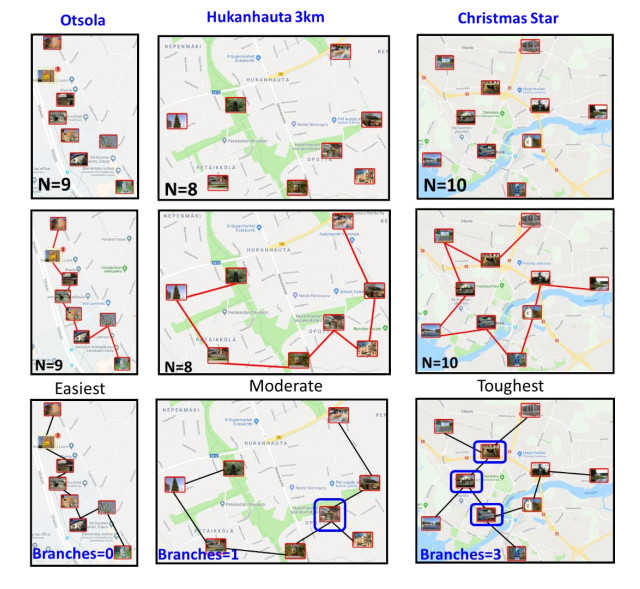
From the theory of algorithms, we know that the time complexity of finding the optimal solution for a traveling salesman problem (TSP) grows exponentially with the number of targets. However, the size of the problem instance is not the only factor that affects its difficulty. In this paper, we review existing measures to estimate the difficulty of a problem instance. We also introduce MST branches and two other measures called greedy path and greedy gap. The idea of MST branches is to generate minimum spanning tree (MST) and then calculate the number of branches in the tree. A branch is a target, which is connected to at least two other targets. We perform an extensive comparison of 11 measures to see how well they correlate to human and computer performance. We evaluate the measures based on time complexity, prediction capability, suitability, and practicality. The results show that while the MST branches measure is simple, fast to compute, and does not need to have the optimal solution as a reference unlike many other measures. It correlates equally good or even better than the best of the previous measures ‑ the number of targets, and the number of targets on the convex hull.
Citation: Lahari Sengupta, Pasi Fränti. Comparison of eleven measures for estimating difficulty of open-loop TSP instances[J]. Applied Computing and Intelligence, 2021, 1(1): 1-30. doi: 10.3934/aci.2021001
[1] | Pasi Fränti, Jun Shen, Chih-Cheng Hung . Applied Computing and Intelligence: A new open access journal. Applied Computing and Intelligence, 2024, 4(1): 19-23. doi: 10.3934/aci.2024002 |
[2] | Guanyu Yang, Zihan Ye, Rui Zhang, Kaizhu Huang . A comprehensive survey of zero-shot image classification: methods, implementation, and fair evaluation. Applied Computing and Intelligence, 2022, 2(1): 1-31. doi: 10.3934/aci.2022001 |
[3] | Pasi Fränti, Lingyi Kong . Puzzle-Mopsi: A location-puzzle game. Applied Computing and Intelligence, 2023, 3(1): 1-12. doi: 10.3934/aci.2023001 |
[4] | Elizaveta Zimina, Kalervo Järvelin, Jaakko Peltonen, Aarne Ranta, Kostas Stefanidis, Jyrki Nummenmaa . Linguistic summarisation of multiple entities in RDF graphs. Applied Computing and Intelligence, 2024, 4(1): 1-18. doi: 10.3934/aci.2024001 |
[5] | Henri Tiittanen, Liisa Holm, Petri Törönen . Novel split quality measures for stratified multilabel cross validation with application to large and sparse gene ontology datasets. Applied Computing and Intelligence, 2022, 2(1): 49-62. doi: 10.3934/aci.2022003 |
[6] | Xian Wen Sim, Sie Long Kek, Sy Yi Sim . State estimation and optimal control of an inverted pendulum on a cart system with stochastic approximation approach. Applied Computing and Intelligence, 2023, 3(1): 79-92. doi: 10.3934/aci.2023005 |
[7] | Muhammad Amir Shafiq, Zhiling Long, Haibin Di, Ghassan AlRegib . A novel attention model for salient structure detection in seismic volumes. Applied Computing and Intelligence, 2021, 1(1): 31-45. doi: 10.3934/aci.2021002 |
[8] | Marko Niemelä, Mikaela von Bonsdorff, Sami Äyrämö, Tommi Kärkkäinen . Classification of dementia from spoken speech using feature selection and the bag of acoustic words model. Applied Computing and Intelligence, 2024, 4(1): 45-65. doi: 10.3934/aci.2024004 |
[9] | Isaiah Kiprono Mutai, Kristof Van Laerhoven, Nancy Wangechi Karuri, Robert Kimutai Tewo . Using multiple linear regression for biochemical oxygen demand prediction in water. Applied Computing and Intelligence, 2024, 4(2): 125-137. doi: 10.3934/aci.2024008 |
[10] | Pasi Fränti, Sami Sieranoja . Clustering accuracy. Applied Computing and Intelligence, 2024, 4(1): 24-44. doi: 10.3934/aci.2024003 |
From the theory of algorithms, we know that the time complexity of finding the optimal solution for a traveling salesman problem (TSP) grows exponentially with the number of targets. However, the size of the problem instance is not the only factor that affects its difficulty. In this paper, we review existing measures to estimate the difficulty of a problem instance. We also introduce MST branches and two other measures called greedy path and greedy gap. The idea of MST branches is to generate minimum spanning tree (MST) and then calculate the number of branches in the tree. A branch is a target, which is connected to at least two other targets. We perform an extensive comparison of 11 measures to see how well they correlate to human and computer performance. We evaluate the measures based on time complexity, prediction capability, suitability, and practicality. The results show that while the MST branches measure is simple, fast to compute, and does not need to have the optimal solution as a reference unlike many other measures. It correlates equally good or even better than the best of the previous measures ‑ the number of targets, and the number of targets on the convex hull.
Traveling salesman problem (TSP) aims to find a tour that visits all targets and then returns to the origin. We refer to this as the closed loop variant of TSP. The less studied open loop variant excludes the return to the origin. Solving TSP is challenging for both computers and humans. Both open and closed loop variants are shown to be NP hard [1], and consequently, exponential time consuming for computers. Therefore, computers can efficiently solve only small-size instances.
Orienteering is a popular outdoor sport where the goal is to visit targets in nature. A variant of orienteering is rogaining [2] where the goal is to visit as many targets as possible within a given time limit. Another variant is a mobile orienteering game called Mopsi orienteering, O-Mopsi [3], where players navigate using smart phone positioning. Their difference to classical orienteering is there is no pre-defined order of visiting the targets. The game playing implicitly includes the open-loop traveling salesman problem.
Although the sizes of the O-Mopsi game instances are small (N = 4 to 27), only 18% of the players managed to find the optimal order while playing the game instances [4]. Players very often settle into simpler heuristics like the nearest neighbor strategy or some randomized strategy, which rarely results in the optimal solution. This indicates that even small size instances can be difficult for human players. However, some problem instances appear to be significantly more difficult than other instances.
Human problem-solving skills have also been studied in the literature. Solving TSP instances is like solving a puzzle that requires visual-spatial abilities [5,6]. From the algorithm theory, we know that the time complexity of finding the optimal solution is exponential with the number of targets. However, results from the literature have also shown that human efficiency reduces linearly or near-linearly with the increasing problem size [7,8]. The difference is that these papers measure how close the human solution was to the optimal, not how much effort they need to find the exact optimal tour.
It was concluded both in [9] and in [10] that size is not the only factor that affects the difficulty of a problem instance. Three O-Mopsi game instances shown in Fig. 1 have almost the same number of targets. Despite this, the first example (Otsola) is significantly easier to solve than the other two because the targets have an almost linear structure that is easy to follow from north to south. The second example (Hukanhauta 3 km) is somewhat more difficult, but the third example (Christmas Star) is the most difficult among these for humans to solve.
An open question is how to estimate the difficulty of a given TSP problem instance for humans and algorithms to solve. Exact solvers require a considerable amount of time and heuristics often produce only sub-optimal results. The number of targets is clearly one affecting factor but there are other factors.
It was claimed in [11] that the structure of the instance is very important to measure its complexity. They analyzed the behavior of two algorithms: one exact solver – Concorde [12] – and one heuristic – Helsgaun's implementation of Lin-Kernighan's algorithm [13]. They recognized that structured instances (grid structure and fractal curve) require less computational time to solve by the algorithms than random targets.
In [14] it was claimed that meticulous statistical methods can efficiently estimate the execution time of an algorithm for computationally hard problems. They surveyed the performance of such a statistical model called empirical hardness model for different types of combinatorial hard problems including TSP.
The difficulty of the TSP instance was measured in [15] using ant colony optimization algorithm without local search and compared the cases whether the targets are clustered or distributed randomly. They represented a TSP instance as a network structure using the so-called LRNet algorithm [16]. They found out that clustered instances are more difficult to solve than non-structured instances.
We have selected 8 measures from the existing literature and introduce 3 new measures of our own for detailed comparative testing. The measures are:
● Problem size (N) [1]
● Number of targets on the convex hull (H) [9]
● Convexity (C) [17]
● Number of indentations (I) [18]
● Number of intersections (X) [19]
● Regularity of targets (R) [6]
● Path complexity (PC) [5]
● Circularity (O) [17]
● MST branches (M) (new)
● Greedy path (GP) (new)
● Greedy gap (GG) (new)
To estimate the usefulness of the measures, we evaluate their performance using the following four criteria, which all are relevant to practitioners:
● Prediction capability
● Need to have the optimal solution
● Processing time
● Simplicity of the implementation
The prediction capability estimates how well the measure correlates to human performance or computer processing time. A good measure should also work in real life situations outside the laboratory environment.
A major bottleneck of several existing measures is that they need to have the optimal solution as a reference. This is acceptable for problem instances with known optimum, or small problems, for which the optimum can be solved in a reasonable time. However, computing the optimal solution can be too slow for real-time situations and can be a severe restriction on the practical usefulness of such measures.
The measure should also be computable and simple to implement. A reasonable amount of time can be allowed for the implementation and running, but huge implementation complexity tends to decrease the willingness of practitioners to adopt the measure. Fortunately, most of the existing measures are fast to compute and very simple to implement, and therefore, meet these two criteria.
Minimum spanning tree (MST) is a closely related graph problem to TSP. Its goal is to connect all the targets using a tree structure by minimizing the total distance of the selected connections. MST is significantly easier to solve than the TSP. Kruskal [20] and Prim [21] are two well-known examples of greedy algorithms to find the optimal solution. With sophisticated data structures like union-heap, they require only about O(N2) time. This is several orders of magnitude faster than the exponential O(2N) time required to find the optimal solution.
From the algorithmic perspective, while TSP and MST problems are at completely different complexity levels, they share a lot of common. Specifically, algorithms for the easier MST problems have been utilized for developing algorithms for the more difficult TSP problem. For example, the classical Christofides algorithm [22] starts from the minimum spanning tree. Creating additional links allows us to generate an approximation TSP tour in polynomial time. A greedy heuristic called Insertion algorithm as introduced in [23]. It corresponds to Prim's algorithm, while [24] showed how Kruskal's algorithm can also be modified to create a solution for the openloop TSP. It is also possible to convert MST to TSP path by a series of link swaps [25]. MST was utilized in [26] to provide an estimation of the lower limit for the TSP tour length.
From the human problem-solving perspective, MST and TSP also share a lot of common features. It was shown in [5] that human performance in solving MST and TSP correlates highly (0.66). The common ability to solve these two problems is to perceive the visual structure. In [6] it was shown that human solutions for both MST and TSP are affected by the spatial distribution of the targets. For both problems, human performance degrades gradually when the problem instance changes from highly clustered to random and regular instances. O-Mopsi players were recognized to often settle into a simple nearest neighbor strategy in [27], which is very close to the greedy strategy used for creating the minimum spanning tree. All these evidences indicate that MST and TSP are closely related problems in the context of human problem solving.
In this paper, apart from the review of the existing methods, we also propose a method based on the MST solution to estimate the difficulty of a TSP problem instance. We first note that the solution for an open-loop TSP is also a spanning tree, although not necessarily the minimum one. The idea here is to count the number of branch targets in the minimum spanning tree. A branch is a target that connects with two or more other targets. A spanning tree can have branches while TSP cannot. We hypothesize that the more there are branches in the minimum spanning tree, the more difficult it is to find the optimal TSP solution for the corresponding problem instance.
Fig. 1 shows three problem instances and their corresponding MST solutions. The first case (Otsola) is also optimal for TSP because there are no branches. The second case (Hukanhauta 3 km) has one branch; the solution is close to the optimal TSP path. The third case (Christmas star) contains three branches, and it is the most difficult among these three. It is not obvious how the MST solution could help to find the TSP solution.
We evaluate the methods how well they correlate to human performance. We measure human performance in two alternative ways. First, we study how close humans can reach the optimal when playing O-Mopsi games in the real-world environment. Second, we study how fast humans can find exactly the optimal solution using a computer interface. The results show that MST branches has as good predicting capability as the best existing measures and is better when compared against human performance. For computer processing time, the number of targets (N) is a slightly better predictor than MST branches, but only by a small margin.
The rest of the paper is organized as follows. In Section 2, we review the measures and the related results found in the literature. In Section 3, we define our evaluation methodology and then provide experimental results in Section 4. Conclusions are drawn in Section 5.
Several researchers have studied the complexity of the TSP instances to estimate the difficulty either to a human or to a computer algorithm. Next, we review the following existing measures and add three others that have not been considered before:
● Problem size (N) [1]
● Number of targets on the convex hull (H) [9]
● Convexity (C) [17]
● Number of indentations (I) [18]
● Number of intersections (X) [19]
● Regularity of targets (R) [6]
● Path complexity (PC) [5]
● Circularity (O) [17]
● Number of MST branches (M) (new)
● Greedy path (GP) (new)
● Greedy gap (GG) (new)
Papadimitriou showed in [1] that both the Euclidean closed- and open-loop TSPs are NP hard problems. This means that the time for finding the optimal solution by computer grows exponentially with the problem size. Thereby, the size of the problem is the most obvious measure.
A convex hull is the shortest enclosing polygon of a given point set in the plane. MacGregor and Ormerod [9] collected TSP solving data from volunteer participants. They calculated the percentage of extra path length over optimal (PAO) for those data. We refer to this as gap. They also compared the solutions of all participants for each instance. They calculated the frequency of all existing links for all different solutions the participants chose for a problem instance. Then they calculated the response uncertainty using Shannon entropy from those frequency values. From these two measures, they showed that the number of targets located on the convex hull affects human performance. They first demonstrated that human performance is better for an instance with more hull targets. Similar results were later obtained in [28].
Although the measure was originally designed for closed-loop cases, it does apply (to a certain extent) to open-loop cases as well. Simply by removing the longest link from the hull we can have a relevant reference path for the open-loop case. The difference of removing one link can be significant with small instances but is negligible with large-scale instances [29]. Chronicle et al. [10] studied human performance in solving the open-loop instance, and based on the gap and response uncertainty, they concluded that human performance in solving the open-loop TSP improves when there are fewer points on the hull.
To count the number of targets on the convex hull, we first calculate the convex hull using Monotone chain algorithm by [30] and then divide the number of targets on the convex hull by the total number of targets:
P=CHN.100% | (1) |
Here P is the relative number of targets on the convex hull (%), CH is the total number of targets on the hull, and N is the problem size. Examples of the calculations are shown in Fig. 2.
Ormerod and Chronicle [31] showed that the optimal solution of a TSP is more attractive to a human than a sub-optimal solution. Vickers et al. [17] showed that convexity is a better measure of attractiveness than the number of targets on the convex hull based on a survey of volunteer participants. Convexity is based on the convex hull. It estimates how well the optimal solution resembles the convex hull polygon by measuring the area of the polygon (ATSP) created by the optimal TSP tour, relative to the area of the convex hull of the problem instance (ACH). The measure is calculated as the ratio of these two:
Convexity=ATSPACH | (2) |
Dry and Fontaine [32] experimented on the convexity measure but with their dataset, convexity did not predict the difficulty well. They studied closed problem instances. In the open-loop case, the optimal solution is a path not a tour. To be able to use the measure in our case, we first connect the terminal nodes of the path to create a polygon of the optimal TSP path, see Fig. 3.
The optimal solution for the closed-loop TSP is always a polygon that does not cross itself. We can calculate the area of such a simple polygon by Surveyor's area formula [33]. In contrast, polygons created from the open-loop TSP solutions can be self-intersecting, see Fig. 3. For this reason, we need to divide such a polygon into non-intersecting sub-polygons based on the intersecting targets before applying the Surveyor's formula. The last two examples of Fig. 3 have one intersecting point in each.
Another issue with the convexity measure is that we need to calculate the optimal TSP solution before being able to apply the measure. We use Concorde solver [34]. However, the Concorde solver is designed for closed-loop cases only. As explained in [1], and later shown in practice in [27], we add a phantom node to the open-loop instance and solve the closed-loop problem using Concorde. Thereafter, we remove the phantom node from the solution to recover the open-loop solution.
This measure counts the number of times the optimal solution deviates from the convex hull (see Fig. 4). MacGregor [18] showed that human solutions contain fewer indentations than the optimal solutions. Hence, it was speculated in [32] that the optimal solutions, which have lesser indentations, are easier to solve by humans. Their experimental results showed evidence to support this hypothesis by showing that human solutions of instances with higher indentations deviated significantly from the optimal compared to instances with fewer indentations. From this conclusion, we can assume that an instance is easier to solve when the optimal tour follows the convex hull more closely.
Quintas and Supnick [35] proved that the optimal solution of a Euclidean TSP does not intersect itself. Van Rooij et al. [36] showed that humans also prefer to avoid intersections in their solutions. Vickers et al. [19] first proposed that the total number of intersecting links of the complete graph of a TSP instance could predict human performance in solving that instance. They measured the gap and response uncertainty for the results obtained from volunteer participants and showed that with increasing intersections, the instances become easier for humans.
The idea of this measure is to generate a complete graph by connecting all the targets and then counting how many links intersect each other. Fig. 5 shows how the number of intersections varies with the difficulty of the instance.
Clustered problem instances are easier to solve by humans than randomly distributed targets as we can solve the clusters separately as smaller sub-problems and combine the overall solutions from the sub-solutions. This kind of divide-and-conquer approach is also commonly used in computer algorithms [37], especially in the case of large-scale instances [29]. In general, divide-and-conquer algorithms are significantly faster than brute force or exhaustive search.
Dry et al. [6] introduced a measure called regularity (R), which estimates the difficulty based on the level of the cluster of the data. According to the gap, response uncertainty and solution time obtained in their experiment, human performs better when targets are highly clustered than randomly distributed both in the TSP and MST problem. They also showed that human provides better solutions for random targets than highly regular targets. MacGregor [38] extended this study and showed that the cluster location of a highly clustered instance also matters. For a highly clustered instance, when the cluster locates near the border of the instance layout, humans solve that instance easily. If the cluster is closer to the center of the layout, the problem instance is more difficult (gap increases).
The measure is based on the distances of each target to its nearest neighbor. The average of all nearest neighbor distances, observed distance (ro), is then compared against the expected distance (re) of randomly distributed data. The measure is defined as the ratio between these two:
R=rore | (3) |
where,
ro=1N∗∑Ni≠jmin(dij) and re=0.5∗√A╱N |
Here N is the problem size, dij is the distance between ith and jth targets, and A is the area of the bounding box of the instance.
However, the nearest neighbor distances do not solely explain the clustering property of the instance. High R value can also be caused by density variations or spatial layout of the targets even without any clusters. Instead of R, similar (or better) results would probably be obtained by calculating the standard deviation of the distances normalized by the size of the bounding box. Nevertheless, the measure provides some clues about the difficulty of the instance as can be seen by the examples in Fig. 6. Smaller values of R demonstrate higher distance variations, which are often caused by clusters that reduce the difficulty of the instance.
Path complexity is another measure calculated based on the nearest neighbor distances. Every link in the optimal path is analyzed by calculating the nearest neighbors (NN) of the source node. The traveled link is scored by 1 if it was the 1st nearest neighbor, 2 if it was the 2nd nearest and so on. In other words, the score is the rank of the link when all links are sorted according to their lengths. The measure is then the average of the individual rank scores (see Fig. 7). If the optimal path always follows the shortest link, the resulting path corresponds to a greedy strategy and gives the value 1.
Vickers et al. [5] claimed that path complexity could predict human performance on TSP. They experimented with over 69 volunteer participants and found that higher path complexity values increase the gap value, and thus, increase the difficulties of the problem instances. Dry and Fontaine [32] experimented with the path complexity with human performance by measuring the choice probability and choice time but did not find a significant correlation.
We also note that the order of travel can produce different results since the nearest neighbor function is not symmetric. We, therefore, calculate the complexity value for both directions and use their average as the path complexity value (Fig. 7). The difference between the two directions is usually insignificant but the averaging can balance the measure in case of significant asymmetry. Human solvers are not expected to follow the optimal path anyway; they have a hard time even for finding the best start point [27].
Circularity was introduced by Vickers et al. [17] to calculate how similar the shape of the optimal solution is to a circle. They claimed that circularity correlates to the attractiveness of the optimal TSP solution based on a survey of several volunteer participants. The more circular the solution, the easier it is to solve. However, the results in [32] did not show a significant correlation with the circularity.
The idea is to use the length (L) of the optimal tour and then draw a circle that has a circumference of length L. The area surrounded by the optimal tour is then divided by the area of the circle. The closer the ratio is to 1 the more accurately the tour corresponds to the circle; and vice versa, the more complex is the shape, the smaller is the area it surrounds compared to the area that can be obtained by a perfect circle with the circumference.
The circularity is derived from the radius r, the circumference d, and the area A:
d=2πr⟺r=d2π |
A=πr2=d24π |
Fixing d = L, we can calculate the circularity as follows:
Circularity=4πAL2 | (4) |
In literature of mathematics, the measure is known as 2-D compactness [39,40]. Fig. 8 shows a few examples of how the measure works. We can see that the measure has some relationship with the complexity of the instance. We also note that the measure was defined for the closed-loop TSP. We use the same strategy for open-loop instances as with the convexity measure.
Next, we present a new measure based on the relationship between the greedy path and the optimal path. First, we generate a greedy path by always traveling to the nearest unvisited target. We consider every node as a possible start node, and thus, obtain N different greedy solutions. We then compare the greedy paths with the optimal path, see Fig. 9. We count the number of links in each greedy path that are absent in the optimal path. We call these mismatch links. The greedy path measure is the average number of mismatches. The bigger the value, the more difficult the instance.
The greedy path measure considers merely the structure of the paths, which is expected to correlate to human performance on finding the exact optimal tour. However, some measures aim at estimating the difficulty of how close humans get to the optimal. For this reason, we consider an alternative variant called the greedy gap.
The measure is otherwise the same as the greedy path with one exception. Instead of counting the number of mismatches, we calculate the relative difference of the greedy and optimal path lengths:
GreedyGap=GL−LGL=1−LGL | (5) |
Here L is the length of the optimal path, and GL is the average length of the greedy paths. The bigger the ratio, the worse is the greedy path and the more difficult is the problem instance. Fig. 10 shows an example of the calculation.
Minimum spanning tree (MST) is a tree that connects all the targets so that the sum of the connections is minimum. The solution for an open-loop TSP is also a spanning tree, although not necessarily the minimum one. Fig. 11 shows two problem instances and their corresponding MST and TSP solutions. In the case of the first instance, both solutions have the same connections. In the case of the second instance, solutions still resemble each other but there are two connections in the TSP that have been replaced by shorter ones in the MST solution. Nevertheless, the relationship between the MST and TSP is clear.
We define a branch as a node in the MST that has three or more links associated with it. Fig. 12 shows three examples with one, two and three branches, respectively. A preliminary version of this measure was introduced in [4] with the name MST knots.
Every branch divides the spanning tree into sub-trees, called branches. Every branch makes the creation of a TSP path more difficult. The number of branches varies from 0 to N/2-1. A special case is a minimum spanning tree without any branches, which is also the optimal solution for the open-loop TSP because |MST| ≤ |TSP|. In general, we hypothesize that the more branches there are, the more difficult it is to solve TSP.
We measure the number of MST branches as follows:
● We create MST by any algorithm (Prim or Kruskal)
● While adding new links, we update the counts
● If a count exceeds 2, we mark the node as a branch
● Lastly, we count the total number of branches
We summarize the four properties introduced in Section 1 for the measures. The properties are speed, simplicity of the implementation, need for the ground truth, and prediction capability. The first three are stated in Table 1. We also mark if the measure is well suitable for the openloop case.
Measure: |
Properties: | |||
Time complexity | Needs optimal | Simple | Suitable for open-loop | |
N | O(1) | - | Yes | Yes |
H | O(N*logN) | - | Yes | Yes |
C | O(N2) | Yes | - | - |
I | O(N*logN) | Yes | Yes | Yes |
X | O(N4) | - | Yes | Yes |
R | O(N2) | - | Yes | Yes |
PC | O(N2) | Yes | Yes | Yes |
O | O(N) | Yes | - | - |
GP | O(N3) | Yes | Yes | Yes |
GG | O(N2) | Yes | Yes | Yes |
M | O(N2) | - | Yes | Yes |
Most of the measures are simple to implement. Only convexity and circularity require the calculation of the area surrounded by the TSP tour, and these two measures do not generalize to the open-loop case as such. The measures based on the convex hull polygon (targets on the hull, indentions) require a non-trivial algorithm for calculating the convex hull. However, there are plenty of easy-to-use packages for this. Therefore, we count them as easy to implement.
Some measures have high time complexity and six measures require having the optimal tour, which can take exponential time. This is a serious limitation. Among the others, targets on hull and indentations require O(N*logN) time while regularity and MST branches take quadratic, O(N2) time.
To measure the performance of the measures, we compare how well they correlate to human performance and computer execution time. We use the following four evaluation methods:
● Mistakes by a human
● Time taken by a human
● Time taken by the Concorde algorithm [34]
● Operations taken by a local search algorithm [41]
We analyze the tours constructed by humans when playing OMopsi games in the real-world. The game itself sets no requirement to find the optimal TSP solution; any path is acceptable. However, since the performance is measured by the playing time; players naturally try to minimize the distance they need to travel. To measure human performance, we consider the following three measures:
● Number of mismatches
● Number of mistakes
● Gap to optimal
For the mismatch and mistake, we do not care about the length of the path for visiting the targets. Instead, we analyze the order in which the targets were visited against the corresponding order of the optimal path. The number of differences between the two paths defines human performance.
We denote a link as a pair of two consequent targets in the path. The mismatch is defined as the number of links in the optimal tour that are missed in the player's path. An example is shown in Fig. 13 where the player's path is the same as the optimal with two exceptions.
The problem of the mismatch is it cumulates the faults. Even a single different choice will cause another mismatch later in the path. In Fig. 13, the player reversed the optimal visiting order of the 2nd and 3rd target. Therefore, the measure penalizes this single mistake twice.
The idea is to count every mistake only once and update the optimal tour dynamically after every mistake. Otherwise, the measure works exactly as the mismatch: we count the number of links in the optimal tour that were missed by the player. After every mistake, we resolve a new optimal solution for the remaining unvisited nodes starting from the current node. Fig. 14 shows the same example as in Fig. 13 when the optimal path is updated after every mistake.
Some mistakes are more significant than others. For example, the mistakes made in Fig. 15 causes only a minor increase in the path length while other mistakes can have more dramatic effects. Therefore, it can be more meaningful to measure the effect of the choices rather than their count. Hence, we also consider the concept called the gap.
We measure the gap as the relative difference of the lengths of the optimal path (TSP) and the path generated from the player's choices (path):
gap=|path|−|TSP||TSP| | (6) |
The gap is also known as path length over optimal (PAO) in literature. We note that we do not use the real path traveled by the player. Instead, we generate an artificial tour using the order of the targets chosen by the player. In this way, we eliminate the effect of GPS errors and navigational skills of the player, and measure merely the TSP problem-solving skill.
The distance of the path is measured using Euclidean distance between the targets. In some cases, routing along the street network might provide a more accurate measurement. However, many of the O-Mopsi games appear in parks and campus areas, where using only the street network would be too restrictive because players can easily make shortcuts. According to [27], Euclidean distance correlates slightly better (0.95) to reality than routing via the open street map (0.93).
Fig. 15 shows two example paths. There is only one mistake in the first example, but its effect is relatively large making the overall path 14% longer than the optimal path. In the second example, the player made four mistakes and yet the path is only 13% longer than the optimal path.
We evaluate human performance by measuring how long it took to find the optimal solution. This can be significantly more challenging than just find a 'good' solution. For this, we presented problem instances to participants on the computer screen. The task was to create paths using an easy-to-use web interface, which not only allows to create a new path but also to modify existing ones by dragging and by mouse clicks. We then measured the time it took the participant to find the optimal solution.
In these computer simulations, the gap of the player's current solution to the optimal was shown on screen in real-time. This guides the player towards finding the optimal solutions, which might otherwise be too hard with some more difficult problem instances. The optimal tour was generated online by the local search algorithm in [41] as the instances were needed in real-time. The algorithm does not guarantee the optimality of the solution always. We later verified offline that they were optimal using the Concorde solver [34].
Our third ground truth is to measure the execution time for the computer to find the optimal solution. We use the Concorde algorithm, which is one of the fastest TSP solvers for large problem instances [34]. In theory, running time should grow exponentially with the problem size. In practice, the performance depends also on other factors than the number of nodes. In specific, we expect that more difficult game instances take considerably more time than easier ones with the same number of targets.
Apart from the exact solver, we also study the complexity with respect to a simple local search heuristic proposed in [14] for O-Mopsi and Dots games. It uses two parameters called iteration and repetition so that their product corresponds to the total number of trial solutions generated. Easier instances need fewer trials to achieve the optimal solution compared to more difficult instances. The number of trial solutions generated can therefore define the difficulty of the instance.
Table 2 summarizes the evaluation methods used in the literature. Most works have calculated correlation between the gap of solutions generated by human solvers to the optimum. Some researchers have also used the time for humans taken to find the optimal solution [7,8]. A few researchers have also used methods that we do not consider here. These include response uncertainty, solved-% and choice time.
Measure | Studied | Methods | Conclusions |
N | Graham et al. (2000) [7] Dry et al. (2006) [8] |
Human time | Linear or near-linear growth |
H | MacGregor & Ormerod (1996) [9] | Gap, Response uncertainty | More targets easier |
Macgregor et al. (2004) [28] | Gap | More targets easier | |
Vickers et al. (2003) [19] | Gap, Response uncertainty | Fewer targets easier | |
Chronicle et al. (2006) [10] | Gap, Solved-% | More targets easier | |
Dry and Fontaine (2014) [32] | Choice time, Choice probabilities | Fewer targets easier | |
C | Dry and Fontaine (2014) [32] | Choice time, Choice probabilities | Does not matter |
I | MacGregor (2012) [18] | Gap, Solved-% | Fewer indentations easier |
Dry and Fontaine (2014) [32] | Choice time, Choice probabilities | Fewer indentations easier | |
X | Vickers et al. (2003) [19] | Gap, Response uncertainty | More intersections easier |
Dry and Fontaine (2014) [32] | Choice time, Choice probabilities | More intersections easier | |
R | Dry et al. (2012) [6] | Gap, Response uncertainty, Solving time | Clustered easier |
MacGregor (2013) [38] | Gap | Cluster locations is important | |
PC | Vickers et al. (2004) [5] | Gap | Lower value easier |
O | Vickers et al. (2006) [17] | Survey | Circularity more attractive |
Dry and Fontaine [32] introduced a methodology where participants were shown several problem instances and asked to choose the ones they want to solve. The hypothesis is that humans are more likely to choose easier problems. Two counts were then used: how much time a participant spent to make the choice (choice time) and how frequently an instance was chosen (choice probability). Several researchers [10,18] also counted how many times the optimal solution was found (solved) for a given problem instance.
The main results of the previous works are summarized in Table 2. Most results clearly show either an increasing or decreasing the correlation of a given measure to the difficulty of the problem instance. Some methodologies give only indirect evidence. For example, [32] measured human preference in choosing the instances. While it is expected that easier instances are chosen more often, the results also showed that humans preferred instances with fewer targets on the convex hull. Vickers et al. [17] also reported the attractiveness of the instances based on a survey.
We use O-Mopsi and Dots datasets presented in [41]. They are publicly available on the web1, and their statistics are summarized in Table 3 and Fig.16. The problem sizes vary from N = 4 to 50, being 13 on average. Both O-Mopsi and Dots datasets are used for measuring human performance (accuracy and speed), and processing time required by the computer.
1 http://cs.uef.fi/o-mopsi/datasets/
Dataset | Type | Distance | Instances | Sizes |
O-Mopsi | Openloop | Haversine | 147 | 4−27 |
Dots | Openloop | Euclidean | 12124 | 4−50 |
In total, O-Mopsi has 147 game instances available, of which 55 have been completed by at least one player. Most playing records were collected during the SciFest Annual Science festivals in 2011−2018 [42]. At least one new game instance was created every year and played by elementary school kids, usually without any previous knowledge of the game. These 14 game instances produced 153 playing records in total. The remaining 99 playing records were produced by individuals who played game instances they happened to like. Many of these players had a competitive attitude aiming at outperforming the time of the others. In total, 252 playing records were collected.
Dots instances are randomly generated points on the computer screen [41]. A game-like web application was created with an easy-to-use interface that allows players to connect the dots and modify the existing path by clicking and dragging links. The gap value of the current path was visible when all dots were connected, and the game ended once it reached 0% value. The total time taken was recorded. The players were computer science researchers or students who knew TSP and enjoyed puzzle solving. In total, 12,244 playing records were collected.
O-Mopsi data is used to measure human performance in terms of how close they reached the optimal result. Three measures are used: gap, mismatch, mistakes. Dots data is used to measure how long (time) it took for humans to find the optimal solution. The time varied hugely. In this study, we excluded cases when playing time exceeded 5 minutes; we noticed that players sometimes left the game idle and continued it later.
We test all the measures presented in Section 2. In addition, we also calculate normalized values for three measures: intersections, indentations, and MST branches because these three measures have a clear dependency on the problem size. We normalize intersections by dividing the original value by N2, and the other two by dividing by N. The hypothesis is that these measures might perform well merely because of the dependency on the problem size, which is the mediating factor. If correlation remains also after normalization, then they carry additional information.
As per our hypotheses, human mistake, human time, and algorithm time all are expected to correlate with the measures. From Table 4, we can observe that most of the measures correlate highly with all the ground truths except regularity, circularity, and greedy gap. Circularity and regularity could be useful measures for the closed-loop case but not much for the open-loop case.
Human performance | Computer processing time | ||||||
O-Mopsi | Dots | O-Mopsi | Dots | ||||
Human mistake | Human gap | Human time | Concorde time | LS time | Concorde time | LS time | |
N | 0.45 | 0.06 | 0.38 | 0.75 | 0.66 | 0.61 | 0.63 |
H | 0.48 | 0.12 | 0.30 | 0.67 | 0.52 | 0.50 | 0.42 |
C | 0.39 | 0.02 | 0.22 | 0.50 | 0.39 | 0.35 | 0.29 |
I | 0.28 | 0.05 | 0.30 | 0.52 | 0.48 | 0.51 | 0.43 |
I (normalized) | 0.02 | 0.04 | 0.01 | 0.01 | 0.01 | 0.10 | 0.03 |
X | 0.40 | 0.01 | 0.39 | 0.72 | 0.72 | 0.62 | 0.75 |
X (normalized) | 0.49 | 0.13 | 0.29 | 0.70 | 0.44 | 0.49 | 0.35 |
R | 0.22 | 0.06 | 0.01 | 0.45 | 0.37 | 0.05 | 0.08 |
PC | 0.48 | 0.14 | 0.03 | 0.48 | 0.23 | 0.06 | 0.06 |
O | 0.43 | 0.11 | 0.29 | 0.51 | 0.37 | 0.47 | 0.37 |
GP | 0.53 | 0.16 | 0.35 | 0.68 | 0.50 | 0.58 | 0.64 |
GG | 0.02 | 0.08 | 0.15 | 0.24 | 0.21 | 0.25 | 0.22 |
M | 0.53 | 0.15 | 0.35 | 0.69 | 0.55 | 0.56 | 0.60 |
M (normalized) | 0.44 | 0.11 | 0.13 | 0.48 | 0.30 | 0.22 | 0.18 |
Legends: Problem size (N), Targets on the convex hull (H), Convexity (C), Indentations (I), Intersections (X), Regularity (R), Path complexity (PC), Circularity (O), MST branches (M), Greedy path (GP), Greedy gap (GG). |
All measures are capable to predict human mistakes well. MST branches and greedy path result in the highest correlation (0.53) while targets on the hull (0.49), normalized intersections (0.49), path complexity (0.48), and problem size (0.46) also perform well. When compared to the time taken to find the optimal solution with the Dots instances, the same measures also correlate well based on the ranks in Table 5. However, intersections (0.39) has now the highest correlation before problem size (0.38).
Human performance | Computer processing time | Mean | ||||||
O-Mopsi | Dots | O-Mopsi | Dots | |||||
Human mistake | Human gap | Human time | Concorde time | LS time | Concorde time | LS time | ||
N | 3 | 8 | 2 | 1 | 2 | 2 | 3 | 3.00 |
H | 2 | 5 | 4 | 6 | 4 | 6 | 6 | 4.71 |
C | 7 | 11 | 6 | 9 | 8 | 9 | 9 | 8.43 |
I | 8 | 10 | 4 | 7 | 6 | 5 | 5 | 6.43 |
I (normalized) | 10 | 10 | 10 | 13 | 13 | 12 | 14 | 11.71 |
X | 6 | 12 | 1 | 2 | 1 | 1 | 1 | 3.43 |
X (normalized) | 2 | 4 | 5 | 3 | 7 | 7 | 8 | 5.14 |
R | 9 | 9 | 10 | 11 | 9 | 14 | 12 | 10.57 |
PC | 2 | 3 | 9 | 10 | 11 | 13 | 13 | 8.71 |
O | 5 | 6 | 5 | 8 | 9 | 8 | 7 | 6.86 |
GP | 1 | 1 | 3 | 5 | 5 | 3 | 2 | 2.86 |
GG | 10 | 7 | 7 | 12 | 12 | 10 | 10 | 9.71 |
M | 1 | 2 | 3 | 4 | 3 | 4 | 4 | 3.00 |
M (normalized) | 4 | 6 | 8 | 10 | 10 | 11 | 11 | 8.57 |
There is one notable difference in human performance. Path complexity is calculated using the nearestneighbor values along the optimal path, which correlates well with the human mistake (0.48) because the measure matches well with the strategy of most human players to go to the nearesttarget rather than using a more sophisticated tour optimization. Yet, the path complexity does not correlate (0.04) to the time taken to find the optimal solution.
Another general observation is that gap in the human performance does not correlate well to any of the measures. There are two possible explanations for this. The possible reason is that the other factors in playing the O-Mopsi games in the real environment affect so much more that they overwhelm the effect of the difficulty of the instance. In specific, the human players were not aware that they are indirectly solving TSP but merely aimed at visiting the targets while the navigation and observing the environment took most of their attention.
The correlation values with computer processing time are more consistent. Most measures have a high correlation to all ground truths. The correlation values of the Concorde time and the LS time are almost equal for all the cases. Based on the rankings, problem size (2, 2, 2, 3) and intersections (3, 1, 1, 1) are the best predictors, which is understandable because of the known exponential dependency of the time complexity to the size of the problems. The intersections also have quadratic relation to the problem size; for N targets there are O(N2) connections, and by default, the more connections, the more intersections.
The few notable differences are path complexity and regularity. Dots instances are computer generated with random distribution and have less regularity than humangenerated O-Mopsi games. These instances have smaller variations on the regularity. The processing time for a computer can barely predict such measures, which are based on factors that are less apparent in the data.
Fig. 17 shows the distribution of human performance evaluations (mistakes and time) and computer processing time. Selected O-Mopsi examples are also shown visually in Fig. 18. Next, we summarize how well our observations confirm (or contradict) those found in the literature.
First, human mistakes increase with the problem size, which supports the results by [7,8]. However, even if we also found a linear correlation, the amount of data is too small and the variation too large to conclude that the correlation is indeed linear and not some higher polynomial or even exponential. In the case of human time, we confirm the linear correlation (0.38) with the regression line f = 1.4N–3.2. Although a polynomial regression line f = 0.03N2 + 0.19N + 4.9 seems a better fit.
However, according to the theory of algorithms, the problem-solving time increases exponentially with the problem size, and humans are not expected to perform any better than the best possible computer algorithms. If we increase the problem size from 100 to 1000, much steeper than linear growth in human time is expected to appear. However, it would become overwhelming for humans to test this in practice. Linear or near-linear dependency has been reported by other researchers, and probably exists, but only with small problem instances, or using accuracy (gap) to measure human performance. When measuring time to find the optimal solution we still expect a linear dependency only with small instances. There is a clear difference in the difficulty of finding a very good sub-optimal solution than to find the exact optimal solution.
Second, human mistake and time decrease with the increasing number of targets on the convex hull. Hence, the more targets on the convex hull polygon, the more similar the optimal solution is to the convex hull polygon, and the easier the problem is to solve. These observations support the results of [9,10,28,43,44].
Third, the more convex is the optimal solution, humans make fewer mistakes. Although the correlation is not as strong as with several other measures; it is a new observation. A correlation with attractiveness was reported in [17] while [32] did not find any remarkable correlation with the difficulty of the problem instance.
Human mistakes and time also increase with the number of indentations. This supports [18] who made the same observation when evaluated by the gap and the number of problems solved. They reasoned humans prefer fewer indentations in their solutions than what the optimal solution requires. However, when the measure was normalized by the problem size, all correlations disappear completely. It seems that the mediating factor is merely the problem size.
The number of intersections was one of the measures with the strongest correlation to the difficulty of the problem. This is in direct accordance with the results by [19] and [32]. Even after normalization, the correlation stays relatively strong.
Regularity had only a mild correlation when used the measure by [6]. One reason is that our data did not include many clusters. Another reason is that the proposed regularity measures more the variation of the distances rather than randomness or the level of cluster of the data. We think it would make more sense to derive a measure from some clustering validity index such as WB-index [45] instead.
Among the other observations, we found that path complexity and circularity correlate with human mistakes. These observations support the results by [5,17].
Cross-correlations between the measures are reported in Table 6. We can see there is a high correlation between most of them. For example, the problem size and intersections measure essentially the same thing. The greedy gap deviates most having maximum cross-correlations of 0.50 with targets on hull and convexity.
N | H | C | I | X | R | PC | O | GP | GG | M | |
N | 1.00 | ||||||||||
H | 0.72 | 1.00 | |||||||||
C | 0.51 | 0.66 | 1.00 | ||||||||
I | 0.67 | 0.64 | 0.46 | 1.00 | |||||||
X | 0.98 | 0.69 | 0.49 | 0.62 | 1.00 | ||||||
R | 0.63 | 0.57 | 0.28 | 0.19 | 0.63 | 1.00 | |||||
PC | 0.43 | 0.46 | 0.61 | 0.26 | 0.43 | 0.27 | 1.00 | ||||
O | 0.63 | 0.63 | 0.83 | 0.50 | 0.57 | 0.28 | 0.56 | 1.00 | |||
GP | 0.73 | 0.70 | 0.60 | 0.62 | 0.72 | 0.30 | 0.63 | 0.64 | 1.00 | ||
GG | 0.24 | 0.50 | 0.50 | 0.16 | 0.24 | 0.35 | 0.39 | 0.44 | 0.39 | 1.00 | |
M | 0.72 | 0.67 | 0.59 | 0.58 | 0.71 | 0.32 | 0.61 | 0.60 | 0.87 | 0.30 | 1.00 |
We next study the joint prediction power of the different measures as follows. We consider all pairs of measures and find their correlations to human performances (mistake, gap, and time). We use the following definition of the joint correlation:
Rz,xy=√r2xz+r2yz−2∗rxz∗ryz∗rxy1−r2xy | (7) |
where, x and y denote the two measures and z denotes human performance. Rz, xy is the joint correlation between (x, y) and z. The variables rxz. ryz, rxy are the pairwise correlations. Here we need to mention that we consider the signs of all individual correlation coefficients to calculate the correct joint correlation values.
The results are summarized in Tables 7, 8, and 9. The MST branches and path complexity provide the most powerful joint prediction for human mistake (0.57). Human playing time is best predicted by all combinations with intersection (0.39) and the combination of the number of indentations and problem size. In this case, the problem size provides almost no additional benefit to any other measures. Human gap is the most difficult to predict due to the realworld factors. The problem size and the number of intersections provide the best joint prediction (0.25).
Human Mistake | Individual | N | H | C | I | X | R | PC | O | GP | GG | M |
N | 0.45 | - | ||||||||||
H | 0.48 | 0.50 | - | |||||||||
C | 0.39 | 0.49 | 0.49 | - | ||||||||
I | 0.28 | 0.45 | 0.48 | 0.41 | - | |||||||
X | 0.40 | 0.49 | 0.49 | 0.46 | 0.41 | - | ||||||
R | 0.23 | 0.46 | 0.48 | 0.41 | 0.33 | 0.41 | - | |||||
PC | 0.48 | 0.55 | 0.56 | 0.50 | 0.51 | 0.53 | 0.49 | - | ||||
O | 0.43 | 0.49 | 0.51 | 0.43 | 0.44 | 0.47 | 0.44 | 0.52 | - | |||
GP | 0.53 | 0.54 | 0.55 | 0.54 | 0.54 | 0.53 | 0.54 | 0.56 | 0.54 | - | ||
GG | 0.02 | 0.46 | 0.55 | 0.44 | 0.29 | 0.41 | 0.23 | 0.51 | 0.47 | 0.57 | - | |
M | 0.53 | 0.54 | 0.56 | 0.54 | 0.53 | 0.53 | 0.54 | 0.57 | 0.55 | 0.55 | 0.55 | - |
Human Gap | Individual | N | H | C | I | X | R | PC | O | GP | GG | M |
N | 0.06 | - | ||||||||||
H | 0.12 | 0.13 | - | |||||||||
C | 0.02 | 0.06 | 0.15 | - | ||||||||
I | 0.05 | 0.06 | 0.13 | 0.05 | - | |||||||
X | 0.01 | 0.25 | 0.16 | 0.02 | 0.06 | - | ||||||
R | 0.06 | 0.13 | 0.20 | 0.06 | 0.08 | 0.08 | - | |||||
PC | 0.14 | 0.14 | 0.15 | 0.16 | 0.14 | 0.15 | 0.17 | - | ||||
O | 0.11 | 0.11 | 0.13 | 0.18 | 0.11 | 0.13 | 0.14 | 0.15 | - | |||
GP | 0.16 | 0.19 | 0.16 | 0.19 | 0.18 | 0.23 | 0.20 | 0.17 | 0.16 | - | ||
GG | 0.08 | 0.12 | 0.21 | 0.11 | 0.11 | 0.09 | 0.09 | 0.20 | 0.19 | 0.23 | - | |
M | 0.15 | 0.17 | 0.15 | 0.18 | 0.16 | 0.21 | 0.19 | 0.16 | 0.15 | 0.17 | 0.20 | - |
Human time | Individual | N | H | C | I | X | R | PC | O | GP | GG | M |
N | 0.38 | - | ||||||||||
H | 0.30 | 0.38 | - | |||||||||
C | 0.22 | 0.38 | 0.30 | - | ||||||||
I | 0.30 | 0.39 | 0.30 | 0.30 | - | |||||||
X | 0.39 | 0.39 | 0.39 | 0.39 | 0.39 | - | ||||||
R | 0.01 | 0.38 | 0.30 | 0.22 | 0.30 | 0.39 | - | |||||
PC | 0.03 | 0.38 | 0.30 | 0.23 | 0.30 | 0.39 | 0.03 | - | ||||
O | 0.29 | 0.38 | 0.30 | 0.30 | 0.31 | 0.39 | 0.29 | 0.29 | - | |||
GP | 0.35 | 0.38 | 0.35 | 0.35 | 0.36 | 0.39 | 0.36 | 0.36 | 0.36 | - | ||
GG | 0.15 | 0.38 | 0.30 | 0.22 | 0.30 | 0.39 | 0.15 | 0.15 | 0.29 | 0.36 | - | |
M | 0.35 | 0.38 | 0.35 | 0.35 | 0.35 | 0.39 | 0.35 | 0.35 | 0.35 | 0.36 | 0.35 | - |
We studied 11 measures to predict the difficulty of a TSP instance. We implemented those measures and tested them on O-Mopsi and Dots datasets. Our results confirm the previously reported results in the literature. Among the existing measures, the number of targets, intersections, and the points on the convex hull have the best prediction capability in the case of open-loop TSP. Among these, the number of intersections is suitable only for small-scale data due to its O(N2) time complexity.
We have also studied the connection between two related problems, MST and TSP. While their connection is known and utilized in the theory of algorithm, it is much less studied in the context of human problem-solving skills. In this paper, we extend this connection by introducing a measure to estimate the difficulty of TSP instances by counting the number of MST branches in the minimum spanning tree. Experiments show that it has the highest correlation to human mistake, second highest to human gap and third highest correlation to human time for finding the optimal TSP solution. The measure is easy to calculate from the minimum spanning tree and it does not require the optimal solution as a reference.
Two other measures, greedy path and greedy gap, were also introduced. The greedy path has slightly better prediction capability, but it requires the optimal TSP solution as a reference. The best practical predictors seem to be the number of MST branches, the number of nodes, and the number of points on the convex hull.
We also studied the joint correlations of all pairs of measures to human performance to assure the prediction capabilities. The joint correlation results show that the powerful measures are still powerful when used jointly with other measures. Potential future research could be training a machine learning model from all measures jointly to maximize the prediction power.
[1] |
Papadimitriou CH, The Euclidean travelling salesman problem is NP-complete. Theoretical Computer Science, 4(3), 237-244, 1977. doi: 10.1016/0304-3975(77)90012-3 doi: 10.1016/0304-3975(77)90012-3
![]() |
[2] | Phillips N and Phillips R, Rogaining: Cross-Country Navigation. Outdoor Recreation in Australia. ISBN 0-9593329-2-8. |
[3] | Fränti P, Mariescu-Istodor R, and Sengupta L, O-Mopsi: Mobile Orienteering Game for Sightseeing, Exercising, and Education. ACM Transactions on Multimedia Computing, Communications, and Applications, 13(4), 56, 1-12, 2017. doi: 10.1145/3115935 |
[4] | Sengupta L and Fränti P, Predicting the difficulty of TSP instances using MST. IEEE Int. Conf. on Industrial Informatics (INDIN), pp. 847-852, Helsinki 2019. |
[5] |
Vickers D, Mayo T, Heitmann M, Lee MD, and Hughes P, Intelligence and individual differences on three types of visually presented optimisation problems. Personality and Individual Differences, 36, 1059-1071, 2004. doi:10.1016/S0191-8869(03)00200-9 doi: 10.1016/S0191-8869(03)00200-9
![]() |
[6] |
Dry MJ, Preiss K, and Wagemans J, Clustering, Randomness, and Regularity: Spatial Distributions and Human Performance on the Traveling Salesperson Problem and Minimum Spanning Tree Problem. The Journal of Problem Solving, 4(1), 2, 2012. doi: 10.7771/1932-6246.1117 doi: 10.7771/1932-6246.1117
![]() |
[7] |
Graham SM, Joshi A, and Pizlo Z, The traveling salesman problem: A hierarchical model. Memory and Cognition, 28(7), 1191-1204, 2000. doi: 10.3758/BF03211820 doi: 10.3758/BF03211820
![]() |
[8] | Dry MJ, Lee MD, Vickers D, and Hughes P, Human performance on visually presented traveling salesperson problems with varying numbers of nodes. Journal of Problem Solving, 1(1), 4, 2006. doi: 10.7771/1932-6246.1004 |
[9] | Macgregor JN and Ormerod T, Human performance on the traveling salesman problem. Perception and Psychophysics 58: 527-539, 1996. doi: 10.3758/BF03213088 |
[10] |
Chronicle EP, MacGregor JN, Ormerod TC, and Burr A, It looks easy! Heuristics for combinatorial optimisation problems. Quarterly Journal of Experimental Psychology, 59,783–800, 2006. doi: 10.1080/02724980543000033 doi: 10.1080/02724980543000033
![]() |
[11] | Fischer T, Stützle T, Hoos H, and Merz P, An analysis of the hardness of TSP instances for two high performance algorithms. Metaheuristics International Conference, pp. 361-367, 2005. |
[12] |
Applegate D, Bixby R, Chvátal V, and Cook W, Implementing the Dantzig-Fulkerson-Johnson algorithm for large traveling salesman problems. Mathematical Programming, 97, 91-153, 2003. doi: 10.1007/s10107-003-0440-4 doi: 10.1007/s10107-003-0440-4
![]() |
[13] |
Helsgaun K, An effective implementation of the Lin–Kernighan traveling salesman heuristic. European Journal of Operational Research, 126(1), 106-130, 2000. doi 10.1016/S0377-2217(99)00284-2 doi: 10.1016/S0377-2217(99)00284-2
![]() |
[14] |
Leyton-Brown K, Hoos HH, Hutter F, and Xu L, Understanding the empirical hardness of NP-complete problems. Communications of the ACM, 57(5), 98-107, 2014. doi: 10.1145/2594413.2594424 doi: 10.1145/2594413.2594424
![]() |
[15] | Kromer P, Platos J, and Kudelka M, Network measures and evaluation of traveling salesman instance hardness. IEEE Symposium Series on Computational Intelligence (SSCI), pp. 1-7, Honululu, 2017. |
[16] | Ochodkova E, Zehnalova S, and Kudelka M, Graph construction based on local representativeness. International Computing and Combinatorics Conference, pp 654-665. Springer, Cham, 2017. |
[17] |
Vickers D, Lee MD, Dry M, Hughes P, and McMahon JA, The aesthetic appeal of minimal structures: Judging the attractiveness of solutions to traveling salesperson problems. Perception and Psychophysics, 68 (1), 32-42, 2006. doi: 10.3758/BF03193653 doi: 10.3758/BF03193653
![]() |
[18] |
MacGregor JN, Indentations and starting points in traveling sales tour problems: Implications for theory. Journal of Problem Solving, 5(1), 2–17, 2012. doi: 10.7771/1932-6246.1140 doi: 10.7771/1932-6246.1140
![]() |
[19] |
Vickers D, Lee MD, Dry M, and Hughes P, The roles of the convex hull and the number of potential intersections in performance on visually presented traveling salesperson problems. Memory and Cognition, 31(7), 1094-1104, 2003. doi: 10.3758/bf03196130 doi: 10.3758/bf03196130
![]() |
[20] |
Kruskal JB, On the shortest spanning subtree of a graph and the traveling salesman problem. Proceedings of the American Mathematical society, 7(1), 48-50, 1956. doi: 10.1090/S0002-9939-1956-0078686-7 doi: 10.1090/S0002-9939-1956-0078686-7
![]() |
[21] |
Prim RC, Shortest connection networks and some generalizations. The Bell System Technical Journal, 36(6), 1389-1401, 1957. doi: 10.1002/j.1538-7305.1957.tb01515.x doi: 10.1002/j.1538-7305.1957.tb01515.x
![]() |
[22] | Christofides N, Worst-case analysis of a new heuristic for the travelling salesman problem, Report 388, Graduate School of Industrial Administration, CMU, 1976. |
[23] |
Rosenkrantz J, Stearns RE, Lewis II PM, An analysis of several heuristics for the travelling salesman problem, SIAM journal on Computing, 6,563-581, 1977. doi: 10.1137/0206041 doi: 10.1137/0206041
![]() |
[24] | Fränti P and Nenonen H, Modifying Kruskal algorithm to solve open loop TSP. Multidisciplinary International Scheduling Conference (MISTA), pp. 584-590, Ningbo, China, 2019. |
[25] |
Fränti P, Nenonen T, and Yuan M, Converting MST to TSP path by branch elimination, Applied Sciences, 11(177), 1-17, 2021. doi: 10.3390/app11010177 doi: 10.3390/app11010177
![]() |
[26] |
Laporte G, The traveling salesman problem: An overview of exact and approximate algorithms. European Journal of Operational Research, 59(2), 231-247, 1992. doi: 10.1016/0377-2217(92)90138-Y
![]() |
[27] |
Sengupta L, Mariescu-Istodor R, and Fränti P, Planning your route: Where to start?. Computational Brain and Behavior, 1(3), 252-265, 2018. doi: 10.1007/s42113-018-0018-0 doi: 10.1007/s42113-018-0018-0
![]() |
[28] |
MacGregor JN, Chronicle EP, and Ormerod TC, Convex hull or crossing avoidance? Solution heuristics in the traveling salesperson problem. Memory and Cognition, 32(2), 260-270, 2004. doi: 10.3758/bf03196857 doi: 10.3758/bf03196857
![]() |
[29] | Mariescu-Istodor R and Fränti P, Solving large-scale TSP problem in 1 hour: Santa Claus Challenge 2020. Frontiers in Robotics and AI (submitted), 2021. |
[30] |
Andrew AM, Another efficient algorithm for convex hulls in two dimensions. Information Processing Letters, 9(5), 216-219, 1979. doi: 10.1016/0020-0190(79)90072-3 doi: 10.1016/0020-0190(79)90072-3
![]() |
[31] | Ormerod TC, Chronicle EP, Global perceptual processing in problem solving: The case of the traveling salesperson. Perception and Psychophysics 61, 1227–1238, 1999. doi: 10.3758/bf03207625 |
[32] |
Dry MJ and Fontaine EL, Fast and efficient discrimination of traveling salesperson problem stimulus difficulty. The Journal of Problem Solving, 7(1), 9, 2014. doi: 10.7771/1932-6246.1160 doi: 10.7771/1932-6246.1160
![]() |
[33] |
Braden B, The surveyor's area formula. The College Mathematics Journal, 17(4), 326-337, 1986. doi: 10.1080/07468342.1986.11972974 doi: 10.1080/07468342.1986.11972974
![]() |
[34] | Applegate DL, Bixby RE, Chvatal V, and Cook WJ, The traveling salesman problem: a computational study. Princeton University Press, 2011 |
[35] | Quintas LV, Supnick F, Extreme Hamiltonian circuits. Resolution of the convex-even case. Proceedings of the American Mathematical Society, 16(5), 1058-1061, 1965. doi: 10.2307/2035616 |
[36] |
Van Rooij I, Stege U, and Schactman A, Convex hull and tour crossings in the Euclidean traveling salesperson problem: Implications for human performance studies. Memory and Cognition, 31(2), 215-220, 2003. doi: 10.3758/BF03194380 doi: 10.3758/BF03194380
![]() |
[37] |
Yang JQ, Yang JG, and Chen GL, Solving large-scale TSP using adaptive clustering method. IEEE International Symposium on Computational Intelligence and Design, 1, 49-51, 2009. doi: 10.1109/ISCID.2009.19 doi: 10.1109/ISCID.2009.19
![]() |
[38] |
MacGregor JN, Effects of cluster location on human performance on the Traveling Salesperson Problem. The Journal of Problem Solving, 5(2), 3, 2013. doi: 10.7771/1932-6246.1151 doi: 10.7771/1932-6246.1151
![]() |
[39] | Žunić J and Hirota K, Measuring shape circularity. Iberoamerican Congress on Pattern Recognition (pp. 94-101). Springer, Berlin, Heidelberg, 2008. |
[40] | Schick A, Fischer M, and Stiefelhagen R, Measuring and evaluating the compactness of superpixels. International Conference on Pattern Recognition (ICPR), pp. 930-934, IEEE, 2012. |
[41] | Sengupta L, Mariescu-Istodor R, and Fränti P, Which local search operator works best for the open-loop TSP? Applied Sciences, 9(19), 3985, 2019. doi: 10.3390/app9193985 |
[42] | Jormanainen I and Korhonen P, Science festivals on computer science recruitment. Koli Calling International Conference on Computing Education Research (Koli Calling'10). ACM, New York, 72–73, 2010. |
[43] | MacGregor JN, Ormerod TC, and Chronicle EP, Spatial and contextual factors in human performance on the travelling salesperson problem. Perception. 28(11): 1417-1427, 1999. doi: 10.1068/p2863 |
[44] | MacGregor JN, Ormerod TC, and Chronicle EP, A model of human performance on the traveling salesperson problem. Memory and Cognition. 28: 1183-1190, 2000. doi: 10.3758/BF03211819 |
[45] |
Zhao Q and Fränti P, WB-index: A sum-of-squares based index for cluster validity. Data & Knowledge Engineering, 92, 77-89, 2014. doi: 10.1016/j.datak.2014.07.008 doi: 10.1016/j.datak.2014.07.008
![]() |
1. | Víctor Hugo Pacheco-Valencia, Nodari Vakhania, Frank Ángel Hernández-Mira, José Alberto Hernández-Aguilar, A Multi-Phase Method for Euclidean Traveling Salesman Problems, 2022, 11, 2075-1680, 439, 10.3390/axioms11090439 | |
2. | Pasi Fränti, Lingyi Kong, Puzzle-Mopsi: A location-puzzle game, 2023, 3, 2771-392X, 1, 10.3934/aci.2023001 | |
3. | Pasi Fränti, Nancy Fazal, Design Principles for Content Creation in Location-Based Games, 2023, 19, 1551-6857, 1, 10.1145/3583689 | |
4. | Pasi Fränti, Jun Shen, Chih-Cheng Hung, Applied Computing and Intelligence: A new open access journal, 2024, 4, 2771-392X, 19, 10.3934/aci.2024002 |
Measure: |
Properties: | |||
Time complexity | Needs optimal | Simple | Suitable for open-loop | |
N | O(1) | - | Yes | Yes |
H | O(N*logN) | - | Yes | Yes |
C | O(N2) | Yes | - | - |
I | O(N*logN) | Yes | Yes | Yes |
X | O(N4) | - | Yes | Yes |
R | O(N2) | - | Yes | Yes |
PC | O(N2) | Yes | Yes | Yes |
O | O(N) | Yes | - | - |
GP | O(N3) | Yes | Yes | Yes |
GG | O(N2) | Yes | Yes | Yes |
M | O(N2) | - | Yes | Yes |
Measure | Studied | Methods | Conclusions |
N | Graham et al. (2000) [7] Dry et al. (2006) [8] |
Human time | Linear or near-linear growth |
H | MacGregor & Ormerod (1996) [9] | Gap, Response uncertainty | More targets easier |
Macgregor et al. (2004) [28] | Gap | More targets easier | |
Vickers et al. (2003) [19] | Gap, Response uncertainty | Fewer targets easier | |
Chronicle et al. (2006) [10] | Gap, Solved-% | More targets easier | |
Dry and Fontaine (2014) [32] | Choice time, Choice probabilities | Fewer targets easier | |
C | Dry and Fontaine (2014) [32] | Choice time, Choice probabilities | Does not matter |
I | MacGregor (2012) [18] | Gap, Solved-% | Fewer indentations easier |
Dry and Fontaine (2014) [32] | Choice time, Choice probabilities | Fewer indentations easier | |
X | Vickers et al. (2003) [19] | Gap, Response uncertainty | More intersections easier |
Dry and Fontaine (2014) [32] | Choice time, Choice probabilities | More intersections easier | |
R | Dry et al. (2012) [6] | Gap, Response uncertainty, Solving time | Clustered easier |
MacGregor (2013) [38] | Gap | Cluster locations is important | |
PC | Vickers et al. (2004) [5] | Gap | Lower value easier |
O | Vickers et al. (2006) [17] | Survey | Circularity more attractive |
Dataset | Type | Distance | Instances | Sizes |
O-Mopsi | Openloop | Haversine | 147 | 4−27 |
Dots | Openloop | Euclidean | 12124 | 4−50 |
Human performance | Computer processing time | ||||||
O-Mopsi | Dots | O-Mopsi | Dots | ||||
Human mistake | Human gap | Human time | Concorde time | LS time | Concorde time | LS time | |
N | 0.45 | 0.06 | 0.38 | 0.75 | 0.66 | 0.61 | 0.63 |
H | 0.48 | 0.12 | 0.30 | 0.67 | 0.52 | 0.50 | 0.42 |
C | 0.39 | 0.02 | 0.22 | 0.50 | 0.39 | 0.35 | 0.29 |
I | 0.28 | 0.05 | 0.30 | 0.52 | 0.48 | 0.51 | 0.43 |
I (normalized) | 0.02 | 0.04 | 0.01 | 0.01 | 0.01 | 0.10 | 0.03 |
X | 0.40 | 0.01 | 0.39 | 0.72 | 0.72 | 0.62 | 0.75 |
X (normalized) | 0.49 | 0.13 | 0.29 | 0.70 | 0.44 | 0.49 | 0.35 |
R | 0.22 | 0.06 | 0.01 | 0.45 | 0.37 | 0.05 | 0.08 |
PC | 0.48 | 0.14 | 0.03 | 0.48 | 0.23 | 0.06 | 0.06 |
O | 0.43 | 0.11 | 0.29 | 0.51 | 0.37 | 0.47 | 0.37 |
GP | 0.53 | 0.16 | 0.35 | 0.68 | 0.50 | 0.58 | 0.64 |
GG | 0.02 | 0.08 | 0.15 | 0.24 | 0.21 | 0.25 | 0.22 |
M | 0.53 | 0.15 | 0.35 | 0.69 | 0.55 | 0.56 | 0.60 |
M (normalized) | 0.44 | 0.11 | 0.13 | 0.48 | 0.30 | 0.22 | 0.18 |
Legends: Problem size (N), Targets on the convex hull (H), Convexity (C), Indentations (I), Intersections (X), Regularity (R), Path complexity (PC), Circularity (O), MST branches (M), Greedy path (GP), Greedy gap (GG). |
Human performance | Computer processing time | Mean | ||||||
O-Mopsi | Dots | O-Mopsi | Dots | |||||
Human mistake | Human gap | Human time | Concorde time | LS time | Concorde time | LS time | ||
N | 3 | 8 | 2 | 1 | 2 | 2 | 3 | 3.00 |
H | 2 | 5 | 4 | 6 | 4 | 6 | 6 | 4.71 |
C | 7 | 11 | 6 | 9 | 8 | 9 | 9 | 8.43 |
I | 8 | 10 | 4 | 7 | 6 | 5 | 5 | 6.43 |
I (normalized) | 10 | 10 | 10 | 13 | 13 | 12 | 14 | 11.71 |
X | 6 | 12 | 1 | 2 | 1 | 1 | 1 | 3.43 |
X (normalized) | 2 | 4 | 5 | 3 | 7 | 7 | 8 | 5.14 |
R | 9 | 9 | 10 | 11 | 9 | 14 | 12 | 10.57 |
PC | 2 | 3 | 9 | 10 | 11 | 13 | 13 | 8.71 |
O | 5 | 6 | 5 | 8 | 9 | 8 | 7 | 6.86 |
GP | 1 | 1 | 3 | 5 | 5 | 3 | 2 | 2.86 |
GG | 10 | 7 | 7 | 12 | 12 | 10 | 10 | 9.71 |
M | 1 | 2 | 3 | 4 | 3 | 4 | 4 | 3.00 |
M (normalized) | 4 | 6 | 8 | 10 | 10 | 11 | 11 | 8.57 |
N | H | C | I | X | R | PC | O | GP | GG | M | |
N | 1.00 | ||||||||||
H | 0.72 | 1.00 | |||||||||
C | 0.51 | 0.66 | 1.00 | ||||||||
I | 0.67 | 0.64 | 0.46 | 1.00 | |||||||
X | 0.98 | 0.69 | 0.49 | 0.62 | 1.00 | ||||||
R | 0.63 | 0.57 | 0.28 | 0.19 | 0.63 | 1.00 | |||||
PC | 0.43 | 0.46 | 0.61 | 0.26 | 0.43 | 0.27 | 1.00 | ||||
O | 0.63 | 0.63 | 0.83 | 0.50 | 0.57 | 0.28 | 0.56 | 1.00 | |||
GP | 0.73 | 0.70 | 0.60 | 0.62 | 0.72 | 0.30 | 0.63 | 0.64 | 1.00 | ||
GG | 0.24 | 0.50 | 0.50 | 0.16 | 0.24 | 0.35 | 0.39 | 0.44 | 0.39 | 1.00 | |
M | 0.72 | 0.67 | 0.59 | 0.58 | 0.71 | 0.32 | 0.61 | 0.60 | 0.87 | 0.30 | 1.00 |
Human Mistake | Individual | N | H | C | I | X | R | PC | O | GP | GG | M |
N | 0.45 | - | ||||||||||
H | 0.48 | 0.50 | - | |||||||||
C | 0.39 | 0.49 | 0.49 | - | ||||||||
I | 0.28 | 0.45 | 0.48 | 0.41 | - | |||||||
X | 0.40 | 0.49 | 0.49 | 0.46 | 0.41 | - | ||||||
R | 0.23 | 0.46 | 0.48 | 0.41 | 0.33 | 0.41 | - | |||||
PC | 0.48 | 0.55 | 0.56 | 0.50 | 0.51 | 0.53 | 0.49 | - | ||||
O | 0.43 | 0.49 | 0.51 | 0.43 | 0.44 | 0.47 | 0.44 | 0.52 | - | |||
GP | 0.53 | 0.54 | 0.55 | 0.54 | 0.54 | 0.53 | 0.54 | 0.56 | 0.54 | - | ||
GG | 0.02 | 0.46 | 0.55 | 0.44 | 0.29 | 0.41 | 0.23 | 0.51 | 0.47 | 0.57 | - | |
M | 0.53 | 0.54 | 0.56 | 0.54 | 0.53 | 0.53 | 0.54 | 0.57 | 0.55 | 0.55 | 0.55 | - |
Human Gap | Individual | N | H | C | I | X | R | PC | O | GP | GG | M |
N | 0.06 | - | ||||||||||
H | 0.12 | 0.13 | - | |||||||||
C | 0.02 | 0.06 | 0.15 | - | ||||||||
I | 0.05 | 0.06 | 0.13 | 0.05 | - | |||||||
X | 0.01 | 0.25 | 0.16 | 0.02 | 0.06 | - | ||||||
R | 0.06 | 0.13 | 0.20 | 0.06 | 0.08 | 0.08 | - | |||||
PC | 0.14 | 0.14 | 0.15 | 0.16 | 0.14 | 0.15 | 0.17 | - | ||||
O | 0.11 | 0.11 | 0.13 | 0.18 | 0.11 | 0.13 | 0.14 | 0.15 | - | |||
GP | 0.16 | 0.19 | 0.16 | 0.19 | 0.18 | 0.23 | 0.20 | 0.17 | 0.16 | - | ||
GG | 0.08 | 0.12 | 0.21 | 0.11 | 0.11 | 0.09 | 0.09 | 0.20 | 0.19 | 0.23 | - | |
M | 0.15 | 0.17 | 0.15 | 0.18 | 0.16 | 0.21 | 0.19 | 0.16 | 0.15 | 0.17 | 0.20 | - |
Human time | Individual | N | H | C | I | X | R | PC | O | GP | GG | M |
N | 0.38 | - | ||||||||||
H | 0.30 | 0.38 | - | |||||||||
C | 0.22 | 0.38 | 0.30 | - | ||||||||
I | 0.30 | 0.39 | 0.30 | 0.30 | - | |||||||
X | 0.39 | 0.39 | 0.39 | 0.39 | 0.39 | - | ||||||
R | 0.01 | 0.38 | 0.30 | 0.22 | 0.30 | 0.39 | - | |||||
PC | 0.03 | 0.38 | 0.30 | 0.23 | 0.30 | 0.39 | 0.03 | - | ||||
O | 0.29 | 0.38 | 0.30 | 0.30 | 0.31 | 0.39 | 0.29 | 0.29 | - | |||
GP | 0.35 | 0.38 | 0.35 | 0.35 | 0.36 | 0.39 | 0.36 | 0.36 | 0.36 | - | ||
GG | 0.15 | 0.38 | 0.30 | 0.22 | 0.30 | 0.39 | 0.15 | 0.15 | 0.29 | 0.36 | - | |
M | 0.35 | 0.38 | 0.35 | 0.35 | 0.35 | 0.39 | 0.35 | 0.35 | 0.35 | 0.36 | 0.35 | - |
Measure: |
Properties: | |||
Time complexity | Needs optimal | Simple | Suitable for open-loop | |
N | O(1) | - | Yes | Yes |
H | O(N*logN) | - | Yes | Yes |
C | O(N2) | Yes | - | - |
I | O(N*logN) | Yes | Yes | Yes |
X | O(N4) | - | Yes | Yes |
R | O(N2) | - | Yes | Yes |
PC | O(N2) | Yes | Yes | Yes |
O | O(N) | Yes | - | - |
GP | O(N3) | Yes | Yes | Yes |
GG | O(N2) | Yes | Yes | Yes |
M | O(N2) | - | Yes | Yes |
Measure | Studied | Methods | Conclusions |
N | Graham et al. (2000) [7] Dry et al. (2006) [8] |
Human time | Linear or near-linear growth |
H | MacGregor & Ormerod (1996) [9] | Gap, Response uncertainty | More targets easier |
Macgregor et al. (2004) [28] | Gap | More targets easier | |
Vickers et al. (2003) [19] | Gap, Response uncertainty | Fewer targets easier | |
Chronicle et al. (2006) [10] | Gap, Solved-% | More targets easier | |
Dry and Fontaine (2014) [32] | Choice time, Choice probabilities | Fewer targets easier | |
C | Dry and Fontaine (2014) [32] | Choice time, Choice probabilities | Does not matter |
I | MacGregor (2012) [18] | Gap, Solved-% | Fewer indentations easier |
Dry and Fontaine (2014) [32] | Choice time, Choice probabilities | Fewer indentations easier | |
X | Vickers et al. (2003) [19] | Gap, Response uncertainty | More intersections easier |
Dry and Fontaine (2014) [32] | Choice time, Choice probabilities | More intersections easier | |
R | Dry et al. (2012) [6] | Gap, Response uncertainty, Solving time | Clustered easier |
MacGregor (2013) [38] | Gap | Cluster locations is important | |
PC | Vickers et al. (2004) [5] | Gap | Lower value easier |
O | Vickers et al. (2006) [17] | Survey | Circularity more attractive |
Dataset | Type | Distance | Instances | Sizes |
O-Mopsi | Openloop | Haversine | 147 | 4−27 |
Dots | Openloop | Euclidean | 12124 | 4−50 |
Human performance | Computer processing time | ||||||
O-Mopsi | Dots | O-Mopsi | Dots | ||||
Human mistake | Human gap | Human time | Concorde time | LS time | Concorde time | LS time | |
N | 0.45 | 0.06 | 0.38 | 0.75 | 0.66 | 0.61 | 0.63 |
H | 0.48 | 0.12 | 0.30 | 0.67 | 0.52 | 0.50 | 0.42 |
C | 0.39 | 0.02 | 0.22 | 0.50 | 0.39 | 0.35 | 0.29 |
I | 0.28 | 0.05 | 0.30 | 0.52 | 0.48 | 0.51 | 0.43 |
I (normalized) | 0.02 | 0.04 | 0.01 | 0.01 | 0.01 | 0.10 | 0.03 |
X | 0.40 | 0.01 | 0.39 | 0.72 | 0.72 | 0.62 | 0.75 |
X (normalized) | 0.49 | 0.13 | 0.29 | 0.70 | 0.44 | 0.49 | 0.35 |
R | 0.22 | 0.06 | 0.01 | 0.45 | 0.37 | 0.05 | 0.08 |
PC | 0.48 | 0.14 | 0.03 | 0.48 | 0.23 | 0.06 | 0.06 |
O | 0.43 | 0.11 | 0.29 | 0.51 | 0.37 | 0.47 | 0.37 |
GP | 0.53 | 0.16 | 0.35 | 0.68 | 0.50 | 0.58 | 0.64 |
GG | 0.02 | 0.08 | 0.15 | 0.24 | 0.21 | 0.25 | 0.22 |
M | 0.53 | 0.15 | 0.35 | 0.69 | 0.55 | 0.56 | 0.60 |
M (normalized) | 0.44 | 0.11 | 0.13 | 0.48 | 0.30 | 0.22 | 0.18 |
Legends: Problem size (N), Targets on the convex hull (H), Convexity (C), Indentations (I), Intersections (X), Regularity (R), Path complexity (PC), Circularity (O), MST branches (M), Greedy path (GP), Greedy gap (GG). |
Human performance | Computer processing time | Mean | ||||||
O-Mopsi | Dots | O-Mopsi | Dots | |||||
Human mistake | Human gap | Human time | Concorde time | LS time | Concorde time | LS time | ||
N | 3 | 8 | 2 | 1 | 2 | 2 | 3 | 3.00 |
H | 2 | 5 | 4 | 6 | 4 | 6 | 6 | 4.71 |
C | 7 | 11 | 6 | 9 | 8 | 9 | 9 | 8.43 |
I | 8 | 10 | 4 | 7 | 6 | 5 | 5 | 6.43 |
I (normalized) | 10 | 10 | 10 | 13 | 13 | 12 | 14 | 11.71 |
X | 6 | 12 | 1 | 2 | 1 | 1 | 1 | 3.43 |
X (normalized) | 2 | 4 | 5 | 3 | 7 | 7 | 8 | 5.14 |
R | 9 | 9 | 10 | 11 | 9 | 14 | 12 | 10.57 |
PC | 2 | 3 | 9 | 10 | 11 | 13 | 13 | 8.71 |
O | 5 | 6 | 5 | 8 | 9 | 8 | 7 | 6.86 |
GP | 1 | 1 | 3 | 5 | 5 | 3 | 2 | 2.86 |
GG | 10 | 7 | 7 | 12 | 12 | 10 | 10 | 9.71 |
M | 1 | 2 | 3 | 4 | 3 | 4 | 4 | 3.00 |
M (normalized) | 4 | 6 | 8 | 10 | 10 | 11 | 11 | 8.57 |
N | H | C | I | X | R | PC | O | GP | GG | M | |
N | 1.00 | ||||||||||
H | 0.72 | 1.00 | |||||||||
C | 0.51 | 0.66 | 1.00 | ||||||||
I | 0.67 | 0.64 | 0.46 | 1.00 | |||||||
X | 0.98 | 0.69 | 0.49 | 0.62 | 1.00 | ||||||
R | 0.63 | 0.57 | 0.28 | 0.19 | 0.63 | 1.00 | |||||
PC | 0.43 | 0.46 | 0.61 | 0.26 | 0.43 | 0.27 | 1.00 | ||||
O | 0.63 | 0.63 | 0.83 | 0.50 | 0.57 | 0.28 | 0.56 | 1.00 | |||
GP | 0.73 | 0.70 | 0.60 | 0.62 | 0.72 | 0.30 | 0.63 | 0.64 | 1.00 | ||
GG | 0.24 | 0.50 | 0.50 | 0.16 | 0.24 | 0.35 | 0.39 | 0.44 | 0.39 | 1.00 | |
M | 0.72 | 0.67 | 0.59 | 0.58 | 0.71 | 0.32 | 0.61 | 0.60 | 0.87 | 0.30 | 1.00 |
Human Mistake | Individual | N | H | C | I | X | R | PC | O | GP | GG | M |
N | 0.45 | - | ||||||||||
H | 0.48 | 0.50 | - | |||||||||
C | 0.39 | 0.49 | 0.49 | - | ||||||||
I | 0.28 | 0.45 | 0.48 | 0.41 | - | |||||||
X | 0.40 | 0.49 | 0.49 | 0.46 | 0.41 | - | ||||||
R | 0.23 | 0.46 | 0.48 | 0.41 | 0.33 | 0.41 | - | |||||
PC | 0.48 | 0.55 | 0.56 | 0.50 | 0.51 | 0.53 | 0.49 | - | ||||
O | 0.43 | 0.49 | 0.51 | 0.43 | 0.44 | 0.47 | 0.44 | 0.52 | - | |||
GP | 0.53 | 0.54 | 0.55 | 0.54 | 0.54 | 0.53 | 0.54 | 0.56 | 0.54 | - | ||
GG | 0.02 | 0.46 | 0.55 | 0.44 | 0.29 | 0.41 | 0.23 | 0.51 | 0.47 | 0.57 | - | |
M | 0.53 | 0.54 | 0.56 | 0.54 | 0.53 | 0.53 | 0.54 | 0.57 | 0.55 | 0.55 | 0.55 | - |
Human Gap | Individual | N | H | C | I | X | R | PC | O | GP | GG | M |
N | 0.06 | - | ||||||||||
H | 0.12 | 0.13 | - | |||||||||
C | 0.02 | 0.06 | 0.15 | - | ||||||||
I | 0.05 | 0.06 | 0.13 | 0.05 | - | |||||||
X | 0.01 | 0.25 | 0.16 | 0.02 | 0.06 | - | ||||||
R | 0.06 | 0.13 | 0.20 | 0.06 | 0.08 | 0.08 | - | |||||
PC | 0.14 | 0.14 | 0.15 | 0.16 | 0.14 | 0.15 | 0.17 | - | ||||
O | 0.11 | 0.11 | 0.13 | 0.18 | 0.11 | 0.13 | 0.14 | 0.15 | - | |||
GP | 0.16 | 0.19 | 0.16 | 0.19 | 0.18 | 0.23 | 0.20 | 0.17 | 0.16 | - | ||
GG | 0.08 | 0.12 | 0.21 | 0.11 | 0.11 | 0.09 | 0.09 | 0.20 | 0.19 | 0.23 | - | |
M | 0.15 | 0.17 | 0.15 | 0.18 | 0.16 | 0.21 | 0.19 | 0.16 | 0.15 | 0.17 | 0.20 | - |
Human time | Individual | N | H | C | I | X | R | PC | O | GP | GG | M |
N | 0.38 | - | ||||||||||
H | 0.30 | 0.38 | - | |||||||||
C | 0.22 | 0.38 | 0.30 | - | ||||||||
I | 0.30 | 0.39 | 0.30 | 0.30 | - | |||||||
X | 0.39 | 0.39 | 0.39 | 0.39 | 0.39 | - | ||||||
R | 0.01 | 0.38 | 0.30 | 0.22 | 0.30 | 0.39 | - | |||||
PC | 0.03 | 0.38 | 0.30 | 0.23 | 0.30 | 0.39 | 0.03 | - | ||||
O | 0.29 | 0.38 | 0.30 | 0.30 | 0.31 | 0.39 | 0.29 | 0.29 | - | |||
GP | 0.35 | 0.38 | 0.35 | 0.35 | 0.36 | 0.39 | 0.36 | 0.36 | 0.36 | - | ||
GG | 0.15 | 0.38 | 0.30 | 0.22 | 0.30 | 0.39 | 0.15 | 0.15 | 0.29 | 0.36 | - | |
M | 0.35 | 0.38 | 0.35 | 0.35 | 0.35 | 0.39 | 0.35 | 0.35 | 0.35 | 0.36 | 0.35 | - |