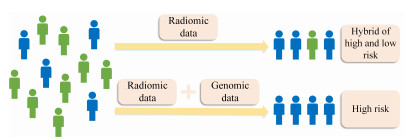
The extraction of the total soluble solid compounds from the pulp fruit Phoenix dactylifera L. is a major challenge for their valorization in the date fruit industry. However, conventional aqueous extraction methods are limited in terms of efficiency and processing time. In order to optimize this process, this study explores and compares three extraction methods: microwave-assisted extraction (MAE), ultrasound-assisted extraction (UAE), and conventional water bath–assisted extraction (WAE). The primary objective of this study is to maximize extraction recovery (ER) by performing an optimization using a Box–Behnken design (BBD). The second objective is to analyze the impact of the three extraction methods on extraction recovery, functional attributes, biochemical characteristics, antioxidant and antibacterial activities of the optimized date pulp aqueous extract (DPAE). Results obtained using mathematical models showed significant differences (p < 0.05) between the three methods tested. Optimum extraction conditions were determined as follows: for WAE, a solid/liquid (S/L) ratio of 1/4.68 g/mL, a temperature of 80 ℃, and a duration of 38.63 minutes; for MAE, an S/L ratio of 1/6 g/mL, an irradiation power of 480 W, and a duration of 11 minutes; for UAE, an S/L ratio of 1/5 g/mL, a sonication amplitude of 40%, and a duration of 60 minutes. The MAE method stood out for its extraction efficiency, with ER = 79.90% ± 1.54% and being three times faster than WAE and MAE. It also provided the highest concentrations of total soluble solids (14.33% ± 0.11% FW), total sugars (22.23 ± 0.23 g/100 mL DPAE), and total polyphenols (1.69 ± 0.04 mg GAE/mL DPAE). The antioxidant activity of DPAE was high, with an IC50 of 729.80 ± 12.87 μg/mL of the methanolic extract. These results suggest that the optimization of extraction processes, particularly using microwave technology, could offer promising prospects for the valorization of date fruit products by improving the production efficiency of a natural and nutritious DPAE, potentially beneficial to the date fruit industry in terms of product quality and cost reduction.
Citation: Naima Belguedj, Ghayth Rigane, Ridha Ben Salem, Khodir Madani. Conventional and eco-friendly aqueous extraction methods of date palm fruit compounds: Optimization, comparison, characterization of the date pulp extract and value-added potential[J]. AIMS Agriculture and Food, 2025, 10(1): 218-246. doi: 10.3934/agrfood.2025012
[1] | Jingren Niu, Qing Tan, Xiufen Zou, Suoqin Jin . Accurate prediction of glioma grades from radiomics using a multi-filter and multi-objective-based method. Mathematical Biosciences and Engineering, 2023, 20(2): 2890-2907. doi: 10.3934/mbe.2023136 |
[2] | Hakan Özcan, Bülent Gürsel Emiroğlu, Hakan Sabuncuoğlu, Selçuk Özdoğan, Ahmet Soyer, Tahsin Saygı . A comparative study for glioma classification using deep convolutional neural networks. Mathematical Biosciences and Engineering, 2021, 18(2): 1550-1572. doi: 10.3934/mbe.2021080 |
[3] | Sonam Saluja, Munesh Chandra Trivedi, Shiv S. Sarangdevot . Advancing glioma diagnosis: Integrating custom U-Net and VGG-16 for improved grading in MR imaging. Mathematical Biosciences and Engineering, 2024, 21(3): 4328-4350. doi: 10.3934/mbe.2024191 |
[4] | Yutao Wang, Qian Shao, Shuying Luo, Randi Fu . Development of a nomograph integrating radiomics and deep features based on MRI to predict the prognosis of high grade Gliomas. Mathematical Biosciences and Engineering, 2021, 18(6): 8084-8095. doi: 10.3934/mbe.2021401 |
[5] | Sonam Saluja, Munesh Chandra Trivedi, Ashim Saha . Deep CNNs for glioma grading on conventional MRIs: Performance analysis, challenges, and future directions. Mathematical Biosciences and Engineering, 2024, 21(4): 5250-5282. doi: 10.3934/mbe.2024232 |
[6] | Xiaowei Zhang, Jiayu Tan, Xinyu Zhang, Kritika Pandey, Yuqing Zhong, Guitao Wu, Kejun He . Aggrephagy-related gene signature correlates with survival and tumor-associated macrophages in glioma: Insights from single-cell and bulk RNA sequencing. Mathematical Biosciences and Engineering, 2024, 21(2): 2407-2431. doi: 10.3934/mbe.2024106 |
[7] | Hongwei Sun, Qian Gao, Guiming Zhu, Chunlei Han, Haosen Yan, Tong Wang . Identification of influential observations in high-dimensional survival data through robust penalized Cox regression based on trimming. Mathematical Biosciences and Engineering, 2023, 20(3): 5352-5378. doi: 10.3934/mbe.2023248 |
[8] | Moxuan Zhang, Quan Zhang, Jilin Bai, Zhiming Zhao, Jian Zhang . Transcriptome analysis revealed CENPF associated with glioma prognosis. Mathematical Biosciences and Engineering, 2021, 18(3): 2077-2096. doi: 10.3934/mbe.2021107 |
[9] | Yuan Yang, Lingshan Zhou, Xi Gou, Guozhi Wu, Ya Zheng, Min Liu, Zhaofeng Chen, Yuping Wang, Rui Ji, Qinghong Guo, Yongning Zhou . Comprehensive analysis to identify DNA damage response-related lncRNA pairs as a prognostic and therapeutic biomarker in gastric cancer. Mathematical Biosciences and Engineering, 2022, 19(1): 595-611. doi: 10.3934/mbe.2022026 |
[10] | Wei Niu, Lianping Jiang . A seven-gene prognostic model related to immune checkpoint PD-1 revealing overall survival in patients with lung adenocarcinoma. Mathematical Biosciences and Engineering, 2021, 18(5): 6136-6154. doi: 10.3934/mbe.2021307 |
The extraction of the total soluble solid compounds from the pulp fruit Phoenix dactylifera L. is a major challenge for their valorization in the date fruit industry. However, conventional aqueous extraction methods are limited in terms of efficiency and processing time. In order to optimize this process, this study explores and compares three extraction methods: microwave-assisted extraction (MAE), ultrasound-assisted extraction (UAE), and conventional water bath–assisted extraction (WAE). The primary objective of this study is to maximize extraction recovery (ER) by performing an optimization using a Box–Behnken design (BBD). The second objective is to analyze the impact of the three extraction methods on extraction recovery, functional attributes, biochemical characteristics, antioxidant and antibacterial activities of the optimized date pulp aqueous extract (DPAE). Results obtained using mathematical models showed significant differences (p < 0.05) between the three methods tested. Optimum extraction conditions were determined as follows: for WAE, a solid/liquid (S/L) ratio of 1/4.68 g/mL, a temperature of 80 ℃, and a duration of 38.63 minutes; for MAE, an S/L ratio of 1/6 g/mL, an irradiation power of 480 W, and a duration of 11 minutes; for UAE, an S/L ratio of 1/5 g/mL, a sonication amplitude of 40%, and a duration of 60 minutes. The MAE method stood out for its extraction efficiency, with ER = 79.90% ± 1.54% and being three times faster than WAE and MAE. It also provided the highest concentrations of total soluble solids (14.33% ± 0.11% FW), total sugars (22.23 ± 0.23 g/100 mL DPAE), and total polyphenols (1.69 ± 0.04 mg GAE/mL DPAE). The antioxidant activity of DPAE was high, with an IC50 of 729.80 ± 12.87 μg/mL of the methanolic extract. These results suggest that the optimization of extraction processes, particularly using microwave technology, could offer promising prospects for the valorization of date fruit products by improving the production efficiency of a natural and nutritious DPAE, potentially beneficial to the date fruit industry in terms of product quality and cost reduction.
Low-grade glioma (LGG) is a uniformly fatal tumor, and the survival from this tumor is approximately 7 years [1]. Because of the heterogeneity in LGG patients, different LGG subtypes increase the difficulty of optimizing management of adult low-grade gliomas [2,3]. Magnetic Resonance Imaging (MRI) is an imaging technique that can capture tumors of the brain clearly [4]. Clinicians often use MRI images to diagnose the agammaessiveness of the tumor. Therefore, the analysis of MRI data and feature extraction are becoming more challenging. To address these issues, many studies have used MRI data to extract prognostic factors for LGG patients. In a study by Pignatti et al. [5], the authors established a score system that can be used to determine the prognostic score. In adult patients with LGG, the age of the patients, the astrocytoma histology, the largest diameter of the tumor, the tumor crossing the midline and the presence of a neurologic deficit before surgery are all important prognostic factors for survival. These factors can be used to identify low-risk and high-risk patients. In a study by Chen et al. [6], the authors developed a computer-assisted algorithm for tumor segmentation and characterization using both kinetic information and morphological features of 3-D DCE-MRI. They differentiated benign and malignant lesions by analyzing 3-D morphological features including shape features and texture features of the segmented tumor. In a study by Agravat et al. [7], the authors implemented the DeepMedic CNN architecture for tumor segmentation and the extracted features are fed to a random forest classifier to obtain 59% overall survival accuracy. In another study by Shboul et al. [8], 40 features were extracted from the predicted brain tumor mask and fed to a random forest regression to predict the overall survival of a glioma patient, with an accuracy of 67% on the training dataset and 57.9% on the testing dataset. In an attempt at prediction of survival [9], the authors extracted 26 image-derived geometrical features and used SVM to predict the risk of death and classify glioma patients into three groups, with an accuracy of 56.8%. In another attempt [10], hundreds of intensity and texture features were extracted from MR images of glioblastoma multiforme, and principal component analysis (PCA) was used to reduce dimensionality. Then, these features were fed to an artificial neural network (ANN). A result with accuracy of 65.1% was obtained based on two classes: short-overall survivor and long-overall survivor. In another study [11], Chato et al. attempted the use of support vector machines (SVMs), k-nearest neighbors (KNNs), linear discriminants, tree, ensembles and logistic regression to classify survivors into two or three classes. The features from segmentations are used to train the linear discriminant for prediction of survival. The texture features resulted in the accuracy of 46%, and histogram features achieved an accuracy of 68.5% for the test dataset.
The above methods predicted survival by using only image information or clinical information. However, the tumor heterogeneity possibly comes from strong phenotypic differences, and it is difficult to predict prognosis accurately by using only medical imaging analysis (see Figure 1), thus motivating the need for integrating another kind of data. Along with the rapid development of deep-sequencing technology, the output of sequencing has made huge progress not only in equality but also in speed [12]. If radiomic data and genomic data can be integrated, this integration will build a bridge between micro and macro and increase the accuracy of the precision diagnosis and treatment of the brain tumor [13]. Grossmann et al. [14] found that prognostic biomarkers performed better in lung cancer when radiomic, genetic, and clinical information was combined. The C-index was 0.73, while the result is only 0.66 when lacking genetic information. Xia et al. [15] created a radiogenomic strategy that can obtain significant associations between imaging features and gene expression patterns in hepatocellular carcinoma. However, similar work is lacking in LGG. Therefore, in this study we integrated two different types of data, i.e., radiomic features of MRI and gene signatures, to develop a new integrated survival prediction measure for LGG.
The framework of this study is shown in Figure 2. First, we used gene expression data to construct a gene regulatory network and identify network modules and then used imaging data to extract significant radiomic biomarkers that are associated with the survival of the patient (Parts (a) and (b)), respectively. Then, we calculated the correlation between gene modules and image features to obtain a small number of gene signatures that are connected with these image features (Part (c)). Furthermore, we established a Lasso (least absolute shrinkage and selection operator) model to predict the image features with only gene expression values (Parts (d) and (e)). Based on gene expression data, we used support vector machines (SVMs) to identify the gene signatures (Parts (f) and (g)). We combined the predicted image features and the gene signatures to establish an integrated measure that can predict survival of the LGG patient (Parts (h) and (i)). The results show that the integrated measure performed better on survival prediction than any other single index.
Computer-aided and manually corrected segmentation labels for the preoperative multi-institutional scans of 65 LGG patients and 724 radiomic features along with the corresponding skull-stripped and coregistered multimodal (i.e., T1, T1-Gd, T2, T2-FLAIR) MRI data were collected from the Cancer Imaging Archive (TCIA) [16,17,18]. The corresponding RNA-seq data and Disease Free Survival (DSS) data for these 65 patients were also obtained from The Cancer Genome Atlas (TCGA) database. These data were used in this study as the training dataset.
The gene expression data and the corresponding DSS data of 455 LGG patients were downloaded from TCGA and used in this study as the validation dataset.
A gene coexpression network was constructed using gene expression data in the training dataset. We deleted genes that express in less than 20% of the patients or have no expression values. Then, we retained genes that have the highest 25% variance. A pairwise correlation matrix was calculated, and then we adjusted the matrix by raising it to the power of five using the R package WGCNA [19,20]. The minimum module size was set to 50, and the minimum height for merging modules was set to 0.25.
We identified significant image features that are associated with patient DSS by training a multivariate Cox regression model [21] on the training dataset. Image features were filtered with the standard that p value must be less than 0.01. Then, these image features were treated as image biomarkers and survival prediction indexes. For each image feature, we divided patients on the validation dataset into two groups—high-risk group and low-risk group—by taking the median value of the feature as the threshold and plotted the Kaplan-Meier curves. The concordance index (C-index) [22] and the log-rank test were also used to assess the prognostic prediction performance.
The basic formula of the multivariate Cox regression model is described as follows:
h(t,X)=h0(t)⋅exp(β1X1+β2X2+...+βmXm) | (1) |
h(t,X) represents the hazard function and h0(t) is the baseline hazard function. The factor X1, X2, ..., Xm correspond to the image features here and β1, β2, ..., βm are the corresponding regression coefficients.
We calculated Pearson correlation coefficients and their statistical significance to obtain the correlations between gene modules and selected image features. Because there are many genes in each module, the principal component analysis (PCA) was used to reduce the dimension of gene expression data of 65 patients in the training dataset. Then, image features were filtered. Features that showed significant correlation (p value less than 0.05) with at least one gene module were retained, and others were removed. Then, gene modules associated with the same image feature were integrated. The enrichment analysis was performed to identify the significantly enriched molecular pathways on these modules.
We established a radiogenomic map by identifying gene signatures associated with the prognostic imaging features. Lasso (least absolute shrinkage and selection operator) is a regression analysis method that performs both variable selection and regularization [23,24]. This method can enhance the prediction accuracy and interpretability of the statistical model it produces.
Q(β)=‖y−Xβ‖2+λ‖β‖1 | (2) |
Among the above formulas, X is the variable and y is the label. β is the coefficient that we want to optimize. Q(β) is the objective function that we want to minimize. Compared with the method of least squares, the objective function in the Lasso model has a regularization term λ‖β‖1. With this L1 norm regularization term, Lasso can control the number of variables used and improve the generalization ability of the model. For each image feature remaining in the gene module analysis, Lasso was trained to select gene signatures from related gene modules and make a prediction on image features with MRI data and gene expression data in the training dataset. We determined the regularization coefficient λ by minimizing the MSE (mean squared error) of the model.
In this step, we obtained a survival prediction index using only gene signatures, without the information of image features. SVMs (support vector machines) are supervised learning models that can be used for classification and regression problems [25,26,27]. For a classification problem, the optimal hyperplane is searched to separate data into two classes with the max margin. For new data, the trained hyperplane is used to predict the label or the probability of each class. Sometimes, data may not be separated completely, and a soft margin [25] can be used by adding a penalty parameter C and slack variables ξi to obtain the minimum error. The SVM optimization problem is
minω,C12‖ω‖2+CN∑i=1ξi | (3) |
subject to
yif(xi)≥1−ξi, and ξi≥0 | (4) |
The vector ω is the vector orthogonal to the hyperplane. xi, yi are an observation pair of data points, and f(xi) is the label of xi predicted by the SVM. SVM-RFE (support vector machine-recursive feature elimination) [28] is a powerful feature selection algorithm based on SVM that can avoid overfitting when the number of features is high. In each iteration, features are scored and sorted through model training and the least important feature is removed. Remaining features are used for a next training, and the above step is repeated. The score for sorting of the ith feature is defined as
ci=ω2i | (5) |
ωi is the ith dimension of the hyperplane orthogonal vector ω in SVM. Finally, the optimal number of features that have the minimum error is determined.
We use SVM-FRE to select gene signatures and train a classification SVM model with expression data of these selected gene signatures and DSS data in the training dataset. The patient labels are set to 0 or 1 based on their prognostic situation—survival or death. Then, the predicted probability is treated as a survival prediction index. Survival curve and C-index are used to access the prediction performance.
Further, we consider a combination of selected image biomarkers and the index calculated by SVM with gene signatures. To ensure improvement of the new agammaegated index, we transform the calculation of optimal combination coefficients of all features into an optimization problem. Specifically, suppose that N image features are considered to be associated with DSS independently—which are recorded as f1, f2, ..., fN and the gene index value from SVM is recorded as g. The integrated measure we want to determine is recorded as f. The optimization problem needing to be solved can be described as follows.
maxfCf | (6) |
subject to
f=N∑i=1αi⋅fi+β⋅g | (7) |
N∑i=1αi+β=1 | (8) |
where Cf is the C-index of integrated measure f on the training dataset. Our goal is to search optimal parameters α1, α2, ..., αN and β in Eq (7) to maximize the Cf in (6).
The Particle Swarm Optimization (PSO) algorithm [29] is used to solve the optimization problem (6) in this study. PSO is an evolutionary computation algorithm inspired by bird activities that can solve any optimization problem. Initial population with some random particle is created first. For each particle, the position represents a solution, and the corresponding fitness means a value of target function. The object of PSO is to find the optimal particle that has the minimized fitness by updating the velocity and position of particle as the following formula:
vi=ω⋅vi+c1⋅r1⋅(pbesti−xi)+c2⋅r2 | (9) |
xi=xi+vi | (10) |
xi, vi is the position and velocity of the ith particle. pbesti is the best position of the ith particle in history and gbest is the best position of all particles currently. r1, r2 are random numbers between 0 and 1. ω is the inertia weight, and c1, c2 are the acceleration constants.
We take a log-rank test on 724 image features using DSS data of 65 patients in TCIA and filter these features with a standard that the p value is less than 0.01. Then, 21 features remain. Features with high similarity to each other are removed: we calculate the Pearson correlation coefficient between features and remove the one that has the bigger log-rank p value if the Pearson correlation coefficient between two image features is greater than 0.8. After this step, 6 features are removed, and 15 features remain. Based on the above univariable analysis, we first implement the proportional hazard test [21]. Each image feature meets the proportional hazard assumption (detailed information is shown in Additional file 1: Table S1). Then, we train a multivariate Cox regression model on these remaining image features with gene expression data and DSS data in the training dataset. The result is shown in Table 1, and eight features marked with ∗ are considered to be independently correlated with DSS (p<0.05).
Image features | exp(coef) | exp(coef) lower 95% | exp(coef) upper 95% | Wald test | p value |
TEXTURE_GLSZM_ET_T1Gd_SZLGE* | 0 | 0 | 0 | −3.12 | 0.00178 |
HISTO_ED_T2_Bin8* | 0.7 | 0.55 | 0.88 | −3.02 | 0.00254 |
TEXTURE_GLOBAL_ET_T1Gd_Skewness* | 3.03E+05 | 47.29 | 1.94E+09 | 2.82 | 0.00477 |
TEXTURE_GLRLM_NET_FLAIR_LRHGE* | 1 | 0.99 | 1 | −2.66 | 0.0078 |
HISTO_NET_T1_Bin4* | 0.89 | 0.8 | 0.98 | −2.42 | 0.01559 |
HISTO_ET_T1Gd_Bin10* | 1.19 | 1.03 | 1.38 | 2.35 | 0.01877 |
TEXTURE_GLSZM_NET_T1Gd_ZSV* | 0 | 0 | 0 | −2.34 | 0.01906 |
TEXTURE_GLRLM_NET_T1Gd_GLV* | 2.00E+42 | 2230.45 | 1.79E+81 | 2.13 | 0.0333 |
HISTO_ET_T1_Bin10 | 0.81 | 0.63 | 1.05 | −1.59 | 0.11219 |
TEXTURE_GLCM_ET_T2_SumAverage | 0 | 0 | 9.95E+83 | −1.57 | 0.11569 |
TEXTURE_GLRLM_NET_T1_LGRE | 0 | 0 | 2.73E+38 | −1.49 | 0.13526 |
TEXTURE_GLRLM_ED_T1_RLV | inf | 0 | inf | 1.4 | 0.16185 |
HISTO_ED_T2_Bin4 | 0.96 | 0.88 | 1.05 | −0.91 | 0.36241 |
TEXTURE_GLCM_ED_FLAIR_Energy | inf | 0 | inf | 0.78 | 0.43387 |
TEXTURE_GLSZM_NET_T1_LZLGE | 0.99 | 0.96 | 1.03 | −0.39 | 0.69976 |
A gene coexpression network is constructed using gene expression data of 65 patients in the training dataset. We delete genes that express in less than 20% of the patients or have no expression values (n = 1875). Then, we retain genes that have the highest 25% variance (n = 4663). A pairwise correlation matrix is calculated, and then we adjust the matrix by raising it to the power of five using the R package WGCNA [19,20]. The minimum module size is set to 50 and the minimum height for merging modules is set to 0.25. Then, we get 12 gene modules. Detailed information on the modules is shown in Additional file 2: Table S2.
The Pearson correlation coefficient and their statistical significance were calculated between the 12 gene modules and the 8 image features. The result is shown in Figure 3. Four image features that show significant correlation (p<0.05) with at least one gene module were obtained. HISTO_ED_T2_Bin8 is the 8-bin histogram feature of the peritumoral edema in T2-weighted precontrast, TEXTURE_GLSZM_NET_T1Gd_ZSV is the zone size variance of gray level size zone matrix (GLSZM) of the nonenhancing part of the tumor core in T1-weighted postcontrast, TEXTURE_GLRLM_NET_FLAIR_LRHGE is the long run high gray level emphasis of gray level run length matrix (GLRLM) of the nonenhancing part of the tumor core in T2 Fluid-Attenuated Inversion Recovery, and TEXTURE_GLRLM_NET_T1Gd_GLV is the gray level variance of GLRLM of the nonenhancing part of the tumor core in T1-weighted postcontrast. Then, their corresponding gene modules were integrated. The statistical results are shown in Table 2 and the detailed list of genes is shown in Additional file 3: Table S3.
Image features | Associated gene modules | Number of associated genes |
HISTO_ED_T2_Bin8 | module2, module4, module5, module7 | 2794 |
TEXTURE_GLSZM_NET_T1Gd_ZSV | module6 | 506 |
TEXTURE_GLRLM_NET_FLAIR_LRHGE | module6 | 506 |
TEXTURE_GLRLM_NET_T1Gd_GLV | module2, module4, module6, module8, module10 | 1421 |
A further KEGG enrichment analysis was performed on integrated gene modules using the Metascape website [30], which is shown in Figure 4. The complete list of biological annotations is shown in Additional file 4: Table S4. Among these, the neuroactive ligand-receptor interaction pathway is mostly enriched in all integrated gene modules with the minimum p value of 1.259×10−41, which is reported to be associated with glioma [31,32].
Then, the Lasso method described in section 2.5 was used to select gene signatures from the related gene modules and establish a map from genes to image features. We determined the regularization coefficient λ by minimizing the MSE (mean squared error) of the model. The process is shown in Figure 6. The optimal coefficient λ and the corresponding RMSE (root mean squared error) of 65 patients are shown in Table 3. The number of selected gene signatures is also shown. The detailed list of gene signatures is shown in Additional file 5: Table S5.
Image feature | Number of genes in associated modules | Optimal λ | RMSE | Number of genes selected by Lasso |
HISTO_ED_T2_Bin8 | 2794 | 1.6627 | 6.0847 | 12 |
TEXTURE_GLSZM_NET_T1Gd_ZSV | 506 | 7.81E-06 | 2.0195E-5 | 3 |
TEXTURE_GLRLM_NET_FLAIR_LRHGE | 506 | 163.9677 | 528.16 | 6 |
TEXTURE_GLRLM_NET_T1Gd_GLV | 1421 | 3.01E-03 | 0.0120 | 18 |
We made a prediction on the 4 image features using Lasso with gene expression data of 455 patients in TCGA as the validation dataset. We then took the value of each image feature as a survival prediction index. We calculated the C-index and plotted the Kaplan-Meier curves on the validation dataset. The result is shown in Figure 7. The C-index of these four survival prediction indexes are 0.6945, 0.7321, 0.7926, and 0.7985. These results indicate that these four image features perform well in survival prediction.
From the selected 4663 genes with high variance, we fed gene expression data and DSS data of 65 patients in TCIA to SVM-FRE and obtained 43 gene signatures (shown in Additional file 6: Table S6). Then, we trained a classification SVM model with these selected genes. The variables were gene expression data of 65 patients, and the labels were set to 0 or 1 based on the patient prognostic situation—survival or death. Penalty parameter C was set to 2 and 5-fold cross-validation was used to evaluate the error in the recursive feature elimination process. We trained the SVM model and took the predicted probability of survival as a survival prediction index. C-index and survival curve are shown in Figure 8. The C-index is 0.7627.
We took a linear combination of four significant image features and the index calculated by SVM with gene signatures. A better integrated measure was obtained that represents patient survival situation. Set N=4 in formula (7). Four normalized image feature values were recorded as f1, f2, f3, and f4, and the index value from SVM was recorded as g. The integrated measure is recorded as f. Then, we get
f=4∑i=1αi⋅fi+β⋅g | (11) |
We used PSO algorithm to calculate the optimal coefficient to maximize the C-index of 65 patients in the training dataset, with parameters ω, C1 and C2 of 0.8, 0.5 and 0.5. The initial population size was set to 20, 25, 30, 35 and 40, and the corresponding iteration number was set to 30 to ensure the convergence of PSO. We repeated numerical experiments 10 times and recorded the average result for different parameters. Detailed results of each experiment are shown in Additional file 7: Table S7. For each population size, we then brought the coefficients into formula (11) and obtained integrated measure f with different forms. C-index was calculated using gene expression data on the validation dataset. The validation result is shown in Table 4.
Populations sizes | 20 | 25 | 30 | 35 | 40 |
α1 | 0.2926 | 0.3187 | 0.2792 | 0.3303 | 0.276 |
α2 | 0.0663 | 0.0394 | 0.0739 | 0.0505 | 0.068 |
α3 | 0.2171 | 0.2329 | 0.2076 | 0.2214 | 0.2102 |
α4 | 0.0091 | 0.019 | 0.0107 | 0.0298 | 0.0159 |
β | 0.4149 | 0.39 | 0.4288 | 0.368 | 0.4298 |
C-index | 0.8065 | 0.807 | 0.8061 | 0.807 | 0.8057 |
From Table 4, we observe that β is more or less than 0.4 with different parameters. Therefore, the proportion of gene signatures in integration is approximately 40%. α1 is approximately 0.3, α2 is approximately 0.06 and α3 is approximately 0.24. α4 is nearly 0, indicating that the gray level variance of GLRLM of the nonenhancing part of the tumor core in T1-weighted postcontrast can be removed in the integration. We then set parameters α1, α2, α3, α4 and β to 0.3, 0.06, 0.24, 0 and 0.4. We brought these coefficients into formula (11) and calculated the integrated measure f on the validation dataset. The Kaplan-Meier curve is shown in Figure 9. The C-index of the four independent image features, gene signatures and integrated measures are shown in Table 5.
Image features | f1 | f2 | f3 | f4 | g | f |
C-index | 0.6945 | 0.7321 | 0.7926 | 0.7985 | 0.7627 | 0.8071 |
The C-index of the integrated measure f is 0.8071 and is higher than any other measure based on image signatures or gene signature. This result indicates that the integrated measure can improve the prediction accuracy. The integrated measure is recorded as follows.
f=0.3f1+0.06f2+0.24f3+0.4g | (12) |
Furthermore, we use the time dependent Receiver Operating Characteristic (ROC) [33] to further assess the predictive power and compare different prediction models. Time-dependent ROC analysis showed that the integrated measure improved our ability to predict prognosis [AUC, 0.79; and 95% confidence intervals (CI), 0.71 to 0.87] (see Figure 10), when compared with other measures based on image signatures or gene signatures.
Patients are defined into two groups—high-risk group and low-risk group, based on their prognosis—DSS value in this study, by taking the median value of DSS of 65 patients in the training dataset as a threshold. Then, classification is conducted on 455 patients in the validation dataset by taking a threshold of the median value of the integrated measure in the training dataset. The accuracy is 72.1%, which is higher than the accuracy of the published studies [7,8,9,10,11].
The primary goal of phenotyping and classifying a human tumor is to capture tumor heterogeneity and realize personalized precision diagnosis and therapy. In clinical practice, the massive and multiple types of big medical data are available with the rapid development of biomedical engineering and computer application technology. However, one of the biggest challenges in clinical applications is how to integrate these different types of data to extract accuracy information.
In this study, we attempted to integrate both MRI data and gene expression data to propose a new feature measure that could be used to identify subsets of LGG patients at low and high risk for progression to DSS. Based on gene expression data, we first used the WGCNA method to construct the network and identify twelve network modules. With MRI data, eight image biomarkers were obtained by using the Cox regression model. Furthermore, through correlation analysis between gene modules and image features, four radiomic biomarkers were identified. Because MRI data are not available in our test dataset, the Lasso method was applied to build a map from gene expression data to these image features. In addition, we also independently used gene expression data to predict image biomarkers through the SVM method. Finally, an integrated measure (IM) for combining image and gene signatures was obtained through the PSO algorithm. We validated IM with gene expression data and DSS data on 455 patients in the validation dataset. The C-index of IM is 0.8071 and its Area Under Curve (AUC) of the ROC curve is 0.79, higher than any other single measure. The accuracy of classification of patients is 72.1%, which is higher than the accuracy of the published work using only radiomic data [7,8,9,10,11]. The results demonstrate that the proposed IM enhances the prediction accuracy for lower grade gliomas.
In summary, the accuracy of DSS prediction of LGG patients is successfully improved by integrating radiomic features in Macro with the gene expression data in Micro. The proposed method in this study can also be extended to analyze different data sources of other tumors.
This work was supported by the National Key Research and Development Program of China (No. 2018YFC1314600), the Key Program of the National Natural Science Foundation of China (No. 11831015) and the Chinese National Natural Science Foundation (No. 61672388).
All authors declare no conflicts of interest in this paper.
[1] | FAOSTAT (2023) Production/Yield quantities of Dates in Algeria 2023. Available from: https://www.fao.org/faostat/fr/#data/QCL/visualize. |
[2] |
Alharbi KL, Raman J, Shin HJ (2021) Date fruit and seed in nutricosmetics. Cosmetics 8: 59. https://doi.org/10.3390/cosmetics8030059 doi: 10.3390/cosmetics8030059
![]() |
[3] |
Ouamnina A, Alahyane A, Elateri I, et al. (2024) Relationship between phenolic compounds and antioxidant activity of some Moroccan date palm fruit varieties (Phoenix dactylifera L.): A two-year study. Plants 13: 1119. https://doi.org/10.3390/plants13081119 doi: 10.3390/plants13081119
![]() |
[4] |
Hinkaew J, Aursalung A, Sahasakul Y, et al. (2021) A comparison of the nutritional and biochemical quality of date palm fruits obtained using different planting techniques. Molecules 26: 2245. https://doi.org/10.3390/molecules26082245 doi: 10.3390/molecules26082245
![]() |
[5] |
Khalil N, Elbeltagy AE, Aljutaily T, et al. (2023) Organoleptic, antioxidant activity and microbial aspects of functional biscuit formulated with date fruit fibers grown in Qassim Region. Food Sci Technol (Brazil) 43: e95222. https://doi.org/10.1590/fst.95222 doi: 10.1590/fst.95222
![]() |
[6] |
Julai K, Sridonpai P, Ngampeerapong C, et al. (2023). Effects of extraction and evaporation methods on physico-chemical, functional, and nutritional properties of syrups from Barhi dates (Phoenix dactylifera L.). Foods 12: 1268. https://doi.org/10.3390/foods12061268 doi: 10.3390/foods12061268
![]() |
[7] |
Idowu AT, Igiehon OO, Adekoya AE, et al. (2020) Dates palm fruits: A review of their nutritional components, bioactivities and functional food applications. AIMS Agric Food 5: 734. https://doi.org/10.3934/agrfood.2020.4.734 doi: 10.3934/agrfood.2020.4.734
![]() |
[8] |
Al-Okbi SY (2022) Date palm as source of nutraceuticals for health promotion: A review. Curr Nutr Rep 11: 574–591. https://doi.org/10.1007/s13668-022-00437-w doi: 10.1007/s13668-022-00437-w
![]() |
[9] |
Ben Yahmed N, Dauptain K, Lajnef I, et al. (2021) New sustainable bioconversion concept of date by-products (Phoenix dactylifera L.) to biohydrogen, biogas and date-syrup. Int J Hydrogen Energy 46: 297–305. https://doi.org/10.1016/j.ijhydene.2020.09.203 doi: 10.1016/j.ijhydene.2020.09.203
![]() |
[10] |
Djaoud K, Arkoub-Djermoune L, Remini H, et al. (2020) Syrup from common date variety (Phoenix dactylifera L.): Optimization of sugars extraction and their quantification by high performance liquid chromatography. Curr Nutr Food Sci 16: 530–542. https://doi.org/10.2174/1573401315666190115160950 doi: 10.2174/1573401315666190115160950
![]() |
[11] |
Mahdi ZI, El-Sharnouby GA, Sharoba A (2022) Physicochemical properties and microbiological quality of dates syrup prepared from some Egyptian and Iraqi dates palm (Phoenix dactylifera L.) fruits. Egypt J Chem 65: 175–184. https://doi.org/10.21608/ejchem.2022.150877.6535 doi: 10.21608/ejchem.2022.150877.6535
![]() |
[12] |
AlYammahi J, Hai A, Krishnamoorthy R, et al. (2022) Ultrasound-assisted extraction of highly nutritious date sugar from date palm (Phoenix dactylifera) fruit powder: Parametric optimization and kinetic modeling. Ultrason Sonochem 88: 106107. https://doi.org/10.1016/j.ultsonch.2022.106107 doi: 10.1016/j.ultsonch.2022.106107
![]() |
[13] |
Pal P, Corpuz AG, Hasan SW, et al. (2024) Soluble natural sweetener from date palm (Phoenix dactylifera L.) extract using colloidal gas aphrons generated with a food-grade non-ionic surfactant. J Food Sci Technol 61: 1374–1382. https://doi.org/10.1007/s13197-023-05907-9 doi: 10.1007/s13197-023-05907-9
![]() |
[14] |
Ganbi HHA (2012) Production of nutritious high quality date (Phoenix dactylifera) fruits syrup (Dibs) by using some novel technological approaches. J Appl Sci Res 2012: 1524–1538. https://doi.org/10.5555/20133012564 doi: 10.5555/20133012564
![]() |
[15] | Beg S, Akhter S (2021) Box–Behnken designs and their applications in pharmaceutical product development. In: Beg S (Eds.), Design of Experiments for Pharmaceutical Product Development Volume I: Basic and Fundamental Principles, Springer, Singapore, 77–85. https://doi.org/10.1007/978-981-33-4717-5 |
[16] |
Chaira N, Ferchichi A, Mrabet A, et al. (2007) Characterization of date juices extracted from the rest of sorting of Deglet Nour variety. Biotechnology 6: 251–256. https://doi.org/10.3923/biotech.2007.251.256 doi: 10.3923/biotech.2007.251.256
![]() |
[17] |
Trigui M, Gabsi K, Amri IE, et al. (2011) Modular feed forward networks to predict sugar diffusivity from date pulp Part I. Model validation. Int J Food Prop 14: 356–370. https://doi.org/10.1080/10942910903191609 doi: 10.1080/10942910903191609
![]() |
[18] |
Messadi N, Mechmeche M, Setti K, et al. (2023) Optimization of extraction parameters and characterization of Tunisian date extract: A scientific approach toward their utilization. Sugar Tech 25: 460–472. https://doi.org/10.1007/s12355-022-01223-2 doi: 10.1007/s12355-022-01223-2
![]() |
[19] |
Nishad J, Saha S, Dubey AK, et al. (2019) Optimization and comparison of non-conventional extraction technologies for Citrus paradisi L. peels: A valorization approach. J Food Sci Technol 56: 1221–1233. https://doi.org/10.1007/s13197-019-03585-0 doi: 10.1007/s13197-019-03585-0
![]() |
[20] |
Flórez N, Conde E, Domínguez H, et al. (2015) Microwave assisted water extraction of plant compounds. J Chem Technol Biotechnol 90: 590–607. https://doi.org/10.1002/jctb.4519 doi: 10.1002/jctb.4519
![]() |
[21] |
Weremfo A, Adulley F, Adarkwah-Yiadom M (2020) Simultaneous optimization of microwave-assisted extraction of phenolic compounds and antioxidant activity of avocado (Persea americana Mill.) seeds using response surface methodology. J Anal Methods Chem 2020: 7541. https://doi.org/10.1155/2020/7541927 doi: 10.1155/2020/7541927
![]() |
[22] |
López CJ, Caleja C, Prieto MA, et al. (2018) Optimization and comparison of heat and ultrasound assisted extraction techniques to obtain anthocyanin compounds from Arbutus unedo L. Fruits. Food Chem 264: 81–91. https://doi.org/10.1016/j.foodchem.2018.04.103 doi: 10.1016/j.foodchem.2018.04.103
![]() |
[23] | AFNOR (French Association of Standardization. Association Française de NORmalisation, in French) (1984) Collection of French standards. Fruit and vegetable juice derivates. (Recueil de normes françaises. Produits dérivés des fruits et légumes jus de fruits, in French). 2nd edition, Paris, France, 343p. |
[24] |
Vickers JE, Grof CPL, Bonnett GD, et al. (2005) Overexpression of polyphenol oxidase in transgenic sugarcane results in darker juice and raw sugar. Crop Sci 45: 354–362. https://doi.org/10.2135/cropsci2005.0354 doi: 10.2135/cropsci2005.0354
![]() |
[25] |
Muñoz A, Caminiti IM, Palgan I, et al. (2012) Effects on Escherichia coli inactivation and quality attributes in apple juice treated by combinations of pulsed light and thermosonication. Food Res Int 45: 299–305. https://doi.org/10.1016/j.foodres.2011.08.020 doi: 10.1016/j.foodres.2011.08.020
![]() |
[26] |
Rai P, Majumdar GC, Sharma G, et al. (2006) Effect of various cutoff membranes on permeate flux and quality during filtration of Mosambi (Citrus sinensis (L.) Osbeck) juice. Food Bioprod Process 84: 213–219. https://doi.org/10.1205/fbp.05181 doi: 10.1205/fbp.05181
![]() |
[27] |
Dubois M, Gilles KA, Hamilton JK, et al. (1956) Colorimetric method for determination of sugars and related substances. Anal Chem 28: 350. https://doi.org/10.1021/ac60111a017 doi: 10.1021/ac60111a017
![]() |
[28] | Miller, GL (1959) Modified DNS method for reducing sugars. Anal Chem 31: 426–428. |
[29] |
Amira EA, Guido F, Behija SE, et al. (2011) Chemical and aroma volatile compositions of date palm (Phoenix dactylifera L.) fruits at three maturation stages. Food Chem 127: 1744–1754. https://doi.org/10.1016/j.foodchem.2011.02.051 doi: 10.1016/j.foodchem.2011.02.051
![]() |
[30] |
Assadi I, Elfalleh W, Benabderrahim MA, et al. (2019) Nutritional quality and antioxidant capacity of a combination of pomegranate and date juices. Int J Fruit Sci 19: 1512438. https://doi.org/10.1080/15538362.2018.1512438 doi: 10.1080/15538362.2018.1512438
![]() |
[31] |
Begum R, Yusof Y, Aziz M, et al. (2017) Screening of fruit wastes as pectin source. J Environ Sci Nat Resour 10: 65–70. https://doi.org/10.3329/jesnr.v10i1.34696 doi: 10.3329/jesnr.v10i1.34696
![]() |
[32] |
Subedi T (2023) An assessment of mineral contents in fruits. Prithvi Acad J 6: 21–21. https://doi.org/10.3126/paj.v6i1.54603 doi: 10.3126/paj.v6i1.54603
![]() |
[33] |
Ruiz-Matute AI, Rodrıguez-Sanchez S, Sanz ML, et al. (2010) Detection of adulterations of honey with high fructose syrups from inulin by GC analysis. J Food Compos Anal 23: 273–276. https://doi:10.1016/j.jfca.2009.10.004 doi: 10.1016/j.jfca.2009.10.004
![]() |
[34] |
Saleh EA, Tawfik MS, Abu-Tarboush HM (2011) Phenolic contents and antioxidant activity of various date palm (Phoenix dactylifera L.) fruits from Saudi Arabia. Food Nutr Sci 2: 16364. https://doi.org/10.4236/fns.2011.210152 doi: 10.4236/fns.2011.210152
![]() |
[35] |
Braca A, Sortino C, Politi M, et al. (2002) Antioxidant activity of flavonoids from Licania licaniaeflora. J Ethnopharmacol 79: 379–381. https://doi.org/10.1016/s0378-8741(01)00413-5 doi: 10.1016/s0378-8741(01)00413-5
![]() |
[36] |
Hernández-Pérez M, Rabanal RM, Arias A, et al. (1999) Aethiopinone, an antibacterial and cytotoxic agent from Salvia aethiopis roots. Pharm Biol 37: 17–21. https://doi.org/10.1076/phbi.37.1.17.6321 doi: 10.1076/phbi.37.1.17.6321
![]() |
[37] |
Shill DK, Kumar U, al Hossain AM, et al. (2022) Development and optimization of RP-UHPLC method for mesalamine through QbD approach. Dhaka Univ J Pharm Sci 21: 77–84. https://doi.org/10.3329/dujps.v21i1.60399 doi: 10.3329/dujps.v21i1.60399
![]() |
[38] |
Mgoma ST, Basitere M, Mshayisa VV (2021) Kinetics and thermodynamics of oil extraction from South African hass avocados using hexane as a solvent. S Afr J Chem Eng 37: 244–251. https://doi.org/10.1016/j.sajce.2021.06.007 doi: 10.1016/j.sajce.2021.06.007
![]() |
[39] |
El-Sharnouby GA, Eleid SM, Al-Otaibi MM (2014) Liquid sugar extraction from date palm (Phoenix dactylifera L.) fruits. J Food Process Technol 5: 402. https://doi.org/10.4172/2157-7110.1000402 doi: 10.4172/2157-7110.1000402
![]() |
[40] |
Kadlezir F, Mohagir AM, Desobgo SCZ (2024) Extracting juice from dates (Phoenix dactylifera L.) using response surface methodology: Effect on pH, vitamin C, titratable acidity, free amino nitrogen (FAN) and polyphenols. Appl Food Res 4: 100375. https://doi.org/10.1016/j.afres.2023.100375 doi: 10.1016/j.afres.2023.100375
![]() |
[41] | Mandal, V, Mohan, Y, Hemalatha, SJPR (2007) Microwave assisted extraction—An innovative and promising extraction tool for medicinal plant research. Pharmacogn Rev 1: 7–18. |
[42] |
Chambaud, M, Colas, C, Destandau, E (2023) Water-based microwave-assisted extraction of pigments from madder optimized by a box-Behnken design. Separations 10: 433. https://doi.org/10.3390/separations10080433 doi: 10.3390/separations10080433
![]() |
[43] |
Rocha S, Marzialetti T, Kopp M, et al. (2021). Reaction mechanism of the microwave-assisted synthesis of 5-hydroxymethylfurfural from sucrose in sugar beet molasses. Catalysts 11: 1458. https://doi.org/10.3390/catal11121458 doi: 10.3390/catal11121458
![]() |
[44] |
Entezari MH, Hagh Nazary S, Haddad Khodaparast MH (2004) The direct effect of ultrasound on the extraction of date syrup and its micro-organisms. Ultrason Sonochem 11: 379–384. https://doi.org/10.1016/j.ultsonch.2003.10.005 doi: 10.1016/j.ultsonch.2003.10.005
![]() |
[45] |
Hasni S, Rigane G, Ghazghazi H, et al. (2021) Optimum conditions and LC-ESI-MS analysis of phenolic rich extract from Eucalyptus Marginata L. under maceration and ultrasound-assisted extraction methods using response surface methodology. J Food Qual 2021: 5591022. https://doi.org/10.1155/2021/5591022 doi: 10.1155/2021/5591022
![]() |
[46] |
Vinatoru M, Mason TJ, Calinescu I (2017) Ultrasonically assisted extraction (UAE) and microwave assisted extraction (MAE) of functional compounds from plant materials. TrAC Trends Anal Chem 97: 159–178. https://doi.org/10.1016/j.trac.2017.09.002 doi: 10.1016/j.trac.2017.09.002
![]() |
[47] |
Sridhar A, Vaishampayan V, Senthil Kumar P, et al. (2022) Extraction techniques in food industry: Insights into process parameters and their optimization. Food Chem Toxicol 166: 113207. https://doi.org/10.1016/j.fct.2022.113207 doi: 10.1016/j.fct.2022.113207
![]() |
[48] |
Cendres A, Chemat F, Page D, et al. (2012) Comparison between microwave hydrodiffusion and pressing for plum juice extraction. LWT-Food Sci Technol 49: 229–237. https://doi.org/10.1016/j.lwt.2012.06.027 doi: 10.1016/j.lwt.2012.06.027
![]() |
[49] |
Turk M, Perino S, Cendres A, et al. (2017) Alternative process for strawberry juice processing: Microwave hydrodiffusion and gravity. LWT-Food Sci Technol 84: 626–633. https://doi.org/10.1016/j.lwt.2017.06.030 doi: 10.1016/j.lwt.2017.06.030
![]() |
[50] | Ogblechi SR, Ige MT (2014) Development and evaluation of a mechanical extractor for date palm fruit juice. In: 2nd International Conference on Applied Social Science Research (ICASSR 2014). Atlantis Press, 104: 85–88. https://doi.org/10.2991/icassr-14.2014.24 |
[51] |
Samsalee N, Sothornvit R (2022) Different novel extraction techniques on chemical and functional properties of sugar extracts from spent coffee grounds. AIMS Agric Food 7: 897–915. https://doi.org/10.3934/agrfood.2022055 doi: 10.3934/agrfood.2022055
![]() |
[52] |
Masmoudi M, Besbes S, Blecker C, et al. (2010). Preparation and characterization of jellies with reduced sugar content from date (Phoenix dactylifera L.) and lemon (Citrus limon L.) by-products. Fruits 65: 21–29. https://doi.org/10.1051/fruits/2009038 doi: 10.1051/fruits/2009038
![]() |
[53] |
Kulkarni SG, Vijayanand P, Shubha L (2010) Effect of processing of dates into date juice concentrate and appraisal of its quality characteristics. J Food Sci Technol 47: 157–161. https://doi.org/10.1007/S13197-010-0028-Y doi: 10.1007/S13197-010-0028-Y
![]() |
[54] |
Yan-xi HOU, Ming-rui WANG, Xin LI, et al. (2023) Changes of methanol content during pectinase clarification of honey melon juice. Food Mach 39: 32–36. https://doi.org/10.13652/j.spjx.1003.5788.2022.60155 doi: 10.13652/j.spjx.1003.5788.2022.60155
![]() |
[55] |
Fikry M, Yusof YA, Al-Awaadh AM, et al. (2023) Assessment of physical and sensory attributes of date-based energy drink treated with ultrasonication: Modelling changes during storage and predicting shelf life. Processes 11: 1399. https://doi.org/10.3390/pr11051399 doi: 10.3390/pr11051399
![]() |
[56] |
Patrignani M, Brantsen JF, Awika JM, et al. (2021) Application of a novel microwave energy treatment on brewers' spent grain (BSG): Effect on its functionality and chemical characteristics. Food Chem 346: 128935. https://doi.org/10.1016/j.foodchem.2020.128935 doi: 10.1016/j.foodchem.2020.128935
![]() |
[57] |
El-Nagga EA, Abd El–Tawab YA (2012) Compositional characteristics of date syrup extracted by different methods in some fermented dairy products. Ann Agric Sci 57: 29–36. https://doi.org/10.1016/j.aoas.2012.03.007 doi: 10.1016/j.aoas.2012.03.007
![]() |
[58] |
Yilmaz Y, Toledo R (2005) Antioxidant activity of water-soluble Maillard reaction products. Food Chem 93: 273–278. https://doi.org/10.1016/j.foodchem.2004.09.043 doi: 10.1016/j.foodchem.2004.09.043
![]() |
[59] |
Zahid I, Nazir MH, Javed MA (2024) Extraction of bioactive components from date palm waste, various extraction processes and their applications: A review. Biomass Bioenergy 190: 107433. https://doi.org/10.1016/j.biombioe.2024.107433 doi: 10.1016/j.biombioe.2024.107433
![]() |
[60] |
Yusoff IM, Taher ZM, Rahmat Z, et al. (2022) A review of ultrasound-assisted extraction for plant bioactive compounds: Phenolics, flavonoids, thymols, saponins and proteins. Food Res Int 157: 111268. https://doi.org/10.1016/j.foodres.2022.111268 doi: 10.1016/j.foodres.2022.111268
![]() |
[61] |
Nariya PB, Bhalodia NR, Shukla VJ, et al. (2013). In vitro evaluation of antioxidant activity of Cordia dichotoma (Forst f.) bark. AYU (An International Quarterly Journal of Research in Ayurveda) 34:124–128. https://doi.org/10.4103/0974-8520.115451 doi: 10.4103/0974-8520.115451
![]() |
[62] |
Djaoud K, Daglia M, Sokeng AJT, et al. (2020) RP-HPLC-PDA-ESI-MS/MS screening of bioactive compounds from Degla-Beida dates: Conventional and green extraction technologies. An Univ "Dunărea de Jos" Galaţi, Fasc Ⅵ Food Technol 44: 58–81. https://doi.org/10.35219/foodtechnology.2020.1.04 doi: 10.35219/foodtechnology.2020.1.04
![]() |
[63] |
Manai S, Boulila A, Silva AS, et al. (2024) Recovering functional and bioactive compounds from date palm by-products and their application as multi-functional ingredients in food. Sustainable Chem Pharm 38: 101475. https://doi.org/10.1016/j.scp.2024.101475 doi: 10.1016/j.scp.2024.101475
![]() |
[64] |
Ashraf Z, Hamidi-Esfahani Z (2011) Date and date processing: A review. Food Rev Int 27: 101–133. https://doi.org/10.1080/87559129.2010.535231 doi: 10.1080/87559129.2010.535231
![]() |
[65] | Whitney R, Taylor M, Monti J (2007) The challenges of changing retention times in GC-MS. Available from: https://www.spectroscopyonline.com/view/challenges-changing-retention-times-gc-ms. |
[66] |
Kranenburg RF, García-Cicourel AR, Kukurin C, et al. (2019) Distinguishing drug isomers in the forensic laboratory: GC-VUV in addition to GC-MS for orthogonal selectivity and the use of library match scores as a new source of information. Forensic Sci Int 302: 109900. https://doi.org/10.1016/j.forsciint.2019.109900 doi: 10.1016/j.forsciint.2019.109900
![]() |
[67] |
Gabsi K, Trigui M, Helal AN, et al. (2013) CFD modeling to predict diffused date syrup yield and quality from sugar production process. J Food Eng 118: 205–212. https://doi.org/10.1016/j.jfoodeng.2013.04.011 doi: 10.1016/j.jfoodeng.2013.04.011
![]() |
[68] | Belguedj M (2002) Genetic resources of the date palm, characteristics of date cultivars in the palm groves of southeastern Algeria (Les ressources génétiques du palmier dattier, caractéristiques des cultivars de dattiers dans les palmeraies du Sud-Est Algérien, in french). National Agricultural Research Institute of Algeria INRAA (Institut national de la recherche agronomique d'Algérie INRAA, in French), El-Harrach, Algiers, Algeria, 1: 289. |
[69] |
Masmoudi M, Besbes S, Chaabouni M, et al. (2008) Optimization of pectin extraction from lemon by-product with acidified date juice using response surface methodology. Carbohydr Polym 74: 185–192. https://doi.org/10.1016/j.carbpol.2008.02.003 doi: 10.1016/j.carbpol.2008.02.003
![]() |
[70] |
Hassim N, Markom M, Anuar N, et al. (2015). Antioxidant and antibacterial assays on polygonum minus extracts: different extraction methods. Int J Chem Eng 2015: 826709. https://doi.org/10.1155/2015/826709 doi: 10.1155/2015/826709
![]() |
[71] | El Sohaimy S, Abdelwahab AE, Brennan CS (2015). Phenolic content, antioxidant and antimicrobial activities of Egyptian date palm (Phoenix dactylifera L.) fruits. Aust J Basic Appl Sci 9: 141–147. |
[72] |
Saleh FA, Otaibi MM (2013) Antibacterial activity of date palm (Phoenix dectylifera L.) fruit at different ripening stages. J Food Process Technol 4: 1–6. https://doi.org/10.4172/2157-7110.10002 doi: 10.4172/2157-7110.10002
![]() |
[73] |
Al-daihan S, Shafi Bhat R (2012) Antibacterial activities of extracts of leaf, fruit, seed and bark of Phoenix dactylifera. Afr J Biotechnol 11: 10021–10025. https://doi.org/10.5897/ajb11.4309 doi: 10.5897/ajb11.4309
![]() |
[74] |
Bhaskaracharya RK, Bhaskaracharya A, Stathopoulos C (2023) A systematic review of antibacterial activity of polyphenolic extract from date palm (Phoenix dactylifera L.) kernel. Front Pharmacol 13: 1043548. https://doi.org/10.3389/fphar.2022.104354 doi: 10.3389/fphar.2022.104354
![]() |
[75] |
Pitout JD (2012) Extraintestinal pathogenic Escherichia coli: A combination of virulence with antibiotic resistance. Front Microbiol 3: 9. https://doi.org/10.3389/fmicb.2012.00009 doi: 10.3389/fmicb.2012.00009
![]() |
[76] |
Gowda NN, Gurikar C, Anusha MB, et al. (2022) Ultrasound-assisted and microwave-assisted extraction, GC-MS characterization and antimicrobial potential of freeze-dried L. camara flower. J Pure Appl Microbiol 16: 526–540. https://doi.org/10.22207/JPAM.16.1.50 doi: 10.22207/JPAM.16.1.50
![]() |
[77] |
Chan CH, Yusoff R, Ngoh GC, et al. (2011) Microwave-assisted extractions of active ingredients from plants. J Chromatogr A 1218: 6213–6225. https://doi.org/10.1016/j.chroma.2011.07.040 doi: 10.1016/j.chroma.2011.07.040
![]() |
[78] |
Lasunon P, Phonkerd N, Tettawong P, et al. (2021) Effect of microwave-assisted extraction on bioactive compounds from industrial tomato waste and its antioxidant activity. Food Res 5: 468–474. https://doi.org/10.26656/fr.2017.5(2).516 doi: 10.26656/fr.2017.5(2).516
![]() |
[79] |
Khalfi A, Garrigós MC, Ramos M, et al. (2024) Optimization of the microwave-assisted extraction conditions for phenolic compounds from date seeds. Foods 13: 3771. https://doi.org/10.3390/foods13233771 doi: 10.3390/foods13233771
![]() |
[80] |
Peng S, Zhu M, Li S, et al. (2023). Ultrasound-assisted extraction of polyphenols from Chinese propolis. Front Sustainable Food Syst 7: 1131959. https://doi.org/10.3389/fsufs.2023.1131959 doi: 10.3389/fsufs.2023.1131959
![]() |
[81] | Abdennabi R, Gaboriaud N, Ahluwalia V, et al. (2017) Microwave-assisted extraction of phenolic compounds from date palm saps (Phoenix dactylifera L.) and their antioxidant, antidiabetic and antibacterial activities evaluation. Mathews J Diabetes Obes 2: 1–6. |
[82] |
Sandeep G, Arumugam T, Janavi GJ, et al. (2023) A Comparative study on conventional and non-conventional extraction methodologies for extraction yield, quality and antibacterial properties of moringa (Moringa oleifera Lam.). J Appl Hortic 25: 17–24. https://doi.org/10.37855/jah.2023.v25i01.03 doi: 10.37855/jah.2023.v25i01.03
![]() |
[83] |
Deng X, Huang H, Huang S, et al. (2022) Insight into the incredible effects of microwave heating: Driving changes in the structure, properties and functions of macromolecular nutrients in novel food. Front Nutr 9: 941527. https://doi.org/10.3389/fnut.2022.941527 doi: 10.3389/fnut.2022.941527
![]() |
[84] |
Bouarab-Chibane L, Forquet V, Lantéri P, et al. (2019) Antibacterial properties of polyphenols: characterization and QSAR (Quantitative structure-activity relationship) models. Front Microbiol 10: 829. https://doi.org/10.3389/fmicb.2019.00829 doi: 10.3389/fmicb.2019.00829
![]() |
[85] |
Mondal J, Lakkaraju R, Ghosh P, et al. (2021) Acoustic cavitation-induced shear: A mini-review. Biophys Rev 13: 1229–1243. https://doi.org/10.1007/s12551-021-00896-5 doi: 10.1007/s12551-021-00896-5
![]() |
[86] |
Rahmatia L, Irawan C, Sukiman M, et al. (2023) Optimization of ultrasonic-assisted extraction methods of Gymnanthemum amygdalinum del for antioxidant and antibacterial activities. Egypt J Chem 66: 379–387. https://doi.org/10.21608/EJCHEM.2023.187526.7490 doi: 10.21608/EJCHEM.2023.187526.7490
![]() |
[87] |
Gonelimali FD, Lin J, Miao W, et al. (2018) Antimicrobial properties and mechanism of action of some plant extracts against food pathogens and spoilage microorganisms. Front Microbiol 9: 1639. https://doi.org/10.3389/fmicb.2018.01639 doi: 10.3389/fmicb.2018.01639
![]() |
[88] |
Bessalah S, Khorchani T, Hammadi M, et al. (2023) Inhibitory potential of natural plant extracts against Escherichia coli strain isolated from diarrheic camel calves. Open Vet J 13: 1082–1090. http://dx.doi.org/10.5455/OVJ.2023.v13.i9.3 doi: 10.5455/OVJ.2023.v13.i9.3
![]() |
[89] | Gattupalli N, Gattupalli A (2021) Potential of Escherichia coli probiotics for improved health and disease management. In: Edition: Erjavec MS (Ed.), Escherichia coli-Old and New Insights. IntechOpen. https://doi.org/10.5772/intechopen.100380 |
1. | Farinaz Forouzannia, Vahid Shahrezaei, Mohammad Kohandel, The impact of random microenvironmental fluctuations on tumor control probability, 2021, 509, 00225193, 110494, 10.1016/j.jtbi.2020.110494 | |
2. | Anuraag Bukkuri, Kenneth J. Pienta, Robert H. Austin, Emma U. Hammarlund, Sarah R. Amend, Joel S. Brown, A mathematical investigation of polyaneuploid cancer cell memory and cross-resistance in state-structured cancer populations, 2023, 13, 2045-2322, 10.1038/s41598-023-42368-8 | |
3. | Anuraag Bukkuri, Modeling stress-induced responses: plasticity in continuous state space and gradual clonal evolution, 2024, 143, 1431-7613, 63, 10.1007/s12064-023-00410-3 |
Image features | exp(coef) | exp(coef) lower 95% | exp(coef) upper 95% | Wald test | p value |
TEXTURE_GLSZM_ET_T1Gd_SZLGE* | 0 | 0 | 0 | −3.12 | 0.00178 |
HISTO_ED_T2_Bin8* | 0.7 | 0.55 | 0.88 | −3.02 | 0.00254 |
TEXTURE_GLOBAL_ET_T1Gd_Skewness* | 3.03E+05 | 47.29 | 1.94E+09 | 2.82 | 0.00477 |
TEXTURE_GLRLM_NET_FLAIR_LRHGE* | 1 | 0.99 | 1 | −2.66 | 0.0078 |
HISTO_NET_T1_Bin4* | 0.89 | 0.8 | 0.98 | −2.42 | 0.01559 |
HISTO_ET_T1Gd_Bin10* | 1.19 | 1.03 | 1.38 | 2.35 | 0.01877 |
TEXTURE_GLSZM_NET_T1Gd_ZSV* | 0 | 0 | 0 | −2.34 | 0.01906 |
TEXTURE_GLRLM_NET_T1Gd_GLV* | 2.00E+42 | 2230.45 | 1.79E+81 | 2.13 | 0.0333 |
HISTO_ET_T1_Bin10 | 0.81 | 0.63 | 1.05 | −1.59 | 0.11219 |
TEXTURE_GLCM_ET_T2_SumAverage | 0 | 0 | 9.95E+83 | −1.57 | 0.11569 |
TEXTURE_GLRLM_NET_T1_LGRE | 0 | 0 | 2.73E+38 | −1.49 | 0.13526 |
TEXTURE_GLRLM_ED_T1_RLV | inf | 0 | inf | 1.4 | 0.16185 |
HISTO_ED_T2_Bin4 | 0.96 | 0.88 | 1.05 | −0.91 | 0.36241 |
TEXTURE_GLCM_ED_FLAIR_Energy | inf | 0 | inf | 0.78 | 0.43387 |
TEXTURE_GLSZM_NET_T1_LZLGE | 0.99 | 0.96 | 1.03 | −0.39 | 0.69976 |
Image features | Associated gene modules | Number of associated genes |
HISTO_ED_T2_Bin8 | module2, module4, module5, module7 | 2794 |
TEXTURE_GLSZM_NET_T1Gd_ZSV | module6 | 506 |
TEXTURE_GLRLM_NET_FLAIR_LRHGE | module6 | 506 |
TEXTURE_GLRLM_NET_T1Gd_GLV | module2, module4, module6, module8, module10 | 1421 |
Image feature | Number of genes in associated modules | Optimal λ | RMSE | Number of genes selected by Lasso |
HISTO_ED_T2_Bin8 | 2794 | 1.6627 | 6.0847 | 12 |
TEXTURE_GLSZM_NET_T1Gd_ZSV | 506 | 7.81E-06 | 2.0195E-5 | 3 |
TEXTURE_GLRLM_NET_FLAIR_LRHGE | 506 | 163.9677 | 528.16 | 6 |
TEXTURE_GLRLM_NET_T1Gd_GLV | 1421 | 3.01E-03 | 0.0120 | 18 |
Populations sizes | 20 | 25 | 30 | 35 | 40 |
α1 | 0.2926 | 0.3187 | 0.2792 | 0.3303 | 0.276 |
α2 | 0.0663 | 0.0394 | 0.0739 | 0.0505 | 0.068 |
α3 | 0.2171 | 0.2329 | 0.2076 | 0.2214 | 0.2102 |
α4 | 0.0091 | 0.019 | 0.0107 | 0.0298 | 0.0159 |
β | 0.4149 | 0.39 | 0.4288 | 0.368 | 0.4298 |
C-index | 0.8065 | 0.807 | 0.8061 | 0.807 | 0.8057 |
Image features | f1 | f2 | f3 | f4 | g | f |
C-index | 0.6945 | 0.7321 | 0.7926 | 0.7985 | 0.7627 | 0.8071 |
Image features | exp(coef) | exp(coef) lower 95% | exp(coef) upper 95% | Wald test | p value |
TEXTURE_GLSZM_ET_T1Gd_SZLGE* | 0 | 0 | 0 | −3.12 | 0.00178 |
HISTO_ED_T2_Bin8* | 0.7 | 0.55 | 0.88 | −3.02 | 0.00254 |
TEXTURE_GLOBAL_ET_T1Gd_Skewness* | 3.03E+05 | 47.29 | 1.94E+09 | 2.82 | 0.00477 |
TEXTURE_GLRLM_NET_FLAIR_LRHGE* | 1 | 0.99 | 1 | −2.66 | 0.0078 |
HISTO_NET_T1_Bin4* | 0.89 | 0.8 | 0.98 | −2.42 | 0.01559 |
HISTO_ET_T1Gd_Bin10* | 1.19 | 1.03 | 1.38 | 2.35 | 0.01877 |
TEXTURE_GLSZM_NET_T1Gd_ZSV* | 0 | 0 | 0 | −2.34 | 0.01906 |
TEXTURE_GLRLM_NET_T1Gd_GLV* | 2.00E+42 | 2230.45 | 1.79E+81 | 2.13 | 0.0333 |
HISTO_ET_T1_Bin10 | 0.81 | 0.63 | 1.05 | −1.59 | 0.11219 |
TEXTURE_GLCM_ET_T2_SumAverage | 0 | 0 | 9.95E+83 | −1.57 | 0.11569 |
TEXTURE_GLRLM_NET_T1_LGRE | 0 | 0 | 2.73E+38 | −1.49 | 0.13526 |
TEXTURE_GLRLM_ED_T1_RLV | inf | 0 | inf | 1.4 | 0.16185 |
HISTO_ED_T2_Bin4 | 0.96 | 0.88 | 1.05 | −0.91 | 0.36241 |
TEXTURE_GLCM_ED_FLAIR_Energy | inf | 0 | inf | 0.78 | 0.43387 |
TEXTURE_GLSZM_NET_T1_LZLGE | 0.99 | 0.96 | 1.03 | −0.39 | 0.69976 |
Image features | Associated gene modules | Number of associated genes |
HISTO_ED_T2_Bin8 | module2, module4, module5, module7 | 2794 |
TEXTURE_GLSZM_NET_T1Gd_ZSV | module6 | 506 |
TEXTURE_GLRLM_NET_FLAIR_LRHGE | module6 | 506 |
TEXTURE_GLRLM_NET_T1Gd_GLV | module2, module4, module6, module8, module10 | 1421 |
Image feature | Number of genes in associated modules | Optimal λ | RMSE | Number of genes selected by Lasso |
HISTO_ED_T2_Bin8 | 2794 | 1.6627 | 6.0847 | 12 |
TEXTURE_GLSZM_NET_T1Gd_ZSV | 506 | 7.81E-06 | 2.0195E-5 | 3 |
TEXTURE_GLRLM_NET_FLAIR_LRHGE | 506 | 163.9677 | 528.16 | 6 |
TEXTURE_GLRLM_NET_T1Gd_GLV | 1421 | 3.01E-03 | 0.0120 | 18 |
Populations sizes | 20 | 25 | 30 | 35 | 40 |
α1 | 0.2926 | 0.3187 | 0.2792 | 0.3303 | 0.276 |
α2 | 0.0663 | 0.0394 | 0.0739 | 0.0505 | 0.068 |
α3 | 0.2171 | 0.2329 | 0.2076 | 0.2214 | 0.2102 |
α4 | 0.0091 | 0.019 | 0.0107 | 0.0298 | 0.0159 |
β | 0.4149 | 0.39 | 0.4288 | 0.368 | 0.4298 |
C-index | 0.8065 | 0.807 | 0.8061 | 0.807 | 0.8057 |
Image features | f1 | f2 | f3 | f4 | g | f |
C-index | 0.6945 | 0.7321 | 0.7926 | 0.7985 | 0.7627 | 0.8071 |