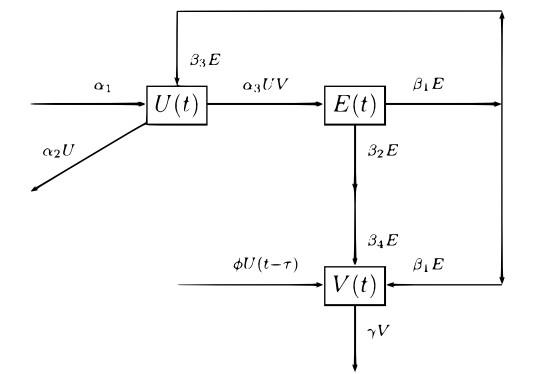
This paper considers the maneuvering penetration methods of missile which do not know the intercepting strategies of the interceptor beforehand. Based on reinforcement learning, the online intelligent maneuvering penetration methods of missile are derived. When the missile is locked by the interceptor, in terms of the tracking characteristics of the interceptor, the missile carries out tentative maneuvers which lead to the interceptor makes the responses respectively, in the light of the information on interceptor responses which can be gathered by the missile-borne detectors, online game confrontation learning is employed to increase the miss distance of the interceptor in guidance blind area by reinforcement learning algorithm, the results of which are used to generate maneuvering strategies that make the missile to achieve the successful penetration. The simulation results show that, compared with no maneuvering methods or random maneuvering methods, the methods proposed not only present higher probability of successful penetration, but also need less overload and lower command switching frequency. Moreover, the effectiveness of this maneuvering penetration methods can be realized under the condition of limited number of training.
Citation: Yaokun Wang, Kun Zhao, Juan L. G. Guirao, Kai Pan, Huatao Chen. Online intelligent maneuvering penetration methods of missile with respect to unknown intercepting strategies based on reinforcement learning[J]. Electronic Research Archive, 2022, 30(12): 4366-4381. doi: 10.3934/era.2022221
[1] | Bruno Buonomo, Deborah Lacitignola . On the stabilizing effect of cannibalism in stage-structured population models. Mathematical Biosciences and Engineering, 2006, 3(4): 717-731. doi: 10.3934/mbe.2006.3.717 |
[2] | Yuxuan Zhang, Xinmiao Rong, Jimin Zhang . A diffusive predator-prey system with prey refuge and predator cannibalism. Mathematical Biosciences and Engineering, 2019, 16(3): 1445-1470. doi: 10.3934/mbe.2019070 |
[3] | Wei Feng, Michael T. Cowen, Xin Lu . Coexistence and asymptotic stability in stage-structured predator-prey models. Mathematical Biosciences and Engineering, 2014, 11(4): 823-839. doi: 10.3934/mbe.2014.11.823 |
[4] | Jessica L. Hite, André M. de Roos . Pathogens stabilize or destabilize depending on host stage structure. Mathematical Biosciences and Engineering, 2023, 20(12): 20378-20404. doi: 10.3934/mbe.2023901 |
[5] | Shunyi Li . Hopf bifurcation, stability switches and chaos in a prey-predator system with three stage structure and two time delays. Mathematical Biosciences and Engineering, 2019, 16(6): 6934-6961. doi: 10.3934/mbe.2019348 |
[6] | Lazarus Kalvein Beay, Agus Suryanto, Isnani Darti, Trisilowati . Hopf bifurcation and stability analysis of the Rosenzweig-MacArthur predator-prey model with stage-structure in prey. Mathematical Biosciences and Engineering, 2020, 17(4): 4080-4097. doi: 10.3934/mbe.2020226 |
[7] | Fang Liu, Yanfei Du . Spatiotemporal dynamics of a diffusive predator-prey model with delay and Allee effect in predator. Mathematical Biosciences and Engineering, 2023, 20(11): 19372-19400. doi: 10.3934/mbe.2023857 |
[8] | Tingting Ma, Xinzhu Meng . Global analysis and Hopf-bifurcation in a cross-diffusion prey-predator system with fear effect and predator cannibalism. Mathematical Biosciences and Engineering, 2022, 19(6): 6040-6071. doi: 10.3934/mbe.2022282 |
[9] | John Cleveland . Basic stage structure measure valued evolutionary game model. Mathematical Biosciences and Engineering, 2015, 12(2): 291-310. doi: 10.3934/mbe.2015.12.291 |
[10] | G.A.K. van Voorn, D. Stiefs, T. Gross, B. W. Kooi, Ulrike Feudel, S.A.L.M. Kooijman . Stabilization due to predator interference: comparison of different analysis approaches. Mathematical Biosciences and Engineering, 2008, 5(3): 567-583. doi: 10.3934/mbe.2008.5.567 |
This paper considers the maneuvering penetration methods of missile which do not know the intercepting strategies of the interceptor beforehand. Based on reinforcement learning, the online intelligent maneuvering penetration methods of missile are derived. When the missile is locked by the interceptor, in terms of the tracking characteristics of the interceptor, the missile carries out tentative maneuvers which lead to the interceptor makes the responses respectively, in the light of the information on interceptor responses which can be gathered by the missile-borne detectors, online game confrontation learning is employed to increase the miss distance of the interceptor in guidance blind area by reinforcement learning algorithm, the results of which are used to generate maneuvering strategies that make the missile to achieve the successful penetration. The simulation results show that, compared with no maneuvering methods or random maneuvering methods, the methods proposed not only present higher probability of successful penetration, but also need less overload and lower command switching frequency. Moreover, the effectiveness of this maneuvering penetration methods can be realized under the condition of limited number of training.
Unemployment is a serious problem for many nations since the problem is related to economic and social factors such as inflation, increase/decrease in GDP, and economic depression. Moreover, prolonged unemployment can lead to an erosion of skill, a negative effect on mental health, and worsen the physical health of unemployed people. For these reasons, the government of each country has been attempting to reduce the number of unemployed persons. A goal of many governments is full employment. There are various policies used to solve the problem such as monetary policy and fiscal policy. The government intervention helps to speed up the matching process between unemployed persons and entrepreneurs through the support of vacancies creation. Mathematical models for unemployment are one of the tools that are being used to introduce methods that can be used to manage the unemployment problem.
To understand the dynamic system of unemployment, we have followed up on the following work. In [21], Nikolopoulos and Tzanetis introduced and analyzed a mathematical model for a housing allocation of homeless families because of a natural disaster. Based on some concept of [21], Misra and Singh [15,16] introduced and analyzed mathematical models for the unemployment problem and focused on the stability analysis of models. In [15], the authors proposed a nonlinear differential equation of the unemployment model. For this study, three variables have been considered, namely, the numbers of unemployed, temporarily employed, and regularly employed persons. They assumed that the number of unemployed persons is continuously increasing due to graduation and it decreases because unemployed persons migrate and get a job. Moreover, the total numbers of each available vacancies are assumed to be constants. Furthermore, they assumed that employed persons become unemployed persons because of layoff, and the number of employed person decreases due to retirement. In [16], based on their previous work, the authors proposed a nonlinear dynamical system with time delay. In their study, a mathematical model with three variables has been proposed, namely, the numbers of unemployed persons, employed persons, and newly created vacancies. Besides, they assumed that firms may reduce vacancies because of a lack of financial resources. Moreover, they assumed that the government helps to support vacancies creation and the new vacancies are determined by considering previously collected data of unemployment, namely, a time delay is incorporated in creating new vacancies. Therefore, the model is presented by delay differential equations. In [19], Munoli and Gani proposed a dynamic unemployment model based on concept of [15,16]. They assumed that the new vacancies are determined by considering the number of unemployed persons. They also assumed that vacancies are incurred due to layoffs and retirement. The model without time delay is considered by three states, namely, the number of employed persons, unemployed persons, and vacancies. The optimal control analysis of the model was derived. In [8,9,17,20,23,25], the authors have constructed and analyzed the models of unemployment based on the above-mentioned works. In [13,18,22,24,28,31,32], the authors have studied and analyzed some viral infection models with intracellular delays. Some authors have focused on the local asymptotic stability and Hopf-bifurcation by taking delay as a bifurcating parameter, such as [22,24,28]. On the other hand, some authors have focused on the global asymptotic stability by using the Lyapunov functional technique and LaSalle theorem, such as [13,18,31,32].
Based on the concepts of [15,16,19], we have developed an unemployment model, taking into consideration the role of government for the support of vacancies creation. In this study, we modify the unemployment model of Munoli and Gani with new assumptions. The model is considered by three variables, namely, the number of unemployed persons, employed persons, and vacancies. Under some additional assumptions, we assume that workers are dismissed and they may become unemployed persons, migrate to other countries, or change to a business owner. We also assume that vacancies are incurred due to retirement and some job positions are maintained and job positions are not terminated. Moreover, we assume that the new vacancies are incurred by the government policy and the government uses previously collected data of unemployment to determine the new vacancies. In this paper, the unemployment model is presented by delay differential equations. We have analyzed the local and global stability of the system. Numerical results are given to illustrate the effectiveness of theoretical results. Furthermore, we have observed behavior of solutions of the system when some parameters are varied.
Let U(t), E(t), and V(t) be the number of unemployed persons, employed persons, and vacancies at time t, respectively. In this study, we assume the following:
(ⅰ) all entrants to unemployment class are fully qualified for doing any job;
(ⅱ) the unemployed persons are continuously increasing in number with a constant rate;
(ⅲ) the unemployed persons migrate from their local place to other places and the migration rate of unemployed persons is assumed to be proportional to their number;
(ⅳ) the rate of movement from the unemployment class to the employed class is jointly proportional to the numbers of unemployed persons and available vacancies;
(ⅴ) the employed persons may leave their jobs because of layoff and join the unemployed class;
(ⅵ) layoff of employed persons is regarded as for vacancies. They were dismissed to become unemployed persons but some persons do not become unemployed persons because they may migrate to other countries or become a business owner;
(ⅶ) the employed persons may leave their jobs because of retirement and vacancies are incurred after they are retired but some job positions may be terminated;
(ⅷ) firms may reduce vacancies because of lack of financial resources and the reduction rate of vacancies is assumed to be proportional to their numbers;
(ⅸ) the new vacancies are created at a rate proportional to the number of unemployed persons and time delay is incorporated in creating new vacancies.
The flow schematic diagram and parameters of the model considered in this study are given in Figure 1 and Table 1, respectively. In view of the above consideration, the mathematical model may be written as follows:
˙U(t)=α1−α2U(t)−α3U(t)V(t)+β3E(t)˙E(t)=α3U(t)V(t)−β1E(t)−β2E(t)˙V(t)=β1E(t)+β4E(t)+ϕU(t−τ)−γV(t) | (2.1) |
Parameters | Explanation |
α1 | The constant rate at which the number of unemployed persons |
is increasing continuously | |
α2 | The migration rate of unemployed persons |
α3 | The rate at which the unemployed persons are becoming employed persons |
β1 | The layoff rate |
β2 | The rate of retirement |
β3 | The rate at which the persons are dismissed become the unemployed persons |
β4 | The rate of vacancies because of retirement |
ϕ | The rate of creating new vacancies |
γ | The reduction rate of vacancies because of lack of financial resources |
τ | Time delay as point of past time |
where β1>β3 and β2>β4.
The initial conditions and history functions for system (2.1) are assumed to be of the forms:
U(ζ)=Φ1(ζ),E(ζ)=Φ2(ζ),V(ζ)=Φ3(ζ),Φ1(ζ)≥0,Φ2(ζ)≥0,Φ3(ζ)≥0,ζ∈[−τ,0),Φ1(0)>0,Φ2(0)>0,Φ3(0)>0, |
where (Φ1(ζ),Φ3(ζ),Φ3(ζ))∈C([−τ,0),R3+).
In this section, we analyze the system (2.1) using the stability theory of differential equations [6,10,14] and delay differential equations [5,7,11,27]. The following Lemmas show that we can find the region of attraction and the positive equilibrium points of the system (2.1), respectively.
Lemma 3.1. The set Ω={(U,E,V):0≤U+E≤α1δ1,0≤V(t)≤δ2α1δ1γ}, where δ1=min{α2,β1+β2−β3} and δ2=max{β1+β4,ϕ} is a region of attraction for the system (2.1) and attracts all solution initiating in the interior of positive octant.
Proof. From the system (2.1), we have
˙U(t)+˙E(t)=α1−α2U(t)−(β1+β2−β3)E(t). |
Let δ1=min{α2,β1+β2−β3}, we obtain
ddt[U(t)+E(t)]≤α1−δ1[U(t)+E(t)]. |
This implies
limt→+∞sup[U(t)+E(t)]≤α1δ1. |
Consider the third equation of system (2.1) and let δ2=max{β1+β4,ϕ}, we get
˙V(t)≤δ2α1δ1−γV(t). |
Therefore,
limt→+∞sup V(t)≤δ2α1δ1γ. |
Lemma 3.2. Suppose A=α2α3(β1+β4)−α3(β1+β2−β3)ϕ, B=α1α3(β1+β4)+α2γ(β1+β2), C=α1γ(β1+β2), ˉD=(β1+β2)γ−(β1+β4)α3ˉU and ˇD=(β1+β2)γ−(β1+β4)α3ˇU. Then we have the following:
(i) If A=0 holds, then positive equilibrium point E1(ˆU,ˆE,ˆV) exists, where
ˆU=CB,ˆE=α3ˆUˆVβ1+β2,ˆV=ϕα1γα2. |
(ii) If A>0 and ˉD>0 hold, then positive equilibrium point E2(ˉU,ˉE,ˉV) exists, where
ˉU=B−√B2−4AC2A,ˉE=α3ˉUˉVβ1+β2,ˉV=(β1+β2)ϕˉUˉD. |
(iii) If A<0 and ˇD>0 hold, then positive equilibrium point E3(ˇU,ˇE,ˇV) exists, where
ˇU=B−√B2−4AC2A,ˇE=α3ˇUˇVβ1+β2,ˇV=(β1+β2)ϕˇUˇD. |
Proof. To obtain all positive equilibrium points of the system (2.1), we set the right-hand side of (2.1) equal to zero. The equations for the equilibrium points are as follows:
α1−α2U−α3UV+β3E=0, | (3.1) |
α3UV−(β1+β2)E=0, | (3.2) |
(β1+β4)E+ϕU−γV=0. | (3.3) |
From (3.2), we get
E=α3UVβ1+β2. | (3.4) |
Substituting (3.4) into (3.3), we obtain
(β1+β4)α3UVβ1+β2+ϕU−γV=0. |
This gives,
V=(β1+β2)ϕU(β1+β2)γ−(β1+β4)α3U. | (3.5) |
Substituting (3.4) and (3.5) into (3.1), we get the following equations in U:
α1−α2U−α3(β1+β2)ϕU2(β1+β2)γ−(β1+β4)α3U+α3β3ϕU2(β1+β2)γ−(β1+β4)α3U=0,(α1−α2U)[(β1+β2)γ−(β1+β4)α3U]−α3(β1+β2)ϕU2+α3β3ϕU2=0, |
[α2α3(β1+β4)−α3(β1+β2−β3)ϕ]U2−[α1α3(β1+β4)+α2γ(β1+β2)]U+α1γ(β1+β2)=0. |
As a result, we have the quadratic equation
AU2−BU+C=0. | (3.6) |
For A=0, the equilibrium point E1(ˆU,ˆE,ˆV) exists.
For A≠0, we solve the quadratic equation and obtain
U=B±√B2−4AC2A. | (3.7) |
Since B2−4AC>0, the values of U are real numbers. If A<0, then one root of U is positive and the other is negative. If A>0, then both roots of U are positive. However, we require positive equilibrium points. Therefore, two feasible equilibrium points exist, namely, E2(ˉU,ˉE,ˉV) for A>0 and ˉD>0 or E3(ˇU,ˇE,ˇV) for A<0 and ˇD>0.
We now examine the local asymptotic stability of the positive equilibrium points we have found in Lemma (3.2) and determine the conditions for the nonexistence of delay induced instability by analyzing characteristic equations. For this purpose, we assume that an equilibrium point E(˜U,˜E,˜V) exists and then linearize the system (2.1) by using the following transformation
x(t)=[u(t) e(t) v(t)]T=[˜U ˜E ˜V]T+[U(t) E(t) V(t)]T |
where u,e and v are small perturbations around the equilibrium point E(˜U,˜E,˜V).
The linearized system of (2.1) may be written in the matrix form as
˙x(t)=L1x(t)+L2x(t−τ) | (3.8) |
where
L1=[−(α3˜V+α2)β3−α3˜Uα3˜V−(β1+β2)α3˜U0(β1+β4)−γ], |
L2=[000000ϕ00]. |
Let us take the solution of model (3.8) as u(t)=k1eλt,e(t)=k2eλt, and v(t)=k3eλt, where k1,k2 and k3 are arbitrary constants. Thus, we have
λIx(t)=L1x(t)+L2x(t)e−λτ, |
[λI−L1−L2e−λτ]x(t)=0. |
By setting det(λI−L1−L2e−λτ)=0, this leads to the following characteristic equation:
λ3+p1λ2+p2λ+p3+(q1λ+q2)e−λτ=0, | (3.9) |
where
p1=α3˜V+α2+β1+β2+γ,p2=α3(β1+β2−β3+γ)˜V+α2(β1+β2)+(β1+β2)γ−(β1+β4)α3˜U,p3=α3γ(β1+β2−β3)˜V+α2[(β1+β2)γ−(β1+β4)α3˜U],q1=α3ϕ˜U,q2=α3ϕ(β1+β2−β3)˜U. |
Next, we analyze (3.9) by substituting λ=iω in (3.9) and then rearranging it as follows,
(iω)3+p1(iω)2+p2iω+p3+[q1(iω)+q2]e−(iω)τ=0, |
−iω3−p1ω2+p2iω+p3+[q1iω+q2][cos(−ωτ)+isin(−ωτ)]=0, |
i[ω3−p2ω−q1ωcos(ωτ)+q2sin(ωτ)]+p1ω2−p3−q1ωsin(ωτ)−q2cos(ωτ)=0. | (3.10) |
Then, by separating real and imaginary parts, we obtain
p1ω2−p3=q1ωsin(ωτ)+q2cos(ωτ), | (3.11) |
ω3−p2ω=q1ωcos(ωτ)−q2sin(ωτ). | (3.12) |
Squaring both sides of (3.11) and (3.12), we get
(p1ω2−p3)2=(q1ω)2sin2(ωτ)+2q1q2ωsin(ωτ)cos(ωτ)+q22cos2(ωτ), | (3.13) |
(ω3−p2ω)2=(q1ω)2cos2(ωτ)−2q1q2ωsin(ωτ)cos(ωτ)+q22sin2(ωτ). | (3.14) |
Next, after adding (3.13) and (3.14) and rearranging, we have
(p1ω2−p3)2+(ω3−p2ω)2=(q1ω)2+q22, |
ω6+(p21−2p2)ω4+(p22−2p1p3−q21)ω2+p23−q22=0. |
Therefore, we get
ω6+c1ω4+c2ω2+c3=0, | (3.15) |
where c1=p21−2p2,c2=p22−2p1p3−q21 and c3=p23−q22.
By letting σ=ω2, (3.15) becomes a cubic equation given by
σ3+c1σ2+c2σ+c3=0. | (3.16) |
We now define
F(σ)=σ3+c1σ2+c2σ+c3. | (3.17) |
Thus, we have shown that the Eq (3.9) has no purely imaginary roots if and only if (3.16) has no positive real roots. Next, we analyze Eq (3.16) using the methods for third-degree exponential polynomials given by Ruan and Wei [26]. According to the work in [26], for a polynomial in the form of (3.16), the following lemma is obtained.
Lemma 3.3. Suppose σ0=13(−c1+√c21−3c2) and equilibrium point E(˜U,˜E,˜V) exists. If one of the following holds:
(i) c3≥0 and c21<3c2,
(ii) c3≥0 and σ0≤0,
(iii) c3≥0, σ0>0 and F(σ0)>0,
then Eq (3.16) has no positive roots.
From the lemma above, if the conditions of Lemma 3.3 are satisfied, then the system cannot have purely imaginary eigenvalues and therefore a Hopf-bifurcation cannot exist. Then, we apply Lemma 3.3 to the following propositions.
Proposition 3.4. Suppose A=0 and ˆσ=13(−^c1+√^c12−3^c2) where
^c1=^p12−2^p2,^c2=^p22−2^p1^p3−^q12,^c3=^p32−^q22,^p1=α3ˆV+α2+β1+β2+γ,^p2=α3(β1+β2−β3+γ)ˆV+α2(β1+β2)+α2γ2(β1+β2)2B,^p3=α3γ(β1+β2−β3)ˆV+α22γ2(β1+β2)2B,^q1=α3ϕˆU,^q2=α3ϕ(β1+β2−β3)ˆU. |
If one of the following holds:
(i) ^c12<3^c2,
(ii) ^c2≥0,
(iii) ^c2<0 and F(ˆσ)>0,
then the equation
ˆσ3+^c1ˆσ2+^c2ˉσ+^c3=0 | (3.18) |
has no positive roots.
Proposition 3.5. Suppose A>0, ˉD>0 and ˉσ=13(−¯c1+√¯c12−3¯c2) where
¯c1=¯p12−2¯p2,¯c2=¯p22−2¯p1¯p3−¯q12,¯c3=¯p32−¯q22,¯p1=α3ˉV+α2+β1+β2+γ,¯p2=α3(β1+β2−β3+γ)ˉV+α2(β1+β2)+ˉD,¯p3=α3γ(β1+β2−β3)ˉV+α2ˉD,¯q1=α3ϕˉU,¯q2=α3ϕ(β1+β2−β3)ˉU. |
If one of the following holds:
(i) ¯c12<3¯c2,
(ii) ¯c2≥0,
(iii) ¯c2<0 and F(ˉσ)>0,
then the equation
ˉσ3+¯c1ˉσ2+¯c2ˉσ+¯c3=0 | (3.19) |
has no positive roots.
Proposition 3.6. Suppose A<0, ˇD>0 and ˇσ=13(−ˇc1+√ˇc12−3ˇc2) where
ˇc1=ˇp12−2ˇp2,ˇc2=ˇp22−2ˇp1ˇp3−ˇq12,ˇc3=ˇp32−ˇq22,ˇp1=α3ˇV+α2+β1+β2+γ,ˇp2=α3(β1+β2−β3+γ)ˇV+α2(β1+β2)+ˇD,ˇp3=α3γ(β1+β2−β3)ˇV+α2ˇD,ˇq1=α3ϕˇU,ˇq2=α3ϕ(β1+β2−β3)ˇU. |
If one of the following holds:
(i) ˇc12<3ˇc2,
(ii) ˇc2≥0,
(iii) ˇc2<0 and F(ˇσ)>0,
then the equation
ˇσ3+ˇc1ˇσ2+ˇc2ˇσ+ˇc3=0 | (3.20) |
has no positive roots.
Note that, it is easy to show that ^c3>0, ¯c3>0 and ˇc3>0. Next, by using the above propositions and analyzing characteristic equations, we obtain the following theorems.
Theorem 3.7. Suppose A=0. If one of the conditions in Proposition 3.4 holds, then E1(ˆU,ˆE,ˆV) is locally asymptotically stable for all τ≥0.
Proof. Since A=0, E1(ˆU,ˆE,ˆV) exists. The characteristic equation of the linearized the system (2.1) is
λ3+^p1λ2+^p2λ+^p3+(^q1λ+^q2)e−λτ=0. | (3.21) |
When τ=0, the characteristic Eq (3.21) becomes
λ3+^p1λ2+(^p2+^q1)λ+^p3+^q2=0. | (3.22) |
Clearly, ^p1, ^p2, ^p3, ^q1 and ^q2 are positive and ^p1(^p2+^q1)>^p3+^q2. By using the Routh-Hurwitz criterion [3], all the roots of (3.22) have negative real parts. For τ>0, if one of the conditions in Proposition 3.4 holds then (3.18) has no positive real roots, namely, all solutions of (3.18) should be negative or zero. But zero roots cannot occur since ^c3>0. Hence, we obtain only negative roots for (3.18). This implies that (3.21) has negative real parts for all τ≥0. Consequently, E1(ˆU,ˆE,ˆV) is locally asymptotically stable for all τ≥0.
Theorem 3.8. Suppose A>0 and ˉD>0. If one of the conditions in Proposition 3.5 holds, then E2(ˉU,ˉE,ˉV) is locally asymptotically stable for all τ≥0.
Proof. Since A>0 and ˉD>0, E2(ˉU,ˉE,ˉV) exists. The characteristic equation of the linearized the system (2.1) is
λ3+¯p1λ2+¯p2λ+¯p3+(¯q1λ+¯q2)e−λτ=0. | (3.23) |
Similar to the proof of Theorem 3.7, we may show that E2(ˉU,ˉE,ˉV) is locally asymptotically stable for all τ≥0.
Theorem 3.9. Suppose A<0 and ˇD>0. If one of the conditions in Proposition 3.6 holds, then E3(ˇU,ˇE,ˇV) is locally asymptotically stable for all τ≥0.
Proof. Since A<0 and ˇD>0, E3(ˇU,ˇE,ˇV) exists. The characteristic equation of the linearized the system (2.1) is
λ3+ˇp1λ2+ˇp2λ+ˇp3+(ˇq1λ+ˇq2)e−λτ=0. | (3.24) |
Similar to the proof of Theorem 3.7, we may show that E3(ˇU,ˇE,ˇV) is locally asymptotically stable for all τ≥0. Next, we study the global stability of the system (2.1). For convenience, we define a function g:R+→R+ as g(x)=x−1−lnx. Here, g(x)≥0 for all x>0 and g(1)=0. We have the following theorems.
Theorem 3.10. Suppose A>0 and ˉD>0. If α2≥ϕ, β2−β4≥β3, γα3≥ˉU, and one of the conditions in Proposition 3.5 holds, then E2(ˉU,ˉE,ˉV) is globally asymptotically stable for all τ≥0.
Proof. Since A>0 and ˉD>0, E2(ˉU,ˉE,ˉV) exists. Now, we define a Lyapunov functional as follows:
W1=ˉUg(U(t)ˉU)+ˉEg(E(t)ˉE)+ˉVg(V(t)ˉV)+ϕˉU∫τ0g(U(t−ζ)ˉU)dζ. | (3.25) |
Note that, W1≥0 for all positive U, E, V and ζ∈[0,τ]. Moreover, the global minimum W1=0 occurs at E2(ˉU,ˉE,ˉV). Then, by differentiating W1 along the solution of system (2.1) with respect to t, we obtain
dW1dt=(1−ˉUU(t))˙U+(1−ˉEE(t))˙E+(1−ˉVV(t))˙V+ϕˉU[g(U(t)ˉU)−g(U(t−τ)ˉU)],=[1−ˉUU(t)][α1−α2U(t)−α3U(t)V(t)+β3E(t)]+[1−ˉEE(t)][α3U(t)V(t)−(β1+β2)E(t)]+[1−ˉVV(t)][(β1+β4)E(t)+ϕU(t−τ)−γV(t)]+ϕˉU[g(U(t)ˉU)−g(U(t−τ)ˉU)]. |
Using the equalities α1=α2ˉU+α3ˉUˉV−β3ˉE, α3ˉUˉV=(β1+β2)ˉE, and γˉV=ϕˉU+(β1+β4)ˉE into the above equation, we get
dW1dt=[1−ˉUU(t)][α2ˉU+α3ˉUˉV−β3ˉE−α2U(t)−α3U(t)V(t)+β3E(t)]+[1−ˉEE(t)][α3U(t)V(t)−α3ˉUˉV+α3ˉUˉV−α3ˉUˉVˉEE(t)]+[1−ˉVV(t)][(β1+β4)E(t)+ϕU(t−τ)−γˉV−γV(t)+γˉV]+ϕˉU[g(U(t)ˉU)−g(U(t−τ)ˉU)]. |
By rearrangement, we have
dW1dt=α2[1−ˉUU(t)][ˉU−U(t)]−α3[1−ˉUU(t)][U(t)V(t)−ˉUˉV]+β3[1−ˉUU(t)][E(t)−ˉE]+α3[1−ˉEE(t)][U(t)V(t)−ˉUˉV]+α3ˉUˉV[1−ˉEE(t)][1−E(t)ˉE]+(β1+β4)[1−ˉVV(t)][E(t)−ˉE]+ϕ[1−ˉVV(t)][U(t−τ)−ˉU]+γ[1−ˉVV(t)][ˉV−V(t)]+ϕˉU[g(U(t)ˉU)−g(U(t−τ)ˉU)], |
dW1dt=−α2ˉU[g(U(t)ˉU)+g(ˉUU(t))]+α3ˉUˉV[g(V(t)ˉV)−g(ˉUU(t))−g(U(t)V(t)ˉUˉV)]−β3ˉE[g(ˉUE(t)U(t)ˉE)−g(ˉUU(t))−g(E(t)ˉE)]−α3ˉUˉV[g(ˉEU(t)V(t)E(t)ˉUˉV)−g(ˉEE(t))−g(U(t)V(t)ˉUˉV)]−α3ˉUˉV[g(E(t)ˉE)+g(ˉEE(t))]−(β1+β4)ˉE[g(ˉVE(t)V(t)ˉE)−g(ˉVV(t))−g(E(t)ˉE)]−ϕˉU[g(ˉVU(t−τ)V(t)ˉU)−g(ˉVV(t))−g(U(t−τ)ˉU)]−γˉV[g(V(t)ˉV)+g(ˉVV(t))]+ϕˉU[g(U(t)ˉU)−g(U(t−τ)ˉU)],=−(α2−ϕ)ˉUg(U(t)ˉU)−(α2ˉU+α3ˉUˉV−β3ˉE)g(ˉUU(t))−β3ˉEg(ˉUE(t)U(t)ˉE)−(α3ˉUˉV−β3ˉE−β1ˉE−β4ˉE)g(E(t)ˉE)−α3ˉUˉVg(ˉEU(t)V(t)E(t)ˉUˉV)−(β1+β4)ˉEg(ˉVE(t)V(t)ˉE)−(γˉV−α3ˉUˉV)g(V(t)ˉV)−(γˉV−β1ˉE−β4ˉE−ϕˉU)g(ˉVV(t))−ϕˉUg(ˉVU(t−τ)V(t)ˉU),=−(α2−ϕ)ˉUg(U(t)ˉU)−(α2ˉU+β1ˉE+β2ˉE−β3ˉE)g(ˉUU(t))−β3ˉEg(ˉUE(t)U(t)ˉE)−(β2−β3−β4)ˉEg(E(t)ˉE)−α3ˉUˉVg(ˉEU(t)V(t)E(t)ˉUˉV)−(β1+β4)ˉEg(ˉVE(t)V(t)ˉE)−(γ−α3ˉU)ˉVg(V(t)ˉV)−ϕˉUg(ˉVU(t−τ)V(t)ˉU). |
From assumptions that α2≥ϕ, β2−β4≥β3, and γα3≥ˉU, i.e., α2−ϕ≥0, β2−β3−β4≥0 and γ−α3ˉU≥0, we have dW1dt≤0. As a result, W1 is bounded and non-increasing and thus limt→+∞W1(t) exists. Moreover, dW1dt=0 if and only if U(t)=U(t−τ)=ˉU, E(t)=ˉE and V(t)=ˉV for all t≥0. It follows that the largest invariant set in {(U,E,V)|dW1dt=0} is E2(ˉU,ˉE,ˉV). Thus, by LaSalle invariant principle [12], we conclude that the solution of the system (2.1) tends to the largest invariant set. Consequently, E2(ˉU,ˉE,ˉV) is globally asymptotically stable for all τ≥0.
Theorem 3.11. Suppose A<0 and ˇD>0. If α2≥ϕ, β2−β4≥β3, γα3≥ˇU, and one of the conditions in Proposition 3.6 holds, then E3(ˇU,ˇE,ˇV) is globally asymptotically stable for all τ≥0.
Proof. Since A<0 and ˇD>0, E3(ˇU,ˇE,ˇV) exists. Then, we define
W3=ˇUg(U(t)ˇU)+ˇEg(E(t)ˇE)+ˇVg(V(t)ˇV)+ϕˇU∫τ0g(U(t−ζ)ˇU)dζ. | (3.26) |
Similar to the proof of Theorem 3.10, we may show that E3(ˇU,ˇE,ˇV) is globally asymptotically stable for all τ≥0.
Theorem 3.12. Suppose A=0. If α2≥ϕ, β2−β4≥β3, γα3≥ˆU, and one of the conditions in Proposition 3.4 holds, then E1(ˆU,ˆE,ˆV) is globally asymptotically stable for all τ≥0.
Proof. Since A=0, E1(ˆU,ˆE,ˆV) exists. Then, we define
W2=ˆUg(U(t)ˆU)+ˆEg(E(t)ˆE)+ˆVg(V(t)ˆV)+ϕˆU∫τ0g(U(t−ζ)ˆU)dζ. | (3.27) |
Similar to the proof of Theorem 3.10, we may show that E1(ˆU,ˆE,ˆV) is globally asymptotically stable for all τ≥0.
Remark 3.13. In the case of the unemployment model without time-delay, namely for the case τ=0, it is assumed that the new vacancies are determined by using unemployment data in the current time.
Remark 3.14. The conditions α2≥ϕ, β2−β4≥β3, and γα3≥Ul for l=1,2,3 mean that the rate of creating new vacancies should not exceed the migration rate of unemployed persons, the layoff rate should not exceed the rate of vacancies lost because of retirement, and an equilibrium point value of unemployed persons should not exceed the ratio of the reduction rate of vacancies and employment rate. These conditions are assumed to analyze the global asymptotic stability of the system. If we could find a more suitable Lyapunov functional, then this may lead to improved sufficient conditions for global asymptotic stability.
In this section, we provide a practical application and a numerical example to illustrate the effectiveness of theoretical results.
Example 4.1 We first consider a practical example of an unemployment situation in Thailand. According to the latest education statistics of Thailand from the academic year of 2016-2017 [4] provided by the Ministry of Education of Thailand, we may estimate the average number of new graduates as α1=288081. According to the labor statistics yearbook, 2019 of Thailand [29] and social security statistics 2019 of Thailand [30] provided by the Ministry of Labour of Thailand, we may estimate the rate of overseas labor migration as α2=0.071, the rate at which the unemployed persons become employed persons as α3=0.0000055, the layoff rate as β1=0.0081, and the retirement rate as β2=0.028, respectively. Moreover, from the labor statistics yearbook, 2019 of Thailand [29], the numbers of unemployed persons (U), employed persons (E), and newly created vacancies (V) are 145000, 8196600, and 168396, respectively. As a result, we may obtain the initial values as U(0)=145000, E(0)=8196600, and V(0)=168396, respectively. However, due to the lack of some population statistical information, we are unable to estimate the unemployment rate due to layoff (β3), the rate of vacancies due to retirement (β4), the reduction rate of vacancies because of lack of financial resources (γ), and the rate of creating new vacancies from the government policy (ϕ). As a result, to study the unemployment model in Thailand, we need to estimate β3, β4, γ, and ϕ as follows. First, we assume that the unemployment rate due to layoff is 90 percent of the layoff rate, namely, β3=0.9β1=0.00729. Next, we assume that the rate of vacancies because of retirement is 5 percent of retirement, namely, β4=0.05β2=0.0014. Then, we assume that the reduction rate of vacancies because of lack of financial resources is γ=0.05. Finally, we assume that the rate of creating new vacancies from the government policy is ϕ=0.01.
Now, we provide numerical simulations of the unemployment model in Thailand for the system (2.1) without time delay by using the parameters and initial values from statistical data of Thailand as mentioned above. It is straight forward to verify that these parameter values satisfy the condition (ii) of Lemma 3.2, Proposition 3.5, and Theorem 3.8. Therefore, the equilibrium point of the system exists which can be found from the condition (ii) of Lemma 3.2 as (34400,9914500,1890600). Furthermore, it follows from Theorem 3.8 that the equilibrium point is locally asymptotically stable. In Figure 2, we illustrate the change of numbers of unemployed persons, employed persons, and vacancies as time evolves. The simulation indicates that, as time evolved, the number of vacancies increases due to layoff, retirement, and the creation of new vacancies from the government policy. Moreover, the number of unemployed persons is increasing at the beginning and eventually it is decreasing as unemployed persons are getting jobs, as time evolves. On the other hand, the number of employed persons is decreasing for early-stage but eventually, it is increasing because unemployed persons have become employed persons. Furthermore, the number of vacancies is greater than the number of unemployed persons, namely, there are enough vacancies for unemployed persons. In general, in crises such as COVID-19 pandemic, economic recession, government as well as private sectors are not creating job vacancies, but rather providing other policies such as emergency funds for unemployment, food bank, tax-exempts, respectively.
Next, we illustrate the change in the number of unemployed persons as the rate of creating new vacancies from the government policy is being varied, as time evolves. To this end, we provide numerical simulations of the unemployment model in Thailand for the system (2.1) without time delay when the parameters and initial values are the same as those used in Figure 2 but the values of ϕ is being varied, namely, ϕ=0.01, 0.11, and 0.21, respectively. From Figure 3, the numbers of unemployed persons are given, as time evolved, when the rate of creating new vacancies is being varied as ϕ=0.01, 0.11, and 0.21, respectively. The simulation indicates that the number of unemployed persons is increasing at the beginning and eventually it is decreasing as time evolves. Moreover, it can be seen that the number of unemployed persons decreases as the rate of creating new vacancies increases. Note that all three sets of parameters used in Figure 3 satisfy theoretical results for locally asymptotically stable.
Then, we compare the numbers of unemployed persons as time evolved when the rate at which the unemployed persons become employed persons is being varied. We provide numerical simulations of the unemployment model in Thailand for the system (2.1) without time delay when the parameters and initial values are the same as those used to obtain the Figure 2 but the value of α3 is being varied, namely, α3=0.0000055,0.000055, and 0.00055, respectively. From figure 4, it is shown that the numbers of unemployed persons as time evolved when the rate at which the unemployed persons become employed persons is being varied as α3=0.0000055,0.000055, and 0.00055, respectively. It can be seen that the number of unemployed persons decreases as the rate at which the unemployed persons become employed person increases. Moreover, the number of unemployed persons is quite small when the rate at which the unemployed persons become employed persons is set as α3=0.00055. A plausible reason is that at this rate of the unemployed persons become employed persons, the matching between vacancies to unemployed persons takes place quickly. Finally, we note that all three sets of parameters used in Figure 4 satisfy theoretical results for equilibrium states to be locally asymptotically stable.
Example 4.2 In this example, we give numerical results to support the theoretical results for system (2.1). The numerical results based on the Runge-Kutta methods for differential equations [2] and delay differential equations [1]. The sets of parameters used in this numerical example are shown in Table 2.
Parameters | Set 1 | Set 2 | Set 3 | Set 4 |
α1 | 400000 | 400000 | 400000 | 400000 |
α2 | 0.02 | 0.02 | 0.02 | 0.02 |
α3 | 0.00000001 | 0.00000001 | 0.0000000075 | 0.0000001 |
β1 | 0.01 | 0.01 | 0.01 | 0.01 |
β2 | 0.01 | 0.01 | 0.015 | 0.01 |
β3 | 0.005 | 0.005 | 0.005 | 0.005 |
β4 | 0.005 | 0.005 | 0.005 | 0.005 |
ϕ | 0.02 | 0.016 | 0.02 | 0.02 |
γ | 0.05 | 0.06 | 0.05 | 0.05 |
The parameter value set 1 satisfies condition (i) of Lemma 3.2, Proposition 3.4, Theorem 3.7, and Theorem 3.12. Therefore, E1(5000000,20000000,8000000) exists and it is locally and globally asymptotically stable. Next, if we use the parameter value set 2 which satisfies (ii) of Lemma 3.2, Proposition 3.5, Theorem 3.8, and Theorem 3.10. Therefore, E2(5969000,18708000,6269000) exists and it is locally and globally asymptotically stable. Then, we consider the parameter value set 3. The parameter value set 3 corresponds to conditions (iii) of Lemma 3.2, Proposition 3.6, Theorem 3.9, and Theorem 3.11. Therefore, E3(6667000, 13333000,6667000) exists and it is locally and globally asymptotically stable. Figure 5, Figure 6, and Figure 7 show numerical simulations of the system (2.1) by using the parameter value sets 1, 2, and 3, respectively, with the initial values (1000000,15000000,1000000) for τ=0 and the initial functions (1000000e−0.063ζ,15000000,1000000) for τ=10, ζ∈[−τ,0). From Figure 5, Figure 6, and Figure 7, we see that trajectories of solutions converge to E1, E3, and E3, respectively which are in agreement with theoretical results.
Next, we consider the parameter value set 4 given in Table 2. This set satisfies condition (ⅰ) of Lemma 3.2, Proposition 3.4, and Theorem 3.7, but not Theorem 3.12. It follows that ¯E1(645000,25806000,8000000) exists and this point is locally asymptotically stable. However, the conditions in Theorem 3.12 is sufficient conditions for global asymptotic stability. Now, we give the initial conditions far from the equilibrium point ¯E1 and simulate the numerical results of the system (2.1) by using the parameter value set 4 with the initial values (5000000,10000000, 400000) for τ=0 and the initial functions (5000000e−0.063ζ,10000000,400000) for τ=10, ζ∈[−τ,0). These two situations are shown in Figures 8. In this case, although we give initial conditions far from the equilibrium point, the solutions converge to ¯E1.
In this paper, the unemployment model with time delay is proposed. It has been shown that the model has three feasible positive equilibrium points in Lemma 3.2. Next, we have analyzed the local and global stability of all positive equilibrium points. The conditions for the nonexistence of delay induced instability are determined in Proposition 3.4 to Proposition 3.6. The analysis has indicated that the phenomena of a Hopf-bifurcation cannot exist under conditions given in these propositions. Moreover, the conditions for locally and globally asymptotically stable are obtained in Theorem 3.7 to Theorem 3.11. Numerical results confirmed the theoretical analysis as well. Furthermore, we observe that if the rate of creating new vacancies or the employment rate increase, then the number of unemployed persons decreases. This observation has indicated that the strategy of a government to create new vacancies and speed up the matching process between unemployed persons and entrepreneurs has an effect to reduce the number of unemployed persons.
This work is supported by Chiang Mai University. The authors would like to thank reviewers and editors for their valuable comments and suggestions for improving the quality of the manuscript. The first author is supported by the Development and Promotion of Science and Technology Talents Project (DPST).
The authors declare that they have no known competing financial interests or personal relationships that could have appeared to influence the work reported in this paper.
[1] |
P. Zarchan, Proportional navigation and weaving targets, J. Guid. Control Dyn., 18 (1995), 969–974. https://doi.org/10.2514/3.21492 doi: 10.2514/3.21492
![]() |
[2] |
J. I. Lee, C. K. Ryoo, Impact angle control law with sinusoidal evasive maneuver for survivability enhancement, Int. J. Aeronaut. Space Sci., 19 (2018), 433–442. https://doi.org/10.1007/s42405-018-0042-2 doi: 10.1007/s42405-018-0042-2
![]() |
[3] |
E. Garcia, D. W. Casbeer, M. Pachter, Design and analysis of state-feedback optimal strategies for the differential game of active defense, IEEE Trans. Autom. Control, 64 (2019), 553–568. https://doi.org/10.1109/TAC.2018.2828088 doi: 10.1109/TAC.2018.2828088
![]() |
[4] | J. Ding, C. L. Li, G. S. Zhu, Two-person zero-sum matrix games on credibility space, in 2011 Eighth International Conference on Fuzzy Systems and Knowledge Discovery (FSKD), (2011), 912–916. https://doi.org/10.1109/FSKD.2011.6019721 |
[5] | T. Yang, L. Geng, M. Duan, K. Zhang, X. Liu, Research on the evasive strategy of missile based on the theory of differential game, in 2015 34th Chinese Control Conference (CCC), (2015), 5182–5187. https://doi.org/10.1109/ChiCC.2015.7260447 |
[6] | M. Flasiński, Symbolic artificial intelligence, in Introduction to Artificial Intelligence, (2016), 15–22. https://doi.org/10.1007/978-3-319-40022-8_2 |
[7] |
L. P. Kaelbling, M. L. Littman, A. W. Moore, Reinforcement learning: a survey, J. Artif. Intell. Res., 4 (1996), 237–285. https://doi.org/10.1613/jair.301 doi: 10.1613/jair.301
![]() |
[8] | R. S. Sutton, A. G. Barto, Reinforcement Learning: An Introduction, MIT press, 2018. |
[9] |
S. Lei, Y. Lei, Z. Zhu, Research on missile intelligent penetration based on deep reinforcement learning, J. Phys. Conf. Ser., 1616 (2020), 012107. https://doi.org/10.1088/1742-6596/1616/1/012107 doi: 10.1088/1742-6596/1616/1/012107
![]() |
[10] | X. Wang, Y. Cai, Y. Fang, Y. Deng, Intercept strategy for maneuvering target based on deep reinforcement learning, in 2021 40th Chinese Control Conference (CCC), (2021), 3547–3552. https://doi.org/10.23919/CCC52363.2021.9549458 |
[11] |
L. Jiang, Y. Nan, Z. H. Li, Realizing midcourse penetration with deep reinforcement learning, IEEE Access, 9 (2021), 89812–89822. https://doi.org/10.1109/ACCESS.2021.3091605 doi: 10.1109/ACCESS.2021.3091605
![]() |
[12] |
K. Arulkumaran, M. P. Deisenroth, M. Brundage, A. A. Bharath, Deep reinforcement learning: a brief survey, IEEE Signal Process Mag., 34 (2017), 26–38. https://doi.org/10.1109/MSP.2017.2743240 doi: 10.1109/MSP.2017.2743240
![]() |
[13] |
S. Arora, P. Doshi, A survey of inverse reinforcement learning: challenges, methods and progress, Artif. Intell., 297 (2021), 103500. https://doi.org/10.1016/j.artint.2021.103500 doi: 10.1016/j.artint.2021.103500
![]() |
[14] | S. Bradtke, M. Duff, Reinforcement learning methods for continuous-time markov decision problems, in Advances in Neural Information Processing Systems, 7 (1994). Available from: https://proceedings.neurips.cc/paper/1994/file/07871915a8107172b3b5dc15a6574ad3-Paper.pdf. |
[15] |
B. Abdulhai, L. Kattan, Reinforcement learning: introduction to theory and potential for transport applications, Can. J. Civ. Eng., 30 (2003), 981–991. https://doi.org/10.1139/l03-014 doi: 10.1139/l03-014
![]() |
[16] |
F. L. Lewis, D. Vrabie, K. G. Vamvoudakis, Reinforcement learning and feedback control: using natural decision methods to design optimal adaptive controllers, IEEE Control Syst. Mag., 32 (2012), 76–105. https://doi.org/10.1109/MCS.2012.2214134 doi: 10.1109/MCS.2012.2214134
![]() |
[17] |
J. Kober, J. A. Bagnell, J. Peters, Reinforcement learning in robotics: a survey, Int. J. Rob. Res., 32 (2013), 1238–1274. https://doi.org/10.1177/0278364913495721 doi: 10.1177/0278364913495721
![]() |
[18] |
A. Mosavi, Y. Faghan, P. Ghamisi, P. Duan, S. F. Ardabili, E. Salwana, et al., Comprehensive review of deep reinforcement learning methods and applications in economics, Mathematics, 8 (2020), 1640. https://doi.org/10.3390/math8101640 doi: 10.3390/math8101640
![]() |
[19] |
V. Shalumov, Cooperative online guide-launch-guide policy in a target-missile-defender engagement using deep reinforcement learning, Aerosp. Sci. Technol., 104 (2020), 105996. https://doi.org/10.1016/j.ast.2020.105996 doi: 10.1016/j.ast.2020.105996
![]() |
[20] |
S. He, H. S. Shin, A. Tsourdos, Computational missile guidance: a deep reinforcement learning approach, J. Aerosp. Inf. Syst., 18 (2021), 571–582. https://doi.org/10.2514/1.I010970 doi: 10.2514/1.I010970
![]() |
[21] | B. Gaudet, R. Furfaro, Missile homing-phase guidance law design using reinforcement learning, in AIAA Guidance, Navigation, and Control Conference, (2012), 4470. https://doi.org/10.2514/6.2012-4470 |
[22] |
D. Hong, S. Park, Avoiding obstacles via missile real-time inference by reinforcement learning, Appl. Sci., 12 (2022), 4142. https://doi.org/10.3390/app12094142 doi: 10.3390/app12094142
![]() |
[23] |
B. Gaudet, R. Furfaro, R. Linares, Reinforcement learning for angle-only intercept guidance of maneuvering targets, Aerosp. Sci. Technol., 99 (2020), 105746. https://doi.org/10.1016/j.ast.2020.105746 doi: 10.1016/j.ast.2020.105746
![]() |
[24] |
W. Li, Y. Zhu, D. Zhao, Missile guidance with assisted deep reinforcement learning for head-on interception of maneuvering target, Complex Intell. Syst., 8 (2022), 1205–1216. https://doi.org/10.1007/s40747-021-00577-6 doi: 10.1007/s40747-021-00577-6
![]() |
[25] | X. Qiu, C. Gao, W. Jing, Maneuvering penetration strategies of ballistic missiles based on deep reinforcement learning, Proc. Inst. Mech. Eng., Part G: J. Aerosp. Eng., 2022 (2022), 09544100221088361. |
[26] | B. Gaudet, R. Furfaro, Integrated and adaptive guidance and control for endoatmospheric missiles via reinforcement learning, preprint, arXiv: 2109.03880. |
[27] |
A. Candeli, G. de Tommasi, D. G. Lui, A. Mele, S. Santini, G. Tartaglione, A deep deterministic policy gradient learning approach to missile autopilot design, IEEE Access, 10 (2022), 19685–19696. https://doi.org/10.1109/ACCESS.2022.3150926 doi: 10.1109/ACCESS.2022.3150926
![]() |
[28] | C. Yang, J. Wu, G. Liu, Y. Zhang, Ballistic missile maneuver penetration based on reinforcement learning, in 2018 IEEE CSAA Guidance, Navigation and Control Conference (CGNCC), (2018), 1–5. https://doi.org/10.1109/GNCC42960.2018.9018872 |
[29] | R. T. Yanushevsky, Modern Missile Guidance, CRC Press, 2018. https://doi.org/10.1201/9781351202954 |
[30] | A. Wong, F. Nitzsche, M. Khalid, Formulation of reduced-order models for blade-vortex interactions using modified volterra kernels, 2004. Available from: https://citeseerx.ist.psu.edu/viewdoc/download?doi=10.1.1.632.1295&rep=rep1&type=pdf. |
[31] |
S. H. Hollingdale, The mathematics of collision avoidance in two dimensions, J. Navig., 14 (1961), 243–261. https://doi.org/10.1017/S037346330002960X doi: 10.1017/S037346330002960X
![]() |
[32] |
Y. Chen, J. Wang, C. Wang, J. Shan, M. Xin, A modified cooperative proportional navigation guidance law, J. Franklin Inst., 356 (2019), 5692–5705. https://doi.org/10.1016/j.jfranklin.2019.04.013 doi: 10.1016/j.jfranklin.2019.04.013
![]() |
1. | Eric M. Takyi, Charles Ohanian, Margaret Cathcart, Nihal Kumar, Dynamical analysis of a predator-prey system with prey vigilance and hunting cooperation in predators, 2024, 21, 1551-0018, 2768, 10.3934/mbe.2024123 |
Parameters | Explanation |
α1 | The constant rate at which the number of unemployed persons |
is increasing continuously | |
α2 | The migration rate of unemployed persons |
α3 | The rate at which the unemployed persons are becoming employed persons |
β1 | The layoff rate |
β2 | The rate of retirement |
β3 | The rate at which the persons are dismissed become the unemployed persons |
β4 | The rate of vacancies because of retirement |
ϕ | The rate of creating new vacancies |
γ | The reduction rate of vacancies because of lack of financial resources |
τ | Time delay as point of past time |
Parameters | Set 1 | Set 2 | Set 3 | Set 4 |
α1 | 400000 | 400000 | 400000 | 400000 |
α2 | 0.02 | 0.02 | 0.02 | 0.02 |
α3 | 0.00000001 | 0.00000001 | 0.0000000075 | 0.0000001 |
β1 | 0.01 | 0.01 | 0.01 | 0.01 |
β2 | 0.01 | 0.01 | 0.015 | 0.01 |
β3 | 0.005 | 0.005 | 0.005 | 0.005 |
β4 | 0.005 | 0.005 | 0.005 | 0.005 |
ϕ | 0.02 | 0.016 | 0.02 | 0.02 |
γ | 0.05 | 0.06 | 0.05 | 0.05 |
Parameters | Explanation |
α1 | The constant rate at which the number of unemployed persons |
is increasing continuously | |
α2 | The migration rate of unemployed persons |
α3 | The rate at which the unemployed persons are becoming employed persons |
β1 | The layoff rate |
β2 | The rate of retirement |
β3 | The rate at which the persons are dismissed become the unemployed persons |
β4 | The rate of vacancies because of retirement |
ϕ | The rate of creating new vacancies |
γ | The reduction rate of vacancies because of lack of financial resources |
τ | Time delay as point of past time |
Parameters | Set 1 | Set 2 | Set 3 | Set 4 |
α1 | 400000 | 400000 | 400000 | 400000 |
α2 | 0.02 | 0.02 | 0.02 | 0.02 |
α3 | 0.00000001 | 0.00000001 | 0.0000000075 | 0.0000001 |
β1 | 0.01 | 0.01 | 0.01 | 0.01 |
β2 | 0.01 | 0.01 | 0.015 | 0.01 |
β3 | 0.005 | 0.005 | 0.005 | 0.005 |
β4 | 0.005 | 0.005 | 0.005 | 0.005 |
ϕ | 0.02 | 0.016 | 0.02 | 0.02 |
γ | 0.05 | 0.06 | 0.05 | 0.05 |