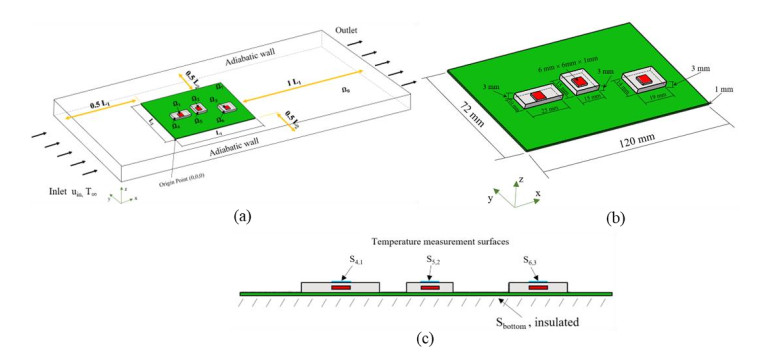
Citation: Alma Sobrino-Figueroa, Sergio H. Álvarez Hernandez, Carlos Álvarez Silva C. Evaluation of the freshwater copepod Acanthocyclops americanus (Marsh, 1983) (Cyclopidae) response to Cd, Cr, Cu, Hg, Mn, Ni and Pb[J]. AIMS Environmental Science, 2020, 7(6): 449-463. doi: 10.3934/environsci.2020029
[1] | Bauyrzhan Derbissaly, Makhmud Sadybekov . Inverse source problem for multi-term time-fractional diffusion equation with nonlocal boundary conditions. AIMS Mathematics, 2024, 9(4): 9969-9988. doi: 10.3934/math.2024488 |
[2] | Varadharaj Dinakar, Natesan Barani Balan, Krishnan Balachandran . Identification of Source Terms in a Coupled Age-structured Population Model with Discontinuous Diffusion Coefficients. AIMS Mathematics, 2017, 2(1): 81-95. doi: 10.3934/Math.2017.1.81 |
[3] | Zui-Cha Deng, Liu Yang . Unicity of solution for a semi-infinite inverse heat source problem. AIMS Mathematics, 2022, 7(4): 7026-7039. doi: 10.3934/math.2022391 |
[4] | Dun-Gang Li, Fan Yang, Ping Fan, Xiao-Xiao Li, Can-Yun Huang . Landweber iterative regularization method for reconstructing the unknown source of the modified Helmholtz equation. AIMS Mathematics, 2021, 6(9): 10327-10342. doi: 10.3934/math.2021598 |
[5] | Xuemin Xue, Xiangtuan Xiong, Yuanxiang Zhang . Two fractional regularization methods for identifying the radiogenic source of the Helium production-diffusion equation. AIMS Mathematics, 2021, 6(10): 11425-11448. doi: 10.3934/math.2021662 |
[6] | Zui-Cha Deng, Fan-Li Liu, Liu Yang . Numerical simulations for initial value inversion problem in a two-dimensional degenerate parabolic equation. AIMS Mathematics, 2021, 6(4): 3080-3104. doi: 10.3934/math.2021187 |
[7] | M. J. Huntul . Inverse source problems for multi-parameter space-time fractional differential equations with bi-fractional Laplacian operators. AIMS Mathematics, 2024, 9(11): 32734-32756. doi: 10.3934/math.20241566 |
[8] | Yu Xu, Youjun Deng, Dong Wei . Numerical solution of forward and inverse problems of heat conduction in multi-layered media. AIMS Mathematics, 2025, 10(3): 6144-6167. doi: 10.3934/math.2025280 |
[9] | Özlem Kaytmaz . The problem of determining source term in a kinetic equation in an unbounded domain. AIMS Mathematics, 2024, 9(4): 9184-9194. doi: 10.3934/math.2024447 |
[10] | Shuang-Shuang Zhou, Saima Rashid, Asia Rauf, Khadija Tul Kubra, Abdullah M. Alsharif . Initial boundary value problems for a multi-term time fractional diffusion equation with generalized fractional derivatives in time. AIMS Mathematics, 2021, 6(11): 12114-12132. doi: 10.3934/math.2021703 |
The realm of heat source estimation concerning encapsulated chips affixed to printed circuit boards (PCBs) stands as a vital field of inquiry within the electronics industry. As the dimensions of electronic devices consistently shrink while integration escalates, comprehending the intricacies of heat generation and dissipation mechanisms within these systems is of paramount importance. The pursuit of accurately estimating heat generation on encapsulated chips holds significance for a multitude of reasons, encompassing the enhancement of thermal management tactics, mitigation of thermal failure risks, and the optimization of overall system performance.
Multiple factors come into play when considering the influence on heat source in encapsulated chips. Among these factors are the electrical and thermal characteristics of both the chip and the encapsulating materials, in addition to the design and arrangement of the PCB. Within the realm of research, a diversity of methodologies has been employed to estimate heat source in encapsulated chips. These approaches span numerical simulation techniques, like the analysis of inverse problems, and experimental strategies, such as the utilization of infrared thermal imaging and thermocouple measurements.
Inverse problem techniques have found application within electronics packaging systems for the purpose of estimating chip heat sources [1,2,3,4,5]. Leveraging this estimation approach facilitates precise quantification of heat source magnitudes, subsequently enhancing the broader realm of electronic thermal management.
As an illustration, Krane et al. [5] employed a least-squares algorithm to ascertain both the magnitude and positioning of heat sources within an electronics system. Their investigation revealed that the fine-grid method exhibits computational expediency surpassing that of the coarse-grid alternative, all while maintaining a negligible compromise on overall accuracy. It's important to note that the Inverse Heat Conduction Problem (IHCP) studies cited earlier did not incorporate air velocity as a factor in their mathematical models. Consequently, a more lifelike scenario, encompassing the coupled heat conduction and convection conditions encountered by chips mounted on a PCB, remains an unexplored avenue.
The CGM [6] stands as an iterative algorithm rooted in gradients and self-regularization. What sets it apart from alternative techniques is its lack of necessity for a priori insights into the functional structure of the unknown variables, rendering it adept at producing dependable estimates for these enigmatic quantities. The robust capabilities of the CGM make it especially fitting for tackling the intricacies of function estimation within three-dimensional inverse problems, particularly those unfolding in irregular domains. Notably, despite its prowess, such problems have remained relatively less explored within the existing literature [7,8,9,10].
Huang et al. [7] delved into a three-dimensional inverse problem aimed at estimating the heat flux applied to the surface of a drilling tool during the drilling process. This estimation was driven by simulated time-dependent temperature distributions captured by sensors embedded onto the drilling surfaces. The outcomes of this investigation underscore the prowess of the gradient method in furnishing dependable estimates, all achieved without the need for foreknowledge regarding the functional nature of the enigmatic functions in play. Notably, the analysis revealed an initial increase in applied heat flux over time owing to escalating torque, followed by a subsequent decline until the point of workpiece penetration.
In another study, Huang and Lee [8] undertook an IHCP using the CGM. Their focus was on the simultaneous estimation of six hitherto unknown internal surface heat fluxes within a square internal combustion chamber. The estimation process hinged on simulated surface temperature measurements. The outcomes of their efforts showcased the feasibility of accurate estimations when dealing with error-free temperature readings. Notably, the maximum relative error for the estimated heat fluxes remained below 3.3%. Furthermore, the study concludes that even in the presence of substantial measurement errors, dependable estimates of internal surface heat fluxes are attainable.
Huang and He [9] undertook the examination of an inverse heat transfer problem, aiming to deduce the elusive spatially varying surface heat flux using the conjugate gradient method (CGM). The veracity of the inverse solutions was substantiated through an array of numerical simulations, encompassing diverse inlet air velocities and plate thicknesses. In addition, an assessment of the impact of measurement errors on the inverse solutions was undertaken. The authors arrived at the conclusion that due to the ill-posed nature intrinsic to the inverse problem, the precision of the estimated heat flux diminishes as the plate thickness increases.
A recent study by Huang and Zhong [10] has undertaken an exploration of an inverse problem, harnessing the potential of the CGM, with the objective of deducing the heat source for a solitary encapsulated chip. This endeavor relied on simulated temperature measurements. Notably, their investigation revolved around the challenging context of conjugated heat transfer conditions. Their study holds the distinction of being the inaugural instance of such an investigation within existing literature. Through a series of numerical experiments, their findings illuminated the potency of the CGM as a robust optimization tool that is well-suited for addressing the intricacies of the three-dimensional inverse heat transfer problem.
In practical scenarios, encapsulated chips are consistently affixed to printed circuit boards (PCBs) to serve functional purposes. Consequently, the real-world context demands the simultaneous estimation of heat generation across multiple chips. Building upon the groundwork laid by Huang and Zhong [10], we aspire to broaden the scope of inquiry. Specifically, our focus lies in extending the investigations to encompass the concurrent estimation of numerous spatially dependent heat sources attributed to chips mounted on a PCB.
The ongoing investigation centers around addressing the 3-D steady-state inverse heat transfer problem using the CGM algorithm. This approach involves a transformative process that divides the problem into two distinct sub-problems, specifically the sensitivity and adjoint problems. The validity of using the CGM in this inverse problem will be examined by estimating multiple heat sources with various inlet velocities and simulated error temperature measurements.
Three unknown spatial-dependent volumetric heat generations of encapsulated chips mounted on a PCB are estimated in this study based on the technique of inverse problems. Ω is a domain of computational and it consists of eight subdomains Ωi, i = 1 to 8. The solid domains include Ω1 to Ω7, where Ω1, Ω2 and Ω3 represent three chips, Ω4, Ω5 and Ω6 represent the encapsulation materials for three chips, and Ω7 is a PCB, while the air domain is indicated as Ω8. The domain of computation considered in this work is given in Figure 1(a), while Figure 1(b) and 1(c) indicate the 3-D and side view of the physical geometry considered in the present study, respectively. Since conjugate heat transfer is considered in the present inverse model, thus it is classified as an Inverse Heat Conduction-Convection Conjugated Problem (IHCCCP).
In solid domains, there exist three volumetric heat generations ψ(Ω1), ψ(Ω2) and ψ(Ω3) for three chips, and an insulation condition is applied on the bottom surface of the PCB, Sbottom. An incompressible inlet air enters the computational domain Ω with velocity uin and ambient temperature T∞, and a perfect thermal contact condition applied to all interfacial surfaces of Ω.
The partial differential equations for the considered direct problem can be given below:
(Ⅰ) Solid domains (Ωi, i = 1 to 7 and s = 1 to 3):
ki∇2T(Ωi)+ψ(Ωs)δ(i−s)=0, | (1) |
where ki denotes the thermal conductivity of the solid regions, and ψ(Ωs) is the heat source in Ωs. Here δ(∙) is the Dirac delta function, and s = 1 to 3 indicates the number of source positions.
An insulation condition is plied to the bottom wall of the PCB.
−k7∂T(Ω7)∂n=0;onSbottom. | (2) |
Therefore, seven equations are in solid domains Ωi.
(Ⅱ) Air domain (Ω8):
In Ω8, the differential equations for continuity, momentum and energy equations can be obtained as:
∇⋅U=0, | (3) |
ρ(U⋅∇U)=−∇P+μ∇2U+13μ∇(∇⋅U)+ρg, | (4) |
k8∇2T(Ω8)=ρCp[U⋅∇T(Ω8)], | (5) |
where U = {u, v, w} with Uin = uin and T8,in = T∞ and k8 represents the thermal conductivity of air.
(Ⅲ) Interface conditions (SΩi∩Ωj,i≠j):
A perfect thermal contact condition is applied to all the interface surfaces of Ω :
−ki∂T(Ωi)∂n=−kj∂T(Ωj)∂n and T(Ωi) = T(Ωj),onSΩi∩Ωj,i≠j. | (6) |
The commercial package CFD-ACE+ (by ESI-CFD Inc., 2020). is utilized to compute the solutions of the above direct problem.
For the inverse problem examined in this work, volumetric heat sources ψ(Ω1), ψ(Ω2), and ψ(Ω3) are considered unknown and are functions of space. In addition, temperature readings for S4,1, S5,2, and S6,3 are considered available. These surfaces are the perpendicular projection areas of chips Ω1, Ω2, and Ω3 on the top surfaces of S4, S5 and S6, respectively, and they are illustrated in Figure 1(c).
Let the simulated temperature measurements on Ss+3,s, s = 1 to 3, be denoted by Ym(Ss+3,s), m = 1 to M. The procedure for this IHCCCP is as follows: by utilizing the abovementioned temperature measurements Ym(Ss+3,s), predict the three unknown volumetric heat sources ψ(Ω1), ψ(Ω2) and ψ(Ω3).
The following cost function is thus defined and minimized for obtaining the estimation of three sources:
J[ψ(Ωs)]=3∑s=1M∑m=1[Tm(Ss+3,s)−Ym(Ss+3,s)]2=3∑s=1∫Ss+3,s[T(Ss+3,s)−Y(Ss+3,s)]2δ(x−xm)δ(y−ym)dSs+3,s, | (7) |
where Tm(Ss+3,s) are the computed temperatures on Ss+3,s with estimated sources for the exact sources.
The following CGM [11] is used to minimize the cost function J[ψ(Ωs)] and then to estimate unknown volumetric heat generations ψ(Ωs):
ψn+1(Ωs)=ψn(Ωs)−βsnPn(Ωs)fors=1to3andn=0,1,2,…, | (8) |
Pn(Ωs)=J′n(Ωs)+γsnPn−1(Ωs), | (9) |
γsn=∫Ωs(J′n)2dΩs∫Ωs(J′n−1)2dΩswithγs0=0, | (10) |
where βsn and J′n(Ωs) can be obtained based on the solutions of the following sensitivity and adjoint problems.
Given that the predicament at hand entails three enigmatic volumetric heat sources, denoted as ψ(Ωs), where s ranges from 1 to 3, the derivation of the sensitivity problem necessitates a sequential approach. This involves perturbing a solitary unknown heat source at a time, enabling the isolation and analysis of each distinct unknown in the context of the larger conundrum.
The sensitivity problems are derived from the foundational direct problem as delineated by Eq (1), employing the subsequent approach. For s = 1, it is assumed that when ψ(Ω1) undergoes a variation Δψ(Ω1), while ψ(Ω2) and ψ(Ω3) remain unchanged, and T(Ωi) is perturbed by ΔT1(Ωi). Then, replacing ψ(Ω1) by ψ(Ω1)+Δψ(Ω1) and T(Ωi) by T(Ω)+ ΔT1(Ωi) in the direct problem, subtracting from the resulting expressions in the direct problem and neglecting the second-order terms, the following sensitivity problem for the first sensitivity function ΔT1(Ωi) is obtained as:
(Ⅰ) Solid domains (Ωi, i = 1 to 7):
ki∇2ΔT1(Ωi)+Δψ(Ω1)δ(i−1)=0, | (11) |
where an insulation condition is applied to the base surface Sbottom:
−k7∂ΔT1(Ω7)∂n=0;onSbottom. | (12) |
(Ⅱ) Air domain (Ω8):
All the thermal parameters considered in this work are assumed to be constants, i.e. it is a linear problem; therefore, Eqs (3) and (4) need to be solved only once in the direct problem at the first iteration, and these solutions can be used for the remaining iterations. In Ω8, the energy equation for the sensitivity function can be derived as:
k8∇2ΔT1(Ω8)=ρCp[U⋅∇ΔT1(Ω8)]. | (13) |
The inlet conditions for the first sensitivity function can be obtained as Uin = uin and ΔTi,in = 0.
(Ⅲ) Interface conditions (SΩi∩Ωj,i≠j):
The interface conditions on the interface surfaces are given below:
−ki∂ΔT1(Ωi)∂n=−kj∂ΔT1(Ωj)∂n and ΔT1(Ωi) = ΔT1(Ωj),onSΩi∩Ωj,i≠j. | (14) |
Similarly, for s = 2 and 3, two additional groups of sensitivity equations for sensitivity functions ΔT2(Ωi) and ΔT3(Ωi) are derived below in the solid domain (Ωi, i = 1 to 7):
ki∇2ΔT2(Ωi)+Δψ(Ω2)δ(i−2)=0. | (15) |
ki∇2ΔT3(Ωi)+Δψ(Ω3)δ(i−3)=0. | (16) |
The sensitivity functions in the air domain are the same as those given in Eq (13). In addition, the boundary condition (14) is also applied to all the interface surfaces of Eqs (15) and (16). To obtain the expression for the calculation of step size βsn, an alternative form of cost function J(ψsn+1) at iteration n+1 must be derived.
The computed temperatures Tm(Ss+3,s) that occur in Eq (7) should be functions of ψ1, ψ2, and ψ3. However, because the measured temperatures Ym(S4,1), Ym (S5,2), and Ym (S6,3) were affected mostly by ψ1, ψ2, and ψ3, respectively, the cost function can be approximately expressed as:
J[ψ(Ωs)n+1]=3∑s=1M∑m=1[Tm(Ss+3,s;ψsn−βsPsn)−Ym]2. | (17) |
Equation (18) is subjected to linearization through a Taylor series expansion. As a result of this process, we ultimately arrive at:
J[ψ(Ωs)n+1]=3∑s=1M∑m=1[Tm(ψsn)−βsΔTs,m(Psn)−Ym]2. | (18) |
βsn can then be obtained by minimizing Eq (18) with respect to βsn; thereafter, the following expression can be obtained:
βsn=M∑m=1[Tm−Ym]ΔTs,mM∑m=1(ΔTs,m)2 ; s = 1 to 3. | (19) |
The following procedure is performed to derive the adjoint problem: For s = 1, the adjoint functions λ1(Ωi), i = 1 to 7, and λ1(Ω8) are multiplied to Eqs (1) and (5), respectively, and are integrated over the Ωi domains. The expressions are then added to the right-hand side of Eq (7) to obtain a new cost function J[ψ(Ωs)]:
J[ψ(Ωs)] = 3∑s=1∫Ss+3,s[T(Ss+3,s)−Y(Ss+3,s)]2δ(x−xm)δ(y−ym)dSs+3,s+7∑i=1∫Ωiλ1(Ωi)[ki∇2T(Ωi)+ψ(Ω1)δ(Ωi−Ω1)]dΩi+∫Ω8λ1(Ω8)[k8∇2T(Ω8)−ρCp[U⋅∇T(Ω8)−μΦ]]dΩ8. | (20) |
The variation of Eq (20) ΔJ1 can be derived by perturbing ψ(Ω1) by ψ(Ω1)+Δψ(Ω1) and T(Ωi)+ΔT1(Ωi) in Eq (21), while ψ(Ω2) and ψ(Ω3) remain unchanged, subtracting from Eq (20) and neglecting the higher-order terms. The expression given below is obtained
ΔJ1[ψ(Ωs)] = 3∑s=1∫Ss+3,s2[T(Ss+3,s)−Y(Ss+3,s)]ΔT1δ(x−xm)δ(y−ym)dSs+3,s+7∑i=1∫Ωiλ1(Ωi)[ki∇2ΔT1(Ωi)+Δψ(Ω1)δ(Ωi−Ω1)]dΩi+∫Ω8λ1(Ω8)[k4∇2ΔT1(Ω8)−ρCp[U⋅∇ΔT1(Ω8)]]dΩ8. | (21) |
In Eq (21), the domain integral terms are reformulated based on Green's second identity; the boundary and interface conditions of the sensitivity problems are used, and then ΔJ1 is allowed to approach zero. The vanishing of the integrands containing ΔT1 leads to the following adjoint problem λ1(Ωi):
(Ⅰ) Solid domain (Ωi, i = 1 to 7):
ki∇2λ1(Ωi)=0,i=1to7. | (22) |
−k7∂λ1(Ω7)∂n=0 ,onSbottom. | (23) |
(Ⅱ) Air domain (Ω8):
k8∇2λ1(Ω8) = ρCpU⋅∇λ1(Ω8), | (24) |
The inlet conditions are Uin = uin and λ1,in(Ω8) = 0, and U and P are computed from Eqs (3) and (4).
(Ⅲ) Interface conditions (SΩi∩Ωj,i≠j):
The perfect thermal contact condition is used to all the interface surfaces of Ω, except for S4,1, S5,2 and S6,3.
−ki∂λ1(Ωi)∂n=−kj∂λ1(Ωj)∂n and λ1(Ωi) = λ1(Ωj),onSΩi∩Ωj,i≠j. | (25) |
The interface conditions on S4,1, S5,2 and S6,3 are given as:
{−ks+3∂λ1(Ωs+3)∂n = −k8∂λ1(Ω8)∂n−2[T(Ss+3,s)−Y(Ss+3,s)]δ(x−xm)δ(y−ym),λ1(Ωs+3)=λ1(Ω8), | (26) |
on Ss + 3,s,s=1 to 3. Clearly, extra heat source terms exist on S41, S52 and S63 in the interface surfaces of the adjoint problem.
Finally, the following integral term remains:
ΔJ1=∫Ω1λ1(Ω1)Δψ(Ω1)dΩ1. | (27) |
The functional increment can be defined as [11]:
ΔJ1=∫Ω1J′[ψ(Ω1)]Δψ(Ω1)dΩ1. | (28) |
The comparison of Eqs (27) and (28) yields the gradient of the cost function:
J′[ψ(Ω1)]=λ1(Ω1). | (29) |
Similarly, for s = 2 and 3, two sets of adjoint equations for adjoint functions λ2(Ωi) and λ3(Ωi) can be derived, and it is found that they are all identical to λ1(Ωi); therefore, the adjoint equations need to be solved only once. Finally, the following two gradient equations for ψ(Ω2) and ψ(Ω3) are obtained:
J′[ψ(Ω2)]=λ1(Ω2), | (30) |
J′[ψ(Ω3)]=λ1(Ω3). | (31) |
The residual between the computed and simulated measured temperatures can be approximated by:
[Tm(Ss+3,s)−Ym(Ss+3,s)]≈σ, | (32) |
here σ represents the standard deviation of the temperature readings. Substituting the above temperature residual expression into Eq (7), the following stopping criterion value ε, based on the discrepancy principle [11], can be obtained
ε=3Mσ2 | (33) |
A 3-D inverse problem using the technique of function estimation is investigated in the present study to determine the distribution of unknown strength of heat generations ψ(Ω1), ψ(Ω2), and ψ(Ω3) of three chips mounted on a PCB with the CGM.
Three silicon chips with k = 124 W/(m-K) and lengths Lx = Ly = 6 mm and Lz = 1 mm were filled with CEL-9200, k = 0.97 W/(m-K) [12], and they were mounted on a PCB with dimensions of 120 mm ×72 mm ×1 mm and k = 0.2 W/(m-K). The sizes (in mm) of the three encapsulated chips in the x, y and z directions are 25×10×3, 15×15×3 and 19×14×3, respectively. The computational domain of the present work is given in Figure 1(a), where uin denotes the inlet air velocity and T∞ = 300 K represents the ambient air temperature. The chips are very thin in this work, therefore it is reasonable to assume that ψ(Ωs) = ψ(x, y) for the numerical experiments.
The center point temperature Tcenter of chip 2 with ψ(Ωs) = 31250 W/m3, uin = 2 m/s and T∞ = 300 K was calculated using the four grid numbers of 162,468,239,162,416,832 and 529,280 as 326.7 K, 341.3 K, 342.5 K and 342.7 K, respectively. It is clear that the error of Tcenter in chip 2 between the grid numbers 416,832 and 529,280 was only 0.058%; therefore, grid numbers of 416,832 were considered for the computations.
The exact temperature readings, Yexact, with random errors, ωσ, were used to simulate the measurement data Y:
Y=Yexact +ωσ | (34) |
here, the symbol ω represents a randomly generated number, facilitated through the IMSL subroutine DRNNOR (by Perforce Software Inc., 2021), encompassing a range from -2.576 to 2.576, corresponding to a 99% confidence bound.
An advantage of using the CGM to compute an inverse problem is the ability to arbitrarily choose the values of initial guesses; therefore, the initial guess for the heat sources is chosen as a small number, ψ(Ωs)0 = 1 W/m3, for all numerical experiments in the present study.
The unknown heat generations ψ(Ω1), ψ(Ω2) and ψ(Ω3) are considered as:
ψ(Ω1)=ψ(x,y)=22222 W/m3, {0⩽x⩽Lx,0⩽y⩽Ly, | (35) |
ψ(Ω2)=ψ(x,y)=[5+(3×xLx+3×yLx)]×103 W/m3, {0⩽x⩽Lx,0⩽y⩽Ly, | (36) |
ψ(Ω3)=ψ(x,y)=[60−(30×xLx−30yLx)]×103 W/m3, {0⩽x⩽Lx,0⩽y⩽Ly, | (37) |
where ψ(Ω1) is assumed to be a constant heat source, and the heat source distributions for ψ(Ω2) and ψ(Ω3) are assumed to be two inclined planes.
The 3-D plots for the exact ψ(Ω2) and ψ(Ω3) are given in Figure 2(a) and 2(b), respectively, and contour plots for ψ(Ω1), ψ(Ω2) and ψ(Ω3) are given in Figure 3(a)–3(c), respectively. The grid sizes of the chip are taken as 20 and 20 in the x and y directions, respectively; thus a total of M = 400 unknown discretized values of heat source are to be estimated at each chip, and 400 temperature readings are needed on Ss+3,s surfaces.
First, inverse estimates are executed using σ = 0.0, uin = 2 m/s, T∞ = 300 K and ψ(Ω1)0 = ψ(Ω2)0 = ψ(Ω3)0 = 1 W/m3. Contour plots of the simulated exact measurement temperatures Y(Ss+3,s), s = 1 to 3, with σ = 0.0 are presented in Figure 4(a)–4(c), respectively. Figure 5(a) and 5(b) illustrate the temperature distributions of air and encapsulated chips on the measurement and bottom surfaces, respectively. Since the magnitude of heat generation varies for chips, the resultant temperatures of the air and encapsulated chips also vary. In addition, because of the motion of air, the air temperatures will be higher in the downstream regions of chips than at the inlet, and the temperatures of the encapsulated chips are higher on the bottom surface than on the measurement surface.
Figure 6(a) illustrates the progression of convergence concerning the number of iterations. It is readily apparent that the cost function experiences a decrease, ultimately converging to 0.0003 after 50 iterations. This trend also underscores that the estimated heat sources exhibit swift convergence towards the precise heat generations, facilitated by the rapid rate of convergence observed over the initial iterations. Subsequent to this swift convergence, the ensuing iterations are employed to meticulously refine and optimize the estimated heat sources within a localized context.
It is observed that the values for constant heat generation ψ(Ω1) can be estimated accurately in only 3 iterations. This result implies that if all the heat generations of chips are constants or nearly constants, which is the case for practical applications, the iteration numbers required to obtain converged estimates should be very small, and it makes the algorithm very efficient in practice.
The estimated 3-D ψ(Ω2) and ψ(Ω3) are given in Figure 2(a) and Figure 2(b), respectively, the contour plots of ψ(Ω1), ψ(Ω2) and ψ(Ω3) are plotted in Figure 3(a)–3(c), respectively, and the corresponding estimated temperatures T(Ss+3,s), s = 1 to 3, are presented in Figure 4(a)–4(c), respectively. The figures indicated that using σ = 0.0, the exact and estimated values are nearly the same for both the heat generations and temperatures.
The computed errors for the estimated temperatures ERR1, s and estimated heat generations ERR2, s are summarized in Table 1 and are defined below.
ERR1,s%=400∑m=1|Tm(Ss+3,s)−Ym(Ss+3,s)Ym(Ss+3,s)|÷400×100%, s=1to3. | (38a) |
ERR2, s%=400∑m=1|ψestimated,m(x,y)−ψexact,m(x,y)ψexact,m(x,y)|÷400×100%,s=1to3. | (38b) |
The influence of temperature readings with errors on heat source estimations ψ(Ω1), ψ(Ω2) and ψ(Ω3) is one of the major concerns for inverse problems and is examined now. The error of the temperatures readings is first considered as σ = 0.01 and then increased to σ = 0.05. The estimation of three heat sources can be obtained after 9 and 8 iterations for σ = 0.01 and 0.05, respectively, and the estimated ψ(Ω1), ψ(Ω2) and ψ(Ω3) are shown in Figure 7(a)–7(c), respectively, for σ = 0.01 and 0.05. The errors ERR1, s and ERR2, s are also summarized in Table 1. These results indicate that the heat generation estimates remain reliable when error readings are considered.
![]() |
![]() |
u∞= 2 m/s | u∞= 4 m/s | ||||
σ=0 | σ=0.01 | σ=0.05 | σ=0 | σ=0.01 | σ=0.05 | ||
Iteration numbers | 50 | 9 | 8 | 50 | 8 | 8 | |
J | 0.0003 | 0.0764 | 0.2129 | 0.0002 | 0.0219 | 0.2665 | |
Chip 1 | ERR1, 1 % | 0.00002 | 0.00005 | 0.00006 | 0.00002 | 0.00005 | 0.00005 |
ERR2, 1 % | 0.1387 | 0.1477 | 0.1582 | 0.1270 | 0.1618 | 0.1769 | |
Chip 2 | ERR1, 2 % | 0.00002 | 0.0020 | 0.0026 | 0.00002 | 0.0024 | 0.0029 |
ERR2, 2 % | 1.3849 | 2.6339 | 2.9935 | 1.1964 | 1.8792 | 3.5412 | |
Chip 3 | ERR1, 3 % | 0.0002 | 0.0054 | 0.0199 | 0.0001 | 0.0067 | 0.0235 |
ERR2, 3 % | 1.9696 | 2.5668 | 2.9244 | 1.6506 | 2.0141 | 2.2662 |
To illustrate the estimated heat generations more clearly, the exact and estimated ψ(Ω1), ψ(Ω2), and ψ(Ω3), at the midpoint of the x position of each chip with y positions varied, are plotted in Figure 8(a)–8(c), respectively. If the measurement errors are increased, the estimates may become poor, but they will be reliable, although the unknown heat source can be replicated accurately for errorless measurements.
In order to validate the proposed algorithm and investigate the impact of the inlet air velocity on the estimated heat generation, it was increased to uin = 4 m/s when σ was set to 0.0. The graph in Figure 6(b) depicts the cost function against the number of iterations for errorless measurements. After 50 iterations, the objective function J decreases to 0.0002, illustrating that the estimated heat source rapidly converges towards the averaged exact heat source in the initial iterations, with the remaining iterations serving to fine-tune it locally.
The estimated contour plots of ψ(Ω1), ψ(Ω2) and ψ(Ω3) are given in Figure 9(a)–9(c), respectively, and the measured and estimated temperatures Y(Ss+3,s) and T(Ss+3,s) are given in Figure 10(a)–10(c) for chip 1, chip 2, and chip 3, respectively. Comparing Figures 4 and 10 shows that a higher air velocity will result in lower surface temperatures of encapsulated chips.
Furthermore, a striking resemblance between the precise and estimated values of both temperature and heat generation was noted. This observation was supported by the analysis of average errors, denoted as ERR1, s and ERR2, s, which are succinctly presented in Table 1. The inverse estimation of heat generations for inexact measurements, σ = 0.01 and 0.05, are also conducted, and the estimated results are listed in Table 1.
Similarly, to compare the exact and estimated heat generations more clearly, the exact and estimated ψ(Ω1), ψ(Ω2), and ψ(Ω3) with uin = 4 m/s at the center point of the x positions of each chip and varying the y position are illustrated in Figure 8(a)–8(c). Again, the heat generations can be reproduced well for σ = 0, but increasing the measurement errors results in acceptable estimates. These results indicate that the air velocities considered in this work have an insignificant influence on the estimated results; in addition, the estimates are reliable when considering error measurements.
Based on the results of the above numerical experiments, it can be concluded that the unknown magnitude of spatially dependent heat generations of multiple chips mounted on a PCB are accurately estimated in this work, and if the temperature measurements are exact or inexact, the inverse solutions remain reliable.
By employing simulated surface-temperature data, we adeptly delved into the realm of a 3-D steady-state inverse heat transfer problem, successfully unraveling the enigma of unknown volumetric heat sources attributed to multiple encapsulated chips adorning a PCB. The linchpin of this investigation lay in the adept utilization of the CGM optimization algorithm. Notably, the CGM's distinct advantage stems from its capacity to obviate the need for presupposing a particular functional form for the heat source. This attribute renders it exceptionally suited for addressing the intricate domain of function-estimation problems, wherein arbitrary initial conjectures for the heat sources are often the norm. It's pertinent to highlight that, while the CGM has formerly been harnessed to unravel inverse problems associated with either heat conduction or heat convection, this study represents its virgin application within the context of the inverse heat transfer problem. Rigorous evaluation ensued through the analysis of multiple test cases, spanning varying heat generations across each chip, an array of inlet velocities, and the inclusion of diverse measurement errors. Notably, simulation results consistently underscored the reliability of CGM-derived estimations for the heat generation of multiple chips, even when confronted with escalating air velocities and measurement inaccuracies. An example of this reliability emerges in the form of the maximum ERR2, 2, which stood resolutely below 3.5412% for σ = 0.05 and uin = 4 m/s. Emanating from the findings of these meticulous numerical experiments, the study culminates with the resounding verdict that the CGM, a potent optimization tool, aptly serves as the key to unlock the solutions to the complex 3-D steady-state inverse heat transfer problem.
The authors declare they have not used Artificial Intelligence (AI) tools in the creation of this article.
The research was funded by the National Science and Technology Council of Taiwan, under Grant NSTC-112-2221-E-006-140-MY3.
The authors declare that they have no conflicts of interest.
[1] | De La Vega-Salazar Y (2003) Situación de los peces dulceacuícolas en México. Revista Ciencias UNAM 72: 20-30. |
[2] | SEMARNAT (2016) Informe de la situación del medio ambiente en México. Compendio de estadísticas ambientales, indicadores clave de desempeñ o ambiental y crecimiento verde. Mexico: Semarnat. |
[3] | Naranjo EJ, Dirzo R (2009) Impacto de los factores antropogénicos de afectación directa a las poblaciones silvestres de flora y fauna. In: CONABIO, Capital natural de México, Vol. II: Estado de conservación y tendencias de cambio. México: CONABIO 247-276. |
[4] | Paulín-Vaca R, Hernández-Silva G, Lugo de la Fuente J (1997) Extracción secuencial de Cd, Co, Cu, Mn, Ni, Pb y Zn en sedimentos de la cuenca alta del Río Lerma. Actas INAGEQ 4: 85-96. |
[5] | Avila-Perez P, Zarazua G, Tejeda S, et al. (2007) Evaluation of distribution and bioavailability of Cr, Mn, Fe, Cu, Zn and Pb in the waters of the upper course of the Lerma River. X-Ray Spectrom 36: 361-368. |
[6] | Carrion C, Ponce-de Leon C, Cram S, et al. (2012) Potential use of water hyacinth (Eichhornia crassipes) in Xochimilco for metal phytoremediation. Agrociencia 46: 609-620. |
[7] | Zarazúa G, Avila-Pérez P, Tejeda S, et al. (2013) Evaluación de los metales pesados Cr, Mn, Fe, Cu, Zn y Pb en sombrerillo de agua (Hydrocotyle ranunculoides) del curso alto del río Lerma, México. Rev Int Contam Ambie 29: 17-24. |
[8] | Mercado-Borrayo BM, Heydrich SC, Rosas PI, et al. (2015) Organophosphorus and organochlorine pesticides bioaccumulation by Eichhornia crassipes in irrigation canals in an urban agricultural system. Int J Phytorem 17: 701-708. |
[9] | Aldana G, Hernández M, Cramb S, et al. (2018) Trace metal speciation in a wastewater wetland and its bioaccumulation in tilapia Oreochromis niloticus. Chem Speciat Bioavailab 30: 23-32. |
[10] | Tchounwou PB, Yedjou CG, Patlolla AK, et al. (2012) Heavy metals toxicity and the environment. EXS 101: 133-164. |
[11] | Wu X, Cobbina SJ, Guanghua M, et al. (2016)A review of toxicity and mechanisms of individual and mixtures of heavy metals in the environment. Environ Sci Pollut Res 23:8244-8259. |
[12] | Brito EMS, De la Cruz M, Barróna C, et al. (2015) Impact of hydrocarbons, PCBs and heavy metals on bacterial communities in Lerma River, Salamanca, Mexico: Investigation of hydrocarbon degradation potential. Sci Total Environ 521: 1-10. |
[13] | Bojórquez L. Esquivel-Herrera A (2017) Contaminación química de la zona lacustre de Xochimilco. In: Bojórquez L. Contaminación química y biológica de la zona lacustre de Xochimilco.Mexico: UAM, 85-169. |
[14] | Norma Oficial Mexicana NOM-001-Semarnat. Que establece los Límites Máximos Permitidos de contaminantes en las descargas de aguas residuales en aguas y bienes nacionales. Publicada en el Diario Oficial de la Federación el 6 de enero de 1997, México. |
[15] | Golovanova IL (2008) Effects of heavy metals on the physiological and biochemical status of fishes and aquatic invertebrates. Inland Water Biol 1: 93-101. |
[16] | Barata C, Varo I, Navarro JC, et al. (2005) Antioxidant enzyme activities and lipid peroxidation in the freshwater cladoceran Daphnia magna exposed to redox cycling compounds. Comp Biochem Physiol C 140: 175-186. |
[17] | Lushchak VI (2011) Environmentally induced oxidative stress in aquatic animals. Aquat Toxicol 101: 13-30. |
[18] | Chang LW, Suzuki T (1996) Toxicology of Metals. Lewis Publishers, 432-567. |
[19] | Pulido MD, Parrish AR (2003) Metal-induced apoptosis: mechanisms. Mutat Res 533: 227-241. |
[20] | Forget J, Pavillon JF, Beliaeff B, et al. (1999) Joint action of pollutant combinations (pesticides and metals) on survival (LC50 values) and acetylcholinesterase activity of Tigriopus brevicornis (copepoda, harpacticoida). Environ Toxicol Chem 18: 912-918 |
[21] | Tsangaris C, Papathanasiou E, Cotou E (2007) Assessment of the impact of heavy metal pollution from a ferro-nickel smelting plant using biomarkers. Ecotoxicol Environ Saf 66: 232-43. |
[22] | Enríquez-García C, Nandini S, Sarma SSS (2011) Demographic characteristics of the copepod Acanthocyclops americanus (Sars, 1863), (Copepoda:Cyclopoida) fed mixed algal (Scenedesmus acutus) rotifer (Brachionus havanensis) diet. Hydrobiologia 666: 59-69. |
[23] | Elías-Gutiérrez M, Suárez Morales E, Silva Briano M, et al. (2008) Cladocera y Copepoda de las aguas continentales de México. Guía ilustrada. México: UNAM, ECOSUR, SEMARNAT-CONACYT, CONABIO. 57: 67-92. |
[24] | Azad M, Agard JBR (2006) Comparative sensitivity of three tropical Cladoceran species (Diaphanosoma brachyurum, Ceriodaphnia rigaudii and Moinodaphnia macleayi) to six chemicals. J Environ Sci Health 41: 2713-2720. |
[25] | NMX-AA-087-SCFI-2010 Análisis de agua - Evaluación de toxicidad aguda con Daphnia magna, Straus (Crustacea -Cladocera) - Método de prueba, México, 1-44. |
[26] | USEPA (US Environmental Protection Agency) (2002) Methods for Measuring the Acute Toxicity of Effluents and Receiving Waters to Freshwater and Marine Organisms. Washington DC: U.S: Environmental Protection Agency, EPA-821-R-02-012. |
[27] | FAO (1986) Manual of methods in aquatic environment research. Part 10. Short-term static bioassays. FAO Fisheries Technical Paper. 247: 1-62. |
[28] | APHA, AWWA y WPFC (1994) Métodos estándar para el examen de aguas y aguas de desecho, 64º México: Ed. Interamericana, 124-278. |
[29] | Diz FR, Araújo CV, Moreno-Garrido I, et al. (2009) Short-term toxicity tests on the harpacticoid copepod Tisbe battagliai: lethal and reproductive endpoints. Ecotoxicol Environ Saf 72: 1881-1886. |
[30] | Buege JA, Aust SD (1978) Microsomal lipid peroxidation. Meth Enzym51: 302. |
[31] | Ellman L, Courtey KD, Andreas VJr, et al. (1961) A new rapid calorimetric determination of cholinesterase activity. Biochem Pharmacol 7: 88-98 |
[32] | Guilhermino L, Lopes MC, Carvalho AP, et al. (1996) Inhibition of acetylcholinesterase activity as effect criterion in acute tests with juvenile Daphnia magna. Chemosphere 32: 727-738. |
[33] | Bradford M (1976) A rapid and sensitive method for the quantification of microgram quantities of protein utilizing the principle of protein-dye binding. Annals Biochem 72: 248-254. |
[34] | Hintze JL (1997) NCSS 97: Statistical System for Windows. NCSS. Kaysville, UT. |
[35] | Raisuddin S, Kwok KWH, Leung KMY, et al. (2007) The copepod Tigriopus: A promising marine model organism for ecotoxicology and environmental genomics. Aquatic Toxicol 83: 161-173. |
[36] | Kulkarni D, Gergs A, Hommen U, et al. (2013) A plea for the use of copepods in freshwater ecotoxicology. Environ Sci Pollut Res 20: 75-85. |
[37] | Bo-Mi K, Jae-Sung R, Chang-Bum J, et al. (2014) Heavy metals induce oxidative stress and trigger oxidative stress-mediated heat shock protein (hsp) modulation in the intertidal copepod Tigriopus japonicus. Comp Biochem Physiol Part C 166: 65-74. |
[38] | Brown RJ, Rundle SD, Hutchinson TH, et al. (2005) A microplate freshwater copepod bioassay for evaluating acute and chronic effects of chemical. Environ Toxicol Chem 24: 1528-1531. |
[39] | Soto E, Oyarce G, Inzunza B, et al. (2003) Acute toxicity of organic and inorganic compounds on the freshwater cyclopoid copepod Eucyclops neumani neumani (Pesta, 1927). Bull Environ Contam Toxicol 70: 1017-1021. |
[40] | Devdutt K, Gergs A, Hommen U, et al. (2013) A plea for the use of copepods in freshwater ecotoxicology. Environ Sci Pollut Res 20: 75-85. |
[41] | Baudouin MF, Scoppa P (1974) Acute toxicity of various metals of freshwater zooplankton. Bull Environ Contam Toxicol 12: 741-751. |
[42] | WongCK, Pak AP (2004) Acute and Subchronic Toxicity of the Heavy Metals Copper, Chromium, Nickel, and Zinc, Individually and in Mixture, to the Freshwater Copepod Mesocyclops pehpeiensis. Bull Environ Contam Toxicol 73: 190-196. |
[43] | Lalande M, Pinel-Alloul B (1986) Acute toxicity of cadmium, copper, mercury and zinc to tropocyclops prasinus mexicanus (cyclopoida, copepoda) from three quebec lakes. Environ Toxicol Chem 5: 95-102. |
[44] | Abbasi SA, Nipaney PC, Soni R (1988) Studies on environmental management of mercury (II), Chromium (VI) and zinc (II) with respect to the impact on some arthropods and protozoans. Inter J Environmental Studies 32: 181-187. |
[45] | Ecotox Database. EPA Available at https://cfpub.epa.gov/ecotox/ |
[46] | Moraitou-Apostolopoulou M, Verriopoulos G (1982) Individual and combined toxicity of three heavy metals, Cu, Cd and Cr for marine copepod Tisbe holothuriae. Hydrobiologia 87: 83-87 |
[47] | Lee KW, Raisuddin S, Hwang DS, et al. (2007) Acute Toxicities of Trace Metals and Common Xenobiotics to the Marine Copepod Tigriopus japonicus: Evaluation of Its Use as a Benchmark Species for Routine Ecotoxicity Tests in Western Pacific Coastal Regions. Environ Toxicol 22: 532-538 |
[48] | Emadeldeen HM (2017) Acute Toxicity of Cadmium and Nickel to Three Marine Copepods. Sci J King Faisal Univer 18: 9-16. |
[49] | Pane L, Mariottini GL, Lodi A, et al. (2008) Effects of heavy metals on laboratory reared Tigriopus fulvus Fischer (Copepoda: Harpacticoida). In: Brown SE, Welton WC Heavy Metal Pollution. Nova Science Publishers, Inc, 158-165. |
[50] | Hutchinson TH, Williams TD, Eales GJ (994) Toxicity of cadmium, hexavalent chromium and copper to marine fish larvae (Cyprinodon variegatus) and copepods (Tisbe battagliai). Mar Environ Res. 38: 275-290. |
[51] | Barka S, Pavillon JF, Amiard JC (2001) Influence of different essential and nonessentialmetals on MTLP levels in the copepod Tigriopus brevicornis. Comp Biochem Physiol C 128: 479-493. |
[52] | Mance G(1987) Pollution threat of heavy metals in aquatic environments. London: Elsevier Aplied Science, 45-123. |
[53] | Traudt EM, Ranville JF, Meye JS (2017) Effect of age on acute toxicity of cadmium, copper, nickel, and zinc in individual-metal exposures to Daphnia magna neonates. Environ Toxicol Chem 36: 113-119. |
[54] | Moldovan OT, Melega IN, Leveib E, et al. (2013) A simple method for assessing biotic indicators and predicting biodiversity in the hyporheic zone of a river polluted with metals. Ecol Indic 24: 123-136. |
[55] | El-Shabrawy GM, Elowa SE, Rizk ST, et al. (2005) Impact of industrial pollution on zooplankton community structure in Rosetta Nile Branch at Kafr El-Zayat area Egypt. Afr J Biol Sci 1: 1-14. |
[56] | Abdel-Halim AS, Waheed ME, El-ShabrawyGM, Fawzia MF (2013)Sewage pollution and zooplankton assemblages along the Rosetta Nile branch at El Rahawy area, Egypt. Int J Environ Sci Engin 4: 29-45. |
[57] | Krupa EG (2007) Structural characteristics of zooplankton of the Shardarinskoe reservoir and their use in water quality assessment. Water Resour 34: 712-717. |
[58] | Gagneten AM, Paggi JC (2009) Effects of heavy metal contamination (Cr, Cu, Pb, Cd) and eutrophication on zooplankton in the lower basin of the Salado River (Argentina). Water Air Soil Pollut 198: 317-334. |
[59] | United Nations (2009) The globally harmonized system of classification and labelling of chemicals. ST/SG/AC.10/30/Rev.3. Available from: https://www.unece.org/fileadmin/DAM/trans/danger/publi/ghs/ghs_rev04/English/ST-SG-AC10-30-Rev4e.pdf |
[60] | Vasseur P, Cossu-Leguille C (2006) Linking molecular interactions to consequent effects of persistent organic pollutants (POPs) upon populations. Chemosphere 62: 1033-42. |
[61] | Hagger J, Malcolm JB, Lowe D, et al. (2008) Application of biomarkers for improving risk assessments of chemicals under the Water Framework Directive: A case study. Mar Poll Bull 56: 1111-1118. |
[62] | Broeg K, Westernhagen H, Zander S, et al. (2005) The bioeffect assessment index (BAI) a concept for the quantification of effects of marine pollution by an integrated biomarker approach. Mar Poll Bull 50: 495-503. |
[63] | Volodymyr I L (2011) Environmentally induced oxidative stress in aquatic animals. Aquatic Toxicol 101: 13-30. |
[64] | Wang MH, Wang GZ (2009) Biochemical response of the copepod Tigriopus japonicusMori experimentally exposed to cadmium. Arch Environ Contam Toxicol 57: 707-717 |
[65] | Wang MH, Wang GZ (2010) Oxidative damage effects in the copepod Tigriopus japonicusMori experimentally exposed to nickel. Ecotoxicol 19: 273-284. |
[66] | USEPA (1998). SCE policy issues related to the food quality protection act. Office of pesticide programs science policy on the use of cholinesterase inhibition for risk assessment of organophosphate and carbamate pesticides. Office of Pesticide Programs. Washington DC: US Environmental Protection Agency 20460. |
[67] | Dailianis S, Domouhtsidou GP, Raftopoulou E, et al. (2003). Evaluation of neutral red retention assay, micronucleus test, acetylcholinesterase activity and a signal transduction molecule (cAMP) in tissues of Mytilus galloprovincialis (L.), in pollution monitoring. Mar Environ Res 56: 443-470. |
[68] | Stefano B, Ilaria C, Silvano F (2008). Cholinesterase activities in the scallop Pecten jacobaeus: Characterization and effects of exposure to aquatic contaminants. Sci Total Environ 392: 99-109. |
![]() |
![]() |
u∞= 2 m/s | u∞= 4 m/s | ||||
σ=0 | σ=0.01 | σ=0.05 | σ=0 | σ=0.01 | σ=0.05 | ||
Iteration numbers | 50 | 9 | 8 | 50 | 8 | 8 | |
J | 0.0003 | 0.0764 | 0.2129 | 0.0002 | 0.0219 | 0.2665 | |
Chip 1 | ERR1, 1 % | 0.00002 | 0.00005 | 0.00006 | 0.00002 | 0.00005 | 0.00005 |
ERR2, 1 % | 0.1387 | 0.1477 | 0.1582 | 0.1270 | 0.1618 | 0.1769 | |
Chip 2 | ERR1, 2 % | 0.00002 | 0.0020 | 0.0026 | 0.00002 | 0.0024 | 0.0029 |
ERR2, 2 % | 1.3849 | 2.6339 | 2.9935 | 1.1964 | 1.8792 | 3.5412 | |
Chip 3 | ERR1, 3 % | 0.0002 | 0.0054 | 0.0199 | 0.0001 | 0.0067 | 0.0235 |
ERR2, 3 % | 1.9696 | 2.5668 | 2.9244 | 1.6506 | 2.0141 | 2.2662 |
![]() |
![]() |
u∞= 2 m/s | u∞= 4 m/s | ||||
σ=0 | σ=0.01 | σ=0.05 | σ=0 | σ=0.01 | σ=0.05 | ||
Iteration numbers | 50 | 9 | 8 | 50 | 8 | 8 | |
J | 0.0003 | 0.0764 | 0.2129 | 0.0002 | 0.0219 | 0.2665 | |
Chip 1 | ERR1, 1 % | 0.00002 | 0.00005 | 0.00006 | 0.00002 | 0.00005 | 0.00005 |
ERR2, 1 % | 0.1387 | 0.1477 | 0.1582 | 0.1270 | 0.1618 | 0.1769 | |
Chip 2 | ERR1, 2 % | 0.00002 | 0.0020 | 0.0026 | 0.00002 | 0.0024 | 0.0029 |
ERR2, 2 % | 1.3849 | 2.6339 | 2.9935 | 1.1964 | 1.8792 | 3.5412 | |
Chip 3 | ERR1, 3 % | 0.0002 | 0.0054 | 0.0199 | 0.0001 | 0.0067 | 0.0235 |
ERR2, 3 % | 1.9696 | 2.5668 | 2.9244 | 1.6506 | 2.0141 | 2.2662 |