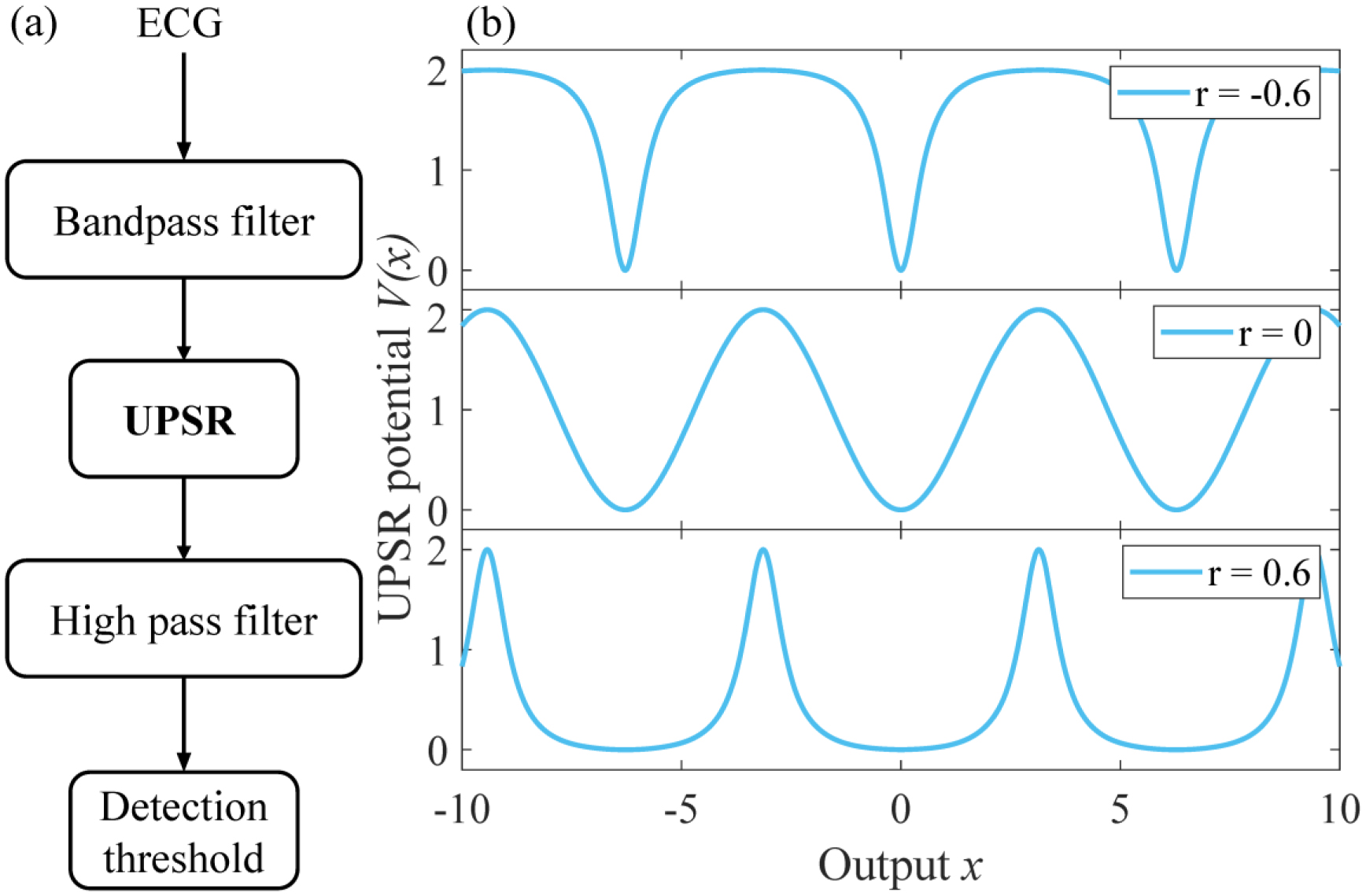
Robust QRS detection is crucial for accurate diagnosis and monitoring of cardiovascular diseases. During the detection process, various types of noise and artifacts in the electrocardiogram (ECG) can degrade the accuracy of algorithm. Previous QRS detectors have employed various filtering methods to minimize the negative impact of noise. However, their performance still significantly deteriorates in large-noise environments. To further enhance the robustness of QRS detectors on noisy electrocardiograms (ECGs), we proposed a QRS detection algorithm based on an underdamped. This method utilizes the period nonlinearity-induced stochastic resonance to enhance QRS complexes while suppressing noise and non-QRS components in the ECG. In contrast to neural network-based algorithms, our proposed algorithm does not rely on large datasets or prior knowledge. Through testing on three widely used ECG datasets, we demonstrated that the proposed algorithm achieves state-of-the-art detection performance. Furthermore, compared to traditional stochastic resonance-based method, our algorithm has increased noise robustness by 25% to 100% across various real-world environments. This enables the proposed method to maintain its optimal performance within a certain range even in the presence of additional injected noise, thus providing an excellent approach for robust QRS detection in noisy ECGs.
Citation: Zheng Guo, Siqi Li, Kaicong Chen, Xuehui Zang. Robust QRS complex detection in noisy electrocardiogram based on underdamped periodic stochastic resonance[J]. AIMS Bioengineering, 2023, 10(3): 283-299. doi: 10.3934/bioeng.2023018
[1] | Abdulmajeed Alsufyani . Performance comparison of deep learning models for MRI-based brain tumor detection. AIMS Bioengineering, 2025, 12(1): 1-21. doi: 10.3934/bioeng.2025001 |
[2] | Islam Uddin, Salman A. AlQahtani, Sumaiya Noor, Salman Khan . Deep-m6Am: a deep learning model for identifying N6, 2′-O-Dimethyladenosine (m6Am) sites using hybrid features. AIMS Bioengineering, 2025, 12(1): 145-161. doi: 10.3934/bioeng.2025006 |
[3] | Vasudeva Reddy Tatiparthi, Madhava Rao, Santosh Kumar, Hindumathi . Detection and analysis of coagulation effect in vein using MEMS laminar flow for the early heart stroke diagnosis. AIMS Bioengineering, 2023, 10(1): 1-12. doi: 10.3934/bioeng.2023001 |
[4] | Poovayar Priya Mohan, Ezhilarasan Murugesan . A novel approach for detecting the presence of a lymphatic rosary in the iris using a 3-stage algorithm. AIMS Bioengineering, 2024, 11(4): 506-526. doi: 10.3934/bioeng.2024023 |
[5] | Priya Vizzini, Lucilla Iacumin, Giuseppe Comi, Marisa Manzano . Development and application of DNA molecular probes. AIMS Bioengineering, 2017, 4(1): 113-132. doi: 10.3934/bioeng.2017.1.113 |
[6] | N. Supraja, T.N.V.K.V. Prasad, M. Soundariya, R. Babujanarthanam . Synthesis, characterization and dose dependent antimicrobial and anti-cancerous activity of phycogenic silver nanoparticles against human hepatic carcinoma (HepG2) cell line. AIMS Bioengineering, 2016, 3(4): 425-440. doi: 10.3934/bioeng.2016.4.425 |
[7] | Maria Waqas, Urooj Ainuddin, Umar Iftikhar . An analog electronic circuit model for cAMP-dependent pathway—towards creation of Silicon life. AIMS Bioengineering, 2022, 9(2): 145-162. doi: 10.3934/bioeng.2022011 |
[8] | Kayode Oshinubi, Augustina Amakor, Olumuyiwa James Peter, Mustapha Rachdi, Jacques Demongeot . Approach to COVID-19 time series data using deep learning and spectral analysis methods. AIMS Bioengineering, 2022, 9(1): 1-21. doi: 10.3934/bioeng.2022001 |
[9] | Thi Kim Thoa Thieu, Roderick Melnik . Coupled effects of channels and synaptic dynamics in stochastic modelling of healthy and Parkinson's-disease-affected brains. AIMS Bioengineering, 2022, 9(2): 213-238. doi: 10.3934/bioeng.2022015 |
[10] | Jingwen Zhang, Lu Wang, Huolin Chen, Tieying Yin, Yanqun Teng, Kang Zhang, Donghong Yu, Guixue Wang . Effect of Caspase Inhibitor Ac-DEVD-CHO on Apoptosis of Vascular Smooth Muscle Cells Induced by Artesunate. AIMS Bioengineering, 2014, 1(1): 13-24. doi: 10.3934/bioeng.2014.1.13 |
Robust QRS detection is crucial for accurate diagnosis and monitoring of cardiovascular diseases. During the detection process, various types of noise and artifacts in the electrocardiogram (ECG) can degrade the accuracy of algorithm. Previous QRS detectors have employed various filtering methods to minimize the negative impact of noise. However, their performance still significantly deteriorates in large-noise environments. To further enhance the robustness of QRS detectors on noisy electrocardiograms (ECGs), we proposed a QRS detection algorithm based on an underdamped. This method utilizes the period nonlinearity-induced stochastic resonance to enhance QRS complexes while suppressing noise and non-QRS components in the ECG. In contrast to neural network-based algorithms, our proposed algorithm does not rely on large datasets or prior knowledge. Through testing on three widely used ECG datasets, we demonstrated that the proposed algorithm achieves state-of-the-art detection performance. Furthermore, compared to traditional stochastic resonance-based method, our algorithm has increased noise robustness by 25% to 100% across various real-world environments. This enables the proposed method to maintain its optimal performance within a certain range even in the presence of additional injected noise, thus providing an excellent approach for robust QRS detection in noisy ECGs.
Electrocardiogram (ECG) is a recording of the cardiac electrical activity and is commonly used to assess cardiovascular function and health [1]–[3]. Besides cardiology, ECG is also instrumental in the diagnosis of other medical departments. For instance, changes in ECG can aid in determining the severity of fractures in orthopedic patients [4]. ECG is also indispensable for health monitoring of intensive care patients, newborns, and elderly patients [5]–[7]. ECG is also critical in diagnosing and predicting the prognosis of diabetic neuropathy [8]. As an essential metric of ECG evaluation, both standard and instantaneous heart rates can be obtained by counting the number of QRS complexes on the ECG within a specific time frame [9]. QRS complexes indicate the ventricular depolarization process, and their frequency should match the heartbeat frequency. Owing to the critical importance of a reliable clinical decision support system for patient diagnosis and monitoring [10],[11], an algorithm that provides robust QRS detection results for the decision system is of utmost significance. However, the accurate automatic detection of QRS complexes is hampered by various forms of noise in practical applications. Based on existing reports [12]–[14], ECG information is primarily affected by three types of noise, namely, electrode motion artifacts, DC drift, and high-frequency noise. Factors such as movement of the body during measurement and external forces applied to the electrodes can cause some low-frequency components, ranging from 0 to 20 Hz, that form electrode motion artifacts [12],[14]. In the frequency range of 20 to 50 Hz, the electrochemical activity of muscle cells in bones can cause high-frequency noise [12],[14], while DC drift noise can be observed at low frequencies between 8 and 50 Hz due to lead and body movement [13]. Although many QRS detectors utilizing various traditional filters have been proposed, they often struggle to demonstrate stable robustness against one or two of the aforementioned three types of noise [15]–[17]. This indicates that the traditional filters used in previous studies are not entirely successful in removing noise that overlaps with the QRS complex spectrum, posing significant challenges for accurate and automated heart rate detection from noisy ECG in real clinical environments [18].
Recently, a counterintuitive physical phenomenon called stochastic resonance (SR) has attracted the attention of scholars in the field of cardiac signal processing. The SR effect can transfer noise energy, which overlaps with the informative components in terms of spectral content, into the informative components themselves [19]. Therefore, it can suppress in-band noise without sacrificing the informative content of ECG signals. Liao et al. first applied overdamped SR to the processing of noisy weak magnetocardiogram and successfully observed all characteristic waveforms in the presence of time-varying noise [20]. In the field of electrocardiogram, Güngör et al. initially employed underdamped monostable SR (UMSR) for QRS complex detection [21]. Even in the environment with artificially injected noise, the detector based on UMSR robustly localized the QRS complexes. Subsequent studies have also demonstrated the hardware feasibility of the QRS detectors with an SR module [22]. In other fields, SR has also been extensively studied, such as signal enhancement [23]–[25], synchronization control [26]–[28], and noisy intelligent computing [29]–[32]. It is worth noting that noise robustness enhancement brought about by nonlinearity-induced SR with a small number of stable states is generally limited [33]. Particularly, in the case of monostable potentials, their lack of potential barrier can result in high output fidelity at the expense of sacrificing output signal-to-noise ratio (SNR) [34]. In the context of QRS enhancement, the magnitude of the output amplitude is more important than the signal fidelity, as it directly determines the output SNR [20],[22].
To further enhance the noise robustness, we propose an underdamped periodic SR (UPSR) module in this study to improve the performance of QRS detector under large noise environment. Compared to the traditional UMSR, the UPSR used in our proposed algorithm has infinite number of potential wells, which leads to a large noise margin for maintaining optimal performance. In comparison to neural network-based QRS detectors [35],[36], the proposed method does not require a large amount of training data and complex network structures. By testing on three publicly available datasets, we demonstrate that our proposed method has superior performance and robustness in QRS complex detection compared to traditional methods.
According to function difference, the entire process of our proposed method can be divided into two parts: the noise suppression part and the QRS localization part. Among them, the UPSR module serves as the core of the noise suppression part, and its mathematical expression is as follows:
where V(x), γ, and x(t) are the nonlinear potential function, damping factor, and the trajectory of the UPSR output, respectively. D is the standard deviation, also known as noise intensity, of the noise term ζ(t). V0 is a constant used to normalize the potential height according to the normalized amplitude of the input S(t). Owing to the opposite direction of S(t), V(x)′ can be considered as the damping force provided by the periodic nonlinearity. The damping factor γ is used for tunning different damping effects for different parts of ECG. Specifically, γ0 * 10, where γ0 is a constant, is set for the section outside the QRS complex, while γ0/100 is set for the QRS complex. A larger value of γ is used to suppress disturbances outside the target effective information, thus preventing noise spikes from being falsely identified as QRS complexes. Conversely, a smaller value of γ is employed to reduce the damping effect of the UPSR module on QRS complexes, thereby reducing the occurrence of missed QRS complexes. Besides, the damping of the UPSR module can also be indirectly controlled by adjusting the shape of V(x) through the modification of r, as illustrated in Figure 1b. By optimizing r, we can configure different damping effects for ECG signals from different patients and recording conditions.
Because the proposed QRS detection process is nonlinear, it inevitably causes changes in the morphology of the ECG. Figure 2 illustrates the morphological changes at different stages when applying the proposed algorithm to the ECG in the MIT-BIH Arrhythmia dataset. Firstly, as shown in Figure 2a,b, bandpass filters with cutoff frequencies of 0.05 and 100 Hz are used to remove out-of-band information without affecting the ECG morphology primarily concentrated within the passband. Secondly, the SR module suppresses noise and information outside the QRS complex. During this process, some high-frequency baseline variations are introduced, as shown in Figure 2c. To eliminate these high-frequency variations, a high-pass filter with a cutoff frequency of 10 Hz is applied. Finally, by applying a threshold, we can locate the QRS complexes, as shown in Figure 2d.
The SR function requires the use of numerical methods to solve it due to its complex nature. The Runge-Kutta fourth-order algorithm is commonly employed for solving such functions because of its accuracy and stability [37]. For solving a Eq. (1), the solving process of Runge-Kutta fourth-order algorithm satisfies the following formula [38]:
where w[n] and x[n] are discretized from
For the proposed QRS detector, the parameters to be optimized are concentrated in Algorithm 1 (η, h, V0, r, γ0). In our study, the parameter ranges for η, h, V0, r, and γ0 are set to (0, 10], (0, 10], (0, 10], (0, 100], and (0, 10], respectively. For such a multi-parameter nonlinear dynamic module, it is challenging to determine the optimal parameters through empirical methods. Therefore, we employed an open-source Ant Lion Optimizer (ALO) toolbox to automate the parameter optimization process [39]. This choice is motivated by the excellent performance of the ALO in optimizing the dynamics of SR-related systems [40],[41]. In the ALO algorithm, antlions grow by capturing ants, representing the potential optimal solutions reached by the algorithm. The ants represent variables that can be altered through random search. They are initially normalized using the following equation:
where maxj and minj are the maximum and minimum of random walk for j-th variable, respectively. i represents i-th iteration. d and c are the upper and lower bound of random variable respectively, and they can be updated using information of antlion positions as follows:
where AL, ω and T represent the antlion position, convergence constant and the maximum iteration, respectively. During the iteration, the right sides of the Eqs. (6) and (7) decrease gradually, leading to a closer distance between the antlion and ant. The antlion also updates its position to actively predate ants according to the average of random walk around each particular antlion and the antlion closest to the objective.
In the case of the QRS detector, a higher amplitude of QRS complexes indicates that they are more easily detectable. Therefore, the objective function of the optimizer is defined as follows:
where OF is the opposite of QRS complex SNR. The calculation of Eq. (8) involved 100 randomly chosen segments comprising the noise interval and QRS complex. Each segment for the QRS complex, centered around the identified R peak (referred to as QRSdetected), lasted for 100 ms. Additionally, 100 one-second noise intervals are arbitrarily selected from the ECG section outside the QRS complex for calculating the standard deviation.
After undergoing all filtering processes, the QRS localization module applies a detection threshold to perform binary conversion of the ECG. In this study, the threshold was set to a constant value of 0.1. Consequently, points above 0.1 and below 0.1 are transformed into 1 and 0, respectively. For each segment with a value of 1, the center is defined as the position of the QRS complex, denoted as QRSdetected. To verify the accuracy of QRS complex localization, the annotations in the dataset can be examined to determine the true positions of the QRS complexes, denoted as QRSture. According to ANSI/AAMI EC38, EC57, and previous studies [42]–[45], it is considered valid if the distance between QRSdetected and QRSture is within 150 ms. Through the aforementioned comparison process, several basic statistical measures such as true positives (TP), true negatives (TN), false positives (FP), and false negatives (FN) can be obtained. Subsequently, following commonly used evaluation metrics can be calculated based on the acquired statistical information:
As widely used test datasets for QRS detectors, MIT-BIH Arrythmia [12], European ST-T (EDB) [46], and MIT-BIH Noise Stress Test (NST) [47] datasets were selected for this study, and their detailed information is provided in Table 1. The MIT-BIH Arrythmia dataset is collected from patients with cardiac arrhythmia, while the EDB dataset is collected from patients with myocardial ischemia. The collection of the MIT-BIH NST dataset primarily serves for analyzing ECG acquisition challenges under different noise conditions. To demonstrate the superior performance and noise robustness of our proposed algorithm, the testing was divided into two categories. The first category involved testing directly on the original dataset without injecting additional noise. The second category involved subjecting the proposed algorithm to noise stress testing by injecting additional noise. The process of injecting additional noise satisfies the following formula:
where RawECG(t) is the raw ECG signal in the dataset. ξ(t) is the real-word noise sequence recorded in the MIT/BIH NST dataset. The three previously mentioned types of noise, namely muscle artifact (MA), baseline wander (BW), and electrode motion (EM) artifacts, were individually recorded in the MIT/BIH NST dataset. In this study, we considered the individual injection of the aforementioned three types of noise as well as the combined injection of all types of noise, resulting in four different scenarios.
Datasets | Recording number | Sampling frequency (Hz) | Recording length (min) | Total heartbeat number |
MIT-BIH Arrhythmia | 48 | 360 | 30 | 109518 |
EDB | 90 | 250 | 120 | 790558 |
MIT-BIH NST | 12 | 360 | 30 | 25590 |
Works | QRS detection method | Sensitivity (%) | Predictivity (%) | F1-score (%) |
Yakut, 2018 [15] | Pan-Tompkins-based | 99.83 | 99.83 | 99.83 |
Burguera, 2019 [48] | Smoothing and Peak-valley detector | 99.57 | 99.37 | 99.47 |
Jia, 2020 [35] | Convolutional neural network | 99.89 | 99.90 | 99.90 |
Peimankar, 2021 [36] | Convolutional neural network and Long short-term memory | 99.61 | 99.52 | 99.57 |
Rahul, 2021 [49] | Third power and adaptive thresholding | 99.82 | 99.85 | 99.84 |
Pander, 2022 [42] | Fuzzy c-median Clustering | 99.82 | 99.88 | 99.85 |
Güngör, 2022 [21] | UMSR | 99.95 | 99.96 | 99.96 |
This work | UPSR | 99.95 | 99.96 | 99.96 |
Works | QRS detection method | Sensitivity (%) | Predictivity (%) | F1-score (%) |
Burguera, 2019 [48] | Smoothing and Peak-valley detector | 99.88 | 99.98 | 99.93 |
Xiong, 2021 [50] | Energy Segmentation | 99.77 | 99.65 | 99.71 |
Rahul, 2021 [49] | Third power and adaptive thresholding | 99.71 | 99.80 | 99.76 |
Pander, 2022 [42] | Fuzzy c-median Clustering | 99.67 | 99.86 | 99.76 |
Güngör, 2022 [21] | UMSR | 99.93 | 99.97 | 99.95 |
This work | UPBSR | 99.93 | 99.97 | 99.95 |
Works | QRS detection method | Sensitivity (%) | Predictivity (%) | F1-score (%) |
Khamis, 2016 [51] | Smoothing and Peak-valley detector | 93.14 | 86.23 | 89.55 |
Jia, 2020 [35] | Convolutional neural network | 99.25 | 96.31 | 94.63 |
Rahul, 2021 [49] | Third power and adaptive thresholding | 97.58 | 96.04 | 96.80 |
Pander, 2022 [42] | Fuzzy c-median Clustering | 95.27 | 94.70 | 94.98 |
Güngör, 2022 [21] | UMSR | 98.65 | 99.11 | 98.87 |
This work | UPSR | 98.65 | 99.11 | 99.87 |
Even without injecting noise, the selected databases for ECG contain noise contamination from the acquisition process. Therefore, we compared the performance of the proposed algorithm with other state-of-the-art QRS detectors without injecting additional noise. Tables 2–4 respectively present the comparative results of different QRS detectors on datasets MIT-BIH Arrhythmia, EDB, and MIT-BIH NST. It is clear that both algorithms based on the SR effect outperform the other algorithms. This can be attributed to the SR-induced suppression of in-band noise. Additionally, the performance based on UMSR and UPSR shows no significant difference. This is because the SR-induced gain increases within a certain range with an increase in noise intensity, while the noise intensity in the original databases is relatively weak, resulting in a relatively weak SR effect.
Owing to the superior noise robustness of the UMSR-based QRS detector that has been demonstrated in previous studies compared to traditional algorithms such as Elgendi and Pan-Tomkins [21], we selected it as the comparative baseline for noise robustness testing in this work. Taking the MIT-BIH Arrhythmia dataset as an example, Figure 3a illustrates the sensitivity variation of the UMSR-based and UPSR-based QRS detectors when MA noise is injected. When the MA noise intensity D is relatively low, both SR modules achieve a sensitivity of 99.95% for the QRS detector. As D increases, the sensitivity of the UMSR-based QRS detector starts to decrease at D > 0.1, while the sensitivity of the UPSR-based QRS detector begins to decrease at D > 0.2. Similarly, as shown in Figures 3b,c, the predictivity and F1-score of the UPSR-based QRS detector exhibit a higher D value at the point of decrease compared to the UMSR-based QRS detector. This indicates that UPSR provides stronger robustness against MA noise for the QRS detector compared to traditional UMSR.
Figures 4–6 present the performance variations of the two QRS detectors when injected with BW, EM, and mixed noise, respectively. The trends depicted in these three figures follow similar patterns as shown in Figure 3. In all cases, the UPSR-based QRS detector consistently maintains better performance at larger D compared to the UMSR-based QRS detector. These results indicate that the UPSR module significantly improves the noise robustness of the QRS detection algorithm for ECG data with cardiac arrhythmias.
As discussed in Section 3.2, the noise robustness of the SR-based QRS detectors can be evaluated by comparing the value of D at which the performance starts to degrade. In this work, considering that the F1-score combines sensitivity and predictive value, we define the value of D at which the F1-score of the QRS detector starts to degrade as the robustness boundary Dmax. Figure 7 presents the results of the noise stress testing on EDB and MIT-BIH NST datasets for the SR-based QRS detection algorithms. Clearly, under all test conditions, the Dmax of the UPSR-based QRS detection algorithm is larger than that of the UMSR-based algorithm. This indicates that, similar to the case of MIT-BIH Arrhythmia dataset, our proposed UPSR can provide the QRS detector with stronger noise robustness compared to the traditional UMSR.
To investigate the enhancement effects of the two SR modules on QRS complexes, we defined the SNR gain of the ECG signal after SR module processing as ΔSNR. Figure 8 presents the relationship curve between ΔSNR and D for the two SR-based QRS detectors under different injected noise conditions. Clearly, as D increases, both UMSR and UPSR initially exhibit an increase in ΔSNR followed by a decrease, providing evidence of successfully induced SR effects [52]. In related studies, the noise robustness of SR systems is typically considered to be positively correlated with the SNR gain of the processed signal [53]. Therefore, this suggests that the UPSR achieves stronger noise robustness for the QRS detection algorithm compared to the UMSR by inducing a stronger SR effect. It is noteworthy that the complexity of the UPSR described in Algorithm 1 is the same as that of UMSR presented in reference [21]. This implies that under identical optimization conditions, the UPSR-based QRS detector can achieve the same computational complexity as the UMSR-based QRS detector, i.e., O(n).
In this study, we proposed a QRS detection algorithm based on UPSR. Compared to neural network-based QRS detectors, the proposed algorithm does not require a large amount of training data. Testing on three widely used public datasets demonstrated that the proposed algorithm outperforms many state-of-the-art traditional algorithms in terms of detection performance. Compared to traditional UMSR-based detectors, UPSR induces stronger SR effects, thereby enhancing the noise robustness of the QRS detector. From the perspective of the Robustness boundary Dmax, the noise margin of the UPSR-based QRS detector is increased by 25% to 100% compared to that of the UMSR-based detector.
From a clinical application perspective, our proposed algorithm does not require a large amount of training data like neural network-based QRS detectors. Therefore, it can be used even in the absence of clinical data. However, due to the significant variations in heart conditions caused by different cardiovascular diseases, it is inevitable to re-optimize the parameters of this algorithm. This limitation actually increases the burden of optimization computations in general environments such as outpatient service. In contrast, in some customized application scenarios, such as ECG monitoring of elderly individuals with cardiovascular diseases in their homes, parameter optimization only needs to be performed once at the beginning and periodically calibrated thereafter, resulting in a relatively low computational burden.
Furthermore, the importance of portable hardware for customized physiological monitoring is gradually increasing. Although this study discusses the implementation of the QRS detection algorithm based on UPSR, the configuration of this algorithm in hardware has not been discussed. Generally, there are two approaches to implementing smart portable hardware: one based on neuromorphic devices utilizing material properties [54]; the other based on miniaturized electronic devices and circuit chips [55]. Considering that periodic nonlinearity exists both in materials [56] and circuit structures [57], hardware QRS detectors based on UPSR are expected to be realizable in both frameworks. However, achieving adaptive parameter optimization in hardware will be a challenge worth exploring in the future. In addition, as machine learning algorithms play an increasingly important role in biomedical engineering [58]–[60], it is also valuable to investigate how UPSR can be utilized to enhance the noise robustness of these algorithms.
The authors declare they have not used Artificial Intelligence (AI) tools in the creation of this article.
[1] |
Böhm M, Swedberg K, Komajda M, et al. (2010) Heart rate as a risk factor in chronic heart failure (SHIFT): the association between heart rate and outcomes in a randomised placebo-controlled trial. Lancet 376: 886-894. https://doi.org/10.1016/S0140-6736(10)61259-7 ![]() |
[2] |
Hillis GS, Woodward M, Rodgers A, et al. (2012) Resting heart rate and the risk of death and cardiovascular complications in patients with type 2 diabetes mellitus. Diabetologia 55: 1283-1290. https://doi.org/10.1007/s00125-012-2471-y ![]() |
[3] |
Templos-Hernández DJ, Quezada-Téllez LA, González-Hernández BM, et al. (2021) A fractional-order approach to cardiac rhythm analysis. Chaos Solitons Fractals 147: 110942. https://doi.org/10.1016/j.chaos.2021.110942 ![]() |
[4] |
Potaris K, Gakidis J, Mihos P, et al. (2002) Management of sternal fractures: 239 cases. Asian Cardiovasc Thorac Ann 10: 145-149. https://doi.org/10.1177/021849230201000212 ![]() |
[5] |
Kekade S, Hseieh C, Islam MM, et al. (2018) The usefulness and actual use of wearable devices among the elderly population. Comput Methods Programs Biomed 153: 137-159. https://doi.org/10.1016/j.cmpb.2017.10.008 ![]() |
[6] |
Olmi B., Manfredi C, Frassineti L, et al. (2022) Heart rate variability analysis for seizure detection in neonatal intensive care units. Bioengineering 9: 165. https://doi.org/10.3390/bioengineering9040165 ![]() |
[7] |
Nakatani S, Yamamoto K, Ohtsuki T (2023) Fetal arrhythmia detection based on labeling considering heartbeat interval. Bioengineering 10: 48. https://doi.org/10.3390/bioengineering10010048 ![]() |
[8] |
Pappachan JM, Sebastian J, Bino BC, et al. (2008) Cardiac autonomic neuropathy in diabetes mellitus: prevalence, risk factors and utility of corrected QT interval in the ECG for its diagnosis. Postgrad Med J 84: 205. https://doi.org/10.1136/pgmj.2007.064048 ![]() |
[9] |
Sabor N, Gendy G, Mohammed H, et al. (2022) Robust arrhythmia classification based on QRS detection and a compact 1D-CNN for wearable ECG devices. IEEE J Biomed Health Inform 26: 5918-5929. https://doi.org/10.1109/JBHI.2022.3207456 ![]() |
[10] | Liao Z, Wang J, Shi Z, et al. (2023) Revolutionary potential of ChatGPT in constructing intelligent clinical decision support systems. Ann Biomed Eng 2023: 1-5. https://doi.org/10.1007/s10439-023-03288-w |
[11] |
Khalfallah HB, Jelassi M, Demongeot J, et al. (2023) Decision support systems in healthcare: systematic review, meta-analysis and prediction, with example of COVID-19. AIMS Bioeng 10: 27-52. https://doi/10.3934/bioeng.2023004 ![]() |
[12] | Moody GB, Mark RG (1990) The MIT-BIH Arrhythmia Database on CD-ROM and software for use with it. [1990] Proceedings Computers in Cardiology1990. Chicago: IEEE 185-188. |
[13] | Sörnmo L, Laguna P (2006) Electrocardiogram (ECG) signal processing. Wiley Encyclopedia of Biomedical Engineering . https://doi.org/10.1002/9780471740360.ebs1482 |
[14] |
Jekova I, Krasteva V, Christov I, et al. (2012) Threshold-based system for noise detection in multilead ECG recordings. Physiol Meas 33: 1463. https://doi.org/10.1088/0967-3334/33/9/1463 ![]() |
[15] |
Yakut Ö, Bolat ED (2018) An improved QRS complex detection method having low computational load. Biomed Signal Process Control 42: 230-241. https://doi.org/10.1016/j.bspc.2018.02.004 ![]() |
[16] |
Bachi L, Billeci L, Varanini M (2021) QRS detection based on medical knowledge and cascades of moving average filters. Appl Sci 11: 6995. https://doi.org/10.3390/app11156995 ![]() |
[17] |
Mukhopadhyay SK, Krishnan S (2020) Robust identification of QRS-complexes in electrocardiogram signals using a combination of interval and trigonometric threshold values. Biomed Signal Process Control 61: 102007. https://doi.org/10.1016/j.bspc.2020.102007 ![]() |
[18] |
Tereshchenko LG, Josephson ME (2015) Frequency content and characteristics of ventricular conduction. J Electrocardiol 48: 933-937. https://doi.org/10.1016/j.jelectrocard.2015.08.034 ![]() |
[19] |
Liao Z, Wang Z, Yamahara H, et al. (2022) Low-power-consumption physical reservoir computing model based on overdamped bistable stochastic resonance system. Neurocomputing 468: 137-147. https://doi.org/10.1016/j.neucom.2021.09.074 ![]() |
[20] |
Liao Z, Jin S, Kuwahata A, et al. (2021) Coherent detection stochastic resonance assisted biomagnetometer for measuring magnetocardiography at room temperature. Appl Phys Express 14: 097001. https://doi.org/10.35848/1882-0786/ac1de5 ![]() |
[21] |
Güngör CB, Mercier PP, Töreyin H (2022) A stochastic resonance electrocardiogram enhancement algorithm for robust QRS detection. IEEE J Biomed Health Inform 26: 3743-3754. https://doi.org/10.1109/JBHI.2022.3178109 ![]() |
[22] |
Güngör CB, Mercier PP, Töreyin H (2023) A 2.2 nW analog electrocardiogram processor based on stochastic resonance achieving a 99.94% QRS complex detection sensitivity. IEEE Trans Biomed Circuits Syst 17: 33-44. https://doi.org/10.1109/TBCAS.2023.3235786 ![]() |
[23] |
Zhang W, Shi P, Li M, et al. (2021) A novel stochastic resonance model based on bistable stochastic pooling network and its application. Chaos Solitons Fractals 145: 110800. https://doi.org/10.1016/j.chaos.2021.110800 ![]() |
[24] |
Shi Z, Liao Z, Tabata H (2023) Enhancing performance of convolutional neural network-based epileptic electroencephalogram diagnosis by asymmetric stochastic resonance. IEEE J Biomed Health Inform 27: 4228-4239. https://doi.org/10.1109/JBHI.2023.3282251 ![]() |
[25] | Zhai Y, Fu Y, Kang Y (2023) Incipient bearing fault diagnosis based on the two-state theory for stochastic resonance systems. IEEE Trans Instrum Meas 72: 1-11. https://doi.org/10.1109/TIM.2023.3241066 |
[26] |
Nakamura O, Tateno K (2019) Random pulse induced synchronization and resonance in uncoupled non-identical neuron models. Cogn Neurodyn 13: 303-312. https://doi.org/10.1007/s11571-018-09518-5 ![]() |
[27] |
Liao Z, Ma K, Tang S, et al. (2021) Influence of levy noise on subthreshold synchronization of spintronic stochastic neurons. Results Phys 27: 104475. https://doi.org/10.1016/j.rinp.2021.104475 ![]() |
[28] |
Liao Z, Ma K, Tang S, et al. (2021) Phase locking of ultra-low power consumption stochastic magnetic bits induced by colored noise. Chaos Solitons Fractals 151: 111262. https://doi.org/10.1016/j.chaos.2021.111262 ![]() |
[29] |
Ikemoto S (2021) Noise-modulated neural networks for selectively functionalizing sub-networks by exploiting stochastic resonance. Neurocomputing 448: 1-9. https://doi.org/10.1016/j.neucom.2020.05.125 ![]() |
[30] |
Liao Z, Ma K, Sarker MS, et al. (2022) Quantum analog annealing of gain-dissipative ising machine driven by colored gaussian noise. Adv Theory Simul 5: 2100497. https://doi.org/10.1002/adts.202100497 ![]() |
[31] |
Duan L, Ren Y, Duan F (2022) Adaptive stochastic resonance based convolutional neural network for image classification. Chaos Solitons Fractals 162: 112429. https://doi.org/10.1016/j.chaos.2022.112429 ![]() |
[32] |
Liao Z, Ma K, Tang S, et al. (2022) Nonbistable rectified linear unit-based gain-dissipative Ising spin network with stochastic resonance effect. J Comput Sci 62: 101722. https://doi.org/10.1016/j.jocs.2022.101722 ![]() |
[33] |
Liao Z, Ma K, Sarker MS, et al. (2022) Quadstable logical stochastic resonance-based reconfigurable Boolean operation subjected to heavy noise floor. Results Phys 42: 105968. https://doi.org/10.1016/j.rinp.2022.105968 ![]() |
[34] |
Lu S, He Q, Dai D, et al. (2016) Periodic fault signal enhancement in rotating machine vibrations via stochastic resonance. J Vib Control 22: 4227-4246. https://doi.org/10.1177/1077546315572205 ![]() |
[35] |
Jia M, Li F, Wu J, et al. (2020) Robust QRS detection using high-resolution wavelet packet decomposition and time-attention convolutional neural network. IEEE Access 8: 16979-16988. https://doi.org/10.1109/ACCESS.2020.2967775 ![]() |
[36] |
Peimankar A, Puthusserypady S (2021) DENS-ECG: A deep learning approach for ECG signal delineation. Expert Syst Appl 165: 113911. https://doi.org/10.1016/j.eswa.2020.113911 ![]() |
[37] |
Shi Z, Liao Z, Tabata H (2022) Boosting learning ability of overdamped bistable stochastic resonance system based physical reservoir computing model by time-delayed feedback. Chaos Solitons Fractals 161: 112314. https://doi.org/10.1016/j.chaos.2022.112314 ![]() |
[38] |
Zhang W, Shi P, Li M, et al. (2019) Signal detection based on second-order underdamped tristable stochastic resonance and its application to weak fault diagnosis. IEEE Access 7: 173753-173765. https://doi.org/10.1109/ACCESS.2019.2955605 ![]() |
[39] |
Mirjalili S (2015) The ant lion optimizer. Adv Eng Softw 83: 80-98. https://doi.org/10.1016/j.advengsoft.2015.01.010 ![]() |
[40] |
Singh M, Verma A, Sharma N (2018) Optimized multistable stochastic resonance for the enhancement of pituitary microadenoma in MRI. IEEE J Biomed Health Inform 22: 862-873. https://doi.org/10.1109/JBHI.2017.2715078 ![]() |
[41] |
Liao Z, Huang K, Tang S, et al. (2023) Reconfigurable logical stochastic resonance in a hyperbolic one-site lattice with variable-barrier potential. Results Phys 49: 106469. https://doi.org/10.1016/j.rinp.2023.106469 ![]() |
[42] |
Pander T (2022) A new approach to adaptive threshold based method for QRS detection with fuzzy clustering. Biocybern Biomed Eng 42: 404-425. https://doi.org/10.1016/j.bbe.2022.02.007 ![]() |
[43] |
Cai W, Hu D (2020) QRS complex detection using novel deep learning neural networks. IEEE Access 8: 97082-97089. https://doi.org/10.1109/ACCESS.2020.2997473 ![]() |
[44] |
Chandra BS, Sastry CS, Jana S (2019) Robust heartbeat detection from multimodal data via CNN-based generalizable information fusion. IEEE Trans Biomed Eng 66: 710-717. https://doi.org/10.1109/TBME.2018.2854899 ![]() |
[45] |
Lee M, Park D, Dong SY, et al. (2018) A novel R peak detection method for mobile environments. IEEE Access 6: 51227-51237. https://doi.org/10.1109/ACCESS.2018.2867329 ![]() |
[46] |
Taddei A, Distante G, Emdin M, et al. (1992) The European ST-T database: standard for evaluating systems for the analysis of ST-T changes in ambulatory electrocardiography. Eur Heart J 13: 1164-1172. https://doi.org/10.1093/oxfordjournals.eurheartj.a060332 ![]() |
[47] | MIT-BIH Noise Stress Test Database, PhysioNet (1999). Available from: https://www.physionet.org/content/nstdb/1.0.0/ |
[48] |
Burguera A (2019) Fast QRS detection and ECG compression based on signal structural analysis. IEEE J Biomed Health Inform 23: 123-131. https://doi.org/10.1109/JBHI.2018.2792404 ![]() |
[49] |
Rahul J, Sora M, Sharma LD (2021) Dynamic thresholding based efficient QRS complex detection with low computational overhead. Biomed Signal Process Control 67: 102519. https://doi.org/10.1016/j.bspc.2021.102519 ![]() |
[50] |
Xiong H, Liang M, Liu J (2021) A real-time QRS detection algorithm based on energy segmentation for exercise electrocardiogram. Circuits Syst Signal Process 40: 4969-4985. https://doi.org/10.1007/s00034-021-01702-z ![]() |
[51] |
Khamis H, Weiss R, Xie Y, et al. (2016) QRS detection algorithm for telehealth electrocardiogram recordings. IEEE Trans Biomed Eng 63: 1377-1388. https://doi.org/10.1109/TBME.2016.2549060 ![]() |
[52] |
Liao Z, Wang Z, Yamahara H, et al. (2021) Echo state network activation function based on bistable stochastic resonance. Chaos Solitons Fractals 153: 111503. https://doi.org/10.1016/j.chaos.2021.111503 ![]() |
[53] |
Fu Y, Kang Y, Chen G (2020) Stochastic resonance based visual perception using spiking neural networks. Front Comput Neurosci 14: 24. https://doi.org/10.3389/fncom.2020.00024 ![]() |
[54] |
Liao Z, Yamahara H, Terao K, et al. (2023) Short-term memory capacity analysis of Lu3Fe4Co0.5Si0.5O12-based spin cluster glass towards reservoir computing. Sci Rep 13: 5260. https://doi.org/10.1038/s41598-023-32084-8 ![]() |
[55] |
Cai F, Yen S, Uppala A, et al. (2022) A fully integrated system-on-chip design with scalable resistive random-access memory tile design for analog in-memory computing. Adv Intell Syst 4: 2200014. https://doi.org/10.1002/aisy.202200014 ![]() |
[56] |
Christodoulides DN, Joseph RI (1989) Slow bragg solitons in nonlinear periodic structures. Phys Rev Lett 62: 1746. https://doi.org/10.1103/PhysRevLett.62.1746 ![]() |
[57] |
Böhm F, Sahakian S, Dooms A, et al. (2020) Stable high-speed encryption key distribution via synchronization of chaotic optoelectronic oscillators. Phys Rev Appl 13: 064014. https://doi.org/10.1103/PhysRevApplied.13.064014 ![]() |
[58] | Mao Y, Tan KXQ, Seng A, et al. (2022) Stratification of patients with diabetes using continuous glucose monitoring profiles and machine learning. Health Data Sci 2022: 9892340. https://doi.org/10.34133/2022/9892340 |
[59] | Yan Y, Hong S, Zhang W, et al. (2022) Artificial intelligence in skin diseases: fulfilling its potentials to meet the real needs in dermatology practice. Health Data Sci 2022: 9791467. https://doi.org/10.34133/2022/9791467 |
[60] |
Kazi Tani LF, Kazi Tani MY, Kadri B (2022) Gas-Net: A deep neural network for gastric tumor semantic segmentation. AIMS Bioeng 9: 266-282. https://doi.org/10.3934/bioeng.2022018 ![]() |
1. | Peihong Li, Zhiqiang Liao, Hitoshi Tabata, Parameter-induced logical stochastic resonance in substrate potential with deformable sine-Gordon shape, 2024, 92, 05779073, 542, 10.1016/j.cjph.2024.09.025 | |
2. | Jian Suo, Haiyan Wang, Yongsheng Yan, Xiaohong Shen, Deep stochastic resonance array and its application in enhancing underwater weak signals, 2024, 0924-090X, 10.1007/s11071-024-10464-7 | |
3. | Jinhui Song, Xingxing Shi, Jiu Hui Wu, Tengyue Zheng, Zhiwei Song, Nonlinear multi-order coupled stochastic resonance modeling under extremely low signal-to-noise ratios, 2025, 224, 08883270, 112208, 10.1016/j.ymssp.2024.112208 |
Datasets | Recording number | Sampling frequency (Hz) | Recording length (min) | Total heartbeat number |
MIT-BIH Arrhythmia | 48 | 360 | 30 | 109518 |
EDB | 90 | 250 | 120 | 790558 |
MIT-BIH NST | 12 | 360 | 30 | 25590 |
Works | QRS detection method | Sensitivity (%) | Predictivity (%) | F1-score (%) |
Yakut, 2018 [15] | Pan-Tompkins-based | 99.83 | 99.83 | 99.83 |
Burguera, 2019 [48] | Smoothing and Peak-valley detector | 99.57 | 99.37 | 99.47 |
Jia, 2020 [35] | Convolutional neural network | 99.89 | 99.90 | 99.90 |
Peimankar, 2021 [36] | Convolutional neural network and Long short-term memory | 99.61 | 99.52 | 99.57 |
Rahul, 2021 [49] | Third power and adaptive thresholding | 99.82 | 99.85 | 99.84 |
Pander, 2022 [42] | Fuzzy c-median Clustering | 99.82 | 99.88 | 99.85 |
Güngör, 2022 [21] | UMSR | 99.95 | 99.96 | 99.96 |
This work | UPSR | 99.95 | 99.96 | 99.96 |
Works | QRS detection method | Sensitivity (%) | Predictivity (%) | F1-score (%) |
Burguera, 2019 [48] | Smoothing and Peak-valley detector | 99.88 | 99.98 | 99.93 |
Xiong, 2021 [50] | Energy Segmentation | 99.77 | 99.65 | 99.71 |
Rahul, 2021 [49] | Third power and adaptive thresholding | 99.71 | 99.80 | 99.76 |
Pander, 2022 [42] | Fuzzy c-median Clustering | 99.67 | 99.86 | 99.76 |
Güngör, 2022 [21] | UMSR | 99.93 | 99.97 | 99.95 |
This work | UPBSR | 99.93 | 99.97 | 99.95 |
Works | QRS detection method | Sensitivity (%) | Predictivity (%) | F1-score (%) |
Khamis, 2016 [51] | Smoothing and Peak-valley detector | 93.14 | 86.23 | 89.55 |
Jia, 2020 [35] | Convolutional neural network | 99.25 | 96.31 | 94.63 |
Rahul, 2021 [49] | Third power and adaptive thresholding | 97.58 | 96.04 | 96.80 |
Pander, 2022 [42] | Fuzzy c-median Clustering | 95.27 | 94.70 | 94.98 |
Güngör, 2022 [21] | UMSR | 98.65 | 99.11 | 98.87 |
This work | UPSR | 98.65 | 99.11 | 99.87 |
Datasets | Recording number | Sampling frequency (Hz) | Recording length (min) | Total heartbeat number |
MIT-BIH Arrhythmia | 48 | 360 | 30 | 109518 |
EDB | 90 | 250 | 120 | 790558 |
MIT-BIH NST | 12 | 360 | 30 | 25590 |
Works | QRS detection method | Sensitivity (%) | Predictivity (%) | F1-score (%) |
Yakut, 2018 [15] | Pan-Tompkins-based | 99.83 | 99.83 | 99.83 |
Burguera, 2019 [48] | Smoothing and Peak-valley detector | 99.57 | 99.37 | 99.47 |
Jia, 2020 [35] | Convolutional neural network | 99.89 | 99.90 | 99.90 |
Peimankar, 2021 [36] | Convolutional neural network and Long short-term memory | 99.61 | 99.52 | 99.57 |
Rahul, 2021 [49] | Third power and adaptive thresholding | 99.82 | 99.85 | 99.84 |
Pander, 2022 [42] | Fuzzy c-median Clustering | 99.82 | 99.88 | 99.85 |
Güngör, 2022 [21] | UMSR | 99.95 | 99.96 | 99.96 |
This work | UPSR | 99.95 | 99.96 | 99.96 |
Works | QRS detection method | Sensitivity (%) | Predictivity (%) | F1-score (%) |
Burguera, 2019 [48] | Smoothing and Peak-valley detector | 99.88 | 99.98 | 99.93 |
Xiong, 2021 [50] | Energy Segmentation | 99.77 | 99.65 | 99.71 |
Rahul, 2021 [49] | Third power and adaptive thresholding | 99.71 | 99.80 | 99.76 |
Pander, 2022 [42] | Fuzzy c-median Clustering | 99.67 | 99.86 | 99.76 |
Güngör, 2022 [21] | UMSR | 99.93 | 99.97 | 99.95 |
This work | UPBSR | 99.93 | 99.97 | 99.95 |
Works | QRS detection method | Sensitivity (%) | Predictivity (%) | F1-score (%) |
Khamis, 2016 [51] | Smoothing and Peak-valley detector | 93.14 | 86.23 | 89.55 |
Jia, 2020 [35] | Convolutional neural network | 99.25 | 96.31 | 94.63 |
Rahul, 2021 [49] | Third power and adaptive thresholding | 97.58 | 96.04 | 96.80 |
Pander, 2022 [42] | Fuzzy c-median Clustering | 95.27 | 94.70 | 94.98 |
Güngör, 2022 [21] | UMSR | 98.65 | 99.11 | 98.87 |
This work | UPSR | 98.65 | 99.11 | 99.87 |