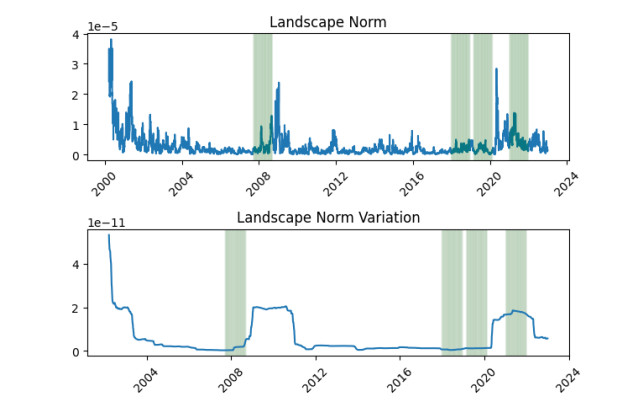
We investigate market crashes and downturns through the lens of persistent homology and persistence landscape norms. Using individual stock price data from Yahoo! Finance, we find that the variation in the persistence landscape norm as well as other measures of persistence exhibit a marked increase followed by a decline prior to historic incidents. We show that basic descriptions of persistent homology may be useful in addition to more sophisticated tools like the persistence landscape norm.
Citation: Aaron D Valdivia. Topological variability in financial markets[J]. Quantitative Finance and Economics, 2023, 7(3): 391-402. doi: 10.3934/QFE.2023019
[1] | Fredrik Hobbelhagen, Ioannis Diamantis . A comparative study of symbolic aggregate approximation and topological data analysis. Quantitative Finance and Economics, 2024, 8(4): 705-732. doi: 10.3934/QFE.2024027 |
[2] | Janina Engel, Markus Wahl, Rudi Zagst . Forecasting turbulence in the Asian and European stock market using regime-switching models. Quantitative Finance and Economics, 2018, 2(2): 388-406. doi: 10.3934/QFE.2018.2.388 |
[3] | Didier Sornette, Peter Cauwels, Georgi Smilyanov . Can we use volatility to diagnose financial bubbles? lessons from 40 historical bubbles. Quantitative Finance and Economics, 2018, 2(1): 486-590. doi: 10.3934/QFE.2018.1.1 |
[4] | Akash Deep . Advanced financial market forecasting: integrating Monte Carlo simulations with ensemble Machine Learning models. Quantitative Finance and Economics, 2024, 8(2): 286-314. doi: 10.3934/QFE.2024011 |
[5] | Miquel Carreras-Simó, Germà Coenders . The relationship between asset and capital structure: a compositional approach with panel vector autoregressive models. Quantitative Finance and Economics, 2021, 5(4): 571-590. doi: 10.3934/QFE.2021025 |
[6] | Wenyan Hao, Claude Lefèvre, Muhsin Tamturk, Sergey Utev . Quantum option pricing and data analysis. Quantitative Finance and Economics, 2019, 3(3): 490-507. doi: 10.3934/QFE.2019.3.490 |
[7] | Wenhao Chen, Kinkeung Lai, Yi Cai . Topic generation for Chinese stocks: a cognitively motivated topic modelingmethod using social media data. Quantitative Finance and Economics, 2018, 2(2): 279-293. doi: 10.3934/QFE.2018.2.279 |
[8] | Makoto Nakakita, Teruo Nakatsuma . Analysis of the trading interval duration for the Bitcoin market using high-frequency transaction data. Quantitative Finance and Economics, 2025, 9(1): 202-241. doi: 10.3934/QFE.2025007 |
[9] | Oleg Sukharev, Ekaterina Voronchikhina . Financial and non-financial investments: comparative econometric analysis of the impact on economic dynamics. Quantitative Finance and Economics, 2020, 4(3): 382-411. doi: 10.3934/QFE.2020018 |
[10] | Ana Sousa, Ana Braga, Jorge Cunha . Impact of macroeconomic indicators on bankruptcy prediction models: Case of the Portuguese construction sector. Quantitative Finance and Economics, 2022, 6(3): 405-432. doi: 10.3934/QFE.2022018 |
We investigate market crashes and downturns through the lens of persistent homology and persistence landscape norms. Using individual stock price data from Yahoo! Finance, we find that the variation in the persistence landscape norm as well as other measures of persistence exhibit a marked increase followed by a decline prior to historic incidents. We show that basic descriptions of persistent homology may be useful in addition to more sophisticated tools like the persistence landscape norm.
Topological data analysis introduced by Carlsson (2009) refers to the ways in which topology can be used to describe discrete information. In particular we will be interested in persistent homology which essentially, given a discrete set of points and a metric, is a record of the homology classes of the manifolds obtained by taking the union of ϵ-neighborhoods around each point for each ϵ>0. With this record, one can view how the geometry changes at different values of ϵ or infer the geometry of the underlying manifold that the data is sampled from. The aim is to use these topological features to help describe complicated nonlinear relationships in large datasets of high dimension. One area of study where these methods have been applied is econometrics, see for instance Gidea and Katz (2018) and Yen and Cheong (2021), which in part is interested in the behavior and the driving forces of financial markets. Understanding patterns of behavior in these markets may enable analysts to better craft financial policies and more easily prevent large economic downturns or crashes. We will show that persistent homology can be used to identify time periods during which crashes become more likely and examine new ways of summarizing the information in the persistent homology making it more accessible.
Identifying indicators or early warning signals (EWS) of market crashes often takes the approach of viewing markets as dynamical systems with crashes appearing as critical transitions in the system Bascompte et al. (2009). The critical transitions in some nonlinear complex dynamical systems exhibit a phenomenon called critical slowing down which is not found in financial markets, Goel et al. (2016), distinguishing economic transitions from other physical phenomenon. However, the authors in Goel et al. (2016) notice that approaching historical crashes a marked rise in the variance of those prices can be observed. Variance of prices or volatility is a heavily studied measure of financial markets see Antonakakis et al. (2018), Edwards et al. (2003) and Hsieh (1995). However, volatility and its relationship with co-movement of stock prices is usually studied through an individual assets price or through correlations, comparing two assets prices at a time. Topological data analysis allows us to create measures whose variance, as a single value, relates to the changing geometric relationships of large classes of stocks. This is a stark change in view of what the volatility of an index is, changing the view from "what is the variance of the value of the index" to "how is the geometry of the index shifting over time."
A first step at considering this topological variance is investigated in Gidea and Katz (2018), in which the authors consider the persistent homology using four market indices as coordinates and a measure called the persistence landscape norm developed in Bubenick (2015). In their paper, Gidea and Katz find that the persistence landscape norm of the rank 1 homology increases quickly towards the crashes associated to the dot-com bubble and Lehman Brothers collapse. They also show that the variability in the persistence landscape norm increases over a 250 day window before those crashes.
However, when considering the crash associated to the US-China trade war in 2018, the crash associated to the covid-19 pandemic and the market downturn called the 2022 decline, we see a weaker rise in norm for the trade war and fail to see the increasing norm for the covid-19 pandemic and 2022 market decline. Considering the variation of these norms, we find weak increases for the trade war and covid-19 pandemic and do not observe the rise in variation for the 2022 decline. We consider persistent homology in dimensions 0, 1 and 2 to look for a clearer picture of all market declines.
We consider each stock as a point whose distance to other stocks is defined by their Pearson correlation distance over a 50 day window. We will be interested in the persistent homology in dimensions up to and including 2. We examine the persistence landscape norms and their variations in each dimension as well as the interaction of these norms. We will consider two analogies with the Euler characteristic: i) the alternating sum of these norms after normalization, much like the alternating sum of the Betti numbers and ii) the ratio of the product of the rank 0 and rank 2 homology norms to the rank 1 homology norm after normalization. The second analogy is akin to a multiplicative version of the Euler characteristic. We find large spikes and drops in the variations of these measures prior to crashes.
We will demonstrate that the persistent homology differs among crashes and market changes. We will look at simple descriptions of the persistent homology like the width and height, the window in which the topological features appear in terms of ϵ and the maximum persistence of a class respectively. We also consider ways in which these simpler measures relate to the persistence landscape norms to develop simpler descriptions of overall persistence and propose a measure of market instability that appears to capture indications of all market crashes and downturns considered here.
In section 2 we review relevant background material. In section 3.1 we discuss how the Lehman Brothers collapse differs from later market changes while viewing days as points. In section 3.2 we will discuss the persistence homology for all four market changes while viewing individual stocks as points. In section 3.3 we will discuss the possible early warning signals for market transitions based on our results.
While the topology of a point cloud is trivial, topological data analysis, developed in Carlsson (2009), allows us to view point clouds as simplicial manifolds. For a given point cloud D={x1…xn} we define a simplicial complex for every ϵ≥0 such that a set of points defines a k−simplex S={x1…xk} for each collection S where d(xi,xj)≤ϵ for all xi,xj∈S. The simplicial complex C(D,ϵ)⊂C(D,ϵ′) for all ϵ≤ϵ′. These inclusions induce canonical homomorphisms on the homology groups, with given field coefficients, associated to these complexes Hk(C(D,ϵ))→Hk(C(D,ϵ′)). These maps ensure that for any non-zero homology class α∈Hk(C(D,ϵ)) there is a minimum and maximum value ϵ1 and ϵ2 for which the class α exists and is non-zero. Furthermore α exists and is non-zero for all ϵ1≤ϵ≤ϵ2. We call ϵ1 the birth for α and ϵ2 the death. The collection of all these pairs in dimension n is denoted Pn.
A persistence diagram is then a graph of the points Pn often with multiple values of n displayed in different colors. These persistence diagrams give a summary of the persistent homology, the information on all homology classes for all values of ϵ. We define the n-width, wn, of the persistence diagram to be bmax−bmin, where the bmax is the largest birth time for a class in dimension n and bmin the minimum birth time for a class in dimension n. We also define the height of a class hn=maxi(di−bi), where bi is the birth of a homology class and di is the death of a homology class we let i range over all classes in Pn. With these measures, we can define the area an=wnhn and what we call the inv invn=hnwn.
A fuller description of the persistence diagram is the persistence landscape. The persistence landscape is a function defined on the birth and death times of the homology classes in a particular dimension. For each homology class α, there is an associated point (bα,dα) whose coordinates are the birth and death times for the homology class. A function for each homology class, α, is then defined by
fα(x)={x−bαbα<x≤bα+dα2dα−xbα+dα2<x<dα0x∉(bα,dα) |
The landscape in dimension n is then the function
λn(x,k)=k−max(fα(x)∣α∈Pn) | (1) |
where k−max gives the kth largest value or 0 if such a value does not exist. This function gives a stratification of the topological features the persistence diagram is picking out. When k=1, we are looking at a function describing the most persistent classes. As k increases, we see the functions describing less persistent classes. For each value of k, the function is a series of triangular peaks like a mountain range, hence the name landscape. Considering different values of k, one can view a function that says something about the overall abundance and distribution of topological features with relatively similar persistence. To more easily view this information and quantify it as a single value, we introduce a norm. The norm we will use is actually just the standard L1 norm. The Lp norm on these functions for any integer p is defined by
Λ(λn(x,k),p)=||λn(x,k)||p=(∞∑k=1(∫Rλ(x,k)p∂x))1p. | (2) |
The persistence landscape norm with p=1 will be denoted Λn for the rank n homology.
In this section we will reproduce the method of analysis in Gidea and Katz (2018) and see that the increasing behavior in the persistence landscape norm continues strongly after the Lehman Brothers collapse but also is not as predictive for the market crashes and downturns since then. It would appear that these more recent events require different tools to identify.
First, we recall the methods of analysis. The data is taken from Yahoo! Finance and the TDA algorithms come from the python package, scikit-tda. We will be considering the log returns for the Dow Jones Industrial, the S & P 500 index, the NASDAQ, and the Russel 2000. We use a sliding window of 50 trading days sliding by one day at a time computing the persistent homology on each window. To be explicit, each day is a point with coordinates the log returns of the different indices. We compute the persistence landscape norm on each persistence diagram and the variation of this norm over a window of length 500.
We consider the persistence landscape norm and its variation over decades in Figure 1. We can clearly see the rise in norm and variation prior to the Lehman Brothers Collapse. However that behavior does not appear in the subsequent crashes (the year prior to the crash is highlighted in green). We also note that the rise associated to the Lehman Brothers Collapse continues significantly after the crash.
In order to try to uncover some differences in these time periods we take a look at cruder measures of the persistent homology. The persistence landscape norm captures, in a way, the full sum of the persistent homology group. We will look at descriptions or pieces of this sum defined in the previous section. The width, wn, height hn, area an and inverse invn.
The reasoning behind looking at each of these measures is their relation to the persistence landscape norm. The persistence landscape norm measures overall persistence of all classes, measuring the most persistent classes first. The width measures the range over which this sum must be taken. A large width can contribute to the persistence landscape norm in that there are more classes over a longer range. However, width can detract from the norm since given a set number of points having geometric features at too many different scales might indicate a lack of persistence of those features. The height is directly related to the persistence, but only the largest class's persistence. The area then gives a very cursory and one dimensional look at the persistence norm capturing a box in which all the pieces of the landscape lie. On the other hand, the inv gives a measure of how persistent the geometric features might be together with a sense of how concentrated the geometric information is. For instance, a small width indicates that all the homology classes are born closer together. Therefore, it is more likely that when computing the persistence landscape norm the k−max must be computed for larger values of k. Clearly these are all heuristic and the picture is not so simple.
We consider these different measures across large time frames. We can notice that on their own these measures do not show clear behavior but looking at their variations is a different story. Area seems to be a very close representation of the persistence landscape norm variation. We can also see that the width and height variations resemble the picture for the landscape variation. Looking at all the images, it seems that the Lehman brothers collapse happens at the beginning of a rise in the landscape variation where the decline of 2022 occurs at the top of a local maximum. The Trade war crash and covid crash appear to happen at a minimum for most measures. Figure 2 shows the height and its variation as an example. Width and area are similar while inv has one large peak well after the Lehman crash.
In an attempt to look for more nuanced information in the dimension 2 persistent homology we notice there rarely is any. This may be due to the small number of points used or to the flattening of the market information into four indices. In the next section we will attempt to take a more granular look at the market in general.
In this section, we try to get a closer look at market behavior by considering the prices of all stocks on an index rather than just the price of the index in total. Again, all data comes from Yahoo! Finance with persistent homology calculated using the python package scikit-tda. We choose the S & P 500 and consider the trading days from 2000 to 2023. Then, we calculate the returns for each day as a percentage above or below the previous day. From the percentages, we consider 50 trading day windows sliding by 1 trading day at a time. For each window, we define the distance between 2 stocks as their Pearson correlation distance, d(x,y)=√1−ρ(x,y) where ρ(x,y) is the Pearson correlation between x and y. From these distances, we can calculate the persistent homology. This approach is different than that in Gidea and Katz (2018). Our reasoning for this change is two fold, while still considering information over a 50 day window we can gather more points. Second, if we had used each stock price as a coordinate we would have a very high dimensional space, the number of points would then be extremely sparse in this space and not be likely to give a good picture of the geometric behavior.
We will compute the persistence homology up to and including rank 2. We again will be interested in the persistence landscape norms and their variations in ranks 0, 1 and 2 as well as the variations of the width, height, area and inv in each of dimensions 1 and 2. We exclude rank 0 when computing the width, height, area and inv because the width in rank 0 is always 0. Additionally, we will be interested in the how these homology groups interact. In their paper, Yen and Cheong (2021) assert that the Euler characteristic is positive prior to market crashes. This relies on two choices of cutoff values, one the rank in which to cease the alternating sum of Betti numbers and two the time at which all remaining classes will be counted towards the Betti numbers. Rather than attempt to make up such a cutoff, we will try to come up with ways to indicate rise or fall in Euler Characteristic. Heuristically, the persistence landscape norms indicate a stronger likelihood of more classes, higher Betti number. Lower rank 1 Betti numbers and higher rank 0 or rank 2 Betti numbers contribute to higher Euler Characteristic so we define the Euler ratio as the ratio of the product of the zero and second persistence landscape norms to first persistence landscape norm after 0−1 normalization. Denoting the ratio as re and the normalized persistence landscape norms as ¯Λn we have re=ˉΛ0ˉΛ2ˉΛ1. We also consider the alternating sum in line with the Euler characteristic, χϵ=ˉΛ0−ˉΛ1+ˉΛ2.
Considering our different measures over the time period chosen we can see some interesting behavior around the crashes and downturns being discussed. Figure 3 shows a summary of the long term behavior of the persistence landscape norms' variations.
In comparison, with the view of days as points we notice that rather than the increase indicating a crash it would seem that the critical values in the variations of the norms is the indicator. There is a rise but also a fall that precedes crashes. The windows highlighted are a year of trading days prior to the date of the crash. The Lehman Brothers collapse is most pronounced in ranks 1 and 2 as well as the trade war and covid related crashes. The decline of 2022 is most apparent in the drop in the rank 0 norm. Knowing that the topology of a manifold is better understood through all the homology groups rather than an individual group we might try to combine this information in the natural ways, the alternating sum of the persistence landscape norms and the ratio of the norms is graphed in Figure 4.
Here we can more clearly see the peaks and declines associated to each crash. There is one major peak unaccounted for in the center of the image but interestingly this leads up to a market correction in 2014 where the market dropped 10 percent and then quickly recovered. Inserting this event and the year prior to it, in Figures 5 and 6, we can more clearly see that the fall in these measures indicating a change in market dynamics that leads to a drop in total market value.
Next, we will investigate the other measures of the persistent homology we have previously defined in an effort to tease out the parts of these persistence landscape norms that are most indicative of the crashes and declines we want to predict as well as being less computationally expensive.
From the previous discussion we can see that we may be able to gain insight about when crashes are about to occur or understand differences in the behavior of crashes based on the geometry of stock movements. Previous candidates for early warning systems have included rising variation in price of an index, rising correlation in prices across an index or rising variation in the persistence landscape norms where the days are viewed as points. When viewing individual stocks as points we see maximums in the persistence landscape norms as an indicator of an upcoming crash or downturn in the market. We also see that combining these norms after normalization as ratios or alternating sums, mimicking the Euler characteristic, we get clearer pictures of when crashes may occur.
We have examined the widths, heights, area and inv for the rank 1 and 2 homology groups. We have found that only the variation of the inv of the rank 1 homology is of interest to pick out all crashes.
Recall that inv=hw and that large height indicates higher persistence while low width indicates that the geometry happening on similar scale. Higher inv indicates stronger evidence for organized geometric behavior. Interestingly we seem to capture the same information here as we did from the analogies to the Euler characteristic. We see that each downturn or crash is preceded by a peak and a drop in the variation of this value defined by basic descriptions of the persistent homology. There are two advantages in using the inv. For one, we only need to compute up to the first homology to view this information, but also the height and width are much less computationally expensive than the persistence landscape norm. In any case, these descriptions of persistent homology, i.e., width, height and persistence landscape norm, give new tools and show promise in helping to predict changes in market behavior.
Volatility or variation in index prices has been shown to be an indicator of market crashes. Our present investigation is part of an expansion of the scope of the term volatility to include not just the variation in the individual prices but also the variation in the geometry of the prices as a group. Previous work by Gidea and Katz showed that variation of the persistence landscape norm in dimension 1 rises prior to some market crashes. However, these results do not generalize to more recent crashes. We include information on a more granular level, considering all stocks in the S&P 500 index rather than using indexes as summaries, and consider persistence in dimensions 0, 1 and 2 rather than just dimension 1. We further suggest two possible ways of condensing the information from multiple dimensions re and χϵ. Each of these measures has a rise and fall behavior prior to three of the four market crashes considered. This is more robust than the methods presented in Gidea and Katz. The definitions of re and χϵ are easily expanded to include higher dimensional information if and when it becomes available. This makes them good candidates for overall summaries as persistence homology in higher dimension is computed in the future.
We also suggest measures that are less computationally expensive than the persistence landscape norm and more closely related to what one can see visually in a persistence diagram, wn, hn and invn. We found that among these measures and their various sums, differences, products and ratios, the most obviously connected to the market crashes was inv1. The inv1 falls from a peak prior to each of the market crashes considered. Surprisingly, this contrasts with the results using the persistence landscape norm, where the most effective measures were those that considered information over multiple dimensions. This also suggests that the rudimentary descriptions wn, hn and invn provide different information than the persistence landscape norm and are worthy of study in their own right.
Deciding what the topology of a data set means about the data set is a hard question that people tend to land on when encountering topological data analysis. It is possible to discuss the meaning of different homology classes as clusters in dimension 0, closed paths around a void in dimension 1 or surfaces that bound a void in dimension 2. Rather than thinking about these individual classes, we have focused on ways of summarizing the information and tracking how these summaries change. In general, we might think of highly structured data having high persistence landscape norm. Then, the variation is linked to the speed of the continual restructuring of that information. In this view, high persistence is linked to a variance in the price of assets, not just individually but also in how those prices are rising and falling in relation to one another. We emphasize that we are not suggesting any causal relationship between the variation and prices only describing objective behavior of the data.
We have shown that there are warning signals of financial crashes that can be extracted through topological data analysis. In particular, inv1 is the most indicative of a coming market crash or downturn. We have also shown that it may be the case that some coarser measures like height and width of persistent diagrams may be more predictive than measures like the persistence landscape norm. We note that measures defined here, including hn, wn, invn, re and χϵ, are not dependent on the application to financial markets and can be used for investigating any time series dataset once persistent homology is applied.
The authors declare they have not used Artificial Intelligence (AI) tools in the creation of this article.
The author declares no conflicts of interest in this paper.
[1] | Bubenik P (2015) Statistical topological data analysis using persistence landscapes. J Mach Learn Res 16: 77–102. |
[2] | Carlsson G (2009) Topology and data. Bull Am Math Soc 46. |
[3] |
Gidea M, Katz Y(2018) Topological data analysis of financial time series: Landscapes of crashes. Physica A 491: 820–834. https://doi.org/10.1016/j.physa.2017.09.028. doi: 10.1016/j.physa.2017.09.028
![]() |
[4] |
Bascompte J, Brock W, Scheffer M, et al. (2009) Early-warning signals for critical transitions. Nature 461: 53–59. https://doi.org/10.1038/nature08227 doi: 10.1038/nature08227
![]() |
[5] |
Guttal V, Raghavendra S, Goel N, et al. (2016) Lack of critical slowing down suggests that financial meltdowns are not critical transitions, yet rising variability could signal systemic risk. PL0S ONE 11: e0144198. https://doi.org/10.1371/journal.pone.0144198 doi: 10.1371/journal.pone.0144198
![]() |
[6] |
Yen PTW, Cheong SA (2021) Using topological data analysis (tda) and persistent homology to analyze the stock markets in Singapore and Taiwan. Front Physics. https://doi.org/10.3389/fphy.2021.572216 doi: 10.3389/fphy.2021.572216
![]() |
[7] |
Antonakakis N, Cunado J, Filis G, et al. (2018) Oil volatility, oil and gas firms and portfolio diversification. Energ Econ 70: 499–515. https://doi.org/10.1016/j.eneco.2018.01.023 doi: 10.1016/j.eneco.2018.01.023
![]() |
[8] |
Edwards S, Biscarri JG, De Gracia FP (2003) Stock market cycles, financial liberalization and volatility. J Int Money Financ 22: 925–955. https://doi.org/10.1016/j.jimonfin.2003.09.011 doi: 10.1016/j.jimonfin.2003.09.011
![]() |
[9] |
Hsieh DA (1995) Nonlinear Dynamics in Financial Markets: Evidence and Implications. Financ Analy J 51: 55–62. https://doi.org/10.2469/faj.v51.n4.1921 doi: 10.2469/faj.v51.n4.1921
![]() |