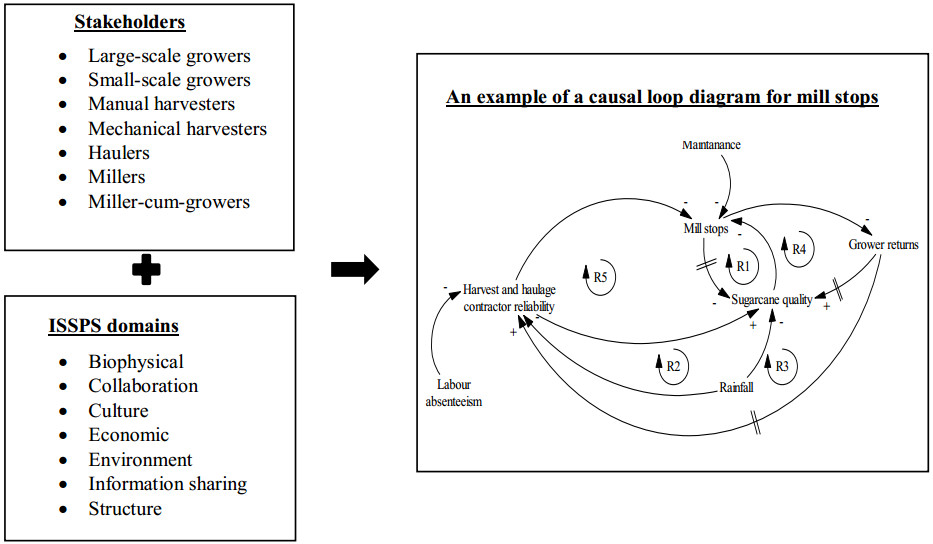
Citation: Mduduzi Innocent Shongwe, Carel Nicolaas Bezuidenhout. A heuristic for the selection of appropriate diagnostic tools in largescale sugarcane supply systems[J]. AIMS Agriculture and Food, 2019, 4(1): 1-26. doi: 10.3934/agrfood.2019.1.1
[1] | João Paulo Curto, Pedro Dinis Gaspar . Traceability in food supply chains: SME focused traceability framework for chain-wide quality and safety-Part 2. AIMS Agriculture and Food, 2021, 6(2): 708-736. doi: 10.3934/agrfood.2021042 |
[2] | Ralph Kwadwo Osei, Marco Medici, Martin Hingley, Maurizio Canavari . Exploring opportunities and challenges to the adoption of blockchain technology in the fresh produce value chain. AIMS Agriculture and Food, 2021, 6(2): 560-577. doi: 10.3934/agrfood.2021033 |
[3] | Surni, Doppy Roy Nendissa, Muhaimin Abdul Wahib, Maria Haryulin Astuti, Putu Arimbawa, Miar, Maximilian M. J. Kapa, Evi Feronika Elbaar . Socio-economic impact of the Covid-19 pandemic: Empirical study on the supply of chicken meat in Indonesia. AIMS Agriculture and Food, 2021, 6(1): 65-81. doi: 10.3934/agrfood.2021005 |
[4] | Zarbà Carla, La Via Giovanni, Pappalardo Gioacchino, Hamam Manal Samir Mustafa . The sustainability of Novel foods in the transition phase to the circular economy; the trade “Algae fit for human consumption” in European Union. AIMS Agriculture and Food, 2020, 5(1): 54-75. doi: 10.3934/agrfood.2020.1.54 |
[5] | Wei Yang, Bryan Anh, Phuc Le . Do consumers care about environmentally sustainable attributes along the food supply chain? —A systematic literature review. AIMS Agriculture and Food, 2023, 8(2): 513-533. doi: 10.3934/agrfood.2023027 |
[6] | Mohamed-Yousif Ibrahim Mohamed . Campylobacteriosis in North Africa. AIMS Agriculture and Food, 2024, 9(3): 801-821. doi: 10.3934/agrfood.2024043 |
[7] | Katharina Biely, Susanne von Münchhausen, Steven van Passel . Vertical integration as a strategy to increase value absorption by primary producers: The Belgian sugar beet and the German rapeseed case. AIMS Agriculture and Food, 2022, 7(3): 659-682. doi: 10.3934/agrfood.2022041 |
[8] | Giuseppe Timpanaro, Paolo Guarnaccia, Silvia Zingale, Vera Teresa Foti, Alessandro Scuderi . The sustainability role in the purchasing choice of agri-food products in the United Arab Emirates and Italy. AIMS Agriculture and Food, 2022, 7(2): 212-240. doi: 10.3934/agrfood.2022014 |
[9] | Abdulwakil Olawale Saba, Victor Oscar Eyo, Isa Olalekan Elegbede, Kafayat Adetoun Fakoya, Akinloye Emmanuel Ojewole, Fareed Olatunji Dawodu, Rashidat Adebola Adewale, Mohammad Noor Azmai Amal . Sustaining the blue bounty: Fish food and nutrition security in Nigeria's evolving blue economy. AIMS Agriculture and Food, 2024, 9(2): 500-530. doi: 10.3934/agrfood.2024029 |
[10] | João Paulo Curto, Pedro Dinis Gaspar . Traceability in food supply chains: Review and SME focused analysis-Part 1. AIMS Agriculture and Food, 2021, 6(2): 679-707. doi: 10.3934/agrfood.2021041 |
Integrated sugarcane supply and processing systems (ISSPS) are complex systems characterised by multiple stakeholders with various, often conflicting objectives [1]. Furthermore, these systems contain several domains that causally interact to regulate behaviour [2]. As a consequence, ISSPS exhibit several complex systems characteristics viz. non-linearity, feedback, delays, constant change, counterintuitive behaviour, emergence, and trade-offs [3]. Integrated sugarcane supply and processing systems as used in this research refers to the flow of sugarcane and information between sugarcane growing and raw sugar production. This segment includes components of cultivation, harvesting, transport and milling. Up to the point of raw sugar, ISSPS are driven by a wide range of biophysical push factors such as pest and diseases, unpredictable weather, and fluctuating qualities. However, post-milling the supply chain factors change significantly as the product (raw sugar) becomes biologically stable and also becomes the responsibility of one firm.
Similar to many other industries, the sugar industry is mature, well established and systems are relatively efficient. However, due to the higher degree of connectivity, the interactions between growers, harvesters, haulers, and the millers influence the overall effectiveness of ISSPS. Complexity as indicated in Figure 1 emanates from the interactions within and/or between these stakeholders and the many ISSPS domains. As a result, significant inefficiencies remain present in ISSPS e.g., vehicle over-fleeting [4], unnecessary risk averse behaviour [5] and problematic forecasting and planning [6]. Many of these inefficiencies are attributed to economics, collaboration issues, system governance and misaligned stakeholder objectives.
It can be seen from the causal loop diagram in Figure 1 that the issue of mill stops directly affects four key stakeholder groups viz. millers, growers, harvesters and haulers. Furthermore, complexity from interactions between domains is well pronounced on the sugarcane quality variable and on the reliability of harvesters and hauliers. As indicated in Figure 1, the quality of sugarcane delivered at the mill is a function of rainfall (environment), the reliability of harvesting and haulage (collaboration) and grower returns (economic). Delays due to low harvest and haulage reliability can cause considerable sugarcane moisture loss, sucrose inversion and a decline in recoverable sugar. Excessive rainfall on the other hand, reduces the ability to pre-harvest burn sugarcane and increases the chances of soil contamination on the harvest. The onset of rainy weather early on in the milling season therefore, requires some trade-offs between sugarcane quality and the length of the milling season. As the length of the milling season is adjusted to accommodate delayed crushing, this sometimes coincides with the wet season. Sugarcane harvested around the wet season is usually high in fibre. Furthermore, a combination of rainfall and high temperatures increases the rate of sugarcane deterioration.
To make sense of complex systems it is important to recognise that most issues do not exist in isolation, but are imbedded within complex interrelationships and interdependencies between system elements. Holistic sugarcane supply chain research has been an important contributor to the industry and has in the past identified several system-scale opportunities. Such research is mostly multidisciplinary and employs a range of research methodologies, such as interviews, questionnaires, stakeholder workshops, statistical data analysis, and modelling. A "one size fits all" approach to optimising all the systems at the same time, however, is unlikely. Even though the fundamentals of sugarcane supply chains are the same, each mill area exhibits a number of relatively unique combination of issues, which need to be contextualised at the local level [7]. Complex systems theory presents valuable universal laws such as Ashby's law [8] and the Theory of Constraints [9], to help unlock such localised opportunities. Ashby posits that the precise measure of complexity is variety [8]. Through the Law of Requisite Variety, Ashby argues that the variety of a system which regulates has to be at least equal to the variety of the system it is regulating [8]. The Theory of Constraints on the other hand, is premised on the assumption that within any complex system there exist a certain constraint, or a few. The Theory of Constraints as such, makes it is possible to identify such constraint(s) for improvement purposes [9].
System improvements are however, likely to occur through an evolutionary small step-by-step approach as opposed to a complete top-down restructuring and system overhaul [10]. This guided self-organisation notion is supported by amongst others [11], who claim that modern systems have a high degree of connectivity which makes these systems unpredictable, rigid and slow to change. Within a rigid and complex system it becomes difficult to follow standard optimisation-based research strategies. In fact, it often takes a significant amount of time to prioritise the importance of different issues that seem to negatively affect the overall system. Researchers can find themselves entangled in a web of unstructured, interconnected and multidisciplinary issues, which restricts the opportunity to apply unbiased scientific methodologies.
In this context, a heuristic research approach helps the researcher to fast track progress and to select appropriate research methodologies that appear promising. Heuristics are problem solving strategies designed to arrive at satisfactory solutions with the modest amount of effort. A review of heuristics is provided by [12] who further define the term as a strategy "with the goal of making decisions more quickly, frugally, and/or accurately than more complex methods". Heuristics reduce the cognitive burden associated with complex decision making and offer decision-makers an opportunity to examine only a few signals and/or alternative choices before reaching a conclusion [13]. Gigerenzer and Gaissmaier argue that many researchers, including Einstein, extensively used and supported heuristic research approaches [12]. Given the background, this article develops an overarching diagnostic heuristic for ISSPS aimed at diagnosing relatively small but pertinent, in situ constraints and opportunities. Contrary to most research in ISSPS which focuses on long-term issues [14], the proposed heuristic advocates for short-term focused solutions with an aim of making small, incremental changes. An integrated sugarcane supply and processing system as defined in this research refers to the physical flow of sugarcane between growing, harvesting, transport, as well as the processing components.
The health of a supply chain should be evaluated before making any interventions into the system [15]. Determining the "overall health" of the system implies a systematic process that considers all components that constitute the supply chain hence, diagnosis is critical for continuous improvement [16,17]. The term "diagnosis" refers to identification and investigation of the cause and nature of a condition, situation or a problem. Supply chain diagnosis is thus, a structured examination of issues within a supply system in order to identify improvement opportunities. Poor diagnosis remains a huge challenge within agricultural supply systems [18]. Various researchers attribute the low adoption of technologies in agricultural systems to misdiagnosis of issues [18,19]. Although literature provides many examples of diagnostic tools and their applications within ISSPS, these tools, however, are largely tailored to deal with specific problem areas and mostly, within certain paradigms [20,21]. Even those tools that are able to diagnose issues in multiple paradigms and/or dimensions, more often give less attention to the integrated nature of some of the problems. A comprehensive view is therefore more possible only through the use of a combination of tools, a concept widely referred to as multimethodology.
The large number of diagnostic tools available further makes it difficult to choose the best tool or a combination thereof, an obvious gap to developing criteria for accurate comparison of tools. The objectives of the research were therefore, to develop criteria against which diagnostic tools could be evaluated and also to compare the performance of different tools against such criteria. The broad nature of ISSPS (multiple domains), however, means that not all tools could be applied across all ISSPS domains. Depending on the domain(s), each tool can (to a certain extent) be applicable or not applicable. This research therefore further seeks to capture the diagnostic suitability of each tool against the domains. The heuristic will provide a mechanism to objectively compare, select, use, and/or commission various systemic diagnostic tools. Although the focus is on sugarcane systems, the attributes of ISSPS make the heuristic a relatively general approach to integrated agricultural systems. It is therefore, envisaged that the heuristic will also be transferable to other agricultural industries, including the large number of new and rapidly developing bio-fuel and bio-refinery supply systems.
An integrated sugarcane supply and processing system as conceptualised in this study is a sum of nine domains viz. biophysical, collaboration, culture, economics, environment, future strategy, information sharing, political forces, and structures [2]. The biophysical domain describes the physical equipment and processes involved in an ISSPS. These include raw material, work-in-process inventory, and finished products. Collaboration in contrast, describes an act where two or more independent supply chain members mutually work together to achieve more benefits than when acting in isolation. According to Wilding and Humphries, supply chain collaboration is defined by the level of trust, commitment, cooperation, and coordination [22]. Culture is defined as a pattern of shared values, beliefs, assumptions, and behaviour [23].
The environment describes the context within which a supply chain exists. The environment is thus multi-dimensional consisting of macro and micro factors [24]. Conversely, supply chain strategy is the intelligence function that monitors the environment for threats and opportunities. The economic domain describes all activities that progressively create value for the supply chain. Economic factors determine the success and profitability of ISSPS as they affect capital availability, cost and demand. Structure refers to the distribution of tasks and responsibilities within supply chains. Issues of power within a supply system are described by political forces. Lastly, information sharing describes the extent to which critical and proprietary information is communicated between supply chain partners.
The article is structured into five sections. The first section provides an overview of the practice of multimethodology outlining its applications, strengths and weaknesses. Methods undertaken to conduct the study are described in Section 3. Results and Discussion are presented in Section 4 followed by a section on Conclusion and Recommendations.
Multimethodology is a form of methodological pluralism that describes the creative combination of different methodologies, or parts thereof, within a single intervention [25]. It is not a methodology or a specific way of combining methodologies but rather, "a whole area of utilising a plurality of methodologies and techniques" [26]. The term "methodology" refers to a structured set of procedures or guidelines employed by researchers to undertake interventions [27]. Techniques or methods on the other hand, are well-defined primary activities or a sequence of operations within a methodology. Methodologies thus consist of various techniques. The term "tool" is used interchangeable throughout the study to refer to methodologies and/or techniques.
Each methodology is based on particular philosophical assumptions it makes about the nature of the world in which it can be applied (paradigms) hence, the scepticism around multimethodology especially when partitioning and/or combining methodologies and/or techniques from different paradigms [28]. Paradigms specify ontology (what is assumed to exist), epistemology (possibilities of, and limitations on the nature of valid knowledge), axiology (what is considered right), and methodology. Traditionally, two paradigms exist viz. soft (interpretivism/constructivism) and hard (positivism/post-positivism) paradigm. The hard paradigm views the world as objective whilst soft paradigms are based on a subjective meaning [29]. Another paradigm that is widely used is the critical paradigm. The critical paradigm has political overtones and obliges that the researcher(s) should uncover hidden assumptions about a specific context [30]. The paradigm assumes a transactional epistemology and a historical ontology [31].
Researchers advocating for methodological pluralism allude to the fact that the real world is multidimensional whilst particular paradigms focus on specific aspects of the problem context [20]. Adopting only one paradigm for certain problem contexts only reveals certain aspects of that context but is completely blind to others. Methodological pluralism and hence, multimethodology views all methods as complementary. According to the Theory of Communicative Action [32], the real world is made up of interactions between three constructs viz. material, personal and social. Currently there is "no existing methodology" that comprehensively covers all of these worlds simultaneously, hence, the need to draw upon a plurality of methodologies [33]. The research/intervention process itself proceeds through a number of phases and as such, multimethodology offers a comprehensive option by exploiting different tools for different phases [34]. This ability, even when the tools cover similar functions, provides triangulation. Triangulation, which is the use of multiple methods on the same phenomenon, generates new insights and provides possibilities for validating results [35].
There are two commonly used approaches to multimethodology viz. the M-B framework [27] and the Mingers approach [20]. The M-B framework uses a 2-dimensional grid with the problem context (material, social and personal worlds) on one side and the four general phases of research/intervention [36] on the other. The research/intervention phases, as portrayed by [36], are the appreciation phase, analysis, assessment, and the action phase. The appreciation phase is a design and conceptualisation phase that describes the problem context as experienced by stakeholders. The analysis phase depicts the underlying structures and constraints that maintain a specific problem. Assessment weighs up postulated explanations and potential changes to the problem context whilst the action phase brings about change if necessary. Assigning tools on the M-B framework is somewhat subjective and ad hoc. According to Mingers, the M-B framework does not critically specify the dimensions and phases in which a particular tool is more useful [20]. Furthermore, Mingers J [20] argues that the M-B framework does not focus on specific tasks but is rather more general towards the problem context. To overcome some of the M-B framework limitations, Mingers J [20] developed another framework with added dimensions. The Mingers framework [20] outlines the purpose of the intended intervention and also surfaces the philosophical assumptions (ontology, epistemology and axiology) underpinning each methodology and/or method under consideration. These "dimensions" are then synthesised into a Soft Systems Methodology root definition form [37].
The main criticism towards multimethodology concerns paradigm incommensurability [38]. The issue of incommensurability, largely based on the history of science [39], has been widely challenged by researcher [40,41]. Kuhn asserted that paradigms succeed each other and therefore, reconciliation between the "old" and the "new" cannot be possible [39]. The Theory of Knowledge Constitutive Interest [42] was used by [43] as a foundation to challenge incommensurability. The Theory of Knowledge Constitutive Interest states that all knowledge is aimed at serving three human interests viz. technical, practical and emancipatory. Jackson, however, argues that these interests are aligned with the hard, soft, and critical paradigms, respectively and as such, paradigms are complementary. Accordingly, the Theory of Communicative Action [32] is used by [44] to justify paradigm complementarity. The hard, soft and critical paradigms pursue the material, social and personal worldviews, respectively [45]. Mingers maintains that there is no universal classification of paradigms and that the concept is simply a heuristic [44]. It is upon these arguments [44,45,46] that paradigms are considered complimentary in this research. Multimethodology, as considered in this study therefore, refers to a bespoke methodology where various methodologies are partitioned into components and then combined together.
Cultural and cognitive feasibility are also considered to be barriers towards the development and adoption of multimethodology [44]. Cultural feasibility refers to the extent to which existing paradigm subcultures facilitate or act against the use of multimethodology. According to Mingers and Brocklesby, crossing and/or combining paradigms requires individuals to overcome socially constructed obstacles [27]. Mingers pointed out that there are interdependencies between personality traits, entrenched cognition and research preference [44]. These links cause individuals to experience difficulties in moving between paradigms.
The availability of multiple stakeholders and their varying perspectives means that the diagnosis of issues in ISSPS should not only be guided by cause and effect but also the appreciation of different worldviews [1]. This is further complicated by the fact that most of the diagnostic tools available are only tailored for specific context. A heuristic that could provide a comprehensive diagnosis therefore, requires a combination of tools from different paradigms and strong criteria that could guide such. The development of the ISSPS diagnostic heuristic was therefore, based on pragmatism. The pragmatic paradigm is founded on the assumption that either or both positivism and interpretivism provide acceptable knowledge dependent upon the research question. Pragmatism as such, is pluralistic and is congruent with the practice of multimethodology taken within the predisposition of practitioner-based research.
Following a thorough literature review, diagnostic criteria were developed. The criteria were founded on Bhaskar's appreciation and analysis phases [36]. The criteria are important given Mingers' argument [20] that the M-B framework does not critically specify the dimensions and phases in which particular tools are more useful. The developed criteria therefore, critically expanded on each phase (appreciation and analysis) and specified exactly what was expected from the diagnosis process. A numerical ranking scale of 1–5 was used to determine the performance of various diagnostic tools on the criteria, where a score of 5 indicated excellent and 1, very poor. Zero (0) was used to specify no relationship whatsoever. The systemic tools considered were the current reality trees, fuzzy cognitive maps, network analysis approaches, rich pictures, stock and flow diagrams, cause and effect diagrams, and causal loop diagrams. Various researchers have compared the performance of some of these tools on numerous criteria and under diverse contexts. For example, Soft Systems Methodology was compared to System Dynamics in a health services context using qualitative and graphical scales. Similarly, a qualitative scale was used to compare the performance of the current reality tree and cause and effect diagram amongst other tools. A comprehensive literature review was further used to determine the appropriateness of each of the tools to diagnose issues within each of the many ISSPS domains viz. biophysical domain, collaboration, culture, economics, environment, future strategy, information sharing, political forces, and structures. Formulating ISSPS as domains provided a more specific context than expressing the system along Habermas worlds [32].
The developed performance matrix was used as an input into the Technique for Order of Preference by Similarity to Ideal Solution (TOPSIS) to prioritise and to select appropriate diagnostic tool(s). The TOPSIS is a multi-criteria decision analysis (MCDA) tool. Multi-criteria decision analysis techniques seek to integrate objective measurements with value judgment [47]. The TOPSIS selects alternatives that simultaneously have the shortest distance from an ideal solution and the furthest distance from a negative ideal solution. It was considered attractive in this research due to its simplicity, rationality, comprehensibility and good computational efficiency. The TOPSIS uses a five step process as indicated in Figure 2. The technique is, however, only an aid to decision making and does not give a right or a wrong answer.
Initially (as indicated in Figure 2), the performance matrix is normalised before being multiplied by a weight assigned to each criterion. Decision makers define these weights according to their preferences and the sum of all weights should be equal to one. Steps 4 and 5 compute the ideal solutions and the separation measures, respectively. The TOPSIS in this research uses the benefit criteria as opposed to the cost function [49]. Hence, for all criteria the positive ideal solution in Step 3 remains a maximum value.
This Results and Discussion section is divided into three sub-sections. Using extensive literature, systemic criteria for comparing the performance of various diagnostic tools was developed in Section 4.1. Some of the diagnostic tools that are widely used in ISSPS and/or agricultural systems were thereafter reviewed in Section 4.2. The last section synthesises the information from Sections 4.1 and 4.2 to develop the diagnostic heuristic.
Integrated sugarcane supply and processing systems are complex characterised by multiple domains and stakeholders. Under such contexts, the sum of local optimisation solutions does not often translate to an overall system solution. It is for this reason that various researchers report on a number of systemic inefficiencies within ISSPS. For example, Gaucher et al. [50] and Wynne et al. [51] noted that the existence of multiple growers makes coordination of sugarcane supply difficult. Complex systems such as ISSPS are characterised by both tame and wicked or messy problem contexts. However, despite such contexts, most interventions into the system often view ISSPS as hard, technical systems characterised by tame issues [7].
Messy problems are a class of social problems where there are differences of opinions about the problem or even on the question of whether a problem exists or not [52]. These types of problems are continually evolving, have many causal levels and have no single solution. On the contrary, tame problems are well-defined and can be solved linearly using reductionist and/or sequential techniques [53]. In this research, "wicked problems" also refers to what [54] describes as "messy problems" and what [55] define as "unstructured problems".
Tame and wicked problems are not governed by the same logic hence, treating a wicked context as tame creates confusion and provides ineffective solutions [56]. In the same vein, strategies developed for wicked problems may not be suitable for tame contexts. This study embraces Bhaskar's [36] appreciation and analysis phases of research/intervention to represent wicked and tame problem contexts, respectively. Conceptualising ISSPS diagnosis along the appreciation and analysis phases is consistent with the complex systems' diagnostic process as suggested by [36]. After comparing the M-B framework, the process model [54] and the conceptualisation of problem-solving and decision-making model [57,58] came to the conclusion that complex systems diagnosis involves these two phases. The remainder of this section describes the appreciation and analysis phases and develops criteria that should be considered when comparing various tools.
Wicked problems are socially-constructed and as such, research into such contexts should be interpretive [59]. Messy problem contexts should therefore be approached using systematic social processes [60]. An interpretive approach understands reality as defined by subjective experiences of individuals. Interpretive research seeks to understand the world through an individuals' own background and experiences [61]. The appreciation phase as described by Bhaskar [36] is therefore more appropriate for such problem contexts. The appreciation phase is interpretive and based on the rationale that different worldviews give a full representation of a problem context.
Various researchers advocate for the use of participatory approaches within the appreciation phase [62,63,64]. Participatory approaches improve the legitimacy of findings as participants collectively learn about issues and discover a common ground [65]. Although convergence of views is not necessarily the aim of appreciation, in practice partial convergence emerges [63]. Consequently, appreciation tools should be both iterative and interactive [66].
Interactiveness is important as appreciation seeks to elicit resolutions through debate and negotiation [66]. Interactions between participants and that of participants with the facilitator(s) are required in order to mutually capture issues. Interactions between participants and the model reshape the modelling process [67]. Iterative-ness on the other hand is important in order to ensure that problem representation adjust to reflect the state and stage of discussion [64]. Appreciation tools should be able to operate non-linearly, switching freely between different modes of interventions [68]. Franco and Montibeller refer to this iterative-ness as "phased-ness" and posit that iterative tools ensure a tangible product without having to pass through all the phases of a process [67]. Iteration leads to the premature termination of the tendency towards satisficing.
Appreciation tools should also be cognitively accessible in order to accommodate audiences from a range of background [64]. Accessibility as defined by [69] refers to the tool's ease of use and whether it requires specialised skill (or software) or not. It is argued that tools annotated with mathematical equations and symbols require a certain level of skill and as such, promote unease among most people [70]. Appreciation tools should also be transparent and nothing should be done in secrecy [71]. The ownership of the diagnosis process is only guaranteed through transparency of representation [68]. Representing problem complexity graphically rather than algebraically or in numerical tables as such, improves participation [70].
The analysis phase is a cause-and-effect stage of diagnosis. Analysis explains the underlying causal structures that maintain certain problems. According to Raia, asking "why" and "how" establishes causal determinants of an observed phenomenon [72]. The analysis phase as a result, embraces a wide range of tools, both quantitative and qualitative.
Cause-and-effect describes the relationship between an event (cause) and a second event (effect), where the first event is understood to be responsible for the second. According to the Wiener's framework [73], the causality of a variable in relation to another can be measured by how well that variable helps to predict the other. Causality can take the form of directionality, information transfer and independence [74]. Cause-and-effect is also viewed as a combination of both factor relationships and causal interdependence [75].
Cause-and-effect in complex systems is not only linear but is also characterised by feedback which shows how actions reinforce or balance each other. Complex systems therefore, require tools that capture feedback structures. Human mental models are, however, based on linear thinking and as a result, often neglect feedback [76]. Consistent with Sterman's view [76], most cause-and-effect tools neglect feedback [74]. In such cases cause-and-effect is only described with respect to events rather than behaviour.
Analysis tools should be able to capture time delays and stock and flows [76]. Time delays, in combination with feedback, create system instability and the tendency to oscillate [77]. Stocks on the other hand act as delays or buffers in a system. Analysis tools should clearly present a mechanism for testing cause-and-effect logic [78]. This should be done to ensure validity of the revealed root cause. Accordingly, causality requires three conditions: (a) covariation of cause-and-effect, (b) temporal precedence (cause precedes the effect in time) and (c) non-spuriousness (no plausible alternative explanation) [79]. Moreover, the validity of causal connections in trees and diagrams should be governed by a set of logic rules called the "Categories of Legitimate Reservation" (CLR) [80]. The purpose of these rules as stated in Burns and Musa, is to espouse the criteria that govern causal connections acceptability [81]. There are eight logic rules categorised into three levels viz. level 1 reservation (clarity), level 2 reservations (entity existence, causality existence) and level 3 reservations (cause sufficiency, additional cause, cause-effect reversal, predicted effect, and tautology).
The clarity rule explains the extent to which a given model communicates the implied causality. It checks for complete understanding of the cause entity, effect entity and the causal link. Questions addressed by clarity rules include: (a) is the connection between cause and effect convincing at "face value"; (b) is there any verbal explanation required to understand cause and effect and; (c) is the link too long (i.e., missing intermediate steps). The entity existence rule verifies the existence of the statement or fact. It challenges the existence of either the cause or effect entity in reality. In causal existence, however, the existence of the link is called into question.
Level 3 reservations are used only after levels 1 and 2. The cause sufficiency rule examines whether a cause entity (on its own) is sufficient enough to have a specific effect. It asks the question "can the cause on its own create the effect or must it exist in concert with other causes?" The additional cause rule on the other hand, searches for the existence of a completely separate and independent cause to a specific effect. This reservation examines whether there are circumstances where the effect would still persist even after removing the cause in question. Cause-effect reversal questions the direction of causal links. This reservation is used to challenge the thought pattern where the cause and effect seem reversed. The predicted effect reservation searches for additional expected and verifiable effects of a particular cause. It seeks to determine whether the cause itself is tangible. If not, it searches whether there exists one or more additional predicted effects. Lastly, tautology or the circular logic reservation checks whether the effect is not a sole and insufficient proof offered for cause existence. Tautology is often a result of an abstract cause that is difficult to determine and define.
This section reviews some of the systemic diagnostic tools that are widely used in ISSPS and/or agricultural systems. A brief description of each tool including its history, application within agriculture and its limitations is provided. The review does not, however, represent an exhaustive list but rather focuses on tools that the researchers believe are suitable within agri-industrial systems. The reviewed tools are the current reality tree, fuzzy cognitive maps, network approaches, rich pictures, stock and flow diagram, causal loop diagram, and cause and effect diagram.
The current reality tree is a technique from Goldrattt's Theory of Constraints (TOC). The TOC as a methodology is premised on the assumption that within any system there exist a constraint or a few that limit systems' performance and that it is possible to identify such constraint(s) for improvement purposes. First developed by Eliyahu Goldratt in the late 1970's, TOC links hard and soft system issues [82].
Current reality trees (CRTs) are logic-based cause-and-effect tools that identify observed undesirable effects (UDE) and postulate probable causes. These UDE can be physical or non-physical. According to Oglethorpe and Heron, TOC tools encompass physical, behavioural, institutional, and political constraints [83]. Current reality trees are, however, most effective in policy-related constraints as opposed to physical [84]. This is largely due to their subjective approach. Machado used CRTs to capture factors that affected the efficiency of an ethanol supply chain [85]. Mena et al. applied CRTs when diagnosing causes of food waste in the UK and Spain [86]. Current reality trees have also been used to identify UDE in a fresh fruit and vegetables supply chain [87].
Logic rules, often referred to as Categories of Legitimate Reservation, are the core "ingredients" of CRTs construction. According to Kim et al., logic rules provide "analytical rigour" to CRTs modelling process [84]. They help a researcher identify the validity of the constructed logic relations. A current reality tree generally includes at least one positive feedback loop. The loop position provides guidance on remedial action as a change in or below the loop have an effect on the system [88].
Current reality trees can be drawn from interviews, brainstorms, open discussions, and/or a combination thereof. The complex nature of constructing CRTs and their logic system does not only make CRTs difficult to comprehend but also time-consuming. Goldratt alluded to the fact that CRTs require a skilled facilitator and cooperation from participants [9].
Fuzzy cognitive maps (FCMs) are signed digraph models first introduced by Kosko [89] as an extension to cognitive maps. They are a combination of cognitive maps with fuzzy logic and neural networks. Fuzzy cognitive maps depict and analyse human perceptions. Instead of only using signs to indicate the direction of cause-and-effect (as is the case with cognitive maps), FCMs also associate a weight with each causal link. Lopolito applied FCMs to capture stakeholders' perceptions in a bio-refinery [90]. Fairweather used FCMs to model perceptions in a dairy supply chain [91]. Fuzzy cognitive maps have further been used to diagnose collaboration issues [92], inter-firm trust [93], political forces [94], and cultural issues [95].
Fuzzy cognitive mapping is conducted through interviews, worksheets, pattern notes, and/or reports. The process involves: (a) the identification of key system concepts (trends, actions, events, or goals), (b) identification of causal relationships between concepts, and (c) determining the strength of each causal relationship. In a graphical form these concepts are represented as nodes and the causal relationships as edges . Edges express the type and degree of causality and can be one of three types; either positive , negative or no relationship whatsoever . Cheah et al. allude to the fact that in most cases a scheme of linguistic modifiers is prepared beforehand to convert discrete linguistic weights into continuous numerical values [96]. This is necessitated by the fact that most people relate easier to linguistic weights than numerical [96]. Papageorgiou and Salmeron argue that FCMs dynamics are based on first order logic and as such, FCMs cannot handle randomness associated with complex systems [97]. The actual mapping process itself can be demanding, especially when large systems with multiple nodes are considered. The combination of FCMs from different sources into a single map as indicated by Hanafizadeh and Aliehyaei [98], however, is oblivious of the fact that each individual map represents only a partial view of the system.
Network analysis approaches (NA) use techniques from graph theory, algebra and statistics to study relational and structural properties. Belamy and Basole are of the view that network analysis approaches offer a bridge between technical and social issues [99]. Network analysis approaches integrate qualitative and quantitative data and as such, are applied in both hard and soft contexts. Network analysis approaches have been used to diagnose issues within ISSPS [2,100,101]. They have been used to research collaboration issues [102], culture [103] and information sharing [104].
Network analysis approaches utilise information gathered through interviews and records. A network analysis model consists of a set of elements and a collection of links or connectors between these entities. Graph theory is applied to links to determine relationships between individual, detect singular nodes and to identify properties of the entire network. An important attribute of NA is finding actors that have a central position within a particular network. From graph theory, centrality has three measures viz. degree, betweenness and closeness [105]. Degree centrality describes the number of ties that a given node has whilst closeness is a measure of global centrality. A high degree centrality reflects high connectivity. Closeness centrality gives an estimate of how closely connected a node is to others in a particular network. Betweenness on the hand is a measure of brokerage and measures how often a particular node appears on the shortest path between nodes [106]. Researcher's perceptions in the network construction process could introduce bias into a map [2]. Also, large data can overwhelm generic network software [106]. Results from NA are only a "snapshot" in an evolution process and should therefore, not be generalised as they are time-specific.
Rich pictures (RP) are a flexible graphical tool from Peter Checkland's Soft Systems Methodology (SSM). Soft Systems Methodology is a popular soft approach widely used to unlock, structure and interpret social complexity [37]. It is premised on the fact that complex systems are social constructs characterised by multiple perspectives. Rich pictures as a tool provide a detailed representation of these problem contexts. According to Parker et al., RP give a broad, high-grained view of a problem context. Rich pictures perform three kinds of inquiries viz. intervention, social and political analysis [37]. Shongwe [1] and Gerwel-Proches [107] amongst others, used RP to diagnose systemic issues within the South African ISSPS.
Rich pictures can be drawn by participants and/or the facilitator in a participative environment or by a researcher during interviews. The drawing of RP, however, does not have a specific format or language but rather depends much on the skill and purposes of the person(s) doing the drawing. This characteristic makes third party interpretation difficult as people may mistake and misconstrue meaning. In addition, the whole rich picture process can take a long time to complete considering multiple revisions.
Causal loop diagrams (CLDs) are foundational System Dynamics tools used to conceptualise and structure complex issues. They seek to develop a holistic view of how relationships between variables influence the dynamics of a system. Causal loop diagrams are used to represent and communicate feedback. A causal loop diagram consists of variables connected by cause-and-effect links. These links have either a positive (+) or negative (−) polarity, which indicates the direction of causality between the variables when all other variables are conceptually constant. When the causal links close (in a circular fashion) feedback loops form and these are of two types viz. positive or negative. A negative feedback loop exhibits a goal-seeking behaviour whilst a positive loop shows a reinforcing behaviour.
Causal loop diagrams are developed from information gathered through interviews, observations, archives, and focus groups [76]. Causal loop diagrams have been used to diagnose issues in the Brazillian ISSPS [108]. Ibarra-Vega used CLDs to model waste management issues in a bioethanol plant [109]. Causal loop diagrams have further been used to diagnose supply chain collaboration, culture and strategic issues [110,111,112]. Causal loop diagrams do not distinguish between stock and flow structures and as a result the logic behind some causal links may be misinterpreted. Schaffernicht is of the view that the most common limitation of CLDs is mislabelling of loop polarity [113].
Unlike causal loop diagrams, stock and flow diagrams (SFDs) are a more detailed System Dynamics tool. They distinguish between the different types of variables and causal links. Stocks describe the state of the system over time and represent major accumulations whilst flow variables denote the rate of change in stock. Stocks provide systems with inertia and memory and as such, are a source of delays. They also decouple rates of flow, a characteristic that makes them to be a source of disequilibrium dynamics. Stock and flow diagrams have been used to model ethanol production in Mexico [114]. Sandvik and Moxnes used SFDs to evaluate the effects of ethanol production on the price of oil [115].
The construction of SFDs includes the identification of critical stocks, determining the flows and defining converters. Stock and flow diagrams can also be constructed by converting CLDs [113]. However, due to their technical orientation, SFDs are considered too complex to comprehend [116]. Lambert and Loiselle are of the view that SFDs often fail to communicate the location of feedback loops [117].
Cause and effect diagrams (CEDs) are used in many fields to identify and group potential causes to problems. The tool was first introduced by Kaoru Ishikawa in the early 1940's. Kumar and Nigmatulin used CEDs to determine demand uncertainty in a non-perishable food supply chain [118]. Trybus and Johnson applied CEDs to determine causes of food contamination [119]. Similarly, Mariajayaprakash and Senthilvelan used CEDs to identify parameters that caused conveyor failure at a sugar plant [120].
Cause and effect diagrams use interviews and brainstorming to identify potential causal factors. Andersen and Fagerhaug suggest a three-step procedure to drawing CEDs: (a) the problem is written on the right end of a large arrow, (b) the main categories that causes the problem are written as major branch arrows emanating from the main arrow and, (c) for each major branch, detailed causal factors are written as twigs, and these are analysed to determine the likely root causes [121]. According to Jayswal et al., the major categories should not exceed eight per diagram [122]. Cause and effect diagrams, however, do not show causal relationships between interrelated issues.
This section develops the ISSPS heuristic through synthesis of information discussed in Sections 4.1 and 4.2. Table 1 shows the performance of the various systemic tools discussed in Section 4.2 on the diagnostic criteria developed in Section 4.1. Also indicated in Table 1 is the suitability of each tool to diagnose issues on each of the ISSPS domains. Doggett used a combination of nominal and ordinal scales to compare the performance of root-cause analysis tools [75]. Jun et al. on the other hand, employed a cardinal relative scale to compare the performance of several methodologies on resource-based criteria [123]. Through the use of TOPSIS this research developed a qualitative heuristic that allows objective comparison of tools. The criteria and performance scores are discussed immediately after Table 1.
Tools | Diagnostic criteria | ||||||
Appreciation criteria | Analysis criteria | ||||||
Accessibility | Interactive | Iterative | Transparency | Feedback | Delays | Cause & effect logic | |
Cause and effect diagrams | 4 | 2 | 3 | 5 | 0 | 0 | 1 |
Causal loop diagrams | 3 | 3.5 | 4 | 4 | 5 | 4 | 3 |
Current reality trees | 3 | 5 | 2 | 5 | 2 | 0 | 5 |
Fuzzy cognitive maps* | 3.5 | 3 | 3 | 3 | 3 | 0 | 2 |
Rich pictures | 5 | 4 | 1 | 5 | 0 | 0 | 0 |
Network analysis approaches | 1 | 1 | 3 | 1 | 3 | 0 | 2 |
Stock and flow diagrams | 2 | 4 | 5 | 4 | 5 | 5 | 4 |
*Note: Should be applied with caution within the biophysical domain. |
The criteria as deliberated in the Section 4.1 are accessibility, iterativeness, interactiveness, transparency, feedback, time delays, and cause-and-effect logic. Cause-and-effect logic as a criterion was conceptualised along the eight logic rules viz. clarity, entity existence, causality existence, cause sufficiency, additional cause, cause-effect reversal, predicted effect, and tautology. As stated in Section 3, a score of 5 indicates excellent performance and 1, very poor. A score of zero (0) is used to specify no relationship whatsoever.
Ontologically, NA, RP and the CRTs are well-suited across all ISSPS domains. These three tools plus FCMs are the only tools suitable for less abstract problem contexts. The rest of the tools begin from a more structured context. As a result, CRTs have been applied before CLDs [124] and SFDs [125]. In the same vein, rich pictures have preceded CLDs [126], FCMs [98] and Bayesian networks [1]. Similarly, cognitive maps have been used with CLDs [127], and Bayesian networks [128].
Compared to the rest of the tools in the heuristic, RP are the most transparent and accessible tools more especially because humans identify easily with picture representation. Rich pictures were, however, the least iterative tool in the heuristic as the drawing of pictures cannot be "phased". Current reality trees on the other hand, were the most interactive of all the tools in Table 1. [129] posits that the CRTs logic and construction rules promote dialogue and discussion. A study by Doggett pointed out that CEDs were more accessible than CRTs [75]. As indicated in Table 1, RP do not have "analysis" capabilities and as a consequence, have a score of zero for feedback, cause-and-effect logic, and time delays.
Causal loop diagrams are less iterative and interactive compared to SFDs based on the fact that SFDs are constructed (sometimes) from CLDs [113]. Furthermore, the construction of SFDs requires a certain level of technical skills which renders them even less accessible than most of the tools in the heuristic. Compared to CLDs, FCMs are less interactive due to the fact that their causal links are based on first order logic, which happens to be the first step in the development of CLDs links. The use of language modifiers within FCMs reduces their transparency especially when compared to CED, CLDs, CRTs, RP and SFDs. Besides the language modifiers, FCMs are based on a "natural" language that is easily understood by most people hence, they were more accessible compared to CLDs, CRTs, NA and SFDs. Networks analysis approaches were least accessible, least transparent and were poor interactively compared to all the tools in Table 1. The use of special software immediately after compiling worldviews and the fact that cause-and-effect requires some knowledge of the entire system makes this tool less suitable for participatory modelling.
Network analysis approaches, FCMs, CRTs, CLDs and SFDs were the only tools in the heuristic that capture feedback. Nevertheless, feedback in NA, FCMs and CRTs is not conceptualised and signalled separately as is the case with CLDs and SFDs. Furthermore, these tools (NA, CRTs, and FCMs) do not capture feedback loop polarity. Feedback polarity is important for converting information about structure into behaviour. McNally views feedback in CRTs as "occasional" [130]. Youngman, however, argues that a current reality tree is not complete without a feedback loop [131].
None of the tools in Table 1 capture time delays except CLDs and SFDs. Park and Kim acknowledge this "weakness" with FCMs and suggest the use of dummy delay nodes in what they call "fuzzy time cognitive map" [132]. Between the two system dynamics tools, SFDs explicitly capture delays through stocks and decoupling rates whilst CLDs only indicate delays through a "hash" sign. Current reality trees critically validate cause-and-effect logic as their construction is based on CLR. Burns and Musa proposed that CLR should be incorporated into CLDs to improve model validity [81]. Cause and effect diagrams sort and relate causes within a classification schema. Hence, in terms of the cause-and-effect logic criterion, CEDs are susceptible to low clarity levels. The classification schema in general, makes the application of level 3 reservations difficult.
The nodes in FCMs and NA are more abstract (concepts) compared to those of CLDs and SFDs which utilise variables. Fuzzy cognitive maps and NA as such, are more susceptible to tautology than their System Dynamics counterparts. The circular conceptualisation of causality in System Dynamics in addition, is more rigorous compared to that of FCMs and NA. As a result, CLDs and SFDs are less prone to the cause-and-effect reversal rule. In relation to the cause sufficiency reservation, the use of Behaviour Over Time Charts within SFDs makes these superior to CLDs (Table 1).
All the tools in the heuristic could be applied across all the ISSPS domains except for FCMs which are explicitly subjective. Fuzzy cognitive maps' contribution lies within the soft paradigm rather than an objective world. This research therefore, recommends that FCMs should not be used in isolation when diagnosing issues within the biophysical domain. Despite the fact that all the System Dynamics tools in Table 1 could be applied across all domains, it is important to understand that SFDs assume quantifiable relations and as such, were historically favoured for the material world. However, variables that were previously omitted due to unavailability of data are now included with assumed mathematical relations that are transparent. Causal loop diagrams on the other hand, are mostly applied within the social world even though [133] argues that these contribute weakly socially since social practices are largely subjective.
Table 2 shows the output of the TOPSIS model obtained after using Table 1 as a decision matrix and assuming an equal criteria weight of 0.143 (). From this example SFDs were the highest ranked tools followed by CLDs . It is important to note that SFDs and CLDs were the only tools in the Table 1 that met all the criteria. Stock and flow diagrams, however, are superior to CLDs in terms of iterativeness, cause-and-effect logic and the time delays criterion. They were nonetheless, the second least accessible tool after NA (Table 1) since they are considered too complex to comprehend. Hence, as advocated for by [81], the use of SFDs can be strengthened by the adoption of all CLR.
Tool | Rank | |||
Cause and effect diagrams | 0.169 | 0.083 | 0.329 | 6 |
Causal loop diagrams | 0.063 | 0.158 | 0.715 | 2 |
Current reality trees | 0.136 | 0.134 | 0.495 | 3 |
Fuzzy cognitive maps | 0.142 | 0.091 | 0.393 | 4 |
Rich pictures | 0.182 | 0.096 | 0.346 | 5 |
Network approaches | 0.170 | 0.071 | 0.295 | 7 |
Stock and flow diagrams | 0.056 | 0.184 | 0.765 | 1 |
*Note: = separation from positive ideal solution. = separation from negative ideal solution. = relative closeness to ideal solution. |
The low rank of NA in Table 2 is a consequence of its low performance on the appreciation criteria viz. accessibility, interactiveness and transparency (Table 1). The ranking of CEDs on the other hand, was low because these tools have less analysis capabilities (only cause-and-effect logic). Although RP are strictly appreciation tools, they were, however, ranked higher than CEDs (Table 2) owing to the fact that RP performed better than CEDs on the accessibility and interactiveness criteria. Cause and effect diagrams and RP, however, can be used in tandem with other analysis tools. Doggett suggested that CEDs could be used in tandem with CRTs where the output from CEDs is used to develop a list of UDEs for the current reality tree [75]. As seen in Table 2, the selection criteria and the criteria weight had a huge influence on the tools ranking. A change in criteria, for example to appreciation criteria only, could probable result in a different conclusion. Similarly, a change in criteria weight is expected to have an impact on rankings.
Using the equal weighting (0.143) as a basis, sensitivity analysis of the tools to criteria weight was conducted. The analysis followed a method by [134] where the weight of each criterion is varied whilst that of other criteria is multiplied by a common ratio. For application examples of this method refer to [135]. In this study, sensitivity analysis was conducted by varying the appreciation criteria viz. accessibility, interactiveness, iterativeness, and transparency. Selection of these criteria was founded on the fact that the performance of all the tools against the appreciation criteria was more than zero (Table 1). Figure 3 shows the results of the sensitivity analysis where the weight of (a) accessibility, (b) interactiveness (c) iterativeness, and (d) transparency increased from scenario 1 to scenario 9 towards a value of 1.
All the tools were sensitive to criteria weights, which is critical for the MCDA model (Figure 3). Stock and flow diagrams were the most sensitive as indicated by the change in rankings throughout the criteria (6 in Figure 3 (a), 2 in (b), 1 in (c), and 4 in (d)). The sensitivity of SFDs under the iterativeness criterion (Figure 3 (c)), however, was low as these tools were ranked first in almost all of the scenarios. This is a result of the fact that SFDs were strong iteratively compared to the rest of the tools in Table 1. Network analysis approaches were the least sensitive as revealed by their continuous low rank (7) in the accessibility, interactiveness, and the transparency criteria. This is compatible to the low performance scores for these criteria as indicated in Table 1. Similarly, high performance scores (5) in the transparency and interactiveness criteria resulted in a positive rank change for CRTs to first.
The complex nature of ISSPS makes it practically difficult to diagnose issues that constrain productivity within these systems. The matter is further complicated by the fact that most of the diagnostic tools available are only tailored for specific problem contexts. As such, selecting appropriate tool(s) for the diagnosis of complex ISSPS issues becomes a challenge. This research developed a diagnostic heuristic that will be used to compare and to select diagnostic tools. The heuristic offers a basis for the construction of comprehensive methodological approaches and as such, will improve the efficiency of systemic diagnosis of issues in such systems. The attributes of ISSPS make the heuristic a relatively general approach to integrated agricultural supply and processing systems. It is therefore envisaged that the heuristic will be transferable to other agri-industrial systems.
Systemic diagnostic criteria were developed and a suite of diagnostic tools was compiled. The performance of each tool on the criteria was determined for tool comparison and/or selection. The suitability of each tool to diagnose issues within each of the ISSPS domains was also determined. The diagnostic criteria were accessibility, interactiveness, iterativeness, transparency, feedback, cause-and-effect logic, and time delays. The suite of tools consisted of CEDs, CLDs, CRTs, NA, RP, and SFDs. It was shown in the study that each tool provides a different facet to complexity. Hence, the apparent need for multimethodology in ISSPS. All of the tools in the heuristic could be applied across both criteria (appreciation and analysis) except for RP. It was further revealed that issues in the soft (culture, collaboration and political forces) and strategic domains (environment, future strategy and structure) could be diagnosed by any of the tools in the suite. Issues in the material world (biophysical domain), however, could not be fully diagnosed by FCMs as these tools are explicitly subjective. Sensitivity analysis of the TOPSIS model revealed that SFDs were the most sensitive tools in the heuristic whilst NA were least sensitive. The study excluded resource-based criteria (e.g., time and cost) as these are not entirely dependent on the tool. For future research it is recommended that such criteria be incorporated into the heuristic. With such criteria incorporated, pairwise comparison of tools by industry experts could be explored. It is further recommended that the heuristic be demonstrated in an actual ISSPS.
The authors declare no conflict of interest.
[1] | Shongwe MI (2018) A systems thinking approach to investigating complex sugarcane supply and processing systems: Integrating rich pictures and Bayesian networks. Syst Pract Action Res 31: 75–85. |
[2] | Bezuidenhout CN, Kadwa M, Sibomana MS (2013) Using theme and domain networking approaches to understand complex agriindustrial systems: A demonstration from the south african sugar industry. Outlook Agric 42: 9–16. |
[3] | Bezuidenhout CN, Bodhanya S, Sanjika T, et al. (2011) Network-analysis approaches to deal with causal complexity in a supply network. Int J Prod Res 50: 1840–1849. |
[4] | Giles RC, Bezuidenhout CN, Lyne PWL (2008) Evaluating the feasibility of a sugarcane vehicle delivery scheduling system-A theoretical study. Int Sugar J 110: 242–247. |
[5] | Bezuidenhout, CN (2008) A Farmers market at the local sugar mill: Lean versus agile. In: Proceedings of the South African Sugar Technologists Association, Durban, 81: 68–71. |
[6] | Kadwa M, Bezuidenhout CN, Ferrer SRD (2012) Cane supply benefits associated with the mitigation of labour absenteeism in the Eston sugarcane supply chain. In: Proceedings of the South African Sugar Technologists Association, Durban, 85: 47–49. |
[7] | Bezuidenhout CN, Baier TJA (2011) An evaluation of the literature on integrated sugarcane production systems: A scientometrical approach. Outlook Agric 40: 79–88. |
[8] | Ashby WR (1958) Requisite variety and its implications for the control of complex systems. Cybernetica 1: 83–99. |
[9] | Goldratt EM (1990) What Is This Thing Called Theory Of Constraints And How Should It Be Implemented? Great Barrington: North River Press. |
[10] | Lichtenstein BB, Plowman DA (2009) The leadership of emergence: A complex systems leadership theory of emergence at successive organizational levels. Leadersh Q 20: 617–630. |
[11] | Helbing D (2013) Globally networked risks and how to respond. Nature 497: 51–59. |
[12] | Gigerenzer G, Gaissmaier W (2011) Heuristic decision making. Annu Rev Psychol 62: 451–482. |
[13] | Dietrich C (2010) Decision making: Factors that influence decision making, heuristics used, and decision outcomes. Inq J Stud Pulse 2: 1–3. |
[14] | Gerwel CN, Hildbrand S, Bodhanya SA, et al. (2011) Systemic approaches to understand the complexities at the Umfolozi and Felixton mill areas. In Proceedings of the 84th South African Sugar Technologists' Association, 177–181. |
[15] | Childerhouse P, Towill DR (2011) Effective supply chain research via the quick scan audit methodology. Supply Chain Manag An Int J 16: 5–10. |
[16] | Singh J, Singh H (2015) Continuous improvement philosophy–literature review and directions. Benchmarking An Int J 22: 75–119. |
[17] | Yatskovskaya E, Srai JS, Kumar M (2018) Integrated supply network maturity model: Water scarcity perspective. Sustain 10: 896–921. |
[18] | Schut M, Rodenburg J, Klerkx L, et al. (2015) RAAIS: Rapid Appraisal of Agricultural Innovation Systems (Part Ⅱ). Integrated analysis of parasitic weed problems in rice in Tanzania. Agric Syst 132: 12–24. |
[19] | Higgins AJ, Miller CJ, Archer AA, et al. (2010) Challenges of operations research practice in agricultural value chains. J Oper Res Soc 61: 964–973. |
[20] | Mingers J (2003) A classification of the philosophical assumptions of management science methods. J Oper Res Soc 54: 559–570. |
[21] | Zawedde A, Lubega J, Kidde S, et al. (2010) Methodological pluralism: An emerging paradigmatic approach to information systems research. In: Strengthening the Role of ICT in Development, Kizza MJ, Lynch K, Aisbett J, et al., Ed., Kampala, 99–128. |
[22] | Wilding R, Humphries A (2009) Building relationships that create value. In: Dynamic Supply Chain Alignment: A New Business Model for Peak Performance in Enterprise Supply Chains Across All Geographies; Gattorna JL, Ed., London, 67–80. |
[23] | Schein EH (2010) Organizational Culture and Leadership, John Wiley & Sons: San Francisco. |
[24] | Koumparoulis DN (2013) PEST Analysis: The case of E-shop. Int J Econ Manag Soc Sci 2: 31–36. |
[25] | Green P, Hardman S (2013) A conceptual framework for evaluating an academic department: A systems approach. Int Bus Econ Res J 12: 1535–1546. |
[26] | Mingers J (1997) Multi-paradigm multimethodology. In: Multimethodology: The Theory and Practice of Combining Management Science Methodologies, Mingers J, Gill A, Ed., Wiley & Sons: Chichester, 1–22. |
[27] | Mingers J, Brocklesby J (1997) Multimethodology: Towards a framework for mixing methodologies. Omega 25: 489–509. |
[28] | Westwood R, Clegg S (2009) The discourse of organisation studies: dissensus, politics, and paradigms. In: Debating Organisation: Point-Counterpoint in Organisation Studies, Westwood R, Clegg S, Ed., Blackwell: Oxford, 1–42. |
[29] | Pollack J (2009) Multimethodology in series and parallel: Strategic planning using hard and soft OR. J Oper Res Soc 60: 156–167. |
[30] | Creswell JW, Miller DL (2000) Determining validity in qualitative inquiry. Theory Pract 39: 124–130. |
[31] | Kivunja C, Kuyini AB (2017) Understanding and applying research paradigms in educational contexts. Int J High Educ 6: 26–41. |
[32] | Habermas J (1984) The Theory of Communicative Action: Reason and the Rationalization of Society. Beacon Press, Boston. |
[33] | Midgley G (2011) Theoretical pluralism in systemic action research. Syst Pract Action Res 24: 1–15. |
[34] | Ferreira JS (2013) Multimethodology in Metaheuristics. J Oper Res Soc 64: 876–883. |
[35] | Bekhet AK, Zauszniewski JA (2012) Methodological triangulation: An approach to understanding data. Nurse Res 20: 40–43. |
[36] | Bhaskar R (1981) The Possibility of Naturalism. Harvester Press, Sussex. |
[37] | Checkland P, Poulter J (2006) Learning For Action: A Short Definitive Account of Soft Systems Methodology, and its use Practitioners, Teachers and Students. Wiley & Sons: Chichester. |
[38] | Zhu Z (2011) After paradim: Why mixing-methodology theorising fails and how to make it work again. J Oper Res Soc 62: 784–798. |
[39] | Kuhn TS (1962) The Structure of Scientific Revolutions. University of Chicago Press: Chicago. |
[40] | Harwood SA (2011) Mixing methodologies and paradigmatic commensurability. J Oper Res Soc 62: 806–809. |
[41] | Callaghan CW (2016) Critical theory and contemporary paradigm differentiation. Acta Commer 16: 59–99. |
[42] | Habermas J (1972) Knowledge and Human Interests. Heinemann, London. |
[43] | Jackson MC (2013) Systems Methodology for the Management Sciences. Springer, New York. |
[44] | Mingers J (2001) Combining IS research methods: Towards a pluralist methodology. Inf Syst Res 12: 240–259. |
[45] | Midgley G (1997) Mixing methods: Developing systemic intervention. In: Multimethodology: The Theory and Practice of Integrating OR and Systems Methodologies; Mingers J, Gill A, Ed., Wiley, Chichester, 249–290. |
[46] | Jackson MC (1991) Systems Methodology for the Management Sciences. Plenum, New York. |
[47] | Angelis A, Kanavos P (2017) Multiple criteria decision analysis (MCDA) for evaluating new medicines in health technology assessment and beyond: The advance value framework. Soc Sci Med 188: 137–156. |
[48] | Behzadian M, Otaghsara SK, Yazdani M, et al. (2012) A state-of the-art survey of TOPSIS applications. Expert Syst Appl 39: 13051–13069. |
[49] | Kelemenis A, Askounis D (2010) A new TOPSIS-based multi-criteria approach to personnel selection. Expert Syst Appl 37: 4999–5008. |
[50] | Gaucher S, Le Gal PY, Soler LG (2004) Modelling supply chain management in the sugar industry. Sugar Cane Int 22: 8–16. |
[51] | Wynne AT, Murray TJ, Gabriel AB (2009) Relative cane payment: realigning grower incentives to optimise sugar recoveries. In Proceedings of the 82nd Annual Congress-South African Sugar Technologists' Association : Durban, 50–57. |
[52] | Horn RE, Weber RP (2007) New Tools for Resolving Wicked Problems: Mess Mapping and Resolution Mapping Processes; Strategy Kinetics LLC: Watertown. |
[53] | Wexler MN (2009) Exploring the moral dimension of wicked problems. Int J Sociol Soc Policy 29: 531–542. |
[54] | Ackoff RL (1978) The Art of Problem Solving. Wiley, New York. |
[55] | Mintzberg H, Raisinghani D, Thérêt A (1976) The structure of "unstructured" decision processes. Adm Sci Q 21: 246–275. |
[56] | Nelson GH, Stolterman E (2012) The Design Way: Intentional Change in an Unpredictable World. MIT Press, Cambridge. |
[57] | Simon HA, Dantzig GB, Hogarth R, et al. (1987) Decision making and problem solving. Interfaces (Providence)17: 11–31. |
[58] | Davies J, Mabin VJ, Balderstone SJ (2005) The theory of constraints: A methodology apart? — A comparison with selected OR/MS methodologies. Omega 55: 506–524. |
[59] | Houghton L, Tuffley D (2015) Towards a methodology of wicked problem exploration through concept shifting and tension point analysis. Syst Res Behav Sci 32: 283–297. |
[60] | Camillus JC (2008) Strategy as a wicked problem. Harv Bus Rev 86: 98–106. |
[61] | Yanow D, Schwartz-Shea P (2015) Interpretive Approaches to Research Design: Concepts and Processes. Routledge, New York. |
[62] | Zlatanovic D (2017) A multi-methodological approach to complex problem solving: The case of Serbian enterprise. Systems 5: 40–55. |
[63] | Small A, Wainwright D (2014) SSM and technology management: Developing multimethodology through practice. Eur J Oper Res 233: 660–673. |
[64] | Mingers J, Rosenhead J (2004) Problem structuring methods in action. Eur J Oper Res 152: 530–554. |
[65] | Von Korff Y, Daniell KA, Moellenkamp S, et al. (2012) Implementing participatory water management: Recent advances in theory, practice, and evaluation. Ecol Soc 17: 30–44. |
[66] | Belton V, Stewart T (2010) Problem structuring and multiple criteria decision analysis. In: Trends in Multiple Criteria Decision Analysis, Greco S, Ehrgott M, Figueira JR, Ed.; Springer Science & Business Media, New York, 209–239. |
[67] | Franco LA, Montibeller G (2010) Facilitated modelling in operational research. Eur J Oper Res 205: 489–500. |
[68] | Rosenhead J (1996) What's the problem? An introduction to problem structuring methods. Interfaces (Providence) 26: 117–131. |
[69] | Sibbesen LK, Leleur S (2006) Decision support and multimethodology: Diffrent strategies for combination of OR methods Available from: http://www.feg.unesp.br/~fmarins/seminarios/MaterialdeLeitura/artigosm%E9todos/Sibbesen&_Leleur-Multimethodology.pdf (accessed on Mar 15, 2016). |
[70] | Rosenhead J (1992) Into the swamp: The analysis of social issues. J Oper Res Soc 43: 293–305. |
[71] | Myllyviita T, Hujala T, Kangas A, et al. (2014) Mixing methods–assessment of potential benefits for natural resources planning. Scand J For Res 29: 20–29. |
[72] | Raia F (2008) Causality in complex dynamic systems : A challenge in earth systems science education. J Geosci Educ 56: 81–94. |
[73] | Wiener N (1966) I Am a Mathematician: The Later Life of a Prodigy. MIT Press, Massachusettts. |
[74] | Razak AF, Jensen HJ (2014) Quantifying "causality" in complex systems: Understanding transfer entropy. PLoS One 9: 1–14. |
[75] | Doggett AM (2005) Root cause analysis: A framework for tool selection. Qual Manag J 12: 34–45. |
[76] | Sterman JD (2000) Business Dynamics: Systems Thinking and Modeling for a Complex World. McGraw-Hill, Boston. |
[77] | Schaffernicht M, Groesser SN (2011) A comprehensive method for comparing mental models of dynamic systems. Eur J Oper Res 210: 57–67. |
[78] | Goldratt EM (1992) The Jonah Program. The Goldratt Institute, New Hampshire. |
[79] | Cook TD, Campbell DT, Shadish W (2002) Experimental and Quasi-Experimental Designs for Generalized Causal Inference. Houghton Mifflin, Boston. |
[80] | Dettmer HW (2007) The Logical Thinking Process: A Systems Approach to Complex Problem Solving. ASQ Quality Press, Milwaukee. |
[81] | Burns JR, Musa P (2001) Structural validation of causal loop diagrams. Proc 19th Int Conf Syst Dyn Soc, 1–13. |
[82] | Siriram R (2012) A soft and hard systems approach to business process management. Syst Res Behav Sci 29: 87–100. |
[83] | Oglethorpe D, Heron G (2013) Testing the theory of constraints in UK local food supply chains. Int J Oper Prod Manag 33: 1346–1367. |
[84] | Kim S, Mabin VJ, Davies J (2008) The theory of constraints thinking processes: Retrospect and prospect. Int J Oper Prod Manag 28: 155–184. |
[85] | Machado RL (2015) An analysis of the Brazilian ethanol supply chain. In Proceedings of 26th POMS Annual Conference. |
[86] | Mena C, Adenso-Diaz B, Yurt O (2011) The causes of food waste in the supplier-retailer interface: Evidences from the UK and Spain. Resour Conserv Recycl 55: 648–658. |
[87] | Taylor LJ, Esan TO (2012) Goldratt's theory applied to the problems associated with the mode of transportation, storage and sale of fresh fruits and vegetables in Nigeria. J African Re Bus Technol 2012: 1–16. |
[88] | Gupta A, Bhardwaj A, Kanda A (2010) Fundamental concepts of theory of constraints: An emerging philosophy. World Acad Sci Eng Technol 46: 686–692. |
[89] | Kosko B (1986) Fuzzy cognitive maps. Int J Man–Mach Stud 24: 65–75. |
[90] | Lopolito A, Prosperi M (2009) Socio-economic implications of the development of a bio-refinery: An analysis with fuzzy cognitive maps. Landscape 1: 2–27. |
[91] | Fairweather J (2010) Farmer models of socio-ecologic systems: Application of causal mapping across multiple locations. Ecol Modell 221: 555–562. |
[92] | Buyukozkan G, Vardaloglu Z (2009) Analyzing of collaborative planning, forecasting and replenishment approach using fuzzy cognitive map. In Proceedings of the 2009 International Conference on Computers & Industrial Engineering; Kacem I, Ed., Institute of Electrical and Electronic Engineers Inc, New York, 1763–1768. |
[93] | Abbas NH (2014) The impact of trust relationships on environmental management in north Lebanon. University of Twente. |
[94] | Al Shayji S, El Kadhi NEZ, Wang Z (2011) Fuzzy cognitive map theory for the political domain. In Proceedings of the Federated Conference on Computer Science and Information Systems; Ganzha M, Maciaszek L, Paprzycki M, Ed.; IEEE, 179–186. |
[95] | Ruan D, Mkrtchyan L (2012) Using belief degree-distributed fuzzy cognitive maps for safety culture assessment. Adv Intell Soft Comput 124: 501–510. |
[96] | Cheah WP, Kim YS, Kim KY, et al. (2011) Systematic causal knowledge acquisition using FCM constructor for product design decision support. Expert Syst Appl 38: 15316–15331. |
[97] | Papageorgiou EI, Salmeron JL (2013) A review of fuzzy cognitive maps research during the last decade. IEEE Trans Fuzzy Syst 21: 66–79. |
[98] | Hanafizadeh P, Aliehyaei R (2011) The application of fuzzy cognitive map in soft system methodology. Syst Pract Action Res 24: 325–354. |
[99] | Bellamy MA, Basole RC (2013) Network analysis of supply chain systems: A systematic review and future research. Syst Eng 16: 235–249. |
[100] | Kadwa M, Bezuidenhout CN, Ortmann GF (2014) Quantifying and modelling disruptions in the Eston sugarcane supply chain. In Proceedings of the South African Sugarcane Technologists Association 87, Durban, 474–477. |
[101] | Sanjika TM, Bezuidenhout CN, Bodhanya S, et al. (2012) A network analysis approach to identify problems in integrated sugarcane production and processing systems. In Proceedings of the 85th South African Sugar Technologists Association, 50–53. |
[102] | Borg R, Toikka A, Primmer E (2015) Social capital and governance: A social network analysis of forest biodiversity collaboration in Central Finland. For Policy Econ 50: 90–97. |
[103] | Zagenczyk TJ, Scott KD, Gibney R, et al. (2010) Social influence and perceived organizational support: A social networks analysis. Organ Behav Hum Decis Process 111: 127–138. |
[104] | Capo-Vicedo J, Mula J, Capo J (2011) A social network-based organizational model for improving knowledge management in supply chains. Supply Chain Manag Int J, 16: 379–388. |
[105] | Baruah D, Bharali A (2017) A comparative study of vertex deleted centrality measures. Ann Pure Appl Math 14: 199–205. |
[106] | Martinez-Lopez B, Perez AM, Sanchez-Vizcaino JM (2009) Social network analysis-review of general concepts and use in preventive veterinary medicine. Transbound Emerg Dis 56: 109–120. |
[107] | Gerwel-Proches CN, Bodhanya S (2015) An application of Soft Systems Methodology in the sugar industry. Int J Qual Methods 14: 1–15. |
[108] | Mishra MK, Khare N, Agrawal AB (2004) Bagasse cogeneration in India: Status, barriers. IOSR J Mech Civ Eng 11: 69–78. |
[109] | Ibarra-Vega DW (2016) Modeling waste management in a bioethanol supply chain: A system dynamics approach. Dyna 83: 99–104. |
[110] | Lourenzani AEB, Silva AL (2010) Systematic model of collective actions: Evidences from Brazilian agribusiness. In: 5th Research Workshop On Institutions And Organizations. Concalves, Brazil. |
[111] | Mathew AO, Rodrigues LLR, Vittaleswar A (2012) Human factors & knowledge management : A system dynamics based analysis. J Knowl Manag Pract 13: 1–21. |
[112] | bitrus Goyol A, Dala BG. Causal loop diagrams (CLD) as an instrument for strategic planning process. Int J Bus Manag 9: 77–89. |
[113] | Schaffernicht M (2010) Causal loop diagrams between structure and behaviour: A critical analysis of the relationship between polarity, behaviour and events. Syst Res Behav Sci 27: 653–666. |
[114] | Rendon-Sagardi MA, Sanchez-Ramirez C, Cortes-Robles G, et al. (2014) Dynamic analysis of feasibility in ethanol supply chain for biofuel production in Mexico. Appl Energy 123: 358–367. |
[115] | Sandvik S, Moxnes E (2009) Peak oil, biofuels, and long-term food security. In: Proceedings of the 27th International Conference of the System Dynamics Society; Ford A, Ford DN, Anderson EG, Ed.; System Dynamics Society: New York, 1–19. |
[116] | Zlatanovic D (2012) System dynamics models in management problems solving. Econ Horiz 14: 25–38. |
[117] | Lambert SD, Loiselle CG (2008) Combining individual interviews and focus groups to enhance data richness. J Adv Nurs 62: 228–237. |
[118] | Kumar S, Nigmatullin A (2011) A system dynamics analysis of food supply chains-Case study with non-perishable products. Simul Model Pract Theory 19: 2151–2168. |
[119] | Trybus E, Johnson G (2010) The role of supply chain product safety: A study on food safety regulations. Calif J Oper Manag 8: 93–99. |
[120] | Mariajayaprakash A, Senthilvelan T (2013) Failure detection and optimization of sugar mill boiler using FMEA and Taguchi method. Eng Fail Anal 30: 17–26. |
[121] | Andersen B, Fagerhaug T (2006) Root Cause Analysis: Simplified Tools and Techniques; ASQ Quality Press: Milwaukee. |
[122] | Jayswal A, Li X, Zanwar A, et al. (2011) A sustainability root cause analysis methodology and its application. Comput Chem Eng 35: 2786–2798. |
[123] | Jun GT, Morris Z, Eldabi T, et al (2011) Development of modelling method selection tool for health services management: From problem structuring methods to modelling and simulation methods. BMC Health Serv Res 11: 108–119. |
[124] | Mohammadi H, Ghazanfari M, Nozari H, et al. (2015) Combining the theory of constraints with system dynamics: A general model (case study of the subsidized milk industry). Int J Manag Sci Eng Manag 10: 102–108. |
[125] | Ahmad N, Zulkepli J, Ramli R, et al. (2017) Understanding the dynamic effects of returning patients toward emergency department density. In: Proceedings of the AIP Conference; Ibrahim H, Aziz N, Zulkepli J, et al., Ed., AIP Publishing: USA. |
[126] | Setianto NA, Cameron D, Gaughan JB (2014) Identifying archetypes of an enhanced system dynamics causal loop diagram in pursuit of strategies to improve smallholder beef farming in Java, Indonesia, Syst Res Behav Sci 31: 642–654. |
[127] | Duryan M, Nikolik D, van Merode G, et al. (2014) Using cognitive mapping and qualitative system dynamics to support decision making in intellectual disability care. J Policy Pract Intellect Disabil 11: 245–254. |
[128] | Wee YY, Cheah WP, Tan SC, et al. (2015) A method for root cause analysis with a Bayesian belief network and fuzzy cognitive map. Expert Syst Appl 42: 468–487. |
[129] | Doggett AM (2004) A statistical comparison of three root cause analysis tools. J Ind Technol 20: 20–28. |
[130] | McNally R (2011) Thinking with Flying Logic; Sciral: Glendora. |
[131] | Youngman KJA (2016) A guide to implementing the Theory of Constraints (TOC) Available from: http://www.dbrmfg.co.nz/ThinkingProcessCRT.htm (accessed on Mar 15, 2016). |
[132] | Park KS, Kim HS (1995) Fuzzy cognitive maps considering time relationships. Int J Hum Comput Stud 42: 157–168. |
[133] | Mingers J (2006) Philosophical foundations: Critical realism. In Realising Systems Thinking: Knowledge and Action in Management Science; Mingers J, Ed.; Springer: New Jersey, 11–31. |
[134] | Alinezhad A, Amini A, Alinezhad A (2011) Sensitivity analysis of TOPSIS technique: The result of change in the weight of one attribute on the final ranking of alternatives. J Ind Eng 7: 23–28. |
[135] | Hanine M, Boutkhoum O, Tikniouine A, et al. (2016) Application of an integrated multi-criteria decision making AHP-TOPSIS methodology for ETL software selection. Springerplus 5: 263–279. |
1. | Mohammad Ridwane Mungroo, Ayaz Anwar, Naveed Ahmed Khan, Ruqaiyyah Siddiqui, Gold-Conjugated Curcumin as a Novel Therapeutic Agent against Brain-Eating Amoebae, 2020, 5, 2470-1343, 12467, 10.1021/acsomega.0c01305 | |
2. | A F Fudhlaa, W Rachmawati, D Retnowati, Analysis of sugar import policy effects on sugar cane farmer’s income in East Java: A system dynamic approach, 2021, 1072, 1757-8981, 012023, 10.1088/1757-899X/1072/1/012023 | |
3. | Huilin Chen, Zheyi Chen, Feiting Lin, Peifen Zhuang, Effective Management for Blockchain-Based Agri-Food Supply Chains Using Deep Reinforcement Learning, 2021, 9, 2169-3536, 36008, 10.1109/ACCESS.2021.3062410 | |
4. | Renu Mishra, Mohammad Kaif, Ayush Raj, Ritika Deep, 2023, Block chain Enabled Farmer Centric Supply chain Management using Reinforcement learning, 979-8-3503-3509-5, 1, 10.1109/ICCCNT56998.2023.10307187 | |
5. | Leyla Beba Pozharani, Esra Baloglu, Kaya Suer, Emrah Guler, E. Vildan Burgaz, Imge Kunter, Development and optimization of in-situ gels for vaginal delivery of metronidazole and curcumin via box-behnken design: In vitro characterization and anti-trichomonas activity, 2023, 86, 17732247, 104739, 10.1016/j.jddst.2023.104739 |
Tools | Diagnostic criteria | ||||||
Appreciation criteria | Analysis criteria | ||||||
Accessibility | Interactive | Iterative | Transparency | Feedback | Delays | Cause & effect logic | |
Cause and effect diagrams | 4 | 2 | 3 | 5 | 0 | 0 | 1 |
Causal loop diagrams | 3 | 3.5 | 4 | 4 | 5 | 4 | 3 |
Current reality trees | 3 | 5 | 2 | 5 | 2 | 0 | 5 |
Fuzzy cognitive maps* | 3.5 | 3 | 3 | 3 | 3 | 0 | 2 |
Rich pictures | 5 | 4 | 1 | 5 | 0 | 0 | 0 |
Network analysis approaches | 1 | 1 | 3 | 1 | 3 | 0 | 2 |
Stock and flow diagrams | 2 | 4 | 5 | 4 | 5 | 5 | 4 |
*Note: Should be applied with caution within the biophysical domain. |
Tool | Rank | |||
Cause and effect diagrams | 0.169 | 0.083 | 0.329 | 6 |
Causal loop diagrams | 0.063 | 0.158 | 0.715 | 2 |
Current reality trees | 0.136 | 0.134 | 0.495 | 3 |
Fuzzy cognitive maps | 0.142 | 0.091 | 0.393 | 4 |
Rich pictures | 0.182 | 0.096 | 0.346 | 5 |
Network approaches | 0.170 | 0.071 | 0.295 | 7 |
Stock and flow diagrams | 0.056 | 0.184 | 0.765 | 1 |
*Note: = separation from positive ideal solution. = separation from negative ideal solution. = relative closeness to ideal solution. |
Tools | Diagnostic criteria | ||||||
Appreciation criteria | Analysis criteria | ||||||
Accessibility | Interactive | Iterative | Transparency | Feedback | Delays | Cause & effect logic | |
Cause and effect diagrams | 4 | 2 | 3 | 5 | 0 | 0 | 1 |
Causal loop diagrams | 3 | 3.5 | 4 | 4 | 5 | 4 | 3 |
Current reality trees | 3 | 5 | 2 | 5 | 2 | 0 | 5 |
Fuzzy cognitive maps* | 3.5 | 3 | 3 | 3 | 3 | 0 | 2 |
Rich pictures | 5 | 4 | 1 | 5 | 0 | 0 | 0 |
Network analysis approaches | 1 | 1 | 3 | 1 | 3 | 0 | 2 |
Stock and flow diagrams | 2 | 4 | 5 | 4 | 5 | 5 | 4 |
*Note: Should be applied with caution within the biophysical domain. |
Tool | Rank | |||
Cause and effect diagrams | 0.169 | 0.083 | 0.329 | 6 |
Causal loop diagrams | 0.063 | 0.158 | 0.715 | 2 |
Current reality trees | 0.136 | 0.134 | 0.495 | 3 |
Fuzzy cognitive maps | 0.142 | 0.091 | 0.393 | 4 |
Rich pictures | 0.182 | 0.096 | 0.346 | 5 |
Network approaches | 0.170 | 0.071 | 0.295 | 7 |
Stock and flow diagrams | 0.056 | 0.184 | 0.765 | 1 |
*Note: = separation from positive ideal solution. = separation from negative ideal solution. = relative closeness to ideal solution. |