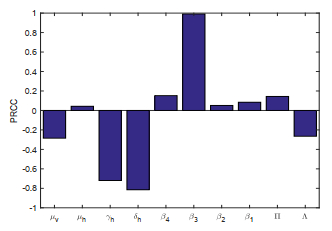
A stochastic model of leptospirosis with vector and environmental transmission is established in this paper. By mathematical analysis of the model, the threshold for eliminating the disease is obtained. The partial rank correlation coefficient was used to analyze the parameters that have a greater impact on disease elimination, and a sensitivity analysis was conducted on the parameters through numerical simulation. Further, combined with the data of leptospirosis case reports in China from 2003 to 2021, two parameter estimation methods, Least Squares method (LSM) and Markov Chain Monte Carlo-Metropolis Hastings method (MCMC-MH), are applied to estimate the important parameters of the model and the future trend of leptospirosis in China are predicted.
Citation: Xiangyun Shi, Dan Zhou, Xueyong Zhou, Fan Yu. Predicting the trend of leptospirosis in China via a stochastic model with vector and environmental transmission[J]. Electronic Research Archive, 2024, 32(6): 3937-3951. doi: 10.3934/era.2024176
[1] | Wenxuan Li, Suli Liu . Dynamic analysis of a stochastic epidemic model incorporating the double epidemic hypothesis and Crowley-Martin incidence term. Electronic Research Archive, 2023, 31(10): 6134-6159. doi: 10.3934/era.2023312 |
[2] | Tao Zhang, Mengjuan Wu, Chunjie Gao, Yingdan Wang, Lei Wang . Probability of disease extinction and outbreak in a stochastic tuberculosis model with fast-slow progression and relapse. Electronic Research Archive, 2023, 31(11): 7104-7124. doi: 10.3934/era.2023360 |
[3] | Zhimin Li, Tailei Zhang, Xiuqing Li . Threshold dynamics of stochastic models with time delays: A case study for Yunnan, China. Electronic Research Archive, 2021, 29(1): 1661-1679. doi: 10.3934/era.2020085 |
[4] | Gaohui Fan, Ning Li . Application and analysis of a model with environmental transmission in a periodic environment. Electronic Research Archive, 2023, 31(9): 5815-5844. doi: 10.3934/era.2023296 |
[5] | Wenhui Niu, Xinhong Zhang, Daqing Jiang . Dynamics and numerical simulations of a generalized mosquito-borne epidemic model using the Ornstein-Uhlenbeck process: Stability, stationary distribution, and probability density function. Electronic Research Archive, 2024, 32(6): 3777-3818. doi: 10.3934/era.2024172 |
[6] | Feng Wang, Taotao Li . Dynamics of a stochastic epidemic model with information intervention and vertical transmission. Electronic Research Archive, 2024, 32(6): 3700-3727. doi: 10.3934/era.2024168 |
[7] | Xianfei Hui, Baiqing Sun, Indranil SenGupta, Yan Zhou, Hui Jiang . Stochastic volatility modeling of high-frequency CSI 300 index and dynamic jump prediction driven by machine learning. Electronic Research Archive, 2023, 31(3): 1365-1386. doi: 10.3934/era.2023070 |
[8] | Yanjiao Li, Yue Zhang . Dynamic behavior on a multi-time scale eco-epidemic model with stochastic disturbances. Electronic Research Archive, 2025, 33(3): 1667-1692. doi: 10.3934/era.2025078 |
[9] | Zhiliang Li, Lijun Pei, Guangcai Duan, Shuaiyin Chen . A non-autonomous time-delayed SIR model for COVID-19 epidemics prediction in China during the transmission of Omicron variant. Electronic Research Archive, 2024, 32(3): 2203-2228. doi: 10.3934/era.2024100 |
[10] | Xueyong Zhou, Xiangyun Shi . Stability analysis and backward bifurcation on an SEIQR epidemic model with nonlinear innate immunity. Electronic Research Archive, 2022, 30(9): 3481-3508. doi: 10.3934/era.2022178 |
A stochastic model of leptospirosis with vector and environmental transmission is established in this paper. By mathematical analysis of the model, the threshold for eliminating the disease is obtained. The partial rank correlation coefficient was used to analyze the parameters that have a greater impact on disease elimination, and a sensitivity analysis was conducted on the parameters through numerical simulation. Further, combined with the data of leptospirosis case reports in China from 2003 to 2021, two parameter estimation methods, Least Squares method (LSM) and Markov Chain Monte Carlo-Metropolis Hastings method (MCMC-MH), are applied to estimate the important parameters of the model and the future trend of leptospirosis in China are predicted.
Leptospirosis is an acute systemic infectious disease caused by various pathogenic leptospira, which belongs to natural foci disease. It is epidemic almost all over the world, especially severe in Southeast Asia. Most provinces, cities, and autonomous regions in China have the existence and epidemic of this disease. Rodents and pigs are the two major sources of infection, while other livestock such as cattle, pigs, and pets like cats, dogs, and mice may also transmit leptospirosis. Typically, pathogenic leptospira can survive longer in a warm and humid environment. People may contract the disease through ingestion of contaminated food or water, or when the bacteria enter the body through scratches on the skin or mucous membranes [1].
The application of mathematical models in leptospirosis research has also become increasingly widespread. Through numerical simulations and data analysis, we can delve deeper into the transmission mechanisms and dynamic characteristics of the disease, providing more precise and effective means for disease prevention and control. Regarding the research on mathematical models of leptospirosis, please refer to the literature [2,3,4,5,6]. These models analyze the factors that influence the transmission dynamics of leptospirosis, pointing out that disease transmission is not only related to the interaction between rodents and humans [4], but also to their contact with free bacteria in the environment [6]. They also demonstrate that adopting appropriate intervention mechanisms, such as reducing the transmission rate, increasing the recovery rate, reducing rodent populations, and reducing bacterial contamination in water sources, can greatly assist in reducing the spread of the disease in the population.
In the real world, infectious disease models are inevitably affected by environmental noise, and deterministic models alone cannot accurately reflect the dynamic behavior of the system when describing disease transmission processes. In recent years, most scholars have explored stochastic infectious disease models that consider environmental perturbations [7,8,9,10,11,12]. The research results indicate that random perturbations have a certain impact on the spread of diseases.
Therefore, it is highly necessary to further establish and study leptospirosis models that consider vector-environment interactions and random disturbances.
To establish the model, we make the following assumptions.
(ⅰ) Susceptible individuals who come into contact with infected vectors or free bacteria in the environment can become infected individuals, and susceptible vectors that come into contact with infected individuals or free bacteria in the environment can also become infected vectors.
(ⅱ) Infected individuals and vectors both release free bacteria into the environment.
(ⅲ) The host population Sh(t),Ih(t),Sh(t), vector population Sv(t),Iv(t), and the concentration of bacteria in the environment are all influenced by Gaussian white noise.
(ⅳ) The recruitment rate Λ and the birth rate Π of the vectors are constants. Every parameter within the system is a nonnegative real number.
Base on the above assumptions, we establish and study a stochastic model of leptospirosis with host-vector-environment interactions:
{dSh(t)=[Λ−μhSh−β1ShIvNh−β3ShBK+B+λhRh]dt+σ1ShdB1(t),dIh(t)=[β1ShIvNh+β3ShBK+B−μhIh−δhIh−γhIh]dt+σ2IhdB2(t),dRh(t)=[γhIh−λhRh−μhRh]dt+σ3RhdB3(t),dSv(t)=[Π−β2IhSvNh−β4SvBK+B−μvSv]dt+σ4SvdB4(t),dIv(t)=[β2IhSvNh+β4SvBK+B−μvIv]dt+σ5IvdB5(t),dB(t)=[α1Ih+α2Iv−kB]dt+σ6BdB6(t), | (1.1) |
where the host population, which represents the human population, is divided into three categories at time t: susceptible individuals Sh(t), infected individuals Ih(t), and recovered individuals Rh(t). The vector population is divided into susceptible vectors Sv(t) and infected vectors Iv(t) at time t. Additionally, B(t) represents the free-floating bacterial population in the environment. The meanings of the parameters are as follows. β1 and β2 represent the infection rates of diseased vectors transmitting the disease to humans and of infected humans transmitting the disease to vectors, respectively. β3 and β4 represent the rates at which susceptible humans and susceptible vectors become infected through contact with bacteria in the environment. μh and μv are natural mortality rate for the human population and the vector population, and γh represents the disease-induced mortality rate among humans. δh represents the recovery rate for infected humans, while λh represents the rate at which recovered humans revert back to the susceptible state. α1 and α2 represent the rates at which infected humans and infected vectors release bacteria into the environment, respectively. K serves as a half-saturation infection parameter, and k is the decay rate of bacteria in the environment. Bi(t)(i=1,2,3,4,5,6) are standard Brownian motions. Parameters σi(i=1,2,⋯,6) are the intensities of noise, representing variability and stochastic effects: σ1 represents the variability in the susceptible individuals Sh(t), which arise from fluctuating contact rates or changes in population behavior that affect exposure to the virus environment and infected vectors; σ2 reflects the random fluctuations in the number of the infected population Ih(t) due to variations in the disease's infectiousness, or response to treatment; σ3 represents stochastic factors affecting the recovered population Rh(t), such as loss of immunity or the impact of interventions; σ4 represents the variability in the susceptible vectors Sv(t), which arise from fluctuating contact rates or changes in population behavior that affect exposure to the Leptospira virus environment and infected individuals; σ5 reflects the random fluctuations in the number of the infected vectors Iv(t) due to variations in the disease¡¯s infectiousness; σ6 represents the random variation intensity of Leptospira virus B(t) released into the environment by infected humans or disease vectors.
We assume the initial conditions are
Sh(0)≥0,Ih(0)≥0,Rh(0)≥0,Sv(0)≥0,Iv(0)≥0,B(0)≥0. | (1.2) |
The aim of this paper is to build a stochastic model of leptospirosis that incorporates both vector-borne and environmental transmission to more comprehensively describe the disease's transmission characteristics. Furthermore, by combining this model with actual reported data on leptospirosis in China in recent years, we aim to estimate important parameters of the model using statistical methods and predict the future trends of leptospirosis in China.
To demonstrate that our proposed model is meaningful, we prove that there exists a unique global positive solution of the system (1.1).
Theorem 2.1. For any initial value (Sh(0),Ih(0),Rh(0),Sv(0),Iv(0),B(0))∈R6+, the system (1.1) has a unique positive solution (Sh(t),Ih(t),Rh(t),Sv(t),Iv(t),B(t)), and the solution will remain in R6+ with probability 1, i.e., (Sh(t),Ih(t),Rh(t),Sv(t),Iv(t),B(t))∈R6+ for all t>0 almost surly (a.s.).
Proof. Obviously, the system (1.1) has locally Lipschitz continuous coefficients, for any initial value (Sh(0),Ih(0),Rh(0),Sv(0),Iv(0),B(0))∈R6+, and the system (1.1) exists a unique maximal local solution (Sh(t),Ih(t),Rh(t),Sv(t),Iv(t),B(t)), t∈[0,τe), where τe is the explosion time. To verify that this solution of the system (1.1) is global, we just have to prove that τe=∞ a.s. For this, assume k0≥1 is large enough such that (Sh(0),Ih(0),Rh(0),Sv(0),Iv(0),B(0)) all fall within the interval [1/k0,k0]. For each integer k≥k0, define the stopping time as:
τk=inf{t∈[0,τe):Sh(t)∉(1k,korIh(t)∉(1k,korRh(t)∉(1k,k)orSh(t)∉(1k,korIv(t)∉(1k,k)orB(t)∉(1k,k)}, |
where inf∅=∞ (∅ denotes the empty set). Clearly, when k→∞, τk are increasing. Let τ∞=limk→∞τk, then τ∞≤τe a.s. If τ∞=∞ a.s. holds, then τe=∞ a.s., which means that (Sh(t),Ih(t),Rh(t),Sv(t),Iv(t),B(t))∈R6+ a.s. for t≥0. Therefore, it suffices to prove that τ∞=∞ a.s.
Next, we assume that there exist constants T>0 and ε∈(0,1), such that P{τ∞≤T}>ε, then, there exists an integer k1≥k0, such that for any k≥k1,
P{τk≤T}≥ε. | (2.1) |
Define the function Q:R6+→R+ as follows:
Q(Sh,Ih,Rh,Sv,Iv,B)=(Sh−a1−a1lnSha1)+(Ih−1−lnIh)+(Rh−1−lnRh)+(Sv−b1−b1lnSvb1)+(Iv−1−lnIv)+ln(1+1B), |
where a1,b1 are positive constants to be determined later. Obviously, the function u−1−lnu is non-negative for all u>0.
Applying Itô's formula, we obtain
dQ=LQdt+σ1(Sh−a1)dB1(t)+σ2(Ih−1)dB2(t)+σ3(Rh−1)dB3(t)+σ4(Sv−b1)dB4(t)+σ5(Iv−1)dB5(t)−σ61+BdB6(t), |
where
LQ=Λ−μh(Sh+Ih+Rh)−δhIh+Π−μv(Sv+Iv)−α1IhB(1+B)−α2IvB(1+B)+k1+B−a1ΛSh+a1μh+a1β1IvNh+a1β3BK+B−a1λhRhSh−β1ShIvNhIh−β3ShB(K+B)Ih+μh+δh+γh−γhIhRh+λh+μh−b1ΠSv+b1β2IhNh+b1β4BK+B+b1μv−β2SvIhNhIv−β4SvB(K+B)Iv+μv+12a1σ21+12σ22+12σ23+12b1σ24+12σ25+121+2B(1+B)2σ26≤Λ+Π+k+a1μh+(a1β1M1−μv)Iv+a1β3+μh+δh+γh+λh+μh+(b1β2M1−μh)Ih+b1β4+b1μv+μv+12a1σ21+12σ22+12σ23+12b1σ24+12σ25+12σ26. |
Choose a1=μvM1β1,b1=μhM1β2, such that a1β1M1−μv=0,b1β2M1−μh=0, and
LQ≤Λ+Π+k+a1μh+a1β3+μh+δh+γh+λh+μh+b1β4+b1μv+μv+12a1σ21+12σ22+12σ23+12b1σ24+12σ25+12σ26:=K, |
where K>0 is a constant. The remainder of the proof follows the similar approach given in [13].
Now, the sufficient conditions for the elimination of Ih,Iv are presented. Denote ⟨f⟩=1t∫t0f(s)ds, and the parameter as follows:
Rm=(β1+β2+β4)μhΠ+β3μvΛμhμv(Λ+Π)+(δh+γh)μvΛ. |
To facilitate the proof of the theorem, we first give a related lemma.
Lemma 2.1. [14,15,16] For any initial value (Sh(0),Ih(0),Rh(0),Sv(0),Iv(0),B(0))∈R6+, the solution (Sh(t),Ih(t),Rh(t),Sv(t),Iv(t),B(t))∈R6+ of model (1.1) possesses the following properties:
limt→∞∫t0Sh(s)dB1(s)t=0, limt→∞∫t0Ih(s)dB2(s)t=0, limt→∞∫t0Rh(s)dB3(s)t=0, |
limt→∞∫t0Sv(s)dB4(s)t=0, limt→∞∫t0Iv(s)dB5(s)t=0, limt→∞∫t0B(s)dB6(s)t=0 a.s. |
Proof of Lemma 2.1 can be similarly obtained by following the proof of Lemma 2.2 in reference [14]. The details are omitted here.
Theorem 2.2. Assume (Sh(t),Ih(t),Rh(t),Sv(t),Iv(t),B(t))∈R6+ is the solution of model (1.1) that satisfies the initial condition (Sh(0),Ih(0),Rh(0),Sv(0),Iv(0),B(0))∈R6+. If Rm<1, then (Ih(t),Iv(t),B(t)) converges to (0,0,0) exponentially with probability one (a.s.), indicating the elimination of the disease, and furthermore,
limt→∞Sh(t)=Λμh, limt→∞Sv(t)=Πμv, limt→∞Rh(t)=0 a.s. |
Proof. Let P(t)=Ih(t)+Iv(t). Applying Itô's formula, we have
dP(t)=[β1ShNhIv+β3ShBK+B−μhIh−δhIh−γhIh+β2IhNhSv+β4SvBK+B−μvIv]dt+σ2IhdB2(t)++σ5IvdB5(t). | (2.2) |
Integrating both sides of (2.2) from 0 to t and dividing by t, we obtain
P(t)t=P(0)t+β1⟨ShNhIv⟩+β3⟨ShBK+B⟩−(μh+δh+γh)⟨Ih⟩+β2⟨IhNhSv⟩+β4⟨SvBK+B⟩−μv⟨Iv⟩+1t∫t0σ2Ih(s)dB2(s)+1t∫t0σ5Iv(s)dB5(s)≤P(0)t+β1⟨Iv⟩+β3⟨Sh⟩−(μh+δh+γh)⟨Ih⟩+β2⟨Sv⟩+β4⟨Sv⟩−μv⟨Iv⟩+1t∫t0σ2Ih(s)dB2(s)+1t∫t0σ5Iv(s)dB5(s). | (2.3) |
Notice
d(Sh(t)+Ih(t)+Rh(t))≤[Λ−μ(Sh+Ih+Rh)]dt+σ1Sh(t)dB1(t)+σ2Ih(t)dB2(t)+σ3Rh(t)dB3(t) | (2.4) |
and
d(Sv(t)+Iv(t))=[Π−μv(Sv+Iv)]dt+σ4Sv(t)dB4(t)+σ5Iv(t)dB5(t). | (2.5) |
Integrating both sides of (2.4) and (2.5) from 0 to t and dividing by t, then, taking the upper limit, we obtain
lim supt→∞⟨Sh(t)+Ih(t)+Rh(t)⟩≤Λμh a.s. |
lim supt→∞⟨Sv(t)+Iv(t)⟩=Πμv a.s. |
Thus
lim supt→∞⟨Sh(t)⟩≤Λμh, lim supt→∞⟨Ih(t)⟩≤Λμh, lim supt→∞⟨Rh(t)⟩≤Λμh a.s. |
lim supt→∞⟨Sv(t)⟩≤Πμv, lim supt→∞⟨Iv(t)⟩≤Πμv a.s. |
Taking the upper limit of both sides of (2.3), and according to Lemma 2.1, we can obtain the desired result
lim supt→∞P(t)t≤β1⋅Πμv+β3⋅Λμh−(μh+δh+γh)⋅Λμh+β2⋅Πμv+β4⋅Πμv−μv⋅Πμv=μh(Λ+Π)+(δh+γh)Λμh(Rm−1)<0. |
Then
limt→∞P(t)=0. |
Hence
limt→∞Ih(t)=0, limt→∞Iv(t)=0. |
For the sixth equation in (1.1), by integrating both sides from 0 to t, dividing by t, and then taking the upper limit, we can derive that limt→∞B(t)=0.
Similarly, applying the same method to the third equation in (1.1), we can obtain limt→∞Rh(t)=0.
Since
d(Sh(t)+Ih(t))=[Λ−μhSh−μhIh−δhIh−γhIh+λhRh]dt+σ1Sh(t)dB1(t)+σ2Ih(t)dB2(t), |
based on the conclusions obtained above, we can derive that limt→∞Sh(t)=Λμh. Similarly, we can obtain that limt→∞Sv(t)=Πμv.
To better analyze the impact of different parameters on the spread of infectious diseases on the surface, we will proceed with a further parameter sensitivity analysis. We conduct 1000 samplings of the parameters using the Latin Hypercube Sampling (LHS) method [17]. By calculating the Partial Rank Correlation Coefficient (PRCC), we will be able to screen out the parameters that have a significant impact on the population size. This will help us identify more accurate measures to control the epidemic.
Observing Figure 1, it is evident that the parameters with significant impacts on disease transmission are β3,δh,γh,μv. Here, β3 is positively correlated with Rm, while δh,γh,μv are negatively correlated with Rm. In other words, the smaller the contact rate of humans with free bacteria in the environment, the higher the human mortality rate due to the disease and the natural mortality rate of the vector population; and the faster the recovery rate from the disease, the smaller the basic reproduction number will be, making it easier to eliminate the disease. In fact, as the contact rate of humans with free bacteria in the environment declines, so does the likelihood of contracting the virus. Similarly, when the mortality rate stemming from the illness is high, infected individuals may perish during the infection period, thereby diminishing their capacity to spread the disease to others, resulting in a lower average transmission rate per infected individual. Furthermore, a high natural mortality rate among vectors lessens their chances of transmitting the disease to humans and curtails the release of virus particles into the environment. Lastly, an increase in the recovery rate of infected individuals reduces their chances of transmitting the disease to vectors. All these scenarios contribute significantly to a decrease in the Rm value.
Next, we perform numerical simulations on the system (1.1) by using the high-order Milstein method mentioned in [18,19], which is based on the concept of Itô's formula and stochastic Taylor expansion. The Milstein method improves the accuracy of the estimates by introducing higher-order infinitesimals. Compared to the Euler-Maruyama method, the Milstein method is more precise. However, the Milstein method requires the stochastic process to be twice differentiable, which can make its implementation more complex. It is primarily suitable for stochastic differential equations with continuous sample paths. For stochastic differential equations with discontinuous sample paths or jump processes, other types of numerical methods may be required.
Assuming an initial condition of (Sh(0),Ih(0),Rh(0),Sv(0),Iv(0),B(0))=(400,100,150,500, 120, 1000), the specific parameter values are as follows: Λ=35day−1, Π=30day−1, β1=0.004day−1, β2=0.001day−1, β3=0.003day−1, β4=0.002day−1, λh=0.1day−1, δh=0.6day−1, μh=0.01day−1, μv=0.1day−1, K=10cells⋅ml−1, k=0.5day−1, α1=0.08cells⋅ml−1⋅day−1, α2=0.09cells⋅ml−1⋅day−1 and γh=0.7day−1.
Figures 2 and 3 demonstrate the specific time-varying situation of the number of infected individuals or infected vectors when these four parameters β3,δh,γh,μv change, while other parameters remain unchanged, respectively. From these two figures, it can be observed that despite changes in the parameters, both the infected population and the infected vectors ultimately go extinct, but the time of extinction differs. Specifically, as β3 decreases, the extinction time of Ih shortens. Similarly, when δh and γh increase, the extinction time of Ih decreases. Additionally, as μv increases, the extinction time of Iv also shortens.
In this section, we utilize the reported leptospirosis case data in China from 2003 to 2021 to predict the future epidemic situation of the disease. The data comes from China's statistical Yearbook [20], as shown in Figure 4. The population recruitment rate of Λ=7.74×106 is estimated based on China's population statistics from 2003 to 2021, the natural death rate of humans is μh=0.0064, and the number of newly reported leptospirosis cases in 2003 was 1728 [20]. Assuming that the recruitment rate of vectors carrying leptospira is Π=1.0812×105, these vectors are susceptible to external factors that can lead to death, with a natural death rate of μv=0.8125 [21]. The specific values of the parameters are listed in Table 1.
Parameter | Parameter value | Source | Parameter | Parameter value | Source |
Λ | 7.74×106 year−1 | [20] | Π | 1.0812×105 year−1 | [22] |
[1mm] β2 | 1×10−5 year−1 | Fitted | β4 | 1×10−5 year−1 | Fitted |
K | 4.65×108 cells⋅ml−1 | Fitted | k | 0.162 year−1 | [20] |
μh | 0.0064 year−1 | [20] | μv | 0.8125 year−1 | [21] |
α1 | 3 cells⋅ml−1⋅year−1 | [20] | α2 | 100 cells⋅ml−1⋅year−1 | Fitted |
λh | 0.08082 year−1 | [21] | δh | 0.03328 year−1 | [23] |
γh | 0.08889 year−1 | [23] |
Let the cumulative number of leptospirosis cases in the human population be defined as Dh(t), and
dDh(t)dt=β1ShIvNh+β3ShBK+B. | (3.1) |
To predict the disease, it is necessary to first estimate the two important parameters that affect the spread of the disease, namely, β1,β3. We utilize the numerical solution Dh(t) from model (3.1) to fit the data. Let Θ(β1,β3) represent the vector of parameters to be estimated, and Dh(t,Θ) represent the numerical solution of model (3.1) corresponding to the parameters Θ. The vector Y(Yk,k=1,2,3,...,19) represents the 19 statistical data points, and tk is the corresponding time for each data point. Take the initial value of the variable as (Sh(0),Ih(0),Rh(0),Sv(0),Iv(0),B(0),Dh(0))=(7.74×106,1728,307,1.0812×105,1.867×103,1.42×102,1728), and the initial value of the parameter (β1,β3)=(3.2326×10−3,1.2×10−4). Random disturbance intensities are taken as σ1=σ2=σ3=σ4=σ5=σ6=0.1. We estimate the parameters using two methods below: one is the least squares method, and the other is the Markov Chain Monte Carlo (MCMC) method.
1) The least squares method (LSM). The goal is to find the optimal values of Θ(β1,β3) that minimize the least squares criterion:
LS=19∑k=1|Dh(tk,Θ)−Yk|2. | (3.2) |
To achieve this, we utilize the fmincon command in the mathematical software MATLAB for numerical optimization. Based on the biological background, we set the ranges of Θ to be ((0,0),[0.5,0.5]), which serve as the constraint conditions. Using the optimization algorithm, we obtain the estimated values of the parameters. Then, we run the program 100 times and calculate the average of the output parameters β1=0.0032308,β3=0.00011993, which serve as the required parameter estimates. Figure 5(a), (b) present numerical simulations of the cumulative number of leptospirosis cases in 100 sample paths and their mean output path, respectively.
2) Markov Chain Monte Carlo-Metropolis Hastings method (MCMC-MH). Now, we estimate the parameters using the MCMC parameter estimation method combined with MH sampling. Let Θ(β1,β3) be the proposed parameter and Θ′(β1,β3) be the current parameter. The proposed parameter follows Θ=Θ′+ε, where ε is the step size of random walk that follows a uniform distribution. According to Bayesian statistical inference, the posterior distribution is given by:
P(Θ|Y)=L(Y|Θ)P(Θ), | (3.3) |
where the likelihood function is L(Y|Θ)=−19∑k=1|Dh(tk,Θ)−Yk|2, and P(Θ) is the non-informative prior distribution, assumed to be a constant C. The acceptance probability is defined as: α(Θ,Θ′)=min{1,exp(L(Y|Θ)−L(Y|Θ′))}. The ranges of Θ are also ((0,0), [0.5,0.5]). After performing 5000 iterations of MCMC calculations, with a burn-in period of 1000 iterations, we computed the average of the last 4000 iterations to obtain the estimated values of the parameters as β1=0.0050193,β3=0.000096193. The 95 percent confidence interval for β1 and β3 is (1.432×10−3−9.941×10−3), (1.5036×10−5−2.2604×10−4), respectively. By substituting the estimated parameters into the model (3.1), we can obtain any 100 paths of Dh(t). Figure 6(a), (b) present numerical simulations of the cumulative number of leptospirosis cases in 100 sample paths and their mean output path, respectively. Figure 6(c), (d) show the posterior distribution plots and trace plots for β1, β3, respectively.
It can be seen from Figures 5 and 6 that both simulation results of the model (3.1) by two methods match the cumulative data of leptospirosis cases in China from 2003 to 2021. Next, we calculate the error value between the average curve and the real data, and compare the results from both two methods. It can be seen from Table 2 that the parameter values estimated by the two methods are very close, but the estimation error by the MCMC-MH method is smaller than LSM. Finally, using the parameters estimated by the MCMC-MH method, we calculate the basic reproduction number for the transmission of leptospirosis in China, Rm≈0.00075197<1, and predict that leptospirosis will be eliminated in China in 26 years (see Figure 7).
Method | The estimated value of β1 | The estimated value of β3 | MAPE | RSME |
LSM | 0.0032308 | 0.00011993 | 0.6236 | 4190.7348 |
MCMC | 0.0050193 | 0.000096193 | 0.61821 | 3968.3587 |
This article establishes a stochastic leptospirosis model with both vector and environmental transmission. Through mathematical analysis of the model, a threshold for disease elimination is derived. Then, using the partial rank correlation coefficient, an impact analysis was conducted on the model parameters to identify the key parameters that have a significant influence on disease elimination. Furthermore, a sensitivity analysis of these parameters was carried out through numerical simulations, which further revealed the mechanisms of their role in the disease transmission process. This analytical approach provides a powerful tool for gaining a deeper understanding of how model parameters affect disease transmission. In the end, using data from China's leptospirosis case reports from 2003 to 2021, two parameter estimation methods, LSM and MCMC-MH, are applied to estimate the crucial parameters of the model. The simulation results of the number of infections in model (1.1) using parameters obtained from two parameter estimation methods align well with the cumulative data of leptospirosis cases in China from 2003 to 2021. It is predicted that under the current control measures, leptospirosis in China will be completely eliminated after 26 years.
Common leptospirosis models [3,5,22] tend to only consider the interaction between hosts and vectors, overlooking the influence of environmental factors. In this paper, by incorporating environmental transmission factors into the model design and considering environmental disturbance, we construct a more comprehensive and realistic stochastic infectious disease model, providing a new perspective for a more accurate understanding of the transmission mechanisms of leptospirosis. Specifically, the parameter estimation method used in this article, which combines MH sampling with MCMC, has served as a good demonstration for parameter estimation in stochastic differential systems with numerous parameters. This approach of combining actual data with parameter estimation not only enhances the accuracy and reliability of the model, but also provides strong support for predicting the future trends of leptospirosis in China. The stochastic model of leptospirosis and its related analysis methods established in this article have important theoretical and practical significance for understanding the transmission patterns of other similar vector-borne diseases and predicting future epidemic trends.
However, it must be said that when we make predictions, we only estimate two important parameters, and some parameters are based on subjective assumptions fitted to the data, which may reduce the accuracy of the prediction. In addition, the model does not fully consider the impact of human behavior, socioeconomic factors, and climate change on disease transmission. The neglect of these factors may limit the accuracy and applicability of the model. In the future, we will incorporate human behavior, socioeconomic factors, and climate change into our model, and strive to utilize actual data to estimate more parameters in order to improve the accuracy and applicability of the model. This will help us gain a deeper understanding of the dynamics of disease transmission and design effective interventions to protect public health.
The authors declare they have not used Artificial Intelligence (AI) tools in the creation of this article.
This work is sponsored by Nanhu Scholars Program for Young Scholars of XYNU.
The authors declare there is no conflicts of interest.
[1] | L. Liu, H. Zhu, G. Yang, Current situation of endemic status, prevention and control of neglected zoonotic diseases in China (in Chinese), Chin. J. Schistosomiasis Control, 25 (2013), 307–311. |
[2] |
H. T. Alemneh, A co-infection model of dengue and leptospirosis diseases, Adv. Differ. Equations, 2020 (2020), 664–687. https://doi.org/10.1186/s13662-020-03126-6 doi: 10.1186/s13662-020-03126-6
![]() |
[3] | A. Bhalraj, A. Azmi, M. H. Mohd, Analytical and numerical solutions of leptospirosis model, Comput. Sci., 16 (2021), 949–961. |
[4] |
M. A. Gallego, M. V. Simoy, Mathematical modeling of leptospirosis: a dynamic regulated by environmental carrying capacity, Chaos, Solitons Fractals, 152 (2021), 111425. https://doi.org/10.1016/j.chaos.2021.111425 doi: 10.1016/j.chaos.2021.111425
![]() |
[5] |
H. A. Engida, D. M. Theuri, D. Gathungu, J. Gachohi, H. T. Alemneh, A mathematical model analysis for the transmission dynamics of leptospirosis disease in human and rodent populations, Comput. Math. Methods Med., 2022 (2022), 1806585. https://doi.org/10.1155/2022/1806585 doi: 10.1155/2022/1806585
![]() |
[6] |
D. Baca-Carrasco, D. Olmos, I. Barradas, A mathematical model for human and animal leptospirosis, J. Biol. Syst., 23 (2015), S55–S65. https://doi.org/10.1142/S0218339015400057 doi: 10.1142/S0218339015400057
![]() |
[7] |
D. Zhou, X. Y. Shi, X. Y. Zhou, Dynamic analysis of a stochastic delayed SEIRS epidemic model with Lévy jumps and the impact of public health education, Axioms, 12 (2023), 560. https://doi.org/10.3390/axioms12060560 doi: 10.3390/axioms12060560
![]() |
[8] |
J. Djordjevic, J. C. Silva, F. D. Torres, A stochastic SICA epidemic model for HIV transmission, Appl. Math. Lett., 84 (2018), 168–175. https://doi.org/10.1016/j.aml.2018.05.005 doi: 10.1016/j.aml.2018.05.005
![]() |
[9] |
Y. Zhao, D. Jiang, The threshold of a stochastic SIS epidemic model with vaccination, Appl. Math. Comput., 243 (2014), 718–727. https://doi.org/10.1016/j.amc.2014.05.124 doi: 10.1016/j.amc.2014.05.124
![]() |
[10] |
X. Meng, S. Zhao, T. Feng, T. Zhang, Dynamics of a novel nonlinear stochastic SIS epidemic model with double epidemic hypothesis, J. Math. Anal. Appl., 433 (2016), 227–242. https://doi.org/10.1016/j.jmaa.2015.07.056 doi: 10.1016/j.jmaa.2015.07.056
![]() |
[11] |
A. Din, Bifurcation analysis of a delayed stochastic HBV epidemic model: Cell-to-cell transmission, Chaos, Solitons Fractals, 181 (2024), 114714. https://doi.org/10.1016/j.chaos.2024.114714 doi: 10.1016/j.chaos.2024.114714
![]() |
[12] |
A. Din, Y. Li, A. Yusuf, Delayed hepatitis B epidemic model with stochastic analysis, Chaos, Solitons Fractals, 146 (2021), 110839. https://doi.org/10.1016/j.chaos.2021.110839 doi: 10.1016/j.chaos.2021.110839
![]() |
[13] |
X. Mao, G. Marion, E. Renshaw, Environmental Brownian noise suppresses explosions in population dynamics, Stochastic Processes Appl., 97 (2002), 95–110. https://doi.org/10.1016/S0304-4149(01)00126-0 doi: 10.1016/S0304-4149(01)00126-0
![]() |
[14] |
Y. Zhao, D. Jiang, The threshold of a stochastic SIS epidemic model with vaccination, Appl. Math. Comput., 243 (2014), 718–727. https://doi.org/10.1016/j.amc.2014.05.124 doi: 10.1016/j.amc.2014.05.124
![]() |
[15] |
Q. T. Ain, Nonlinear stochastic cholera epidemic model under the influence of noise, J. Math. Tech. Model., 1 (2024), 52–74. https://doi.org/10.56868/jmtm.v1i1.30 doi: 10.56868/jmtm.v1i1.30
![]() |
[16] | R. Khasminskii, Stochastic Stability of Differential Equations, Springer Berlin, Heidelberg, 2011. |
[17] |
S. Marino, I. B. Hogue, C. Ray, et al., A methodology for performing global uncertainty and sensitivity analysis in systems biology, J. Theor. Biol., 254 (2009), 178–196. https://doi.org/10.1016/j.jtbi.2008.04.011 doi: 10.1016/j.jtbi.2008.04.011
![]() |
[18] |
D. J. Higham, An algorithmic introduction to numerical simulation of stochastic differential equations, SIAM Rev., 43 (2001), 525–546. https://doi.org/10.1137/S0036144500378302 doi: 10.1137/S0036144500378302
![]() |
[19] |
Q. T. Ain, J. Shen, P. Xu, X. Qiang, Z. Kou, A stochastic approach for co-evolution process of virus and human immune system, Sci. Rep., 14 (2024), 10337. https://doi.org/10.1038/s41598-024-60911-z doi: 10.1038/s41598-024-60911-z
![]() |
[20] | China's Statistical Yearbook, the National Bureau of Statistics of China, 2003–2021. Available from: https://www.stats.gov.cn/sj/ndsj/. |
[21] | W. Triampo, D. Baowan, I. M. Tang, N. Nuttavut, J. Wong-Ekkabut, G. Doungchawee, A simple deterministic model for the spread of leptospirosis in Thailand, Int. J. Bio. Med. Sci., 2 (2007), 22–26. |
[22] |
M. A. Khan, S. Islam, S. A. Khan, G. Zaman, Global stability of vector-host disease with variable population size, Biomed Res. Int., 2013 (2013), 710917. http://dx.doi.org/10.1155/2013/710917 doi: 10.1155/2013/710917
![]() |
[23] | W. Tangkanakul, H. L. Smits, S. Jatanasen, D. A. Ashford, Leptospirosis: an emerging health problem in Thailand, Southeast Asian J. Trop. Med. Public Health, 36 (2005), 281–288. |
Parameter | Parameter value | Source | Parameter | Parameter value | Source |
Λ | 7.74×106 year−1 | [20] | Π | 1.0812×105 year−1 | [22] |
[1mm] β2 | 1×10−5 year−1 | Fitted | β4 | 1×10−5 year−1 | Fitted |
K | 4.65×108 cells⋅ml−1 | Fitted | k | 0.162 year−1 | [20] |
μh | 0.0064 year−1 | [20] | μv | 0.8125 year−1 | [21] |
α1 | 3 cells⋅ml−1⋅year−1 | [20] | α2 | 100 cells⋅ml−1⋅year−1 | Fitted |
λh | 0.08082 year−1 | [21] | δh | 0.03328 year−1 | [23] |
γh | 0.08889 year−1 | [23] |
Method | The estimated value of β1 | The estimated value of β3 | MAPE | RSME |
LSM | 0.0032308 | 0.00011993 | 0.6236 | 4190.7348 |
MCMC | 0.0050193 | 0.000096193 | 0.61821 | 3968.3587 |
Parameter | Parameter value | Source | Parameter | Parameter value | Source |
Λ | 7.74×106 year−1 | [20] | Π | 1.0812×105 year−1 | [22] |
[1mm] β2 | 1×10−5 year−1 | Fitted | β4 | 1×10−5 year−1 | Fitted |
K | 4.65×108 cells⋅ml−1 | Fitted | k | 0.162 year−1 | [20] |
μh | 0.0064 year−1 | [20] | μv | 0.8125 year−1 | [21] |
α1 | 3 cells⋅ml−1⋅year−1 | [20] | α2 | 100 cells⋅ml−1⋅year−1 | Fitted |
λh | 0.08082 year−1 | [21] | δh | 0.03328 year−1 | [23] |
γh | 0.08889 year−1 | [23] |
Method | The estimated value of β1 | The estimated value of β3 | MAPE | RSME |
LSM | 0.0032308 | 0.00011993 | 0.6236 | 4190.7348 |
MCMC | 0.0050193 | 0.000096193 | 0.61821 | 3968.3587 |