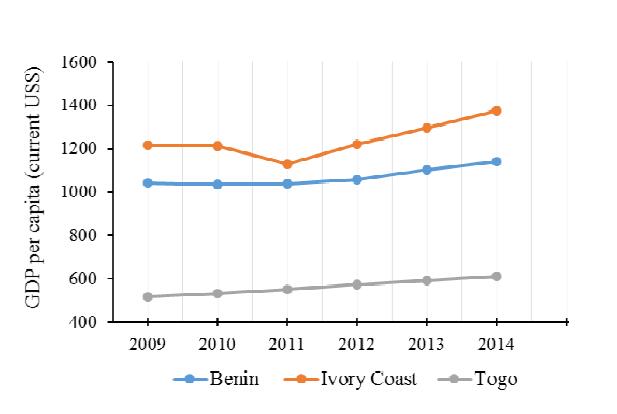
In several regions worldwide, demand for electricity can be highly dependent on weather conditions. This study investigates the relationships between weather and electricity consumption in three West African cities. Monthly electricity consumption datasets for the cities of Abidjan (Ivory Coast), Cotonou (Benin) and Lomé (Togo) for the 1990–2015, 2000–2015 and 2008–2014 periods respectively were collected from national electricity companies, and meteorological data of the synoptic stations were used to compute Cooling Degree-Days in the three cities. The Cooling Degree-Days indices were estimated using air temperature and two temperature indices (the Humidex and the Heat Index). For the statistical analysis, classical multiplicative decomposition was applied to consumption data for subperiods for which consumption was considered to show relatively homogeneous evolutionary behavior (Abidjan and Lomé from 2011 to 2014 and Cotonou from 2009 to 2014). Regardless of the temperature indices considered in the three cities, the Cooling Degree-Days indices are well correlated with the seasonal variability of power consumption and particularly, the peak consumption observed in March and the lower consumption in August. Slightly better correlations are obtained for Cotonou and Abidjan when the heat index (combining both temperature and relative humidity) are used to calculate the Cooling Degree-Days.
Citation: Ghafi Kondi Akara, Benoit Hingray, Adama Diawara, Arona Diedhiou. Effect of weather on monthly electricity consumption in three coastal cities in West Africa[J]. AIMS Energy, 2021, 9(3): 446-464. doi: 10.3934/energy.2021022
[1] | Rahaman Abu, John Amakor, Rasaq Kazeem, Temilola Olugasa, Olusegun Ajide, Nosa Idusuyi, Tien-Chien Jen, Esther Akinlabi . Modeling influence of weather variables on energy consumption in an agricultural research institute in Ibadan, Nigeria. AIMS Energy, 2024, 12(1): 256-270. doi: 10.3934/energy.2024012 |
[2] | Lamya Lairgi, Rachid Lagtayi, Yassir Lairgi, Abdelmajid Daya, Rabie Elotmani, Ahmed Khouya, Mohammed Touzani . Optimization of tertiary building passive parameters by forecasting energy consumption based on artificial intelligence models and using ANOVA variance analysis method. AIMS Energy, 2023, 11(5): 795-809. doi: 10.3934/energy.2023039 |
[3] | Lilias Makashini, Austine Ng'ombe, Henry Abanda, Albert Malama, Priscilla Mudenda . Household lifestyle, energy related practices and perceptions of energy efficiency: Evidence from Kitwe, Zambia. AIMS Energy, 2014, 2(3): 276-294. doi: 10.3934/energy.2014.3.276 |
[4] | Degaga Abdi, Addisu Bekele, Chandraprabu Venkatachalam, Mohanram Parthiban . Energy performance analysis of pasta and macaroni factory-a case study. AIMS Energy, 2021, 9(2): 238-256. doi: 10.3934/energy.2021013 |
[5] | Alemayehu T. Eneyaw, Demiss A. Amibe . Annual performance of photovoltaic-thermal system under actual operating condition of Dire Dawa in Ethiopia. AIMS Energy, 2019, 7(5): 539-556. doi: 10.3934/energy.2019.5.539 |
[6] | Mburamatare Daniel, William K. Gboney, Hakizimana Jean de Dieu, Akumuntu Joseph, Fidele Mutemberezi . Empirical assessment of drivers of electricity prices in East Africa: Panel data experience of Rwanda, Uganda, Tanzania, Burundi, and Kenya. AIMS Energy, 2023, 11(1): 1-30. doi: 10.3934/energy.2023001 |
[7] | Kaovinath Appalasamy, R Mamat, Sudhakar Kumarasamy . Smart thermal management of photovoltaic systems: Innovative strategies. AIMS Energy, 2025, 13(2): 309-353. doi: 10.3934/energy.2025013 |
[8] | Norman Maswanganyi, Edmore Ranganai, Caston Sigauke . Long-term peak electricity demand forecasting in South Africa: A quantile regression averaging approach. AIMS Energy, 2019, 7(6): 857-882. doi: 10.3934/energy.2019.6.857 |
[9] | Mohamed Elweddad, Muhammet Güneşer, Ziyodulla Yusupov . Designing an energy management system for household consumptions with an off-grid hybrid power system. AIMS Energy, 2022, 10(4): 801-830. doi: 10.3934/energy.2022036 |
[10] | Araz Alizadeh, MohammadBehshad Shafii, Alireza hajiseyed Mirzahosseini, Abtin Ataei . Experimental and simulation investigation of pulsed heat pipes in gas compressors. AIMS Energy, 2020, 8(3): 438-454. doi: 10.3934/energy.2020.3.438 |
In several regions worldwide, demand for electricity can be highly dependent on weather conditions. This study investigates the relationships between weather and electricity consumption in three West African cities. Monthly electricity consumption datasets for the cities of Abidjan (Ivory Coast), Cotonou (Benin) and Lomé (Togo) for the 1990–2015, 2000–2015 and 2008–2014 periods respectively were collected from national electricity companies, and meteorological data of the synoptic stations were used to compute Cooling Degree-Days in the three cities. The Cooling Degree-Days indices were estimated using air temperature and two temperature indices (the Humidex and the Heat Index). For the statistical analysis, classical multiplicative decomposition was applied to consumption data for subperiods for which consumption was considered to show relatively homogeneous evolutionary behavior (Abidjan and Lomé from 2011 to 2014 and Cotonou from 2009 to 2014). Regardless of the temperature indices considered in the three cities, the Cooling Degree-Days indices are well correlated with the seasonal variability of power consumption and particularly, the peak consumption observed in March and the lower consumption in August. Slightly better correlations are obtained for Cotonou and Abidjan when the heat index (combining both temperature and relative humidity) are used to calculate the Cooling Degree-Days.
Access to electricity has become a sine qua non condition for strengthening economic activities and improving people's living standards. Agricultural and industrial production is optimized through the use of electricity. Families require electricity for many uses, including housekeeping, education and economic activities at home. Basic infrastructure, such as hospitals, requires electricity for cooling, sterilization and refrigeration [1]. Electricity plays an important role in development and is a key component of economic growth [2]. Its access affects the economy and countries' progress, as it is closely linked to poverty, mostly in developing countries such as those of Africa. Admittedly, the proportion of electrification in Africa is lower than that in other regions of the world. According to the United Nations Development Program (UNDP), the share of electrification in Africa is estimated at 42%, compared to 75% worldwide [2]. In sub-Saharan African countries, the situation is more precarious, with 30% electrical coverage found in urban areas versus 14% found in rural areas [2]. Furthermore, in West Africa, the rate of electrification over years before 2012 was roughly only 20% [3], showing a significant difference between rates of access in urban (40% on average) and rural areas (between 6% and 8%) [4,5].
According to the World Bank, the proportion of population having access to electricity was in 2014, 34% in Benin, 46% in Togo and 62% in Ivory Coast [6]. In 2018, the share of electricity consumers has increased compare to that of 2014 to 42%, 51% and 67% respectively in Benin, Togo and in Ivory Coast.
This increase in the access to electricity is mainly due to the rising of population in urban areas, especially in West African capitals and megacities. Between 2010 and 2014 the share of population with access to electricity has increased from 80% to 85% in Abidjan, from 66% to 84% in Lomé, and from 55% to 69% in Cotonou [6]. Within a country, the access to electricity in the capital is much higher than in other urban areas [6]. The increase of electricity demand is linked to the increase of comsumers, especially in urban areas.
National production of electricity is mainly provided by thermal groups, which limit the orientations set out by states of the region to promote emergence by 2030 [4,7].
The West African subregion has abundant reserves of fossil fuels such as coal, oil and natural gas. This part of the world is also rich in natural and sustainable energy resources, including variable renewable energy (VRE) sources such as wind, solar and water [8]. In line with the conclusions of the last Conferences of the Parties (COP) 21 and 22 held in Paris and Marrakech, respectively, a high use of VRE is in fact the only way to improve access to electricity in Africa without having a negative impact on the climate [8].
However, the variability and intermittent nature of VREs, from the subdaily to multidecadal timescales, will increase the complexity of managing electricity systems. To identify and support efficient electrification pathways with high shares of VRE, it is important to have adequate knowledge and understanding of weather variability and its possible effects on electricity systems. This especially involves characterizing the multiscale variability of production from VRE and of electricity demand.
The weather sensitivity of electricity systems is also important to characterize in the context of climate variability and change. Climate variability and change are obviously expected to have an impact on water resources, wind and solar radiation and therefore on potential VRE production [9]. Climate change is also expected to modify demand and the risk of electricity system failure. An increase of the per capita consumption is for instance expected in a number of regions. This would result from more frequent and extreme temperatures (heat waves), which could cause higher peaks of energy demand for cooling needs and, as a result, cause the malfunctioning of thermal power plants due to overheating (e.g., [10,11,12,13]). This is expected to be the case in West Africa especially [14].
In the present work, we focus on the urban electricity consumption of coastal regions in West Africa. We explore its sensitivity to weather variability. To date, there are very few studies of Africa and even fewer of West Africa that address this issue. Better understanding of this weather sensitivity is expected to allow for the development of prediction models to be used for demand forecasts, which in turn could support the operational management of electricity systems in the region. Additionally, such work is expected to estimate how electricity demand could change as a result of climate change in the region.
The dependence of electricity consumption on climatic factors has been highlighted in a number of regions worldwide. A first factor is daylight time [15]. The period of the day when lighting is required is determined by this astronomical factor. In contrast to high latitude regions, where large variations in daylight duration occur between seasons, no meaningful result is expected in the case of tropical regions. Seasonal and day-to-day variations in weather can be important drivers of electricity consumption [16]. Atmospheric temperature is presented as the main weather driver of electricity consumption worldwide (e.g., [15,17]. Within high-latitude countries (which differ from our context), higher consumption for heating is observed in the winter. There is increased consumption during hot cycles for cooling. In both cases, consumption is extremely sensitive to air temperature. The relationship between temperature and consumption is nonlinear. This relationship is classically modeled at a daily time step with a threshold-based approach. When the daily mean temperature is lower or higher than the thermal comfort temperature threshold, the energy demand for heating or cooling is often considered to be proportional to the difference between both temperatures [18,19].
Various temperature indices have been proposed to highlight dependence on meteorological factors. Heating and cooling degree days allow the energy sector to frequently measure consumers' energy demand for heating/cooling [21]. Based on this, several definitions of these temperature indices have been proposed; however, they are usually calculated on a day-to-day basis and are generated from daily average, daily minimum and maximum and hourly temperatures [18].
In addition to temperature, other weather variables can influence electricity demand, including cloudiness, solar radiation, wind speed, humidity and precipitation (e.g., [18,20,21,22]). Weather variables are sometimes reported to have a joint effect on consumption. For example, in Togo in West Africa, higher electricity consumption is, for instance, obtained during dry periods for high values of daily maximum temperature and relative humidity in the air [23]. Under high temperature conditions, humidity intensifies the sensation of heat and thus the demand for cooling via air conditioning. Similarly, during cold weather, strong winds increase the sensation of coldness and thus the need for heating. Temperature/humidity or temperature/wind weather variables are thus sometimes considered in combination to produce integrated weather indices that better explain consumption variations (e.g., [20,21]).
The weather-consumption relationship can be rather difficult to identify from weather and consumption data. The sensitivity of consumption to weather can change over time. For instance, Bertrand and Reysset [26] recorded a change in French residents' electricity consumption patterns with a drop in heating needs in recent years but an increase in summer cooling needs for air conditioning. This evolution was attributed to regional warming in recent years. For Italy, the sensitivity of consumption to temperature was identified by Scapin et al. [22], and their study presented a strong positive trend between 1990 and 2013, increasing by a factor of 3.5. This increase, much greater than the increase in Italian total electricity demand, was related to increased equipment used for air conditioning. The main factors that influence electricity consumption are obviously socioeconomic and technical [27,28]. Western African cities are shaped by demographics, gross domestic product (GDP), and urbanization [16,18,29]. Consumption data, from which the relationship must be estimated, therefore present temporal variations that are not only shaped by weather. Some preprocessing work must therefore be done to remove temporal variations in consumption data that are due to nonclimate factors. For example, Scapin et al. [22] related Italian residents' national electricity demands to climate variables, such as temperature and solar radiation, for the 1990–2013 period. The authors fitted to the raw consumption data a 3-degree polynomial trend. Assumed to represent the nonclimatic part of consumption variations, this trend model was used to normalize daily consumption data, which in turn highlighted the strong relationship between normalized data and degree days (DDs). Similar preprocessing work is carried out in the present analysis.
The current study focuses on the analysis of electricity consumption sensitivity to weather in Abidjan, Cotonou and Lomé, three cities located within the tropical climatic zone. In this context, the aim is to determine how variation in electricity consumption is linked to changes in cooling degree days (CDDs). To address this, we estimated different CDDs based on raw temperature and other temperature indices. These indices include the Humidex and heat index, which are both calculated from combinations of temperature, humidity and dew point [21,24,25].
In section 2, we present the study area and the consumption and weather data. Then, a description of the different weather indices used to estimate the CDDs is done. The relationships between CDDs and consumption are described and commented in section 3, and the paper concludes in section 4.
The investigations related to this research work were conducted in (3) capitales (Abidjan, Cotonou and Lomé) located on the western coast of Africa (Figure 1).
These three cities were selected given their political and economic importance [30]. Their selection was also guided by the fact that the cities are characterized by precarious energy coverage, low electricity access and a lack of related studies linking weather or climate change to energy conditions [31,32]. This explains why the countries' (Ivory Coast, Togo and Benin) annual electricity production levels are low relative to those found across the globe, with values of 6688 million Kwh/year, 1529 million Kwh/year and 154 million Kwh/year, respectively [33]. Among the three countries, Togo has been selected as a pilot country for pursuing the Seventh Sustainable Development Goals in West Africa (ensuring access to clean affordable, reliable, sustainable and modern energy) [34].
On the other hand, the cities were selected based on their lower development levels based on the premise that increasing electrification rates by closing the access gap in these countries with affordable sustainable energy will also improve economic, education, health and job growth levels.
Abidjan, the economic capital of the Ivory Coast, had approximately 4, 707, 404 inhabitants in 2014, and the population of Cotonou, the economic capital of Benin, had a population of 1, 720, 105 in 2013, with Lomé having a population of approximately 1, 570, 283 in 2014 [35]. The economic growth rate of these three cities was approximately 4.2% in 2015 [30], with an estimated rate of urbanization of between 3.7% and 4.2% per year from 2000 to 2015. The country contributions of GDP were 9.2%, 2.1% and 5.4% in 2015 [36].
During the period 2009-2014, an increase in GDP per capita is recorded in the study area. In average, the GDP per capita is about 500 US dollars (US$) in Togo and 1000 US$ in Benin. In Ivory Coast, two trends were noted: a first decreasing trend from 2009 to 2011 with an average of 1250 US$ associated with social crisis and political instability, a second increasing trend from 2011 to 2014 (1400 US$ in average). This increase of GDP is related to the economic growth in terms of commercial and other socio-economic activities and is known to have the same trend than the electricity consumption [16].
Abidjan, Cotonou and Lomé are located in the humid region of the Guinean tropical climate zone. In Abidjan, the climate is characterized by a tropical monsoon climate with a short dry season. Lomé and Cotonou have a tropical savannah climate marked by a longer dry season. For these coastal cities, annual rainfall varies between 1100 and 1600 mm. In terms of relative humidity, values range from 80 to 95%. Like most coastal countries in West Africa, the countries in which these cities are located are bounded to the south by a coastal plain. The two types of wet and dry seasons observed are the consequence of the interaction of two migrating air masses following a constant cycle of atmospheric dynamics [38].
The mean annual temperature variation in these cities is over 26.5 ℃. Temperatures usually range from 22 ℃ to 32 ℃ per year [39]. The dry season covers the months of January to May. However, heat waves (beyond the threshold of 29 ℃) extend from February to March [40]. The monthly average temperature from July to September (JAS) is approximately 25 ℃ [40]. In the study areas, the dew point temperature frequently used for the measurement of thermal comfort ranges between 12 and 28 ℃.
Data on electricity consumption for Abidjan, Cotonou and Lomé were obtained from the national electrical companies of each country (CEET in Togo, SBEE in Benin and CIE in Ivory Coast). Monthly electricity consumption data were acquired for 2008–2015, 1990–2015 and 2000–2015 for Lomé (Figure 5a), Abidjan (Figure 3a) and Cotonou (Figure 4a), respectively. For the three cities, GDP growth is the most often factor used to explain the increase in electricity consumption. GDP growth results in regional population growth and improved quality of life [27]. The raw data time series of electricity consumption illustrated for these three cities (Figures 3a, 4a and 5a) shows a nonsmooth and gradual increase over time, which is reflected by discontinuities and breaks. For the three sites, in addition to the growth trend, there are strong seasonal variations in consumption. This seasonality is characterized by high values in the dry seasons and remarkably low values in the wet seasons.
In addition to overall growth and seasonal variations, Abidjan's electricity consumption from 1990 to 2015 showed a number of phases that correspond to each of the four subperiods (Figure 3). Within the individual time periods, the curve's behavior is approximately homogenous (stable or gradual increment), while the consumption pattern varies greatly from one subperiod to the next, in some cases sharply. Specifically, an initial pattern is found for 1990 to 1999. This pattern is more or less static with fairly stable yearly consumption. After an abrupt increase in 1999, a second period, still roughly stationary, extends to 2002. Third and fourth phases are observed from 2002–2010 and 2010–2015, respectively, and involve fast consumption growth. The two time periods are divided by the previous one with a sharp drop in demand (2003 and 2010).
Various economic and social indicators are probably the cause of this temporal evolution [28].
The decrease in 2003 is likely a response to unstable political conditions in Ivory Coast between 2002 and 2004. According to the Technical Distribution Department (TDD) of the power company CIE, the incident in 2003 occurred at the epicenter of the country's political crisis in 2002, leading to plant closures and then to a decrease in electricity consumption.
The three phases of instability listed below explain the drop in 2010 occurring due to a postelectoral crisis in the country:
-First, the period running from January to April 2010, referred to as the postelection crisis, impacted socioeconomic activity as well as electricity consumption;
-Second, in the beginning of 2010, load shedding occurred due to damages to a 150 MW turbine used to generate electricity;
-Last, in October 2010, political instability from the postelectoral crisis; residents moving and plants, businesses and administrations closing resulted in lower electricity consumption.
An analysis of the year 2015 was not done for the considered period even though a singular point occurs in a specific month of this year.
The temporal trend of power demand in Cotonou for 2000 to 2015 (Figure 4) is significantly smoother and more gradual than that for Abidjan (Figure 3). The results show a noticeable upward trend with strong seasonality and increased demand in the winter. Despite being less marked than those of Abidjan, 3 distinct periods are identified: there are two principal subperiods with a consistent and uniform increase (2000_2005 and 2008_2015) that are separated by a transitory phase (2006–2007) characterized by a moderately low increment and lower levels of seasonal variability. No definite social, economic or technical explanations for the suspected discontinuity between 2006 and 2008 could be found so far.
Removing the breakdown in raw electricity data as done above, prior to using the demand time series, prevents problems associated with data. This capacity provided by Lomé's electricity demand time series for 2008 to 2014 (Figure 5) enables us to choose the 2011 to 2014 year interval as our working period.
For the cities of Lomé, Cotonou and Abidjan, the temporal evolution of monthly consumption is primarily driven by nonweather factors. These non-climate factors are among those linked to the socio-economic status such as population and GDP. Referring to Figure 1, it is clear that the per capita GDP's profile over the study period at the country level follows the same trend as that for the smoothing curve of electricity consumption of the relevant country's city. To avoid including those nonweather factors in the analysis, in the first place, we removed from the main time series the subperiods for which consumption's evolution was observed. This means that an upward trend can occur, although it should be gradual without sudden fluctuations from one year to the next. The working periods selected are therefore 2011–2014 for Abidjan and Lomé and 2009–2014 for Cotonou. For these 3 cities, the periods we consider are similar in terms of their behavior and the number of years covered (ranging from 4 to 6 years). Our study of the part of consumption related to weather conditions led to the omission of consumption drift considered to be related to the economic growth rate of each country. The first step involved standardizing the consumption data. Specifically, a trend model was fitted to raw consumption data named C(t) for the study period. To remove the aforementioned trend (T(t)), we proceeded as described below: Each monthly data item was divided by the corresponding monthly value obtained from the trend model. The normalized consumption for day t called Nc(t) (Figure 6) is expressed as shown below. It should be noted that for normalization, we used the classical multiplicative time-series decomposition method.
NC(t)=C(t)T(t), where C(t) is the monthly consumption and T(t) is the trend value of monthly consumption for month t [17,22] | (1) |
The subtractive approach could also have been used for normalizing, where the trend is deducted from the raw data. For example, Bessec et al. [17] and Scapin et al. [22] propose this form of additive decomposition, which assumes that the climate-related portion of the demand is temporally stable, whereas this is most likely not the case. The increase in revenue generation is assumed to be due to an increase in air conditioning facilities and thus a greater dependence on weather conditions (e.g., [22]). Using multiplicative decomposition, we hypothesized that the intensity of seasonal and discontinuous variations grows as the trend increases. In other words, sensitivity to meteorological variables is assumed to also rise over time and to be fairly similar to the long-term drift. While this is not evident for Abidjan due to the significant extent of this socioeconomic problem over time, the hypothesis appears to be valid for Cotonou and Lomé for the entire range of the data used. Hence, multiplicative decomposition is a reasonable hypothesis in this case [41].
In the following, CDDs are computed on a daily basis using different weather indices: temperature, the humidex and the heat index. When a CDD is calculated from temperature, we can use its daily average directly (Tm) or both its minimum (Tn) and maximum (Tx) for the calculation [42]. If the humidex or heat index is considered, we then use the daily dew point temperature (Td) and daily relative humidity (R). The meteorological data used were obtained at a daily time step from synoptic stations of the respective weather services in each of the considered cities. We obtained data for 1980–2014 for the three cities.
-The Humidex (HU) is an index of temperature established by the Weather Service of Canada in 1979 to characterize how people perceive the weather based on the combined impacts of heat and humidity. The index is a very popular tool for calculating human discomfort in the summer as equivalent to dry temperature [43].
This temperature- and humidity-based index was applied to define the degree of heat discomfort (Figure 4). A slight risk of discomfort is observed when the humidex values are in the range of 20 to 29 ℃; a moderate degree of discomfort is obtained at humidex values of 30 to 39 ℃; and the greatest discomfort occurs when the humidex reaches or surpasses 46 ℃ (hazardous conditions) [44].
-The heat index (HI), which George Winterling proposed in 1978 [25], is defined similarly to the humidex. This thermal index can also be considered to represent the temperature felt by the body through the combined effects of relative humidity and air temperature [45]. The heating and cooling degree days are commonly used in energy systems to estimate heating and cooling energy consumption in buildings [46].
While heating degree days focus on heating requirements during cold weather, cooling degree days focus on cooling requirements during hot weather. Cooling degree days are a measure of the degree (in terms of degrees) and period of time (in terms of days) in which the outdoor air temperature has exceeded a certain level [47]. For a given time period, the measure assesses the cumulative temperature that exceeds (for CDDs) or goes below (for HDDs) a predetermined temperature called the threshold temperature [48].
CDDs may be estimated on a per day basis. Various formulas have been developed the basis of various temperature data. The CDD, when calculated from daily mean temperature Tm, is expressed as:
wherever hourly temperature information is available, a similar formula is applied to each hour of the day, whereby CDDs for the whole day are generated as an average of all hourly estimates. In the latter case, the calculation relies on minimum (Tn) and maximum (Tx) daily air temperatures, which are also frequently available [49,50].
Index | Formulae |
Humidex (HU) | HU = T + R, where (2)
T is the temperature under normalized shelter (℃); R is relative humidity: R = 0.55 * (e − 10), e issaturatingvaporpressure e=6.11∗e(5417.75∗((1273.16)−(1Td))); with Td is dew point temperature (℃). |
Heat Index (HI) | Heat Index(HI)=−42.37+2.05T+10.14R−0.22TR−6.83∗10−3T2−5.48∗10−2R2+1.23∗10−3T2R+8.531∗10−2TR2−1.99∗10−6T2R2(3)
with T denoting air temperature (°F) and R is relative humidity (%) |
Cooling Degree Days (CDD) | CDD(Tm) = (Tm – Tr) if Tr < Tm CDD(Tm) = 0 if Tm < Tr (4) Tr is the threshold temperature. No consensus exists on this threshold value, as this threshold can differ from one region to the other. It is, however, often taken to be 25℃. |
CDD(Tx,Tn)=((Tx+Tn)2)–Tr) if Tr<Tn
CDD(Tx,Tn)=(Tx−Tr)∗(0.08+0.42∗(Tx−Tr)(Tx−Tn)) if Tn<Tr<Tx CDD(Tx,Tn)=0 if Tx<Tr(5) |
Here, we will look at 6 possible different assessments of CDDs based on several available CDD expressions and on a variety of temperature indices, such as air temperature, the humidex and the heat index. For the first three CDD calculations, with Eq (4) the CDD value is determined using the mean temperature for each day. In this case, both the daily Humidex value and heat index are obtained from daily values of air temperature, relative humidity and dew point temperature. These assessments using air temperature, the Humidex and the heat index are denoted as CDDTm, CDDHUm and CDDHIm, respectively.
Each of the remaining three categories of CDDs utilizes the minimum and maximum temperature values made available each day. See Eq (5) above. With this approach, the Humidex index is calculated from minimum and maximum daily temperatures in turn. Both Humidex values for individual days are applied as temperature index values in Eq (5) by replacing Tx and Tn. Similar steps are applied to the Heat Index (HI). An estimate of the heat index is made for the minimum and maximum temperatures for a given day. These two estimates are used for the calculation of the heat index in Eq (5). The CDD estimates obtained from the minimum and maximum temperatures, Humidex and heat index are referred to as CDDTxn, CDDHUxn and CDDHIxn, respectively.
CDDs were estimated at a daily time interval. The daily time series of CDD estimates were agammaegated to monthly time intervals. The relationship between the calculated monthly CDDs and the monthly normalized consumptions was then evaluated for each case.
The time series of normalized monthly electricity consumption for the localities of Abidjan, Cotonou and Lomé using the classic multiplicative decomposition of the time series (formula 1) are displayed below in Figure 6.
The interannual variation in the normalized electricity consumption time series (Figure 6) shows the same behavior in terms of curve variations from one year to the next. From Figure 6, there is an annual peak for each city in March and a low point in August of each year. The approximately consistent seasonal variations across the whole period confirm the multiplicative decomposition hypothesis proposed previously.
Figure 7 below illustrates the relationship between the monthly CDDs estimated from the different temperature indices above and monthly normalized consumptions.
Figure 7 presents the interannual evolution of monthly CDDs and monthly electricity consumption for the cities of Lomé (C), Cotonou (B) and Abidjan (A). For these three areas, there is a rise in both consumption and temperature indices from January, with a peak in March (maximum point) and a downturn from May to August (minimum point). Regardless of the temperature indices, consumption patterns essentially followed the same seasonal variations as those of the CDDs. Consequently, the temperature indices and electricity consumption fluctuate simultaneously. The fact that consumption depends on CDDs means that by knowing their values, one can predict, to a certain extent, the monthly demand of the population in terms of electricity consumption. In other words, if NC(t) depends on CDDs, we have function f such that NC(t) = f (CDDs). Notably, the interannual variation in consumption is most similar to that of the CDDs calculated directly from the average temperature. As a consequence, temperature appears to be the variable that best predicts demand for electricity consumption. At first glance, it is easy to see that there is the same predictive ability regardless of the predictor chosen. It should also be noted that, depending on the cases mentioned above, it is possible to reproduce the seasonality of consumption. The scatter plots below (Figure 7) illustrate this point as well and show estimated monthly cooling consumption as a result of the estimated monthly CDD differences.
The scatter and correlation diagrams presented in Figure 8 were computed to confirm the aforementioned relationship between consumption and CDDs calculated using all temperature indices presented above. Figure 8 shows that regardless of the city, there is a liner relationship between consumption and CDDs calculated using temperature (CDDTm and CDDTxn) meaning that there is a positive correlation between temperature and electricity consumption. For CDDs calculated using the Humidex index (CDDHUm and CDDHUxn), we notice that they are slightly scattered, and in this case, the linear correlation between comsumption and humidity is weak. For CDDs computed with the heat index (CDDHIm and CDDHIxn), the correlation with consumption are also weak but they are higher than that of the Humidex. Consequently, there is a relationship between the power consumption and the temperature and humidity.
To conclude, from the above confirmation of correlation, we conduct a statistical analysis to this end by considering the Pearson correlation coefficient (r) between CDDs and monthly consumption in Table 2.
CDDTm | CDDHUm | CDDHIm | CDDTxn | CDDHUxn | CDDHIxn | |
Abidjan power consumption | 0.76 | 0.75 | 0.75 | 0.76 | 0.75 | 0.75 |
Cotonou power consumption | 0.78 | 0.73 | 0.75 | 0.78 | 0.73 | 0.78 |
Lomé power consumption | 0.66 | 0.59 | 0.63 | 0.66 | 0.59 | 0.63 |
In all cases, the correlation coefficient between CDDs and power consumption shows high significant values greater than 0.59 (p-value of lower than 0.001). For the thress cities, there is no significant difference between CDDs using mean temperature and those from both minimum and maximum temperatures(CDDTm, CDDTxn).
For Abidjan, the best correlations are found using temperature data only. Nevertheless, the values obtained with the other weather predictors are not so different. For Lomé, better relationships between weather and power comsumption are observed when considering CDD with temperature only. For Cotonou, the best values are those with CDD from the both minimum and maximum heat index (CDDHIxn), and from both minimum and maximum temperature (CDDTxn). The values are considerably higher than those obtained for Abidjan and Lomé, indicating the importance of humidity to Cotonou's consumption. The relationships between power compsumtion and weather are weaker when using CDD with Humidex.
In summary, the power consumption is sensitive to weather conditions in these three West African cities. The obvious consequence of hot periods is a sharp increase in consumption. This is likely related to the use of cooling equipment to improve thermal comfort (use of fans or air conditioners). For the cities of Cotonou and Abidjan, high correalation values between consumption and CDD from heat index suggest that humidity may also influence the variations of power comsumption.
Electricity consumption is affected by several factors, such as GDP, population growth and increasing urbanization. In this paper, the relationship between electricity consumption in three West African coastal cities and weather is established. It was not possible to perform an econometric study because there are not enough data at relevant scale to do such a study and the aim of this manuscript is not to look at the causes of the long-term trend that can be explained by the increase in population and economic growth. For this purpose, we worked on standardized chronicles in which we removed the trend that can be explained by GDP, demographics, standard of living or other socio-economic factors. The general objective of this paper is to highlight the link between weather and electricity consumption and specifically, to identify which weather variable among CDDs computed with Temperature (CDDTm and CDDTxn), with humidex (CDDHUm and CDDHUxn) or with HeatIndex (CDDHIm and CDDHIxn) is more related to variations of power consumption.
Normalized consumption data for Abidjan, Cotonou and Lomé highlight strong seasonality with a high peak in March and a drop in August. The relationship between consumption and weather was explored in reference to cooling degree days, reflecting the need for additional consumption for building cooling with every degree Celsius increase in temperature. CDDs were elaborated from different temperature indices based on daily temperature and then with relative humidity (the heat index and humidex). For these three cities, a strong relationship exists between temperature and consumption. Humidity is another important driver of demand, mainly for Cotonou and Abidjan. In this case, how humidity and temperature are combined to produce the equivalent temperature index also matters: the heat index indeed has significantly better explanatory power than the Humidex.
These preliminary results call for further investigation. The results are likely dependent on the threshold temperature used in the CDD estimations. A sensitivity analysis of this threshold should be carried out to determine which temperature threshold would lead to the best relationship between consumption and CDDs. The sensitivity of consumption to other weather predictors could also be explored. Wind speed and solar radiation may also be relevant predictors in this context. In the present case, we examined a very small fraction of available consumption data. A better use of full consumption time series at a daily time scale may improve weather sensitivity characterization.
Our findings are restricted to the three cities of Abidjan, Cotonou and Lomé. An extension of this work to other urban areas will be important and useful in establishing its extent to the regional level. The level of weather sensitivity found for these three cities is consistent with those found in a number of other studies, such as those mentioned in the introduction. The presented results are also in line with those obtained by [51] for Lomé, Togo, within the same climatic region. In [51], the authors find strong dependence, for the dry season, of electricity demand on maximum air temperature and minimum relative humidity. However, Togo is located close to Abidjan, and why relative humidity does not exert the same influence in both cases is worth investigating.
Most importantly, the relationships identified from these analyses could lead to the development of an electricity consumption forecasting model in this area. To investigate further the relationship between weather variables and consumption at daily scale, we can assumed that the relationship between consumption D and Weather M can be expressed as an affine relationship (D = D0 + aM) where D0 is the minimum Consumption which is independent to the weather variables and « a » the fraction of consumption due to the weather variables. However, the development and testing of such a model will require the use of high-resolution consumption data. A database of daily data would likely be useful in this context. Moreover, it is important to carry out an econometric study using relevant data to better highlight the influence of demographic (population) and economic (GDP) on the variations in electricity consumption according to the daily and weekly profile.
The present work benefited from the inputs of M. Sylvain GNAN, Prof. Romain Lucas GLÈLÈ KAKAЇ and Dr. Achille Hounkpèvi, who provided valuable comments, ideas, and assistance with data operations in the completion of this research. We thank them for directing us in the right direction. In addition, we acknowledge other contributors to the study, including the administrations of the CEA CCBAD/UFHB Postgraduate School and LASMES, who hosted us at their laboratories. Finally, we thank National Electricity (CIE, SBEE and CEET) and Meteorology Services (ASECNA) and the Higher Education Ministers of Ivory Coast, Benin and Togo for assisting with the acquisition of consumption and meteorological data used in the present work.
The research leading to this publication is funded by France National Research Institute for Sustainable Development (IRD; Institut de Recherche pour le Développement), grant number UMR IGE Imputation 252RA5".
The authors declare that there is no conflict of interest.
[1] | IRENA (2012) Prospects for the African Power Sector; 60. |
[2] |
Pineau PO (2008) Electricity sector integration in West Africa. Energy Policy 36: 210-223. doi: 10.1016/j.enpol.2007.09.002
![]() |
[3] | REN21 (2014) ECOWAS Renewable Energy and Energy Efficiency Status Report; 86. |
[4] | Karekezi S, Kithyoma W (2002) Renewable energy strategies for rural Africa: is a PV-led renewable energy strategy the right approach for providing modern energy to the rural poor of sub-Saharan Africa? Energy Policy 30: 1071-1086. |
[5] | ECREEE (2015) Politique d'Efficacité Énergétique de la CEDEAO; 74. |
[6] | World Bank Group (2021) Access to electricity (% of population). Available from: https://data.worldbank.org. |
[7] | Rojey A (2011) Comment assurer la transition énergétique; 37. |
[8] | REN21 (2016) Renewables 2016 Global Status Report; 272. |
[9] | REN21 (2015) Renewables 2015 Global Status Report; 251. |
[10] | Schipper L, Meyers S, Howarth RB, et al. (1992) Energy efficiency and human activity: Past trends, future prospects. Cambridge; New York, NY, USA: Cambridge University Press; 400. |
[11] | Akbari H (2005) Energy saving potentials and air quality benefits of urban heat island mitigation[Internet]. Ernest Orlando Lawrence Berkeley NationalLaboratory, Berkeley, 19. |
[12] | Shahmohamadi P, Che-Ani AI, Ramly A, et al. (2010) Reducing urban heat island effects: A systematic review to achieve energy consumption balance. Int J Phys Sci, 11. |
[13] |
Hamlet AF, Lee S-Y, Mickelson KEB, et al. (2010) Effects of projected climate change on energy supply and demand in the Pacific Northwest and Washington State. Clim Change 102: 103-128. doi: 10.1007/s10584-010-9857-y
![]() |
[14] | Aivalioti S (2015) Electricity Sector Adaptation to Heat Waves, 51. |
[15] | Hernández L, Baladrón C, Aguiar JM, et al. (2012) A study of the relationship between weather variables and electric power demand inside a smart grid/smart world framework. 12: 11571-11591. |
[16] |
Akinlo AE (2009) Electricity consumption and economic growth in Nigeria: Evidence from cointegration and co-feature analysis. J Policy Model 31: 681-693. doi: 10.1016/j.jpolmod.2009.03.004
![]() |
[17] |
Bessec M, Fouquau J (2008) The non-linear link between electricity consumption and temperature in Europe: A threshold panel approach. Energy Econ 30: 2705-2721. doi: 10.1016/j.eneco.2008.02.003
![]() |
[18] |
Isaac M, van Vuuren DP (2009) Modeling global residential sector energy demand for heating and air conditioning in the context of climate change. Energy Policy 37: 507-21. doi: 10.1016/j.enpol.2008.09.051
![]() |
[19] |
Franç ois B, Borga M, Creutin JD, et al. (2016) Complementarity between solar and hydro power: Sensitivity study to climate characteristics in Northern-Italy. Renewable Energy 86: 543-553. doi: 10.1016/j.renene.2015.08.044
![]() |
[20] |
Apadula F, Bassini A, Elli A, et al. (2012) Relationships between meteorological variables and monthly electricity demand. Appl Energy 98: 346-356. doi: 10.1016/j.apenergy.2012.03.053
![]() |
[21] |
Marvuglia A, Messineo A (2012) Using recurrent artificial neural networks to forecast household electricity consumption. Energy Procedia 14: 45-55. doi: 10.1016/j.egypro.2011.12.895
![]() |
[22] |
Scapin S, Apadula F, Brunetti M, et al. (2016) High-resolution temperature fields to evaluate the response of Italian electricity demand to meteorological variables: an example of climate service for the energy sector. Theor Appl Climatol 125: 729-742. doi: 10.1007/s00704-015-1536-5
![]() |
[23] | Adjamagbo C, Ngae P, Vianou A, et al. (2011) Modeling the demand for electrical energy in Togo. Rev Energy Renouvelables 14: 67-83. |
[24] |
Beccali M, Cellura M, Lo Brano V, et al. (2008) Short-term prediction of household electricity consumption: Assessing weather sensitivity in a Mediterranean area. Renew Sustain Energy Rev 12: 2040-65. doi: 10.1016/j.rser.2007.04.010
![]() |
[25] | US Department of Commerce N (2020) What is the heat index? NOAA's National Weather Service. |
[26] | Bertrand REYSSET (2008) Impact of climate change on the energy sector in France, 5. |
[27] |
Adom PK, Bekoe W, Akoena SKK (2012) Modelling aggregate domestic electricity demand in Ghana: An autoregressive distributed lag bounds cointegration approach. Energy Policy 42: 530-537. doi: 10.1016/j.enpol.2011.12.019
![]() |
[28] |
Ouédraogo IM (2010) Electricity consumption and economic growth in Burkina Faso: A cointegration analysis. Energy Econ 32: 524-531. doi: 10.1016/j.eneco.2009.08.011
![]() |
[29] |
Inglesi-Lotz R, Blignaut JN (2011) South Africa's electricity consumption: A sectoral decomposition analysis. Appl Energy 88: 4779-4784. doi: 10.1016/j.apenergy.2011.06.018
![]() |
[30] | UNECA (2016) Nineteenth (19th) session of the intergovernmental committee of experts (ice-19) of West Africa. Outcome document draft conclusions and recommendations, 8. |
[31] | IEA, OECD (2014) Africa Energy Outlook : a focus on energy prospects in Sub-Saharan Africa. World Energy Outlook Special Report. Fance: International Energy Agency, 242. |
[32] | IEA, OECD (2015) Energy and Climate Change, World Energy Outlook Special Report. International Energy Agency, 200. |
[33] | CIA (2015) World Factbook. Electricity Production/Countries of the World. |
[34] | UNOSD (2016) Seminar on Mainstreaming Energy for Sustainable Development Goals (SDGs), Targets and Indicators into Statistical Programmes in Select African Countries, 27-29 June 2016, Addis Ababa, Ethiopia. |
[35] | IEA (2014) World energy outlook 2014 factsheet: Energy in sub-Saharan Africa today. |
[36] | World Bank (2017) Where we work. World Bank. |
[37] | Perspective monde (2021) Togo-PIB par habitant ($ US constant 2010)|Statistiques. Outil pédagogique des grandes tendances mondiales depuis 1945 École de politique appliquée Faculté des lettres et sciences humaines. |
[38] | Atlas Overview (2010) Climate|West Africa, 157. |
[39] | AccuWeather (2016) Global Current Weather|AccuWeather. |
[40] | Cedar Lake Ventures, Inc (2016) The Typical Weather Anywhere on Earth-Weather Spark. |
[41] | Commonwealth of Australia (2002) Appliance Electricity End-Use: Weather and Climate Sensitivity, 69. |
[42] |
Robinson PJ (2001) On the definition of a heat wave. J Appl Meteorol 40: 762-775. doi: 10.1175/1520-0450(2001)040<0762:OTDOAH>2.0.CO;2
![]() |
[43] | Masterton JM, Richardson FA (1979) Humidex : a method of quantifying human discomfort due to excessive heat and humidity. Meteorological Service of Canada, 45. |
[44] | Rome S, Oueslati B, Moron V, et al. (2016) Heat waves in Sahel : definition and main spatial-temporal characteristics (1973-2014). In: J.-M. Fallot DJ & NB, éditeur. 29ème Colloque de l'Association Internationale de Climatologie, 345-350. |
[45] | Oueslati B, Sambou M-JG, Pohl B, et al. (2016) Sahelian heat waves: characterization, mechanisms, predictability. 29e Colloque de l'Association Internationale de Climatologie. Besanç on, France: Association Internationale de Climatologie, 327-332. |
[46] | Martin Bromley (2016) Degree Days : Understanding Heating and Cooling Degree Days. |
[47] |
Jiang F, Li X, Wei B, et al. (2009) Observed trends of heating and cooling degree-days in Xinjiang Province, China. Theor Appl Climatol 97: 349-360. doi: 10.1007/s00704-008-0078-5
![]() |
[48] | BizEE Software (2020) Degree Days—An Introduction. |
[49] |
Aceituno P (1979) Statistical formula to estimate heating or cooling degree-days. Agric Meteorol 20: 227-232. doi: 10.1016/0002-1571(79)90023-2
![]() |
[50] |
d'AMBROSIO ALFANO FR, Palella BI, Riccio G (2011) Thermal Environment Assessment Reliability Using Temperature —Humidity Indices. Ind Health 49: 95-106. doi: 10.2486/indhealth.MS1097
![]() |
[51] | Adjamagbo C, Ngae P, Vianou A (2011) Modélisation de la demande en énergie électrique au Togo, 17. |
1. | Thierry C. Fotso-Nguemo, Derbetini A. Vondou, Ismaila Diallo, Arona Diedhiou, Torsten Weber, Roméo S. Tanessong, Jean P. Nghonda, Zéphirin D. Yepdo, Potential impact of 1.5, 2 and 3 °C global warming levels on heat and discomfort indices changes over Central Africa, 2022, 804, 00489697, 150099, 10.1016/j.scitotenv.2021.150099 | |
2. | Oluwarotimi Delano Thierry Odou, Heidi Heinrichs Ursula, Rabani Adamou, Thierry Godjo, Mounkaila S Moussa, Potential changes in cooling degree day under different global warming levels and shared socioeconomic pathways in West Africa, 2023, 18, 1748-9326, 034029, 10.1088/1748-9326/acbc8f | |
3. | Maurizio Tiepolo, Andrea Galligari, Fabio Giulio Tonolo, Enrico Moretto, Silvana Stefani, LST-R: A method for assessing land surface temperature reduction in urban, hot and semi-arid Global South, 2023, 10, 22150161, 101977, 10.1016/j.mex.2022.101977 | |
4. | Qingyao Qiao, Akilu Yunusa-Kaltungo, Rodger E. Edwards, Feature selection strategy for machine learning methods in building energy consumption prediction, 2022, 8, 23524847, 13621, 10.1016/j.egyr.2022.10.125 | |
5. | Thierry C. Fotso‐Nguemo, Torsten Weber, Arona Diedhiou, Steven Chouto, Derbetini A. Vondou, Diana Rechid, Daniela Jacob, Projected Impact of Increased Global Warming on Heat Stress and Exposed Population Over Africa, 2023, 11, 2328-4277, 10.1029/2022EF003268 | |
6. | E T N’Datchoh, K Kouadio, S Silué, A Bamba, E Naabil, K B Djè, A Diedhiou, M B Sylla, S Anquetin, C Lennard, Potential changes in temperature extreme events under global warming at 1.5 °C and 2 °C over Côte d’Ivoire * , 2022, 1, 2752-5295, 015007, 10.1088/2752-5295/ac7acb | |
7. | Kagou Dicko, Emmanuel Tanko Umaru, Souleymane Sanogo, Appollonia Aimiosino Okhimamhe, Ralf Loewner, A Trend Analysis of Changes in Cooling Degree Days in West Africa Under Global Warming, 2024, 15, 2073-4433, 1376, 10.3390/atmos15111376 | |
8. | Suleman Sarwar, Ghazala Aziz, Aviral Kumar Tiwari, Implication of machine learning techniques to forecast the electricity price and carbon emission: Evidence from a hot region, 2024, 15, 16749871, 101647, 10.1016/j.gsf.2023.101647 | |
9. | Tolulope David Makanju, Thokozani Shongwe, Oluwole John Famoriji, Machine Learning Approaches for Power System Parameters Prediction: A Systematic Review, 2024, 12, 2169-3536, 66646, 10.1109/ACCESS.2024.3397676 | |
10. | Jing Huang, Dalia Patino Echeverri, Zhengfeng Zhang, Planting trees is a cost-effective way to reduce residential electricity consumption and abate atmospheric CO2, 2024, 373, 03062619, 123842, 10.1016/j.apenergy.2024.123842 | |
11. | Ghafi Kondi-Akara, Benoit Hingray, Baptiste Francois, Arona Diedhiou, Recent trends in urban electricity consumption for cooling in West and Central African countries, 2023, 276, 03605442, 127597, 10.1016/j.energy.2023.127597 | |
12. | Yusri Syam Akil, Saiful Mangngenre, Sri Mawar Said, Kifayah Amar, A. M. Shiddiq Yunus, Factors influencing urban consumers on selecting electricity saving home appliances for managing energy consumption in Indonesia, 2023, 10, 2331-1916, 10.1080/23311916.2023.2287299 | |
13. | Tolulope David Makanju, Oluwole John Famoriji, Ali N. Hasan, Thokozani Shongwe, Machine learning approaches for identifying and predicting voltage conditions in power system networks using network topology behavior input formulation., 2024, 24682276, e02493, 10.1016/j.sciaf.2024.e02493 | |
14. | Konin Pierre Claver Kakou, Dungall Laouali, Boko Aka, Janet Appiah Osei, Nicaise Franck Kassi Ette, Georg Frey, 2024, Validation of Satellite-derived Daily Sunshine Duration Estimates Against Campbell-Stokes Sensor Measurements in Côte d’Ivoire, 979-8-3503-5464-5, 1, 10.1109/IHTC61819.2024.10855142 |
Index | Formulae |
Humidex (HU) | HU = T + R, where (2)
T is the temperature under normalized shelter (℃); R is relative humidity: R = 0.55 * (e − 10), e issaturatingvaporpressure e=6.11∗e(5417.75∗((1273.16)−(1Td))); with Td is dew point temperature (℃). |
Heat Index (HI) | Heat Index(HI)=−42.37+2.05T+10.14R−0.22TR−6.83∗10−3T2−5.48∗10−2R2+1.23∗10−3T2R+8.531∗10−2TR2−1.99∗10−6T2R2(3)
with T denoting air temperature (°F) and R is relative humidity (%) |
Cooling Degree Days (CDD) | CDD(Tm) = (Tm – Tr) if Tr < Tm CDD(Tm) = 0 if Tm < Tr (4) Tr is the threshold temperature. No consensus exists on this threshold value, as this threshold can differ from one region to the other. It is, however, often taken to be 25℃. |
CDD(Tx,Tn)=((Tx+Tn)2)–Tr) if Tr<Tn
CDD(Tx,Tn)=(Tx−Tr)∗(0.08+0.42∗(Tx−Tr)(Tx−Tn)) if Tn<Tr<Tx CDD(Tx,Tn)=0 if Tx<Tr(5) |
CDDTm | CDDHUm | CDDHIm | CDDTxn | CDDHUxn | CDDHIxn | |
Abidjan power consumption | 0.76 | 0.75 | 0.75 | 0.76 | 0.75 | 0.75 |
Cotonou power consumption | 0.78 | 0.73 | 0.75 | 0.78 | 0.73 | 0.78 |
Lomé power consumption | 0.66 | 0.59 | 0.63 | 0.66 | 0.59 | 0.63 |
Index | Formulae |
Humidex (HU) | HU = T + R, where (2)
T is the temperature under normalized shelter (℃); R is relative humidity: R = 0.55 * (e − 10), e issaturatingvaporpressure e=6.11∗e(5417.75∗((1273.16)−(1Td))); with Td is dew point temperature (℃). |
Heat Index (HI) | Heat Index(HI)=−42.37+2.05T+10.14R−0.22TR−6.83∗10−3T2−5.48∗10−2R2+1.23∗10−3T2R+8.531∗10−2TR2−1.99∗10−6T2R2(3)
with T denoting air temperature (°F) and R is relative humidity (%) |
Cooling Degree Days (CDD) | CDD(Tm) = (Tm – Tr) if Tr < Tm CDD(Tm) = 0 if Tm < Tr (4) Tr is the threshold temperature. No consensus exists on this threshold value, as this threshold can differ from one region to the other. It is, however, often taken to be 25℃. |
CDD(Tx,Tn)=((Tx+Tn)2)–Tr) if Tr<Tn
CDD(Tx,Tn)=(Tx−Tr)∗(0.08+0.42∗(Tx−Tr)(Tx−Tn)) if Tn<Tr<Tx CDD(Tx,Tn)=0 if Tx<Tr(5) |
CDDTm | CDDHUm | CDDHIm | CDDTxn | CDDHUxn | CDDHIxn | |
Abidjan power consumption | 0.76 | 0.75 | 0.75 | 0.76 | 0.75 | 0.75 |
Cotonou power consumption | 0.78 | 0.73 | 0.75 | 0.78 | 0.73 | 0.78 |
Lomé power consumption | 0.66 | 0.59 | 0.63 | 0.66 | 0.59 | 0.63 |