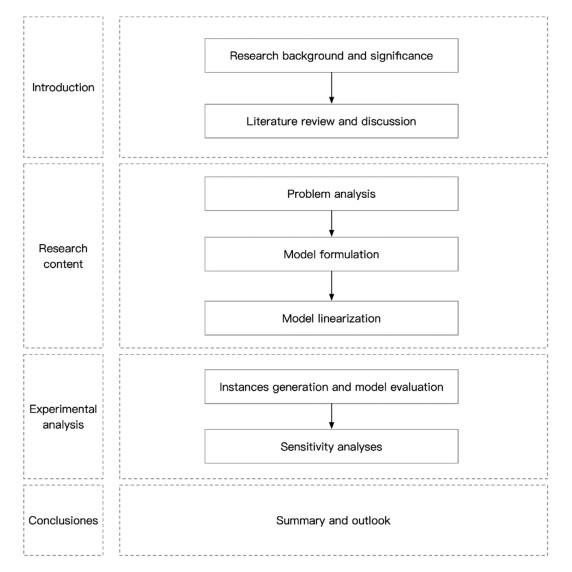
This paper is considered with the quasilinear elliptic equation Δpu=b(x)f(u),u(x)>0,x∈Ω, where Ω is an exterior domain with compact smooth boundary, b∈C(Ω) is non-negative in Ω and may be singular or vanish on ∂Ω, f∈C[0,∞) is positive and increasing on (0,∞) which satisfies a generalized Keller-Osserman condition and is regularly varying at infinity with critical index p−1. By structuring a new comparison function, we establish the new asymptotic behavior of large solutions to the above equation in the exterior domain. We find that the lower term of f has an important influence on the asymptotic behavior of large solutions. And then we further establish the uniqueness of such solutions.
Citation: Yongxiu Shi, Haitao Wan. Refined asymptotic behavior and uniqueness of large solutions to a quasilinear elliptic equation in a borderline case[J]. Electronic Research Archive, 2021, 29(3): 2359-2373. doi: 10.3934/era.2020119
[1] | Yiwei Wu, Yadan Huang, H Wang, Lu Zhen . Nonlinear programming for fleet deployment, voyage planning and speed optimization in sustainable liner shipping. Electronic Research Archive, 2023, 31(1): 147-168. doi: 10.3934/era.2023008 |
[2] | Xu Xin, Xiaoli Wang, Tao Zhang, Haichao Chen, Qian Guo, Shaorui Zhou . Liner alliance shipping network design model with shippers' choice inertia and empty container relocation. Electronic Research Archive, 2023, 31(9): 5509-5540. doi: 10.3934/era.2023280 |
[3] | Xu Zhan, Yang Yong, Wang Xiao . Phased mission reliability analysis of unmanned ship systems. Electronic Research Archive, 2023, 31(10): 6425-6444. doi: 10.3934/era.2023325 |
[4] | Ran Yan, Ying Yang, Yuquan Du . Stochastic optimization model for ship inspection planning under uncertainty in maritime transportation. Electronic Research Archive, 2023, 31(1): 103-122. doi: 10.3934/era.2023006 |
[5] | Simon Tian, Xinyi Zhu . Data analytics in transport: Does Simpson's paradox exist in rule of ship selection for port state control?. Electronic Research Archive, 2023, 31(1): 251-272. doi: 10.3934/era.2023013 |
[6] | Liling Huang, Yong Tan, Jinzhu Ye, Xu Guan . Coordinated location-allocation of cruise ship emergency supplies under public health emergencies. Electronic Research Archive, 2023, 31(4): 1804-1821. doi: 10.3934/era.2023093 |
[7] | Shuaian Wang . Shipping Information Modeling (SIM): bridging the gap between academia and industry. Electronic Research Archive, 2022, 30(10): 3632-3634. doi: 10.3934/era.2022185 |
[8] | Ran Yan, Shuaian Wang . Ship detention prediction using anomaly detection in port state control: model and explanation. Electronic Research Archive, 2022, 30(10): 3679-3691. doi: 10.3934/era.2022188 |
[9] | Kai Zheng, Zengshen Ye, Fanchao Wang, Xi Yang, Jianguo Wu . Custom software development of structural dimension optimization software for the buckling of stiffened plates. Electronic Research Archive, 2023, 31(2): 530-548. doi: 10.3934/era.2023026 |
[10] | Jiali Wang, Xin Jin, Yang Tang . Optimal strategy analysis for adversarial differential games. Electronic Research Archive, 2022, 30(10): 3692-3710. doi: 10.3934/era.2022189 |
This paper is considered with the quasilinear elliptic equation Δpu=b(x)f(u),u(x)>0,x∈Ω, where Ω is an exterior domain with compact smooth boundary, b∈C(Ω) is non-negative in Ω and may be singular or vanish on ∂Ω, f∈C[0,∞) is positive and increasing on (0,∞) which satisfies a generalized Keller-Osserman condition and is regularly varying at infinity with critical index p−1. By structuring a new comparison function, we establish the new asymptotic behavior of large solutions to the above equation in the exterior domain. We find that the lower term of f has an important influence on the asymptotic behavior of large solutions. And then we further establish the uniqueness of such solutions.
Crude oil transportation is highly dependent on maritime transportation. In 2021, nearly 1.83 billion metric tons of crude oil are transported by sea [1], accounting for 43.57% of the total global production [2]. This phenomenon is due to the fact that compared to other modes of transportation, maritime transportation can reliably move large volumes of crude oil around the world and is considered as one of the most efficient ways, even though it requires longer transit times. In general, the stable development of crude oil maritime transportation has an important impact on global economic development and energy supply [3,4].
In 2021, top five countries by crude oil exports are United States, Russia, Saudi Arabia, Canada, and Iraq, holding 50.8% shares of global crude oil production [5]. The prosperity of the global crude oil industry is heavily dependent on the stable output of these five countries. In 2021, i.e., before the Russia-Ukraine war, Europe is the destination for nearly 50% of Russia's crude and petroleum product exports. Specifically, the European Union (EU) imports 104 billion U.S. dollars of oil products from Russia [6]. However, the Russia-Ukraine war brings great uncertainty to the crude oil market. The EU banned imports of Russian oil brought in by sea from December 2022 and all imports of refined oil products from Russia from February 2023 [7]. Besides, the EU banned EU-flagged tankers from transporting Russian crude oil to third countries [7]. EU oil sanctions against Russia undoubtedly bring a thorny challenge to the tanker shipping company's fleet deployment decision, which indicates that tanker shipping companies must consider the influence of EU oil sanctions when deploying tankers.
A new challenge for fleet deployment when EU oil sanctions are taken into account is to consider the flag state of tankers when optimizing crude oil shipping services. The flag state of a merchant tanker is the jurisdiction under whose laws the tanker is registered or licensed. In short, the flag state of a tanker can be considered as the nationality of the tanker. Before the EU oil sanctions, more than half of Russia's oil exports are transported by Greek-flagged tankers [8]. In general, a tanker's flag state has no effect on fleet deployment before the EU oil sanctions. However, to deal with the EU oil sanctions, one of the most efficient methods is fleet redeployment considering the flag state, i.e., deploying non-EU tankers to EU-Russia regions and deploying EU tankers to other regions. In this way, the vacant waste of tankers can be avoided to the greatest extent, and there is no need to spend investment costs to re-purchase new tankers.
This study is motivated by the above mentioned real-world challenge in crude oil maritime shipping, and contributes to the tanker shipping operations management by proposing a quantitative methodology to deal with new challenges in fleet deployment considering EU oil sanctions. With the help of the proposed scientific methodology, tanker shipping companies can optimally determine fleet deployment, fleet repositioning, round trip completion, and speed optimization with the consideration of flag states of tankers to minimize the total cost containing the fleet repositioning cost, the mismatch cost, and the fuel cost. The reason we need to study this integrated problem is that all the decisions are intertwined. The flag state of tankers affects the fleet deployment decision which further affects the fleet repositioning and sailing speed decisions. At the same time, sailing speeds of tankers directly determine the round trip completion. The technical route of this study is shown in Figure 1. Specifically, this paper first introduces the research background and significance of the problem, and reviews related studies. After the above introduction, an integer programming (IP) model for the integrated problem is formulated. 17 numerical experiments are then conducted to assess the efficiency of the proposed model. Moreover, sensitivity analyses including the influences of the unit fuel price, the total crude oil transportation demand, the mismatch cost of completing a round trip by a deployed tanker, and the repositioning cost for each deployed tanker on operations decisions are conducted to explore the influence of these factors on operations decisions for managerial insights.
The remainder of this study is organized as follows. Section 2 reviews related studies. After introducing the problem background, a nonlinear IP model for the integrated problem is first formulated and then linearized in Section 3. Section 4 shows the computational experiments which contains numerical experiments to evaluate the efficiency of the proposed model and sensitivity analyses to obtain managerial insights. Conclusions are summarized in the last section.
The core part of this paper is the widely studied fleet deployment problem [9,10]. Interested readers may refer to Meng et al. [11], Wang and Meng [12], Baykasoğlu et al. [13], Christiansen et al. [14], Dulebenets et al. [15], and Kizilay and Eliiyi [16] for overviews of this problem. This section reviews the related literature from the following three perspectives: fleet deployment considering only the assignment of the type of ships on each route, fleet deployment considering the assignment of the type and number of ships on each route, and fleet redeployment.
In the first research stream, researchers address the fleet deployment problem assuming that ships sail at a given speed. Without considering speed optimization, the fleet deployment problem normally only studies how to assign the type of ships to each ship route to fulfill transportation demand at the lowest cost or the highest profit. Meng and Wang [17] developed a linear IP model with chance constraints for a short-term liner ship fleet planning problem to assign the type of ships to ship routes. On the basis of Meng and Wang [17], Ng and Lin [18] addressed a liner fleet deployment problem when only conditional transportation demand information is known and derived a set of complementary upper and lower bounds on the optimal cost by exploiting the problem structure. Wang and Meng [19] investigated a liner ship fleet deployment problem with container transshipment operations, and proposed a mixed-integer linear programming (MILP) model to determine the type of ships to fulfill container transportation demand at the lowest cost. Meng et al. [20] studied a short-term liner ship fleet planning problem by taking into account container transportation demand. They proposed a two-stage stochastic IP model and a solution algorithm to solve the model. Wang et al. [21] addressed a liner ship fleet deployment problem by providing a sample average approximation method and a mixed-integer nonlinear programming (MINLP) model to help shipping companies assign types of ships deployed on each ship route at the lowest cost while maintaining a certain service level under uncertain container transportation demand. Ng [22] proposed a distribution-free optimization model for a fleet deployment problem to deploy ships with different types on ship routes. Considering uncertainty in transportation demand, Ng [23] investigated a container ship deployment problem and presented analysis results to relax input requirements for the problem. Wang et al. [24] proposed a minimax regret model for a fleet deployment problem with uncertain demand and provided a dynamic scenario inclusion method to solve the model with a small subset of demand scenarios.
However, in practice, decisions regarding sailing speeds and ships type selection are often intertwined. Since sailing speeds and the number of ships on each ship route are correlated, a change in the number of ships deployed on a route usually implies a change in sailing speeds. Therefore, some studies on the fleet deployment problem also optimize the assignment of both the type and number of ships deployed on each route. To minimize the average daily operating cost, Meng and Wang [25] investigated the optimal operating strategy, including frequency, speeds, and the type and number of ships deployed on a service route. They approximated the relationship between fuel consumption and speed by using a piecewise linear function and used a branch-and-bound method to obtain a near-optimal solution. To address the potential overload risk of containers, Zhen et al. [26] formulated an MINLP model for a fleet deployment and demand fulfillment problem in which speed is a variable and developed two efficient algorithms to solve the model. Zhen et al. [27] studied a fleet deployment problem with green technology adoption and proposed an MINLP model to optimally determine the type and number of ships deployed on each route, and sailing speeds on all legs. They developed a three-phase heuristic to solve the problem. Considering fuel consumption, Gao and Hu [28] adopted a speed adjusting strategy to optimize a container ship fleet deployment problem and established a multi-objective MINLP model to optimize the type and number of ships. Pasha et al. [29] proposed an integrated optimization model to deploy heterogeneous ship fleets on ship routes and optimize ship sailing speeds. They designed a decomposition-based heuristic algorithm to solve the model. Lai et al. [30] proposed a two-stage robust optimization model for a ship fleet deployment problem with shipping revenue management, aiming to deploy ships of different types on each ship route under demand uncertainty and optimize the worst-case of total shipping profit. They also designed a column-and-constraint generation-based exact algorithm to solve the model.
In many cases, due to policy adjustments or uncertain demand markets, it is necessary to redeploy (reposition) ships. In contrast to fleet deployment, the fleet redeployment problem may involve repositioning costs [31]. Tierney et al. [32] proposed a novel framework called Temporal Optimization Planning, and formulated an MILP model to solve a liner shipping fleet repositioning problem. Considering seasonal fluctuations in transportation demand of shipping containers, Huang et al. [33] proposed an MILP model to solve a liner service network design and fleet redeployment problem. Tierney et al. [31] introduced a mathematical model and a simulated annealing algorithm for a liner shipping fleet repositioning problem. Müller and Tierney [34] proposed a web-based decision support system designed specifically for liner shipping fleet repositioning. To respond to competitors and changing seasonal customer demand, Wetzel and Tierney [35] proposed an integrated mathematical model and a metaheuristic algorithm for a liner shipping fleet deployment and repositioning problem. Kuhlemann et al. [36] studied a stochastic liner shipping fleet repositioning problem, and formulated an optimization model to handle uncertainty in container transportation demand and ship travel times. Nevertheless, it is noted that the studies reviewed above barely capture speed optimization. Bai et al. [37] provided empirical evidence on the importance of fleet repositioning in the tanker market.
In conclusion, it is necessary for shipping companies to optimize fleet redeployment due to policy adjustments or uncertain transportation demand markets. Few studies studied this problem, but most of them did not incorporate speed optimization. However, speed optimization should be integrated into fleet redeployment because a ship's unit fuel consumption significantly depends on its sailing speed [26] and the fuel cost of ships accounts for more than 50% of the operational cost [38]. Moreover, based on EU oil sanctions, a fleet redeployment and speed optimization problem with the consideration of flag states of tankers needs to be addressed, but the literature lacks it. To the best of our knowledge, our study is the first to attempt to fill this research gap by formulating the problem as an IP model to optimally determine fleet deployment, fleet repositioning, round trip completion, and sailing speeds of tankers, with the aim to minimize the total cost including the fleet repositioning cost, the mismatch cost, and the fuel cost. We believe the problem features considered in this study are realistic and novel with respect to previous research.
Due to EU oil sanctions, the EU banned importing crude oil produced in Russia and transported by sea from 05 December 2022, which means EU-flagged tankers are no longer allowed to ship Russian crude oil. Tanker shipping companies, therefore, need to redeploy their tankers by moving tankers between services in their shipping networks for tanker shipping with the consideration of flag states of tankers. Hence, we consider this new challenge in fleet deployment caused by EU oil sanctions and focus on an integrated optimization problem of fleet deployment, fleet repositioning, round trip completion, and speed optimization. Due to the complexity of the problem, a nonlinear IP model is formulated in Section 3.3 after the detailed problem analysis in Section 3.1 and assumption summary in Section 3.2. Several linearization methods are applied to the nonlinear model in Section 3.4.
Tanker shipping usually serves long-term crude oil trade contracts and its main feature is the stable supply and demand relationship of transportation [39]. Shipping plans for the crude oil trade are designed according to the crude oil trade contract, especially the annual transportation volume and route mileage. Once the shipping plan is designed, there are no major changes unless special issues occur. However, since EU-flagged tankers are no longer allowed to ship Russian crude oil, the fleet redeployment problem considering flag states of tankers needs to be studied. Hence, this study considers a tanker shipping company that is influenced by EU oil sanctions and has a set H of tanker groups before the start of fleet redeployment. Here, notice that tankers in the same group are of the same type in terms of the capacity and the flag state. In terms of the fleet redeployment problem, the tanker shipping company needs to optimize its service network containing a set R of ship routes. Besides, let Hr and Rh represent the subset of all tanker groups whose tankers can be deployed on route r and the subset of all ship routes that can be deployed tankers from tanker group h according to flag states of tanker tankers, respectively. After fleet redeployment, the fleet size on each route will remain the same during the planning period. The length of the planning period is denoted by t.
One of the most important decisions in the integrated optimization problem is the fleet repositioning decision. Hence, we first let αhr represent the number of tankers deployed on ship route r from group h∈Hr. In terms of the restriction on the number of deployed tankers, the total number of tankers from group h deployed on all ship routes (∑r∈Rhαhr) cannot exceed the number of tankers in group h which is represented by nh. Moreover, fleet repositioning operations result in the repositioning cost, and we define chr as the repositioning cost for a tanker from group h to route r. The number of tankers from group h deployed on ship route r is also influenced by the number of round trips completed by tankers from group h deployed on ship route r during the planning period which is denoted by βhr. This is because after a tanker with hundreds of thousands of deadweight tonnage (DWT) arrives at its destination port, the transported oil needs to be temporarily stored at the port, and this storage area has a capacity limit, which requires a minimum frequency requirement for each route r, denoted by fr, for tanker shipping. Hence, the total number of round trips for route r (∑h∈Hrβhr) must be larger than or equal to fr. However, a mismatch between tanker capacity and channel conditions should not be ignored for the fleet redeployment problem. For example, before the fleet redeployment, a 100, 000 DWT EU-flagged tanker is previously deployed on a specific ship route that involves a shallow draft port for seaborne deliveries of Russian oil. However, if a 300, 000 DWT non-EU-flagged tanker has to be deployed on that route in response to EU oil sanctions, which implies that the 300, 000 DWT tanker cannot be fully loaded at the origin port. This mismatch between tanker capacity and channel conditions inevitably wastes the tanker's available capacity and leads to mismatch costs. Let mhr represent the mismatch cost of completing a round trip by a tanker from group h deployed on ship route r. The total mismatch cost generated during the planning horizon can be calculated by ∑r∈R∑h∈Hrmhrβhr. Moreover, due to the mismatch, the actual maximum capacity of each deployed tanker may be affected. Let qhr represent the maximum volume of a round trip by a tanker from group h deployed on ship route r, and we assume that each deployed tanker, fully loaded, i.e., qhr, at an origin port, travels to a destination and then returns to the same origin sailing in ballast condition. Besides, the total transportation demand of each route should also be considered by the tanker shipping company. Since the oil transportation supply and demand relationship is relatively stable, the tanker shipping company usually knows the transportation demand of the whole planning period in advance, and we let dr represent the total crude oil transportation demand of the whole planning period for route r. Hence, during the planning period, deployed tankers need to satisfy the transportation demand of each route. Moreover, fleet redeployment is also related to sailing speeds, which means decisions in this problem are intertwined. We first assume that sailing speeds of all tankers deployed on the same ship route should be the same. Let πr represent the speed of tankers deployed on route r (knot). Hence, the total time of all round trips during the planning period (i.e., βhr(lr24πr+pr24), where lr and pr represent the length of a round trip for route r (n mile) and the total duration of a tanker dwells at all ports of call on ship route r (hour), respectively, should be less than or equal to the total available time of tankers deployed on the route (i.e., tαhr). Finally, sailing speeds of deployed tankers need to meet the speed feasible range, i.e., [v_,¯v] where v_ and ¯v represent the minimum and the maximum speeds (knot) of tankers, respectively.
Under the premise of satisfying the total transportation demand of each route and the minimum shipping frequency, the tanker shipping company hopes to find the optimal fleet deployment, fleet repositioning, round trip completion, and sailing speed plans that minimize the total cost. The total cost contains fleet repositioning cost, the mismatch cost, and the fuel cost. The first two costs can be easily calculated by ∑r∈R∑h∈Hrchrαhr and ∑r∈R∑h∈Hrmhrβhr, respectively. For the fuel cost, we first define n and ar as the unit fuel price (USD/ton) and the amount of fuel consumed by the auxiliary engine of a tanker deployed on ship route r per hour (ton/hour), respectively. Hence, the total fuel cost can be calculated by ∑r∈R∑h∈Hrnβhr(k1πrk2lrπr+prar), where k1 and k2 are coefficients to calculate the unit fuel consumption of a tanker for travelling per hour. In summary, the objective function of this problem is Min∑r∈R∑h∈Hr[chrαhr+mhrβhr+nβhr(k1πrk2lrπr+prar)].
Before introducing the proposed model, three used assumptions are summarized as follows:
1) The shipping network are already determined, which is in line with the setting in Zhen et al. [40].
2) The tanker shipping company uses long time charter contracts. Since this problem involves fleet repositioning and the cost of fleet repositioning is often relatively high [31], the tanker shipping company prefers long-term transportation contracts rather than one-time contracts to earn profits more stably to pay for the repositioning cost.
3) The sailing speed of a tanker must be an integer, which is in line with the setting in Xin et al. [41].
Based on the above analysis of the problem, this study formulates an IP model in this section. Before formulating the mathematical model, we list the notation used in this paper as follows.
Indices and sets:
R : set of all ship routes, r∈R.
H : set of all tanker groups, h∈H.
Hr : subset of all tanker groups whose tankers can be deployed on route r according to flag states of tankers, Hr⊂H.
Rh : subset of all ship routes that can be deployed tankers from tanker group h according to flag states of tankers, Rh⊂R.
Z+ : set of all non-negative integers.
Parameters:
chr : repositioning cost for a tanker from group h to route r (USD).
mhr : mismatch cost of completing a round trip by a tanker from group h deployed on ship route r (USD).
nh : number of tankers in group h.
qhr : maximum volume of a round trip by a tanker from group h deployed on ship route r.
dr : total crude oil transportation demand for route r.
fr : minimum frequency requirement for route r in the tanker shipping operation plan.
lr : length of a round trip for route r (n mile).
t : length of the planning period.
v_,¯v : minimum and maximum speeds of tankers on ship routes, respectively (knot); both of them are integer values.
pr : total duration of a tanker dwells at all ports of call on ship route r (hour).
k1,k2 : coefficients to calculate the unit fuel consumption of a tanker for travelling per hour.
ar : amount of fuel consumed by the auxiliary engine of a tanker on ship route r per hour (ton/hour).
n : unit fuel price (USD/ton).
Variables:
αhr : integer, number of tankers from group h∈H deployed on ship route r.
βhr : integer, number of round trips completed by tankers from group h∈H deployed on ship route r during the planning period.
πr : integer, speed of tankers deployed on route r (knot).
Mathematical model
Based on the above definition of parameters and variables, an IP model is formulated as follows.
[M1] Min∑r∈R∑h∈Hr[chrαhr+mhrβhr+nβhr(k1πrk2lrπr+arpr)] | (1) |
subject to:
∑h∈Hrqhrβhr≥dr ∀r∈R | (2) |
∑h∈Hrβhr≥fr ∀r∈R | (3) |
∑r∈Rhαhr≤nh ∀h∈H | (4) |
βhr(lr24πr+pr24)≤tαhr ∀r∈R,h∈Hr | (5) |
v_≤πr≤¯v ∀r∈R | (6) |
αhr∈Z+,βhr∈Z+ ∀r∈R,h∈H | (7) |
πr∈Z+ ∀r∈R. | (8) |
Objective (1) minimizes the total cost containing the fleet repositioning cost, the mismatch cost, and the fuel cost during the planning period. Constraints (2) ensure that during the planning period, deployed tankers need to satisfy the crude oil transportation demand of each route. Constraints (3) guarantee that the total number of round trips of tankers deployed on each ship route during the planning period must be larger than or equal to the minimum frequency requirement for the route. Constraints (4) ensure that the total number of tankers from each group deployed on all ship routes cannot exceed the number of tankers in the group. Constraints (5) guarantee that the total time of all round trips during the planning period should be less than or equal to the total available time of tankers deployed on the route. Constraints (6) ensure that the sailing speeds of deployed tankers during each voyage on all ship routes satisfy the feasible speed range of tankers. Constraints (7) and (8) state the ranges of the defined decision variables.
The nonlinear model [M1] contains nonlinear objective function (1) and nonlinear constraints (5). Therefore, this study uses some linearization methods to linearize the nonlinear parts. A new binary variable γrv is first defined to replace nonlinear parts related to πr in objective function (1) and constraints (5). To that end, some new notation and constraints are defined as follows.
Newly defined index and set:
V : set of all possible sailing speeds, v∈V, V={v_,v_+1,…,¯v−1,¯v}.
Newly defined variable:
γrv : binary, equals 1 if and only if the speed of the tanker deployed on ship route r is v; 0 otherwise.
Newly defined constraints:
∑v∈Vγrv=1 ∀r∈R | (9) |
πr=∑v∈Vγrvv ∀r∈R | (10) |
γrv∈{0,1} ∀r∈R,v∈V. | (11) |
Hence, objective (1) and constraints (5) are replaced with the following objective function and constraints, respectively.
Min∑r∈R∑h∈Hr[chrαhr+mhrβhr+nβhr(∑v∈Vk1vk2lrvγrv+arpr)] | (12) |
βhr(∑v∈Vlr24vγrv+pr24)≤tαhr ∀r∈R,h∈Hr. | (13) |
Then, a new variable δhrv is defined to replace the product of variables βhr and γrv. To that end, some newly defined parameter, variable, and constraints are added as follows.
Newly defined parameter:
M : big-M for linearization.
Newly defined variable:
δhrv : integer, equals βhr if and only if the speed of the tanker deployed on ship route r is v; 0 otherwise.
Newly defined constraints:
δhrv≥βhr+(γrv−1)M ∀r∈R,h∈Hr,v∈V | (14) |
δhrv≤βhr ∀r∈R,h∈Hr,v∈V | (15) |
δhrv≤γrvM ∀r∈R,h∈Hr,v∈V | (16) |
δhrv∈Z+ ∀r∈R,h∈Hr,v∈V. | (17) |
Here, notice that the value of M in constraints (14) and (16) is set to the maximum possible value of βhr. Hence, objective function (12) and constraints (13) are further replaced with the following objective function and constraints, respectively.
Min∑r∈R∑h∈Hr[chrαhr+mhrβhr+∑v∈Vnk1vk2lrvδhrv+narprβhr] | (18) |
∑v∈Vlr24vδhrv+pr24βhr≤tαhr∀r∈R,h∈Hr. | (19) |
As a result, the final linear version of the model [M1] becomes:
[M2]objectivefunction(18) |
subject to: constraints (2)–(4), (6)–(11), (14)–(17), (19).
In order to evaluate the proposed model, we perform numerous computational experiments on a PC (4 cores of CPUs, 1.4 GHz, Memory 8GB). The mathematical model proposed in this study is implemented in off-the-shelf solver Gurobi 9.5.2 (Anaconda, Python). This section first introduces the value setting of the parameters in Section 4.1, shows experimental results in Section 4.2, and reports sensitivity analyses to seek managerial insights in Section 4.3.
The length of the planning period (i.e., t) is set to 365 days. Seven tanker groups are available in this study: 15 Greek-flagged tankers (tanker capacity 5.0×104 tons) idle at the Port of Piraeus (Greece), 15 Russia-flagged tankers (tanker capacity 8.0×104 tons) idle at the Port of Saint Petersburg (Russia), 20 Chinese-flagged tankers (tanker capacity 2.5×105 tons) idle at the Port of Qingdao (China), 10 Saudi-flagged tankers (tanker capacity 1.8×105 tons) idle at the Port of Jeddah (Saudi Arabia), 10 Saudi-flagged tankers (tanker capacity 5.0×104 tons) idle at the Port of Jeddah (Saudi Arabia), 10 Greek-flagged tankers (tanker capacity 1.8×105 tons) idle at the Port of Piraeus (Greece), and 10 Indian-flagged ships (ship capacity 8.0×104 tons) idle at the Port of Chennai (India). Additionally, ten crude oil transport ship routes summarized in Table 1 need to be optimized. For the sake of simplicity, let bh and er represent the capacity of tankers in tanker group h and the maximum volume of a tanker deployed on ship route r based on channel conditions, respectively. Table 1 records port rotations, the length of a round trip for each route (lr) (calculated from the data in a standard instance LINER-LIB [42]), the maximum volume of a tanker deployed on each route based on channel conditions (er), and the minimum frequency requirement for each route in the tanker shipping operation plan (fr). Besides, values of qhr are set to min(bh,er). The mismatch cost (USD) of completing a round trip by a tanker from group h deployed on ship route r relates to the sailing distance and can be calculated by (0.3+10−4×distance)×max(0,bh−qhr). The crude oil transportation demand for each route (dr) is assumed to be uniformly distributed in [6×106, 8×106] tons.
ID | Port rotations | lr (n mile) | er (105 tons) | fr |
1 | Port of Jeddah (Saudi Arabia)-Port of Rotterdam (Netherlands)-Port of Jeddah (Saudi Arabia) | 8152 | 2.0 | 40 |
2 | Port of Jeddah (Saudi Arabia)-Port of Piraeus (Greece)-Port of Jeddah (Saudi Arabia) | 2734 | 1.1 | 38 |
3 | Port of Jeddah (Saudi Arabia)-Port of Algeciras (Spain)-Port of Jeddah (Saudi Arabia) | 5390 | 1.8 | 40 |
4 | Port of Saint Petersburg (Russia)-Port of Qingdao (China)-Port of Saint Petersburg (Russia) | 24, 054 | 0.8 | 38 |
5 | Port of Saint Petersburg (Russia)-Port of Nagoya (Japan)-Port of Saint Petersburg (Russia) | 24, 918 | 1.5 | 45 |
6 | Port of Saint Petersburg (Russia)-Port of Busan (Korea)-Port of Saint Petersburg (Russia) | 24, 126 | 0.8 | 45 |
7 | Port of Jeddah (Saudi Arabia)-Port of Qingdao (China)-Port of Jeddah (Saudi Arabia) | 13, 596 | 2.0 | 40 |
8 | Port of Jeddah (Saudi Arabia)-Port of Nagoya (Japan)-Port of Jeddah (Saudi Arabia) | 14, 460 | 1.1 | 38 |
9 | Port of Jeddah (Saudi Arabia)-Port of Busan (Korea)-Port of Jeddah (Saudi Arabia) | 13, 668 | 1.8 | 40 |
10 | Port of Saint Petersburg (Russia)-Port of Chennai (India)-Port of Saint Petersburg (Russia) | 17, 180 | 1.1 | 40 |
Since the average global 20 ports price of very low sulfur fuel oil (VLSFO) from 17 December 2021 to 16 December 2022 is 827 USD/ton [43], the unit fuel price (n) is set to 827 USD/ton. The average value of the amount of fuel consumed by the auxiliary engine of a tanker deployed on each ship route per hour (i.e., ar) is set to 0.125 ton/hour (normal distribution with standard deviation 0.1), which is consistent with the setting in Wu et al. [44]. The value of the total duration (hour), i.e., pr, of a tanker on route r is randomly selected from {24,48,72}. The value of repositioning cost (chr) for a tanker from group h to route r is set to the repositioning time multiplied by the operating cost of a tanker. The repositioning time of a tanker is the sailing time between the port where the tanker is currently calling and the departure port on the ship route plus three days for preparation. Daily operating costs of tankers with capacity 5.0×104 tons, 8.0×104 tons, 1.8×105 tons, and 2.5×105 tons are set to 7, 557 USD, 7, 832 USD, 9, 104 USD, and 9, 950 USD, respectively, which is in line with realistic data [45]. Values of v_ and ¯v are set to 8 and 22 knots, respectively, which is in line with the setting in Wu et al. [46]. Values of k1 and k2 are set to 0.00085 and 2, respectively, which is in line with the setting in Wang and Meng [47].
The combination of different tanker groups and ship routes yields 17 computational instances, each denoted by the number of tanker groups (|H|) and the number of ship routes (|R|). Table 2 reports the results of the 17 computational instances. Columns "OBJ", and "Time" list the objective value (USD), and the CPU time (s) used for each instance, respectively. The results show that the CPU time generally increases with the number of ship routes due to the significant increase in constraints. The average CPU time required for the 17 problem instances is 4.5 minutes. Therefore, we can confidently conclude that the proposed model can be applied to address problems of practical scales effectively.
Instance | OBJ (USD) | Time (s) | |
|H|=4 | |R|=2 | 5, 544, 641.74 | 0.18 |
|R|=4 | 40, 441, 430.00 | 0.23 | |
|R|=6 | 241, 095, 201.45 | 13.79 | |
|R|=8 | 304, 786, 839.10 | 628.20 | |
|R|=9 | 329, 396, 892.59 | 738.53 | |
|H|=6 | |R|=2 | 5, 255, 382.84 | 0.14 |
|R|=4 | 39, 445, 247.18 | 0.21 | |
|R|=6 | 193, 742, 202.22 | 1.04 | |
|R|=8 | 235, 799, 329.74 | 11.13 | |
|R|=9 | 257, 248, 140.28 | 121.23 | |
|R|=10 | 332, 110, 436.71 | 177.72 | |
|H|=7 | |R|=2 | 5, 255, 382.83 | 0.15 |
|R|=4 | 39, 445, 247.18 | 0.19 | |
|R|=6 | 175, 425, 648.99 | 1.39 | |
|R|=8 | 208, 993, 356.16 | 35.89 | |
|R|=9 | 224, 815, 730.24 | 2051.53 | |
|R|=10 | 290, 768, 567.96 | 786.51 |
In the above analysis, some important parameters, such as the unit fuel price, the total crude oil transportation demand for each route, the mismatch cost of completing a round trip by a deployed tanker, and the repositioning cost for each deployed tanker, are set to be deterministic. However, these parameters often fluctuate in real life. Therefore, based on the computational instance |H|=6 and |R|=9, sensitivity analyses on these parameters are conducted to investigate the influences of these parameters on operations decisions.
We first study the impact of the unit fuel price (n) on operations decisions. According to S & B [43], the lowest and highest prices of VLSFO in global 20 ports from 17 December 2021 to 16 December 2022 are 593.5 USD/ton and 1125.5 USD/ton, respectively. Hence, we set the value of n from 500 to 1200 USD/ton to investigate its influence. In order to make the results more intuitive, we draw Figure 2 whose abscissa is the unit fuel price (n), and the ordinate is the objective value (OBJ). When the unit fuel price increases, the value of OBJ increases due to the rise in the total fuel cost, which is reasonable because the fuel cost accounts for more than 50% of the operational cost [38]. We also draw Figure 3 whose abscissa is the unit fuel price, and the primary and secondary ordinate axes are the total number of deployed tankers (∑r∈R∑h∈Hrαhr), and the total number of annual round trips completed by deployed tankers (∑r∈R∑h∈Hrβhr), respectively. As the unit fuel price increases, the value of ∑r∈R∑h∈Hrαhr shows a stepped increase, while the value of ∑r∈R∑h∈Hrβhr shows a stepped decrease. Moreover, changes in the unit fuel price directly influence the speed of deployed tankers (πr) which is shown in Table 3. From Table 3, we can see that the speed of tankers becomes slower when the unit fuel price becomes higher. This is because the unit fuel consumption of a tanker for travelling per hour (k1πrk2) increases with the increase of its speed (i.e., πr), and tankers on some routes have to slow down to reduce the fuel consumption. Additionally, in order to meet transportation demand, additional tankers are needed, which further increases the value of OBJ.
n (USD/ton) | πr (knot) |
500 | [20,21,15,22,22,22,22,18,22] |
600 | [20,21,15,22,22,22,22,18,22] |
700 | [20,15,15,22,22,22,22,16,22] |
800 | [20,15,15,22,22,22,22,16,22] |
900 | [20,15,15,22,22,22,22,16,22] |
1000 | [16,11,15,22,22,22,22,17,18] |
1100 | [16,11,15,22,22,22,22,17,18] |
1200 | [16,11,15,22,22,22,22,17,18] |
Note: Numbers in "[]" in the column " πr (knot)" record the speeds of tankers deployed on each route. |
Next, we discuss the impact of the total crude oil transportation demand for each route (dr) on operations decisions. The variance of the transportation demand can be considered as a measure of risk in an uncertain shipping market [21]. According to Rasmussen [48], demand for crude oil shipping in 2024 is expected to increase by 4.5–6.5% compared to 2022. Keeping values of other parameters in the model unchanged, we assign values to dr as its 1 to 1.065 times. Figures 4 and 5 are show regularity in the results. Both abscissas of them are the ratio of change in dr (i.e., the ratio of the present value to the value of dr set in Section 4.1). The ordinate of Figure 4 is OBJ, and the primary and secondary ordinate axes of Figure 5 are ∑r∈R∑h∈Hrαhr and ∑r∈R∑h∈Hrβhr, respectively. As the total crude oil transportation demand increases, OBJ increases, which indicates that with sufficient transport capacity, the tanker shipping company wishes to accept as much transportation demand as possible to maximize its profit. In addition, as the transportation demand increases, ∑r∈R∑h∈Hrαhr shows an upward trend and ∑r∈R∑h∈Hrβhr shows an overall upward trend with occasional downward phases, which is reasonable because the total volume transported by deployed tankers must be larger than or equal to the total crude oil transportation demand. With the constant maximum volume of a round trip by each developed tanker, to satisfy the increased crude oil transportation demand, the total number of round trips completed by deployed tankers (i.e., ∑r∈R∑h∈Hrβhr) increases in most cases, and the total number of deployed tankers (i.e., ∑r∈R∑h∈Hrαhr) may also increase when transportation demand increases to a certain level. Moreover, ∑r∈R∑h∈Hrβhr decreases as the total crude oil transportation demand increases in some cases, which may be due to the deployment of tankers with larger capacity. In this case, it does not need as many round trips to meet the transportation demand.
The mismatch cost of completing a round trip by a deployed tanker (mhr) is considered as the degree of mismatch between tanker capacity and channel conditions. To analyze the impact of mhr on operations decisions, we assign different values to mhr as its 0.1 to 2.5 times. Relevant results including the total number of deployed tankers (∑r∈R∑h∈Hrαhr), the total number of round trips completed by deployed tankers (∑r∈R∑h∈Hrβhr), and the speed of tankers deployed on each route (πr) are recorded in Table 4 partially. Figures 6 and 7 are drawn to visualize the results. The abscissa and ordinate of Figure 6 are the ratio of change in mhr (i.e., the ratio of the present value to the value of mhr set in Section 4.1) and OBJ, respectively. The abscissa of Figure 7 is the same as that of Figure 6; the primary and secondary ordinate axes of Figure 7 are ∑r∈R∑h∈Hrαhr and ∑r∈R∑h∈Hrβhr, respectively. When the mismatch cost of completing a round trip increases, OBJ increases because the total mismatch cost increases. In addition, as the mismatch cost of completing a round trip increases, the value of ∑r∈R∑h∈Hrαhr shows a stepped decrease while the value of ∑r∈R∑h∈Hrβhr shows a stepped increase. From Table 4, as the mismatch cost of completing a round trip increases, the sailing speed increases when the value of ∑r∈R∑h∈Hrαhr decreases. The above observations are reasonable because when the total mismatch cost increases, fewer tankers are needed for the shipping network to reduce the total cost. Due to the fixed frequency requirement as well as the transportation demand, and the reduction in the number of deployed tankers, the total number of round trips completed by deployed tankers increases, and deployed tankers need to sail at higher speeds.
The ratio of change in mhr | ∑r∈R∑h∈Hrαhr | ∑r∈R∑h∈Hrβhr | πr (knot) |
0.1 | 64 | 669 | [16,11,15,22,22,22,22,17,18] |
0.4 | 63 | 683 | [20,15,15,22,22,22,22,16,22] |
0.7 | 63 | 683 | [20,15,15,22,22,22,22,16,22] |
1.0 | 63 | 683 | [20,15,15,22,22,22,22,16,22] |
1.3 | 63 | 683 | [20,15,15,22,22,22,22,16,22] |
1.6 | 63 | 683 | [20,15,15,22,22,22,22,16,22] |
1.9 | 63 | 683 | [20,15,15,22,22,22,22,16,22] |
2.2 | 62 | 698 | [20,21,15,22,22,22,22,18,22] |
2.5 | 62 | 698 | [20,21,15,22,22,22,22,18,22] |
Note: Numbers in "[]" in the column " πr (knot)" record the speeds of tankers deployed on each route. |
Finally, we assign different values to the repositioning cost of each deployed tanker (chr) to analyze the impact of chr on operations decisions. The value of chr is set to the repositioning time multiplied by the operating cost of a tanker. According to Moore Greece [49] in 2021, compared to 2020, Small, Handys, Aframax and Panamax tankers saw a 3–6% increase in operating costs, while Capesize tankers saw a slight decrease. So we set the values of chr to its 0.98 to 1.06 times. Figures 8 and 9 are drew to visualize the results. The abscissa of Figure 8 is the ratio of change in chr (i.e., the ratio of the present value to the value of chr set in Section 4.1), and the ordinate is OBJ. In Figure 9, the abscissa is the ratio of change in chr, and the primary and secondary ordinate axes are ∑r∈R∑h∈Hrαhr and ∑r∈R∑h∈Hrβhr, respectively. When the repositioning cost of each deployed tanker increases, OBJ increases because the total fleet repositioning cost increases. In addition, as the fleet repositioning cost increases, the values of ∑r∈R∑h∈Hrαhr and ∑r∈R∑h∈Hrβhr remain constant because the fleet repositioning cost has less impact on decision-making than other costs, such as the fuel cost. Therefore, when tanker shipping companies make decisions, they may ignore the impact of slight changes in the repositioning cost and pay more attention to other costs, such as the fuel cost.
This study summarizes managerial insights drawn from the experiments in the current international political and economic environment. First, after the Russian-Ukrainian war broke out, international oil prices rose, which inevitably leads to an increase in the operating cost of the tanker shipping company. As a result, tankers have to slow steaming to reduce the fuel consumption and more tankers are needed for each ship route. Second, according to Rasmussen [48], demand for crude oil shipping in 2024 is expected to increase by 4.5–6.5% compared to 2022. We find that with sufficient transport capacity, the tanker shipping company wishes to accept as much transportation demand as possible to maximize its profit. However, with the constant maximum volume of a round trip by each developed tanker, there are two ways to satisfy the increased crude oil transportation demand: increasing the total number of round trips completed by deployed tankers (the total number of deployed tankers may also increase when transportation demand increases to a certain level), and deploying tankers with larger capacity. Of the two methods, the former is more commonly used. Third, the degree of mismatch between tanker capacity and channel conditions, represented by the mismatch cost, caused by fleet repositioning cannot be ignored. It is obvious that when the mismatch cost of completing a round trip increases, the total cost increases because the total mismatch cost increases. Besides, as the mismatch cost increases, the sailing speed should also increase when the number of deployed tankers decreases, which is because when the total mismatch cost increases, fewer tankers are needed for the shipping network to reduce the total cost. Finally, we also investigate the impact of the repositioning cost on operations decisions and find that tanker shipping companies may ignore the impact of slight changes in the repositioning cost and pay more attention to other costs, such as the fuel cost because the impact of this cost on decision-making is not obvious.
There is no doubt that EU oil sanctions bring new challenges to fleet deployment. To best response to the impact of EU oil sanctions, tanker shipping companies need to redesign their shipping services with the consideration of flag states of tankers, but the literature lacks it. Besides, the majority of literature on fleet redeployment do not incorporate speed optimization. To fill this research gap, this paper focuses on an integrated optimization problem of fleet deployment, fleet repositioning, round trip completion, and speed optimization, and proposes an IP model to address this problem. The objective of the model is to minimize the total cost containing the fleet repositioning cost, the mismatch cost, and the fuel cost. We make two main new contributions:
1) We propose an integrated optimization model for a fleet deployment, fleet repositioning, round trip completion, and speed optimization problem. Since the proposed model is nonlinear, some techniques are adopted to transform it to a linear model which can be directly solved by some commercial solvers such as Gurobi. The average solving time required for the 17 computational instances is 4.5 minutes, which means that the model we proposed can be applied to address problems of practical scales effectively.
2) Sensitivity analyses, including the influences of the unit fuel price, the total crude oil transportation demand, the mismatch cost of completing a round trip by a deployed tanker, and the repositioning cost for each deployed tanker on operations decisions, are conducted to obtain managerial insights. For example, as the total crude oil transportation demand increases, the total cost increases, which indicates that with sufficient transport capacity, tanker shipping companies may accept as much transportation demand as possible to maximize their profits.
However, there are some further improvements for future research. First, after the Russian oil price cap and EU oil sanctions, many operators appear reluctant to ship Russian crude oil. Therefore, a shadow fleet of Russian tankers seems to be deployed in many cases [50]. Second, the studied problem does not consider uncertainty [51,52,53], such as the fuel price fluctuation. Since fuel prices change at times and the fuel cost plays a vital role in controlling the total operating cost [54], the fuel price fluctuation may be incorporated into the problem. Third, the impact of late delivery penalty cost may also be considered [55]. Finally, some newly developed technologies can also be incorporated into the studied problem [56,57], such as autonomous tankers [58,59,60], applications of global positioning system (GPS) data [61,62], big data [63,64], blockchain [65,66,67], and green shipping [68,69,70,71,72].
The authors declare they have not used Artificial Intelligence (AI) tools in the creation of this article.
Thanks are due to the referees and editors for their valuable comments that helped improve the quality of this paper.
The authors declare there is no conflict of interest.
[1] |
Uniqueness of the blow-up boundary solution of logistic equations with absorbtion. C. R. Math. Acad. Sci. Paris (2002) 335: 447-452. ![]() |
[2] |
Large and entire large solution for a quasilinear problem. Nonlinear Anal. (2009) 70: 1738-1745. ![]() |
[3] |
Explosive solutions of quasilinear elliptic equations: Existence and uniqueness. Nonlinear Anal. (1993) 20: 97-125. ![]() |
[4] |
Boundary blow-up solutions and their applications in quasilinear elliptic equations. J. Anal. Math. (2003) 89: 277-302. ![]() |
[5] | D. Gilbarg and N. S. Trudinger, Elliptic Partial Differential Equations of Second Order, 3rd ed., Springer-Verlag, Berlin, 1998. |
[6] | F. Gladiali and G. Porru, Estimates for Explosive Solutions to p-Laplace Equations, in: Progress in Partial Differential Equations, Pont-á-Mousson, 1997, vol. 1, in: Pitman Res. Notes Math. Ser., vol. 383, Longman, Harlow, 1998. |
[7] |
S. Huang, Asymptotic behavior of boundary blow-up solutions to elliptic equations, Z. Angew. Math. Phys., 67 (2016), Art. 3, 20 pp. doi: 10.1007/s00033-015-0606-y
![]() |
[8] |
General uniqueness results and blow-up rates for large solutions of elliptic equations. Proc. Roy. Soc. Edinburgh Sect. A (2012) 142: 825-837. ![]() |
[9] |
Quasilinear elliptic equations with boundary blow-up. J. Anal. Math. (1996) 69: 229-247. ![]() |
[10] |
Existence and asymptotic behavior of blow-up solutions to weighted quasilinear equations. J. Math. Anal. Appl. (2004) 298: 621-637. ![]() |
[11] |
Boundary asymptotic and uniqueness of solutions to the p-Laplacian with infinite boundary values. J. Math. Anal. Appl. (2007) 325: 480-489. ![]() |
[12] |
S. I. Resnick, Extreme Values, Regular Variation, and Point Processes, Springer-Verlag, New York, Berlin, 1987. doi: 10.1007/978-0-387-75953-1
![]() |
[13] |
E. Seneta, Regular Varying Functions, in: Lecture Notes in Math., vol. 508, Springer-Verlag, 1976. doi: 10.1007/bfb0079659
![]() |
[14] |
H. Wan, Asymptotic behavior and uniqueness of entire large solutions to a quasilinear elliptic equation, Electron. J. Qual. Theory Differ. Equ., 2017 (2017), Paper No. 30, 17 pp. doi: 10.14232/ejqtde.2017.1.30
![]() |
[15] |
Entire large solutions to semilinear elliptic equations with rapidly or regularly varying nonlinearities. Nonlinear Anal.: Real World Applications (2019) 45: 506-530. ![]() |
[16] |
Existence of explosive positive solutions of quasilinear elliptic equations. Appl. Math. Comput. (2006) 177: 581-588. ![]() |
[17] |
Boundary behavior of large solutions for semilinear elliptic equations with weights. Asymptot. Anal. (2016) 96: 309-329. ![]() |
[18] |
Boundary behavior of large solutions to p-Laplacian elliptic equations. Nonlinear Anal.: Real World Applications (2017) 33: 40-57. ![]() |
1. | Yiwei Wu, Jieming Chen, Yao Lu, Shuaian Wang, Fleet Repositioning, Flag Switching, Transportation Scheduling, and Speed Optimization for Tanker Shipping Firms, 2024, 12, 2077-1312, 1072, 10.3390/jmse12071072 |
ID | Port rotations | lr (n mile) | er (105 tons) | fr |
1 | Port of Jeddah (Saudi Arabia)-Port of Rotterdam (Netherlands)-Port of Jeddah (Saudi Arabia) | 8152 | 2.0 | 40 |
2 | Port of Jeddah (Saudi Arabia)-Port of Piraeus (Greece)-Port of Jeddah (Saudi Arabia) | 2734 | 1.1 | 38 |
3 | Port of Jeddah (Saudi Arabia)-Port of Algeciras (Spain)-Port of Jeddah (Saudi Arabia) | 5390 | 1.8 | 40 |
4 | Port of Saint Petersburg (Russia)-Port of Qingdao (China)-Port of Saint Petersburg (Russia) | 24, 054 | 0.8 | 38 |
5 | Port of Saint Petersburg (Russia)-Port of Nagoya (Japan)-Port of Saint Petersburg (Russia) | 24, 918 | 1.5 | 45 |
6 | Port of Saint Petersburg (Russia)-Port of Busan (Korea)-Port of Saint Petersburg (Russia) | 24, 126 | 0.8 | 45 |
7 | Port of Jeddah (Saudi Arabia)-Port of Qingdao (China)-Port of Jeddah (Saudi Arabia) | 13, 596 | 2.0 | 40 |
8 | Port of Jeddah (Saudi Arabia)-Port of Nagoya (Japan)-Port of Jeddah (Saudi Arabia) | 14, 460 | 1.1 | 38 |
9 | Port of Jeddah (Saudi Arabia)-Port of Busan (Korea)-Port of Jeddah (Saudi Arabia) | 13, 668 | 1.8 | 40 |
10 | Port of Saint Petersburg (Russia)-Port of Chennai (India)-Port of Saint Petersburg (Russia) | 17, 180 | 1.1 | 40 |
Instance | OBJ (USD) | Time (s) | |
|H|=4 | |R|=2 | 5, 544, 641.74 | 0.18 |
|R|=4 | 40, 441, 430.00 | 0.23 | |
|R|=6 | 241, 095, 201.45 | 13.79 | |
|R|=8 | 304, 786, 839.10 | 628.20 | |
|R|=9 | 329, 396, 892.59 | 738.53 | |
|H|=6 | |R|=2 | 5, 255, 382.84 | 0.14 |
|R|=4 | 39, 445, 247.18 | 0.21 | |
|R|=6 | 193, 742, 202.22 | 1.04 | |
|R|=8 | 235, 799, 329.74 | 11.13 | |
|R|=9 | 257, 248, 140.28 | 121.23 | |
|R|=10 | 332, 110, 436.71 | 177.72 | |
|H|=7 | |R|=2 | 5, 255, 382.83 | 0.15 |
|R|=4 | 39, 445, 247.18 | 0.19 | |
|R|=6 | 175, 425, 648.99 | 1.39 | |
|R|=8 | 208, 993, 356.16 | 35.89 | |
|R|=9 | 224, 815, 730.24 | 2051.53 | |
|R|=10 | 290, 768, 567.96 | 786.51 |
n (USD/ton) | πr (knot) |
500 | [20,21,15,22,22,22,22,18,22] |
600 | [20,21,15,22,22,22,22,18,22] |
700 | [20,15,15,22,22,22,22,16,22] |
800 | [20,15,15,22,22,22,22,16,22] |
900 | [20,15,15,22,22,22,22,16,22] |
1000 | [16,11,15,22,22,22,22,17,18] |
1100 | [16,11,15,22,22,22,22,17,18] |
1200 | [16,11,15,22,22,22,22,17,18] |
Note: Numbers in "[]" in the column " πr (knot)" record the speeds of tankers deployed on each route. |
The ratio of change in mhr | ∑r∈R∑h∈Hrαhr | ∑r∈R∑h∈Hrβhr | πr (knot) |
0.1 | 64 | 669 | [16,11,15,22,22,22,22,17,18] |
0.4 | 63 | 683 | [20,15,15,22,22,22,22,16,22] |
0.7 | 63 | 683 | [20,15,15,22,22,22,22,16,22] |
1.0 | 63 | 683 | [20,15,15,22,22,22,22,16,22] |
1.3 | 63 | 683 | [20,15,15,22,22,22,22,16,22] |
1.6 | 63 | 683 | [20,15,15,22,22,22,22,16,22] |
1.9 | 63 | 683 | [20,15,15,22,22,22,22,16,22] |
2.2 | 62 | 698 | [20,21,15,22,22,22,22,18,22] |
2.5 | 62 | 698 | [20,21,15,22,22,22,22,18,22] |
Note: Numbers in "[]" in the column " πr (knot)" record the speeds of tankers deployed on each route. |
ID | Port rotations | lr (n mile) | er (105 tons) | fr |
1 | Port of Jeddah (Saudi Arabia)-Port of Rotterdam (Netherlands)-Port of Jeddah (Saudi Arabia) | 8152 | 2.0 | 40 |
2 | Port of Jeddah (Saudi Arabia)-Port of Piraeus (Greece)-Port of Jeddah (Saudi Arabia) | 2734 | 1.1 | 38 |
3 | Port of Jeddah (Saudi Arabia)-Port of Algeciras (Spain)-Port of Jeddah (Saudi Arabia) | 5390 | 1.8 | 40 |
4 | Port of Saint Petersburg (Russia)-Port of Qingdao (China)-Port of Saint Petersburg (Russia) | 24, 054 | 0.8 | 38 |
5 | Port of Saint Petersburg (Russia)-Port of Nagoya (Japan)-Port of Saint Petersburg (Russia) | 24, 918 | 1.5 | 45 |
6 | Port of Saint Petersburg (Russia)-Port of Busan (Korea)-Port of Saint Petersburg (Russia) | 24, 126 | 0.8 | 45 |
7 | Port of Jeddah (Saudi Arabia)-Port of Qingdao (China)-Port of Jeddah (Saudi Arabia) | 13, 596 | 2.0 | 40 |
8 | Port of Jeddah (Saudi Arabia)-Port of Nagoya (Japan)-Port of Jeddah (Saudi Arabia) | 14, 460 | 1.1 | 38 |
9 | Port of Jeddah (Saudi Arabia)-Port of Busan (Korea)-Port of Jeddah (Saudi Arabia) | 13, 668 | 1.8 | 40 |
10 | Port of Saint Petersburg (Russia)-Port of Chennai (India)-Port of Saint Petersburg (Russia) | 17, 180 | 1.1 | 40 |
Instance | OBJ (USD) | Time (s) | |
|H|=4 | |R|=2 | 5, 544, 641.74 | 0.18 |
|R|=4 | 40, 441, 430.00 | 0.23 | |
|R|=6 | 241, 095, 201.45 | 13.79 | |
|R|=8 | 304, 786, 839.10 | 628.20 | |
|R|=9 | 329, 396, 892.59 | 738.53 | |
|H|=6 | |R|=2 | 5, 255, 382.84 | 0.14 |
|R|=4 | 39, 445, 247.18 | 0.21 | |
|R|=6 | 193, 742, 202.22 | 1.04 | |
|R|=8 | 235, 799, 329.74 | 11.13 | |
|R|=9 | 257, 248, 140.28 | 121.23 | |
|R|=10 | 332, 110, 436.71 | 177.72 | |
|H|=7 | |R|=2 | 5, 255, 382.83 | 0.15 |
|R|=4 | 39, 445, 247.18 | 0.19 | |
|R|=6 | 175, 425, 648.99 | 1.39 | |
|R|=8 | 208, 993, 356.16 | 35.89 | |
|R|=9 | 224, 815, 730.24 | 2051.53 | |
|R|=10 | 290, 768, 567.96 | 786.51 |
n (USD/ton) | πr (knot) |
500 | [20,21,15,22,22,22,22,18,22] |
600 | [20,21,15,22,22,22,22,18,22] |
700 | [20,15,15,22,22,22,22,16,22] |
800 | [20,15,15,22,22,22,22,16,22] |
900 | [20,15,15,22,22,22,22,16,22] |
1000 | [16,11,15,22,22,22,22,17,18] |
1100 | [16,11,15,22,22,22,22,17,18] |
1200 | [16,11,15,22,22,22,22,17,18] |
Note: Numbers in "[]" in the column " πr (knot)" record the speeds of tankers deployed on each route. |
The ratio of change in mhr | ∑r∈R∑h∈Hrαhr | ∑r∈R∑h∈Hrβhr | πr (knot) |
0.1 | 64 | 669 | [16,11,15,22,22,22,22,17,18] |
0.4 | 63 | 683 | [20,15,15,22,22,22,22,16,22] |
0.7 | 63 | 683 | [20,15,15,22,22,22,22,16,22] |
1.0 | 63 | 683 | [20,15,15,22,22,22,22,16,22] |
1.3 | 63 | 683 | [20,15,15,22,22,22,22,16,22] |
1.6 | 63 | 683 | [20,15,15,22,22,22,22,16,22] |
1.9 | 63 | 683 | [20,15,15,22,22,22,22,16,22] |
2.2 | 62 | 698 | [20,21,15,22,22,22,22,18,22] |
2.5 | 62 | 698 | [20,21,15,22,22,22,22,18,22] |
Note: Numbers in "[]" in the column " πr (knot)" record the speeds of tankers deployed on each route. |