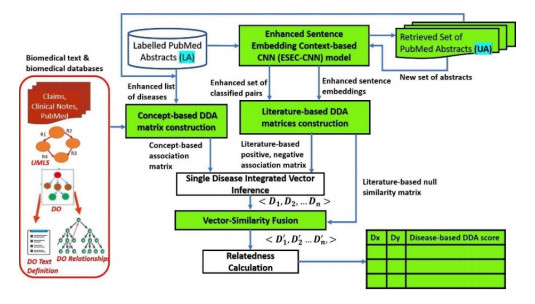
Citation: Patricia Morcillo, Maria Angeles Esteban, Alberto Cuesta. Mercury and its toxic effects on fish[J]. AIMS Environmental Science, 2017, 4(3): 386-402. doi: 10.3934/environsci.2017.3.386
[1] | Karpaga Priyaa Kartheeswaran, Arockia Xavier Annie Rayan, Geetha Thekkumpurath Varrieth . Correction: Enhanced disease-disease association with information enriched disease representation. Mathematical Biosciences and Engineering, 2024, 21(2): 2729-2730. doi: 10.3934/mbe.2024120 |
[2] | Li Hou, Meng Wu, Hongyu Kang, Si Zheng, Liu Shen, Qing Qian, Jiao Li . PMO: A knowledge representation model towards precision medicine. Mathematical Biosciences and Engineering, 2020, 17(4): 4098-4114. doi: 10.3934/mbe.2020227 |
[3] | Lei Chen, Kaiyu Chen, Bo Zhou . Inferring drug-disease associations by a deep analysis on drug and disease networks. Mathematical Biosciences and Engineering, 2023, 20(8): 14136-14157. doi: 10.3934/mbe.2023632 |
[4] | Hangle Hu, Chunlei Cheng, Qing Ye, Lin Peng, Youzhi Shen . Enhancing traditional Chinese medicine diagnostics: Integrating ontological knowledge for multi-label symptom entity classification. Mathematical Biosciences and Engineering, 2024, 21(1): 369-391. doi: 10.3934/mbe.2024017 |
[5] | Peng Wang, Shiyi Zou, Jiajun Liu, Wenjun Ke . Matching biomedical ontologies with GCN-based feature propagation. Mathematical Biosciences and Engineering, 2022, 19(8): 8479-8504. doi: 10.3934/mbe.2022394 |
[6] | Huiqing Wang, Jiale Han, Haolin Li, Liguo Duan, Zhihao Liu, Hao Cheng . CDA-SKAG: Predicting circRNA-disease associations using similarity kernel fusion and an attention-enhancing graph autoencoder. Mathematical Biosciences and Engineering, 2023, 20(5): 7957-7980. doi: 10.3934/mbe.2023345 |
[7] | Lei Chen, Xiaoyu Zhao . PCDA-HNMP: Predicting circRNA-disease association using heterogeneous network and meta-path. Mathematical Biosciences and Engineering, 2023, 20(12): 20553-20575. doi: 10.3934/mbe.2023909 |
[8] | Zhaoyu Liang, Zhichang Zhang, Haoyuan Chen, Ziqin Zhang . Disease prediction based on multi-type data fusion from Chinese electronic health record. Mathematical Biosciences and Engineering, 2022, 19(12): 13732-13746. doi: 10.3934/mbe.2022640 |
[9] | Haipeng Zhao, Baozhong Zhu, Tengsheng Jiang, Zhiming Cui, Hongjie Wu . Identification of DNA-protein binding residues through integration of Transformer encoder and Bi-directional Long Short-Term Memory. Mathematical Biosciences and Engineering, 2024, 21(1): 170-185. doi: 10.3934/mbe.2024008 |
[10] | Zhi Yang, Kang Li, Haitao Gan, Zhongwei Huang, Ming Shi, Ran Zhou . An Alzheimer's Disease classification network based on MRI utilizing diffusion maps for multi-scale feature fusion in graph convolution. Mathematical Biosciences and Engineering, 2024, 21(1): 1554-1572. doi: 10.3934/mbe.2024067 |
DDA acts as a key factor to understanding disease relationships, such as comorbidity, which is essentially the co-occurrence of diseases among the same patients that plays an important role in health care for drug discovery [1] and better treatment plan. To meet the emerging need, several studies in biomedical domain for relating diseases have been carried out [1,2,3,4]. In the work of Suratanee and Plaimas [3], a network-based approach was employed to calculate DDA strength that achieves a performance of 0.71 area under curve (AUC). Zitnik et al. [1] has predicted DDA relationships and found about 66 disease classes have significant high relationships with p-value < 0.001. Another work in [4], a disease similarity database tool was developed that performs hypergeometric test of p-values for different pairs of diseases. On the other hand, the DDA relationships were analysed using disease causality network. Further, the sorted potential association strength were compared between top and bottom group of disease pairs and found 95% of disease pairs in upper group. Since one disease can multiply into another in any patient, treating associated diseases is a great challenge for modern medicine. Hence, exploring DDA helps in gaining better insight of disease relationships, which is helpful for clinicians in proper diagnosis and treatment.
For better understanding of DDA, it is important to know the various underlying aspects with which diseases are associated. One such aspect considers biological entities such as other diseases [5], genes [4,6], pathways [7], drugs [8], and phenotypes [9] as intermediate factors, facilitating indirect DD association. Another aspect, revolves around the vast established heterogeneous biomedical databases such as biomedical datasets including Protein-Protein Interaction Network [4,10], HumanNet [11] and biomedical ontologies like DO [12], GO [13], Human Phenotype Ontology [14], Unified Medical Language System (UMLS) [15], Medical Subject Headings (MeSH) [16]. On the other hand, connection between diseases can be inferred using biomedical text such as PubMed [17,18], MedLine [19], Clinical Notes, Claims Database and PubMed Central (PMC) [20], Electronic Health Records [21] and HealthMap Corpus [22]. In order to widen the range of components affecting disease associations, non-Biomedical Text such as Wikipedia [17,23] has also been considered.
In addition, measuring the strength of DDA helps to improve the clinical decision making. As a quantitative measurement, disease similarity is generally used to indicate the extent to which the diseases are associated, since similar diseases are usually caused by similar semantic aspects such as similar etiology, markers, mechanisms, patterns etc. In this regard, by involving a single biological source, the strength of disease associations is computed by IC-Based methods such as Wang et al. [24], Resnik [25] and Lin [26], accomplished solely based on semantic associations of ontologies such as MeSH, DO, HPO. Taking advantage of biological process terms, some statistical-based approaches are proposed. In the work of Mathur and Dinakarpandian [27] calculated the association strength by overlapping genes of diseases using GO. In another work, association of diseases is computed using both information content and co-occurrence of terms in ontology [28]. Recently some research employed neural network approach, word embedding model, to learn ontological node vector representations used in application of associating diseases through similarity values [29]. Apart from ontologies, DDAs can also be quantified by mining a large corpus of biomedical literature. In the context of text, O'Shea [18] used a network-based shortest path distance method to calculate the relatedness between diseases from occurrence frequency of disease terms. Alternatively, using neural network-based approach, Beam et al. [20] derived distributional vector representations from clinical notes, insurance claims, journal articles and projected the learned context-based concept vector representations to distributional space for relatedness computation. Therefore, in general either semantic aspects or concept-based aspects have been considered for the calculation of DDA strength. However, considering both the above aspects could lead to more effective strength calculation.
Some efforts have been put-forth to combine different biomedical knowledge from various sources to derive representations of biomedical concepts for measuring the relatedness of the concepts. There are works that fused various biomedical knowledge such as biomedical entities, biomedical datasets and ontologies [30,31]. On the other hand, with the growing biomedical literature, some work has attempted to compute relatedness of biomedical concepts, with an integrated vector representations mined from both literature and semantic ontological information [32,33]. However, the integrated vector encoded only limited aspects of contextual relations from literature and semantic relations from ontology. Hence, in this paper, an integrated vector is derived covering a wide range of both contextual and semantic relations for an effective DDA strength calculation.
The structure of the paper is organized as follows: Section 2 briefly reviews the state-of-the-art methods related to biomedical association classification and strength computation. Subsequently, a set of datasets used in this work and the proposed DDA framework is described in detail in Sections 3 and 4 respectively. Section 5 presents the experimental results that evaluates the quantified DDA scores obtained using the proposed framework. Finally, an outline of conclusion is drawn in Section 6.
Biomedical literature contains associations linking diseases with other diseases. Given their significance in health-oriented applications, it is imperative to investigate these digitized data to extract the type of association using text mining approach. Given a sentence and disease pair appearing within the sentence, the DDA type can be of 3 types: positive association, where there exists an explicit mention of association with words like association, comorbidity factors, complicatin, risk factors, etc., negative association, in which a negative word explicitly conveying that no relation exists between the two disease mentions and neutral or null association that does not state about any association between the co-occurring diseases. Towards this end, a number of literature-based methods have been proposed for the extraction of associations between different biomedical entities [17,34,35,36,37].
The co-occurrence statistical technique, assumes that more the frequency of entities occurring together within abstract or sentence higher the chance of being positively associated [8]. Li et al. [38] employed the co-occurrence statistics to detect disease-related associations. Rosário-Ferreira et al. [47] considered diseases to be related if they are co-mentioned in the abstract text. However, entities occurring together may not be semantically connected, and thus result in low precision [39,40,41].
Some manually or automatically formulated rules finds its role in the association extraction task. Lee et al. [42] and Song et al. [43] drafted number of rules manually for PPI and disease-gene relation extraction respectively. In addition, Tari et al. [44] used automatically created rules to identify the biomedical relations from MEDLINE abstracts. The major limitation of rule-based system is that it is difficult to create rules entailing all types of associations and moreover a deeper insight into the biomedical knowledge for creation of such rules is required.
However, with the huge set of annotated training text available for biomedical associations, machine learning approach can overcome the above limitations by its ability to learn relation patterns of sentences which can then automatically detect the association type in unseen texts. Bhasuran and Natarajan [45] used a supervised machine learning method for gene-disease association extraction, which required a large training set and was time-consuming. Zhang and Lu [46] and Rosário-Ferreira et al. [47] eliminated this deficiency by using a semi-supervised method, that utilized a small training set which learns DDA patterns from PubMed abstracts. However, machine learning (ML)-based methods require enormous manual efforts in designing biomedical relation features for the association extraction task as ML methods lack automatic feature extraction.
These issues were addressed by employing deep neural networks for efficient feature engineering in text-mining for curating number of biomedical relation types, as it involved an automatic feature learning process [35,48,49,50]. One of the popular deep neural network models, Convolutional Neural Network (CNN), was widely used for classifying whether sentences contain positive, negative or null associations between biomedical entities using sentence representation, where different representations of various local-level features captured at sentence-level and global-level features captured at corpus-level were used for classification [17,34,37].
A Multi-Channel Dependency based CNN extracted PPIs into positive and negative associations, where the sentence representation covered word embeddings trained only on global-level features from PubMed and PMC [35]. Using additional embeddings from Wikipedia and MEDLINE, the Multi-Channel CNN (MCCNN) model classified DDI and PPI into positive associations such as effect, mechanism, etc and negative associations. An attempt was made to classify different biomedical associations such as gene-disease associations (GDAs) [34], using disease position as the only local-level feature, DDAs [17] using Parts-of-Speech (POS) as additional feature and spice-disease [37] using Parts-of-Speech (POS) and chunk tag as additional local-level features.
However, only a limited number of local-level and global-level features were used in sentence representation for the sentence-level classification of biomedical associations into positive, negative and null.
Similar research considering local and global text and video features have been carried out in the work of Wang et al. [51] for video-text retrieval. In the text part, they considered only the encoded full text representation as global text feature and the decoded global representation is extracted as local text feature. In neither case, no various local-level features nor the global-level features of each word in given text is embedded.
Moreover, most of the above work, only classified associations and did not attempt to calculate the association strength. An attempt was made to calculate only the strength of positively correlated pairs using statistical [18] and pattern-based approaches [52]. While literature-based approaches have mainly been used for the classification of biomedical associations, we need a concept-based approach for effective association strength calculation.
Biomedical ontologies have integrated non-duplicative biomedical concept terms and medical data, providing a high coverage of biomedical concept terms which have been used to compute the semantic association strength between biomedical entities. Quantitative semantic association among diseases help clinicians gain a better knowledge of diseases, since semantically associated diseases reveal similar or common underlying attributes, that further help in proper treatment plan [31]. Therefore, discovering the quantitative semantic biomedical associations using biomedical ontologies plays a crucial role in biomedical field [11,31].
Some work has encoded conceptual sources for computing semantic associations. Wei et al. [53], Beam et al. [20] and Pakhomov et al. [54] used only unstructured corpora such as insurance claims, clinical notes, etc., to include the conceptual aspects into the association computation. While Wei et al. [53] exploited ontology only to retrieve disease concepts. With additional semantic relation types information, Yu et al. [33] attempted to associate biological entities with improved semantics. However, taxonomic relationships conveyed by ontologies are needed for an enhanced semantic association quantification.
Most of the ontology-based methods were node-based, edge/path-based and hybrid-based. The node-based approaches use properties of the node such as Information Content (IC) [25,55] and their variants [56,57,58] for computing semantic association between the concepts based on their lowest common ancestor. However, the IC values computation is based on the annotated corpus and hence is corpus dependant. On the other hand, the edge/path- based approach uses the edges count between the given concepts to measure the association. One such method proposed by Wu and Palmer [59], used the common path from root node to the least common ancestor node while Richardson et al. [60] used the edge weight technique based on node density, depth and connections between parent-child nodes for computing the conceptual associations. Further, Cheng et al. [61] proposed a weighted maximum common ancestor depth and Wu et al. [62] proposed a non-weighted maximum common ancestor depth to measure the semantic associations. Using the topology of DO, Wang et al. [63] calculated the strength of association by considering the semantic impact of ancestors on the entities involved in association. However, the problem with edge-based measure is that the concepts at same depth are not semantically well differentiated. As a hybrid measure, Mazandu and Mulder [64] used the topological positional characteristics of the GO for association strength calculation. Zhao and Wang [58] computed relatedness using the count of children nodes and topology of GO. Kamran and Naveed [65] also exploited the topology of GO along with common descendants to calculate the strength of associations. However, the computation of semantic relatedness using hybrid methods have not incorporated the semantic meaning of the concepts captured within the ontology.
Semantic associations based on semantic meaning of concepts can also be computed using vectors learnt from the ontological graph structure. Camacho-Collados et al. [66] used the graph-based vectors and computed the semantic association, where the vector representation is solely based on the structure of the graph. Guo et al. [67] and Zhong et al. [68], used graph embeddings which can capture the structural information connecting nodes in graph but no relationship information was considered. Smaili et al. [69] represented concepts by general corpus trained aggregated embeddings of all its annotated nodes including the ancestors, where there is no control on the amount of ancestorial information affecting the given concept. Hence, the problem with vector-based association is that representation of vectors has encoded only a limited ontological relationship information without any control of the contribution effect of the entities involved in the association.
Attempts have been made to measure association between diseases by integrating multiple data sources as well as fusing the details of various biological entities extracted from these biomedical sources. Su et al. [31] developed a joint association method combining biological entities such as genes, phenotypes and integrating ontological sources (DO, HPO), where semantic associations determine the disease associations. Similarly, Cheng et al. [30] spans different biomedical sources (DO, HumanNet) fusing functional and semantic associations for measuring the association strength. With the unprecedented growth of biomedical literature, there has been a significant gap between the increasing published scientific knowledge and the tailored biological data knowledge [70]. Hence, it is necessary to integrate the contextual knowledge obtained from biomedical literature with the semantic knowledge of biological data sources for the DDA task. Deng et al. [71] used the biological-process based approach, integrating both literature and ontology (GO) and proposed a combined score of semantic and contextual associations using symptoms, genes and their related functions. In addition, li et al. [72] proposed a relatedness method integrating contextual and functional associations mined from literature (MedLine) and biomedical network (PPI), respectively. Moreover, Jiang et al. [32] proposed a hybrid semantic embedding model incorporating both corpus-based distributional representation into multiple ontologies to gain a better similarity score of biomedical concepts. Similarly, Yu et al. [33] used neural network approach to induce the vector representation of biomedical concepts by retrofitting contextual information from literature (PubMed) using semantic information from ontology (UMLS) such that the resulting vectors can be utilized to measure the association strength. However, both Jiang et al. [32] and Yu et al. [33], generated the corpus-based representation for each concept independently without considering the different types of context (association) of the sentences. On the other hand, the ontological knowledge integrated by Jiang et al. [32], was only edge-based semantic similarity of concept pairs that did not incorporate semantic meaning of concepts as well as their ontological relationship connections. In addition, the existing methods associate the biomedical concepts (entities) using only a limited aspect of contextual and semantic relations, which results in low correlation with human judged association scores.
Thus, for the biomedical association quantification from literature, particularly DDA, the existing classification model has used only a limited number of local-level and global-level features that could capture only limited syntactic, semantic, and contextual features for sentence representation learning. Hence, in order to improve the classification performance, there is a need to include additional local and global-level features. The existing methods either not calculated or calculated only positive association strengths. However, it is important to quantify the strength of DDA pairs based on all types of DDA pairs positively, negatively, and null associated by sentence embeddings under different contexts.
Similarly, for concept-based quantification of DDA, existing methods embedded concepts by considering only the connectivity of concepts in ontology. The semantic meaning of concepts and the various ontological relationships affecting the associations not embedded. In addition, all ancestors are treated equally. However, controlling the impact of ancestorial embedding is important as each ancestor may either be closely or distantly related to each concept in the association.
The integrated approaches fusing literature and ontology, did not consider different context types of sentences from literature and did not incorporate multiple semantic meaning of concepts with ontological relationships. Moreover, the existing methods have fused only limited semantic type relations from ontology with limited contextual relations from literature. However, the association varies based on the taxonomical connection relationship type that exists in the ontology. Therefore, there is a need to integrate both contextual relations from literature and richer semantic relationships from ontologies for an enhanced DDA strength quantification.
Of significance, while there are existing association quantification methods that have fused semantic relations from ontology with contextual relations from literature, we improve the association quantification in this paper:
1) We enhanced literature-based DDA representation by considering all context types of association sentences such as positive, negative and null with improved sentence representation.
2) We also enhanced concept-based DDA representation by the proposed ontology-based joint multi-source association representation where semantic meaning of concepts and the various ontological relationship connections are incorporated for a better DDA quantification.
3) We present an enhanced and integrated DDA framework to widen the coverage of various relationship aspects of association components both contextually A) and conceptually (semantically) B) to build an information enriched disease vector representation.
We initially used the available and already annotated 521 abstracts dataset [17] for training of the proposed ESEC-CNN model. However, in order to achieve better modelling, we expanded this dataset. To assist the DDA dataset expansion, an initial set of approximately 3 million bio-concept annotated disease-related PubMed abstracts have been extracted using PubTator. PubTator, an automatic text-mining tool, recognize various biomedical entities such as genes/proteins, diseases, genetic variants, spices and chemicals in the titles, abstracts of PubMed articles [73]. To ensure sentence-based DDA, only 39,510 abstracts with at least a DDA sentence are retained for further processing.
DO, a taxonomy of diseases, in which each disease term is linked to another in a hierarchical manner by a semantic type "is_a" association has been used [12]. DO mapping each disease term to its disease id DOID along with the term definition and the human disease related knowledge base is downloaded from http://purl.obolibrary.org/obo/doid/releases/2022-06-07/doid.owl (accessed 7 June 2022). In this work, the conceptual linking of diseases for concept-based DDA has been established using various DO relationships. Approximately 8000 diseases out of 14,958 diseases from the enhanced dataset were mapped to DO, whose corresponding term definitions are further utilized in concept embedding.
The UMLS consists of three components, Metathesaurus, Semantic Network and Lexicon tools, that has concepts with concept ID (CUI), definitions and its linkage to other concepts with semantic relations such as CHD "Child", SY "asserted synonymy", RN "has a narrower relationship", RO "has other relationship", RQ "related and possibly synonymous", etc. In this work, only Metathesaurus concepts file, containing the concept pairs relationships are used for concept embedding in concept-based DDA [15].
We evaluate the obtained DDA scores of our approach against the results of DisGeNET, that contains about 10, 48,575 DD pairs from a curated DDA database. DisGeNET defines DDAs based on shared genes and variants among the available gene-disease associations [74]. This well-known database has been used for direct comparison of DDA strengths in both the perspectives. Nicia et al. [47] used DisGeNET to evaluate the results of DDAs obtained using SicknessMiner. The phenotypic similarity of diseases werealso evaluated using the DisGeNET scores for inborn errors of immunity [75]. Further, we created a standard dataset, to compute DDA strength using functional GO as an association criteria. The disease-related GOs are obtained from CTD. Some of the attributes of the datasets are disease1, disease2 and the Jaccard similarity scores using genes, variants and GOs. In this work, we have adopted DisGeNET as well as the created standard dataset for evaluating DDA strength.
In addition, the performance of the obtained DDA strength of our approach is also evaluated using the human rated DDA pairs. Hence, a combined standard DDA dataset with human assessed scores is created using 213 disease-disease pairs obtained from UMNSRS [54] and MayoSRS [76], by mapping the concept terms to disease terms using CTD disease vocabulary [77].
The proposed work effectively measures the association strength between different diseases by integrating various types of disease-disease linking contextual and conceptual relations. In this work, contextual relationships are obtained from biomedical literature such as the PubMed abstracts. Similarly, biomedical databases (DO [12], UMLS [15] and biomedical text (Clinical Notes, Insurance Claims Database, Journal Articles) [20] are utilized to obtain conceptual relations. Deriving DDAs through integration of multiple linking perspectives associating the given disease pair and computing the aggregated DDA strength are important.
Figure 1 describes the proposed framework. With the list of diseases as main input, collection of associated PubMed abstracts is the first step. In Section 4.1, the proposed deep neural network model, Enhanced Sentence Embedding with Context-Based CNN (ESEC-CNN) is trained on preprocessed and labelled (positive, negative and null DD pairs) 521 PubMed abstracts [17]. The built model is further exploited to classify a new set of PubMed abstracts collected iteratively. This dataset is used to improve the general performance of DDA prediction. This dataset is used to improve the general performance of DDA prediction. The set of classified DDAs and sentence embeddings obtained from the enhanced dataset are further utilized to construct literature-based DDA matrices. In addition, the enhanced list of diseases is also used for the construction of concept-based DDA matrix of DDA representations as described in Section 4.2. Using the biomedical text and biomedical databases, Ontology-based joint multi-source association embedding model is proposed to improve concept-based DDA. The integration of literature-based and concept-based DDAs for DD association enhancement is described in Section 4.3 using a modified vector-similarity fusion method [78] to improve the quality of integrated disease vector. Finally, the relatedness score between DDs is calculated using cosine similarity of the integrated disease vector [79].
The DDA dataset derived from initial 521 labelled abstracts are used for construction of enhanced literature-based DDA matrices using sentences with disease pairs classified into positive, negative and null pairs. For this classification, we proposed a neural network architecture as illustrated in Figure 2. The network is designed to capture syntactic and semantic information for a given sentence with DD pairs from three different perspectives using
1) Sentence-based local-level features
At sentence-level, we have used Parts-of-Speech (POS) feature using one-hot encoding scheme represented by 11-bit binary vector [35] and two-dimensional disease distance feature [17]. For DDA, new additional features such as dependency relations [80] and chunk [81] are included and Named Disease Entity (NDE) feature is obtained, similar to the work of Peng and Lu [35]. The NDE feature is applied to each word in a sentence represented by a four dimensional encoding < D1, D2, D, O > , where D1 and D2, represents the disease pair under consideration. Other disease words and non-disease words are represented by D and O respectively.
2) Sentence-based global-level features
Using a popular embedding model word2vec [82], the embedding of each word in a sentence is learnt at corpus-level using both domain-specific context such as PubMed and PMC and general contexts including news, in addition to Wikipedia [83].
3) Document-level features
Similar to the work of Lai et al. [17], the traditional document features such as Bag-Of-Word, word-based Parts of Speech, NDE information and document-based information are represented using one-hot encoding.
Thus, in this work, an enhanced sentence embedding with additional features is framed that helps the proposed classification model in better classification of different types of association.
In Figure 2, the input to ESEC-CNN is the embedding layer representing the sentence followed by convolution and pooling layers outputting an n-dimensional enhanced sentence embedding vector. Similarly, the document representation [17] of m-dimension is merged with enhanced sentence embedding to create (n + m) dimensional final single vector. The fully connected layer with categorical hinge loss in activation function [84] is applied to the obtained merged vector. The combined vector is further passed on to three-dimensional output layer representing the probability of classes: positive, negative, null.
The trained classifier model is effectively utilized in our work to classify the new set of extracted PubMed abstracts. In order to improve the performance of DDA strength calculation, it is essential to widen the range of positive, negative and null contexts of DD pairs, therein, aggregating the contextual information contribution to the DD strength during the construction of enhanced literature-Based DDA matrix. Further, the number of seed diseases is also increased, thus we attempt to measure the strength of association between a larger number of DD pairs. The dataset is constructed by an iterative technique with initial 213 seed DD pairs collected from a combined benchmark datasets including UMNSRS Similarity and Relatedness [54], MayoSRS and MiniMayoSRS between Medical term pairs [76], until we obtain 58,980 unique DD pairs.
In order to effectively quantify DDA strength using literature, considering positive, negative and null associations is important as each type conveys different degrees of association. Hence, the DDA classes (positive, negative and null) predicted by LC-CNN model along with improved sentence representations are further utilized to construct two literature-based DDA matrices namely, literature-based positive, negative DDA matrix of DDA representations and literature-based null similarity matrix.
1) Literature-based positive, negative DDA matrix
As discussed in Section 2.1, sentence-based biomedical associations are classified into only positive, negative [17,34,35,36,37] or only as negative [36]. While during the strength calculation, O'Shea [18] and Xu et al. [52] considered only positively correlated pairs. However, it is important to calculate the strength of association of pairs that occur in both positive and negative contexts and those that occur only in negative context. Considering the above aspects, cumulative association strength is calculated in Eq (1).
LVxy={npos∗∑li=1(Dx−Dy)TDVectori(−)nneg∗∑mj=1(Dx−Dy)TDVectorj} | (1) |
where: LVxy represents association vector of disease pair Dx−Dy, npos and nneg is the number of positive contexts and negative contexts respectively. TDVectori and TDVectorj denote enhanced sentence representations with two disease mentions vector in positive and negative cases respectively.
The association strength of disease pair Dx−Dy, is dealt differently if it falls in any of the three cases.
Case 1 npos∗∑li=1(Dx−Dy)TDVectori, strengths the DDA if Dx−Dy occurs only in positive contexts.
Case 2 nneg∗∑mj=1(Dx−Dy)TDVectorj, identifies negative association strength if Dx−Dy occurs only in negative context.
Case 3 Eq (1) combines case 1 and case 2 using an association modification factor (-) that modifies association strength if Dx−Dy occurs in both positive and negative contexts.
2) Literature-based null similarity matrix
Though Rakhi et al. [37] has classified sentence-based biomedical entity pairs as null, these associations were not considered while calculating the strength of association. However, null pairs with unmentioned associations may also be associated with some strength and hence needs to be taken into consideration. In addition, in this work, we have also extended the concept of null association within same sentence [17,34,37] to across different sentences having single disease mention and therefore, including corresponding embedding information also contributes to DDA strength computation. Accordingly, we have derived an equation Eq (2) representing a disease vector.
LV(Dx)=(∑Ni=1TDVectorDx−Di)+(∑Mj=1ODVectorDx−Dj) | (2) |
where: LV(Dx) denote the disease vector representation of disease Dx, TDVectorDx−DiandODVectorDxj denote two-disease and single disease mention enhanced sentence representations.
The represented disease vector LV(Dx), consists of 2 important components in the context of DDA as follows:
• ∑Ni=1TDVectorDx−Di, accumulates enhanced sentence representations of Dx when it occurs in the same sentence with all other unmentioned or null associated diseases.
• ∑Mj=1ODVectorDxj, accumulates enhanced sentence representations of Dx when it occurs as single disease mention in sentences.
LV(Dy) is calculated in the same way and Dx−Dy strength is calculated using cosine similarity, cos(LV(Dx),LV(Dy)), that helps modify DDA with null associations and discover DDAs that are not directly conveyed by positive/negative associations.
Using Eqs (1) and (2) described in 1) and 2), we are able to construct an enhanced literature-based positive, negative DDA matrix and literature-based null similarity matrix shown in Figure 3. that is later used to calculate literature-based DDA strength.
In order to integrate conceptual aspects for DDA calculation, a detailed ontological mapping covering a wide range of taxonomic relationships, plays a vital role and contributes to the quantification of semantic associations between diseases. Some of the taxonomical ontological relationships include ancestorial parent-child relationship and other relationships like sibling and indirect relationships (uncle, cousin). Wang et al. [63] has not considered the semantic relationship in disease association measurement while only parent-child relationship is considered in the prediction of onset of diseases [85,86]. For DDA, in this work, we consider ancestorial and other closely related taxonomical relationships to derive a better degree of association linking diseases. Given DO as a DAG, having nodes corresponding to the ancestors and disease concepts DxandDy involved in Dx−Dy association, the ancestorial relationship and ontological relationship connection between Dx (disease concept1), Dy( disease-concept2) are used to learn the association representation.
For DDA measurement, when we embed each disease (concept), we need to do so in relation to a disease pair. For this, the connectedness of concepts [68] and semantic information of all ancestors are used [29,85,86]. However, discovering new ancestors sets New_Anc_Set, prior to association representation is important as not all ancestors contribute to the final association.
After discovering the ancestors sets, we introduce a 2-stage DDA quantification, ontology-based joint multi-source association representation, shown in Figure 4. In stage-1, we have included the association effect of the influential factors by infusing multi-source semantic (DO, UMLS) and contextual information (clinical notes, insurance claims, journal articles) of ancestors including the root ancestor node and leaf node. In addition, we add novel level-weight to the multi-source ancestorial representation, where the level-weight is based on new ancestors sets New_Anc_Set discovered initially, thus producing an association embedding matrix. In stage-2, we introduce ontological relationship connection-based DDA quantification that varies the embedded association strength between diseases based on their type of relation connection in the ontology, thus resulting in concept-based association matrix of DDA representations.
Thus, in this work, we try to improve the concept-based DDA by constructing a concept-based DDA matrix of DDA representations using ontology-based joint multi-source association embedding model as shown in Figure 4.
As discussed in Section 4.2, including all ancestors of given disease concept may cause semantic contribution of even the concepts that are not common between diseases in the disease pair and hence, embedding of disease under consideration may lead to incorrect association. In order to tackle this aspect, that is, rather than considering all ancestors of a particular node in the ontology, we consider only those ancestors that contribute to the association between diseases by defining new sets of ancestors New_Anc_Set(Dx)andNew_Anc_Set(Dy) for Dx and Dy respectively for Dx−Dy association. Therefore, the derived ancestors set Anc_Set(Dx) of disease Dx in Dx−Dy association is described in Eq (3), where only common ancestors A′is are considered since two diseases are associated by sharing of common diseases in the DO. In addition, the ancestors on the longest path A′jxs with respect to Dx is also considered to cover a broader etiology of the disease concept.
NewAncSet(Dx)=[A′is∈common(Dx,Dy)]+A′jxsonlongestpathfromLCS(Dx,Dy) | (3) |
where common(Dx,Dy) denotes the common ancestors of Dx and Dy.
Further, by utilizing the discovered ancestors sets, ontology-based joint multi-source association embedding model is proposed, consisting of 2 stages, described in sub-sections 4.2.2 and 4.2.3.
Stage-1 Novel-ancestorial level-based DDA quantification using multi-source embeddings
Figure 5 shows the derived embedded association representation, CVxy for two disease nodes in the given DO, where the representation is divided into two components, A) Multi-source ancestorial Embedding and B) Novel ancestorial level-weight for each of the diseases DxandDy respectively, discussed in following sections.
A) Multi-source ancestorial embedding
As discussed earlier in Section 4.2, Song et al. [86] considered all ancestors and included only semantic embeddings of ancestors excluding the root ancestor node and leaf node (DxinDx−Dy). However, we consider only new ancestors sets, New_Anc_Set(Dx)andNew_Anc_Set(Dy) as discussed in Section 4.2.1 and various conceptual knowledge of ancestors from multiple sources, since, Dx−Dyassociation may be influenced by several factors such as symptoms, biological entities (genes, proteins, etc.), other diseases, affected patient records, etc., which can be covered by infusing embeddings from different sources. In addition, considering multi-source information of root node and leaf node (Dx) is important in the context of DDA as root node is common to both Dx and Dy and leaf node Dx is involved in Dx−Dyassociation. As shown in Figure 5, the multi-source ancestorial embedding of A1∈New_Anc_Set(Dx) is given by the component A, in which we assign multi-source contextual embeddings vDOTextA1, vBioTextA1 from DO and biomedical text [3] and semantic embedding vUMLSA1 from UMLS [33]. For embedding text definition from DO, in this work, we adopted the procedure used by Park et al. [23] to fill in the definition of diseases using the first lead paragraph from Wikipedia, applying an embedding method, Doc2Vec [87]. The combined semantic and contextual information is then infused into the deep neural network embedding model through attention mechanism [85,86]. The attention weights on multi-source embeddings with respect to Dx are denoted by αDOTextA1x,αUMLSA1x,αBioTextA1x. The weight computation for text definition embedding from DO for ancestor A1∈New_Anc_Set(Dx) is computed using equation Eq (4.1) by SoftMax function as follows:
αDOTextA1x=exp(fDOText(vDOTextA1,vDOTextDx))wDOTextDx | (4.1) |
where wDOTextDx is given by Eq (4.2).
wDOTextDx=∑Ak∈New_Anc_Set(Dx)(exp(f(vDOTextAk,vDOTextDx))+exp(f(vUMLSAk,vDOTextDx))+exp(f(vBioTextAk,vDOTextDx))) | (4.2) |
where f(vDOTextA1,vDOTextDx),f(vUMLSA1,vDOTextDx),f(vBioTextA1,vDOTextDx) denotes the scalar score functions defined in Eq (4.2) to find the compatibility between text embedding of Dx from DO and multi-source ancestorial embeddings, which are computed using a single layer feed forward neural network using Eq (4.3).
fDOText(vDOTextA1,vDOTextDx)=zTtanh(N[vDOTextA1vDOTextDx]+bias) | (4.3) |
Z, N and bias are the learning parameters used by the neural network.
Similarly, other attention weights of ancestor A1 w.r.t Dx from other sources are calculated in similar manner. Similar kind of equations are adopted in case of ancestor A2 w.r.t Dy.
B) Novel ancestorial level-weight
The next component of stage-1, controls the semantic and contextual contribution effect of each ancestor by adding level-weight to the aggregated multi-source embeddings obtained using component A. We used the ancestorial level-weights similar to Wang et al. [63] (relative positions in MeSH) and Kamran et al. [65]. Wang et al. [63] and Kamran and Naveed [65], calculated the ancestorial level-weight by choosing the maximum of level-weights among all children of ancestor with respect to each entity in association. This may lead to assigning level-weight of ancestor by children which may be neither common nor on the longest path to Dx and Dy, thus failing to include level-weights of nodes contributing to the association. Thus, selecting the level-weight contributed by children that are common ancestors and those that fall into longest path with respect to Dx and Dy, New_Anc_Set of DxandDy, reveals the actual semantic value or level-weight of ancestors. As a special case of computing level-weight of least common subsumer (LCS), Kamran et al. [65], calculated the semantic value or level-weight of LCS by considering only the level-weights of the ancestors on the longest path from root to LCS which included only the influential effect of ancestors of LCS. However, this will not help in identifying the true level weight of LCS with respect to each of the descendant entities in association. Therefore, for computing the level-weight of LCS, it is required to consider level-weights of children of LCS on deeper or longest path that connects LCS with each of its descendant entities in association as it reveals the actual semantic value of LCS. Therefore, in this work, a novel ancestorial level-weight contributing to the association strength is derived and is denoted by component B in Figure 5 and given in equation Eq (5) for ancestor A1 w.r.t Dx.
Therefore, in this work, a novel ancestorial level-weight contributing to the association strength is derived and is denoted by component B in Figure 5 and given in equation Eq (5) for ancestor A1 w.r.t Dx.
LDx(A1)={LDx(Dx)=1LDx(A1)={Δ∗LDx(t)|t∈children(A1)∈New_Anc_Set(Dx)}Δ→weightfactor | (5) |
where Δ is the weight factor of the edge linking A1 with its child t. The weight factor helps reduce the contribution effect of ancestors that are distant from Dx, ranging from 0 to 1 and we found that Δ=0.4 gives better correlation with the standard DDA scores from DisGeNET. Similarly, level-weight of ancestor A2 w.r.t Dy is derived.
Finally, the derived two components in Section 4.2.2 are then multiplied to get the final association representation, CVxy, for Dx−Dy association. With the derived Dx−Dy association vector CVxy, we further vary the association based on the connectedness ontological relationship between DxandDy, using an additional DDA quantification described in the following Section 4.2.3.
Given a disease pair Dx−Dy, whose association can be established through other diseases in the ontology using ancestorial relationship without considering the variation factor is discussed in Section 4.2.2. However, the type of ontological relationship connection between DxandDy, reveals the actual association. Hence, varying the association based on type of the relationship connection, provides a finer adjustment to the already derived association vector CVxy. Therefore, in this work, we proposed an ontological relationship variation factor (ORVF) for the second level of DDA quantification.
As a diagrammatic illustration, ORVF values for different types of ontological relationship connections are shown in Figure 6.
In Figure 6(a), the ORVF is 0 when both DxandDy are at same distances 0.1 or immediate children of D2, considering the edge weight as 0.1. Similarly, in Figure 6(b), the ORVF is 0 as Dx is the direct parent of Dy, with a distance 0.1. Thus, ORVF 0, represents that there is no variation of association when DxandDy are very closely related as a sibling and direct parent-child relationships. However, the variation occurs when DxandDy are distantly related. For example, the ORVF values are calculated for the indirect relationships shown in Figure 6(c) (d) and (e). In Figure 6(c), Dx acts as grandparent of Dy, producing ORVF 0.2 as Dy is at a distance of 0.2 from Dx, while an uncle relationship connection in Figure 6 (d), calculated ORVF of 0.3as an aggregation of distances 0.1 and 0.2 with respect to Dx and Dy respectively, from LCS(Dx, Dy) (D1). On the other hand, in Figure 6(e), Dx acts as a cousin of Dy resulting in ORVF of 0.4 as both Dx and Dy are at distance 0.2 from LCS (Dx, Dy) (D1). Thus, ORVF helps in varying the extent of DD association by each Ds independent distance from LCS.
Algorithm 1 summarizes the procedure of adjusting the stage-1 association vector CVxy by the proposed ORVF is as follows.
Algorithm 1 CVxy adjustment by ORVF |
1: path(Dx)←ordered_New_Anc_Set(Dx), path(Dy)←ordered_New_Anc_Set(Dy), |
2: LCSDk←LeastCommonSubsumer(path(Dx),path(Dy)) |
3: Compute{Level(LCSDk−Dx),Level(LCSDk−Dy)} |
Compute ORVF: Case 1: Direct parent/Siblings 4: If Level(LCSDk−Dx)==Level(LCSDk−Dy)==1 |
5: CV′xy←CVxy, ORVF=0 i.e. No Variation |
Case 2: Broader/Indirect Relationship 6: If (Level(LCSDk−Dx),Level(LCSDk−Dy))≠1 |
7: CV′xy←CVxy∗ORVF,ORVF←[Level(LCSk−Dx)+Level(LCSk−Dy)] |
An illustration of the above algorithm is given in Figure 7(a) and (b) showing the ORVF calculations for sibling and cousin ontological relationships connecting DxandDy respectively.
Figure 7(a) and (b) follows the same procedure to compute ORVF. The first step gives the LCS(Dx,Dy) denoted as LCSk, by defining the path(Dx) and path(Dy) using the new ancestors sets of Dx and Dy respectively, where LCSk is equal to D2 and D1 corresponding to Figure 7(a) and (b). The next step is to find the distance of LCSk from Dx and Dy independently using Level(LCSk−Dx) and Level(LCSk−Dy) and found to be 0.1 for sibling relationship in Figure 7(a) and found to be of different distances 0.1 and 0.2 for cousin relationship in Figure 7(b). Finally, with the calculated distances, the ORVF is computed for direct/sibling relationships in Figure 7(a) and for broader/indirect relationships in Figure 7(b). For direct/sibling relationship, the association embedding is not varied since ORVF is 0 whereas the association embedding is reduced by a factor of 0.3 which is the total distance of variation between DxandDy, through LCSk. Hence, the association embedding CVxy is the final association embedding CV′xy in case of sibling relationship connection in Figure 7(a) whereas CVxy is reduced by a factor of 0.3 contributed by 0.1 and 0.2 from LCSk from DxandDy respectively.
Using CVxy as shown in Figure 4 and the proposed ORVF, we are able to construct an enhanced concept-based DDA matrix of DDA representations CV′xy that is later used for concept-based DDA strength.
Finally, an information rich single disease vector of Dx in Dx−Dy Association, can be obtained as shown in Figure 8, by the following steps. Extracting literature-based Dx vectors, from the constructed literature-based positive, negative DD association matrix of LVxy and concept-based Dx vectors from concept-based DD association matrix of CV′xy as discussed in Sections 4.1.2 and 4.2. Further, the extracted Dx vectors are integrated into single integrated disease vector. As an enhancement to final DDA strength, the integrated single disease vector is enhanced with additional contextual information obtained from literature-based null DD similarity matrix in Section 4.1.2, using vector-similarity fusion method, in order to obtain the final DDA strength.
For Dx−Dy association, literature-based single disease vector (LVy)x of Dx with respect to Dy, is extracted using association vectors obtained from literature-based positive, negative association matrix in Eq (1) by averaging the literature based DDA vectors LVxi′s of Dx−Di associations, where i←{1,2,..,n}andi≠y and finally concatenating the averaged component with association vector LVxy of Dx−Dy association as shown in Eq (6). For Dx−Dy association, it is important to preserve the actual information component of Dy through concatenation while representing Dx vector. Similarly, single disease vector for Dy is extracted. A similar strategy is followed while extracting disease vector for DxandDy from concept-based DDA matrix, where (CVy)x of Dx with respect to Dy is shown in Eq (7).
(LVy)x=LVxy.∑ni=1LVxin,i≠y | (6) |
(CV′y)x=CV′xy.∑ni=1CV′xin,i≠y | (7) |
where: LVxy and CVxy are literature-based and concept-based association vector of Dx−Dy pair.
(LVy)x represents literature-based single disease vector of Dx with respect to Dy. Similarly, (CV′y)x represents concept-based single disease vector of Dx with respect to Dy.
For an information-enriched disease vector representation, the extracted literature-based and concept-based single disease vectors are integrated into a single information rich disease vector. However, for disease vector representation, only a narrow disease-disease linking relations were fused [32,33]. In order to achieve better association, in this work, the disease vector is represented by integrating vector representations on a wide range of disease-disease linking information from both literature and concept-based biomedical data sources.
Thus, for an information-enriched representation of diseases in Dx−Dy association, the extracted literature-based and concept-based disease vector components in Eqs (6) and (7), respectively, are concatenated into a single integrated disease vector (LVCV′y)x for Dx with respect to Dy as in Eq (8).
(LVCV′y)x=(LVy)x.(CV′y)x | (8) |
where (LVCV′y)x represents the single integrated disease vector Dx with respect to Dy. (LVy)x represents literature-based single disease vector of Dx with respect to Dy. (CV′y)x represents concept-based single disease vector of Dx with respect to Dy. Similarly, (LVCV′x)y for Dy with respect to Dx can be defined using Eq (8).
In addition, the information-enriched integrated disease vector is enhanced with additional contextual relationship with all other diseases obtained from literature-based DD null similarity matrix derived earlier in as discussed in Section 4.1.2. Manchanda and Anand [78] enhanced the disease vector representation by updating the initial vector representation using only literature (PubMed) with the corresponding similarity information with all other diseases. Enhancing such a low informative disease vector with similarity is needed to produce a proper enhanced disease vector. Hence, in this work, we use the information-enriched integrated disease vector derived in Eq (8) as an initial vector for similarity updation using vector-similarity fusion method defined in Eq (9), that uses an objective function [rep learning paper], where the scalar component is replaced by the null similarity scores.
Thus, the enhanced integrated vector (LVCV′y)′x for Dx with respect to Dy in Dx−Dy association is obtained from (LVCV′y)x in Eq (8) when updated if the objective function Fx is minimized as shown in Eq (9)
Fx=∑Ni=1[(LVCV′y)x.(LVCV′i)x|(LVCV′y)x||(LVCV′i)x|−LitNullSim(Dx,Di)]2 | (9) |
where (LVCV′y)x represents the integrated disease vector Dx with respect to Dy, LitNullSim(Dx,Di) denotes the literature-based null similarity scores between Dx and Di, |(LVCV′y)x| denote length of vector (LVCV′y)x, Similarly, the enhanced integrated vector (LVCV′x)′y for Dy with respect to Dx in Dx−Dy association is updated when the objective function Fy is minimized.
Thus, a rich integrated and enhanced disease vector representation is derived that helps DDA both contextually and semantically, leading to a better quality of final DDA Strength.
Finally, with the enhanced-integrated disease vector representations obtained in Section 4.3.3, a cosine similarity is applied to obtain the final score measuring the actual strength of association for the given disease pair as shown in Eq (10).
Assoc_Score(Dx,Dy)=cos((LVCV′y)′x,(LVCV′x)′y) | (10) |
where (LVCV′y)′x and (LVCV′x)′y represent enhanced integrated disease vector Dx with respect to Dy and Dy with respect to Dx respectively.
Therefore, in this section, instead of finding the embedding vector for a disease in isolation, we used a modified method similar to Manchanda and Anand [78], in which the disease embedding is discovered in relation with DDA. We used an integration of literature-based and concept-based conceptual and semantic multi-source embeddings and richer ontological embeddings to obtain and discover DD associations and derive their strengths.
For evaluating the enhanced DDA framework, we first evaluate the performance of the proposed association classification model ESEC-CNN with improved sentence representation, which on training facilitated the construction of enhanced DDAE dataset. The classification model was evaluated by measuring the model's classification performance using Precision, Recall and F-measure. The correlation between the association scores obtained from the enhanced literature-based DDA representations and the association metrics Wang et al. [24], Resnik [25], Schlicker et al. [88] and Lin [26] is evaluated using spearman's rank correlation coefficient. Second, the enhanced concept-based DDA representations is evaluated on both established biomedical dataset DisGeNet and human-rated DDA datasets using spearman's rank correlation coefficient. Third, the evaluation of single disease vector representation is carried out using literature and concept-based approaches independently and using the integration of both in a similar manner. Finally, the quantification of DDA pairs obtained using the enhanced single disease vector representation is compared to the state-of-art methods and evaluated in different perspectives of DDA criteria. Additionally, we have also shown the biological effect of the DDA scores derived by integrated and enhanced disease vector representation for mostly associated disease pairs category-wise.
We conducted experiments to show the effect of additional features in sentence representation using classification performance of various sentence classification models in Table 1 and also in Figure 9. DDA classification performance of the baseline models without (limited local and global-level features) and with (additional local and global-level features) improved sentence representation such as, LSTM [49], BiLSTM [89], CNN [90], BERT [91], BioBERT [92] and LC-CNN [17] are then evaluated on the available annotated DDA dataset, on a 5-fold cross validation. Implementation is carried out on a TensorFlow with hyperparameters of learning rate as 0.025, batch size of 8, epochs of 5, 10, 15 and layer size of 352.
Methods | Without improved sentence representation | With improved sentence representation | ||||
Performance measure | ||||||
Precision (%) |
Recall (%) |
F-measure (%) |
Precision (%) |
Recall (%) |
F-measure (%) |
|
LSTM [49] | 65.13 | 67.11 | 66.11 | 66.92 | 68.37 | 67.64 |
BiLSTM [89] | 64.88 | 66.15 | 65.51 | 65.15 | 68.02 | 66.55 |
CNN [90] | 74.13 | 71.27 | 72.67 | 75.20 | 72.64 | 73.90 |
BERT [91] | 78.65 | 80.32 | 79.48 | 80.63 | 82.12 | 81.37 |
BioBERT [92] | 81.54 | 82.01 | 81.77 | 82.69 | 83.88 | 83.28 |
LC-CNN [17] | 82.16 | 84.89 | 83.50 | − | − | − |
ESEC-CNN* | − | − | − | 83.06 | 86.54 | 84.76 |
ESEC-CNN** | − | − | − | 84.03 | 87.12 | 85.54 |
*- partial improved sentence representation{(PubMed, PMC, Wiki, News), (POS, NE Dist)} **- improved sentence representation {(PubMed, PMC, Wiki, News), (POS, position, Dep. Rel., Chunk, NE)} |
On comparing with all classification models, CNN-based models are found to perform better as LSTM, BiLSTM are sequence-based and hence, CNN-based model shows better sentence classification performance.
The LC-CNN model with additional news embedding feature (global-level) has shown only less improvement of F-measure than that of LC-CNN with limited features. With the combined additional local-level embeddings of NDE, dependency relation, chunk tag along with other global-level embeddings including news, ESEC-CNN model (LC-CNN model with improved sentence representation) outperformed the other baseline models including LC-CNN model without improved sentence representation with F-measure of 85.54%.
A notable observation of F-measure in other baseline models show that models have achieved better F-measure when the sentence representation is improved with additional local and global level features. Hence, the effect of improved sentence representation has a major positive effect on other models also.
The better performing ESEC-CNN model (LC-CNN with improved sentence representation) is further utilized for DDA dataset expansion, where the size of the labelled PubMed abstracts is increased using an initial 213 seed DD pairs obtained from a combined benchmark similarity dataset as discussed in Section 4.1.2.
From PubTator, a set of abstracts are downloaded in BioCXML format from https://ftp.ncbi.nlm.nih.gov/pub/lu/PubTatorCentral/PubTatorCentral_BioCXML/BioCXML.9.tar (accessed 12 July 2022), ensuring only abstracts that contain sentences with the given DD pairs are retrieved. At each iteration, a new unique set of DD pairs are produced from the retrieved set of abstracts. The number of newly produced DD pairs are found to increase at the initial few iterations and the drop in the count of new DD pairs acts as a stopping criterion for the abstracts retrieval process. With the retrieved 39,510 abstracts, a total of 58,980 unique DD pairs are identified. However, for the construction of increased DDA extraction (DDAE) dataset, the LC-CNN model with improved sentence representation is trained on the available labelled abstracts [17] and then applied on to the created dataset. The trained model is able to identify a large number positive, negative and null pairs with only a minimum number of seed pairs. A statistical comparison of the enhanced constructed DDA extraction (DDAE) dataset starting with the available 521 labelled DDAE dataset [17] is tabulated in Table 2.
Details | Available 521 labelled DDAE dataset [17] | Constructed DDAE dataset |
Abstracts | 521 | 39,510 |
Unique Ds | 1103 | 14,598 |
Unique DD pairs | 3600 | 28,980 |
Unique Positive DD pairs | 1626 | 34,481 |
Unique Negative DD pairs | 124 | 5488 |
unique Null DD pairs | 2649 | 36102 |
Unique Positive-Negative DD pairs | 53 | 3254 |
Unique Positive-Negative-Null DD pairs | 33 | 2589 |
DD pairs classified by ESEC-CNN model are of 3 types, namely, both positively and negatively associated, only negatively associated and null associated and their association scores are validated as discussed earlier in this section and the evaluation of the 3 types is shown in Tables 3–5 respectively. The association measures are calculated using DOSim package [5]. Further, the concordance of the classified DD pairs scores with each of the association metrics is evaluated on both 521 DDA labelled abstracts [17] and the constructed DDAE dataset.
Association Type | Method | Wang et al. [24] | Resnik [25] | Schlicker et al. [88] | Lin [26] |
#positively and negatively associated DD pairs = 54 [available 521 labelled dataset [17]] | GloVe-50 [93] | 0.001 | 0.007 | 0.015 | 0.015 |
SicknessMiner [47] | 0.277131 | 0.203487 | 0.340086 | 0.340086 | |
GexText [18] | 0.3982 | 0.3884 | 0.394 | 0.4013 | |
Enhanced literature-based positive, negative DDA representation | 0.402411 | 0.417671 | 0.531724 | 0.531724 | |
#positively and negatively associated DD pairs= 3254 [Enhanced DDA dataset] |
GloVe-50 [93] | 0.005 | 0.009 | 0.024 | 0.026 |
SicknessMiner [47] | 0.323 | 0.321 | 0.352 | 0.349 | |
GexText [18] | 0.468 | 0.463 | 0.476 | 0.476 | |
Enhanced literature-based positive, negative DDA representation | 0.515 | 0.521 | 0.575 | 0.573 |
Association Type | Method | Wang et al. [24] | Resnik [25] | Schlicker et al. [88] | Lin [26] |
#Only negatively associated DD pairs = 70 [available 521 labelled dataset [17]] | GloVe-50 [93] | −0.356 | −0.245 | −0.319 | −0.319 |
SicknessMiner [47] | −0.22114 | −0.18152 | −0.22987 | −0.22987 | |
GexText [18] | −0.008 | −0.125 | −0.012 | −0.011 | |
Enhanced literature-based positive, negative DDA | 0.229156 | 0.208198 | 0.138969 | 0.138969 | |
#Only negatively associated DD pairs= 2234 [Enhanced DDA dataset] |
GloVe-50 [93] | −0.234 | −0.301 | −0.286 | −0.284 |
SicknessMiner [47] | −0.229 | −0.298 | −0.251 | −0.253 | |
GexText [18] | −0.191 | −0.226 | −0.218 | −0.218 | |
Enhanced literature-based positive, negative DDA | 0.306 | 0.299 | 0.274 | 0.269 |
Association Type | Method | Wang et al. [24] | Resnik [25] | Schlicker et al. [88] | Lin [26] |
#Null associated DD pairs = 2649 [available 521 labelled dataset [17]] | GloVe-50 [93] | 0.07 | 0.018 | 0.0195 | 0.03 |
SicknessMiner [47] | −0.0436 | −0.009 | 0.003586 | 0.002185 | |
GexText [18] | 0.231 | 0.236 | 0.196 | 0.193 | |
Literature-based Null DD similarity | 0.333 | 0.307 | 0.281 | 0.281 | |
#Null associated DD pairs= 36102 [Enhanced DDA dataset] |
GloVe-50 [93] | 0.006 | 0.005 | 0.002 | 0.003 |
SicknessMiner [47] | 0.024 | 0.017 | 0.0052 | 0.00549 | |
GexText [18] | 0.168 | 0.186 | 0.210 | 0.208 | |
Literature-based Null DD similarity | 0.423 | 0.419 | 0.454 | 0.456 |
For both positively and negatively associated DD pairs, as shown in Table 3, [47] and [18] derived DDA strength which are less correlated with all metrics when evaluated on both datasets with a count of 54 and 3254 DD pairs. The lower correlation is because, Sicknessminer considered the number of co-mentions ignoring the context and treated all co-mentioned pairs as equally contributing to DD association, while Gextext considered a direct positive association if the DD pair had an average occurrence in the whole corpus, thus missing out the negative context of the pairs. Hence, considering negative context for association quantification will balance the real context by which disease pairs are associated. Further, such consideration could lead to significant correlation achieved by association scores computed using literature-based positive, negative association matrix.
In case of only negatively associated DD pairs as shown in Table 4, a total of 70 and 2234 DD pairs were found from the available and enhanced DDAE dataset, respectively, where their derived scores from the literature-based DDA matrix are positively correlated while other literature-based scores are negatively correlated indicating that considering the context of DD pairs occurrence plays a crucial role rather than taking only their occurrence frequency as in other methods.
Similarly, the associations discovered for 2649 (521 abstracts) and 36102 (enhanced dataset) null pairs from literature-based null similarity matrix, have also correlated better when compared to other methods shown in Table 5, as only few pairs co-occur and therefore Sicknessminer [47] which used the co-mention analysis for association, is less correlated. While GexText [18], resulted in strong association for DD pairs with higher occurrence in the corpus which may not be strongly associated and hence less correlated compared to our null similarity scores, as the null scores obtained, considered the surrounding context influencing the disease in the given pair. On the other hand, GloVe [93] generated less informative embeddings for association calculation and therefore less correlated in all the above cases.
To characterize the concept-based DDA, the derived association embedding consisting of several components such as discovered new ancestors sets, mutli-source ancestorial embedding with root and leaf node, novel ancestorial level-based DDA quantification and finally, the proposed ontology-based joint multi-source association representation with the ontological relationship connections is evaluated with the association scores from DisGeNET and the human assessed combined dataset as discussed in Section 3.4.
For evaluating the concept embeddings represented using newly defined ancestors sets, the ontological sources such as the clinical classifications software for ICD-9-CM (diagnosis) from https://www.hcup-us.ahrq.gov/toolssoftware/ccs/ccs.jsp (accessed 2015) and anatomical therapeutic chemical classification system (ATC) (medications) https://www.whocc.no/atc/ (accessed 2 February 2018) are used.
Table 6 shows the correlation effect of varying combinations of ancestors sets for DxandDy in Dx-Dy association quantification. With diseases in ontology, 7936 DD pairs found in common with DisGeNET, the embeddings derived with discovered new ancestors sets are better correlated compared to embeddings with all ancestors [86]. While considering only common ancestors without ancestors on longest path to DxandDy independently, shows good correlation than all ancestors but still less correlated when compared with the new ancestors sets. Since, association is not only influenced by commonality but also by ancestors on the longest path to each of the disease.
Source of multiple embeddings of ancestor | Ancestors' information | Spearman's rank correlation N=7936 DD pairs (DisGeNET) |
Ontology Sources- CCS, ATC: • Clinical Classifications Software for ICD- 9-CM (CCS) (diagnosis) • Anatomical Therapeutic Chemical classification system (ATC) (medications) [86] |
All Ancestors of DxandDy:∀Axand∀Ay [86] | 0.759 |
Common Ancestors of DxandDy:∀Ax∩∀AyAy | 0.772 | |
New Ancestors Sets of DxandDy:New_Anc_Set(Dx) and New_Anc_Set(Dy) | 0.779 |
With the best correlated newly defined ancestors sets and with all ancestors, the concept embeddings are further evaluated to show the effect of multi-source embeddings of those ancestors with and without including multi-source information of root and leaf nodes. In this regard, the concept embeddings are evaluated as shown in Table 7 for 2658 DD pairs. We observed that the concept embeddings using ancestorial embeddings from multiple conceptual sources including root node and leaf node multiple embeddings in addition to the new ancestors sets gives significantly higher correlation compared to the baseline that considers only semantic sources [86].
Sources of multiple embeddings of ancestor | Different combination of ancestors sets for Dx-Dy quantification | Without Root and leaf node multi-source embeddings | With Root and leaf node multi-source embeddings |
Spearman's rank correlation N = 2658 DD pairs (DisGeNET) |
|||
Ontology Sources: • Clinical Classifications Software for ICD- 9-CM (CCS) and Anatomical Therapeutic Chemical classification system (ATC) [86] |
∀Axand∀Ay [86] | 0.612 | 0.618 |
New_Anc_Set(Dx) and New_Anc_Set(Dy) | 0.643 | 0.695 | |
Ontology Sources • Disease Ontology (DO) • UMLS Biomedical Text • Clinical Notes • Insurance Claims Database • Journal Articles |
∀Axand∀Ay [86] | 0.726 | 0.730 |
New_Anc_Set(Dx) and New_Anc_Set(Dy) | 0.745 | 0.788 |
In order to evaluate the effect of level-weight or semantic value of LCS (Dx, Dy) in Dx-Dy association, we compared the level-weight of LCS computed by longest path of lower DAG with respect to Dx and Dy separately using the proposed novel level-weight and the upper DAG using Baseline_LCA in GOntoSim [65] as shown in Table 8. The results show that the DDA quantification by level-weight of LCS using lower part of DAG connecting Dx and Dy is better correlated with DisGeNET DDA scores than level-weight of LCS using upper part of DAG.
Calculation of level-weight of LCS(Dx, Dy) for Dx-Dy quantification | Spearman's rank correlation N = 1, 75,939 DD pairs (DisGeNET) |
Baseline_LCA of GOntoSim: using upper DAG Level-weight of LCS(Dx,Dy) by ancestors on longest path to LCS(Dx,Dy) [65] |
0.773 |
Novel ancestorial-level weight: using lower DAG Level-weight of LCS(Dx,Dy) by children on longest path to Dx and Dy |
0.782 |
To demonstrate the effectiveness of adding novel level-weight to the multi-source ancestorial embeddings, we first introduce the effect of varying level-weight calculations of ancestors including LCS based on selection of children and then evaluated the effect of various combinations of level-weight with and without multi-source ancestorial embeddings. As shown in Table 9, with 1, 75,939 DD pairs, the novel level weight, where the level-weight is contributed by the children that belongs only to the newly defined ancestors sets, even without multi-source ancestorial embeddings outperformed the baseline level-weight calculation [63]. In addition, the correlation is even better when the novel level-weight is applied on multi-source ancestorial embeddings.
Method | Without multi-source ancestorial embedding | With multi-source ancestorial embedding |
Spearman's rank correlation N = 1, 75,939 DD pairs (DisGeNET) | ||
Semantic similarity of diseases [63] | 0.610 | 0.720 |
Baseline_LCA of GOntoSim [65] | 0.612 | 0.723 |
Novel ancestorial level-weight | 0.619 | 0.756 |
In order to showcase the effect of the proposed ORVF, different combinations of the relationship connections as discussed earlier in Section 4.2.3. are considered. The performance of the effect of various ontological relationships is then evaluated through DDA quantification on DDA dataset as shown in Table 10.
Different concept-based representation methods for concept-based DDA quantification | Spearman's rank correlation N = 1756 DD pairs (DisGeNET) |
MMORE (CCS (diagnosis), ATC (medications)) [86] | 0.703 |
Cui2vec (Clinical Notes, Claims Insurance, Journal articles) [20] | 0.772 |
Retrofitted concept vector representation (PubMed, UMLS) [33] | 0.781 |
Proposed Ontology-based joint multi-source association representation | |
Ancestorial level-based + ontological relationship connection based-Parent, Grandparents only* | 0.787 |
Ancestorial level-based + ontological relationship connection based-Parent, Grandparents & sibling only** | 0.790 |
Ancestorial level-based + ontological relationship connection based-Parent, Grandparents, sibling, uncle & cousin relationships*** | 0.802 |
Further, the proposed ontology-based joint multi-source association representation is evaluated against the state-of-art concept representation methods to project the effect of varying the ontological relationship connection of the given disease pair applied on to the association embedding derived by combining all the better performed components inferred from the sub-experiments as discussed earlier and is shown in Table 10 for 1756 DD pairs. The proposed model considering all subcomponents such as discovered new ancestors sets, multi-source ancestorial embedding with root and leaf node, novel ancestorial level-based DDA quantification and the ontological relationship connections is strongly correlated than other existing methods, because [86] considered only semantic ancestorial embeddings without level weight on all ancestors, is less correlated compared to other methods that considered other contextual and semantic type relations.
The analysis presented so far shows the effectiveness of literature-based DDA and concept-based DDA. However, we need to evaluate integrated literature and concept based DDA representation. This requires representing each disease as a single disease vector representation, integrating literature-based and concept-based methods. This enhanced single vector representation of two diseases is then used to compute the DD association using cosine similarity. In order to show the effect of integrated disease representation, the association scores computed is compared with the other state-of-art methods using only literature-based, only concept-based and those with integrated literature-based and concept-based perspectives.
The disease representations produced by the models is evaluated across different perspectives of datasets. On the basis of type of DDA criteria, various angles of the datasets are used to evaluate the scores obtained by the generated disease representations. In this regard, we relied on disease-related biological domain database DisGeNet, where two association criteria were used to derive DDA scores. One is the disease-associated genes and other is disease-associated variants. Further, the Jaccard index similarity is used to compute association scores. In addition, we created a standard dataset covering the functional aspects of DDA using GO function. The disease-related GOs are obtained from Comparative Toxicogenomics Database (CTD). In order to calculate the DDA score in GO perspective, we employed the Jaccard index. Finally, we also evaluated against the human rated DD pairs obtained from a benchmark dataset. Details of the datasets used is discussed earlier in Section 3.4.
Table 11 summarizes the results of correlation of DDA scores obtained by different methods across various aspects of datasets. The DDA scores derived using only literature-based disease representation, shows better correlation than other literature-based method for DDA quantification in case of Gene-based, GO-based and human-rated scores. The reason may be that considering different context types in which DD pairs occur has a major influence on DDA scores as the additional features during the sentence representation learning can lead to better classified contexts. While, the correlation result on Variant-based dataset, is found to be less as the PubMed abstracts taken may not contain sentences that reveal much about variant related information or only limited contexts since we consider only disease mentioned sentences.
Disease Vector Representation | Spearman's Rank Correlation | |||
Literature-based only | DisGeNet Gene-based | DisGeNet Variant-based | Standard dataset GO-based | Human-rated |
N = 2938 DD pairs (DisGeNET) | N = 199 DD pairs | |||
Cui2vec (Clinical Notes, Claims Insurance, Journal articles) [20] | 0.797 | 0.254 | 0.422 | 0.679 |
Enhanced Literature-Based Disease Vector Representation (Vector-Similarity Fusion Without Integration) | 0.799 | 0.252 | 0.427 | 0.682 |
Concept-based only | DisGeNet Gene-based | DisGeNet Variant-based | Standard dataset GO-based | Human-rated |
N = 2638 DD pairs | N = 50 DD pairs | |||
MMORE (CCS (diagnosis), ATC (medications)) [86] | 0.716 | 0.144 | 0.541 | 0.790 |
Ontology-Based Joint Multi-Source Association Embedding (Ancestral Level-Based + Ontological Relationship Connection Based) | 0.808 | 0.146 | 0.551 | 0.809 |
Integration of literature-based and concept-based | DisGeNet Gene-based | DisGeNet Variant-based | Standard dataset GO-based | Human-rated |
N = 1638 DD pairs | N = 187 DD pairs | |||
Retrofitted Concept Vector Representation (PubMed, UMLS) [33] | 0.801 | 0.213 | 0.592 | 0.810 |
Integration of literature-based and concept-based | DisGeNet Gene-based | DisGeNet Variant-based | Standard dataset GO-based | Human-rated |
N = 1638 DD pairs | N = 187 DD pairs | |||
MORE [32] | 0.811 | 0.220 | 0.609 | 0.813 |
Integrated Disease Vector Representation (Literature-Based Positive, Negative & Concept-Based DDA) | 0.816 | 0.225 | 0.624 | 0.818 |
Enhanced Literature-Based & Concept-Based Joint Embedding Model for Disease Vector Representation (Vector-Similarity Fusion with Integration) | 0.822 | 0.227 | 0.626 | 0.821 |
The DDA scores derived using only concept-based representation, found to have better correlation on all aspects of the datasets with only a slightly higher on variant-based. The proposed ontology-based method tries to embed a narrow information of concepts in ontology rather than generic concepts. This is achieved by controlling the contribution of ancestors on DDA in addition to varying the effect of different taxonomic relationships in ontology. Moreover, we select ancestors with respect to DDA rather than independently with respect to each of the diseases. All these has a major positive effect on DDA scores in different aspects.
On evaluation with the integrated approaches, the proposed method outperforms well compared to other baseline methods on all aspects of datasets. Integrating the enhanced literature-based contextual relations with enriched semantic relationships gives a broader coverage of relationships that might cover various influential factors affecting DDA. This basically includes indirect relationship information that can jointly eliminate false positives. Hence, the proposed work has shown promising results even for different aspects of DDA.
The configurations of the machine include Intel(R) Xenon(R) 3.60 GHz (GPU), 64-bit OS (system) and 64 GB RAM (memory). Our system uses Python to implement the models. For literature-based DDA classification as discussed in Section 5.1.1, Table 12 shows the time taken by the baseline models and the proposed model for training and prediction tasks. On observation, we found that CNN models take less training time compared with other models since it involves less parameters calculation. However, LC-CNN and the proposed ESEC-CNN models, take almost equal time since only additional features have been added in the input sentence representation in ESEC-CNN model.
Literature-based DDA sentence classification models | LSTM [21] | BiLSTM [89] | CNN [90] | BERT [91] | BioBERT [92] | LC-CNN [17] | ESEC-CNN** |
Training time per epoch (in seconds) | 240s | 237s | 184s | 262s | 270s | 196s | 200s |
Concept-based DDA representation | Retrofitted concept vector representation [33] | Cui2vec [20] | MMORE [86] | Ontology-based joint multi-source association representation | N/A | N/A | N/A |
Average seconds to generate concept-based DDA representation | 15s | 16s | 19s | 22s | N/A | N/A | N/A |
N/A-represents not applicable |
For concept-based DDA representation as discussed in Section 5.2.4, the proposed ontology-based joint multi-source embedding representation takes on an average of 22 seconds to derive DDA representation which is higher compared to other models. This arises from calculating different ancestors' information as discussed in earlier sections such as level weight, attention weights as well as the various ontological relationships to generate final representation of DDA. Other concept-based base-line models such as Cui2vec [20], Retrofitted concept vector representation [33] takes less time than MMORE [86] and the proposed model, as the former does not consider the deeper ancestors' information and ontological relationships. Compared with MMORE, the proposed model takes much more time since additional computation of ancestorial level weights and ontological relationships effect are involved. Though the proposed model, takes some time to obtain DDA representation, it is still able to produce quality embedding whose effectiveness is proved by the correlated results in Table 10.
The significance of DDA scores obtained by the proposed framework is analysed in biological aspects: listing top 20 associated disease-disease pairs with normalized scores in Table 13, disease-wise most associated diseases in Table 13, top 5 category-wise associations and also the top 10 associated diseases with corresponding categories for a given disease.
Disease 1 | Disease 2 | Association score |
amyotrophic lateral sclerosis | motor neuron disease | 0.999998 |
Hypertensive retinopathy | Vascular disease | 0.096729 |
cardiovascular disease | intrinsic asthma | 0.043286 |
autosomal dominant polycystic kidney disease | autosomal dominant polycystic kidney disease | 0.033808 |
myopathy | Sjogren's syndrome | 0.022195 |
congenital muscular dystrophy | muscular dystrophy | 0.010661 |
cerebral folate receptor alpha deficiency | Down syndrome | 0.007642 |
muscular dystrophy | myotonic dystrophy type2 | 0.006518 |
Alzheimer's disease | Moyamoya disease | 0.004409 |
migraine without aura | Fibromyalgia | 0.003855 |
diabetes mellitus | diabetic neuropathy | 0.003613 |
diabetes mellitus | Hypoglycemia | 0.003495 |
acute myeloid leukemia | acute monocytic leukemia | 0.003449 |
lepromatous leprosy | Leprosy | 0.002793 |
marasmus | anorexia nervosa | 0.002623 |
lymphoblastic leukemia | lung disease | 0.002265 |
cystic fibrosis | acute pancreatitis | 0.002053 |
acute monocytic leukemia | acute leukemia | 0.001959 |
Azoospermia | oligospermia | 0.001876 |
acute myeloid leukemia | acute leukemia | 0.001875 |
For a given disease, Table 14 shows the most associated disease pairs comparatively to others.
Disease | Most associated diseases |
amyotrophic lateral sclerosis | Motor neuron disease, lateral sclerosis |
motor neuron disease | Motor neuron disease, cardiovascular disease |
Hypertensive retinopathy | Vascular disease |
Vascular disease | Hypertensive retinopathy, cardiovascular disease |
Intrinsic asthma | Cardiovascular disease, lung disease |
autosomal dominant polycystic kidney disease | autosomal recessive polycystic kidney disease |
Myopathy | Sjogren's syndrome |
Sjogren's syndrome | Myopathy, systemic scleroderma, Behcet's disease, systemic lupus erythematosus |
The performance of disease representation in DDA quantification is further validated by disease categories, where the diseases are classified according to top 14-level DO categories such as "disease of cellular proliferation", "nervous system disease", "cardiovascular system disease", "musculoskeletal system disease", "endocrine system disease" and so on [72]. The strength of association between disease categories is measured by averaging the normalized DDA scores between disease categories. The disease category pairs are ranked based on the normalized score.
We find that disease associated within same category have high average association score than with diseases of other categories as shown in Table 15. On observation, diseases in "nervous system disease" category have relatively higher association scores across all other disease categories. On the other hand, we find that average association scores of diseases in "disease by infectious agent", "endocrine system disease", "urinary system disease" have lower association scores with all other categories compared to diseases within itself. In case of "nervous system disease" category, is comparatively higher within and with "cardiovascular system disease" and "musculoskeletal system disease". While the average association score of diseases in "disease of cellular proliferation", are far lower with diseases in "endocrine system disease" and "cardiovascular system disease" than for other categories.
DO Disease Category | Top 5 associated DO Disease Categories | Normalized average score of disease pairs category-wise |
Nervous system disease | Nervous system disease | 0.0223 |
Cardiovascular system disease | 0.0221 | |
Musculoskeletal system disease | 0.0200 | |
Physical disorder | 0.0085 | |
Disease of metabolism | 0.0025 | |
Physical disorder | Physical disorder | 0.014 |
Nervous system disease | 0.0085 | |
Disease of metabolism | 0.0052 | |
Disease of cellular proliferation | 0.0038 | |
Endocrine system disease | 0.00056 | |
Disease of cellular proliferation | Disease of cellular proliferation | 0.00400 |
Respiratory system disease | 0.00382 | |
Physical disorder | 0.00380 | |
Endocrine system disease | 0.000620 | |
Cardiovascular system disease | 0.000621 | |
Urinary system disease | Urinary system disease | 0.00109 |
Disease of cellular proliferation | 0.00062 | |
Endocrine system disease | 0.00059 | |
Gastrointestinal system disease | 0.00054 | |
Disease by infectious agent | 0.00048 | |
Endocrine system disease | Endocrine system disease | 0.000920 |
Disease of cellular proliferation | 0.000626 | |
Urinary system disease | 0.000596 | |
Musculoskeletal system disease | 0.000580 | |
Cardiovascular system disease | 0.000564 | |
Disease by infectious agent | Disease by infectious agent | 0.00072 |
Endocrine system disease | 0.000486 | |
Nervous system disease | 0.000485 | |
Respiratory system disease | 0.000482 | |
Gastrointestinal system disease | 0.000475 |
In addition, we have also shown the category-wise top 10 associated disease pairs for "Diabetes mellitus" of "endocrine system disease" and "cardiovascular disease" of "Cardiovascular system disease" in table 16.
Disease with category | Top 10 associated disease pairs | Category of associated disease pair | Association score |
Diabetes mellitus Endocrine system disease |
diabetic neuropathy | endocrine system disease | 0.003613 |
hypoglycaemia | endocrine system disease | 0.003495 | |
acute myocardial infarction | cardiovascular system disease | 0.000944 | |
diabetic retinopathy | nervous system disease | 0.000938 | |
stomach cancer | disease of cellular proliferations | 0.000918 | |
kidney failure | urinary system disease | 0.000728 | |
Hypothyroidism | endocrine system disease | 0.000649 | |
disease of metabolism | disease of metabolism | 0.000627 | |
autoimmune disease | musculoskeletal system disease | 0.000603 | |
brain cancer | disease of cellular proliferations | 0.000567 | |
Cardiovascular disease Cardiovascular system disease |
intrinsic asthma | respiratory system disease | 0.043286 |
nephrotic syndrome | urinary system disease | 0.001028 | |
vascular disease | cardiovascular system disease | 0.000399 | |
disease by infectious agent | parasetic infectious disease | 0.000376 | |
vein disease | cardiovascular system disease | 0.000359 | |
generalized atherosclerosis | cardiovascular system disease | 0.000353 | |
Moyamoya disease | cardiovascular system disease | 0.000351 | |
peripheral artery disease | cardiovascular sys. disease | 0.000347 | |
Epilepsy | nervous system disease | 0.000331 | |
intermediate coronary syndrome | cardiovascular system disease | 0.000320 |
Representing a richer quality of disease vectors for a qualitative and quantitative measurement of DDA strength provides valuable information to the clinicians for better healthcare planning. The existing methods of integrated vector representation failed to consider various sentence contexts from literature and semantic embedding of concepts along with different ontological relationship connections from ontology for better quantification of biomedical associations. To address this issue, in this paper, we presented an enhanced and integrated DDA framework incorporating various types of sentence contexts such as positive, negative and null from literature with semantically embedded concepts and various ontological relationship connections affecting associations from ontology for a richer quality of disease vector representation. The enriched disease vectors achieved well correlated DDA scores especially on gene-based when evaluated in different aspects of datasets compared to other baseline literature-based, concept-based and integrated representations. Moreover, we also shown the top associated disease pairs and category-pairs. Any biomedical association quantification using biomedical entities representations could greatly be benefited from a richer vector representation using the enhanced and integrated framework. In future, the integrated representation can also be carried out for determining the strength of other biomedical associations such as disease-gene, gene-gene, disease-symptoms etc.
We would like to thank all biomedical data sources for data supporting.
The authors declare that there is no conflict of interest.
[1] | Begam M, Sengupta M (2015) Immunomodulation of intestinal macrophages by mercury involves oxidative damage and rise of pro-in flammatory cytokine release in the fresh water fish Channa punctatus Bloch. Fish Shellfish Immunol 45: 378-385. |
[2] | Clarkson TW, Magos L (2006) The toxicology of mercury and its chemical compounds. Crit Rev Toxicol 36: 609-662. |
[3] | Cossins AR, Crawford DL (2005) Fish as models for environmental genomics. Nat Rev Genet 6: 324-333. |
[4] | Rice KM, Walker EM, Miaozong W, et al. (2014) Environmental mercury and its toxic effects. J Prev Med Public Health 47: 74-83. |
[5] | Serra-Majem L, Román-Viñas B, Salvador G, et al. (2007) Knowledge, opinions and behaviours related to food and nutrition in Catalonia, Spain (1992–2003). Public Health Nutr 10: 1396-1405. |
[6] | EPA, Basic information about mercury. US EPA, 2016. Available from: https://www.epa.gov/mercury/basic-information-about-mercury |
[7] | Cuesta A, Meseguer J, Esteban MA (2011) Immunotoxicological effects of environmental contaminants in teleost fish reared for aquaculture, In: Stoytcheva M, Pesticides in the Modern World-Risks and Benefits, Rijeka, Croatia: Intech, 241-266. |
[8] | Erickson RJ, Nichols JW, Cook PM, et al. (2008) Bioavailability of chemical contaminants in aquatic systems, In: Di Giulio RT, Hinton DE, The Toxicology of Fishes, Florida, USA: CRC Press, 9-45. |
[9] | Sweet LI, Zelikoff JT (2001) Toxicology and immunotoxicology of mercury : a comparative review in fish and humans. J Toxicol Environ Heatlth B 4: 161-205. |
[10] | Aschner M, Onishchenko N, Ceccatelli S (2010) Toxicology of alkylmercury compounds, In: Sigel A, Sigel H, Sigel RKO, Organometallics in Environment and Toxicology, Cambridge, UK: RSC Publishing, 403-434. |
[11] | Kerper LE, Ballatori N, Clarkson TW (1992) Methylmercury transport across the blood-brain barrier by an amino acid carrier. Am J Physiol 262: 761-765. |
[12] | Ebany JMF, Chakraborty S, Fretham SJB, et al. (2012) Cellular transport and homeostasis of essential and nonessential metals. Metallomics 4: 593-605. |
[13] | Giblin FJ, Massaro EJ (1975) The erythrocyte transport and transfer of methylmercury to the tissues of the rainbow trout (Salmo gairdneri). Toxicology 5: 243-254. |
[14] | Farina M, Aschner M, Rocha JBT (2011) Oxidative stress in MeHg-induced neurotoxicity. Toxicol Appl Pharmacol 256: 405-417. |
[15] | Clarkson TW, Magos L, Myers GJ (2003) The toxicology of mercury-current exposures and clinical manifestations. N Engl J Med 349: 1731-1737. |
[16] | Mieiro CL, Ahmad I, Pereira ME, et al. (2010) Antioxidant system breakdown in brain of feral golden grey mullet (Liza aurata) as an effect of mercury exposure. Ecotoxicology 19: 1034-1045. |
[17] | Monteiro DA, Rantin FT, Kalinin AL (2013) Dietary intake of inorganic mercury: bioaccumulation and oxidative stress parameters in the neotropical fish Hoplias malabaricus. Ecotoxicology 22: 446-456. |
[18] | Guardiola FA, Chaves-Pozo E, Espinosa C, et al. (2016) Mercury accumulation, structural damages, and antioxidant and immune status changes in the gilthead seabream (Sparus aurata L.) exposed to methylmercury. Arch Environ Contam Toxicol 70: 734-746. |
[19] | Brandão F, Cappello T, Raimundo J, et al. (2015) Unravelling the mechanisms of mercury hepatotoxicity in wild fish (Liza aurata) through a triad approach: bioaccumulation, metabolomic profiles and oxidative stress. Metallomics 7: 1352-1363. |
[20] | Cappello T, Brandão F, Guilherme S, et al. (2016) Insights into the mechanisms underlying mercury-induced oxidative stress in gills of wild fish (Liza aurata) combining 1H NMR metabolomics and conventional biochemical assays. Sci Total Environ 548-549: 13-24. |
[21] | Cappello T, Pereira P, Maisano M, et al. (2016) Advances in understanding the mechanisms of mercury toxicity in wild golden grey mullet (Liza aurata) by 1H NMR-based metabolomics. Environ Pollut 219: 139-148. |
[22] | Sarmento A, Guilhermino L, Afonso A (2004) Mercury chloride effects on the function and cellular integrity of sea bass (Dicentrarchus labrax) head kidney macrophages. Fish Shellfish Immunol 17: 489-498. |
[23] | Voccia I, Krzystyniak K, Dunier M, et al. (1994) In vitro mercury-related cytotoxicity and functional impairment of the immune cells of rainbow trout (Oncorhynchus mykiss). Aquat Toxicol 29: 37-48. |
[24] | Morcillo P, Chaves-Pozo E, Meseguer J, et al. (2017) Establishment of a new teleost brain cell line (DLB-1) from the European sea bass and its use to study metal toxicology. Toxicol In Vitro 38: 91-100. |
[25] | Morcillo P, Cordero H, Meseguer J, et al. (2015) Toxicological in vitro effects of heavy metals on gilthead seabream (Sparus aurata L.) head-kidney leucocytes. Toxicol In Vitro 30: 412-420. |
[26] | Morcillo P, Esteban MA, Cuesta A (2016) Heavy metals produce toxicity, oxidative stress and apoptosis in the marine teleost fish SAF-1 cell line. Chemosphere 144: 225-233. |
[27] | Elia AC, Galarini R, Taticchi MI, et al. (2003) Antioxidant responses and bioaccumulation in Ictalurus melas under mercury exposure. Ecotoxicol Environ Saf 55: 162-167. |
[28] | Rana SVS, Singh R, Verma S (1995) Mercury-induced lipid peroxidation in the liver, kidney, brain and gills of a fresh water fish Channa punctatus. Jpn J Ichthyol 42: 255-259. |
[29] | Branco V, Canario J, Lu J, et al. (2012) Mercury and selenium interaction in vivo: effects on thioredoxin reductase and glutathione peroxidase. Free Radic Biol Med 52: 781-793. |
[30] | Mela M, Neto FF, Yamamoto FY, et al. (2014) Mercury distribution in target organs and biochemical responses after subchronic and trophic exposure to Neotropical fish Hoplias malabaricus. Fish Physiol Biochem 40: 245-256. |
[31] | Monteiro DA, Rantin FT, Kalinin AL (2010) Inorganic mercury exposure: toxicological effects, oxidative stress biomarkers and bioaccumulation in the tropical freshwater fish matrinxã, Brycon amazonicus (Spix and Agassiz, 1829). Ecotoxicology 19: 105-23. |
[32] | Morcillo P, Cordero H, Meseguer J, et al. (2015) In vitro immunotoxicological effects of heavy metals on European sea bass (Dicentrarchus labrax L.) head-kidney leucocytes. Fish Shellfish Immunol 47: 245-254. |
[33] | Morcillo P, Meseguer J, Esteban MA, et al. (2016) In vitro effects of metals on isolated head-kidney and blood leucocytes of the teleost fish Sparus aurata L. and Dicentrarchus labrax L. head-kidney leucocytes. Fish Shellfish Immunol 54: 77-85. |
[34] | Morcillo P, Romero D, Meseguer J, et al. (2016) Cytotoxicity and alterations at transcriptional level caused by metals on fish erythrocytes in vitro. Environ Sci Pollut Res 23: 12312-12322. |
[35] | Navarro A, Quirós L, Casado M, et al. (2009) Physiological responses to mercury in feral carp populations inhabiting the low Ebro River (NE Spain), a historically contaminated site. Aquat Toxicol 93: 150-157. |
[36] | Mieiro CL, Bervoets L, Joosen S, et al. (2011) Metallothioneins failed to reflect mercury external levels of exposure and bioaccumulation in marine fish. Considerations on tissue and species specific responses. Chemosphere 85: 114-121. |
[37] | Bebianno MJ, Santos C, Canário J, et al. (2007) Hg and metallothionein-like proteins in the black scabbardfish Aphanopus carbo. Food Chem Toxicol 45: 1443-1452. |
[38] | Roméo M, Bennani N, Gnassia-Barelli M, et al. (2000) Cadmium and copper display different responses towards oxidative stress in the kidney of the sea bass Dicentrarchus labrax. Aquat Toxicol 48: 185-194. |
[39] | Carranza-Rosales P, Said-Fernández S, Sepúlveda-Saavedra J, et al. (2005) Morphologic and functional alterations induced by low doses of mercuric chloride in the kidney OK cell line: ultrastructural evidence for an apoptotic mechanism of damage. Toxicology 210: 111-121. |
[40] | Lee, JH, Youm JH, Kwon KS (2006) Mercuric chloride induces apoptosis in MDCK cells. Prov Med Pub Health 39: 199-204. |
[41] | Kim SH, Sharma RP (2004) Mercury-induced apoptosis and necrosis in murine macrophages: role of calcium-induced reactive oxygen species and p38 mitogen-activated protein kinase signaling. Toxicol Appl Pharmacol 196: 47-57. |
[42] | Borner C (2003) The Bcl-2 protein family : sensors and checkpoints for life-or-death decisions. Mol Immunol 39: 615-647. |
[43] | Luzio A, Monteiro SM, Fontainhas-Fernandes AA, et al. (2013) Copper induced upregulation of apoptosis related genes in zebrafish (Danio rerio) gill. Aquat Toxicol 128-129: 183-189. |
[44] | Risso-De Faverney C, Orsini N, De Sousa G, et al. (2004) Cadmium-induced apoptosis through the mitochondrial pathway in rainbow trout hepatocytes: involvement of oxidative stress. Aquat Toxicol 69: 247-258. |
[45] | Zheng GH, Liu CM, Sun JM, et al. (2014) Nickel-induced oxidative stress and apoptosis in Carassius auratus liver by JNK pathway. Aquat Toxicol 147: 105-111. |
[46] | Institoris L, Siroki O, Undeger U, et al. (2001) Immunotoxicological investigation of subacute combined exposure by permethrin and the heavy metals arsenic(III) and mercury(II) in rats. Int Immunopharmacol 1: 925-933. |
[47] | Ynalvez R, Gutierrez J (2016) Mini-review: toxicity of mercury as a consequence of enzyme alteration. BioMetals 29: 781-788. |
[48] | Zelikoff JT, Raymond A, Carlson E, et al. (2000) Biomarkers of immunotoxicity in fish: from the lab to the ocean. Toxicol Lett 112-113: 325-331. |
[49] | Segner H, Wenger M, Möller AM (2012) Immunotoxic effects of environmental toxicants in fish-how to assess them? Environ Sci Pollut Res 19: 2465-2476. |
[50] | Crowe W, Allsopp PJ, Watson GE, et al. (2016) Mercury as an environmental stimulus in the development of autoimmunity - A systematic review. Autoimmun Rev, in press. |
[51] | Guzzi G, Pigatto PD, Minoia C, et al. (2008) Dental amalgam, mercury toxicity, and renal autoimmunity. J Environ Pathol Toxicol Oncol 27: 147-155. |
[52] | Kal BI, Evcin O, Dundar N, et al. (2008) An unusual case of immediate hypersensitivity reaction associated with an amalgam restoration. Br Dent J 10: 547-550. |
[53] | Yadetie F, Karlsen OA, Lanzén A, et al. (2013) Global transcriptome analysis of Atlantic cod (Gadus morhua) liver after in vivo methylmercury exposure suggests effects on energy metabolism pathways. Aquat Toxicol 126: 314-325. |
[54] | Oliveira-Ribeiro CA, Fiipak NF, Mela M, et al. (2006) Hematological findings in neotropical fish Hoplias malabaricus exposed to subchronic and dietary doses of methylmercury, inorganic lead, and tributyltin chloride. Environ Res 101: 74-80. |
[55] | Kong X, Wang S, Jiang H, et al. (2012) Responses of acid/alkaline phosphatase, lysozyme , and catalase activities and lipid peroxidation to mercury exposure during the embryonic development of goldfish Carassius auratus. Aquat Toxicol 120-121: 119-125. |
[56] | Sanchez-Dardon J, Voccia I, Hontela A, et al. (1999) Immunomodulation by heavy metals tested individually or in mixtures in rainbow trout (Oncorhynchus mykiss) exposed in vivo. Environ Toxicol Chem 18: 1492-1497. |
[57] | Fletcher TC (1986) Modulation of nonspecific host defenses in fish. Vet Immunol Immunopathol 12: 59-67. |
[58] | Bennani N, Schmid-Alliana A, Lafaurie M (1996) Immunotoxic effects of copper and cadmium in the sea bass Dicentrarchus labrax. Immunopharmacol Immunotoxicol 18: 129-144. |
[59] | Randall DJ, Perry SF (1992) Catecholamine, In: Hoar WS, Randall DJ, Farrell TP, Fish physiology, New York, Academic Press, 255-300. |
[60] | Wilson RW, Bergman HL, Wood CM (1994) Metabolic costs and physiological consequences of acclimation to aluminum in juvenile rainbow trout (Oncorhynchus mykiss). 1: Gill morphology, swimming performance, and aerobic scope. Can J Fish Aquat Sci 51: 536-544. |
[61] | Oliveira-Ribeiro CA, Pelletier E, Pfeiffer WC, et al. (2000) Comparative uptake, bioaccumulation, and gill damages of inorganic mercury in tropical and Nordic freshwater fish. Environ Res 83: 286-292. |
[62] | Jagoe CH, Faivre A, Newman MC (1996) Morphological and morphometric changes in the gills of mosquitofish (Gambusia holbrooki) after exposure to mercury (II). Aquat Toxicol 31: 163-183. |
[63] | Tatara CP, Newman MC, Mulvey M (2001) Effect of mercury and Gpi-2 genotype on standard metabolic rate of eastern mosquitofish (Gambusia holbrooki). Environ Toxicol Chem 20: 782-786. |
[64] | Hopkins WA, Tatara CP, Brant HA, et al. (2003) Relationships between mercury body concentrations, standard metabolic rate, and body mass in eastern mosquitofish (Gambusia holbrooki) from three experimental populations. Environ Toxicol Chem 22: 586-590. |
[65] | Monteiro DA, Thomaz JM, Rantin FT, et al. (2013) Cardiorespiratory responses to graded hypoxia in the neotropical fish matrinxã (Brycon amazonicus) and traíra (Hoplias malabaricus) after waterborne or trophic exposure to inorganic mercury. Aquat Toxicol 140-141: 346-355. |
[66] | Au DW (2004) The application of histocytopathological biomarkers in marine pollution monitoring: a review. Mar Pollut Bull 48: 817-834. |
[67] | Jiraungkoorskul W, Upatham ES, Kruatrachue M, et al. (2003) Biochemical and histopathological effects of glyphosate herbicide on Nile tilapia (Oreochromis niloticus). Environ Toxicol 18: 260-267. |
[68] | Thophon S, Pokethitiyook P, Chalermwat K, et al. (2004) Ultrastructural alterations in the liver and kidney of white sea bass, Lates calcarifer, in acute and subchronic cadmium exposure. Environ Toxicol 19: 11-19. |
[69] | Dezfuli BS, Simoni E, Giari L, et al. (2006) Effects of experimental terbuthylazine exposure on the cells of Dicentrarchus labrax (L.). Chemosphere 64: 1684-1694. |
[70] | Giari L, Manera M, Simoni E, et al. (2007) Cellular alterations in different organs of European sea bass Dicentrarchus labrax (L.) exposed to cadmium. Chemosphere 67: 1171-1181. |
[71] | Giari L, Simoni E, Manera M, et al. (2008) Histocytological responses of Dicentrarchus labrax (L.) following mercury exposure. Ecotoxicol Environ Saf 70: 400-410. |
[72] | Arabi M (2004) Analyses of impact of metal ion contamination on carp (Cyprinus carpio L.) gill cell suspensions. Biol Trace Element Res 100: 229-245. |
[73] | Arabi M, Alaeddini MA (2005) Metal-ion-mediated oxidative stress in the gill homogenate of rainbow trout (Oncorhynchus mykiss): antioxidant potential of manganese, selenium, and albumin. Biol Trace Element Res 108: 155-168. |
[74] | Fernandes AB, Barros FL, Pecanha FM, et al. (2012) Toxic effects of mercury on the cardiovascular and central nervous systems. J Biomed Biotechnol 2012: 1-12. |
[75] | Sundin LI, Reid SG, Kalinin AL, et al. (1999). Cardiovascular and respiratory reflexes: the tropical fish, traira (Hoplias malabaricus) O2 chemoresponses. Respir Physiol 116: 181-199. |
[76] | Oliveira RD, Lopes JM, Sanches JR, et al. (2004) Cardiorespiratory responses of the facultative air-breathing fish jeju, Hoplerythrinus unitaeniatus (Teleostei, Erythrinidae), exposed to graded ambient hypoxia. Comp Biochem Physiol A 139: 479-485. |
[77] | Reid SG, Sundin L, Milsom WK (2005) The cardiorespiratory system in tropical fishes: structure, function, and control. Fish Physiol 21: 225-275. |
[78] | Crump KL, Trudeau VL (2009) Mercury-induced reproductive impairment in fish. Environ Toxicol Chem 28: 895-907. |
[79] | Meier S, Morton HC, Andersson E, et al. (2011) Low-dose exposure to alkylphenols adversely affects the sexual development of Atlantic cod (Gadus morhua): acceleration of the onset of puberty and delayed seasonal gonad development in mature female cod. Aquat Toxicol 105: 136-150. |
[80] | Arcand-Hoy LD, Benson WH (1998) Fish reproduction: an ecologically relevant indicator of endocrine disruption. Environ Toxicol Chem 17: 49-57. |
[81] | Zhang Q, Li Y, Liu Z, et al. (2016) Reproductive toxicity of inorganic mercury exposure in adult zebrafish : Histological damage, oxidative stress , and alterations of sex hormone and gene expression in the hypothalamic-pituitary-gonadal axis. Aquat Toxicol 177: 417-424. |
[82] | Drevnick PE, Sandheinrich MB (2003) Effects of dietary methylmercury on reproductive endocrinology of fathead minnows. Environ Sci Technol 37: 4390-4396. |
[83] | Klaper R, Rees CB, Drevnick P, et al. (2006) Gene expression changes related to endocrine function and decline in reproduction in fathead minnow (Pimephales promelas) after dietary methylmercury exposure. Environ Health Perspect 114: 1337-1344. |
[84] | Moran PW, Aluru N, Black RW, et al. (2007) Tissue contaminants and associated transcriptional response in trout liver from high elevation lakes of Washington. Environ Sci Technol 41: 6591-6597. |
[85] | Kirubagaran R, Joy KP (1992) Toxic effects of mercury on testicular activity in the fresh water teleost, Clarias batrachus (L.). J Fish Biol 41: 305-315. |
[86] | Liao C, Fu J, Shi J, et al. (2006) Methylmercury accumulation , histopathology effects, and cholinesterase activity alterations in medaka (Oryzias latipes) following sublethal exposure to methylmercury chloride. Environ Toxicol Pharmacol 22: 225-233. |
[87] | Vergilio CS, Moreira RV, Carvalho CE, et al. (2013) Histopathological effects of mercury on male gonad and sperm of tropical fish Gymnotus carapo in vitro. E3S Web of Conferences 12004: 3-6. |
[88] | Victor B, Mahalingam S, Sarojini R (1986) Toxicity of mercury and cadmium on oocyte differentiation and vitellogenesis of the teleost, Lepidocephalichtyhs thermalis (Bleeker). J Environ Biol 7: 209-214. |
[89] | Kirubagaran R, Joy KP (1988) Toxic effects of three mercurial compounds on survival, and histology of the kidney of the catfish Clarias batrachus (L.). Ecotoxicol Environ Saf 15: 171-179. |
[90] | Adams SM, Bevelhimer MS, Greeley MS, et al. (1999) Ecological risk assessment in a large river-reservoir: 6. Bioindicators of fish population health. Environ Toxicol Chem 18: 628-640. |
[91] | Depew DC, Basu N, Burgess NM, et al. (2012) Toxicity of dietary methylmercury to fish: derivation of ecologically meaningful threshold concentrations. Environ Toxicol Chem 31: 1536-1547. |
[92] | Simmons-Willis, TA, Koh AS, Clarkson TW, et al. (2002) Transport of a neurotoxicant by molecular mimicry: the methylmercury-L-cysteine complex is a substrate for human L-type large neutral amino acid transporter LAT1 and LAT2. Biochem J 367: 239-246. |
[93] | Stefansson ES, Heyes A, Rowe CL (2014) Tracing maternal transfer of methylmercury in the sheepshead minnow (Cyprinodon variegatus) with an enriched mercury stable isotope. Environ Sci Technol 48: 1957-1963. |
[94] | Hammerschmidt CR, Sandheinrich MB, Wiener JG, et al. (2002) Effects of dietary methylmercury on reproduction of fathead minnows. Environ Sci Technol 36: 877-883. |
[95] | Bridges KN, Soulen BK, Overturf CL, et al. (2016) Embryotoxicity of maternally transferred methylmercury to fathead minnows (Pimephales promelas). Environ Toxicol Chem 35: 1436-1441. |
[96] | Penglase S, Hamre K, Ellingsen S (2014) Selenium and mercury have a synergistic negative effect on fish reproduction. Aquat Toxicol 149: 16-24. |
[97] | Stohs SJ, Bagchi D (1995) Oxidative mechanisms in the toxicity of metal ions. Free Radic Biol Med 18: 321-336. |
[98] | Aschner M, Syversen T, Souza DO, et al. (2007) Involvement of glutamate and reactive oxygen species in methylmercury neurotoxicity. Braz J Med Biol Res 40: 285-291. |
[99] | Stringari J, Nunes AKC, Franco JL, et al. (2008) Prenatal methylmercury exposure hampers glutathione antioxidant system ontogenesis and causes long-lasting oxidative stress in the mouse brain. Toxicol Appl Pharmacol 227: 147-154. |
[100] | Farina M, Avila DS, Da Rocha JBT, et al. (2013) Metals, oxidative stress and neurodegeneration: a focus on iron, manganese and mercury. Neurochem Int 62: 575-594. |
[101] | Mieiro CL, Pereira ME, Duarte AC, et al. (2011) Brain as a critical target of mercury in environmentally exposed fish (Dicentrarchus labrax)-Bioaccumulation and oxidative stress profiles. Aquat Toxicol 103: 233-240. |
[102] | Pereira P, Puga S, Cardoso V, et al. (2016) Inorganic mercury accumulation in brain following waterborne exposure elicits a deficit on the number of brain cells and impairs swimming behavior in fish (white seabream-Diplodus sargus). Aquat Toxicol 170: 400-412. |
[103] | De Flora S, Bennicelli C, Bagnasco M (1994) Genotoxicity of mercury compounds. A review. Mutat Res Genet Toxicol 317: 57-79. |
[104] | Maulvault AL, Custódio A, Anacleto P, et al. (2016) Bioaccumulation and elimination of mercury in juvenile seabass (Dicentrarchus labrax) in a warmer environment. Environ Res 149: 77-85. |
[105] | Berntssen MHG, Aatland A, Handy RD (2003) Chronic dietary mercury exposure causes oxidative stress, brain lesions, and altered behaviour in Atlantic salmon (Salmo salar) parr. Aquat Toxicol 65: 55-72. |
[106] | Wang Y, Wang D, Lin L, et al. (2015) Quantitative proteomic analysis reveals proteins involved in the neurotoxicity of marine medaka Oryzias melastigma chronically exposed to inorganic mercury. Chemosphere 119: 1126-1133. |
[107] | Gentès S, Maury-Brachet R, Feng C, et al. (2015) Specific effects of dietary methylmercury and inorganic mercury in zebrafish (Danio rerio) determined by genetic, histological, and metallothionein responses. Environ Sci Technol 49: 14560-14569. |
[108] | González P, Dominique Y, Massabuau JC, et al. (2005) Comparative effects of dietary methylmercury on gene expression in liver, skeletal muscle and brain of the zebrafish (Danio rerio). Biometals 39: 3972-3980. |
[109] | WHO (World Health Organization) (1989) Mercury-Environmental Aspects. WHO, Geneva, Switzerland. |
[110] | Bano Y, Hasan M (1990) Histopathological lesions in the body organs of cat-fish (Heteropneustes fossilis) following mercury intoxication. J Environ Sci Health 25: 67-85. |
[111] | Lemaire P, Berhaut J, Lemaire-Gony S, et al. (1992) Ultrastructural changes induced by benzo[a]pyrene in sea bass (Dicentrarchus labrax) liver and intestine: importance of the intoxication route. Environ Res 57: 59-72. |
[112] | Banerjee S, Bhattacharya S (1995) Histopathological changes induced by chronic nonlethal levels of elsan, mercury, and ammonia in the small intestine of Channa punctatus (Bloch). Ecotoxicol Environ Saf 3: 62-68. |
[113] | Oliveira Ribeiro CA, Belger L, Pelletier E, et al. (2002) Histopathological evidence of inorganic mercury and methyl mercury toxicity in the arctic charr (Salvelinus alpinus). Environ Res 90: 217-225. |
[114] | Leaner JJ, Mason RP (2004) Methylmercury uptake and distribution kinetics in sheepshead minnows, Cyprinodon variegatus, after exposure to Ch3Hg-spiked food. Environ Toxicol Chem 23: 2138-2146. |
[115] | Burrows WD, Krenkel PA (1973) Studies on uptake and loss of methylmercury by blue-gills (Lepomis macrochirus Raf.). Environ Sci Technol 7: 1127-1130. |
[116] | Huang SSY, Strathe AB, Fadel JG, et al. (2012) Absorption, distribution, and elimination of graded oral doses of methylmercury in juvenile white sturgeon. Aquat Toxicol 122-123: 163-171. |
[117] | Abreu SN, Pereira E, Vale C, et al. (2000) Accumulation of mercury in sea bass from a contaminated lagoon (Ria de Aveiro, Portugal). Mar Pollut Bull 40: 293-297. |
[118] | Kennedy CJ (2003) Uptake and accumulation of mercury from dental amalgam in the common goldfish, Carassius auratus. Environ Pollut 121: 321-326. |
[119] | Yamamoto Y, Almeida R, Regina S, et al. (2014) Mercury distribution in target organs and biochemical responses after subchronic and trophic exposure to Neotropical fish Hoplias malabaricus. Fish Physiol Biochem 40: 245-256. |
[120] | Lee JW, Kim JW, De Riu N, et al. (2012) Histopathological alterations of juvenile green (Acipenser medirostris) and white sturgeon (Acipenser transmontanus) exposed to graded levels of dietary methylmercury. Aquat Toxicol 109: 90-99. |
[121] | Wester PW, Canton HH (1992) Histopathological effects in Poecilia reticulata (guppy) exposed to methylmercury chloride. Toxicol Pathol 20: 81-92. |
[122] | Kirubagaran R, Joy KP (1988) Toxic effects of three mercurial compounds on survival, and histology of the kidney of the catfish Clarias batrachus (L.). Ecotoxicol Environ Saf 15: 171-179. |
[123] | Bridges CC, Zalups RK (2010) Transport of inorganic mercury and methylmercury in target tissues and organs. J Toxicol Environ Health B 13: 385-410. |
[124] | Patil SS, Jabde SV (1998) Effect of mercury poisoning on some haematological parameters from a fresh water fish, Channa gachua. Pollut Res 17: 223-228. |
[125] | Fletcher TC, White A (1986) Nephrotoxic and hematological effects of mercury chloride in the plaice (Pleuronectes platessa L.). Aquat Toxicol 8: 77-84. |
[126] | Ishikawa NM, Ranzani-Paiva MJT, Vicente J, et al. (2007) Hematological Parameters in Nile Tilápia, Oreochromis niloticus exposed to subletal concentrations of mercury. Braz J Med Biol Res 50: 619-626. |
[127] | Gwoździński K, Roche H, Pérès G (1992) The comparison of the effects of heavy metal ions on the antioxidant enzyme activities in human and fish Dicentrarchus labrax erythrocytes. Comp Biochem Physiol C 102: 57-60. |
[128] | Sanchez-Galan S, Linde AR, Garcia-Vazquez E (1999) Brown trout and European minnow as target species for genotoxicity tests: differential sensitivity to heavy metals. Ecotoxicol Environ Saf 43: 301-304. |
[129] | Yadav KK, Trivedi SP (2009) Sublethal exposure of heavy metals induces micronuclei in fish, Channa punctata. Chemosphere 77: 1495-1500. |
[130] | Guilherme S, Válega M, Pereira ME, et al. (2008) Erythrocytic nuclear abnormalities in wild and caged fish (Liza aurata) along an environmental mercury contamination gradient. Ecotoxicol Environ Saf 70: 411-421. |
1. | Karpaga Priyaa Kartheeswaran, Arockia Xavier Annie Rayan, Geetha Thekkumpurath Varrieth, Correction: Enhanced disease-disease association with information enriched disease representation, 2024, 21, 1551-0018, 2729, 10.3934/mbe.2024120 | |
2. | Karpaga Priyaa Kartheeswaran, Arockia Xavier Annie Rayan, Geetha Thekkumpurath Varrieth, Genetically and semantically aware homogeneous network for prediction and scoring of comorbidities, 2024, 183, 00104825, 109252, 10.1016/j.compbiomed.2024.109252 | |
3. | Shirui Yu, Peng Dong, Junlian Li, Xiaoli Tang, Xiaoying Li, A study on large-scale disease causality discovery from biomedical literature, 2025, 25, 1472-6947, 10.1186/s12911-025-02893-0 |
Methods | Without improved sentence representation | With improved sentence representation | ||||
Performance measure | ||||||
Precision (%) |
Recall (%) |
F-measure (%) |
Precision (%) |
Recall (%) |
F-measure (%) |
|
LSTM [49] | 65.13 | 67.11 | 66.11 | 66.92 | 68.37 | 67.64 |
BiLSTM [89] | 64.88 | 66.15 | 65.51 | 65.15 | 68.02 | 66.55 |
CNN [90] | 74.13 | 71.27 | 72.67 | 75.20 | 72.64 | 73.90 |
BERT [91] | 78.65 | 80.32 | 79.48 | 80.63 | 82.12 | 81.37 |
BioBERT [92] | 81.54 | 82.01 | 81.77 | 82.69 | 83.88 | 83.28 |
LC-CNN [17] | 82.16 | 84.89 | 83.50 | − | − | − |
ESEC-CNN* | − | − | − | 83.06 | 86.54 | 84.76 |
ESEC-CNN** | − | − | − | 84.03 | 87.12 | 85.54 |
*- partial improved sentence representation{(PubMed, PMC, Wiki, News), (POS, NE Dist)} **- improved sentence representation {(PubMed, PMC, Wiki, News), (POS, position, Dep. Rel., Chunk, NE)} |
Details | Available 521 labelled DDAE dataset [17] | Constructed DDAE dataset |
Abstracts | 521 | 39,510 |
Unique Ds | 1103 | 14,598 |
Unique DD pairs | 3600 | 28,980 |
Unique Positive DD pairs | 1626 | 34,481 |
Unique Negative DD pairs | 124 | 5488 |
unique Null DD pairs | 2649 | 36102 |
Unique Positive-Negative DD pairs | 53 | 3254 |
Unique Positive-Negative-Null DD pairs | 33 | 2589 |
Association Type | Method | Wang et al. [24] | Resnik [25] | Schlicker et al. [88] | Lin [26] |
#positively and negatively associated DD pairs = 54 [available 521 labelled dataset [17]] | GloVe-50 [93] | 0.001 | 0.007 | 0.015 | 0.015 |
SicknessMiner [47] | 0.277131 | 0.203487 | 0.340086 | 0.340086 | |
GexText [18] | 0.3982 | 0.3884 | 0.394 | 0.4013 | |
Enhanced literature-based positive, negative DDA representation | 0.402411 | 0.417671 | 0.531724 | 0.531724 | |
#positively and negatively associated DD pairs= 3254 [Enhanced DDA dataset] |
GloVe-50 [93] | 0.005 | 0.009 | 0.024 | 0.026 |
SicknessMiner [47] | 0.323 | 0.321 | 0.352 | 0.349 | |
GexText [18] | 0.468 | 0.463 | 0.476 | 0.476 | |
Enhanced literature-based positive, negative DDA representation | 0.515 | 0.521 | 0.575 | 0.573 |
Association Type | Method | Wang et al. [24] | Resnik [25] | Schlicker et al. [88] | Lin [26] |
#Only negatively associated DD pairs = 70 [available 521 labelled dataset [17]] | GloVe-50 [93] | −0.356 | −0.245 | −0.319 | −0.319 |
SicknessMiner [47] | −0.22114 | −0.18152 | −0.22987 | −0.22987 | |
GexText [18] | −0.008 | −0.125 | −0.012 | −0.011 | |
Enhanced literature-based positive, negative DDA | 0.229156 | 0.208198 | 0.138969 | 0.138969 | |
#Only negatively associated DD pairs= 2234 [Enhanced DDA dataset] |
GloVe-50 [93] | −0.234 | −0.301 | −0.286 | −0.284 |
SicknessMiner [47] | −0.229 | −0.298 | −0.251 | −0.253 | |
GexText [18] | −0.191 | −0.226 | −0.218 | −0.218 | |
Enhanced literature-based positive, negative DDA | 0.306 | 0.299 | 0.274 | 0.269 |
Association Type | Method | Wang et al. [24] | Resnik [25] | Schlicker et al. [88] | Lin [26] |
#Null associated DD pairs = 2649 [available 521 labelled dataset [17]] | GloVe-50 [93] | 0.07 | 0.018 | 0.0195 | 0.03 |
SicknessMiner [47] | −0.0436 | −0.009 | 0.003586 | 0.002185 | |
GexText [18] | 0.231 | 0.236 | 0.196 | 0.193 | |
Literature-based Null DD similarity | 0.333 | 0.307 | 0.281 | 0.281 | |
#Null associated DD pairs= 36102 [Enhanced DDA dataset] |
GloVe-50 [93] | 0.006 | 0.005 | 0.002 | 0.003 |
SicknessMiner [47] | 0.024 | 0.017 | 0.0052 | 0.00549 | |
GexText [18] | 0.168 | 0.186 | 0.210 | 0.208 | |
Literature-based Null DD similarity | 0.423 | 0.419 | 0.454 | 0.456 |
Source of multiple embeddings of ancestor | Ancestors' information | Spearman's rank correlation N=7936 DD pairs (DisGeNET) |
Ontology Sources- CCS, ATC: • Clinical Classifications Software for ICD- 9-CM (CCS) (diagnosis) • Anatomical Therapeutic Chemical classification system (ATC) (medications) [86] |
All Ancestors of DxandDy:∀Axand∀Ay [86] | 0.759 |
Common Ancestors of DxandDy:∀Ax∩∀AyAy | 0.772 | |
New Ancestors Sets of DxandDy:New_Anc_Set(Dx) and New_Anc_Set(Dy) | 0.779 |
Sources of multiple embeddings of ancestor | Different combination of ancestors sets for Dx-Dy quantification | Without Root and leaf node multi-source embeddings | With Root and leaf node multi-source embeddings |
Spearman's rank correlation N = 2658 DD pairs (DisGeNET) |
|||
Ontology Sources: • Clinical Classifications Software for ICD- 9-CM (CCS) and Anatomical Therapeutic Chemical classification system (ATC) [86] |
∀Axand∀Ay [86] | 0.612 | 0.618 |
New_Anc_Set(Dx) and New_Anc_Set(Dy) | 0.643 | 0.695 | |
Ontology Sources • Disease Ontology (DO) • UMLS Biomedical Text • Clinical Notes • Insurance Claims Database • Journal Articles |
∀Axand∀Ay [86] | 0.726 | 0.730 |
New_Anc_Set(Dx) and New_Anc_Set(Dy) | 0.745 | 0.788 |
Calculation of level-weight of LCS(Dx, Dy) for Dx-Dy quantification | Spearman's rank correlation N = 1, 75,939 DD pairs (DisGeNET) |
Baseline_LCA of GOntoSim: using upper DAG Level-weight of LCS(Dx,Dy) by ancestors on longest path to LCS(Dx,Dy) [65] |
0.773 |
Novel ancestorial-level weight: using lower DAG Level-weight of LCS(Dx,Dy) by children on longest path to Dx and Dy |
0.782 |
Method | Without multi-source ancestorial embedding | With multi-source ancestorial embedding |
Spearman's rank correlation N = 1, 75,939 DD pairs (DisGeNET) | ||
Semantic similarity of diseases [63] | 0.610 | 0.720 |
Baseline_LCA of GOntoSim [65] | 0.612 | 0.723 |
Novel ancestorial level-weight | 0.619 | 0.756 |
Different concept-based representation methods for concept-based DDA quantification | Spearman's rank correlation N = 1756 DD pairs (DisGeNET) |
MMORE (CCS (diagnosis), ATC (medications)) [86] | 0.703 |
Cui2vec (Clinical Notes, Claims Insurance, Journal articles) [20] | 0.772 |
Retrofitted concept vector representation (PubMed, UMLS) [33] | 0.781 |
Proposed Ontology-based joint multi-source association representation | |
Ancestorial level-based + ontological relationship connection based-Parent, Grandparents only* | 0.787 |
Ancestorial level-based + ontological relationship connection based-Parent, Grandparents & sibling only** | 0.790 |
Ancestorial level-based + ontological relationship connection based-Parent, Grandparents, sibling, uncle & cousin relationships*** | 0.802 |
Disease Vector Representation | Spearman's Rank Correlation | |||
Literature-based only | DisGeNet Gene-based | DisGeNet Variant-based | Standard dataset GO-based | Human-rated |
N = 2938 DD pairs (DisGeNET) | N = 199 DD pairs | |||
Cui2vec (Clinical Notes, Claims Insurance, Journal articles) [20] | 0.797 | 0.254 | 0.422 | 0.679 |
Enhanced Literature-Based Disease Vector Representation (Vector-Similarity Fusion Without Integration) | 0.799 | 0.252 | 0.427 | 0.682 |
Concept-based only | DisGeNet Gene-based | DisGeNet Variant-based | Standard dataset GO-based | Human-rated |
N = 2638 DD pairs | N = 50 DD pairs | |||
MMORE (CCS (diagnosis), ATC (medications)) [86] | 0.716 | 0.144 | 0.541 | 0.790 |
Ontology-Based Joint Multi-Source Association Embedding (Ancestral Level-Based + Ontological Relationship Connection Based) | 0.808 | 0.146 | 0.551 | 0.809 |
Integration of literature-based and concept-based | DisGeNet Gene-based | DisGeNet Variant-based | Standard dataset GO-based | Human-rated |
N = 1638 DD pairs | N = 187 DD pairs | |||
Retrofitted Concept Vector Representation (PubMed, UMLS) [33] | 0.801 | 0.213 | 0.592 | 0.810 |
Integration of literature-based and concept-based | DisGeNet Gene-based | DisGeNet Variant-based | Standard dataset GO-based | Human-rated |
N = 1638 DD pairs | N = 187 DD pairs | |||
MORE [32] | 0.811 | 0.220 | 0.609 | 0.813 |
Integrated Disease Vector Representation (Literature-Based Positive, Negative & Concept-Based DDA) | 0.816 | 0.225 | 0.624 | 0.818 |
Enhanced Literature-Based & Concept-Based Joint Embedding Model for Disease Vector Representation (Vector-Similarity Fusion with Integration) | 0.822 | 0.227 | 0.626 | 0.821 |
Literature-based DDA sentence classification models | LSTM [21] | BiLSTM [89] | CNN [90] | BERT [91] | BioBERT [92] | LC-CNN [17] | ESEC-CNN** |
Training time per epoch (in seconds) | 240s | 237s | 184s | 262s | 270s | 196s | 200s |
Concept-based DDA representation | Retrofitted concept vector representation [33] | Cui2vec [20] | MMORE [86] | Ontology-based joint multi-source association representation | N/A | N/A | N/A |
Average seconds to generate concept-based DDA representation | 15s | 16s | 19s | 22s | N/A | N/A | N/A |
N/A-represents not applicable |
Disease 1 | Disease 2 | Association score |
amyotrophic lateral sclerosis | motor neuron disease | 0.999998 |
Hypertensive retinopathy | Vascular disease | 0.096729 |
cardiovascular disease | intrinsic asthma | 0.043286 |
autosomal dominant polycystic kidney disease | autosomal dominant polycystic kidney disease | 0.033808 |
myopathy | Sjogren's syndrome | 0.022195 |
congenital muscular dystrophy | muscular dystrophy | 0.010661 |
cerebral folate receptor alpha deficiency | Down syndrome | 0.007642 |
muscular dystrophy | myotonic dystrophy type2 | 0.006518 |
Alzheimer's disease | Moyamoya disease | 0.004409 |
migraine without aura | Fibromyalgia | 0.003855 |
diabetes mellitus | diabetic neuropathy | 0.003613 |
diabetes mellitus | Hypoglycemia | 0.003495 |
acute myeloid leukemia | acute monocytic leukemia | 0.003449 |
lepromatous leprosy | Leprosy | 0.002793 |
marasmus | anorexia nervosa | 0.002623 |
lymphoblastic leukemia | lung disease | 0.002265 |
cystic fibrosis | acute pancreatitis | 0.002053 |
acute monocytic leukemia | acute leukemia | 0.001959 |
Azoospermia | oligospermia | 0.001876 |
acute myeloid leukemia | acute leukemia | 0.001875 |
Disease | Most associated diseases |
amyotrophic lateral sclerosis | Motor neuron disease, lateral sclerosis |
motor neuron disease | Motor neuron disease, cardiovascular disease |
Hypertensive retinopathy | Vascular disease |
Vascular disease | Hypertensive retinopathy, cardiovascular disease |
Intrinsic asthma | Cardiovascular disease, lung disease |
autosomal dominant polycystic kidney disease | autosomal recessive polycystic kidney disease |
Myopathy | Sjogren's syndrome |
Sjogren's syndrome | Myopathy, systemic scleroderma, Behcet's disease, systemic lupus erythematosus |
DO Disease Category | Top 5 associated DO Disease Categories | Normalized average score of disease pairs category-wise |
Nervous system disease | Nervous system disease | 0.0223 |
Cardiovascular system disease | 0.0221 | |
Musculoskeletal system disease | 0.0200 | |
Physical disorder | 0.0085 | |
Disease of metabolism | 0.0025 | |
Physical disorder | Physical disorder | 0.014 |
Nervous system disease | 0.0085 | |
Disease of metabolism | 0.0052 | |
Disease of cellular proliferation | 0.0038 | |
Endocrine system disease | 0.00056 | |
Disease of cellular proliferation | Disease of cellular proliferation | 0.00400 |
Respiratory system disease | 0.00382 | |
Physical disorder | 0.00380 | |
Endocrine system disease | 0.000620 | |
Cardiovascular system disease | 0.000621 | |
Urinary system disease | Urinary system disease | 0.00109 |
Disease of cellular proliferation | 0.00062 | |
Endocrine system disease | 0.00059 | |
Gastrointestinal system disease | 0.00054 | |
Disease by infectious agent | 0.00048 | |
Endocrine system disease | Endocrine system disease | 0.000920 |
Disease of cellular proliferation | 0.000626 | |
Urinary system disease | 0.000596 | |
Musculoskeletal system disease | 0.000580 | |
Cardiovascular system disease | 0.000564 | |
Disease by infectious agent | Disease by infectious agent | 0.00072 |
Endocrine system disease | 0.000486 | |
Nervous system disease | 0.000485 | |
Respiratory system disease | 0.000482 | |
Gastrointestinal system disease | 0.000475 |
Disease with category | Top 10 associated disease pairs | Category of associated disease pair | Association score |
Diabetes mellitus Endocrine system disease |
diabetic neuropathy | endocrine system disease | 0.003613 |
hypoglycaemia | endocrine system disease | 0.003495 | |
acute myocardial infarction | cardiovascular system disease | 0.000944 | |
diabetic retinopathy | nervous system disease | 0.000938 | |
stomach cancer | disease of cellular proliferations | 0.000918 | |
kidney failure | urinary system disease | 0.000728 | |
Hypothyroidism | endocrine system disease | 0.000649 | |
disease of metabolism | disease of metabolism | 0.000627 | |
autoimmune disease | musculoskeletal system disease | 0.000603 | |
brain cancer | disease of cellular proliferations | 0.000567 | |
Cardiovascular disease Cardiovascular system disease |
intrinsic asthma | respiratory system disease | 0.043286 |
nephrotic syndrome | urinary system disease | 0.001028 | |
vascular disease | cardiovascular system disease | 0.000399 | |
disease by infectious agent | parasetic infectious disease | 0.000376 | |
vein disease | cardiovascular system disease | 0.000359 | |
generalized atherosclerosis | cardiovascular system disease | 0.000353 | |
Moyamoya disease | cardiovascular system disease | 0.000351 | |
peripheral artery disease | cardiovascular sys. disease | 0.000347 | |
Epilepsy | nervous system disease | 0.000331 | |
intermediate coronary syndrome | cardiovascular system disease | 0.000320 |
Methods | Without improved sentence representation | With improved sentence representation | ||||
Performance measure | ||||||
Precision (%) |
Recall (%) |
F-measure (%) |
Precision (%) |
Recall (%) |
F-measure (%) |
|
LSTM [49] | 65.13 | 67.11 | 66.11 | 66.92 | 68.37 | 67.64 |
BiLSTM [89] | 64.88 | 66.15 | 65.51 | 65.15 | 68.02 | 66.55 |
CNN [90] | 74.13 | 71.27 | 72.67 | 75.20 | 72.64 | 73.90 |
BERT [91] | 78.65 | 80.32 | 79.48 | 80.63 | 82.12 | 81.37 |
BioBERT [92] | 81.54 | 82.01 | 81.77 | 82.69 | 83.88 | 83.28 |
LC-CNN [17] | 82.16 | 84.89 | 83.50 | − | − | − |
ESEC-CNN* | − | − | − | 83.06 | 86.54 | 84.76 |
ESEC-CNN** | − | − | − | 84.03 | 87.12 | 85.54 |
*- partial improved sentence representation{(PubMed, PMC, Wiki, News), (POS, NE Dist)} **- improved sentence representation {(PubMed, PMC, Wiki, News), (POS, position, Dep. Rel., Chunk, NE)} |
Details | Available 521 labelled DDAE dataset [17] | Constructed DDAE dataset |
Abstracts | 521 | 39,510 |
Unique Ds | 1103 | 14,598 |
Unique DD pairs | 3600 | 28,980 |
Unique Positive DD pairs | 1626 | 34,481 |
Unique Negative DD pairs | 124 | 5488 |
unique Null DD pairs | 2649 | 36102 |
Unique Positive-Negative DD pairs | 53 | 3254 |
Unique Positive-Negative-Null DD pairs | 33 | 2589 |
Association Type | Method | Wang et al. [24] | Resnik [25] | Schlicker et al. [88] | Lin [26] |
#positively and negatively associated DD pairs = 54 [available 521 labelled dataset [17]] | GloVe-50 [93] | 0.001 | 0.007 | 0.015 | 0.015 |
SicknessMiner [47] | 0.277131 | 0.203487 | 0.340086 | 0.340086 | |
GexText [18] | 0.3982 | 0.3884 | 0.394 | 0.4013 | |
Enhanced literature-based positive, negative DDA representation | 0.402411 | 0.417671 | 0.531724 | 0.531724 | |
#positively and negatively associated DD pairs= 3254 [Enhanced DDA dataset] |
GloVe-50 [93] | 0.005 | 0.009 | 0.024 | 0.026 |
SicknessMiner [47] | 0.323 | 0.321 | 0.352 | 0.349 | |
GexText [18] | 0.468 | 0.463 | 0.476 | 0.476 | |
Enhanced literature-based positive, negative DDA representation | 0.515 | 0.521 | 0.575 | 0.573 |
Association Type | Method | Wang et al. [24] | Resnik [25] | Schlicker et al. [88] | Lin [26] |
#Only negatively associated DD pairs = 70 [available 521 labelled dataset [17]] | GloVe-50 [93] | −0.356 | −0.245 | −0.319 | −0.319 |
SicknessMiner [47] | −0.22114 | −0.18152 | −0.22987 | −0.22987 | |
GexText [18] | −0.008 | −0.125 | −0.012 | −0.011 | |
Enhanced literature-based positive, negative DDA | 0.229156 | 0.208198 | 0.138969 | 0.138969 | |
#Only negatively associated DD pairs= 2234 [Enhanced DDA dataset] |
GloVe-50 [93] | −0.234 | −0.301 | −0.286 | −0.284 |
SicknessMiner [47] | −0.229 | −0.298 | −0.251 | −0.253 | |
GexText [18] | −0.191 | −0.226 | −0.218 | −0.218 | |
Enhanced literature-based positive, negative DDA | 0.306 | 0.299 | 0.274 | 0.269 |
Association Type | Method | Wang et al. [24] | Resnik [25] | Schlicker et al. [88] | Lin [26] |
#Null associated DD pairs = 2649 [available 521 labelled dataset [17]] | GloVe-50 [93] | 0.07 | 0.018 | 0.0195 | 0.03 |
SicknessMiner [47] | −0.0436 | −0.009 | 0.003586 | 0.002185 | |
GexText [18] | 0.231 | 0.236 | 0.196 | 0.193 | |
Literature-based Null DD similarity | 0.333 | 0.307 | 0.281 | 0.281 | |
#Null associated DD pairs= 36102 [Enhanced DDA dataset] |
GloVe-50 [93] | 0.006 | 0.005 | 0.002 | 0.003 |
SicknessMiner [47] | 0.024 | 0.017 | 0.0052 | 0.00549 | |
GexText [18] | 0.168 | 0.186 | 0.210 | 0.208 | |
Literature-based Null DD similarity | 0.423 | 0.419 | 0.454 | 0.456 |
Source of multiple embeddings of ancestor | Ancestors' information | Spearman's rank correlation N=7936 DD pairs (DisGeNET) |
Ontology Sources- CCS, ATC: • Clinical Classifications Software for ICD- 9-CM (CCS) (diagnosis) • Anatomical Therapeutic Chemical classification system (ATC) (medications) [86] |
All Ancestors of DxandDy:∀Axand∀Ay [86] | 0.759 |
Common Ancestors of DxandDy:∀Ax∩∀AyAy | 0.772 | |
New Ancestors Sets of DxandDy:New_Anc_Set(Dx) and New_Anc_Set(Dy) | 0.779 |
Sources of multiple embeddings of ancestor | Different combination of ancestors sets for Dx-Dy quantification | Without Root and leaf node multi-source embeddings | With Root and leaf node multi-source embeddings |
Spearman's rank correlation N = 2658 DD pairs (DisGeNET) |
|||
Ontology Sources: • Clinical Classifications Software for ICD- 9-CM (CCS) and Anatomical Therapeutic Chemical classification system (ATC) [86] |
∀Axand∀Ay [86] | 0.612 | 0.618 |
New_Anc_Set(Dx) and New_Anc_Set(Dy) | 0.643 | 0.695 | |
Ontology Sources • Disease Ontology (DO) • UMLS Biomedical Text • Clinical Notes • Insurance Claims Database • Journal Articles |
∀Axand∀Ay [86] | 0.726 | 0.730 |
New_Anc_Set(Dx) and New_Anc_Set(Dy) | 0.745 | 0.788 |
Calculation of level-weight of LCS(Dx, Dy) for Dx-Dy quantification | Spearman's rank correlation N = 1, 75,939 DD pairs (DisGeNET) |
Baseline_LCA of GOntoSim: using upper DAG Level-weight of LCS(Dx,Dy) by ancestors on longest path to LCS(Dx,Dy) [65] |
0.773 |
Novel ancestorial-level weight: using lower DAG Level-weight of LCS(Dx,Dy) by children on longest path to Dx and Dy |
0.782 |
Method | Without multi-source ancestorial embedding | With multi-source ancestorial embedding |
Spearman's rank correlation N = 1, 75,939 DD pairs (DisGeNET) | ||
Semantic similarity of diseases [63] | 0.610 | 0.720 |
Baseline_LCA of GOntoSim [65] | 0.612 | 0.723 |
Novel ancestorial level-weight | 0.619 | 0.756 |
Different concept-based representation methods for concept-based DDA quantification | Spearman's rank correlation N = 1756 DD pairs (DisGeNET) |
MMORE (CCS (diagnosis), ATC (medications)) [86] | 0.703 |
Cui2vec (Clinical Notes, Claims Insurance, Journal articles) [20] | 0.772 |
Retrofitted concept vector representation (PubMed, UMLS) [33] | 0.781 |
Proposed Ontology-based joint multi-source association representation | |
Ancestorial level-based + ontological relationship connection based-Parent, Grandparents only* | 0.787 |
Ancestorial level-based + ontological relationship connection based-Parent, Grandparents & sibling only** | 0.790 |
Ancestorial level-based + ontological relationship connection based-Parent, Grandparents, sibling, uncle & cousin relationships*** | 0.802 |
Disease Vector Representation | Spearman's Rank Correlation | |||
Literature-based only | DisGeNet Gene-based | DisGeNet Variant-based | Standard dataset GO-based | Human-rated |
N = 2938 DD pairs (DisGeNET) | N = 199 DD pairs | |||
Cui2vec (Clinical Notes, Claims Insurance, Journal articles) [20] | 0.797 | 0.254 | 0.422 | 0.679 |
Enhanced Literature-Based Disease Vector Representation (Vector-Similarity Fusion Without Integration) | 0.799 | 0.252 | 0.427 | 0.682 |
Concept-based only | DisGeNet Gene-based | DisGeNet Variant-based | Standard dataset GO-based | Human-rated |
N = 2638 DD pairs | N = 50 DD pairs | |||
MMORE (CCS (diagnosis), ATC (medications)) [86] | 0.716 | 0.144 | 0.541 | 0.790 |
Ontology-Based Joint Multi-Source Association Embedding (Ancestral Level-Based + Ontological Relationship Connection Based) | 0.808 | 0.146 | 0.551 | 0.809 |
Integration of literature-based and concept-based | DisGeNet Gene-based | DisGeNet Variant-based | Standard dataset GO-based | Human-rated |
N = 1638 DD pairs | N = 187 DD pairs | |||
Retrofitted Concept Vector Representation (PubMed, UMLS) [33] | 0.801 | 0.213 | 0.592 | 0.810 |
Integration of literature-based and concept-based | DisGeNet Gene-based | DisGeNet Variant-based | Standard dataset GO-based | Human-rated |
N = 1638 DD pairs | N = 187 DD pairs | |||
MORE [32] | 0.811 | 0.220 | 0.609 | 0.813 |
Integrated Disease Vector Representation (Literature-Based Positive, Negative & Concept-Based DDA) | 0.816 | 0.225 | 0.624 | 0.818 |
Enhanced Literature-Based & Concept-Based Joint Embedding Model for Disease Vector Representation (Vector-Similarity Fusion with Integration) | 0.822 | 0.227 | 0.626 | 0.821 |
Literature-based DDA sentence classification models | LSTM [21] | BiLSTM [89] | CNN [90] | BERT [91] | BioBERT [92] | LC-CNN [17] | ESEC-CNN** |
Training time per epoch (in seconds) | 240s | 237s | 184s | 262s | 270s | 196s | 200s |
Concept-based DDA representation | Retrofitted concept vector representation [33] | Cui2vec [20] | MMORE [86] | Ontology-based joint multi-source association representation | N/A | N/A | N/A |
Average seconds to generate concept-based DDA representation | 15s | 16s | 19s | 22s | N/A | N/A | N/A |
N/A-represents not applicable |
Disease 1 | Disease 2 | Association score |
amyotrophic lateral sclerosis | motor neuron disease | 0.999998 |
Hypertensive retinopathy | Vascular disease | 0.096729 |
cardiovascular disease | intrinsic asthma | 0.043286 |
autosomal dominant polycystic kidney disease | autosomal dominant polycystic kidney disease | 0.033808 |
myopathy | Sjogren's syndrome | 0.022195 |
congenital muscular dystrophy | muscular dystrophy | 0.010661 |
cerebral folate receptor alpha deficiency | Down syndrome | 0.007642 |
muscular dystrophy | myotonic dystrophy type2 | 0.006518 |
Alzheimer's disease | Moyamoya disease | 0.004409 |
migraine without aura | Fibromyalgia | 0.003855 |
diabetes mellitus | diabetic neuropathy | 0.003613 |
diabetes mellitus | Hypoglycemia | 0.003495 |
acute myeloid leukemia | acute monocytic leukemia | 0.003449 |
lepromatous leprosy | Leprosy | 0.002793 |
marasmus | anorexia nervosa | 0.002623 |
lymphoblastic leukemia | lung disease | 0.002265 |
cystic fibrosis | acute pancreatitis | 0.002053 |
acute monocytic leukemia | acute leukemia | 0.001959 |
Azoospermia | oligospermia | 0.001876 |
acute myeloid leukemia | acute leukemia | 0.001875 |
Disease | Most associated diseases |
amyotrophic lateral sclerosis | Motor neuron disease, lateral sclerosis |
motor neuron disease | Motor neuron disease, cardiovascular disease |
Hypertensive retinopathy | Vascular disease |
Vascular disease | Hypertensive retinopathy, cardiovascular disease |
Intrinsic asthma | Cardiovascular disease, lung disease |
autosomal dominant polycystic kidney disease | autosomal recessive polycystic kidney disease |
Myopathy | Sjogren's syndrome |
Sjogren's syndrome | Myopathy, systemic scleroderma, Behcet's disease, systemic lupus erythematosus |
DO Disease Category | Top 5 associated DO Disease Categories | Normalized average score of disease pairs category-wise |
Nervous system disease | Nervous system disease | 0.0223 |
Cardiovascular system disease | 0.0221 | |
Musculoskeletal system disease | 0.0200 | |
Physical disorder | 0.0085 | |
Disease of metabolism | 0.0025 | |
Physical disorder | Physical disorder | 0.014 |
Nervous system disease | 0.0085 | |
Disease of metabolism | 0.0052 | |
Disease of cellular proliferation | 0.0038 | |
Endocrine system disease | 0.00056 | |
Disease of cellular proliferation | Disease of cellular proliferation | 0.00400 |
Respiratory system disease | 0.00382 | |
Physical disorder | 0.00380 | |
Endocrine system disease | 0.000620 | |
Cardiovascular system disease | 0.000621 | |
Urinary system disease | Urinary system disease | 0.00109 |
Disease of cellular proliferation | 0.00062 | |
Endocrine system disease | 0.00059 | |
Gastrointestinal system disease | 0.00054 | |
Disease by infectious agent | 0.00048 | |
Endocrine system disease | Endocrine system disease | 0.000920 |
Disease of cellular proliferation | 0.000626 | |
Urinary system disease | 0.000596 | |
Musculoskeletal system disease | 0.000580 | |
Cardiovascular system disease | 0.000564 | |
Disease by infectious agent | Disease by infectious agent | 0.00072 |
Endocrine system disease | 0.000486 | |
Nervous system disease | 0.000485 | |
Respiratory system disease | 0.000482 | |
Gastrointestinal system disease | 0.000475 |
Disease with category | Top 10 associated disease pairs | Category of associated disease pair | Association score |
Diabetes mellitus Endocrine system disease |
diabetic neuropathy | endocrine system disease | 0.003613 |
hypoglycaemia | endocrine system disease | 0.003495 | |
acute myocardial infarction | cardiovascular system disease | 0.000944 | |
diabetic retinopathy | nervous system disease | 0.000938 | |
stomach cancer | disease of cellular proliferations | 0.000918 | |
kidney failure | urinary system disease | 0.000728 | |
Hypothyroidism | endocrine system disease | 0.000649 | |
disease of metabolism | disease of metabolism | 0.000627 | |
autoimmune disease | musculoskeletal system disease | 0.000603 | |
brain cancer | disease of cellular proliferations | 0.000567 | |
Cardiovascular disease Cardiovascular system disease |
intrinsic asthma | respiratory system disease | 0.043286 |
nephrotic syndrome | urinary system disease | 0.001028 | |
vascular disease | cardiovascular system disease | 0.000399 | |
disease by infectious agent | parasetic infectious disease | 0.000376 | |
vein disease | cardiovascular system disease | 0.000359 | |
generalized atherosclerosis | cardiovascular system disease | 0.000353 | |
Moyamoya disease | cardiovascular system disease | 0.000351 | |
peripheral artery disease | cardiovascular sys. disease | 0.000347 | |
Epilepsy | nervous system disease | 0.000331 | |
intermediate coronary syndrome | cardiovascular system disease | 0.000320 |