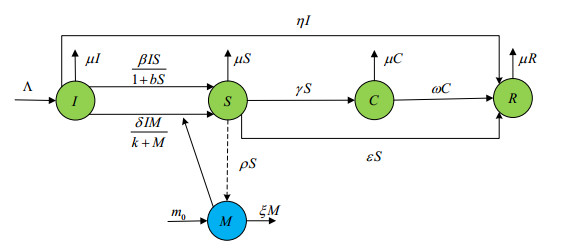
Biological soil crusts (BSCs) are created where a diverse array of microorganisms colonize the surface and upper few millimeters of the soil and create a consolidated crust. They were originally described from arid ecosystems where vascular vegetation is naturally sparse or absent. They have since been discovered in all terrestrial ecosystems. Where present, they perform a variety of important ecological functions, including the capture and accumulation of water and essential plant nutrients, and their release in forms useful to vascular plants. They also stabilize the soil surface against wind and water erosion. BSC organisms include fungi (free-living, lichenized, and mycorrhizal), archaea, bacteria (cyanobacteria and chemotrophic and diazotrophic bacteria), terrestrial algae (including diatoms), and bryophytes (mosses and worts). BSC organisms reproduce primarily asexually via thallus or main body fragmentation or production of asexual spores that are readily dispersed by water and wind. Asexual and sexual propagules of BSC organisms are commonly lifted into the air with vast quantities of dust from the world's arid areas. BSC organisms and/or their propagules have been detected as high as the stratosphere. Some have also been detected in the mesosphere. Airborne dust, microorganisms, and their propagules contribute to the formation of essential raindrop and snowflake nuclei that, in turn, facilitate precipitation events. While airborne in the atmosphere, they also reflect the sun's rays passing laterally through the troposphere and stratosphere at dawn and dusk, often causing brilliant colors at sunrise and sunset.
Citation: Steven D. Warren, Larry L. St. Clair. Atmospheric transport and mixing of biological soil crust microorganisms[J]. AIMS Environmental Science, 2021, 8(5): 498-516. doi: 10.3934/environsci.2021032
[1] | Peter W. Bates, Jianing Chen, Mingji Zhang . Dynamics of ionic flows via Poisson-Nernst-Planck systems with local hard-sphere potentials: Competition between cations. Mathematical Biosciences and Engineering, 2020, 17(4): 3736-3766. doi: 10.3934/mbe.2020210 |
[2] | Yiwei Wang, Mingji Zhang . Finite ion size effects on I-V relations via Poisson-Nernst-Planck systems with two cations: A case study. Mathematical Biosciences and Engineering, 2024, 21(2): 1899-1916. doi: 10.3934/mbe.2024084 |
[3] | Dandan Fan, Dawei Li, Fangzheng Cheng, Guanghua Fu . Effects of congestion charging and subsidy policy on vehicle flow and revenue with user heterogeneity. Mathematical Biosciences and Engineering, 2023, 20(7): 12820-12842. doi: 10.3934/mbe.2023572 |
[4] | Tien-Wen Sung, Wei Li, Qiaoxin Liang, Chuanbo Hong, Qingjun Fang . Research on charging behavior of electric vehicles based on multiple objectives. Mathematical Biosciences and Engineering, 2023, 20(9): 15708-15736. doi: 10.3934/mbe.2023700 |
[5] | Chii-Dong Ho, Jr-Wei Tu, Hsuan Chang, Li-Pang Lin, Thiam Leng Chew . Optimizing thermal efficiencies of power-law fluids in double-pass concentric circular heat exchangers with sinusoidal wall fluxes. Mathematical Biosciences and Engineering, 2022, 19(9): 8648-8670. doi: 10.3934/mbe.2022401 |
[6] | Virginia González-Vélez, Amparo Gil, Iván Quesada . Minimal state models for ionic channels involved in glucagon secretion. Mathematical Biosciences and Engineering, 2010, 7(4): 793-807. doi: 10.3934/mbe.2010.7.793 |
[7] | Mette S. Olufsen, Ali Nadim . On deriving lumped models for blood flow and pressure in the systemic arteries. Mathematical Biosciences and Engineering, 2004, 1(1): 61-80. doi: 10.3934/mbe.2004.1.61 |
[8] | Guichen Lu, Zhengyi Lu . Permanence for two-species Lotka-Volterra cooperative systems with delays. Mathematical Biosciences and Engineering, 2008, 5(3): 477-484. doi: 10.3934/mbe.2008.5.477 |
[9] | Bindu Kumari, Chandrashekhar Sakode, Raghavendran Lakshminarayanan, Prasun K. Roy . Computational systems biology approach for permanent tumor elimination and normal tissue protection using negative biasing: Experimental validation in malignant melanoma as case study. Mathematical Biosciences and Engineering, 2023, 20(5): 9572-9606. doi: 10.3934/mbe.2023420 |
[10] | Suqing Lin, Zhengyi Lu . Permanence for two-species Lotka-Volterra systems with delays. Mathematical Biosciences and Engineering, 2006, 3(1): 137-144. doi: 10.3934/mbe.2006.3.137 |
Biological soil crusts (BSCs) are created where a diverse array of microorganisms colonize the surface and upper few millimeters of the soil and create a consolidated crust. They were originally described from arid ecosystems where vascular vegetation is naturally sparse or absent. They have since been discovered in all terrestrial ecosystems. Where present, they perform a variety of important ecological functions, including the capture and accumulation of water and essential plant nutrients, and their release in forms useful to vascular plants. They also stabilize the soil surface against wind and water erosion. BSC organisms include fungi (free-living, lichenized, and mycorrhizal), archaea, bacteria (cyanobacteria and chemotrophic and diazotrophic bacteria), terrestrial algae (including diatoms), and bryophytes (mosses and worts). BSC organisms reproduce primarily asexually via thallus or main body fragmentation or production of asexual spores that are readily dispersed by water and wind. Asexual and sexual propagules of BSC organisms are commonly lifted into the air with vast quantities of dust from the world's arid areas. BSC organisms and/or their propagules have been detected as high as the stratosphere. Some have also been detected in the mesosphere. Airborne dust, microorganisms, and their propagules contribute to the formation of essential raindrop and snowflake nuclei that, in turn, facilitate precipitation events. While airborne in the atmosphere, they also reflect the sun's rays passing laterally through the troposphere and stratosphere at dawn and dusk, often causing brilliant colors at sunrise and sunset.
A rumor refers to unsubstantiated information, which has the potential to rapidly and extensively disseminate through various communication channels [1]. In the past, rumors were disseminated through oral communication as well as various forms of print media, radio broadcasts, and other mediums. Contemporary social networks can function as an appropriate platform for the rumor propagation, while also satisfying individuals' informational needs [2]. For example, individuals' excessive salt consumption can be attributed to the panic of the Fukushima nuclear accident-related rumor. There have been numerous rumors that emerged a range of adverse impacts on the world since the outbreak of COVID-19 [3]. The security of individuals and their belongings is significantly compromised due to these rumors. Therefore, it is imperative to grasp the rules of communicating with scientists and create effective methods to intervene, defend, and prevent rumors from spreading.
Since rumor spreading is the analogy to the disease's transmission among individuals, many scholars employ similar rumor models. Early studies on rumor propagation focused a tiny community through individual interaction. According to rumor propagation, the crowd can be classified into three categories: ignorant, spreader, and stifler. In 1964, Daley and Kendall proposed the classic DK rumor-spreading model, which is the first cabin model in rumor propagation [4]. Furthermore, in 1973, Maki and Thompson proposed the MT model as an extension of the DK model [5]. According to the MT model, once interpersonal communication occurs, the former will no longer propagate. According to several scholars, the complexity of rumor propagation processes cannot be adequately captured by DK and MK models across various network settings, especially in the context of large-scale social networks. Zanette proposed a conceptual framework to simulate the dynamics of rumor propagation in small-world networks [6]. By integrating the MK model and the SIR model, Moreno et al. developed a novel rumor propagation model, demonstrating that network topologies can significantly influence the spread of rumors on scale-free networks [7]. To gain a deeper comprehension of rumor propagation, an increasing number of factors are being taken into account through the development of more rational models; for instance, hesitating mechanisms [8], inhibiting mechanisms, and attitude adjustment [9], etc. The innovations of these scholars, to a certain extent, promote rumor propagation to advance research under a variety of changing factors.
Nowadays, given the multiple channels of rumor propagation, individuals can quickly obtain information on social media platforms, thereby complicating the regulation of rumor propagation. Due to the significant damage caused by rumors, several control measures aimed at minimizing losses have been proposed by scholars; for instance, optimal control strategies [10], time-dependent controller [11], event-triggered control [12], and so on. In reality, when rumors prevail on social media platforms, the media coverage disseminates information to debunk these rumors and intervene in individuals' behavior, thereby mitigating social panic and upholding social harmony. Cheng et al. incorporated a nonlinear factor into the contact rate by integrating optimal control and time delay [13]. Huo et al. introduced a nonlinear function to effectively capture the impact of misinformation and media reports on the spread of rumors [14]. Pan et al. investigated a novel rumor model that integrated media coverage and rumor refuters [15]. Further on, Guo et al. proposed two models considering media coverage and a refutation mechanism, respectively [16,17]. Numerous mathematical models are available to describe how media coverage affects rumor propagation. Therefore, our proposed model must account for the impact of media coverage on the dissemination of rumors among individuals. Evidently, the aforementioned models have predominantly explored the propagation mechanism of rumors in an integer order.
However, the dynamic behavior of rumors is influenced by historical information. Fractional calculus, as an extension of integral calculus, provides innovative approaches for characterizing the memory effects, which is a generalization of integral calculus. Fractional order Caputo derivatives, extensively employed in various fields such as science, biology, engineering, computer science, chaos theory, and others, have witnessed significant advancements in recent decades [18,19,20,21,22,23,24,25]. The literature offers a wide range of models described by fractional derivatives, such as the SIR or SIRS model [26,27], the SEI model [28], the SEIR model [29,30,31], the SIQR model [32] and the SEIQRS model [33]. Rumor diffusion is similar to virus propagation and can be effectively modeled using fractional calculus. Singh proposed the dynamics of a rumor propagation in a social network, utilizing the Atangana-Baleanu derivative [34]. Ye et al. investigated a fractional-order reaction-diffusion rumor model in two linguistic contexts [35]. The impact of memory was investigated, and the findings clearly demonstrate that fractional calculus can be employed to more accurately characterize the process of rumor propagation.
Revisiting the findings of the previous study, we establish an Ignorants-Spreaders-Calmness-Removers-Media Reports (ISCRM) rumor propagation model with media reports under the Caputo sense by incorporating the memory effect. The subsequent sections of this manuscript are organized as follows: In Section 2, we provide a concise overview of fundamental definitions pertaining to fractional calculus. In Section 3, we propose an enhanced fractional-order ISCRM rumor model. In Section 4, the local and global asymptotic stability of the equilibria is investigated. In Section 5, the optimal control problem is addressed. In Section 6, numerical simulation is examined. In Section 7, the paper culminates with the presentation of our findings.
Various definitions exist regarding fractional derivatives, with the Caputo sense being provided as follows [36].
Definition 2.1. For Riemann-Liouville fractional integration with respect to order α, the function f(t) is presented as
t0Iαtf(t)=D−αtf(t)=1Γ(α)∫tt0f(τ)(t−τ)1−αdτ, | (2.1) |
where α∈R+, t0 represents the initial time, and Γ(⋅), which is established by Γ(z)=∫∞t0tz−1e−tdt, represents the Gamma function.
Definition 2.2. Define l−1<α⩽l, l∈N. The Riemann-Liouville fractional derivative with respect to order α is presented as [36]
RLt0Dαtf(t)=dαf(t)dtα=1Γ(m−α)dldtl∫tt0f(τ)(t−τ)α−l+1dτ, | (2.2) |
Remark 2.1. The combination of Definitions 2.1 and 2.2 yields RLt0Dαt(t0Iαtf(t))=f(t), giving the Riemann-Liouville fractional integrations and derivatives with respect to order α.
Definition 2.3. The Caputo fractional derivative with respect to order α is presented as follows:
Ct0Dαtf(t)={1Γ(l−α)∫tt0fl(τ)(t−τ)α−l+1dτ, l−1<α<l,dldtlf(t),α=l, | (2.3) |
where l denotes the smallest integer number such that α<l.
Remark 2.2. The equivalence between the definitions of Riemann-Liouville fractional integration and Caputo fractional integration are the same. Therefore, from Definitions 2.1 and 2.3, we obtain
Ct0Dαt(t0Iαtf(t))=f(t), | (2.4) |
giving the Caputo fractional integrations and derivatives with respect to order α.
In the subsequent sections of this paper, the notation Dα represents the Riemann-Liouville fractional derivative.
Lemma 2.1. Provide a definition for a function f(t) and a nonzero interval t∈(0,T), the condition |f(t)|⩾F1, where F1>0, is a constant. Next, a positive constant is given L=F1TαΓ(1+α). Thus, the inequality 0Iαt1|f(t)|⩾L is held for t∈(0,t1), t1⩾T.
Proof. The equality is evidently apparent:
0Iαt1|f(t)|=1Γ(α)∫t10|f(τ)|(t−τ)1−αdτ. | (2.5) |
By referring to Eq (2.1), which defines fractional integration, the value of 0Iαt1|f(t)| can be determined. Due to the condition |f(t)|⩾F1, for at least one nonzero interval t∈(0,T), it acquires
0Iαt1|f(t)|=1Γ(α)∫t10|f(τ)|(t−τ)1−αdτ⩾1Γ(α)∫T0|f(τ)|(t−τ)1−αdτ⩾F1Γ(α)∫T01(t−τ)1−αdτ=F1TαΓ(1+α). | (2.6) |
Therefore, the inequality 0Iαt1|f(t)|⩾F1TαΓ(1+α) holds for f(t).
Definition 2.4. Let F(τ) be the Laplace transform of the function f(t). Then, the Caputo fractional derivative Laplace transform is provided as [37]
L[Dαf(t)]=ταF(τ)−n−1∑i=0f(i)(0)τα−n−1, n−1<α⩽n, n∈N. | (2.7) |
Definition 2.5. The expressions for the Mittag-Leffler functions in both one-parameter and two-parameter forms are provided as [36]
Eα(z)=∞∑k=0zkΓ(αk+1), Eα,l(z)=∞∑k=0zkΓ(αk+l), | (2.8) |
where z,α,β∈C.
Theorem 2.1. [36] Consider the autonomous linear fractional-order equation Dαx=Ax, x(0)=x0, where 0<α<1, x∈Rn and A∈Rn×n. The asymptotic stability of this system implies that
|arg(eigA)|⩾απ2. | (2.9) |
In this scenario, each component of the state's decays toward 0 as t−α.
Theorem 2.2. [38] Let x=0 denote an equilibrium point for the equilibrium of the non-autonomous fractional-order equation
Dαx(t)=f(x,t), | (2.10) |
where f(x,t) satisfies the Lipschitz constant l>0 and α∈(0,1). Provided that it constitutes a Lyapunov function V(t,x(t)) and class-K functions α1, α2 and α3 satisfying the condition
α1(‖ |
where \beta \in \left({0, 1} \right) , the equilibrium point of Eq (2.10) is asymptotic stable.
The proposed framework in this section is an Ignorants-Spreaders-Calmness-Removers-Media Reports (ISCRM). All of the crowd are divided into four groups: Ignorants are those who are unfamiliar with the rumors but are willing to believe them, denoted by I\left(t \right) ; Spreaders are those who acquire the rumors and spread them, denoted by S\left(t \right) ; Calmness are those who calm down before they refrain from disseminating rumors [39], denoted by C\left(t \right) ; Removers are those who are aware of the rumors and either refute them or have become disinterested in rumor, denoted by R\left(t \right) . Since rumor propagation varies over time, media reports will undergo changes over time. We employ a quantization method to measure the impact of media reports, denoted by M\left(t \right).
The rules for rumor propagation are as follows:
(ⅰ) When an ignorant contacts a spreader, it will be affected and become a spreader with the exposure rate of \beta /\left({1 + bS} \right) , which reflects the reduced amount of contact rate due to the scale of the rumor propagation. \beta denotes the rumor contact rate and b is the measure of inhibition.
(ⅱ) \delta /\left({k + M} \right) represents the influence of the media reports, where \delta > 1 and k \geqslant 0 . \delta /k means the highest availability of media influence effect. \delta represents the rate of contact between the ignorant and the media reports and k is the effect of media reports. \gamma represents calm down rate of the spreader. \varepsilon and \omega represent the forgetting rates.
(ⅲ) We define that the immigrate rate is \Lambda and the emigrate rate is \mu in our study. Furthermore, \eta represents the influence of rumors under the rumor control measures. {m_0} represents the initial value of the media reports. \rho represents the influence of rumor propagation on media reports. The parameters of the model are assumed to be constant and positive.
The mathematical dynamics of rumor propagation under the Caputo sense is derived based on the propagation rules in Figure 1 as follows:
\begin{array}{l} {D^\alpha }I\left( t \right) = \Lambda - \frac{{\beta I\left( t \right)S\left( t \right)}}{{1 + bS\left( t \right)}} - \frac{{\delta I\left( t \right)M\left( t \right)}}{{k + M\left( t \right)}} - \eta I\left( t \right) - \mu I\left( t \right), \\ {D^\alpha }S\left( t \right) = \frac{{\beta I\left( t \right)S\left( t \right)}}{{1 + bS\left( t \right)}} + \frac{{\delta I\left( t \right)M\left( t \right)}}{{k + M\left( t \right)}} - \gamma S\left( t \right) - \varepsilon S\left( t \right) - \mu S\left( t \right), \\ {D^\alpha }C\left( t \right) = \gamma S\left( t \right) - \omega C\left( t \right) - \mu C\left( t \right), \\ {D^\alpha }R\left( t \right) = \eta I\left( t \right) + \varepsilon S\left( t \right) + \omega C\left( t \right) - \mu R\left( t \right), \\ {D^\alpha }M\left( t \right) = {m_0} + \rho S\left( t \right) - \xi M\left( t \right), \\ \end{array} | (3.1) |
where the initial conditions are denoted by \left({I\left(0 \right), S\left(0 \right), C\left(0 \right), R\left(0 \right), M\left(0 \right)} \right) = \left({{I_0}, {S_0}, {C_0}, {R_0}, {M_0}} \right) .
In this model, the non-negative initial conditions of Eq (3.1) are obtained as
I\left( 0 \right) = {I_0} \geqslant 0, {\text{ }}S\left( 0 \right) = {S_0} \geqslant 0, {\text{ }}C\left( 0 \right) = {C_0} \geqslant 0, {\text{ }}R\left( 0 \right) = {R_0} \geqslant 0, {\text{ }}M\left( 0 \right) = {M_0} \geqslant 0. | (3.2) |
Theorem 3.1. The solution of the Caputo sense Eq (3.1) subject to the conditions (3.2) is non-negative within the closed set
\Omega = \left\{ {\left( {I, S, C, R, M} \right) \in R_ + ^4:N\left( t \right) = I\left( t \right) + S\left( t \right) + C\left( t \right)} \right. \left. { + R\left( t \right) \leqslant \frac{\Lambda }{\mu }, M\left( t \right) \leqslant \frac{{{m_0}}}{\xi } + \frac{{\rho \Lambda }}{{\mu \xi }}} \right\}. |
Proof. For Caputo fractional derivatives (3.1), one obtains
\left\{ \begin{array}{l} {\left. {{D^\alpha }I\left( t \right)} \right|_{I\left( t \right) = 0}} = \Lambda \geqslant 0, \\ {\left. {{D^\alpha }S\left( t \right)} \right|_{S\left( t \right) = 0}} = \frac{{\delta I\left( t \right)M\left( t \right)}}{{k + M\left( t \right)}} \geqslant 0, \\ {\left. {{D^\alpha }S\left( t \right)} \right|_{S\left( t \right) = 0}} = \gamma S\left( t \right) \geqslant 0, \\ {\left. {{D^\alpha }R\left( t \right)} \right|_{R\left( t \right) = 0}} = \eta I\left( t \right) + \varepsilon S\left( t \right) + \omega C\left( t \right) \geqslant 0, \\ {\left. {{D^\alpha }M\left( t \right)} \right|_{M\left( t \right) = 0}} = {m_0} + \rho S\left( t \right) \geqslant 0. \\ \end{array} \right. | (3.3) |
Based on the Laplace transform, it can be demonstrated that I, S, C, R, M \geqslant 0, {\text{ }}t \in \left[{{t_0}, T} \right). Additionally, the total population is determined as N\left(t \right) = I\left(t \right) + S\left(t \right) + C\left(t \right) + R\left(t \right) . Thus, adding the first four equations of Eq (3.1), we obtain
\begin{array}{l} {}_0^CD_t^\alpha N\left( t \right) = \Lambda - \mu \left( {I\left( t \right) + S\left( t \right) + C\left( t \right) + R\left( t \right)} \right) \\ \;\;\;\;\;\;\;\;\;\;\;\;\;\; = \Lambda - \mu N\left( t \right). \\ \end{array} |
By employing the Laplace transform, we obtain N\left(t \right) \leqslant N\left(0 \right){E_\alpha }\left({ - \mu {t^\alpha }} \right) + \frac{\Lambda }{\mu }\left({1 - {E_\alpha }\left({ - \mu {t^\alpha }} \right)} \right). {E_\alpha }\left({ - \mu {t^\alpha }} \right) is the Mittag-Leffler function of parameter \alpha . Since 0 \leqslant {E_\alpha }\left({ - \mu {t^\alpha }} \right) \leqslant 1 , we obtain N\left(t \right) \leqslant \frac{\Lambda }{\mu } + N\left(0 \right). Subsequently, by applying the fifth equation of Eq (3.1), we obtain {D^\alpha }M\left(t \right) \leqslant {m_0} + \frac{{\rho \Lambda }}{\mu } - \xi M\left(t \right), and then, 0 \leqslant M\left(t \right) \leqslant \frac{{{m_0}}}{\xi } + \frac{{\rho \Lambda }}{{\mu \xi }} - M\left(0 \right), where M\left(0 \right) denotes the initial value of the message of media reports. Thus, \mathop {\sup }\limits_{t \to \infty } M\left(t \right) \leqslant \frac{{{m_0}}}{\xi } + \frac{\Lambda }{{\mu \xi }} . This implies that N\left(t \right) is bounded, thus I\left(t \right), S\left(t \right), C\left(t \right), R\left(t \right) and M\left(t \right) are bounded in
\Omega = \left\{ {\left( {I, S, C, R, M} \right) \in R_ + ^4:N\left( t \right) = I\left( t \right) + S\left( t \right) + C\left( t \right) + R\left( t \right) \leqslant \frac{\Lambda }{\mu }, M\left( t \right) \leqslant \frac{{{m_0}}}{\xi } + \frac{{\rho \Lambda }}{{\mu \xi }}} \right\}. |
So, all solutions of Eq (3.1) are non-negative. Now, we will show that equation has a unique solution.
Theorem 3.2. For any given initial condition X\left(0 \right) = \left({{I_0}, {H_0}, {S_0}, {R_0}, {M_0}} \right), {\text{ }}\forall t \geqslant 0 , Eq (3.1) has a unique solution X\left(t \right) \in \Omega .
Proof. The approach employed in [40] is adopted to validate the existence and uniqueness.
Let H\left(X \right) = \left({{H_1}\left(X \right), {H_2}\left(X \right), {H_3}\left(X \right), {H_4}\left(X \right), {H_5}\left(X \right)} \right) as follows:
\begin{array}{l} {H_1}\left( X \right) = \Lambda - \frac{{\beta I\left( t \right)S\left( t \right)}}{{1 + bS\left( t \right)}} - \frac{{\delta I\left( t \right)M\left( t \right)}}{{k + M\left( t \right)}} - \eta I\left( t \right) - \mu I\left( t \right), \\ {H_2}\left( X \right) = \frac{{\beta I\left( t \right)S\left( t \right)}}{{1 + bS\left( t \right)}} + \frac{{\delta I\left( t \right)M\left( t \right)}}{{k + M\left( t \right)}} - \gamma S\left( t \right) - \varepsilon S\left( t \right) - \mu S\left( t \right), \\ {H_3}\left( X \right) = \gamma S\left( t \right) - \omega C\left( t \right) - \mu C\left( t \right), \\ {H_4}\left( X \right) = \eta I\left( t \right) + \varepsilon S\left( t \right) + \omega C\left( t \right) - \mu R\left( t \right), \\ {H_5}\left( X \right) = {m_0} + \rho S\left( t \right) - \xi M\left( t \right). \\ \end{array} |
Furthermore, for any given X, {X_1} \in \Omega , a function can be constructed as follows:
\begin{array}{l} \left\| {H\left( X \right) - H\left( Y \right)} \right\| = \left| {{H_1}\left( X \right) - {H_1}\left( {{X_1}} \right)} \right| + \left| {{H_2}\left( X \right) - {H_2}\left( {{X_1}} \right)} \right| + \left| {{H_3}\left( X \right) - {H_3}\left( {{X_1}} \right)} \right| \\ \;\;\;\;\;\;\;\;\;\;\;\;\;\;\;\;\;\;\;\;\;\;\;\;\;{\text{ }} + \left| {{H_4}\left( X \right) - {H_4}\left( {{X_1}} \right)} \right| + \left| {{H_5}\left( X \right) - {H_5}\left( {{X_1}} \right)} \right| \\ \;\;\;\;\;\;\;\;\;\;\;\;\;\;\;\;\;\;\;\;\;\;\;\;\; = \left| {\Lambda - \frac{{\beta IS}}{{1 + bS}} - \frac{{\delta IM}}{{k + M}} - \eta I - \mu I - \Lambda + \frac{{\beta {I_1}{S_1}}}{{1 + b{S_1}}} + \frac{{\delta {I_1}{M_1}}}{{k + {M_1}}} + \eta {I_1} + \mu {I_1}} \right| \\ \;\;\;\;\;\;\;\;\;\;\;\;\;\;\;\;\;\;\;\;\;\;\;\;\;{\text{ }} + \left| {\frac{{\beta IS}}{{1 + bS}} + \frac{{\delta IM}}{{k + M}} - \gamma S - \varepsilon S - \mu S - \frac{{\beta {I_1}{S_1}}}{{1 + b{S_1}}} - \frac{{\delta {I_1}{M_1}}}{{k + {M_1}}} + \gamma {S_1} + \varepsilon {S_1} + \mu {S_1}} \right| \\ \;\;\;\;\;\;\;\;\;\;\;\;\;\;\;\;\;\;\;\;\;\;\;\;\;{\text{ }} + \left| {\gamma S - \omega C - \mu C - \gamma {S_1} + \omega {C_1} + \mu {C_1}} \right| \\ \;\;\;\;\;\;\;\;\;\;\;\;\;\;\;\;\;\;\;\;\;\;\;\;{\text{ }}\; + \left| {\eta I + \varepsilon S + \omega C - \mu R - \eta {I_1} - \varepsilon {S_1} - \omega {C_1} + \mu {R_1}} \right| \\ \;\;\;\;\;\;\;\;\;\;\;\;\;\;\;\;\;\;\;\;\;\;\;\;\;{\text{ }} + \left| {{m_0} + \rho S - \xi M - {m_0} - \rho {S_1} + \xi {M_1}} \right| \\ \;\;\;\;\;\;\;\;\;\;\;\;\;\;\;\;\;\;\;\;\;\;\;\;\; \leqslant {l_1}\left| {I - {I_1}} \right| + {l_2}\left| {S - {S_1}} \right| + {l_3}\left| {C - {C_1}} \right| + {l_4}\left| {R - {R_1}} \right| + {l_5}\left| {M - {M_1}} \right| \\ \;\;\;\;\;\;\;\;\;\;\;\;\;\;\;\;\;\;\;\;\;\;\;\;\; \leqslant L\left\| {\left( {I, S, C, R, M} \right) - \left( {{I_1}, {S_1}, {C_1}, {R_1}, {M_1}} \right)} \right\| \\ \;\;\;\;\;\;\;\;\;\;\;\;\;\;\;\;\;\;\;\;\;\;\;\;\; \leqslant L\left\| {X - {X_1}} \right\|, \\ \end{array} |
where
\begin{array}{c}{l_1} = \frac{{2\beta }}{b} + 2\delta + 2\eta + \mu , {\text{ }}{l_2} = \frac{{2\beta \Lambda }}{\mu } + 2\gamma + 2\varepsilon + \rho + \mu , {\text{ }}{l_3} = 2\omega + \mu , {\text{ }}{l_4} = \mu , {\text{ }}{l_5} = \frac{{2\delta \Lambda }}{{k\mu }} + \xi ,\\ L = \max \left( {{l_1}, {l_2}, {l_3}, {l_4}, {l_5}} \right). \end{array} |
Therefore, H\left(X \right) satisfies the Lipschitz condition with any initial condition X\left(0 \right) = \left({{I_0}, {H_0}, {S_0}, {R_0}, {M_0}} \right), {\text{ }}\forall t \geqslant 0 . Equations (3.1) always have a unique solution.
In order to explore the rumor-free and rumor-permanence equilibrium points of the fractional order Eq (3.1), we solve the following equations:
\begin{array}{l} \Lambda - \frac{{\beta I\left( t \right)S\left( t \right)}}{{1 + bS\left( t \right)}} - \frac{{\delta I\left( t \right)M\left( t \right)}}{{k + M\left( t \right)}} - \eta I\left( t \right) - \mu I\left( t \right) = 0, \\ \frac{{\beta I\left( t \right)S\left( t \right)}}{{1 + bS\left( t \right)}} + \frac{{\delta I\left( t \right)M\left( t \right)}}{{k + M\left( t \right)}} - \gamma S\left( t \right) - \varepsilon S\left( t \right) - \mu S\left( t \right) = 0, \\ \gamma S\left( t \right) - \omega C\left( t \right) - \mu C\left( t \right) = 0, \\ \eta I\left( t \right) + \varepsilon S\left( t \right) + \omega C\left( t \right) - \mu R\left( t \right) = 0, \\ {m_0} + \rho S\left( t \right) - \xi M\left( t \right) = 0. \\ \end{array} |
By denoting the aforementioned Eq (3.1) with {S_0} = 0 , the rumor-free equilibrium point can be calculated as: {E_0} = \left({\frac{\Lambda }{{\eta + \mu }}, 0, 0, 0, \frac{{{m_0}}}{\xi } + \frac{{\rho \Lambda }}{{\xi \left({\eta + \mu } \right)}}} \right). By employing the next generation approach [41], the basic reproduction number {R_0} is calculated. If Q = {\left({S, C, M} \right)^T} , the original equation can be reformulated as
\frac{{dQ}}{{dt}} = \mathcal{F}\left( x \right) - \mathcal{V}\left( x \right), |
where
\mathcal{F}\left( x \right) = \left( \begin{array}{l} \frac{{\beta I\left( t \right)S\left( t \right)}}{{1 + bS\left( t \right)}} + \frac{{\delta I\left( t \right)M\left( t \right)}}{{k + M\left( t \right)}} \\ \;\;\;\;\;\;\;\;\;\;\;\;\;\;\;\;0 \\ \;\;\;\;\;\;\;\;\;\;\;\;\;\;\;\;0 \\ \end{array} \right), {\text{ }}\mathcal{V}\left( x \right) = \left( \begin{array}{l} \;\;\;\;\left( {\gamma + \varepsilon + \mu } \right)S\left( t \right) \\ \left( {\omega + \mu } \right)C\left( t \right) - \gamma S\left( t \right) \\ \;{m_0} + \xi M\left( t \right) - \rho S\left( t \right) \\ \end{array} \right). |
The Jacobian matrices of \mathcal{F}\left(x \right) and \mathcal{V}\left(x \right) at the rumor-free equilibrium {E_0} , respectively, are
{F_0} = \left( \begin{array}{l} 0\;\;\;\;\beta {I_0}\;\;\;\;0\;\;\;\;\;\;0\;\;\;\;\;\frac{{\delta {I_0}}}{k} \\ 0\;\;\;\;\;0\;\;\;\;\;\;\;0\;\;\;\;\;\;0\;\;\;\;\;\;\;0 \\ 0\;\;\;\;\;0\;\;\;\;\;\;\;0\;\;\;\;\;\;0\;\;\;\;\;\;\;0 \\ \end{array} \right), {\text{ }}{V_0} = \left( \begin{array}{l} 0\;\;\gamma + \varepsilon + \mu \;\;\;\;\;\;0\;\;\;\;\;\;0\;\;\;\;0 \\ 0\;\;\;\;\; - \gamma \;\;\;\;\;\;\;\;\omega + \mu \;\;\;0\;\;\;\;0 \\ 0\;\;\;\; - \rho \;\;\;\;\;\;\;\;\;\;\;0\;\;\;\;\;\;\;0\;\;\;\;\xi \; \\ \end{array} \right). |
The next-generation matrix for Eq (3.1) is presented as follows:
{F_0}V_0^{ - 1} = \left( \begin{array}{l} \;\;\;\;\;\;\;\;\;\;\;\;0\;\;\;\;\;\;\;\;\;\;\;\;\;\;\; 0 \;\;\;\;\;\; 0 \\ \frac{{\beta {I_0}}}{{\gamma + \varepsilon + \mu }} + \frac{{\rho \delta {I_0}}}{{k\xi \left( {\gamma + \varepsilon + \mu } \right)}}\;\;\;\;0\;\;\;\;\frac{{\delta {I_0}}}{{k\xi }} \\ \;\;\;\;\;\;\;\;\;\;\;\;\;0\;\;\;\;\;\;\;\;\; \;\;\;\;\;0\;\;\;\;\;\;0 \\ \end{array} \right). |
Subsequently, the basic reproduction number of Eq (3.1) is determined:
{R_0} = \rho \left( {{F_0}V_0^{ - 1}} \right) = \frac{{\beta {I_0}}}{{\gamma + \varepsilon + \mu }} + \frac{{\rho \delta {I_0}}}{{k\xi \left( {\gamma + \varepsilon + \mu } \right)}} = \frac{{\Lambda \left( {k\xi \beta + \rho \delta } \right)}}{{k\xi \left( {\eta + \mu } \right)\left( {\gamma + \varepsilon + \mu } \right)}}. |
The following theorem is utilized to analyze the local and global stability of the two equilibrium points.
Theorem 4.1. Equation (3.1) exhibit local stability of the rumor-free equilibrium {E_0} if {R_0} < 1 , and if {R_0} > 1 it is unstable.
Proof. The stability of the equilibrium point is investigated by examining the Jacobian matrix of Eq (3.1).
J\left( {{E_0}} \right) = \left( \begin{array}{l} - \eta - \mu \;\;\;\;\;\;\;\;\;\;\;\; - \beta {I_0}\;\;\;\;\;\;\;\;\;\;\;\;\;\;\;0\;\;\;\;\;\;\;\;\;\;\;0\;\;\;\;\; - \frac{{\delta {I_0}}}{k} \\ \;\;\;\;0\;\;\;\;\;\;\beta {I_0} - \left( {\gamma + \varepsilon + \mu } \right)\;\;\;\;\;\;\;\;\;\;0\;\;\;\;\;\;\;\;\;\;\;0\;\;\;\;\;\;\frac{{\delta {I_0}}}{k} \\ \;\;\;\;0\;\;\;\;\;\;\;\;\;\;\;\;\;\;\;\;\;\;\;\;\gamma \;\;\;\;\;\;\;\;\;\;\;\;\; - \omega - \mu \;\;\;\;\;\;0\;\;\;\;\;\;\;\;0 \\ \;\;\;\;\eta \;\;\;\;\;\;\;\;\;\;\;\;\;\;\;\;\;\;\;\varepsilon \;\;\;\;\;\;\;\;\;\;\;\;\;\;\;\;\;\;\omega \;\;\;\;\;\;\;\;\; - \mu \;\;\;\;\;\;0\;\;\;\; \\ \;\;\;\;0\;\;\;\;\;\;\;\;\;\;\;\;\;\;\;\;\;\;\;\rho \;\;\;\;\;\;\;\;\;\;\;\;\;\;\;\;\;\;0\;\;\;\;\;\;\;\;\;\;\;\;0\;\;\;\;\;\; - \xi \\ \end{array} \right). |
The characteristic polynomial of J\left({{E_0}} \right) is derived as follows:
Det\left( {\lambda I - J\left( {{E_0}} \right)} \right) = \left( {\lambda + \mu } \right)\left( {\lambda + \eta + \mu } \right)\left( {\lambda + \omega + \mu } \right)\left[ {\left( {\lambda - \beta {I_0} + \left( {\gamma + \varepsilon + \mu } \right)} \right)\left( {\lambda + \xi } \right) - \frac{{\rho \delta {I_0}}}{k}} \right]. | (4.1) |
If all roots of Det\left({\lambda I - J\left({{E_0}} \right)} \right) possess negative real parts, the rumor-free equilibrium {E_0} can be considered asymptotically stable.
When Det\left({\lambda I - J\left({{E_0}} \right)} \right) = 0 , we obtain
{\lambda _1} = - \mu , {\text{ }}{\lambda _2} = - \eta - \mu , {\text{ }}{\lambda _3} = - \omega - \mu , |
and
{\lambda ^2} + \left( {\gamma + \varepsilon + \mu + \xi - \beta {I_0}} \right)\lambda + \xi \left( {\gamma + \varepsilon + \mu } \right) - \xi \beta {I_0} - \frac{{\rho \delta {I_0}}}{k} = 0. | (4.2) |
It is obvious that {\lambda _1}, {\text{ }}{\lambda _2} and {\lambda _3} are negatives roots and thus, the real component of each of Eq (4.2) roots plays a crucial role.
According to Eq (4.1), it defines
{b_0} = 1, {\text{ }}{b_1} = \gamma + \varepsilon + \mu + \xi - \beta {I_0}, {\text{ }}{b_2} = \xi \left( {\gamma + \varepsilon + \mu } \right) - \xi \beta {I_0} - \frac{{\rho \delta {I_0}}}{k}. |
Evidently, we obtain
{b_0}{\lambda ^2} + {b_1}\lambda + {b_2} = 0. | (4.3) |
When {R_0} < 1 , we acquire
\xi \left( {\gamma + \varepsilon + \mu } \right) - \xi \beta {I_0} - \frac{{\rho \delta {I_0}}}{k} > 0. | (4.4) |
Thus, {b_2} > 0 is deducted from Eq (4.2) by adding \frac{{\rho \delta {I_0}}}{k} to Eq (4.4), and we obtain
\xi \left( {\gamma + \varepsilon + \mu } \right) - \xi \beta {I_0} > \frac{{\rho \delta {I_0}}}{k} > 0. | (4.5) |
From Eq (4.5), we obtain
\gamma + \varepsilon + \mu - \beta {I_0} > \frac{{\rho \delta {I_0}}}{{k\xi }} > 0. | (4.6) |
By adding the parameter \xi to Eq (4.6), we obtain
\gamma + \varepsilon + \mu + \xi - \beta {I_0} > \xi > 0. | (4.7) |
Therefore, {b_1} > 0 is deduced based on Eq (4.7). After evaluation of different coefficients of Eq (4.2), {b_0} > 0, {\text{ }}{b_1} > 0, {\text{ }}{b_2} > 0 and {b_1}{b_2} > 0 are obtained. According to the Routh-Hurwitz conditions [42], all eigenvalues of J\left({{E_0}} \right) have negative real part. The condition \left| {\arg \left({{\lambda _i}} \right)} \right| \geqslant \frac{{\alpha \pi }}{2} is satisfied and the rumor-free equilibrium {E_0} is locally asymptotically stable if {R_0} < 1 , and unstable if {R_0} > 1 .
Theorem 4.2. The rumor-free equilibrium {E_0} of Eq (3.1) is globally asymptotically stable if {R_0} < 1 .
Proof. Let V\left({I, S, C, R, M} \right) , with positive constants {c_1} and {c_2} , serve as a Lyapunov function
V\left( {I, S, C, R, M} \right) = \left( {I - {I_0} - {I_0}\ln \frac{I}{{{I_0}}}} \right) + \left( {C - {C_0} - {C_0}\ln \frac{C}{{{C_0}}}} \right)\; + \left( {R - {R_0} - {R_0}\ln \frac{R}{{{R_0}}}} \right) + {c_1}S + {c_2}M. |
By differentiating the Lyapunov function with respect to time, we obtain
{D^\alpha }V = \left( {1 - \frac{{{I_0}}}{I}} \right){D^\alpha }I\left( t \right) + \left( {1 - \frac{{{C_0}}}{C}} \right){D^\alpha }C\left( t \right) + \left( {1 - \frac{{{R_0}}}{R}} \right){D^\alpha }R\left( t \right)\; + {c_1}{D^\alpha }S\left( t \right) + {c_2}{D^\alpha }M\left( t \right), | (4.8) |
where {c_1} \geqslant 0, {\text{ }}{c_2} \geqslant 0. Substituting {D^\alpha }I\left(t \right), {D^\alpha }S\left(t \right), {D^\alpha }C\left(t \right), {D^\alpha }R\left(t \right) , and {D^\alpha }M\left(t \right) in Eq (3.1) into Eq (4.8) yields
\begin{array}{l} {D^\alpha }V = \left( {1 - \frac{{{I_0}}}{I}} \right)\left[ {\Lambda - \frac{{\beta I\left( t \right)S\left( t \right)}}{{1 + bS\left( t \right)}} - \frac{{\delta I\left( t \right)M\left( t \right)}}{{k + M\left( t \right)}} - \eta I\left( t \right) - \mu I\left( t \right)} \right] \\ \;\;\;\;\;\;\;\;\;\;\;\; + \left( {1 - \frac{{{C_0}}}{C}} \right)\left[ {\gamma S\left( t \right) - \omega C\left( t \right) - \mu C\left( t \right)} \right] \\ \;\;\;\;\;\;\;\;\;\;\;\; + \left( {1 - \frac{{{R_0}}}{R}} \right)\left[ {\eta I\left( t \right) + \varepsilon S\left( t \right) + \omega C\left( t \right) - \mu R\left( t \right)} \right] \\ \;\;\;\;\;\;\;\;\;\;\;\; + {c_1}\left[ {\frac{{\beta I\left( t \right)S\left( t \right)}}{{1 + bS\left( t \right)}} + \frac{{\delta I\left( t \right)M\left( t \right)}}{{k + M\left( t \right)}} - \gamma S\left( t \right) - \varepsilon S\left( t \right) - \mu S\left( t \right)} \right] \\ \;\;\;\;\;\;\;\;\;\;\;\; + {c_2}\left[ {\rho S\left( t \right) - \xi M\left( t \right)} \right], \\ \end{array} |
{D^\alpha }V \leqslant \left[ {\frac{{{c_1}\delta I\left( t \right)}}{{k + M\left( t \right)}} - {c_2}\xi } \right]M\left( t \right) + \left[ {\frac{{{c_1}\beta I\left( t \right)}}{{1 + bS\left( t \right)}} - {c_1}\left( {\gamma + \varepsilon + \mu } \right) + {c_2}\rho } \right]S\left( t \right). | (4.9) |
From Eq (4.9), we obtain
{d_1} = \frac{{{c_1}\delta I\left( t \right)}}{{k + M\left( t \right)}} - {c_2}\xi , {\text{ }}{d_2} = \frac{{{c_1}\beta I\left( t \right)}}{{1 + bS\left( t \right)}} - {c_1}\left( {\gamma + \varepsilon + \mu } \right) + {c_2}. |
Equating the coefficient {d_1} to zero, we obtain
\frac{{{c_1}\delta I\left( t \right)}}{{k + M\left( t \right)}} = {c_2}\xi . |
At the rumor-free equilibrium {E_0} , I \leqslant {I^0} and M \leqslant {M^0} = 0 , we obtain \frac{{{c_1}\delta {I_0}}}{k} = {c_2}\xi . By considering {c_1} = \xi, {\text{ }}{c_2} = \frac{{\delta {I_0}}}{k}, and substituting {c_1} and {c_2} into Eq (4.9), the obtained result is as follows:
\begin{array}{l} {c_2} = \frac{{\xi \beta {I_0}}}{{1 + bS\left( t \right)}} + \frac{{\rho \delta {I_0}}}{k} - \xi \left( {\gamma + \varepsilon + \mu } \right) \\ \;\;\;\; \leqslant \xi \beta {I_0} + \frac{{\rho \delta {I_0}}}{k} - \xi \left( {\gamma + \varepsilon + \mu } \right){\text{ }}\left( {for{\text{ }}1 + bS\left( t \right) \geqslant 1} \right) \\ \;\;\;\; = \xi \left( {\gamma + \varepsilon + \mu } \right)\left( {{R_0} - 1} \right). \\ \end{array} |
If {R_0} \leqslant 1 , then {D^\alpha }V\left(t \right) \leqslant 0 . Specially, {D^\alpha }V\left(t \right) = 0 if and only if S\left(t \right) = 0 . Therefore, the largest compact invariant set in \left\{ {V\left({I, S, C, R, M} \right) \in \Omega :{D^\alpha }V\left(t \right) \leqslant 0} \right\} is the singleton set \left\{ {{E_0}} \right\} . According to LaSalle's invariance principle [43], {E_0} is globally asymptotically stable in \Omega if {R_0} \leqslant 0 .
Theorem 4.3. If {R_0} > 1 and {l_1}{l_2} - {l_3} > 0, {\text{ }}{l_1}{l_2}{l_3} - l_1^2{l_3} - l_3^2 > 0 , Eq (3.1) exhibits local stability of rumor-permanence equilibrium {E^*} .
Proof. Equation (3.1) at the rumor-permanence equilibrium {E^*} can be further simplified as follows:
\begin{array}{l} \Lambda - \frac{{\beta {I^*}{S^*}}}{{1 + b{S^*}}} - \frac{{\delta {I^*}{M^*}}}{{k + {M^*}}} - \eta {I^*} - \mu {I^*} = 0, \\ \frac{{\beta {I^*}{S^*}}}{{1 + b{S^*}}} + \frac{{\delta {I^*}{M^*}}}{{k + {M^*}}} - \gamma {S^*} - \varepsilon {S^*} - \mu {S^*} = 0, \\ \gamma {S^*} - \omega {C^*} - \mu {C^*} = 0, \\ {m_0} + \rho {S^*} - \xi {M^*} = 0. \\ \end{array} |
The Jacobian matrix evaluated at the rumor-permanence equilibrium {E^*} persistence is presented in [16] as
J\left({E}^{*}\right) = \left(\begin{array}{l}{J}_{11}^{*}{J}_{12}^{*}0{J}_{14}^{*}\\ {J}_{21}^{*}{J}_{22}^{*}0{J}_{24}^{*}\\ 0{J}_{32}^{*}{J}_{33}^{*}0\\ 0{J}_{42}^{*}0{J}_{44}^{*}\end{array}\right), |
where
\begin{array}{l} J_{11}^* = - \frac{{\beta {S^*}}}{{1 + b{S^*}}} - \frac{{\delta {M^*}}}{{k + {M^*}}} - \eta - \mu , {\text{ }}J_{12}^* = - \frac{{\beta {I^*}}}{{{{\left( {1 + b{S^*}} \right)}^2}}}, {\text{ }}J_{14}^* = - \frac{{k\delta {I^*}}}{{{{\left( {k + {M^*}} \right)}^2}}}, \\ J_{21}^* = \frac{{\beta {S^*}}}{{1 + b{S^*}}} + \frac{{\delta {M^*}}}{{k + {M^*}}}, {\text{ }}J_{22}^* = \frac{{\beta {I^*}}}{{{{\left( {1 + b{S^*}} \right)}^2}}} - \gamma - \varepsilon - \mu , {\text{ }}J_{24}^* = \frac{{k\delta {I^*}}}{{{{\left( {k + {M^*}} \right)}^2}}}, \\ J_{32}^* = \gamma , {\text{ }}J_{33}^* = - \omega , {\text{ }}J_{42}^* = \rho , {\text{ }}J_{44}^* = - \xi . \\ \end{array} |
The corresponding characteristic equation can be derived as
{\lambda ^4} + {l_1}{\lambda ^3} + {l_2}{\lambda ^2} + {l_3}\lambda + {l_4} = 0, | (4.10) |
where
\begin{array}{l} {l_1} = - J_{11}^* - J_{22}^* - J_{33}^* - J_{44}^*, \\ {l_2} = J_{11}^*J_{22}^* - J_{12}^*J_{21}^* + J_{11}^*J_{33}^* + J_{11}^*J_{44}^* + J_{22}^*J_{33}^* + J_{22}^*J_{44}^* - J_{24}^*J_{42}^* + J_{33}^*J_{44}^*, \\ {l_3} = - J_{11}^*J_{22}^*J_{33}^* + J_{12}^*J_{21}^*J_{33}^* - J_{11}^*J_{24}^*J_{42}^* + J_{12}^*J_{21}^*J_{44}^* - J_{14}^*J_{21}^*J_{42}^* \\ \;\;\;\;\;\; - J_{11}^*J_{33}^*J_{44}^* - J_{22}^*J_{33}^*J_{44}^* + J_{24}^*J_{33}^*J_{42}^*, \\ {l_4} = J_{11}^*J_{22}^*J_{33}^*J_{44}^* - J_{11}^*J_{24}^*J_{33}^*J_{42}^* - J_{12}^*J_{21}^*J_{33}^*J_{44}^* + J_{14}^*J_{21}^*J_{33}^*J_{42}^*. \\ \end{array} |
For {R_0} > 1 , J_{ii}^*{\text{ }}\left({i = 1, 2, 3, 4} \right), J_{12}^* and J_{14}^* are all negative, while J_{21}^*, J_{24}^*, J_{32}^* and J_{42}^* are positive. Subsequently, we obtain
\begin{array}{l} {\Delta _1} = {l_1} = - J_{11}^* - J_{22}^* - J_{33}^* - J_{44}^* > 0, \\ {\Delta _2} = \left| \begin{array}{l} {l_1}\;\;\;\;1 \\ {l_3}\;\;\;\;{l_2} \\ \end{array} \right| = {l_1}{l_2} - {l_3}, \\ {\Delta _3} = \left( \begin{array}{l} {l_1}\;\;\;\;1\;\;\;\;0 \\ {l_3}\;\;\;\;{l_2}\;\;\;{l_1} \\ 0\;\;\;\;{l_4}\;\;\;\;{l_3} \\ \end{array} \right) = {l_1}{l_2}{l_3} - l_1^2{l_3} - l_3^2. \\ \end{array} |
Obviously, it is {\Delta _1} > 0 . If {l_1}{l_2} - {l_3} > 0, {\text{ }}{l_1}{l_2}{l_3} - l_1^2{l_3} - l_3^2 > 0 , then {\Delta _2} > 0, {\text{ }}{\Delta _3} > 0 . According to the Routh-Hurwitz criterion [42], it can be inferred that all eigenvalues of the eigenequation in Eq (4.10) possess negative real parts.
Therefore, the rumor-permanence equilibrium {E^*} is proven to be locally asymptotically stable and Theorem 4.3 is proven.
The objective of our study is to identify the optimal control method and approach in order to minimize the overall cost associated with rumor spreading. The feasible intervention method aims to minimize the dissemination of rumors by individuals. The primary objective of fractional optimal control is to mitigate the relative density of individuals who spread rumors. Furthermore, the consideration of cost control measures is imperative in light of the current circumstances. Therefore, the optimal control strategy for Eq (5.1) is proposed, and its investigation can be facilitated through the application of Pontryagin's maximum principle [44].
Equation (3.1), incorporating the control mechanism, is thus formulated as
\begin{array}{l} {D^\alpha }I\left( t \right) = \Lambda - \frac{{\beta \left( {1 - {u_1}\left( t \right)} \right)I\left( t \right)S\left( t \right)}}{{1 + bS\left( t \right)}} - \frac{{\delta I\left( t \right)M\left( t \right)}}{{k + M\left( t \right)}} - \eta I\left( t \right) - \mu I\left( t \right), \\ {D^\alpha }S\left( t \right) = \frac{{\beta \left( {1 - {u_1}\left( t \right)} \right)I\left( t \right)S\left( t \right)}}{{1 + bS\left( t \right)}} + \frac{{\delta I\left( t \right)M\left( t \right)}}{{k + M\left( t \right)}} - \gamma S\left( t \right) - \varepsilon {u_2}\left( t \right)S\left( t \right) - \mu S\left( t \right), \\ {D^\alpha }C\left( t \right) = \gamma S\left( t \right) - \omega {u_3}\left( t \right)C\left( t \right) - \mu C\left( t \right), \\ {D^\alpha }R\left( t \right) = \eta I\left( t \right) + \varepsilon {u_2}\left( t \right)S\left( t \right) + \omega {u_3}\left( t \right)C\left( t \right) - \mu R\left( t \right), \\ {D^\alpha }M\left( t \right) = {m_0} + \rho S\left( t \right) - \xi M\left( t \right), \\ \end{array} | (5.1) |
where the control strengths to the rumor propagation are represented by {u_1}\left(t \right), {\text{ }}{u_2}\left(t \right) and {u_3}\left(t \right) through education or government management. The objective function of the optimal control is formulated as
Minimize \;J\left({u}_{1}, {u}_{2}, {u}_{3}\right) = {\displaystyle {\int }_{0}^{T}\left({A}_{1}S\left(t\right)+{A}_{2}C\left(t\right)+{K}_{1}\frac{{u}_{1}^{2}}{2}+{K}_{2}\frac{{u}_{2}^{2}}{2}+{K}_{3}\frac{{u}_{3}^{2}}{2}\right)dt}\text{,} | (5.2) |
where {A_1} and {A_2} are the non-negative weights related to S\left(t \right) and C\left(t \right) in Eq (5.2). {K_1}, {\text{ }}{K_2}, and {K_3} are relative to cost of the measures.
To determine the optimal interventions proposed in Eq (5.1),
U\left\{ {\left. {\left( {{u_1}\left( t \right), {u_2}\left( t \right), {u_3}\left( t \right)} \right)} \right|0 \leqslant {u_1}\left( t \right) \leqslant u_1^{\max }, 0 \leqslant {u_2}\left( t \right) \leqslant u_2^{\max }, 0 \leqslant {u_3}\left( t \right) \leqslant u_3^{\max }, t \in \left( {0, T} \right]} \right\}, | (5.3) |
where u_1^{\max } < 1, {\text{ }}u_2^{\max } < 1, and u_3^{\max } < 1 are the upper bounds of {u_1}\left(t \right), {\text{ }}{u_2}\left(t \right), and {u_3}\left(t \right) , respectively. Optimal controls u_1^*, u_2^*, and u_3^* satisfy J\left({u_1^*, u_2^*, u_2^*} \right) = \min \left\{ {J\left({{u_1}\left(t \right), {u_2}\left(t \right), {u_3}\left(t \right)} \right):} \right. \left. {\left({{u_1}\left(t \right), {u_2}\left(t \right), {u_3}\left(t \right)} \right) \in U} \right\} .
We derive the optimal controls for minimizing the rumor propagation at minimal cost. Consequently, the following Lagrangian function is obtained:
\begin{array}{l}{\rm H} = {A}_{1}S\left(t\right)+{A}_{2}C\left(t\right)+{K}_{1}\frac{{u}_{1}^{2}}{2}+{K}_{2}\frac{{u}_{2}^{2}}{2}+{K}_{3}\frac{{u}_{3}^{2}}{2}\\ \text{ }+{\lambda }_{1}\left(\Lambda -\frac{\beta \left(1-{u}_{1}\left(t\right)\right)I\left(t\right)S\left(t\right)}{1+bS\left(t\right)}-\frac{\delta I\left(t\right)M\left(t\right)}{k+M\left(t\right)}-\eta I\left(t\right)-\mu I\left(t\right)\right)\\ \text{ }+{\lambda }_{2}\left(\frac{\beta \left(1-{u}_{1}\left(t\right)\right)I\left(t\right)S\left(t\right)}{1+bS\left(t\right)}+\frac{\delta I\left(t\right)M\left(t\right)}{k+M\left(t\right)}-\left(\gamma +\varepsilon {u}_{2}\left(t\right)+\mu \right)S\left(t\right)\right)\\ \text{ }+{\lambda }_{3}\left(\gamma S\left(t\right)-\omega {u}_{3}\left(t\right)C\left(t\right)-\mu C\left(t\right)\right)\\ \text{ }+{\lambda }_{4}\left(\eta I\left(t\right)+\varepsilon {u}_{2}\left(t\right)S\left(t\right)+\omega {u}_{3}\left(t\right)C\left(t\right)-\mu R\left(t\right)\right)\\ \text{ }+{\lambda }_{5}\left({m}_{0}+\rho S\left(t\right)-\xi M\left(t\right)\right)\text{,}\end{array} | (5.4) |
where {\lambda _1}, {\lambda _2}, {\lambda _3}, {\lambda _4}, {\lambda _5} are adjoint variable functions.
Theorem 5.1. There exists an optimal control with the solution \left({I, S, C, R, M} \right) corresponding to the state equation in Eq (5.1) and the adjoint variables {\lambda _1}, {\lambda _2}, {\lambda _3}, {\lambda _4}, {\lambda _5} , we obtain
\begin{array}{l} {D^\alpha }{\lambda _1} = - {\lambda _1}\left( {\frac{{\beta \left( {1 - {u_1}} \right)S}}{{1 + bS}} - \frac{{\delta M}}{{k + M}} + \eta + \mu } \right) - {\lambda _2}\rho \left( {\frac{{\beta \left( {1 - {u_1}} \right)S}}{{1 + bS}} - \frac{{\delta M}}{{k + M}}} \right) + {\lambda _4}\eta , \\ {D^\alpha }{\lambda _2} = \frac{{ - {\lambda _1}\beta \left( {1 - {u_1}} \right)I}}{{{{\left( {1 + bS} \right)}^2}}} + {\lambda _2}\left( {\frac{{\beta \left( {1 - {u_1}} \right)I}}{{{{\left( {1 + bS} \right)}^2}}} - \left( {\gamma + \varepsilon {u_2}\left( t \right) + \mu } \right)} \right) - {\lambda _3}\gamma - {\lambda _4}\varepsilon {u_2}\left( t \right) - \;{\lambda _5}\rho , \\ {D^\alpha }{\lambda _3} = {\lambda _3}\left( {\omega {u_3}\left( t \right) + \mu } \right) - {\lambda _4}\omega {u_3}\left( t \right), \\ {D^\alpha }{\lambda _4} = {\lambda _4}\mu , \\ {D^\alpha }\;{\lambda _5} = {\lambda _1}\frac{{k\delta I}}{{{{\left( {k + M} \right)}^2}}} - {\lambda _2}\frac{{k\delta I}}{{{{\left( {k + M} \right)}^2}}} + \;{\lambda _5}\xi M, \\ \end{array} |
with boundary conditions {\lambda _1}\left(t \right) = {\lambda _2}\left(t \right) = {\lambda _3}\left(t \right) = {\lambda _4}\left(t \right) = {\lambda _5}\left(t \right) = 0. According to the adjoint variables I\left(t \right), S\left(t \right), C\left(t \right), R\left(t \right) and M\left(t \right) , we differentiate the Hamiltonian {\rm H} in Eq (5.2) with respect to each variable.
Proof. From the adjoint variables I\left(t \right), S\left(t \right), C\left(t \right), R\left(t \right) and M\left(t \right) , we differentiate the Hamiltonian {\rm H} in Eq (5.2) with respect to each variable:
\begin{array}{l} {D^\alpha }{\lambda _1} = \frac{{ - \partial {\rm H}}}{{\partial I}} = {\lambda _1}\left( {\frac{{\beta \left( {1 - {u_1}} \right)S}}{{1 + bS}} - \frac{{\delta M}}{{k + M}} + \eta + \mu } \right) - {\lambda _2}\rho \left( {\frac{{\beta \left( {1 - {u_1}} \right)S}}{{1 + bS}} - \frac{{\delta M}}{{k + M}}} \right) + {\lambda _4}\eta , \\ {D^\alpha }{\lambda _2} = \frac{{ - \partial {\rm H}}}{{\partial S}} = \frac{{ - {\lambda _1}\beta \left( {1 - {u_1}} \right)I}}{{{{\left( {1 + bS} \right)}^2}}} + {\lambda _2}\left( {\frac{{\beta \left( {1 - {u_1}} \right)I}}{{{{\left( {1 + bS} \right)}^2}}} - \left( {\gamma + \varepsilon {u_2}\left( t \right) + \mu } \right)} \right) - {\lambda _3}\gamma - {\lambda _4}\varepsilon {u_2}\left( t \right) - \;{\lambda _5}\rho , \\ {D^\alpha }{\lambda _3} = \frac{{ - \partial {\rm H}}}{{\partial C}} = {\lambda _3}\left( {\omega {u_3}\left( t \right) + \mu } \right) - {\lambda _4}\omega {u_3}\left( t \right), \\ {D^\alpha }{\lambda _4} = \frac{{ - \partial {\rm H}}}{{\partial R}} = {\lambda _4}\mu , \\ {D^\alpha }\;{\lambda _5} = \frac{{ - \partial {\rm H}}}{{\partial M}} = {\lambda _1}\frac{{k\delta I}}{{{{\left( {k + M} \right)}^2}}} - {\lambda _2}\frac{{k\delta I}}{{{{\left( {k + M} \right)}^2}}} + \;{\lambda _5}\xi M. \\ \end{array} |
Moreover, the optimal control of the control variables set {u_1}, {u_2}, {u_3} is attained by partial differential equation as follows:
\frac{{\partial {\rm H}\left( {I, S, C, R, M} \right)}}{{\partial {u_i}}} = 0, {\text{ }}i = 1, 2, 3. |
For u_1^*, {\text{ }}\frac{{\partial {\rm H}\left({I, S, C, R, M} \right)}}{{\partial {u_1}}} = 0,
u_1^* = \frac{{\beta SI\left( {{\lambda _1} - {\lambda _2}} \right)}}{{{K_1}}}. |
For u_2^*, \frac{{\partial {\rm H}\left({I, S, C, R, M} \right)}}{{\partial {u_2}}} = 0,
u_2^* = \frac{{\varepsilon S\left( { - {\lambda _2} + \;{\lambda _4}} \right)}}{{{K_2}}}. |
For u_3^*, \frac{{\partial {\rm H}\left({I, S, C, R, M} \right)}}{{\partial {u_3}}} = 0,
u_3^* = \frac{{\omega C\left( { - {\lambda _3} + {\lambda _4}} \right)}}{{{K_3}}}. |
Therefore,
\begin{array}{l} u_1^* = \max \left\{ {0, \min \left( {1, \frac{{\omega C\left( { - {\lambda _3} + {\lambda _4}} \right)}}{{{K_3}}}} \right)} \right\}, \\ u_2^* = \max \left\{ {0, \min \left( {1, \frac{{\varepsilon S\left( { - {\lambda _2} + \;{\lambda _4}} \right)}}{{{K_2}}}} \right)} \right\}, \\ u_3^* = \max \left\{ {0, \min \left( {1, \frac{{\omega C\left( { - {\lambda _3} + {\lambda _4}} \right)}}{{{K_3}}}} \right)} \right\}. \\ \end{array} |
The uniqueness of the optimal control has been established based on the boundedness and adjoint variables. The standard control with a bound is obtained as follows:
{u}_{1}^{*} = \left\{ \begin{array}{l}0, \;\;\;\;\;\;\;\;\;if\;\;\;\frac{\beta SI\left({\lambda }_{1}-{\lambda }_{2}\right)}{{K}_{1}} < 0, \\ \left(0, 1\right),\;\;\;if\;\;\;\frac{\beta SI\left({\lambda }_{1}-{\lambda }_{2}\right)}{{K}_{1}}\le 1, \\ 1, \;\;\;\;\;\;\;\;\;if\;\;\;\frac{\beta SI\left({\lambda }_{1}-{\lambda }_{2}\right)}{{K}_{1}} > 1, \end{array} \right. |
{u}_{2}^{*} = \left\{ \begin{array}{l}0, \;\;\;\;\;\;\;\;\;if\;\;\;\frac{\varepsilon S\left(-{\lambda }_{2}+{\lambda }_{4}\right)}{{K}_{2}} < 0, \\ \left(0, 1\right),\;\;\;if\;\;\;\frac{\varepsilon S\left(-{\lambda }_{2}+{\lambda }_{4}\right)}{{K}_{2}}\le 1, \\ 1, \;\;\;\;\;\;\;\;\;if\;\;\;\frac{\varepsilon S\left(-{\lambda }_{2}+{\lambda }_{4}\right)}{{K}_{2}} > 1, \end{array} \right. |
{u}_{3}^{*} = \left\{ \begin{array}{l}0, \;\;\;\;\;\;\;\;\;if\;\;\;\frac{\omega C\left(-{\lambda }_{3}+{\lambda }_{4}\right)}{{K}_{3}} < 0, \\ \left(0, 1\right),\;\;\;if\;\;\;\frac{\omega C\left(-{\lambda }_{3}+{\lambda }_{4}\right)}{{K}_{3}}\le 1, \\ 1, \;\;\;\;\;\;\;\;\;if\;\;\;\frac{\omega C\left(-{\lambda }_{3}+{\lambda }_{4}\right)}{{K}_{3}} > 1.\end{array} \right. |
The sensitivity of the parameters in the rumor propagation model is investigated in this section. The following conditions are satisfied:
\begin{array}{l} {A_\beta } = \frac{\beta }{{{R_0}}}\frac{{\partial {R_0}}}{{\partial \beta }} = \frac{{k\xi \beta }}{{k\xi \beta + \rho \delta }} > 0, \\ {A_\rho } = \frac{\rho }{{{R_0}}}\frac{{\partial {R_0}}}{{\partial \rho }} = \frac{{\rho \delta }}{{k\xi \beta + \rho \delta }} > 0, \\ {A_\delta } = \frac{\delta }{{{R_0}}}\frac{{\partial {R_0}}}{{\partial \delta }} = \frac{{\rho \delta }}{{k\xi \beta + \rho \delta }} > 0, \\ {A_\eta } = \frac{\eta }{{{R_0}}}\frac{{\partial {R_0}}}{{\partial \eta }} = - \frac{\eta }{{\left( {\eta + \mu } \right)}} < 0. \\ \end{array} |
In conclusion, \beta , \rho , and \delta are sensitive, and \eta , analogous to \gamma and \varepsilon are not sensitive. Sensitivity analysis shows that it is possible to reduce the rumor contact rate \beta and parameters \rho and \delta , thus lowering the value of {R_0} .
In this section, we present the fractional Euler method [45], which is employed in solving fractional-order differential equations and obtaining a discretized equation, as described below:
\begin{array}{l} I\left( {{t_p}} \right) = I\left( {{t_0}} \right) + \frac{{{h^\alpha }}}{{\Gamma \left( {\alpha + 1} \right)}}\sum\limits_{j = 0}^{p - 1} {{c_{j, p}}\left[ {\Lambda - \frac{{\beta I\left( {{t_j}} \right)S\left( {{t_j}} \right)}}{{1 + bS\left( {{t_j}} \right)}} - \frac{{\delta I\left( {{t_j}} \right)M\left( {{t_j}} \right)}}{{k + M\left( {{t_j}} \right)}} - \left( {\eta + \mu } \right)I\left( {{t_j}} \right)} \right]} , \\ S\left( {{t_p}} \right) = S\left( {{t_0}} \right) + \frac{{{h^\alpha }}}{{\Gamma \left( {\alpha + 1} \right)}}\sum\limits_{j = 0}^{p - 1} {{c_{j, p}}\left[ {\frac{{\beta I\left( {{t_j}} \right)S\left( {{t_j}} \right)}}{{1 + bS\left( {{t_j}} \right)}} + \frac{{\delta I\left( {{t_j}} \right)M\left( {{t_j}} \right)}}{{k + M\left( {{t_j}} \right)}} - \left( {\gamma + \varepsilon + \mu } \right)S\left( {{t_j}} \right)} \right]} , \\ C\left( {{t_p}} \right) = C\left( {{t_0}} \right) + \frac{{{h^\alpha }}}{{\Gamma \left( {\alpha + 1} \right)}}\sum\limits_{j = 0}^{p - 1} {{c_{j, p}}\left[ {\gamma S\left( {{t_j}} \right) - \left( {\omega + \mu } \right)C\left( {{t_j}} \right)} \right]} , \\ R\left( {{t_p}} \right) = R\left( {{t_0}} \right) + \frac{{{h^\alpha }}}{{\Gamma \left( {\alpha + 1} \right)}}\sum\limits_{j = 0}^{p - 1} {{c_{j, p}}\left[ {\eta I\left( {{t_j}} \right) + \varepsilon S\left( {{t_j}} \right) + \omega C\left( {{t_j}} \right) - \mu R\left( {{t_j}} \right)} \right]} , \\ M\left( {{t_p}} \right) = M\left( {{t_0}} \right) + \frac{{{h^\alpha }}}{{\Gamma \left( {\alpha + 1} \right)}}\sum\limits_{j = 0}^{p - 1} {{c_{j, p}}\left[ {{m_0} + \rho M\left( {{t_j}} \right) - \xi M\left( {{t_j}} \right)} \right]} . \\ \end{array} |
for p = 1, 2, \ldots, N where {c_{j, p}} = {\left({p - j} \right)^\alpha } - {\left({p - 1 - j} \right)^\alpha } . The fractional Euler method is employed for the subsequent numerical solutions of the fractional-order differential equations.
In this section, we conduct numerical simulations to validate the findings presented in the preceding sections. The initial values are considered as I\left(0 \right) = 10500, {\text{ }}S\left(0 \right) = 1500, C\left(0 \right) = 750, {\text{ }}R\left(0 \right) = 750, and M\left(0 \right) = 1500 . Additionally, the parametric values are listed in Table 1. Obviously, the basic reproduction number is {{\text{R}}_0}{\text{ = 0}}{\text{.4}} < 1 . Figure 2 provides graphs of the evolution of (a) Ignorants, (b) Spreaders, (c) Calmness, (d) Removers, and (e) Media Reports in the rumor-free case for Eq (3.1), employing various values of the differentiation parameter \alpha = 0.6, 0.7, 0.8, 0.9, 1. With the decrease of the order \alpha , the convergence rate of the rumor propagation curve slows down and eventually tends to stabilize, which shows the memory effect. From the perspective of public opinion dissemination, it implies that such rumors tend to persist for an extended duration. Moreover, it demonstrates an anomalous propagation pattern at a lower order \alpha , characterized by an exponentially rapid growth in the initial phase as depicted in Figure 3(b). Meanwhile, the subsequent phase exhibits a deceleration. When the order is \alpha = 1 , the fractional-order differential equation turns into an integer-order one.
Parameter | Value | Parameter | Value |
\Lambda | 0.4 | \omega | 0.4 |
\beta | 0.1 | \eta | 0.2 |
\delta | 0.2 | \varepsilon | 0.5 |
b | 0.5 | {m_0} | 0.2 |
k | 0.5 | \rho | 0.2 |
\xi | 0.3 | \mu | 0.05 |
\gamma | 0.5 |
The initial values are also set to I\left(0 \right) = 10500, {\text{ }}S\left(0 \right) = 1500, {\text{ }}C\left(0 \right) = 750, {\text{ }}R\left(0 \right) = 750, and M\left(0 \right) = 1500 . Furthermore, the parametric values are provided in Table 2. The calculation result of the basic reproduction number is {{\text{R}}_0}{\text{ = 1}}{\text{.32}} > 1 . The results corresponding to the simulation of the rumors case are presented in Figure 3 using various values of the differentiation parameter \alpha = 0.6, 0.7, 0.8, 0.9, 1. The convergence rates of rumor propagation vary depending on the order value \alpha . The impact of the fractional order is observed through numerical simulation in Figure 3, from which it is demonstrated that reducing the value \alpha can enhance the stability range of model (3.1). The larger the order \alpha , the more rapidly the curve approaches stability in the rumor-permanence case. Therefore, it is imperative to consistently and promptly disseminate scientific knowledge while actively debunking rumors in order to effectively suppress rumor propagation.
Parameter | Value | Parameter | Value |
\Lambda | 1.2 | \omega | 0.4 |
\beta | 0.6 | \eta | 0.2 |
\delta | 0.5 | \varepsilon | 0.6 |
b | 1 | {m_0} | 0.6 |
k | 1 | \rho | 0.2 |
\xi | 0.3 | \mu | 0.3 |
\gamma | 0.5 |
To illustrate the effectiveness of the media reports, for the basic reproduction number {R_0} > 1, {\text{ }}\alpha {\text{ = }}0.95 is established with the parametric values in Table 2. The media reports have an impact on Eq (3.1) governing fractional-order \alpha {\text{ = }}0.95 as illustrated in Figure 4. The peak of the Spreaders group decreases as the value of k increases, as shown in Figure 4(b). The statement suggests that there is a positive correlation between the level of media reports and the influence on rumor propagation. Therefore, employing appropriate media strategies can effectively suppress the rumor propagation.
To demonstrate the effectiveness of the fractional optimal control strategy, for the basic reproduction number {R_0} > 1, {\text{ }}\alpha {\text{ = }}0.95 is established with the parametric values in Table 2. Figure 5 shows the populations of I\left(t \right), S\left(t \right), C\left(t \right), R\left(t \right), and M\left(t \right) , with or without control mechanism. Compared with Eq (5.1), with or without control strategy, the populations of the Ignorants and the Spreaders are significantly influenced. This implies that implementing an effective control mechanism can significantly restrict the rumor diffusion.
A Caputo sense-based ISCRM rumor propagation model incorporating media reports is proposed. The existence of positive solutions is rigorously established in this study, and the equilibrium solutions are determined for both the rumor-free equilibrium and rumor-permanence equilibrium, along with their local properties. The rumor-free equilibrium {E_0} is locally and globally asymptotically stable if {R_0} < 1 . The instability of the unique rumor-permanence equilibrium {E^*} exists when {R_0} > 1 , and for {l_1}{l_2} - {l_3} > 0, {\text{ }}{l_1}{l_2}{l_3} - l_1^2{l_3} - l_3^2 > 0 , it is locally asymptotically stable. The analytical research was validated by conducting numerical simulations, encompassing a range of fractional orders and initial compartment sizes. In the case of {R_0} < 1 , stability is rapidly achieved at lower fractional orders, whereas in the case of {R_0} > 1 , the attainment of stability is rapidly achieved at higher orders. The significance of fractional analysis has been underscored by the achievement of significantly improved and more realistic outcomes. Additionally, a comprehensive analysis of the impact of media reports and an optimal control strategy is presented. The effectiveness of rumor diffusion prevention can be significantly enhanced through the implementation of a control strategy and media reports, thereby mitigating the risk associated with rumor propagation. In future research, the investigation of time delay effects [46] and stochastic factors [47,48] could be expanded within the framework of complex networks in Caputo sense systems.
The authors declare they have not used Artificial Intelligence (AI) tools in the creation of this article.
The authors declare that they have no known competing financial interests or personal relationships that could have appeared to influence the work reported in this paper.
[1] | Warren SD, Clair LLS, Stark LR, et al. (2019) Reproduction and dispersal of biological soil crust organisms. Frontiers in Ecology and Evolution 7: 344. |
[2] | Warren SD Ecological role of microphytic soil crusts in arid ecosystems[C]//Microbial diversity and ecosystem function: proceedings of the IUBS/IUMS Workshop held at Egham, UK, 10-13 August 1993. CAB INTERNATIONAL, 1995: 199-209. |
[3] | Belnap J, (2013) Biological soil crusts: structure, function, and management. Berlin: Springer Science & Business Media. |
[4] | Belnap J, Weber B, Büdel B (2016) Biological soil crusts as an organizing principle in drylands. Biological soil crusts: an organizing principle in drylands. Springer, Cham. 3-13. |
[5] | Warren SD (2001) Synopsis: influence of biological soil crusts on arid land hydrology and soil stability. Biological soil crusts: Structure, function, and management. Springer, Berlin, Heidelberg. 349-360. |
[6] | Belnap J, (2001) Biological soil crusts and wind erosion. Biological soil crusts: Structure, function, and management. Springer, Berlin, Heidelberg, 339-347. |
[7] |
Fick SE, Barger N, Tatarko J, et al. (2020) Induced biological soil crust controls on wind erodibility and dust (PM10) emissions. Earth Surf Proc Land 45: 224-236. doi: 10.1002/esp.4731
![]() |
[8] |
Tisdall JM, OADES JM (1982) Organic matter and water-stable aggregates in soils. J Soil Sci 33: 141-163. doi: 10.1111/j.1365-2389.1982.tb01755.x
![]() |
[9] |
Schulten JA (1985) Soil aggregation by cryptogams of a sand prairie. Am J Bot 72: 1657-1661. doi: 10.1002/j.1537-2197.1985.tb08433.x
![]() |
[10] | Belnap J, Gardner JS (1993) Soil microstructure in soils of the Colorado Plateau: the role of the cyanobacterium Microcoleus vaginatus. Great Basin Nat 1993: 40-47. |
[11] | McCalla TM (1946) Influence of some microbial groups on stabilizing soil structure against falling water drops. Soil Sci Soc Am Pro 11: 260-263. |
[12] | Osborn B (1952) Range soil conditions influence water intake. J Soil Water Conserv 7: 128-132. |
[13] |
Gao GL, Ding GD, Wu B, et al. (2014) Fractal scaling of particle size distribution and relationships with topsoil properties affected by biological soil crusts. Plos One 9: e88559. doi: 10.1371/journal.pone.0088559
![]() |
[14] |
Weber B, Wu D, Tamm A, et al. (2015) Biological soil crusts accelerate the nitrogen cycle through large NO and HONO emissions in drylands. Proc Natl Acad Sci 112: 15384-15389. doi: 10.1073/pnas.1515818112
![]() |
[15] |
Elbert W, Weber B, Burrows S, et al. (2012) Contribution of cryptogamic covers to the global cycles of carbon and nitrogen. Nat Geosci 5: 459-462. doi: 10.1038/ngeo1486
![]() |
[16] | Loope WL, Gifford GF (1972) Influence of soil microfloral crust on select properties of soils under pinyon-juniper in southwestern Utah. J Soil Water Conserv 17: 164-167. |
[17] |
Kleiner EF, Harper KT (1972) Environment and community organization in grasslands of Canyonlands National Park. Ecology 53: 299-309. doi: 10.2307/1934086
![]() |
[18] | Harper KT, Pendleton RL (1993) Cyanobacteria and cyanolichens: can they enhance availability of essential minerals for higher plants? Great Basin Nat 53: 59-72. |
[19] | Metting B, (1991) Biological soil features of semiarid lands and deserts. Pages 257-293 in Skujiņš J[ed.] Semiarid lands and deserts. Marcel Dekker: New York. |
[20] | Edwards HGM, Villar SEJ, Seaward MRD, et al. 2004. Raman spectroscopy of rock biodeterioration by the lichen Lecidea tesselata Flörke in an arid desert environment, Utah, USA, 229-240, In St. Clair LL, Seaward MRD (Eds.), Biodeterioration of Stone Surfaces: Lichens and Biofilms as Weathering Agents of Rocks and Cultural Heritage. Dordrecht, the Netherlands: Kluwer Academic Publishers. |
[21] |
Lange W (1974) Chelating agents and blue-green algae. Can J Microbiol 20: 1311-1321. doi: 10.1139/m74-204
![]() |
[22] |
Lange W (1976) Speculations on a possible essential function of the gelatinous sheath of blue-green algae. Can J Microbiol 22: 1181-1185. doi: 10.1139/m76-171
![]() |
[23] | St Clair, LL, Well BL, Johansen JR, et al. (1984) Cryptogamic soil crusts: enhancement of seedling establishment in disturbed and undisturbed areas. Reclam Reveg Res 3: 129-136. |
[24] |
Li XR, Jia XH, Long LQ, et al. (2005) Effects of biological soil crusts on seed bank, germination and establishment of two annual plant species in the Tengger Desert (N China). Plant Soil 277: 375-385. doi: 10.1007/s11104-005-8162-4
![]() |
[25] | Zhang Y, Aradóttir AL, Serpe M, et al. (2016) Interactions of biological soil crusts with vascular plants. Chapter 19 in Belnap J, Weber B and Büdel B.[Eds.] Biological soil crusts: an organizing principle in drylands, Ecological studies 226. Springer: Cham, Switzerland. |
[26] |
Deines L, Rosentreter R, Eldridge DJ, et al. (2007) Germination and seedling establishment of two annual grasses on lichen-dominated biological soil crusts. Plant Soil 295: 23-35. doi: 10.1007/s11104-007-9256-y
![]() |
[27] | Godínez-Alvarez H, Morín C, Rivera-Aguilar V (2012) Germination, survival and growth of three vascular plants on biological soil crusts from a Mexican tropical desert. Plant Biology 14: 157-162. |
[28] |
Song G, Li X, Hui R (2017) Effect of biological soil crusts on seed germination and growth of an exotic and two native plant species in an arid ecosystem. PLoS One 12: e0185839. doi: 10.1371/journal.pone.0185839
![]() |
[29] |
Warren SD, St Clair LL, Johansen JR, et al. (2015) Effects of prescribed fire on biological soil crusts in a Great Basin juniper woodland. Rangeland Ecol Manage 68: 241-247. doi: 10.1016/j.rama.2015.03.007
![]() |
[30] | Warren SD, Rosentreter R, Pietrasiak N (2020) Biological soil crusts of the Great Plains. Rangeland Ecol Manage in press |
[31] |
Smith SM, Abed RMM, Gercia-Pichel F (2004) Biological soil crusts of sand dunes in Cape Cod National Seashore, Massachusetts, USA. Microbial Ecol 48:200-208. doi: 10.1007/s00248-004-0254-9
![]() |
[32] |
Thiet RK, Boerner REJ, Nagy M, et al. (2005) The effect of biological soil crusts on throughput of rainwater and N into Lake Michigan sand dune soils. Plant Soil 278: 235-251. doi: 10.1007/s11104-005-8550-9
![]() |
[33] |
Zellman KL (2014) Changes in vegetation and biological soil crust communities on sand dunes stabilizing after a century of grazing on San Miguel Island, Channel Islands National Park, California. Monogr West N Am Naturalist 7: 225-245. doi: 10.3398/042.007.0118
![]() |
[34] |
Schulz K, Mikhailyuk T, Dreßler M, et al. (2016) Biological soil crusts from coastal dunes at the Baltic Sea: cyanobacterial and algal biodiversity and related soil properties. Microbial Ecol 71: 178-193. doi: 10.1007/s00248-015-0691-7
![]() |
[35] |
Mikhailyuk T, Glaser K, Tsarenko P, et al. (2019) Composition of biological soil crusts from sand dunes of the Baltic Sea coast in the context of an integrative approach to the taxonomy of microalgae and cyanobacteria. Eur J Phycol 54: 263-290. doi: 10.1080/09670262.2018.1557257
![]() |
[36] |
Schaub I, Baum C, Schumann R, et al. (2019) Effects of an early successional biological soil crust from a temperate coastal sand dune (NE Germany) on soil elemental stoichiometry and phosphatase activity. Microbial Ecol 77: 217-229. doi: 10.1007/s00248-018-1220-2
![]() |
[37] |
Shubert LE, Starks TL (1980) Soil-algal relationships from surface mined soils. Brit Phycol J 15: 417-428. doi: 10.1080/00071618000650421
![]() |
[38] | Spröte R, Fischer T, Veste M, et al. (2010) Biological topsoil crusts at early successional stages on Quaternary substrates dumped by mining in Brandenburg, NE Germany. Géomorphologie 16: no. 4 |
[39] |
Warren SD, Clair LLS, Leavitt SD (2019) Aerobiology and passive restoration of biological soil crusts. Aerobiologia 35: 45-56. doi: 10.1007/s10453-018-9539-1
![]() |
[40] |
Evans Ogden L (2014) Life in the clouds. BioScience 64: 861-867. doi: 10.1093/biosci/biu144
![]() |
[41] |
Bowers RM, Lauber CL, Wiedinmyer C, et al. (2009) Characterization of airborne microbial communities at a high-elevation site and their potential to act as atmospheric ice nuclei. Appl Environ Microb 75: 5121-5130. doi: 10.1128/AEM.00447-09
![]() |
[42] |
Delort A M, Vaïtilingom M, Amato P, et al. (2010) A short overview of the microbial population in clouds: in atmospheric chemistry and nucleation processes. Atmos Res 98: 249-260. doi: 10.1016/j.atmosres.2010.07.004
![]() |
[43] |
Fan J, Leung LR, Rosenfeld D, et al. (2017) Effects of cloud condensation nuclei and ice nucleating particles on precipitation processes and supercooled liquid in mixed-phase orographic clouds. Atmos Chem Phys 17: 1017-1035. doi: 10.5194/acp-17-1017-2017
![]() |
[44] |
Hassett MO, Fischer MWF, Money NP (2015) Mushrooms as rainmakers: how spores act as nuclei for raindrops. PloS One 10(10): e0140407. doi: 10.1371/journal.pone.0140407
![]() |
[45] |
Woo C, An C, Xu S, et al. (2018) Taxonomic diversity of fungi deposited from the atmosphere. The ISME J 12: 2051-2060. doi: 10.1038/s41396-018-0160-7
![]() |
[46] |
Fröhlich-Nowoisky J, Kampf CJ, Weber B, et al. (2016) Bioaerosols in the Earth system: Climate, health, and ecosystem interactions. Atmos Res 182: 346-376. doi: 10.1016/j.atmosres.2016.07.018
![]() |
[47] | Bauer H, Giebl H, Hitzenberger R, et al. (2003) Airborne bacteria as cloud condensation nuclei. J Geophys Res: Atmos 108(D21). |
[48] |
Morris CE, Georgakopoulos DG, Sands DC (2004) Ice nucleation active bacteria and their potential role in precipitation. J de Physique IV Fr 121: 87-103. doi: 10.1051/jp4:2004121004
![]() |
[49] |
Lazardis M (2019) Bacteria as cloud condensation nuclei (CCN) in the atmosphere. Atmosphere 10: 786. doi: 10.3390/atmos10120786
![]() |
[50] |
K Sharma N, K Rai A, Singh S (2006) Meteorological factors affecting the diversity of airborne algae in an urban atmosphere. Ecography 29: 766-772. doi: 10.1111/j.2006.0906-7590.04554.x
![]() |
[51] |
Tesson SVM, Šantl-Temkiv T (2018) Ice nucleation activity and aeolian dispersal success in airborne and aquatic microalgae. Front Microbiol 9: 2681 doi: 10.3389/fmicb.2018.02681
![]() |
[52] | Wynn-Williams DD (1990) Ecological aspects of Antarctic microbiology. Chapter 3 in Marshall, K.C.[Ed], Advances in Microbial Ecol 11. Plenum Press: New York |
[53] | Byers HR (1949) Condensation nuclei and precipitation. J Meteorolo 6: 363. |
[54] |
Christner BC, Morris CE, Foreman CM, et al. (2008) Ubiquity of biological ice nucleators in snowfall. Science 319: 121465. doi: 10.1126/science.1149757
![]() |
[55] | Moffett BF (2015) Ice nucleation in mosses and liverworts. Lindbergia 38: 14-16. |
[56] | Georgia Institute of Technology (2011) Cloud formation: insoluble dust particles can form cloud droplets that affect global and regional climate. ScienceDaily 13 Oct 2011. www.sciencedaily.com/releases/2011/10/111013113814.html |
[57] |
Karydis VA, Kumar P, Barahona D, et al. (2011) On the effect of dust particles on global cloud condensation nuclei and cloud droplet number. J Geophys Res 116: D23204. doi: 10.1029/2011JD016283
![]() |
[58] |
Kerminen VM, Paramonov M, Anttila T, et al. (2012) Cloud condensation nuclei production associated with atmospheric nucleation: a synthesis based on existing literature and new results. Atmos Chem Phys 12: 12037-12059. doi: 10.5194/acp-12-12037-2012
![]() |
[59] |
Pope FD (2010) Pollen grains are efficient cloud condensation nuclei. Environ Res Lett 5: 044015. doi: 10.1088/1748-9326/5/4/044015
![]() |
[60] |
Holle RL (1971) Effects of cloud condensation nuclei and surface sources during south Florida droughts. J Appl Meteorol 10: 62-69. doi: 10.1175/1520-0450(1971)010<0062:EOCCND>2.0.CO;2
![]() |
[61] | Kobziar LN, Pingree MRA, Larson H, et al. (2018) Pyroaerobiology: the aerosolization and transport of viable microbial life by wildland life. Ecosphere 9: article e02507. |
[62] |
Mirskaya E, Agranovski IE (2020) Generation of viable bacterial and fungal aerosols during biomass combustion. Atmosphere 11: 313. doi: 10.3390/atmos11030313
![]() |
[63] |
Orellana MV, Matrai PA, Leck C, et al. (2011) Marine microgels as a source of cloud condensation nuclei in the high Arctic. Proc Natl Acad Sci 108: 13612-13617. doi: 10.1073/pnas.1102457108
![]() |
[64] | Sanchez KJ, Chen CL, Russell LM, et al. (2018) Substantial seasonal contribution of observed biogenic sulfate particles to cloud condensation nuclei. Sci R 8: 3235. |
[65] | Jiang B, Wang D, Shen X, et al. (2019) Effects of sea salt aerosols on precipitation and upper troposphere / lower stratosphere water vapour in tropical cyclone systems. Sci R 9: 15105. |
[66] | Sheil D (2018) Forests, atmospheric water and an uncertain future: the new biology of the global water cycle. Forest Ecosyst 5: article 19. |
[67] | Reguera, G. 2012. The colors of the microbial rainbow. https://schaechter.asmblog.org/schaechter/ |
[68] | Pointing S (2019) Microbes connect the earth, sea and sky. Nature Research: Microbiology: 21 Nov. htpps://go.nature.com/2KFhB |
[69] | Zagury F (2012) The color of the sky. Atmos Climate Sci 2: 510-517. |
[70] | University of Wisconsin-Madison. 2007. What determines sky's colors at sunrise and sunset? ScienceDaily, https://www.sciencedaily.com/releases/2007/11/071108135522.htm |
[71] | Fiegl A (2013) Red sky at night: the science of sunsets. National Geographic https://www.nationalgeographic.com/news/2013/10/131027-sunset-sky-change-color-red-clouds-science/ |
[72] | Corfidi SK (2014) The colors of sunset and twilight. https://www.spc.noaa.gov/publications/corfidi/sunset/ |
[73] | Ballantyne C (2007) Fact or fiction?: Smog creates beautiful sunsets. Sci Am 12 July 2007. |
[74] |
Gardner T, Acosta-Martinez V, Calderón FJ, et al. (2012) Pyrosequencing reveals bacteria carried in different wind-eroded sediments. J Environ Qual 41:744-753. doi: 10.2134/jeq2011.0347
![]() |
[75] |
Abed RMM, Ramette A, Hübner V, et al. (2012) Microbial diversity of eolian dust sources from saline lake sediments and biological soil crusts in arid Southern Australia. Fems Microbiol Ecol 80: 294-304. doi: 10.1111/j.1574-6941.2011.01289.x
![]() |
[76] |
Elliott DR, Thomas AD, Strong CL, et al. (2019) Surface stability in drylands is influenced by dispersal strategy of soil bacteria. J Geophys Res: Biogeosciences 124: 3403-3418. doi: 10.1029/2018JG004932
![]() |
[77] |
Ives RL (1947) Behavior of dust devils. Bulletin American Meteorological Society 28: 168-174 doi: 10.1175/1520-0477-28.4.168
![]() |
[78] |
Smith DJ, Griffin DW, Schuerger AC (2010) Stratospheric microbiology at 20 km over the Pacific Ocean. Aerobiologia 26: 35-46. doi: 10.1007/s10453-009-9141-7
![]() |
[79] |
DasSarma P, Laye VJ, Harvey J, et al. (2016) Survival of halophilic Archaea in Earth's cold stratosphere. Int J Astrobiology 16: 321-327. doi: 10.1017/S1473550416000410
![]() |
[80] |
DasSarma P, DasSarma S (2018) Survival of microbes in Earth's stratosphere. Curr Opin Microbiol 43: 24-30. doi: 10.1016/j.mib.2017.11.002
![]() |
[81] |
Barberán A, Ladau J, Leff J W, et al. (2015) Continental-scale distributions of dust-associated bacteria and fungi. PNAS 112: 5756-5761. doi: 10.1073/pnas.1420815112
![]() |
[82] |
Burrows SM, Elbert W, Lawrence MG, et al. (2009) Bacteria in the global atmosphere - Part 1: Review and synthesis of literature data for different ecosystems. Atmos Chem Phys 9: 9263-9280. doi: 10.5194/acp-9-9263-2009
![]() |
[83] |
Sharma NK, Rai AK, Singh S, et al. (2007) Airborne algae: their present status and relevance. J Phycol 43: 615-627. doi: 10.1111/j.1529-8817.2007.00373.x
![]() |
[84] | Tesson SVM, Skjøth CA, Šantl-Temkiv T, et al. (2016) Airborne microalgae: Insights, opportunities, and challenges. Appl Environ Microb 82: 7. |
[85] |
Frahm JP (2008) Diversity, dispersal and biogeography of bryophytes (mosses). Biodivers Conserv 17: 277-284. doi: 10.1007/s10531-007-9251-x
![]() |
[86] | Lönnell N (2014) Dispersal of bryophytes across landscapes. Stockholm University, Department of Ecology, Environment and Plant Sciences. PhD Thesis, 41 p. |
[87] | Löndahl J (2014) Physical and biological properties of bioaerosols. Chapter 4 in Jonsson, O., Olofsson, G., and Tjä rnhage, T.[Eds] Bioaerosol Detection Technologies. Integrated Analytical Systems. Springer-Verlag: New York. |
[88] | Clauß M (2015) Particle size distribution of airborne microorganisms in the environment-a review. Landbauforsch Appl Agric Forestry Res 65: 77-100. |
[89] | Womack AM, Bohannan BJM, Green JL (2010) Biodiversity and biogeography of the atmosphere. T Roy Soc A 365: 3645-3653. |
[90] |
Acosta-Martinez V, Van Pelt S, Moore-Kucera J, et al. (2015) Microbiology of wind-eroded, sediments: current knowledge and future. Aeolian Res 18: 99-113. doi: 10.1016/j.aeolia.2015.06.001
![]() |
[91] | He Y, Zhao C, Song M, et al. (2015) Onset of frequent dust storms in northern China. Sci R 5: 1711. |
[92] | Yongxiang H, Xiaomin F, Tianliang Z, et al. 2008. Long range trans-Pacific transport and deposition of Asian dust aerosols. J Environ Sci 20: 424-428. |
[93] | Yumimoto K, Eguchi K, Uno I, et al. 2010. Summertime trans-Pacific transport of Asian dust. Geophys Res Lett 37: L18815. |
[94] |
Guo J, Lou M, Miao Y, et al. (2017) Trans-Pacific transport of dust aerosols from East Asia: Insights gained from multiple observations and modeling. Environ Pollut 230: 1030-1039. doi: 10.1016/j.envpol.2017.07.062
![]() |
[95] | Barry PL (2001) The Pacific Dust Express. https://science.nasa.gov/science-news/science-at-nasa/2001/ast17may_1 |
[96] | Doherty OM, Riemer N, Hameed S (2008) Saharan mineral dust transport into the Caribbean: observed atmospheric controls and trends. J Geophys Res 113: DO7211. |
[97] |
Chen KY (2010) The northern path of Asian dust transport from the Gobi Desert to North America. Atmospheric and Oceanic Science Letters 3: 155-159. doi: 10.1080/16742834.2010.11446858
![]() |
[98] |
Creamean JM, Spackman JR, Davis SM, et al. (2014) Climatology of long-range transported Asian dust along the West Coast of the United States. J Geophys Res: Atmosarchaeapheres 119: 171-185. doi: 10.1002/2013JA019325
![]() |
[99] |
Liu L, Guo J, Gong H, et al. (2019) Contrasting influence of Gobi and Taklimakan Deserts on the dust aerosols in western North America. Geophys Res Lett 46: 9064-9071. doi: 10.1029/2019GL083508
![]() |
[100] |
Dunion JP, Velden CS (2004) The impact of the Saharan Air Layer on Atlantic cyclone activity. B Am Meteorol Soc 85: 353-365. doi: 10.1175/BAMS-85-3-353
![]() |
[101] |
Strong JDO, Vecchi GA, Ginoux P (2015) The response of the tropical Atlantic and west African climate to Saharan dust in a fully coupled GCM. J Climate 28: 7071-7092. doi: 10.1175/JCLI-D-14-00797.1
![]() |
[102] | Di Liberto T (2018) Dust from the Sahara Desert stretches across the tropical Atlantic Ocean in late June / early July 2018. National Oceanic and Atmospheric Administration. Climate.gov/print/832174 |
[103] | Kellogg CA, Griffin DW (2003) African dust carries microbes across the ocean: Are they affecting human and ecosystem health? United States Geological Survey Open-File Report 03-028. |
[104] |
Kellogg CA, Griffin DW, Garrison VH, et al. (2004) Characterization of aerosolized bacteria and fungi from desert dust events in Mali, West Africa. Aerobiologia 20: 99-110. doi: 10.1023/B:AERO.0000032947.88335.bb
![]() |
[105] |
Griffin D W, Kellogg C A, Garrison V H, et al. (2002) The global transport of dust: an intercontinental river of dust, microorganisms and toxic chemicals flows through the Earth's atmosphere. Am Sci 90: 228-235. doi: 10.1511/2002.3.228
![]() |
[106] |
Gat D, Mazar Y, Cytryn E, et al. (2017) Origin-dependent variations in the atmospheric microbiome community in eastern Mediterranean dust storms. Environ Sci Technol 51: 6709-6718. doi: 10.1021/acs.est.7b00362
![]() |
[107] |
Behzad H, Mineta K, Gojobori T (2018) Global ramifications of dust and sandstorm microbiota. Genome Biol Evol 10: 1970-1987. doi: 10.1093/gbe/evy134
![]() |
[108] | Dregne HE (1984) North American deserts. In: El-Baz, F. (Ed), Deserts and arid lands. Remote sensing of earth resources and environment. 1. Springer: Dordrecht |
[109] | Achakulwisut P, Shen L, Mickley LJ (2017) What controls springtime fine dust variability in the western United States? Investigating the 2002-2015 increase in fine dust in the U.S southwest. J Geophys Res: Atmos 122: 12449-12467. |
[110] |
Chazette P, Flamant C, Totems J, et al (2019) Evidence of the complexity of aerosol transport in the lower troposphere on the Namibian coast during AEROCLO-sA. Atmos Chem Phys 19: 14979-15005. doi: 10.5194/acp-19-14979-2019
![]() |
[111] |
Nguyen HD, Riley M, Leys J, et al. (2019) Dust storm event of February 2019 in central and east coast of Australia and evidence of long-range transport to New Zealand and Antarctica. Atmosphere 10: 653. doi: 10.3390/atmos10110653
![]() |
[112] | Azua-Bustos A, González-Silva C, Fernández-Martínez M Á, et al. (2019) Aeolian transport of viable microbial life across the Atacama Desert, Chile. Sci R 9: 11024. |
[113] |
Li J, Wang F, Michalski G, et al. (2019) Atmospheric deposition across the Atacama Desert, Chile: Composition, source distribution, and interannual comparisons. Chem Geol 525: 435-446. doi: 10.1016/j.chemgeo.2019.07.037
![]() |
[114] |
Aarons SM, Aciego SM, McConnell JR, et al. (2019) Dust transport in Taylor Glacier, Antarctica, during the last interglacial. Geophys Res Lett 46: 2261-2270. doi: 10.1029/2018GL081887
![]() |
[115] |
Kavan J, Dagsson-Waldhauserova P, Renard JB, et al. (2018) Aerosol concentration in relationship to local atmospheric conditions on James Ross Island, Antarctica. Front Earth Sci 6: 207. doi: 10.3389/feart.2018.00207
![]() |
[116] | Herren CM (2019) Asexual reproduction can account for the high diversity and prevalence of rare taxa observed in microbial communities. Appl Environ Microb 85: e01099-19. |
[117] |
Mayol E, Arrieta J M, Jiménez M A, et al. (2017) Long-range transport of airborne microbes over the global tropical and subtropical ocean. Nature Commun 8: 201. doi: 10.1038/s41467-017-00110-9
![]() |
[118] | Persson, A. 1998. How do we understand the Coriolis force? B Am Meteorol Soc 79: 1373-1385. |
[119] |
Peters DHW, Schneidereit A, Karpechko AY (2018) Enhanced stratosphere/troposphere coupling during extreme warm stratospheric events with strong polar-night jet oscillation. Atmosphere 9: 467. doi: 10.3390/atmos9120467
![]() |
[120] | Aguilera Á, de Diego-Castilla G, Osuna S, et al. 2018. Microbial ecology in the atmosphere: the last extreme environment. In: Extremophilic microbes and metabolites diversity bioprospecting and biotechnological applications. IntechOpen, London. doi: http://dx.doi.org/10.5772/intechopen.81650 |
[121] |
Jaenicke R, Matthias-Maser S, Gruber S (2007) Omnipresence of biological material in the atmosphere. Environ Chem 4: 217-220. doi: 10.1071/EN07021
![]() |
[122] | Laaka-Lindberg S, Korpelainen H, Pohjamo M (2003) Dispersal of asexual propagules in bryophytes. J Hattori Bot Lab 94: 319-330. |
[123] | Fröhlich-Nowoisky J, Pickersgill DA, Després VR, et al. (2009) High diversity of fungi in air particulate matter. Proc Natl Acad Sci 106: no. 31. |
[124] |
Bowers RM, Clements N, Emerson JB, et al. (2013) Seasonal variability in bacterial and fungal diversity of the near-surface atmosphere. Environ Sci Technol 47: 12097-12106. doi: 10.1021/es402970s
![]() |
[125] | Narlikar JV, Wickramasinghe NC, Wainwright M, et al. (2003) Detection of microorganisms at high altitudes. Curr Sci 85: 23-29. |
[126] |
Bryan NC, Christner BC, Guzik TG, et al. (2019) Abundance and survival of microbial aerosols in the troposphere and stratosphere. ISME J 13: 2789-2799. doi: 10.1038/s41396-019-0474-0
![]() |
[127] |
Chen X, Ran P, Ho K, et al. (2012) Concentrations and size distributions of airborne microorganisms in Guangzhou during summer. Aerosol Air Qual Res 12: 1336-1344. doi: 10.4209/aaqr.2012.03.0066
![]() |
[128] | Pulschen AA, de Araujo GG, de Carvalho ACSR, et al. (2018) Survival of extremophilic yeasts in the stratospheric environment during balloon flights and in laboratory simulations. Appl Environ Microb 84: e01942-18. |
[129] |
Smith DJ, Timonen HJ, Jaffe DA, et al. (2013) Intercontinental dispersal of bacteria and archaea by transpacific winds. Appl Environ Microb 79: 1134-1139. doi: 10.1128/AEM.03029-12
![]() |
[130] | Smith DJ, Ravichandar JD, Jain S, et al. (2018) Airborne bacteria in the Earth's lower stratosphere resemble taxa detected in the troposphere: Results from a new NASA Aircraft Bioaerosol Collector (ABC). Front Microbiol 9: article 1752. |
[131] | Smith DJ, Griffin DW, McPeters RD, et al. 2011. Microbial survival in the stratosphere and implications for global dispersal. Aerobiologia 27: 319-332. |
[132] |
Nicholson WL, Munakata N, Horneck G, et al. (2000) Resistance of Bacillus endospores to extreme terrestrial and extraterrestrial environments. Microbiol Mol Biol Rev 64: 548-572. doi: 10.1128/MMBR.64.3.548-572.2000
![]() |
[133] | Huwe B, Fiedler A, Moritz S, et al. 2019. Mosses in low orbit: Implications for the limits of life and the habitability of Mars. Astrobiology 19: 221-232. |
[134] | Trest MT, Will-Wolf S, Keuler R, et al. (2015) Potential impacts of UV exposure on lichen communities: A pilot study of Nothofagus dombeyei trunks in southernmost Chile. Ecosyst Heath Sus 1: article 14. |
[135] | Wainwright M, Alharbi S, Wickramasinghe NC (2006) How do microorganisms reach the stratosphere? Int J Astrobiology 5: 13-15. |
[136] |
Langematz A (2019) Stratospheric ozone: down and up through the Anthropocene. ChemTexts 5: 8. doi: 10.1007/s40828-019-0082-7
![]() |
[137] |
Studlar SM, Eddy C, Spencer J (2007) Survival of four mosses from West Virginia after two hours in the stratosphere. Evansia 24: 17-21. doi: 10.1639/0747-9859-24.1.17
![]() |
[138] |
Horneck G, Klaus DM, Mancinelli RL (2010) Space microbiology. Microbiol Mol Biol Rev 74: 121-156. doi: 10.1128/MMBR.00016-09
![]() |
[139] |
Wainwright M, Wickramasinghe NC, Narlikar JV, et al. (2003) Microorganisms cultured from stratospheric air samples obtained at 41 km. Fems Microbiol Lett 218: 161-165. doi: 10.1111/j.1574-6968.2003.tb11513.x
![]() |
[140] |
Imshenetsky AA, Lysenko SV, Lach SP (1979) Microorganisms of the upper layer of the atmosphere and the protective role of their cell pigments. Life Sci Space R 17: 105-110. doi: 10.1016/B978-0-08-023416-8.50017-9
![]() |
[141] | Sancho LG, De la Torre R, Horneck G, et al. 2007. Lichens survive in space: results from the 2005 LICHENS experiment. Astrobiology 7: 143-454. |
[142] |
Mora M, Perras A, Alekhova TA, et al. (2016) Resilient microorganisms in 12-year old dust samples of the International Space Station - survival of the adaptation specialists. Microbiome 4: 65. doi: 10.1186/s40168-016-0217-7
![]() |
[143] |
Grebennikova TV, Syroeshkin AV, Shubralova EV, et al. (2018) The DNA of bacteria of the world ocean and the Earth in cosmic dust at the International Space Station. Sci World J 2018: 7360147. doi: 10.1155/2018/7360147
![]() |
[144] |
Farman JC, Gardiner BG, Shanklin JD (1985) Large losses of total ozone in Antarctica reveal seasonal C10x/NOx interaction. Nature 315: 307-210. doi: 10.1038/315207a0
![]() |
[145] |
Bhartia PK, McPeters RD (2018) The discovery of the Antarctic Ozone Hole. Cr Geosci 350: 335-340. doi: 10.1016/j.crte.2018.04.006
![]() |
[146] |
Solomon S (2019) The discovery of the Antarctic ozone hole. Nature 575: 46-47. doi: 10.1038/d41586-019-02837-5
![]() |
[147] | Ritchie H, Roser M (2020) Ozone layer. https://ourworldindata.org/ozone-layer |
[148] | Zhang Y, Li J, Zhou L (2017) The relationship between polar vortex and ozone depletion in the Antarctic stratosphere during the period 1979-2016. Adv Meteorol 2017: 3078079. |
[149] |
Onofri S, Barreca D, Selbmann L, et al. (2008) Resistance of Antarctic black fungi and cryptoendolithic communities to simulated space and Martian conditions. Stud Mycol 61: 99-109. doi: 10.3114/sim.2008.61.10
![]() |
[150] |
Monsalves MT, Ollivet-Besson GP, Amenabar MJ, et al. (2020) Isolation of a psychrotolerant and UV-C-resistant bacterium from Elephant Island, Antarctica with a highly thermoactive and thermostable catalase. Microorganisms 8: 95. doi: 10.3390/microorganisms8010095
![]() |
[151] | Romanovskaya VA, Tashirev B, Shilin NA, et al (2011) Resistance of Antarctic microorganisms to UV radiation. Mikrobiolohichnyi Zhurnal 73: 3-8. |
[152] |
Waterman MJ, Nugraha AS, Hendra R, et al. (2017) Antarctic moss bioflavonoids show high antioxidant and ultraviolet-screening activity. J Nat Prod 80: 2224-2231. doi: 10.1021/acs.jnatprod.7b00085
![]() |
[153] | Reis-Mansur MCPP, Cardoso-Rurr JS, Silva JVMA, et al. (2019) Carotenoids from UV-resistant Antarctic Microbacterium sp. LEMMJ01. Sci R 9: 9554. |
[154] |
Yang Y, Itahashi S, Yokobori S, et al. (2008) UV-resistant bacteria isolated from upper troposphere and lower stratosphere. Biol Sci Space 22: 18-25. doi: 10.2187/bss.22.18
![]() |
[155] |
Ehling-Schulz M, Scherer S. (1999) UV protection in cyanobacteria. Eur J Phycol 34: 329-338. doi: 10.1080/09670269910001736392
![]() |
[156] | Taleb NM (2007) The Black Swan. Random House Publishing, New York |
[157] | Ferrari, M. 2009. Anticipating the climate black swan. https://energycentral.com/c/pip/anticipating-climate-black-swan |
[158] | Witze A (2020) Rare ozone hole opens over the Arctic - and it's big. Nature 580: 18-19. |
1. | Hong Li, Zhantao Li, Chaohong Pan, Jie Song, Mingji Zhang, Cubic-like Features of I–V Relations via Classical Poisson–Nernst–Planck Systems Under Relaxed Electroneutrality Boundary Conditions, 2024, 13, 2075-1680, 790, 10.3390/axioms13110790 | |
2. | Xiangshuo Liu, Jie Song, Lijun Zhang, Mingji Zhang, Roles Played by Critical Potentials in the Study of Poisson–Nernst–Planck Models with Steric Effects Under Relaxed Neutral Boundary Conditions, 2025, 14, 2075-1680, 69, 10.3390/axioms14010069 |
Parameter | Value | Parameter | Value |
\Lambda | 0.4 | \omega | 0.4 |
\beta | 0.1 | \eta | 0.2 |
\delta | 0.2 | \varepsilon | 0.5 |
b | 0.5 | {m_0} | 0.2 |
k | 0.5 | \rho | 0.2 |
\xi | 0.3 | \mu | 0.05 |
\gamma | 0.5 |
Parameter | Value | Parameter | Value |
\Lambda | 1.2 | \omega | 0.4 |
\beta | 0.6 | \eta | 0.2 |
\delta | 0.5 | \varepsilon | 0.6 |
b | 1 | {m_0} | 0.6 |
k | 1 | \rho | 0.2 |
\xi | 0.3 | \mu | 0.3 |
\gamma | 0.5 |
Parameter | Value | Parameter | Value |
\Lambda | 0.4 | \omega | 0.4 |
\beta | 0.1 | \eta | 0.2 |
\delta | 0.2 | \varepsilon | 0.5 |
b | 0.5 | {m_0} | 0.2 |
k | 0.5 | \rho | 0.2 |
\xi | 0.3 | \mu | 0.05 |
\gamma | 0.5 |
Parameter | Value | Parameter | Value |
\Lambda | 1.2 | \omega | 0.4 |
\beta | 0.6 | \eta | 0.2 |
\delta | 0.5 | \varepsilon | 0.6 |
b | 1 | {m_0} | 0.6 |
k | 1 | \rho | 0.2 |
\xi | 0.3 | \mu | 0.3 |
\gamma | 0.5 |