9030072 doi: 10.3390/diagnostics9030072
![]() |
[20] | J. Sobek, J. R. M. Inojosa, B. J. M. Inojosa, S. M. Rassoulinejad-Mousavi, G, M. Conte, F. Lopez-Jimenez, et al., MedYOLO: A medical image object detection framework, J. Digit. Imaging. Inform. Med., (2024), 1–9. https://doi.org/10.1007/s10278-024-01138-2 |
[21] | K. Han, Y. Wang, Q. Tian, J. Guo, C. Xu, C. Xu, Ghostnet: More features from cheap operations, in 2020 IEEE/CVF Conference on Computer Vision and Pattern Recognition (CVPR), IEEE, (2020), 1577–1586. https://doi.org/10.1109/CVPR42600.2020.00165 |
[22] | J. Suckling, J. Parker, D. Dance, S. Astley, I. Hutt, C. Boggis, et al., Mammographic Image Analysis Society (MIAS) database v1.21, Apollo-University of Cambridge Repository, 2015. https://doi.org/10.17863/CAM.105113 |
[23] |
M. Heath, K. Bowyer, D. Kopans, P. K. Jr, R. Moore, K. Chang, et al., Current status of the digital database for screening mammography, Digital Mammography, 13 (1998), 457–460. https://doi.org/10.1007/978-94-011-5318-8_75 doi: 10.1007/978-94-011-5318-8_75
![]() |
1. | Lijie Zhou, Liucang Wu, Bin Yang, Estimation and diagnostic for single-index partially functional linear regression model with -order autoregressive skew-normal errors, 2025, 10, 2473-6988, 7022, 10.3934/math.2025321 |
Errors | Method | MISE | MSE() | |||||
Bias | Sd | Bias | Sd | |||||
200 | OLS | 0.2690 | 0.0229 | 0.0048 | 0.1074 | 0.0096 | 0.1059 | |
LAD | 0.3294 | 0.0354 | 0.0099 | 0.1320 | 0.0115 | 0.1333 | ||
ESL | 0.3434 | 0.0394 | 0.0009 | 0.1405 | 0.0013 | 0.1403 | ||
H-ESL | 0.2824 | 0.0259 | 0.0055 | 0.1141 | 0.0059 | 0.1133 | ||
AHR(0.25) | 0.3294 | 0.0786 | 0.0077 | 0.2014 | 0.0105 | 0.1948 | ||
Huber | 0.2758 | 0.0234 | 0.0063 | 0.1090 | 0.0102 | 0.1067 | ||
AHR(0.75) | 0.5251 | 0.0754 | 0.0092 | 0.1894 | 0.0150 | 0.1981 | ||
CAHR | 0.2689 | 0.0233 | 0.0051 | 0.1095 | 0.0091 | 0.1060 | ||
WCAHR | 0.2693 | 0.0230 | 0.0055 | 0.1080 | 0.0101 | 0.1058 | ||
400 | OLS | 0.1004 | 0.0105 | 0.0011 | 0.0710 | 0.0015 | 0.0738 | |
LAD | 0.1304 | 0.0163 | 0.0027 | 0.0880 | 0.0045 | 0.0924 | ||
ESL | 0.1349 | 0.0175 | 0.0010 | 0.0918 | 0.0008 | 0.0955 | ||
H-ESL | 0.1031 | 0.0113 | 0.0011 | 0.0727 | 0.0010 | 0.0773 | ||
AHR(0.25) | 0.1304 | 0.0358 | 0.0048 | 0.1313 | 0.0013 | 0.1361 | ||
Huber | 0.1048 | 0.0107 | 0.0018 | 0.0720 | 0.0027 | 0.0742 | ||
AHR(0.75) | 0.2337 | 0.0405 | 0.0043 | 0.1454 | 0.0019 | 0.1390 | ||
CAHR | 0.1006 | 0.0107 | 0.0014 | 0.0721 | 0.0020 | 0.0743 | ||
WCAHR | 0.1005 | 0.0105 | 0.0014 | 0.0709 | 0.0019 | 0.0736 | ||
200 | OLS | 0.2929 | 0.0241 | 0.0037 | 0.1127 | 0.0012 | 0.1065 | |
LAD | 0.2452 | 0.0137 | 0.0001 | 0.0844 | 0.0007 | 0.0813 | ||
ESL | 0.3665 | 0.0387 | 0.0031 | 0.1412 | 0.0023 | 0.1368 | ||
H-ESL | 0.3377 | 0.0305 | 0.0009 | 0.1244 | 0.0028 | 0.1225 | ||
AHR(0.25) | 0.2260 | 0.0086 | 0.0012 | 0.0652 | 0.0022 | 0.0655 | ||
Huber | 0.1998 | 0.0098 | 0.0011 | 0.0698 | 0.0007 | 0.0702 | ||
AHR(0.75) | 0.2314 | 0.0172 | 0.0038 | 0.0949 | 0.0001 | 0.0903 | ||
CAHR | 0.2122 | 0.0099 | 0.0007 | 0.0717 | 0.0010 | 0.0691 | ||
WCAHR | 0.1884 | 0.0086 | 0.0002 | 0.0659 | 0.0017 | 0.0652 | ||
400 | OLS | 0.0994 | 0.0122 | 0.0024 | 0.0794 | 0.0052 | 0.0769 | |
LAD | 0.0718 | 0.0065 | 0.0004 | 0.0569 | 0.0001 | 0.0568 | ||
ESL | 0.1372 | 0.0193 | 0.0012 | 0.1003 | 0.0031 | 0.0962 | ||
H-ESL | 0.1022 | 0.0139 | 0.0043 | 0.0832 | 0.0056 | 0.0832 | ||
AHR(0.25) | 0.0796 | 0.0037 | 0.0028 | 0.0418 | 0.0010 | 0.0437 | ||
Huber | 0.0688 | 0.0045 | 0.0005 | 0.0468 | 0.0003 | 0.0483 | ||
AHR(0.75) | 0.0912 | 0.0082 | 0.0010 | 0.0627 | 0.0019 | 0.0652 | ||
CAHR | 0.0712 | 0.0043 | 0.0007 | 0.0454 | 0.0009 | 0.0471 | ||
WCAHR | 0.0567 | 0.0035 | 0.0009 | 0.0418 | 0.0018 | 0.0424 | ||
200 | OLS | 0.4781 | 0.0695 | 0.0047 | 0.1815 | 0.0185 | 0.1902 | |
LAD | 0.2461 | 0.0215 | 0.0057 | 0.1052 | 0.0094 | 0.1015 | ||
ESL | 0.3793 | 0.0451 | 0.0023 | 0.1538 | 0.0062 | 0.1462 | ||
H-ESL | 0.3682 | 0.0443 | 0.0059 | 0.1533 | 0.0078 | 0.1437 | ||
AHR(0.25) | 0.2541 | 0.0203 | 0.0014 | 0.0987 | 0.0039 | 0.1025 | ||
Huber | 0.2829 | 0.0284 | 0.0082 | 0.1204 | 0.0013 | 0.1175 | ||
AHR(0.75) | 1.8541 | 0.3745 | 0.0128 | 0.4566 | 0.0243 | 0.4065 | ||
CAHR | 0.3606 | 0.0286 | 0.0037 | 0.1188 | 0.0001 | 0.1202 | ||
WCAHR | 0.2205 | 0.0169 | 0.0018 | 0.0920 | 0.0089 | 0.0915 | ||
400 | OLS | 0.2310 | 0.0325 | 0.0021 | 0.1296 | 0.0008 | 0.1252 | |
LAD | 0.1004 | 0.0109 | 0.0006 | 0.0742 | 0.0001 | 0.0735 | ||
ESL | 0.1563 | 0.0212 | 0.0053 | 0.1002 | 0.0027 | 0.1054 | ||
H-ESL | 0.1516 | 0.0178 | 0.0045 | 0.0917 | 0.0011 | 0.0966 | ||
AHR(0.25) | 0.1000 | 0.0088 | 0.0028 | 0.0671 | 0.0004 | 0.0659 | ||
Huber | 0.1108 | 0.0116 | 0.0019 | 0.0781 | 0.0021 | 0.0743 | ||
AHR(0.75) | 1.5565 | 0.3644 | 0.0416 | 0.4269 | 0.0216 | 0.4242 | ||
CAHR | 0.1496 | 0.0153 | 0.0016 | 0.0873 | 0.0007 | 0.0874 | ||
WCAHR | 0.0838 | 0.0076 | 0.0015 | 0.0616 | 0.0000 | 0.0618 | ||
200 | OLS | 0.8806 | 0.1464 | 0.0134 | 0.2675 | 0.0016 | 0.2732 | |
LAD | 0.3783 | 0.0358 | 0.0005 | 0.1320 | 0.0013 | 0.1355 | ||
ESL | 0.3719 | 0.0363 | 0.0025 | 0.1331 | 0.0044 | 0.1361 | ||
H-ESL | 0.3101 | 0.0280 | 0.0018 | 0.1175 | 0.0028 | 0.1189 | ||
AHR(0.25) | 0.3297 | 0.1148 | 0.0120 | 0.2346 | 0.0105 | 0.2439 | ||
Huber | 0.3685 | 0.0499 | 0.0042 | 0.1613 | 0.0071 | 0.1543 | ||
AHR(0.75) | 0.7037 | 0.1060 | 0.0078 | 0.2321 | 0.0033 | 0.2281 | ||
CAHR | 0.7857 | 0.1035 | 0.0031 | 0.2292 | 0.0035 | 0.2257 | ||
WCAHR | 0.3252 | 0.0340 | 0.0046 | 0.1289 | 0.0033 | 0.1316 | ||
400 | OLS | 0.3822 | 0.0715 | 0.0063 | 0.1887 | 0.0099 | 0.1892 | |
LAD | 0.1307 | 0.0190 | 0.0055 | 0.0983 | 0.0060 | 0.0965 | ||
ELS | 0.1268 | 0.0180 | 0.0032 | 0.0963 | 0.0043 | 0.0932 | ||
H-ESL | 0.1032 | 0.0129 | 0.0048 | 0.0807 | 0.0067 | 0.0793 | ||
AHR(0.25) | 0.1491 | 0.0604 | 0.0052 | 0.1731 | 0.0055 | 0.1742 | ||
Huber | 0.1332 | 0.0156 | 0.0007 | 0.0880 | 0.0033 | 0.0887 | ||
AHR(0.75) | 0.3391 | 0.0505 | 0.0049 | 0.1597 | 0.0040 | 0.1581 | ||
CAHR | 0.3435 | 0.0419 | -0.0030 | 0.1442 | 0.0074 | 0.1449 | ||
WCAHR | 0.1127 | 0.0157 | 0.0055 | 0.0894 | 0.0077 | 0.0872 | ||
200 | OLS | 0.4317 | 0.0560 | 0.0001 | 0.1690 | 0.0018 | 0.1657 | |
LAD | 0.8634 | 0.1201 | 0.0040 | 0.2438 | 0.0008 | 0.2463 | ||
ESL | 2.2921 | 0.4163 | 0.0148 | 0.4541 | 0.0002 | 0.4582 | ||
H-ESL | 0.4417 | 0.0558 | 0.0004 | 0.1687 | 0.0017 | 0.1653 | ||
AHR(0.25) | 0.9240 | 0.3169 | 0.0258 | 0.3973 | 0.0352 | 0.3964 | ||
Huber | 0.5694 | 0.0776 | 0.0056 | 0.2018 | 0.0129 | 0.1914 | ||
AHR(0.75) | 1.8171 | 0.3150 | 0.0110 | 0.4044 | 0.0107 | 0.3889 | ||
CAHR | 0.5191 | 0.0546 | 0.0075 | 0.1652 | 0.0116 | 0.1647 | ||
WCAHR | 0.4215 | 0.0552 | 0.0016 | 0.1679 | 0.0016 | 0.1642 | ||
400 | OLS | 0.1861 | 0.0296 | 0.0032 | 0.1170 | 0.0017 | 0.1262 | |
LAD | 0.4069 | 0.0670 | 0.0068 | 0.1765 | 0.0061 | 0.1891 | ||
ESL | 1.5317 | 0.2511 | 0.0185 | 0.3481 | 0.0033 | 0.3600 | ||
H-ESL | 0.1860 | 0.0298 | 0.0032 | 0.1175 | 0.0021 | 0.1265 | ||
AHR(0.25) | 0.4420 | 0.1620 | 0.0081 | 0.2835 | 0.0025 | 0.2855 | ||
Huber | 0.2369 | 0.0454 | 0.0023 | 0.1483 | 0.0082 | 0.1529 | ||
AHR(0.75) | 0.8504 | 0.1523 | 0.0142 | 0.2774 | 0.0021 | 0.2742 | ||
CAHR | 0.2047 | 0.0296 | 0.0025 | 0.1195 | 0.0014 | 0.1239 | ||
WCAHR | 0.1825 | 0.0291 | 0.0033 | 0.1162 | 0.0019 | 0.1249 |
Errors | Method | MISE | MSE() | |||||
Bias | Sd | Bias | Sd | |||||
200 | OLS | 0.2560 | 0.0237 | 0.0049 | 0.1105 | 0.0052 | 0.1069 | |
LAD | 0.2988 | 0.0300 | 0.0054 | 0.1228 | 0.0009 | 0.1222 | ||
ELS | 0.2990 | 0.0316 | 0.0041 | 0.1272 | 0.0021 | 0.1240 | ||
H-ESL | 0.2655 | 0.0258 | 0.0060 | 0.1163 | 0.0052 | 0.1104 | ||
AHR(0.25) | 0.2988 | 0.1065 | 0.0860 | 0.2178 | 0.0965 | 0.2058 | ||
Huber | 0.2531 | 0.0234 | 0.0050 | 0.1099 | 0.0045 | 0.1062 | ||
AHR(0.75) | 0.5960 | 0.0911 | 0.0958 | 0.1880 | 0.0836 | 0.1990 | ||
CAHR | 0.2562 | 0.0236 | 0.0055 | 0.1099 | 0.0051 | 0.1070 | ||
WCAHR | 0.2519 | 0.0227 | 0.0051 | 0.1081 | 0.0045 | 0.1047 | ||
400 | OLS | 0.1056 | 0.0119 | 0.0029 | 0.0767 | 0.0002 | 0.0777 | |
LAD | 0.1269 | 0.0153 | 0.0027 | 0.0865 | 0.0008 | 0.0882 | ||
ELS | 0.1257 | 0.0157 | 0.0026 | 0.0884 | 0.0019 | 0.0888 | ||
H-ESL | 0.1061 | 0.0116 | 0.0036 | 0.0760 | 0.0018 | 0.0762 | ||
AHR(0.25) | 0.1269 | 0.0588 | 0.1020 | 0.1418 | 0.0889 | 0.1427 | ||
Huber | 0.1060 | 0.0117 | 0.0032 | 0.0764 | 0.0001 | 0.0766 | ||
AHR(0.75) | 0.2695 | 0.0581 | 0.0990 | 0.1428 | 0.0859 | 0.1434 | ||
CAHR | 0.1064 | 0.0120 | 0.0023 | 0.0769 | 0.0005 | 0.0777 | ||
WCAHR | 0.1046 | 0.0115 | 0.0030 | 0.0754 | 0.0002 | 0.0762 | ||
200 | OLS | 0.3164 | 0.0357 | 0.0862 | 0.1070 | 0.0748 | 0.1057 | |
LAD | 0.2417 | 0.0209 | 0.0695 | 0.0789 | 0.0586 | 0.0803 | ||
ELS | 0.3578 | 0.0306 | 0.0140 | 0.1234 | 0.0119 | 0.1228 | ||
H-ESL | 0.3390 | 0.0316 | 0.0308 | 0.1211 | 0.0304 | 0.1228 | ||
AHR(0.25) | 0.2417 | 0.0139 | 0.0555 | 0.0651 | 0.0485 | 0.0650 | ||
Huber | 0.2344 | 0.0209 | 0.0780 | 0.0711 | 0.0684 | 0.0713 | ||
AHR(0.75) | 0.2809 | 0.0427 | 0.1122 | 0.1026 | 0.0969 | 0.1010 | ||
CAHR | 0.2454 | 0.0211 | 0.0774 | 0.0725 | 0.0684 | 0.0718 | ||
WCAHR | 0.2155 | 0.0174 | 0.0727 | 0.0644 | 0.0623 | 0.0642 | ||
400 | OLS | 0.1180 | 0.0242 | 0.0859 | 0.0754 | 0.0776 | 0.0718 | |
LAD | 0.0776 | 0.0149 | 0.0683 | 0.0573 | 0.0622 | 0.0553 | ||
ELS | 0.1267 | 0.0143 | 0.0180 | 0.0841 | 0.0001 | 0.0833 | ||
H-ESL | 0.1199 | 0.0179 | 0.0527 | 0.0831 | 0.0393 | 0.0819 | ||
AHR(0.25) | 0.0776 | 0.0096 | 0.0532 | 0.0478 | 0.0493 | 0.0453 | ||
Huber | 0.0763 | 0.0163 | 0.0753 | 0.0511 | 0.0740 | 0.0500 | ||
AHR(0.75) | 0.1060 | 0.0330 | 0.1043 | 0.0689 | 0.1117 | 0.0700 | ||
CAHR | 0.0813 | 0.0158 | 0.0732 | 0.0491 | 0.0755 | 0.0483 | ||
WCAHR | 0.0672 | 0.0130 | 0.0678 | 0.0451 | 0.0668 | 0.0441 | ||
200 | OLS | 0.5695 | 0.0947 | 0.1071 | 0.1861 | 0.1158 | 0.1876 | |
LAD | 0.3044 | 0.0287 | 0.0704 | 0.0970 | 0.0738 | 0.0941 | ||
ELS | 0.4205 | 0.0400 | 0.0035 | 0.1404 | 0.0162 | 0.1415 | ||
H-ESL | 0.3927 | 0.0405 | 0.0125 | 0.1409 | 0.0038 | 0.1431 | ||
AHR(0.25) | 0.3044 | 0.0219 | 0.0477 | 0.0944 | 0.0466 | 0.0925 | ||
Huber | 0.3450 | 0.0355 | 0.0764 | 0.1077 | 0.0784 | 0.1093 | ||
AHR(0.75) | 2.6347 | 0.5711 | 0.1826 | 0.5139 | 0.1919 | 0.4867 | ||
CAHR | 0.4548 | 0.0423 | 0.0776 | 0.1227 | 0.0826 | 0.1200 | ||
WCAHR | 0.2863 | 0.0246 | 0.0667 | 0.0868 | 0.0703 | 0.0875 | ||
400 | OLS | 0.2663 | 0.0577 | 0.1027 | 0.1286 | 0.1196 | 0.1278 | |
LAD | 0.1092 | 0.0199 | 0.0732 | 0.0694 | 0.0738 | 0.0657 | ||
ELS | 0.1423 | 0.0190 | 0.0083 | 0.0973 | 0.0090 | 0.0968 | ||
H-ESL | 0.1429 | 0.0202 | 0.0184 | 0.0971 | 0.0177 | 0.1003 | ||
AHR(0.25) | 0.1092 | 0.0148 | 0.0456 | 0.0725 | 0.0507 | 0.0698 | ||
Huber | 0.1447 | 0.0237 | 0.0777 | 0.0762 | 0.0784 | 0.0755 | ||
AHR(0.75) | 2.4856 | 0.6166 | 0.2100 | 0.5037 | 0.1899 | 0.5317 | ||
CAHR | 0.1858 | 0.0264 | 0.0726 | 0.0833 | 0.0831 | 0.0852 | ||
WCAHR | 0.0932 | 0.0160 | 0.0645 | 0.0596 | 0.0694 | 0.0592 | ||
200 | OLS | 0.9780 | 0.1653 | 0.0191 | 0.2838 | 0.0090 | 0.2904 | |
LAD | 0.3672 | 0.0409 | 0.0111 | 0.1436 | 0.0065 | 0.1420 | ||
ELS | 0.3644 | 0.0390 | 0.0092 | 0.1407 | 0.0091 | 0.1381 | ||
H-ESL | 0.3186 | 0.0321 | 0.0072 | 0.1260 | 0.0047 | 0.1271 | ||
AHR(0.25) | 0.3672 | 0.1579 | 0.1021 | 0.2647 | 0.0797 | 0.2665 | ||
Huber | 0.3700 | 0.0407 | 0.0134 | 0.1412 | 0.0106 | 0.1431 | ||
AHR(0.75) | 0.7890 | 0.1350 | 0.0802 | 0.2419 | 0.0878 | 0.2498 | ||
CAHR | 0.8570 | 0.0989 | 0.0114 | 0.2229 | 0.0077 | 0.2214 | ||
WCAHR | 0.3324 | 0.0352 | 0.0109 | 0.1326 | 0.0071 | 0.1322 | ||
400 | OLS | 0.4215 | 0.0781 | 0.0126 | 0.2002 | 0.0081 | 0.1944 | |
LAD | 0.1244 | 0.0189 | 0.0000 | 0.0980 | 0.0028 | 0.0964 | ||
ELS | 0.1247 | 0.0173 | 0.0004 | 0.0940 | 0.0028 | 0.0921 | ||
H-ESL | 0.1073 | 0.0131 | 0.0010 | 0.0808 | 0.0019 | 0.0808 | ||
AHR(0.25) | 0.1244 | 0.0759 | 0.0849 | 0.1701 | 0.0953 | 0.1752 | ||
Huber | 0.1222 | 0.0147 | 0.0054 | 0.0861 | 0.0011 | 0.0854 | ||
AHR(0.75) | 0.3546 | 0.0735 | 0.1014 | 0.1642 | 0.0908 | 0.1673 | ||
CAHR | 0.3496 | 0.0531 | 0.0116 | 0.1647 | 0.0069 | 0.1604 | ||
WCAHR | 0.1114 | 0.0148 | 0.0038 | 0.0860 | 0.0001 | 0.0857 | ||
200 | OLS | 0.4259 | 0.0604 | 0.0118 | 0.1733 | 0.0020 | 0.1738 | |
LAD | 0.7261 | 0.1323 | 0.0248 | 0.2573 | 0.0096 | 0.2558 | ||
ELS | 1.9087 | 0.3505 | 0.0283 | 0.4120 | 0.0078 | 0.4241 | ||
H-ESL | 0.4337 | 0.0614 | 0.0118 | 0.1758 | 0.0031 | 0.1743 | ||
AHR(0.25) | 0.7261 | 0.5567 | 0.1960 | 0.5100 | 0.1893 | 0.4716 | ||
Huber | 0.4839 | 0.0763 | 0.0180 | 0.1974 | 0.0064 | 0.1924 | ||
AHR(0.75) | 2.4130 | 0.4776 | 0.1823 | 0.4534 | 0.1885 | 0.4509 | ||
CAHR | 0.6745 | 0.1187 | 0.0223 | 0.2449 | 0.0087 | 0.2411 | ||
WCAHR | 0.4361 | 0.0642 | 0.0096 | 0.1797 | 0.0068 | 0.1783 | ||
400 | OLS | 0.1934 | 0.0295 | 0.0087 | 0.1251 | 0.0113 | 0.1169 | |
LAD | 0.3626 | 0.0578 | 0.0145 | 0.1715 | 0.0169 | 0.1670 | ||
ELS | 1.0701 | 0.1718 | 0.0200 | 0.2893 | 0.0187 | 0.2955 | ||
H-ESL | 0.1948 | 0.0299 | 0.0098 | 0.1256 | 0.0113 | 0.1178 | ||
AHR(0.25) | 0.3626 | 0.3363 | 0.2023 | 0.3440 | 0.2239 | 0.3562 | ||
Huber | 0.2316 | 0.0383 | 0.0093 | 0.1438 | 0.0130 | 0.1320 | ||
AHR(0.75) | 1.2586 | 0.3302 | 0.2280 | 0.3504 | 0.1848 | 0.3484 | ||
CAHR | 0.3292 | 0.0506 | 0.0163 | 0.1645 | 0.0174 | 0.1516 | ||
WCAHR | 0.2058 | 0.0313 | 0.0119 | 0.1298 | 0.0092 | 0.1193 |
Errors | MISE | MSE() | ||||||
Bias | Sd | Bias | Sd | |||||
200 | 4 | 0.2321 | 0.0088 | 0.0034 | 0.0663 | 0.0044 | 0.0665 | |
6 | 0.2295 | 0.0084 | 0.0036 | 0.0646 | 0.0042 | 0.0645 | ||
8 | 0.2279 | 0.0081 | 0.0038 | 0.0635 | 0.0042 | 0.0633 | ||
10 | 0.2269 | 0.0079 | 0.0040 | 0.0629 | 0.0041 | 0.0626 | ||
12 | 0.2264 | 0.0078 | 0.0041 | 0.0627 | 0.0041 | 0.0621 | ||
14 | 0.2262 | 0.0078 | 0.0043 | 0.0627 | 0.0041 | 0.0620 | ||
400 | 4 | 0.0626 | 0.0039 | 0.0071 | 0.0450 | -0.0054 | 0.0425 | |
6 | 0.0611 | 0.0037 | 0.0068 | 0.0440 | 0.0050 | 0.0415 | ||
8 | 0.0601 | 0.0036 | 0.0065 | 0.0434 | 0.0046 | 0.0410 | ||
10 | 0.0595 | 0.0036 | 0.0063 | 0.0432 | 0.0043 | 0.0409 | ||
12 | 0.0591 | 0.0036 | 0.0060 | 0.0432 | 0.0039 | 0.0409 | ||
14 | 0.0589 | 0.0036 | 0.0059 | 0.0433 | 0.0038 | 0.0411 | ||
200 | 4 | 0.2445 | 0.0178 | 0.0027 | 0.0961 | 0.0023 | 0.0927 | |
6 | 0.2388 | 0.0169 | 0.0025 | 0.0938 | 0.0024 | 0.0900 | ||
8 | 0.2349 | 0.0163 | 0.0025 | 0.0923 | 0.0023 | 0.0880 | ||
10 | 0.2321 | 0.0158 | 0.0025 | 0.0911 | 0.0023 | 0.0866 | ||
12 | 0.2301 | 0.0155 | 0.0024 | 0.0903 | 0.0022 | 0.0856 | ||
14 | 0.2287 | 0.0153 | 0.0023 | 0.0898 | 0.0022 | 0.0849 | ||
400 | 4 | 0.0925 | 0.0090 | 0.0079 | 0.0678 | 0.0076 | 0.0652 | |
6 | 0.0899 | 0.0085 | 0.0078 | 0.0659 | 0.0076 | 0.0637 | ||
8 | 0.0880 | 0.0082 | 0.0076 | 0.0646 | 0.0076 | 0.0626 | ||
10 | 0.0867 | 0.0080 | 0.0076 | 0.0636 | 0.0076 | 0.0619 | ||
12 | 0.0857 | 0.0078 | 0.0075 | 0.0629 | 0.0077 | 0.0615 | ||
14 | 0.0850 | 0.0077 | 0.0074 | 0.0623 | 0.0077 | 0.0611 |
Electricity prices | Tecator | |||||
Methods | N=100 | N=200 | N=400 | N=100 | N=200 | N=400 |
OLS | 0.4269 | 0.4132 | 0.4153 | 2.782410 | 2.718210 | 2.725710 |
LAD | 0.4094 | 0.4005 | 0.4068 | 2.938810 | 2.861110 | 2.825310 |
ESL | 0.5751 | 0.5626 | 0.5578 | 2.726810 | 2.670110 | 2.614210 |
H-ESL | 0.4104 | 0.4026 | 0.4064 | 2.739510 | 2.733610 | 2.647610 |
AHR(0.25) | 0.4052 | 0.3985 | 0.4032 | 2.905610 | 2.765810 | 2.775310 |
Huber | 0.4077 | 0.4027 | 0.4074 | 2.763610 | 2.649110 | 2.627810 |
AHR(0.75) | 0.4296 | 0.4198 | 0.4280 | 2.740910 | 2.649410 | 2.606310 |
CAHR | 0.4103 | 0.4019 | 0.4089 | 2.844210 | 2.810610 | 2.745710 |
WCAHR | 0.4030 | 0.3967 | 0.4008 | 2.723610 | 2.615210 | 2.579910 |
Electricity prices | Tecator | |||
OLS | 0.5983 | 0.4672 | 1.1056 | 0.6894 |
LAD | 0.7095 | 0.9438 | 1.0828 | 0.7611 |
ESL | 0.5125 | 0.4629 | 1.0894 | 0.7455 |
H-ESL | 0.6007 | 0.7212 | 1.0983 | 0.7367 |
AHR(0.25) | 0.5618 | 0.4725 | 1.1122 | 0.7026 |
Huber | 0.5799 | 0.4416 | 1.0981 | 0.7235 |
AHR(0.75) | 0.5812 | 0.4302 | 1.0854 | 0.7576 |
CAHR | 0.5394 | 0.4582 | 1.0990 | 0.7274 |
WCAHR | 0.5924 | 0.6182 | 1.0964 | 0.7270 |
Errors | Method | MISE | MSE() | |||||
Bias | Sd | Bias | Sd | |||||
200 | OLS | 0.2690 | 0.0229 | 0.0048 | 0.1074 | 0.0096 | 0.1059 | |
LAD | 0.3294 | 0.0354 | 0.0099 | 0.1320 | 0.0115 | 0.1333 | ||
ESL | 0.3434 | 0.0394 | 0.0009 | 0.1405 | 0.0013 | 0.1403 | ||
H-ESL | 0.2824 | 0.0259 | 0.0055 | 0.1141 | 0.0059 | 0.1133 | ||
AHR(0.25) | 0.3294 | 0.0786 | 0.0077 | 0.2014 | 0.0105 | 0.1948 | ||
Huber | 0.2758 | 0.0234 | 0.0063 | 0.1090 | 0.0102 | 0.1067 | ||
AHR(0.75) | 0.5251 | 0.0754 | 0.0092 | 0.1894 | 0.0150 | 0.1981 | ||
CAHR | 0.2689 | 0.0233 | 0.0051 | 0.1095 | 0.0091 | 0.1060 | ||
WCAHR | 0.2693 | 0.0230 | 0.0055 | 0.1080 | 0.0101 | 0.1058 | ||
400 | OLS | 0.1004 | 0.0105 | 0.0011 | 0.0710 | 0.0015 | 0.0738 | |
LAD | 0.1304 | 0.0163 | 0.0027 | 0.0880 | 0.0045 | 0.0924 | ||
ESL | 0.1349 | 0.0175 | 0.0010 | 0.0918 | 0.0008 | 0.0955 | ||
H-ESL | 0.1031 | 0.0113 | 0.0011 | 0.0727 | 0.0010 | 0.0773 | ||
AHR(0.25) | 0.1304 | 0.0358 | 0.0048 | 0.1313 | 0.0013 | 0.1361 | ||
Huber | 0.1048 | 0.0107 | 0.0018 | 0.0720 | 0.0027 | 0.0742 | ||
AHR(0.75) | 0.2337 | 0.0405 | 0.0043 | 0.1454 | 0.0019 | 0.1390 | ||
CAHR | 0.1006 | 0.0107 | 0.0014 | 0.0721 | 0.0020 | 0.0743 | ||
WCAHR | 0.1005 | 0.0105 | 0.0014 | 0.0709 | 0.0019 | 0.0736 | ||
200 | OLS | 0.2929 | 0.0241 | 0.0037 | 0.1127 | 0.0012 | 0.1065 | |
LAD | 0.2452 | 0.0137 | 0.0001 | 0.0844 | 0.0007 | 0.0813 | ||
ESL | 0.3665 | 0.0387 | 0.0031 | 0.1412 | 0.0023 | 0.1368 | ||
H-ESL | 0.3377 | 0.0305 | 0.0009 | 0.1244 | 0.0028 | 0.1225 | ||
AHR(0.25) | 0.2260 | 0.0086 | 0.0012 | 0.0652 | 0.0022 | 0.0655 | ||
Huber | 0.1998 | 0.0098 | 0.0011 | 0.0698 | 0.0007 | 0.0702 | ||
AHR(0.75) | 0.2314 | 0.0172 | 0.0038 | 0.0949 | 0.0001 | 0.0903 | ||
CAHR | 0.2122 | 0.0099 | 0.0007 | 0.0717 | 0.0010 | 0.0691 | ||
WCAHR | 0.1884 | 0.0086 | 0.0002 | 0.0659 | 0.0017 | 0.0652 | ||
400 | OLS | 0.0994 | 0.0122 | 0.0024 | 0.0794 | 0.0052 | 0.0769 | |
LAD | 0.0718 | 0.0065 | 0.0004 | 0.0569 | 0.0001 | 0.0568 | ||
ESL | 0.1372 | 0.0193 | 0.0012 | 0.1003 | 0.0031 | 0.0962 | ||
H-ESL | 0.1022 | 0.0139 | 0.0043 | 0.0832 | 0.0056 | 0.0832 | ||
AHR(0.25) | 0.0796 | 0.0037 | 0.0028 | 0.0418 | 0.0010 | 0.0437 | ||
Huber | 0.0688 | 0.0045 | 0.0005 | 0.0468 | 0.0003 | 0.0483 | ||
AHR(0.75) | 0.0912 | 0.0082 | 0.0010 | 0.0627 | 0.0019 | 0.0652 | ||
CAHR | 0.0712 | 0.0043 | 0.0007 | 0.0454 | 0.0009 | 0.0471 | ||
WCAHR | 0.0567 | 0.0035 | 0.0009 | 0.0418 | 0.0018 | 0.0424 | ||
200 | OLS | 0.4781 | 0.0695 | 0.0047 | 0.1815 | 0.0185 | 0.1902 | |
LAD | 0.2461 | 0.0215 | 0.0057 | 0.1052 | 0.0094 | 0.1015 | ||
ESL | 0.3793 | 0.0451 | 0.0023 | 0.1538 | 0.0062 | 0.1462 | ||
H-ESL | 0.3682 | 0.0443 | 0.0059 | 0.1533 | 0.0078 | 0.1437 | ||
AHR(0.25) | 0.2541 | 0.0203 | 0.0014 | 0.0987 | 0.0039 | 0.1025 | ||
Huber | 0.2829 | 0.0284 | 0.0082 | 0.1204 | 0.0013 | 0.1175 | ||
AHR(0.75) | 1.8541 | 0.3745 | 0.0128 | 0.4566 | 0.0243 | 0.4065 | ||
CAHR | 0.3606 | 0.0286 | 0.0037 | 0.1188 | 0.0001 | 0.1202 | ||
WCAHR | 0.2205 | 0.0169 | 0.0018 | 0.0920 | 0.0089 | 0.0915 | ||
400 | OLS | 0.2310 | 0.0325 | 0.0021 | 0.1296 | 0.0008 | 0.1252 | |
LAD | 0.1004 | 0.0109 | 0.0006 | 0.0742 | 0.0001 | 0.0735 | ||
ESL | 0.1563 | 0.0212 | 0.0053 | 0.1002 | 0.0027 | 0.1054 | ||
H-ESL | 0.1516 | 0.0178 | 0.0045 | 0.0917 | 0.0011 | 0.0966 | ||
AHR(0.25) | 0.1000 | 0.0088 | 0.0028 | 0.0671 | 0.0004 | 0.0659 | ||
Huber | 0.1108 | 0.0116 | 0.0019 | 0.0781 | 0.0021 | 0.0743 | ||
AHR(0.75) | 1.5565 | 0.3644 | 0.0416 | 0.4269 | 0.0216 | 0.4242 | ||
CAHR | 0.1496 | 0.0153 | 0.0016 | 0.0873 | 0.0007 | 0.0874 | ||
WCAHR | 0.0838 | 0.0076 | 0.0015 | 0.0616 | 0.0000 | 0.0618 | ||
200 | OLS | 0.8806 | 0.1464 | 0.0134 | 0.2675 | 0.0016 | 0.2732 | |
LAD | 0.3783 | 0.0358 | 0.0005 | 0.1320 | 0.0013 | 0.1355 | ||
ESL | 0.3719 | 0.0363 | 0.0025 | 0.1331 | 0.0044 | 0.1361 | ||
H-ESL | 0.3101 | 0.0280 | 0.0018 | 0.1175 | 0.0028 | 0.1189 | ||
AHR(0.25) | 0.3297 | 0.1148 | 0.0120 | 0.2346 | 0.0105 | 0.2439 | ||
Huber | 0.3685 | 0.0499 | 0.0042 | 0.1613 | 0.0071 | 0.1543 | ||
AHR(0.75) | 0.7037 | 0.1060 | 0.0078 | 0.2321 | 0.0033 | 0.2281 | ||
CAHR | 0.7857 | 0.1035 | 0.0031 | 0.2292 | 0.0035 | 0.2257 | ||
WCAHR | 0.3252 | 0.0340 | 0.0046 | 0.1289 | 0.0033 | 0.1316 | ||
400 | OLS | 0.3822 | 0.0715 | 0.0063 | 0.1887 | 0.0099 | 0.1892 | |
LAD | 0.1307 | 0.0190 | 0.0055 | 0.0983 | 0.0060 | 0.0965 | ||
ELS | 0.1268 | 0.0180 | 0.0032 | 0.0963 | 0.0043 | 0.0932 | ||
H-ESL | 0.1032 | 0.0129 | 0.0048 | 0.0807 | 0.0067 | 0.0793 | ||
AHR(0.25) | 0.1491 | 0.0604 | 0.0052 | 0.1731 | 0.0055 | 0.1742 | ||
Huber | 0.1332 | 0.0156 | 0.0007 | 0.0880 | 0.0033 | 0.0887 | ||
AHR(0.75) | 0.3391 | 0.0505 | 0.0049 | 0.1597 | 0.0040 | 0.1581 | ||
CAHR | 0.3435 | 0.0419 | -0.0030 | 0.1442 | 0.0074 | 0.1449 | ||
WCAHR | 0.1127 | 0.0157 | 0.0055 | 0.0894 | 0.0077 | 0.0872 | ||
200 | OLS | 0.4317 | 0.0560 | 0.0001 | 0.1690 | 0.0018 | 0.1657 | |
LAD | 0.8634 | 0.1201 | 0.0040 | 0.2438 | 0.0008 | 0.2463 | ||
ESL | 2.2921 | 0.4163 | 0.0148 | 0.4541 | 0.0002 | 0.4582 | ||
H-ESL | 0.4417 | 0.0558 | 0.0004 | 0.1687 | 0.0017 | 0.1653 | ||
AHR(0.25) | 0.9240 | 0.3169 | 0.0258 | 0.3973 | 0.0352 | 0.3964 | ||
Huber | 0.5694 | 0.0776 | 0.0056 | 0.2018 | 0.0129 | 0.1914 | ||
AHR(0.75) | 1.8171 | 0.3150 | 0.0110 | 0.4044 | 0.0107 | 0.3889 | ||
CAHR | 0.5191 | 0.0546 | 0.0075 | 0.1652 | 0.0116 | 0.1647 | ||
WCAHR | 0.4215 | 0.0552 | 0.0016 | 0.1679 | 0.0016 | 0.1642 | ||
400 | OLS | 0.1861 | 0.0296 | 0.0032 | 0.1170 | 0.0017 | 0.1262 | |
LAD | 0.4069 | 0.0670 | 0.0068 | 0.1765 | 0.0061 | 0.1891 | ||
ESL | 1.5317 | 0.2511 | 0.0185 | 0.3481 | 0.0033 | 0.3600 | ||
H-ESL | 0.1860 | 0.0298 | 0.0032 | 0.1175 | 0.0021 | 0.1265 | ||
AHR(0.25) | 0.4420 | 0.1620 | 0.0081 | 0.2835 | 0.0025 | 0.2855 | ||
Huber | 0.2369 | 0.0454 | 0.0023 | 0.1483 | 0.0082 | 0.1529 | ||
AHR(0.75) | 0.8504 | 0.1523 | 0.0142 | 0.2774 | 0.0021 | 0.2742 | ||
CAHR | 0.2047 | 0.0296 | 0.0025 | 0.1195 | 0.0014 | 0.1239 | ||
WCAHR | 0.1825 | 0.0291 | 0.0033 | 0.1162 | 0.0019 | 0.1249 |
Errors | Method | MISE | MSE() | |||||
Bias | Sd | Bias | Sd | |||||
200 | OLS | 0.2560 | 0.0237 | 0.0049 | 0.1105 | 0.0052 | 0.1069 | |
LAD | 0.2988 | 0.0300 | 0.0054 | 0.1228 | 0.0009 | 0.1222 | ||
ELS | 0.2990 | 0.0316 | 0.0041 | 0.1272 | 0.0021 | 0.1240 | ||
H-ESL | 0.2655 | 0.0258 | 0.0060 | 0.1163 | 0.0052 | 0.1104 | ||
AHR(0.25) | 0.2988 | 0.1065 | 0.0860 | 0.2178 | 0.0965 | 0.2058 | ||
Huber | 0.2531 | 0.0234 | 0.0050 | 0.1099 | 0.0045 | 0.1062 | ||
AHR(0.75) | 0.5960 | 0.0911 | 0.0958 | 0.1880 | 0.0836 | 0.1990 | ||
CAHR | 0.2562 | 0.0236 | 0.0055 | 0.1099 | 0.0051 | 0.1070 | ||
WCAHR | 0.2519 | 0.0227 | 0.0051 | 0.1081 | 0.0045 | 0.1047 | ||
400 | OLS | 0.1056 | 0.0119 | 0.0029 | 0.0767 | 0.0002 | 0.0777 | |
LAD | 0.1269 | 0.0153 | 0.0027 | 0.0865 | 0.0008 | 0.0882 | ||
ELS | 0.1257 | 0.0157 | 0.0026 | 0.0884 | 0.0019 | 0.0888 | ||
H-ESL | 0.1061 | 0.0116 | 0.0036 | 0.0760 | 0.0018 | 0.0762 | ||
AHR(0.25) | 0.1269 | 0.0588 | 0.1020 | 0.1418 | 0.0889 | 0.1427 | ||
Huber | 0.1060 | 0.0117 | 0.0032 | 0.0764 | 0.0001 | 0.0766 | ||
AHR(0.75) | 0.2695 | 0.0581 | 0.0990 | 0.1428 | 0.0859 | 0.1434 | ||
CAHR | 0.1064 | 0.0120 | 0.0023 | 0.0769 | 0.0005 | 0.0777 | ||
WCAHR | 0.1046 | 0.0115 | 0.0030 | 0.0754 | 0.0002 | 0.0762 | ||
200 | OLS | 0.3164 | 0.0357 | 0.0862 | 0.1070 | 0.0748 | 0.1057 | |
LAD | 0.2417 | 0.0209 | 0.0695 | 0.0789 | 0.0586 | 0.0803 | ||
ELS | 0.3578 | 0.0306 | 0.0140 | 0.1234 | 0.0119 | 0.1228 | ||
H-ESL | 0.3390 | 0.0316 | 0.0308 | 0.1211 | 0.0304 | 0.1228 | ||
AHR(0.25) | 0.2417 | 0.0139 | 0.0555 | 0.0651 | 0.0485 | 0.0650 | ||
Huber | 0.2344 | 0.0209 | 0.0780 | 0.0711 | 0.0684 | 0.0713 | ||
AHR(0.75) | 0.2809 | 0.0427 | 0.1122 | 0.1026 | 0.0969 | 0.1010 | ||
CAHR | 0.2454 | 0.0211 | 0.0774 | 0.0725 | 0.0684 | 0.0718 | ||
WCAHR | 0.2155 | 0.0174 | 0.0727 | 0.0644 | 0.0623 | 0.0642 | ||
400 | OLS | 0.1180 | 0.0242 | 0.0859 | 0.0754 | 0.0776 | 0.0718 | |
LAD | 0.0776 | 0.0149 | 0.0683 | 0.0573 | 0.0622 | 0.0553 | ||
ELS | 0.1267 | 0.0143 | 0.0180 | 0.0841 | 0.0001 | 0.0833 | ||
H-ESL | 0.1199 | 0.0179 | 0.0527 | 0.0831 | 0.0393 | 0.0819 | ||
AHR(0.25) | 0.0776 | 0.0096 | 0.0532 | 0.0478 | 0.0493 | 0.0453 | ||
Huber | 0.0763 | 0.0163 | 0.0753 | 0.0511 | 0.0740 | 0.0500 | ||
AHR(0.75) | 0.1060 | 0.0330 | 0.1043 | 0.0689 | 0.1117 | 0.0700 | ||
CAHR | 0.0813 | 0.0158 | 0.0732 | 0.0491 | 0.0755 | 0.0483 | ||
WCAHR | 0.0672 | 0.0130 | 0.0678 | 0.0451 | 0.0668 | 0.0441 | ||
200 | OLS | 0.5695 | 0.0947 | 0.1071 | 0.1861 | 0.1158 | 0.1876 | |
LAD | 0.3044 | 0.0287 | 0.0704 | 0.0970 | 0.0738 | 0.0941 | ||
ELS | 0.4205 | 0.0400 | 0.0035 | 0.1404 | 0.0162 | 0.1415 | ||
H-ESL | 0.3927 | 0.0405 | 0.0125 | 0.1409 | 0.0038 | 0.1431 | ||
AHR(0.25) | 0.3044 | 0.0219 | 0.0477 | 0.0944 | 0.0466 | 0.0925 | ||
Huber | 0.3450 | 0.0355 | 0.0764 | 0.1077 | 0.0784 | 0.1093 | ||
AHR(0.75) | 2.6347 | 0.5711 | 0.1826 | 0.5139 | 0.1919 | 0.4867 | ||
CAHR | 0.4548 | 0.0423 | 0.0776 | 0.1227 | 0.0826 | 0.1200 | ||
WCAHR | 0.2863 | 0.0246 | 0.0667 | 0.0868 | 0.0703 | 0.0875 | ||
400 | OLS | 0.2663 | 0.0577 | 0.1027 | 0.1286 | 0.1196 | 0.1278 | |
LAD | 0.1092 | 0.0199 | 0.0732 | 0.0694 | 0.0738 | 0.0657 | ||
ELS | 0.1423 | 0.0190 | 0.0083 | 0.0973 | 0.0090 | 0.0968 | ||
H-ESL | 0.1429 | 0.0202 | 0.0184 | 0.0971 | 0.0177 | 0.1003 | ||
AHR(0.25) | 0.1092 | 0.0148 | 0.0456 | 0.0725 | 0.0507 | 0.0698 | ||
Huber | 0.1447 | 0.0237 | 0.0777 | 0.0762 | 0.0784 | 0.0755 | ||
AHR(0.75) | 2.4856 | 0.6166 | 0.2100 | 0.5037 | 0.1899 | 0.5317 | ||
CAHR | 0.1858 | 0.0264 | 0.0726 | 0.0833 | 0.0831 | 0.0852 | ||
WCAHR | 0.0932 | 0.0160 | 0.0645 | 0.0596 | 0.0694 | 0.0592 | ||
200 | OLS | 0.9780 | 0.1653 | 0.0191 | 0.2838 | 0.0090 | 0.2904 | |
LAD | 0.3672 | 0.0409 | 0.0111 | 0.1436 | 0.0065 | 0.1420 | ||
ELS | 0.3644 | 0.0390 | 0.0092 | 0.1407 | 0.0091 | 0.1381 | ||
H-ESL | 0.3186 | 0.0321 | 0.0072 | 0.1260 | 0.0047 | 0.1271 | ||
AHR(0.25) | 0.3672 | 0.1579 | 0.1021 | 0.2647 | 0.0797 | 0.2665 | ||
Huber | 0.3700 | 0.0407 | 0.0134 | 0.1412 | 0.0106 | 0.1431 | ||
AHR(0.75) | 0.7890 | 0.1350 | 0.0802 | 0.2419 | 0.0878 | 0.2498 | ||
CAHR | 0.8570 | 0.0989 | 0.0114 | 0.2229 | 0.0077 | 0.2214 | ||
WCAHR | 0.3324 | 0.0352 | 0.0109 | 0.1326 | 0.0071 | 0.1322 | ||
400 | OLS | 0.4215 | 0.0781 | 0.0126 | 0.2002 | 0.0081 | 0.1944 | |
LAD | 0.1244 | 0.0189 | 0.0000 | 0.0980 | 0.0028 | 0.0964 | ||
ELS | 0.1247 | 0.0173 | 0.0004 | 0.0940 | 0.0028 | 0.0921 | ||
H-ESL | 0.1073 | 0.0131 | 0.0010 | 0.0808 | 0.0019 | 0.0808 | ||
AHR(0.25) | 0.1244 | 0.0759 | 0.0849 | 0.1701 | 0.0953 | 0.1752 | ||
Huber | 0.1222 | 0.0147 | 0.0054 | 0.0861 | 0.0011 | 0.0854 | ||
AHR(0.75) | 0.3546 | 0.0735 | 0.1014 | 0.1642 | 0.0908 | 0.1673 | ||
CAHR | 0.3496 | 0.0531 | 0.0116 | 0.1647 | 0.0069 | 0.1604 | ||
WCAHR | 0.1114 | 0.0148 | 0.0038 | 0.0860 | 0.0001 | 0.0857 | ||
200 | OLS | 0.4259 | 0.0604 | 0.0118 | 0.1733 | 0.0020 | 0.1738 | |
LAD | 0.7261 | 0.1323 | 0.0248 | 0.2573 | 0.0096 | 0.2558 | ||
ELS | 1.9087 | 0.3505 | 0.0283 | 0.4120 | 0.0078 | 0.4241 | ||
H-ESL | 0.4337 | 0.0614 | 0.0118 | 0.1758 | 0.0031 | 0.1743 | ||
AHR(0.25) | 0.7261 | 0.5567 | 0.1960 | 0.5100 | 0.1893 | 0.4716 | ||
Huber | 0.4839 | 0.0763 | 0.0180 | 0.1974 | 0.0064 | 0.1924 | ||
AHR(0.75) | 2.4130 | 0.4776 | 0.1823 | 0.4534 | 0.1885 | 0.4509 | ||
CAHR | 0.6745 | 0.1187 | 0.0223 | 0.2449 | 0.0087 | 0.2411 | ||
WCAHR | 0.4361 | 0.0642 | 0.0096 | 0.1797 | 0.0068 | 0.1783 | ||
400 | OLS | 0.1934 | 0.0295 | 0.0087 | 0.1251 | 0.0113 | 0.1169 | |
LAD | 0.3626 | 0.0578 | 0.0145 | 0.1715 | 0.0169 | 0.1670 | ||
ELS | 1.0701 | 0.1718 | 0.0200 | 0.2893 | 0.0187 | 0.2955 | ||
H-ESL | 0.1948 | 0.0299 | 0.0098 | 0.1256 | 0.0113 | 0.1178 | ||
AHR(0.25) | 0.3626 | 0.3363 | 0.2023 | 0.3440 | 0.2239 | 0.3562 | ||
Huber | 0.2316 | 0.0383 | 0.0093 | 0.1438 | 0.0130 | 0.1320 | ||
AHR(0.75) | 1.2586 | 0.3302 | 0.2280 | 0.3504 | 0.1848 | 0.3484 | ||
CAHR | 0.3292 | 0.0506 | 0.0163 | 0.1645 | 0.0174 | 0.1516 | ||
WCAHR | 0.2058 | 0.0313 | 0.0119 | 0.1298 | 0.0092 | 0.1193 |
Errors | MISE | MSE() | ||||||
Bias | Sd | Bias | Sd | |||||
200 | 4 | 0.2321 | 0.0088 | 0.0034 | 0.0663 | 0.0044 | 0.0665 | |
6 | 0.2295 | 0.0084 | 0.0036 | 0.0646 | 0.0042 | 0.0645 | ||
8 | 0.2279 | 0.0081 | 0.0038 | 0.0635 | 0.0042 | 0.0633 | ||
10 | 0.2269 | 0.0079 | 0.0040 | 0.0629 | 0.0041 | 0.0626 | ||
12 | 0.2264 | 0.0078 | 0.0041 | 0.0627 | 0.0041 | 0.0621 | ||
14 | 0.2262 | 0.0078 | 0.0043 | 0.0627 | 0.0041 | 0.0620 | ||
400 | 4 | 0.0626 | 0.0039 | 0.0071 | 0.0450 | -0.0054 | 0.0425 | |
6 | 0.0611 | 0.0037 | 0.0068 | 0.0440 | 0.0050 | 0.0415 | ||
8 | 0.0601 | 0.0036 | 0.0065 | 0.0434 | 0.0046 | 0.0410 | ||
10 | 0.0595 | 0.0036 | 0.0063 | 0.0432 | 0.0043 | 0.0409 | ||
12 | 0.0591 | 0.0036 | 0.0060 | 0.0432 | 0.0039 | 0.0409 | ||
14 | 0.0589 | 0.0036 | 0.0059 | 0.0433 | 0.0038 | 0.0411 | ||
200 | 4 | 0.2445 | 0.0178 | 0.0027 | 0.0961 | 0.0023 | 0.0927 | |
6 | 0.2388 | 0.0169 | 0.0025 | 0.0938 | 0.0024 | 0.0900 | ||
8 | 0.2349 | 0.0163 | 0.0025 | 0.0923 | 0.0023 | 0.0880 | ||
10 | 0.2321 | 0.0158 | 0.0025 | 0.0911 | 0.0023 | 0.0866 | ||
12 | 0.2301 | 0.0155 | 0.0024 | 0.0903 | 0.0022 | 0.0856 | ||
14 | 0.2287 | 0.0153 | 0.0023 | 0.0898 | 0.0022 | 0.0849 | ||
400 | 4 | 0.0925 | 0.0090 | 0.0079 | 0.0678 | 0.0076 | 0.0652 | |
6 | 0.0899 | 0.0085 | 0.0078 | 0.0659 | 0.0076 | 0.0637 | ||
8 | 0.0880 | 0.0082 | 0.0076 | 0.0646 | 0.0076 | 0.0626 | ||
10 | 0.0867 | 0.0080 | 0.0076 | 0.0636 | 0.0076 | 0.0619 | ||
12 | 0.0857 | 0.0078 | 0.0075 | 0.0629 | 0.0077 | 0.0615 | ||
14 | 0.0850 | 0.0077 | 0.0074 | 0.0623 | 0.0077 | 0.0611 |
Electricity prices | Tecator | |||||
Methods | N=100 | N=200 | N=400 | N=100 | N=200 | N=400 |
OLS | 0.4269 | 0.4132 | 0.4153 | 2.782410 | 2.718210 | 2.725710 |
LAD | 0.4094 | 0.4005 | 0.4068 | 2.938810 | 2.861110 | 2.825310 |
ESL | 0.5751 | 0.5626 | 0.5578 | 2.726810 | 2.670110 | 2.614210 |
H-ESL | 0.4104 | 0.4026 | 0.4064 | 2.739510 | 2.733610 | 2.647610 |
AHR(0.25) | 0.4052 | 0.3985 | 0.4032 | 2.905610 | 2.765810 | 2.775310 |
Huber | 0.4077 | 0.4027 | 0.4074 | 2.763610 | 2.649110 | 2.627810 |
AHR(0.75) | 0.4296 | 0.4198 | 0.4280 | 2.740910 | 2.649410 | 2.606310 |
CAHR | 0.4103 | 0.4019 | 0.4089 | 2.844210 | 2.810610 | 2.745710 |
WCAHR | 0.4030 | 0.3967 | 0.4008 | 2.723610 | 2.615210 | 2.579910 |
Electricity prices | Tecator | |||
OLS | 0.5983 | 0.4672 | 1.1056 | 0.6894 |
LAD | 0.7095 | 0.9438 | 1.0828 | 0.7611 |
ESL | 0.5125 | 0.4629 | 1.0894 | 0.7455 |
H-ESL | 0.6007 | 0.7212 | 1.0983 | 0.7367 |
AHR(0.25) | 0.5618 | 0.4725 | 1.1122 | 0.7026 |
Huber | 0.5799 | 0.4416 | 1.0981 | 0.7235 |
AHR(0.75) | 0.5812 | 0.4302 | 1.0854 | 0.7576 |
CAHR | 0.5394 | 0.4582 | 1.0990 | 0.7274 |
WCAHR | 0.5924 | 0.6182 | 1.0964 | 0.7270 |