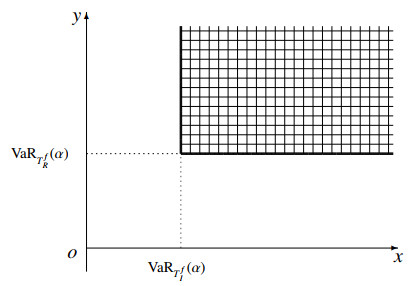
Citation: Thomas Papikinos, Marios Krokidis, Aris Vrahatis, Panagiotis Vlamos, Themis P. Exarchos. Drug repurposing for obsessive-compulsive disorder using deep learning-based binding affinity prediction models[J]. AIMS Neuroscience, 2024, 11(2): 203-211. doi: 10.3934/Neuroscience.2024013
[1] | Weam G. Alharbi, Abdullah F. Shater, Abdelhalim Ebaid, Carlo Cattani, Mounirah Areshi, Mohammed M. Jalal, Mohammed K. Alharbi . Communicable disease model in view of fractional calculus. AIMS Mathematics, 2023, 8(5): 10033-10048. doi: 10.3934/math.2023508 |
[2] | Ateq Alsaadi . Advancing water quality management: A synergistic approach using fractional differential equations and neural networks. AIMS Mathematics, 2025, 10(3): 5332-5352. doi: 10.3934/math.2025246 |
[3] | Jagdev Singh, Jitendra Kumar, Devendra Kumar, Dumitru Baleanu . A reliable numerical algorithm for fractional Lienard equation arising in oscillating circuits. AIMS Mathematics, 2024, 9(7): 19557-19568. doi: 10.3934/math.2024954 |
[4] | Mohammad Sajid, Biplab Dhar, Ahmed S. Almohaimeed . Differential order analysis and sensitivity analysis of a CoVID-19 infection system with memory effect. AIMS Mathematics, 2022, 7(12): 20594-20614. doi: 10.3934/math.20221129 |
[5] | Ahu Ercan . Comparative analysis for fractional nonlinear Sturm-Liouville equations with singular and non-singular kernels. AIMS Mathematics, 2022, 7(7): 13325-13343. doi: 10.3934/math.2022736 |
[6] | Maysaa Al-Qurashi, Saima Rashid, Fahd Jarad, Madeeha Tahir, Abdullah M. Alsharif . New computations for the two-mode version of the fractional Zakharov-Kuznetsov model in plasma fluid by means of the Shehu decomposition method. AIMS Mathematics, 2022, 7(2): 2044-2060. doi: 10.3934/math.2022117 |
[7] | Maysaa Al Qurashi, Saima Rashid, Sobia Sultana, Fahd Jarad, Abdullah M. Alsharif . Fractional-order partial differential equations describing propagation of shallow water waves depending on power and Mittag-Leffler memory. AIMS Mathematics, 2022, 7(7): 12587-12619. doi: 10.3934/math.2022697 |
[8] | M. Mossa Al-Sawalha, Khalil Hadi Hakami, Mohammad Alqudah, Qasem M. Tawhari, Hussain Gissy . Novel Laplace-integrated least square methods for solving the fractional nonlinear damped Burgers' equation. AIMS Mathematics, 2025, 10(3): 7099-7126. doi: 10.3934/math.2025324 |
[9] | Rahat Zarin, Abdur Raouf, Amir Khan, Aeshah A. Raezah, Usa Wannasingha Humphries . Computational modeling of financial crime population dynamics under different fractional operators. AIMS Mathematics, 2023, 8(9): 20755-20789. doi: 10.3934/math.20231058 |
[10] | Peijiang Liu, Taj Munir, Ting Cui, Anwarud Din, Peng Wu . Mathematical assessment of the dynamics of the tobacco smoking model: An application of fractional theory. AIMS Mathematics, 2022, 7(4): 7143-7165. doi: 10.3934/math.2022398 |
Reinsurance is an effective risk management tool for an insurer to mitigate the underwriting risk by transferring part of the risk exposure to a reinsurer. Starting from [1,2], the study of optimal reinsurance has remained a fascinating topic in actuarial science. Most existing literatures on optimal reinsurance are from an insurer's point of view. For example, by maximizing the expected concave utility function of an insurer's wealth, Arrow [3] showed that optimal reinsurance for an insurer is a stop-loss reinsurance. The result has been extended to different settings (see, e.g., [4,5] and references therein). It is well known that the optimal reinsurance for an insurer, which minimizes the variance of the insurer's loss, is also a stop-loss reinsurance (see [6]). However, Vajda [7] showed that the optimal reinsurance for a reinsurer, which minimizes the variance of the reinsurer's loss with a fixed net reinsurance premium, is a quota-share reinsurance among a class of ceded loss functions that include stop-loss reinsurance. Kaluszka and Okolewski [8] showed that if an insurer wants to maximize his expected utility with the maximal possible claim premium principle, the optimal form of reinsurance for the insurer is a limited stop-loss reinsurance. In recent years, Cai et al. [9,10] introduced two classes of optimal reinsurance models by minimizing the value-at-risk (VaR) and the conditional tail expectation (CTE) of the insurer's total risk exposure. Cai et al. [10] proved that depending on the risk measure level of confidence, the optimal reinsurance for an insurer, which minimizes the VaR and CTE of the total risk of the insurer, can be in the form of a stop-loss reinsurance or a quota-share reinsurance or a change-loss reinsurance under the expected value principle and among the increasing convex ceded loss functions. Recent references on VaR-minimization and CTE-minimization reinsurance models can be found in [11,12,13,14,15,16,17] and references therein.
However, a reinsurance contract involves two parties, an insurer and a reinsurer. The two parties have conflicting interests. An optimal reinsurance contract for an insurer may not be optimal for a reinsurer and it might be unacceptable for a reinsurer as pointed out by Borch [18]. Therefore, an interesting question about optimal reinsurance is to design a reinsurance contract so that it considers the interests of both an insurer and a reinsurer. Borch [1] first discussed the optimal quota-share retention and stop-loss retention that maximize the product of the expected utility functions of the two parties' wealth. Cai et al. derived the optimal reinsurance contracts that maximize the joint survival probability and joint profitable probability of the two parties, and gave the sufficient conditions for optimal reinsurance contracts within a wide class of reinsurance policies and under a general reinsurance premium principle, see [19,20]. Cai et al. [21] studied the optimal reinsurance strategy, which based on the minimum convex combination of the VaR of the insurer and the reinsurer under two types of constraints. Lo [22] discussed the generalized problems of [21] by using the Neyman-Pearson approach. Based on the optimal reinsurance strategy of [21], Jiang et al. [23] proved that the optimal reinsurance strategy is a Pareto-optimal reinsurance policy and gave optimal reinsurance strategies using the geometric method. Cai et al. [24] studied Pareto optimality of reinsurance arrangements under general model settings and obtained the explicit forms of the Pareto-optimal reinsurance contracts under TVaR risk measure and the expected value premium principle. By geometric approach, Fang et al. [25] studied Pareto-optimal reinsurance policies under general premium principles and gave the explicit parameters of the optimal ceded loss functions under Dutch premium principle and Wang's premium principle. Lo and Tang [26] characterized the set of Pareto-optimal reinsurance policies analytically and visualized the insurer-reinsurer trade-off structure geometrically. Huang and Yin [27] studied two classes of optimal reinsurance models from perspectives of both insurers and reinsurers by minimizing their convex combination where the risk is measured by a distortion risk measure and the premium is given by a distortion premium principle.
In this paper, we study the optimal reinsurance models by minimizing the insurer and the reinsurer's total costs under the criteria of loss function assuming that the reinsurance premium principles satisfy risk loading and stop-loss ordering preserving. The loss function is defined by the joint VaR based on the binary lower-orthant value-at-risk and the binary upper-orthant value-at-risk, which are proposed by Embrechts and Puccetti [28]. Methodologically, we determine the optimal reinsurance forms using the geometric approach of [11] over three ceded loss function sets, the class of increasing convex ceded loss functions, the class of ceded loss functions which satisfy both ceded and retained loss functions are increasing and the class of increasing concave ceded loss functions1*.
∗1Throughout this paper, the terms "increasing function" and "decreasing function" mean "non-decreasing function" and "non-increasing function", respectively.
The rest of the paper is organized as follows. In Section 2, we give definitions and propose an optimal reinsurance problem that takes into consideration the interests of both an insurer and a reinsurer. In Section 3, we derive optimal reinsurance forms over three ceded loss function sets by the geometric approach of [11], assuming that the reinsurance premium principles satisfy risk loading and stop-loss ordering preserving. In Section 4 and Section 5, we determine the corresponding optimal parameters under expectation premium principle and Dutch premium principle respectively. In Section 6, we provide four numerical examples. Conclusions are given in Section 7.
Let X be the loss or claim initially assumed by an insurer in a fixed time period. We assumed that X is a nonnegative random variable with distribution function F(x)=P{X≤x}, survival function S(x)=P{X≥x} and mean μ=E(X) (0<μ<∞). Under a reinsurance contract, a reinsurer will cover the part of the loss, say f(X) with 0≤f(X)≤X, and the insurer will retain the rest of the loss, which is denoted by If(X)=X−f(X). The losses If(X) and f(X) are called retained loss and ceded loss, respectively. Since the reinsurer shares the risk X, the insurer will pay an additional cost in the form of reinsurance premium to the reinsurer. We denote the reinsurance premium by Πf(X) which corresponds to a ceded loss function f(X). The total cost TfI of the insurer is composed of two components: the retained loss If(X) and the reinsurance premium Πf(X), that is
TfI=If(X)+Πf(X), | (2.1) |
and the total cost of the reinsurer is
TfR=f(X). | (2.2) |
For individual company, an important issue is to determine their maximum agammaegate loss which can occur with some given probability, value-at-risk (VaR) serves this purpose.
Definition 2.1. For 0<α<1, the VaR of a non-negative random variable X with distribution function F(x)=P{X≤x} at confidence level α is defined as
VaRX(α)=inf{x∈R:F(x)≥α}=F−1(α), | (2.3) |
where, F−1 is the generalized inverse function of the distribution function F(x).
The VaR defined by (2.3) is the maximum loss which is not exceeded at a given probability α. We list several properties of the VaR or the generalized inverse function F−1.
Proposition 2.1. For any α∈(0,1) and any nonnegative random variable X with distribution function F(x), the following properties hold:
(1) F(F−1(α))≥α.
(2) F−1(F(x))≤x for x≥0.
(3) If h is an increasing and left-continuous function, then VaRh(X)(α)=h(VaRX(α)).
Proof. Properties (1) and (2) follow immediately from Lemma 2.13 of [29] and the definition of the generalized inverse function, while for property (3), see the proof of Theorem 1 in [30].
In this paper, we assume that the initial loss X has a continuous and strictly increasing distribution function on (0,∞) with a possible mass at 0 and α∈(F(0),1) to avoid trivial cases, then
F(F−1(α))=α. | (2.4) |
For the insurer or the reinsurer, they can use Definition 2.1 to determine their maximum agammaegate cost which can occur with some given probability α. However, if the insurer and the reinsurer are considered as partners, then the total cost Tf is a two-dimensional random vector (TfI,TfR). For this case, Definition 2.1 does not make sense since, even for a one to one continuous distribution function, there are possibly infinitely many vectors (x,y)∈[0,∞)×[0,∞) at which Gf(x,y)=α, where
Gf(x,y)=P{TfI≤x, TfR≤y} |
is the distribution function of (TfI,TfR). Hence we use the definition of multivariate Value-at-Risk which is proposed by Embrechts and Puccetti (see [28]).
Definition 2.2. For α∈(0,1), the binary lower-orthant value-at-risk at confidence level α for the distribution function Gf(x,y) is the boundary of its α-level set, defined as
VaR_f(α):=∂{(x,y)∈R2+:Gf(x,y)≥α}. |
Analogously, the binary upper-orthant value-at-risk at confidence level α for the tail function ¯Gf(x,y) is defined as
¯VaRf(α):=∂{(x,y)∈R2+:¯Gf(x,y)≤1−α}, |
where
¯Gf(x,y)=P{TfI>x, TfR>y}. |
We now provide further analysis on the binary lower-orthant value-at-risk at confidence level α for the distribution function Gf(x,y) and the binary upper-orthant value-at-risk at confidence level α for the tail function ¯Gf(x,y) over the following three admissible sets of ceded loss functions:
F1≜{0⩽f(x)⩽x:f(x) is an increasing convex function }, | (2.5) |
F2≜{0⩽f(x)⩽x: both If(x) and f(x) are increasing functions }, | (2.6) |
F3≜{0⩽f(x)⩽x:f(x) is an increasing concave function }. | (2.7) |
In the set F2, the increasing condition on both ceded and retained loss functions is interesting and important. Both the insurer and the reinsurer are obligated to pay more for larger loss X, hence it potentially reduces moral hazard. In addition, in a reinsurance contract, sometimes in order to better protect the insurer, they let the loss proportion paid by the reinsurer increases in the loss (see [7]). Mathematically, f(x)/x is assumed to be an increasing function. If we assume that f(x) is increasing and convex, then f(x)/x is an increasing function. On the other hand, under the reinsurance policies with no upper limit on the indemnity, the reinsurance may be under a heavy financial burden, especially when the insurer suffers a large unexpected loss. Therefore, reinsurance contracts sometimes involve an upper limit on the indemnity in practice. In such a situation, ceded loss functions must not be convex functions but concave functions sometimes. Motivated by these observations, we consider ceded loss functions in the sets F1 and F3.
Note that F1⊂F2 (see [12]) and F3⊂F2. In addition, if f∈Fi,i=1,2,3, then If and f are increasing and continuous. Thus, from Proposition 1, we have
VaRTfI(α)=If(VaRX(α))+Πf(X), | (2.8) |
VaRTfR(α)=f(VaRX(α)). | (2.9) |
Based on the above analysis, we obtain the following theorem.
Theorem 2.1. For α∈(0,1), the binary lower-orthant value-at-risk at confidence level α for the distribution function Gf(x,y) is
VaR_f(α)=∂{(x,y)∈R2+:x≥VaRTfI(α) and y≥VaRTfR(α)}, |
and the binary upper-orthant value-at-risk at confidence level α for the tail function ¯Gf(x,y) is
¯VaRf(α)=∂{(x,y)∈R2+:x≥VaRTfI(α) or y≥VaRTfR(α)}. |
Proof. Let S1={(x,y)∈R2+:Gf(x,y)≥α} and S2={(x,y)∈R2+:x≥VaRTfI(α) and y≥VaRTfR(α)}. First, it is easy to see that S1⊆S2. Second, note that
Gf(VaRTfI(α),VaRTfR(α))=P{TfI≤VaRTfI(α), TfR≤VaRTfR(α)}=P{If(X)≤If(VaRX(α)), f(X)≤f(VaRX(α)}≥P{X≤VaRX(α)}=α, |
then for any (x,y)∈S2, we have Gf(x,y)≥Gf(VaRTfI(α),VaRTfR(α))≥α, thus we get S2⊆S1.
Similarly, let D1={(x,y)∈R2+:¯Gf(x,y)≤1−α} and D2={(x,y)∈R2+:x≥VaRTfI(α) or y≥VaRTfR(α)}. For any (x,y)∈D2, if x≥VaRTfI(α), then
¯Gf(x,y)=P{TfI>x, TfR>y}≤P{TfI>x}≤P{TfI>VaRTfI(α)}≤1−α. | (2.10) |
By the same arguments, we know that if y≥VaRTfR(α), then ¯Gf(x,y)≤1−α holds as well. Hence, D2⊆D1.
On the other hand, for any (x,y)∈¯D2, we have x<VaRTfI(α) and y<VaRTfR(α). Since TfI and TfR are co-monotonic, we have
¯Gf(x,y)=P{TfI>x, TfR>y}=min{P{TfI>x},P{TfR>y}}. | (2.11) |
Notice that for any random variable Y, if y<VaRY(α), we get P{Y≤y}<α. (Otherwise, suppose P{Y≤y}≥α, then from the definition of VaR, we get y≥VaRY(α).) Then, we have
P{TfI>x}>1−α and P{TfR>y}>1−α | (2.12) |
which implies ¯Gf(x,y)>1−α. Therefore, we have ¯D2⊆¯D1, and hence D2=D1.
The binary lower-orthant value-at-risk VaR_f(α) and the binary upper-orthant value-at-risk ¯VaRf(α) are illustrated in Figures 1 and 2.
Note that the joint VaR (VaRTfI(α),VaRTfR(α)) determines VaR_fα and ¯VaRfα. From both the insurer and the reinsurer's point of view, maximum agammaegate cost Tf which can occur with some given probability is the smaller the better, that is to say, VaR_fα and ¯VaRfα are closer to the origin the better. This motivates us to consider the loss function
L(f)=√[VaRTfI(α)]2+[VaRTfR(α)]2, | (2.13) |
and the optimization criteria for seeking the optimal reinsurance contract:
f∗=argminf L(f). | (2.14) |
In the rest of the paper, we will derive the optimal solutions corresponding to the reinsurance model (2.14) under the admissible ceded loss function sets Fi,i=1,2,3.
In this section, we consider the general reinsurance premium principles which satisfy the following two properties:
1. Risk loading: Π(X)≥E[X];
2. Stop-loss ordering preserving: Π(Y)≤Π(X) if Y is smaller than X in the stop-loss order (Y≤slX)2†.
†2 A random variable Y is said to be smaller than a random variable X in the stop-loss order sense, notation Y≤slX, if and only if Y has lower stop-loss premiums than X: E(Y−d)+≤E(X−d)+, −∞<d<+∞.
We emphasize that there are many premium principles which satisfy these two properties, such as expectation principle, p−mean value principle, Dutch principle, Wang's principle and exponential principle.
In this subsection, we derive the optimal reinsurance policies under the condition that the ceded loss function f∈F1. First, we define a ceded loss function set H1, which consists of all ceded loss functions h(x)=b(x−d)+ with 0≤b≤1 and d≥0. Note that H1 is a subclass of F1. Second, we show that the optimal ceded loss functions which minimize the loss function in the subclass H1 also optimally minimize the loss function in F1. We give the following proposition using the geometric method proposed by [11].
Proposition 3.1. For any f∈F1, there always exists a function h∈H1 such that L(h)≤L(f).
Proof. If f∈F1 is identically zero on [0,VaRX(α)], we consider h:=0∈H1. It is easy to see that h(X)≤f(X) in the usual stochastic order. It further leads to h(X)≤slf(X) according to the theory of stochastic orders in [31]. Then we have Πh(X)≤Πf(X). Consequently, from formulas (2.8) and (2.9), we obtain
VaRTfI(α)=VaRX(α)−f(VaRX(α))+Πf(X)≥VaRX(α)−h(VaRX(α))+Πh(X)=VaRThI(α), |
and
VaRTfR(α)=f(VaRX(α))=0=h(VaRX(α))=VaRThR(α). |
Hence we have L(h)≤L(f).
If f∈F1 is not identically zero on [0,VaRX(α)], let f′−(VaRX(α)) and f′+(VaRX(α)) be the left-hand derivative and right-hand derivative of f at VaRX(α). Let b be an any number in [f′−(VaRX(α)),f′+(VaRX(α))], then we have 0<b≤1. Let d=VaRX(α)−f(VaRX(α))b, define h(x)=b(x−d)+,x≥0. Then h∈H1, f(VaRX(α))=h(VaRX(α)) and f≥h for any x≥0 since f is convex. Hence we have
VaRTfI(α)=VaRX(α)−f(VaRX(α))+Πf(X)≥VaRX(α)−h(VaRX(α))+Πh(X)=VaRThI(α), |
and
VaRTfR(α)=f(VaRX(α))=h(VaRX(α))=VaRThR(α). |
Therefore, L(h)≤L(f) holds. The geometric interpretation of this proof can be seen from Figure 3.
Based on the Proposition 3.1, we know that change-loss reinsurance of the form f(x)=b(x−d)+ with 0≤b≤1 and d≥0 is optimal among F1 in the sense that it minimizes the loss function L(f). The optimal parameters b∗ and d∗ will be given under some specific reinsurance premium principles in the rest of sections.
In this subsection, we focus on the loss function minimization model for any ceded loss function f∈F2. As shown in [12], the ceded loss function f∈F2 is Lipschitz continuous, i.e.,
0⩽f(x2)−f(x1)⩽x2−x1,∀0⩽x1⩽x2. |
Let H2 denote the class of ceded loss function with the following representation h(x)=(x−a)+−(x−VaRX(α))+, a⩽VaRα(X). It is easy to see that H2 is a subclass of F2. Exactly, h(x) is a layer reinsurance with deductible a and upper limit VaRX(α). We will prove that the optimal functions which minimize the loss function in the subclass H2 also optimally minimize the loss function in F2.
Proposition 3.2. Let f∈F2 be a ceded function. There always exists a function h∈H2 such that L(h)⩽L(f).
Proof. For any f∈F2, define a=VaRX(α)−f(VaRX(α))⩾0 and h(x)=(x−a)+−(x−VaRX(α))+=min{(x−(VaRX(α)−f(VaRX(α))))+,f(VaRX(α)}, x⩾0. Then we have h∈H2 and f(VaRX(α))=h(VaRX(α)).
Furthermore, recall that the ceded loss function f∈F2 is non-negative and Lipschitz continuous, hence inequality f(x)⩾(x+f(VaRX(α))−VaRX(α))+ holds for x∈[0,VaRX(α)]. On the other hand, the increasing property of f(x) leads to h(x)=f(VaRX(α))⩽f(x) for all x>VaRX(α). Thus, inequality h(x)⩽f(x) holds for all x⩾0. Since the reinsurance premium preserves stop-loss order, we have
VaRTfI(α)=VaRX(α)−f(VaRX(α))+Πf(X)≥VaRX(α)−h(VaRX(α))+Πh(X)=VaRThI(α), |
and
VaRTfR(α)=f(VaRX(α))=h(VaRX(α))=VaRThR(α). |
Thus, L(h)≤L(f) holds. The geometric interpretation of this proof can be seen from Figure 4.
By Proposition 3.2, we know that layer reinsurance with deductible a and upper limit VaRX(α) is optimal among F2 in the sense that it minimizes the loss function L(f).
In this subsection, we derive the optimal solution to problem of (2.14) over F3. Let H3 be the class of non-negative function h(x) defined on [0,∞] with
h(x)=c(x−(x−VaRX(α))+), | (3.1) |
where 0⩽c⩽1. Note that H3⊂F3 and H3 contains the null function h(x)=0. The following result shows that the optimal ceded loss functions in F3 which minimize the L(f), must take the form of (3.1).
Proposition 3.3. For any f∈F3, there always exists a function h∈H3, such that L(h)⩽L(f).
Proof. For any f∈F3, let c=f(VaRX(α))VaRX(α), then c∈[0,1]. Define h(x)=c(x−(x−VaRX(α))+), obviously h∈H3 and h(VaRX(α))=f(VaRX(α)).
In addition, recall that the ceded loss function f∈F3 is increasing concave, hence f(x)⩾f(VaRX(α))VaRX(α)x=h(x) for x∈[0,VaRX(α)]. On the other hand, the increasing property of f(x) leads to h(x)=f(VaRX(α))⩽f(x) for x>VaRX(α). Since the reinsurance premium preserves stop-loss order, we have
VaRTfI(α)=VaRX(α)−f(VaRX(α))+Πf(X)≥VaRX(α)−h(VaRX(α))+Πh(X)=VaRThI(α), |
and
VaRTfR(α)=f(VaRX(α))=h(VaRX(α))=VaRThR(α). |
Thus, we have L(h)⩽L(f). The geometric interpretation of this proof can be seen from Figure 5.
From Proposition 3.3, we know that the quota-share reinsurance with a policy limit is always optimal among F3 in the sense that it minimizes the loss function L(f).
In this section, we consider the expectation reinsurance premium principle, i.e.,
Πf(X)=(1+θ)E[f(x)], | (4.1) |
where, θ>0 is the safety loading.
As a result of Proposition 3.1, we can deduce optimal ceded loss functions by confining attention to H1. For a change-loss reinsurance with b∈[0,1] and d∈[0,∞), the total costs of the insurer and the reinsurer are
Tb,dI=X−b(X−d)++ΠE(b,d), |
Tb,dR=b(X−d)+, |
where, ΠE(b,d)=(1+θ)E[b(X−d)+]=(1+θ)b∫∞dS(x)dx is the reinsurance premium. Then the VaR of Tb,dI and Tb,dR at confidence level α are
VaRTb,dI(α)=VaRX(α)−b(VaRX(α)−d)++ΠE(b,d), | (4.2) |
VaRTb,dR(α)=b(VaRX(α)−d)+. | (4.3) |
Hence, the loss function is
LE(b,d)={√[(1−b)VaRX(α)+bd+ΠE(b,d)]2+[b(VaRX(α)−d)]2,d≤VaRX(α),VaRX(α)+ΠE(b,d),d>VaRX(α). | (4.4) |
Lemma 4.1. Optimal ceded functions which minimize the loss function LE(b,d) in the class H1 exist.
Proof. Note that the function ΠE(b,d) is an increasing function with respect to b. Then the loss function LE(b,d) attains its minimum value over [0,1]×(VaRX(α),∞) at b=0 (the ceded function is h(x)≡0) and the minimum value is VaRX(α). Hence, the study of optimal ceded functions which minimize the loss function LE(b,d) in the class H1 is simplified to solving the two-parameter minimization problem over closed subset [0,1]×[0,VaRX(α)]. Since LE(b,d) is continuous, then the minimum of LE(b,d) over [0,1]×[0,VaRX(α)] must attain at some stationary point or lie on the boundary.
First, we define A≜[0,1]×[0,VaRX(α)]. In this subsection, we will identify the minimum points of LE(b,d) over A and discuss the optimal ceded function f∗1(x). We split A into five disjoint subsets, i.e. A=⋃5i=1Ai, where, A1={(0,d):0≤d≤VaRX(α)}, A2={(b,d):0<b<1,0<d<VaRX(α)}, A3={(1,d):0<d<VaRX(α)}, A4={(b,0):0<b⩽1} and A5={(b,VaRX(α)):0<b≤1}.
If (b,d)∈A, the loss function is
LE(b,d)=√[(1−b)VaRX(α)+bd+ΠE(b,d)]2+[b(VaRX(α)−d)]2. | (4.5) |
Let HE(b,d)=L2E(b,d)=[(1−b)VaRX(α)+bd+ΠE(b,d)]2+[b(VaRX(α)−d)]2, then HE(b,d) and LE(b,d) have the same minimum points. Thus, we will study the minimization problem of HE(b,d) on A in the rest of this subsection. Note that HE(b,d) is differentiable with partial derivatives
{∂HE(b,d)∂b=2[(VaRX(α)−g(d))2+(VaRX(α)−d)2]b+2VaRX(α)(g(d)−VaRX(α)),∂HE(b,d)∂d=2b[(1−b)VaRX(α)+bg(d)]g′(d)−2b2(VaRX(α)−d), | (4.6) |
where, g(d)=d+(1+θ)∫∞dS(x)dx.
Next, we divide the following analysis into five cases.
● First, we demonstrate that HE(b,d) has no minimum points on A5. For any (b,d)∈A5, HE(b,d)>[VaRX(α)]2=HE(0,d)=minA1HE(b,d), then the minimum value of HE(b,d) over A is not attainable in A5.
● The minimum points of HE(b,d) are located in A1 if and only if
min[0,VaRX(α)]g(d)≥VaRX(α). | (4.7) |
In fact, if inequality (4.7) holds, then it follows from the expression of ∂HE(b,d)∂b in (4.6) that ∂HE(b,d)∂b>0. Thus, HE(b,d) is strictly increasing with respect to b. Furthermore, for any d∈[0,VaRX(α)], HE(0,d)≡[VaRX(α)]2. As a result, the minimum value of HE(b,d) over A is attained at any point (0,d) in A1.
Conversely, if min[0,VaRX(α)]g(d)<VaRX(α), then there exists a ˜d∈[0,VaRX(α)] such that ∂HE(b,˜d)∂b<0 holds in a right neighborhood of b=0. That is to say, (0,˜d) is not a minimum point of HE(b,d). Since HE(0,d)=HE(0,˜d)≡[VaRX(α)]2 for any (0,d)∈A1, then no minimum points of HE(b,d) are located in A1.
● If (b∗,d∗)∈A2 is a minimum point of HE(b,d), then (b∗,d∗) is a stationary point of HE(b,d). Therefore, we have
{∂HE(b,d)∂b|(b,d)=(b∗,d∗)=0,∂HE(b,d)∂d|(b,d)=(b∗,d∗)=0. | (4.8) |
By straightforward algebra, we know that d∗ is a root of equation q(d)=0, where
q(d)=S(d)(VaRX(α)−d)−∫∞dS(x)dx. | (4.9) |
Substituting d∗ in the second equation of (4.8) yields
b∗=VaRX(α)g′(d∗)VaRX(α)−d∗+[VaRX(α)−g(d∗)]g′(d∗). | (4.10) |
Furthermore, b∗ must lie in (0,1), which is equivalent to
p(d∗)>0, | (4.11) |
where, the function p(d) is given by p(d)=VaRX(α)−d−g(d)g′(d).
● If (1,ˉd)∈A3 is a minimum point of HE(b,d), then Fermat's theorem implies
{∂HE(1,d)∂d|d=ˉd=0,∂HE(b,ˉd)∂b|b=1≤0, | (4.12) |
which is equivalent to
{p(ˉd)=0,g(ˉd)[g(ˉd)−VaRX(α)]+[VaRX(α)−ˉd]2≤0. | (4.13) |
● If (ˉb,0)∈A4 is a minimum point of HE(b,d), then ˉb must satisfy the following conditions
{∂HE(b,0)∂b|b=ˉb=0,∂HE(ˉb,d)∂d|d=0≥0. | (4.14) |
From (4.14), we yield
ˉb=VaRX(α)[VaRX(α)−g(0)][VaRX(α)−g(0)]2+[VaRX(α)]2 | (4.15) |
and
[(1−ˉb)VaRX(α)+ˉbg(0)]g′(0)−ˉbVaRX(α)≥0. | (4.16) |
Based on the above arguments, we analyze the conditions for the minimum points of HE(b,d) are located in sets Ai, i=1,2,3,4. The results are summarized in the following theorem.
Theorem 4.1. The optimal solutions to reinsurance problem (2.14) are given as follows.
(1) If one of the three conditions (C1)-(C3) holds, then the optimal ceded loss function is given by f∗1(x)=0, where, d0=S−1(11+θ) and
(C1):α≤θ1+θ,(C2):{F(0)<θ1+θ<α,g(d0)≥VaRX(α),(C3):{S(0)≤11+θ,(1+θ)μ≥VaRX(α). |
(2) If condition (C4) or (C5) holds, then the optimal ceded loss function is given by f∗1(x)=b∗(x−d∗)+, where d∗ is the unique solution of equation q(d)=0, b∗ is given by (4.10) and
(C4):{F(0)<θ1+θ<α,g(d0)<VaRX(α),p(d∗)>0,(C5):{S(0)≤11+θ,μ<S(0)VaRX(α),p(d∗)>0. |
(3) If condition (C6) or (C7) holds, then the optimal ceded loss function is given by f∗1(x)=(x−ˉd)+, where ˉd is the unique solution of equation p(d)=0 and
(C6):{F(0)<θ1+θ<α,g(d0)<VaRX(α),p(d∗)≤0,(C7):{S(0)≤11+θ,μ<S(0)VaRX(α),p(d∗)≤0. |
(4)If condition (C8) holds, then the optimal ceded loss function is given by f∗1(x)=ˉbx, where ˉb is given by (4.15) and
(C8):{S(0)≤11+θ,S(0)VaRX(α)≤μ<11+θVaRX(α). |
Proof. (1) If one of the three conditions (C1)–(C3) holds, it is easy to show that
min[0,VaRX(α)]g(d)≥VaRX(α). |
Then the minimum points of HE(b,d) are located in A1. That is to say, the optimal ceded loss function is f∗1(x)=0.
(2) If condition (C4) holds, then g′(d)<0 for any d∈[0,d0). From the expression of ∂HE(b,d)∂d in (4.6), we have ∂HE(b,d)∂d<0 for any (b,d)∈(0,1]×[0,d0]. Thus, the minimum points are not located in [0,1]×[0,d0]. Furthermore, let d1>d0 such that g(d1)=VaRX(α), from the expression of ∂HE(b,d)∂b in (4.6), we have ∂HE(b,d)∂b>0 for any (b,d)∈(0,1]×[d1,VaRX(α)]. Thus, the minimum points are also not located in [0,1]×[d1,VaRX(α)]. As a result, the minimum points of HE(b,d) over A are located in (0,1]×(d0,d1) and the minimum must be attainable at some stationary point (b∗,d∗) or must lie on the right boundary at some point (1,ˉd). Note that q′(d)=S′(d)(VaRX(α)−d)<0, q(d0)=S(d0)(VaRX(α)−d0)−∫∞d0S(x)dx=11+θ(VaRX(α)−g(d0))>0 and q(d1)=S(d1)(VaRX(α)−d1)−∫∞d1S(x)dx=[(1+θ)S(d1)−1]∫∞d1S(x)dx<0. Thus, the equation q(d)=0 has a unique solution d∗ in (d0,d1). Substituting d∗ in the second equation of (4.8) yields
b∗=VaRX(α)g′(d∗)VaRX(α)−d∗+[VaRX(α)−g(d∗)]g′(d∗). |
It is easy to show 0<b∗<1 since p(d∗)>0. Thus HE(b,d) has a unique stationary point (b∗,d∗). In the following, we show that HE(b,d) attains the minimum at the stationary point (b∗,d∗).
Conversely, we suppose that the minimum of HE(b,d) is attainable at (1,ˉd) if condition (C4) holds. Then we have p(ˉd)=0 and g(ˉd)[g(ˉd)−VaRX(α)]+[VaRX(α)−ˉd]2≤0. Since g′(ˉd)>0, we yield [g(ˉd)[g(ˉd)−VaRX(α)]+[VaRX(α)−ˉd]2]g′(ˉd)≤0. Straightforward algebra leads to q(ˉd)≥0. Note that q′(d)<0 and q(d∗)=0, then we have ˉd≤d∗. However, since p′(d)<0, p(d∗)>0 and p(ˉd)=0, then we have d∗<ˉd. This leads to contradictions. Thus, if condition (C4) holds, the function HE(b,d) attains the minimum at the stationary point (b∗,d∗), that is to say, the optimal ceded loss function is f∗1(x)=b∗(x−d∗)+.
If condition (C5) holds, then ∂HE(b,d)∂b>0 for any (b,d)∈(0,1]×[d1,VaRX(α)]. Thus, the minimum points are not located in [0,1]×[d1,VaRX(α)]. As a result, the minimum points of HE(b,d) over A are located in (0,1]×[0,d1) and the minimum must be attainable at some stationary point (b∗,d∗) or must lie on the right boundary at some point (1,ˉd) or must lie on the lower boundary at some point (ˉb,0). In the following, we consider equation q(d)=0. Note that q′(d)<0, q(0)=S(0)VaRX(α)−μ>0 and
q(d1)=S(d1)(VaRX(α)−d1)−∫∞d1S(x)dx=S(d1)(g(d1)−d1)−∫∞d1S(x)dx=S(d1)(1+θ)∫∞d1S(x)dx−∫∞d1S(x)dx=[S(d1)(1+θ)−1]∫∞d1S(x)dx<0. | (4.17) |
Thus, the equation q(d)=0 has a unique solution d∗ in (0,d1). Further, we know that HE(b,d) has a unique stationary point (b∗,d∗) if condition (C5) holds. By the same argument as above, we show that the minimum of HE(b,d) is not attainable at (1,ˉd) if p(d∗)>0 holds. Meanwhile, we demonstrate that the minimum of HE(b,d) is not attainable at (ˉb,0) if condition (C5) holds. Conversely, we suppose that the minimum value of HE(b,d) is attainable at (ˉb,0) if condition (C5) holds. Then we have conditions (4.15) and (4.16) hold. Substituting (4.15) into (4.16), we get μ−S(0)VaRX(α)≥0, that is contradicted to the second inequality of condition (C5). Thus, if condition (C5) holds, the function HE(b,d) attains the minimum at the stationary point (b∗,d∗).
In summary, if condition (C4) or (C5) holds, the optimal ceded loss function is given by f∗1(x)=b∗(x−d∗)+.
(3) If condition (C6) or (C7) holds, from the above arguments in (2), we know that HE(b,d) has no stationary points because p(d∗)≤0. Furthermore, if the second inequality of (C7) holds, the minimum value of HE(b,d) is not attainable at (ˉb,0). Thus, the function HE(b,d) attains the minimum at the boundary point (1,ˉd) if condition (C6) or (C7) holds, that is to say, the optimal ceded loss function is given by f∗1(x)=(x−ˉd)+.
(4) If condition(C8) holds, then ∂HE(b,d)∂b>0 for any (b,d)∈(0,1]×[d1,VaRX(α)]. Thus, the minimum points are not located in [0,1]×[d1,VaRX(α)]. As a result, the minimum points of HE(b,d) over A are located in (0,1]×[0,d1) and the minimum must be attainable at some stationary point (b∗,d∗) or must lie on the right boundary at some point (1,ˉd) or must lie on the lower boundary at some point (ˉb,0). In the following, we consider equation q(d)=0. Note that q(0)=S(0)VaRX(α)−μ≤0 and q′(d)<0, then the equation q(d)=0 has no solutions in (0,d1), namely, the function HE(b,d) has no stationary points. Thus, the minimum point of HE(b,d) over A must lie on the right boundary at some point (1,ˉd) or must lie on the lower boundary at some point (ˉb,0). If the minimum of HE(b,d) is attainable at (1,ˉd), the we have conditions (4.13) hold. Since g′(ˉd)>0, we yield [g(ˉd)[g(ˉd)−VaRX(α)]+[VaRX(α)−ˉd]2]g′(ˉd)≤0. Straightforward algebra leads to q(ˉd)≥0. This is contradicted to q(0)≤0 and q′(d)<0. Thus, minimum point of HE(b,d) over A must lie on the lower boundary at point (ˉb,0), namely, the optimal ceded loss function is given by f∗1(x)=ˉbx.
As a result of Proposition 3.2, we can deduce optimal ceded loss functions by confining attention to H2. For a layer reinsurance policy h(x)=(x−a)+−(x−VaRX(α))+ with a∈[0,VaRX(α)], the total costs of the insurer and the reinsurer under the VaR risk measure are
VaRTfI(α)=a+(1+θ)∫VaRX(α)aS(x)dx, |
VaRTfR(α)=VaRX(α)−a. |
Hence, the loss function is
LE(a)=√[a+(1+θ)∫VaRX(α)aS(x)dx]2+[VaRX(α)−a]2. |
Theorem 4.2. The optimal ceded loss function that solves (2.14) with F2 constraint is given by
f∗2(x)={(x−a∗1)+−(x−VaRX(α))+,θ1+θ<α,0,otherwise, | (4.18) |
where a∗1 is the unique solution of equation (4.19)
[a+(1+θ)∫VaRX(α)aS(x)dx][1−(1+θ)S(a)]−[VaRX(α)−a]=0. | (4.19) |
Proof. Let HE(a)=[a+(1+θ)∫VaRX(α)aS(x)dx]2+[VaRX(α)−a]2, then
H′E(a)=2(a+(1+θ)∫VaRX(α)aS(x)dx)(1−(1+θ)S(a))−2(VaRX(α)−a), | (4.20) |
HE″ | (4.21) |
If holds, it is easy to show that . According to (4.20) and (4.21), then and attain their minimum at . In this case, .
If holds, then from (4.19) and (4.21), we have
Recall that and . If , then and if , then . So and imply that exists and is the only minimum point of and .
As a result of Proposition , we can deduce optimal ceded loss functions by confining attention to . For a quota-share reinsurance with a policy limit , the total costs of the insurer and the reinsurer under the VaR risk measure are
Hence, the loss function is
Theorem 4.3. The optimal ceded loss function that solves (2.14) with constraint is given by
(4.22) |
where and .
Proof. Let , then
(4.23) |
(4.24) |
If , according to (4.23), we have . Thus, attains its minimum at . Therefore, the optimal ceded loss function is given by .
If , according to (4.23) and (4.24), attains its minimum at . Thus, the optimal ceded loss function is given by .
In this section, we determine the optimal reinsurance policies among the ceded loss function sets under the Dutch premium principle. The Dutch premium principle is given by
(5.1) |
where, .
From Proposition , we know that the optimal ceded loss function in can be determined by confining attention to . For a change-loss reinsurance with and , the total costs of the insurer and the reinsurer under the Dutch premium principle are
where is the reinsurance premium. Then the VaR of and at confidence level are
(5.2) |
(5.3) |
Hence, the loss function is
Let , then
(5.4) |
where, .
Theorem 5.1. The optimal ceded loss function to reinsurance problem (2.14) is given as follows.
(1) If condition (M1) holds, then the optimal ceded loss function is given by , where
(2) If condition (M2) holds, then the optimal ceded loss function is given by , where,
, is the unique solution of equation and , .
(3) If condition (M3)holds, then the optimal ceded loss function is given by , where is the unique solution of equation and
(4)If condition (M4)holds, then the optimal ceded loss function is given by , where and
Proof. Similarly to the proof of Lemma 4.1, the function is an increasing function with respect to . Then the study of optimal ceded functions which minimize the loss function in the class is simplified to solving the two-parameter minimization problem over closed subset . Since is continuous, then the minimum of over must attain at some stationary point or lie on the boundary.
(1) Note that the function is an increasing function. If condition (M1) holds, it is easy to show that
Then from the expression of in (5.4), we know that is an increasing function with respect to . Thus the minimum points of are located in .
Conversely, if condition (M1) does not hold, then there exists a such that holds in a right neighborhood of . That is to say, is not a minimum point of . Since for any , then no minimum points of are located in .
That is to say, the minimum points of are located in if and only if condition (M1) holds. In this case the optimal ceded loss function is .
(2) We first consider the stationary points of . Let
(5.5) |
By straightforward algebra, we obtain
(5.6) |
If condition (M2) holds, then and hold. Since for any , then the equation has a unique root in . Substituting in the second equation of yields
Since , then we have . Thus has a unique stationary point . In the following, we show that attains the minimum at the stationary point .
Conversely, if the minimum value of is attainable at some point on the right boundary, then Fermat's theorem implies
(5.7) |
which is equivalent to
(5.8) |
Since , we yield . Straightforward algebra leads to . Note that and , then we have . However, since , and , then we have . This leads to contradictions. Thus, if condition (M2) holds, the function does not attain the minimum at the right boundary.
If the minimum value of is attainable at some point on the lower boundary, then must satisfy the following conditions
(5.9) |
From (5.9), we yield
(5.10) |
which means , this is contradicted to the condition (M2).
In summary, if condition (M2) holds, the minimum of the function must be attained at the unique stationary point , i.e., the optimal ceded loss function is given by .
(3) If condition (M3) holds, from the above arguments in (2), we know that has no stationary points because and does not attain the minimum at because . Thus, the function attains the minimum at the boundary point if condition (M3) holds, that is to say, the optimal ceded loss function is given by .
(4) If condition (M4) holds, then the equation has no solutions in , namely, the function has no stationary points. Thus, the minimum point of over must lie on the right boundary at some point or must lie on the lower boundary at some point . If the minimum of is attainable at , then the conditions in (5.7) hold. Since , we yield . Straightforward algebra leads to . This is contradicted to and . Thus, the minimum point of over must lie on the lower boundary at point , namely, the optimal ceded loss function is given by .
For a layer reinsurance with , the total costs of the insurer and the reinsurer under the VaR risk measure are
where, . Hence, the loss function is
Theorem 5.2. The optimal ceded loss function that solves (2.14) with constraint is given by
(5.11) |
where is the unique solution of equation
(5.12) |
Proof. Let , then
(5.13) |
(5.14) |
From Eq (5.13), we know that
and
Hence, from (5.12) and (5.14), we have
Therefore, is the unique minimum point of . Since and have the same minimum points, then the optimal ceded loss function that solves (2.14) with constraint is given by (5.11) and (5.12).
From Proposition , we can deduce optimal ceded loss functions by confining attention to . For a quota-share reinsurance with a policy limit , the total costs of the insurer and the reinsurer under the VaR risk measure are
(5.15) |
Hence, the loss function is
Theorem 5.3. The optimal ceded loss function that solves (2.14) with constraint is given by
(5.16) |
where
and .
Proof. Let , then and have the same minimum points. Taking the derivative of , we obtain
(5.17) |
(5.18) |
Note that
(5.19) |
Then, according to (5.17), (5.18) and (5.19), and attain there minimum at , where
In this section, we construct four numerical examples to illustrate the optimal reinsurance policies that we derived in the previous sections. Let the confidence level , safety loading parameters and .
Example 6.1. Assume that the reinsurance premium is expectation premium principle and the loss variable has an exponential distribution with survival function , then , , , . By Theorem (4.1), Theorem (4.2) and Theorem (4.3), we know that the optimal ceded loss function among is , the optimal ceded loss function among is and the optimal ceded loss function among is .
Example 6.2. Assume that the reinsurance premium is expectation premium principle and the loss variable has a Pareto distribution with survival function , then , , , . By Theorem (4.1), Theorem (4.2) and Theorem (4.3), we know that the optimal ceded loss function among is , the optimal ceded loss function among is and the optimal ceded loss function among is .
Example 6.3. Assume that the reinsurance premium is Dutch premium principle and the loss variable has an exponential distribution with survival function , then , , , , . By Theorem (5.1), Theorem (5.2) and Theorem (5.3), we know that the optimal ceded loss function among is , the optimal ceded loss function among is and the optimal ceded loss function among is .
Example 6.4. Assume that the reinsurance premium is Dutch premium principle and the loss variable as a Pareto distribution with survival function , then , , , , . By Theorem (5.1), Theorem (5.2) and Theorem (5.3), we know that the optimal ceded loss function among is , the optimal ceded loss function among is and the optimal ceded loss function among is .
Remark 6.1. Note that the risks have the same mean and the parameters are same in the above four examples. For the exponential case, the optimal reinsurance policy is a stop-loss reinsurance when , while for the Pareto case, the optimal reinsurance policy is a change-loss reinsurance when . Therefore, the form of the optimal reinsurance policy depends on the distribution of loss variable .
The optimal reinsurance policies from the perspective of both the insurer and reinsurer have remained a fascinating topic in actuarial science. Many interesting optimal reinsurance models have been proposed. In contrast to the existing literatures, we provide two new findings to the optimal reinsurance models from both the insurer and reinsurer in this paper. First, we propose an optimization criterion to minimize their total costs under the criteria of loss function which is defined by the joint value-at-risk. Second, we extend the premium principle to a much wide class of premium principles satisfying two axioms: risk loading and stop-loss ordering preserving. Under these conditions, we derive the optimal reinsurance policies over three ceded loss function sets, (ⅰ) the change-loss reinsurance is optimal among the class of increasing convex ceded loss functions; (ⅱ) when the constraints on both ceded and retained loss functions are relaxed to increasing functions, the layer reinsurance is shown to be optimal; (ⅲ) the quota-share reinsurance with a limit is always optimal when the ceded loss functions are in the class of increasing concave functions. We further use the expectation premium principle and Dutch premium principle to illustrate the application of our results by deriving the optimal parameters.
We also wish to point out that further research on this topic is needed. First, for reinsurance, the challenges of classical insurance are amplified, particularly when it comes to dealing with extreme situations like large claims and rare events. We have to rethink classical models in order to cope successfully with the respective challenges. One of the better ways is to focus on modelling and statistics, related literature can be referred to [32,33]. Second, in most of optimal reinsurance problems, it is assumed that the distributions of the insurer's risks are known. However, in practice, only incomplete information on the distributions is available. How to obtain optimal reinsurance contracts with incomplete information is also an interesting topic. An attempt to such a problem is to use the statistical methods, see [34,35]. Third, although some papers have been devoted to deriving optimal reinsurance under model uncertainty, the optimal reinsurance with uncertainty still lacks of available analysis tools, maybe we can draw support from sub-linear expectation, for details, see [36,37]. We hope that these important open problems can be addressed in future research. We also believe that this article can and will foster further research in this direction.
The research was supported by Project of Shandong Province Higher Educational Science and Technology Program (J18KA249) and Social Science Planning Project of Shandong Province (20CTJJ02).
The authors declare that there is no conflict of interest.
[1] |
Schaduangrat N, Lampa S, Simeon S, et al. (2020) Towards reproducible computational drug discovery. J Cheminform 12. https://doi.org/10.1186/s13321-020-0408-x ![]() |
[2] | Rudrapal M, Khairnar SJ, Jadhav AG Drug Repurposing (DR): An Emerging Approach in Drug Discovery. in Drug Repurposing - Hypothesis, Molecular Aspects and Therapeutic Applications (2020). https://doi.org/10.5772/intechopen.93193 |
[3] | Moraca F, Marzano S, D'Amico F, et al. (2022) Ligand-based drug repurposing strategy identified SARS-CoV-2 RNA G-quadruplex binders. Chem Comm 85. https://doi.org/10.1039/D2CC03135C |
[4] |
Huang K, Fu T, Glass LM, et al. (2020) DeepPurpose: a deep learning library for drug–target interaction prediction. Bioinformatics 36: 5545-5547. https://doi.org/10.1093/bioinformatics/btaa1005 ![]() |
[5] | Ramsundar B, Eastman P, Walters P, et al. (2019) Deep Learning for the Life Sciences.O'Reilly Media, Inc. |
[6] | Zhu Z, Shi C, Zhang Z, et al. (2022) TorchDrug: A Powerful and Flexible Machine Learning Platform for Drug Discovery. arXiv : 2202.08320. |
[7] | American Psychiatric Association, in Diagnostic and statistical manual of mental disorders: DSM-52013: 237-242. https://doi.org/10.1176/appi.books.9780890425596 |
[8] | Pittenger C (2021) Pharmacotherapeutic strategies and new targets in OC. The Neurobiology and Treatment of OCD: Accelerating Progress. Current Topics in Behavioral Neurosciences. Cham: Springer. https://doi.org/10.1007/7854_2020_204 |
[9] |
Ramasubbu R, Ravindran A, Lapierre Y (2000) Serotonin and Dopamine Antagonism in Obsessive-Compulsive Disorder: Effect of Atypical Antipsychotic Drugs. Pharmacopsychiatry 33: 236-238. https://doi.org/10.1055/s-2000-8360 ![]() |
[10] |
Moresco RM, Pietra L, Henin M, et al. (2007) Fluvoxamine treatment and D2 receptors: a pet study on OCD drug-naïve patients. Neuropsychopharmacology 32: 197-205. https://doi.org/10.1038/sj.npp.1301199 ![]() |
[11] |
Gilson MK, Liu T, Baitaluk M, et al. (2016) BindingDB in 2015: A public database for medicinal chemistry, computational chemistry and systems pharmacology. Nucleic Acids Res 44: D1045-D1053. https://doi.org/10.1093/nar/gkv1072 ![]() |
[12] |
Mysinger MM, Carchia M, Irwin JJ, et al. (2012) Directory of Useful Decoys, Enhanced (DUD-E): Better Ligands and Decoys for Better Benchmarking. J Med Chem 55: 6582-6594. https://doi.org/10.1021/jm300687e ![]() |
[13] |
Weininger D (1988) SMILES, a chemical language and information system. 1. Introduction to methodology and encoding rules. J Chem Inf Comput Sci 28: 31-36. https://doi.org/10.1021/ci00057a005 ![]() |
[14] | KD value: a quantitative measurement of antibody affinity. [Online]. Available: https://www.abcam.com/primary-antibodies/kd-value-a-quantitive-measurement-of-antibody-affinity |
[15] | Gilmer J, Schoenholz SS, Riley PF, et al. (2017) Neural Message Passing for Quantum Chemistry. arXiv : 1704.01212. |
[16] |
Sarfraz N, Okuampa D, Hansen H, et al. (2022) pitolisant, a novel histamine-3 receptor competitive antagonist, and inverse agonist, in the treatment of excessive daytime sleepiness in adult patients with narcolepsy. Health Psychol Res 10. https://doi.org/10.52965/001c.34222 ![]() |
[17] |
Butler MG (2011) Prader-Willi Syndrome: Obesity due to Genomic Imprinting. Curr Genomics 12: 204-215. https://doi.org/10.2174/138920211795677877 ![]() |
[18] |
Crinò A, Fintini D, Bocchini S, et al. (2018) Obesity management in Prader–Willi syndrome: current perspectives. Diabet Metab Synd Ob 11: 579-593. https://doi.org/10.2147/DMSO.S141352 ![]() |
[19] | FY2007 List of Approved Products: New Drugs (2013) [Online]. Available from: https://web.archive.org/web/20130119182609/http://www.pmda.go.jp/english/service/pdf/list/NewdrugsFY2007.pdf |
[20] |
Nagoshi Y, Tominaga T, Fukui K (2016) Blonanserin Augmentation for Treatment-Resistant Somatic Symptom Disorder: A Case Series. Clin Neuropharmacol 39: 112-114. https://doi.org/10.1097/WNF.0000000000000134 ![]() |
[21] |
Zhang T-T, Xue R, Wang X, et al. (2018) Network-based drug repositioning: A novel strategy for discovering potential antidepressants and their mode of action. Eur Neuropsychopharm 28: 1137-1150. https://doi.org/10.1016/j.euroneuro.2018.07.096 ![]() |
[22] | Biperiden Uses, Side Effects & Warnings. [Online]. Available: https://www.drugs.com/mtm/biperiden.html |
[23] |
Poyurovsky M, Meerovich I, Weizman A (1995) Beneficial effect of low-dose mianserin on fluvoxamine-induced akathisia in an obsessive-compulsive patient. Int Clin Psychopharm 10: 111-114. https://doi.org/10.1097/00004850-199506000-00008 ![]() |
[24] |
Jaskari MO (1979) Observations on mianserin in the treatment of obsessive neuroses. Curr Med Res Opin 6: 128-131. https://doi.org/10.1185/03007998009114812 ![]() |