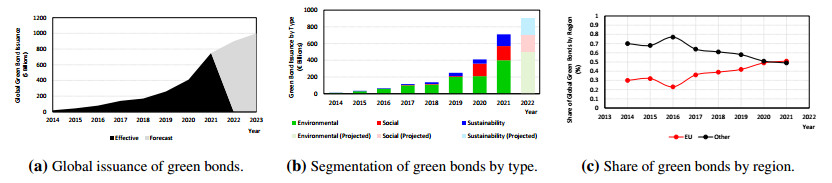
Recent years have been characterized by considerable growth of the green bond market in Europe, particularly in the domain of social bond issuance. Considering the recent pandemic, it is also a stylized fact that this growth is positively correlated with the concept of health-related uncertainty, as the green bond market aims to acquire financing in order to allow the development of projects that comply with the so-called environmental (E), social (S) and governance (G) criteria. This study then applies a dynamic spatial econometric analysis and several robustness checks to assess the extent to which each E, S and G criterion contributes to the societal dynamics of health-related uncertainty. The analysis takes advantage of available data on the number of confirmed cases of COVID-19 to measure health-related uncertainty at the municipal level, so that a higher (lower) number of confirmed cases constitutes a proxy for a greater (smaller) degree of uncertainty, respectively. To reinforce the need to evaluate impacts in a context characterized by health-related uncertainty, the time span covers the first wave of COVID-19, which is the period when uncertainty reached its highest peak. Additionally, the geographical scope is mainland Portugal since this country has become a breeding ground for startups and new ideas, being currently one of the world leaders in hosting businesses that reached Unicorn status. The main result of this research is that only the social dimension has a significant, positive and permanent impact on health-related uncertainty. Therefore, this study empirically confirms that the European green bond market has been and can be further leveraged by the need to finance projects with a social scope.
Citation: Vitor Miguel Ribeiro. Green bond market boom: did environmental, social and governance criteria play a role in reducing health-related uncertainty?[J]. Green Finance, 2023, 5(1): 18-67. doi: 10.3934/GF.2023002
[1] | Mengze Wu, Dejun Xie . The impact of ESG performance on the credit risk of listed companies in Shanghai and Shenzhen stock exchanges. Green Finance, 2024, 6(2): 199-218. doi: 10.3934/GF.2024008 |
[2] | Samuel Asante Gyamerah, Clement Asare . A critical review of the impact of uncertainties on green bonds. Green Finance, 2024, 6(1): 78-91. doi: 10.3934/GF.2024004 |
[3] | Aikaterini (Katerina) Tsoukala, Georgios Tsiotas . Assessing green bond risk: an empirical investigation. Green Finance, 2021, 3(2): 222-252. doi: 10.3934/GF.2021012 |
[4] | Raja Elyn Maryam Raja Ezuma, Nitanan Koshy Matthew . The perspectives of stakeholders on the effectiveness of green financing schemes in Malaysia. Green Finance, 2022, 4(4): 450-473. doi: 10.3934/GF.2022022 |
[5] | Amr ElAlfy, Adel Elgharbawy, Tia Rebecca Driver, Abdul-Jalil Ibrahim . Sustainability disclosure in the Gulf Cooperation Council (GCC) countries: Opportunities and Challenges. Green Finance, 2025, 7(1): 40-82. doi: 10.3934/GF.2025003 |
[6] | Zach Williams, Heather Apollonio . The causation dilemma in ESG research. Green Finance, 2024, 6(2): 265-286. doi: 10.3934/GF.2024011 |
[7] | María Cantero-Saiz, Sergio Sanfilippo-Azofra, Begoña Torre-Olmo, Violeta Bringas-Fernández . ESG and bank profitability: the moderating role of country sustainability in developing and developed economies. Green Finance, 2025, 7(2): 288-331. doi: 10.3934/GF.2025011 |
[8] | Tao Lin, Mingyue Du, Siyu Ren . How do green bonds affect green technology innovation? Firm evidence from China. Green Finance, 2022, 4(4): 492-511. doi: 10.3934/GF.2022024 |
[9] | Juraj Pekár, Ivan Brezina, Marian Reiff . Green investments: Portfolio selection based on risk measure and ESG indicators. Impact of environmental indicators on portfolio selection. Green Finance, 2025, 7(2): 223-246. doi: 10.3934/GF.2025009 |
[10] | Abhisek Mahanta, Naresh Chandra Sahu, Pradeep Kumar Behera, Pushp Kumar . Variations in financial performance of firms with ESG integration in business: The mediating role of corporate efficiency using DEA. Green Finance, 2024, 6(3): 518-562. doi: 10.3934/GF.2024020 |
Recent years have been characterized by considerable growth of the green bond market in Europe, particularly in the domain of social bond issuance. Considering the recent pandemic, it is also a stylized fact that this growth is positively correlated with the concept of health-related uncertainty, as the green bond market aims to acquire financing in order to allow the development of projects that comply with the so-called environmental (E), social (S) and governance (G) criteria. This study then applies a dynamic spatial econometric analysis and several robustness checks to assess the extent to which each E, S and G criterion contributes to the societal dynamics of health-related uncertainty. The analysis takes advantage of available data on the number of confirmed cases of COVID-19 to measure health-related uncertainty at the municipal level, so that a higher (lower) number of confirmed cases constitutes a proxy for a greater (smaller) degree of uncertainty, respectively. To reinforce the need to evaluate impacts in a context characterized by health-related uncertainty, the time span covers the first wave of COVID-19, which is the period when uncertainty reached its highest peak. Additionally, the geographical scope is mainland Portugal since this country has become a breeding ground for startups and new ideas, being currently one of the world leaders in hosting businesses that reached Unicorn status. The main result of this research is that only the social dimension has a significant, positive and permanent impact on health-related uncertainty. Therefore, this study empirically confirms that the European green bond market has been and can be further leveraged by the need to finance projects with a social scope.
The commitment to the set of objectives established in the Paris Agreement and the European Green Deal defines a shift of paradigm in the European Union (EU). Green bonds – fixed-income securities conceived to support social, climate, environmental and sustainable projects – are key instruments of green finance. Conceptually, there are three types of green bonds:
1. Environmental bonds;
2. Social bonds; and
3. Sustainable bonds.
These are expected to play a critical role in the EU's structural change due to their relevance for financing investments, projects and assets aimed at addressing economic, social and environmental issues. Governments and business organizations use them to finance the transition to a low-carbon economy since the European Investment Bank (EIB)'s inauguration of the green bond market in 2007 with its Climate Awareness Bond (CAB). Nevertheless, achieving its full potential requires boosting credibility through a clear definition of what constitutes green and improving the harmonization of practices to make it easier for investors to identify relevant projects.
According to Hamilton et al. (2020), establishing a clear definition of health‐related uncertainty is important for promoting research and improving the comprehension of this phenomenon, and academics from multiple disciplines have presented various descriptions of this construct. Their focus relies on a conceptualization developed by Han et al. (2011), which integrates these perspectives into a multidimensional taxonomy of uncertainty in healthcare to ensure a comprehensive tool for categorizing varieties of health-related uncertainty by specifying three distinct dimensions:
● Source, which includes probability (i.e. uncertainty about the likelihood of an event arising from the randomness or indeterminacy of the future), ambiguity (i.e. uncertainty regarding the strength, validity, consistency or adequacy of risk estimates) and complexity (i.e. aspects of an event that make it difficult to understand, such as potential causes or outcomes);
● Issue, which corresponds to the substantive content of uncertainty and includes scientific uncertainty (e.g., diagnosis or prognosis), practical uncertainty (e.g., procedures to access care) and personal uncertainty (e.g., implications of disease for personal relationships); and
● Locus, which is the perception of uncertainty, so that dyadic exchanges between patients and providers constitute a fundamental step for the management of diseases and the development of a shared understanding of relevant information and associated risks.
While the EU green bond market is growing rapidly, it is still immature and only represents a small fraction of the overall EU bond market. On July 2021, the European Commission (EC) presented a regulatory proposal focused on green bonds. This legislative framework, known as the EU Green Bond Standard (EU GBS), relies on the EU taxonomy for sustainable activities and complements other measures included in the 2018 Action Plan on Sustainable Finance (APSF) and, more recently, in the 2021 New Strategy on Sustainable Finance (NSSF). The green bond market, both globally and at the EU level, grew on average at a rate of 50% per year during the period 2015-2020. Although EC (2022) predicts that the green bond market will reach $1 trillion in 2023, it only represented 3.5% of the overall bond issuance in 2020 (Figure 1a). Recently, the EC launched Next Generation EU, a temporary instrument bringing up to €800 billion in support to the EU's recovery from the coronavirus pandemic and building a greener and more resilient territory. To finance it, the EC will borrow up to €800 billion in current prices on capital markets by the end of 2026. Of this amount, up to €724 billion will be made available under the Recovery and Resilience Plan (RRP), while the additional €76 billion will support key EU programs. To raise the necessary funding under the best possible market conditions, the EC is implementing a strategy that combines different funding techniques with transparent communication to stakeholders, which ensures the ability to quickly react to market turbulences. In a second green bond syndication on February 2022, the EC raised €6 billion in funds. This bond, with a maturity of 20 years, brings the total long-term financing raised under the Next Generation EU program to €99.5 billion, of which €20.5 billion corresponds to green bond emissions. This bond issuance was more than 13 times covered by investor orders and represents the largest bond-related event in 2022. Intuitively, size and quality of the order book confirm investors' confidence in the EU green bond market.
The syndication of February 2022 emerged after EC carried out three joint issuances of social bonds between October 2021 and November 2021, worth of around $40 billion, which reflects the great appetite of investors for this type of bond. This evidence then constitutes the motivation behind the development of this research. As clarified in Figure 1a–1c:
● The green bond market reached $235.4 billion in 2020, which corresponds to a homologous growth rate of 14%;
● In total, 986 operations were carried out to finance social and sustainable projects in 2020, which made it possible to raise $522.1 billion, which represents a jump of 82% compared to funds raised in 2019;
● Emissions simultaneously having social and sustainable objectives more than doubled to $144.6 billion. In September 2021, $94.5 billion in sustainable bonds were raised. As for October (November) 2021, these operations reached the amount of $79.2 ($76.7) billion, respectively. In relative terms, the issuance of debt focused on these two types of green bonds has skyrocketed more than 750% during the biennium 2020–2021;
● The share of the EU Member States in the global green bond market surpassed the share sustained by other parts of the world in 2021;
● Although environmental bonds continue to be a relevant component of green bond issuance, the biennium 2020-2021 marked a shift of paradigm in this segment, with social bonds soaring from $16.6 billion raised in 2019 to $142.2 billion in 2020, which represents an annual rise of 756%; and
● There were also relevant bond emissions from business organizations: Alphabet and Volkswagen carried out the biggest deals, with two issuances of more than $2 billion by the owner of Google and one issue of $1.25 billion by the German car manufacturer.
Intuitively, data suggest that public policy actions to reduce health-related uncertainty proxied by the number of COVID-19 cases can change the landscape of the EU green bond market. While in past years, environmental bonds have constituted the most substantial part of the debt satisfying environmental, social and governance (ESG) criteria, the need for support policies to solve socioeconomic issues associated with the coronavirus pandemic implied a boom in social and sustainable bond issuance. EC (2022) clarifies that the strong growth in these two dimensions is justified by the urgency created by the coronavirus pandemic to attract capital to finance recovery projects aimed at:
● Promoting economic growth; and
● Dissuading health-related uncertainty at the societal level.
The EU leads this movement through joint debt placement operations launched in October 2021 under the Support to mitigate Unemployment Risks in an Emergency (SURE) program, whose goal is to finance social and sustainable projects in Europe.
Since the pandemic outbreak, several studies have been focused on analyzing COVID-19 data, which can be conceptually segmented into three distinct research domains:
1. A strand of research proposes a framework for the detection of outbreaks in advance (Rossman et al., 2020; Hsiang et al., 2020; Tian et al., 2020);
2. Some studies evaluate different measures of actuation to ensure a reduction of transmission rates (Crowley and Doran, 2020); and
3. A strand of research tries to identify environmental, social, governance and economic factors with influential power on health-related uncertainty proxied by metrics related to COVID-19.
The following review is exclusively focused on the third domain. First, one can observe that there is a lively strand of research focused on the relationship between COVID-19 and social mobility, which is captured either by traffic data or air pollution indicators. Studies focused on the impact of travel bans on the diffusion of the new coronavirus have grown exponentially since 2020, either by covering countries such as the United States of America (USA) (Glaeser et al., 2020; Barnes et al., 2010; Engle et al., 2020), China (Fang et al., 2020) and Italy (Favero et al., 2020; Bilgin, 2020; Pepe et al., 2020) or by performing cross-country analyzes (Milani, 2020; Yilmazkuday, 2020). The study presented in Engle et al. (2020) is relevant to emphasize contrasting empirical findings. Their research assesses the connection between changes in average distance traveled by individuals at the county level in the USA and the emergence of new confirmed COVID-19 cases, while controlling for demographic variables and restriction orders to stay-at-home. The authors find that a higher local infection rate up to the order of 0.003 percentage points (p.p.) is associated with a reduction in mobility by 2.31%, which alerts for the relevance of within-county propagation when the ban of intermunicipal mobility is applied. Nevertheless, they also show that stay-at-home restrictions, which have the ability to avoid the within-county propagation, reduce mobility by 7.87% and decrease infection rates. Moreover, counties with a larger share of the population over age 65, lower voting shares for the Republican Party and higher population density are more responsive to stay-at-home orders, which implies the identification of a relationship between demographic characteristics of local areas and the effectiveness of mobility restrictions. Similarly, but focusing on Italy and using a spatial discontinuity approach, Caselli et al. (2020) show that limitations on individual mobility between municipalities were effective in the sense they decreased the mean mobility rate by 7 p.p.; moreover, local features played a key role after travel bans were lifted. In particular, the catching up relative to the pre-pandemic pattern is stronger in municipalities where the labor force is relatively less exposed to the risk of contagion and more likely to be engaged in home working. A machine learning (ML) approach to select instrumental variables (IVs) that strongly predict the new coronavirus transmission is considered in Qiu et al. (2020) to estimate mediating effects of socioeconomic factors on the transmission of COVID-19 in China. In particular, population flow out of Wuhan, the distance between cities, gross domestic product (GDP) per capita, the number of doctors and contemporaneous climate conditions are used as potential socioeconomic factors. By considering meteorological characteristics two weeks prior to a given incidence as the Ⅳ for causal inference, two-stage least squares (2SLS) estimates reveal that stringent quarantine, city lockdowns and local public health measures imposed in late January 2020 significantly decreased the virus transmission rate and ultimately contained its spread by the middle of February 2020. Moreover, the authors find that population outflow from the outbreak source region posed a higher risk to destination regions compared to other factors (e.g., the similarity of economic conditions). From the previous compilation of arguments, two research hypotheses are then formulated:
H1: Environmental factors intrinsic to a given spatial unit reduce health-related uncertainty.
H2: Social factors intrinsic to a given spatial unit reduce health-related uncertainty.
Second, past studies also reveal that public governance can be a relevant driver for the spatial and time propagation of health-related uncertainty. Khalatbari-Soltani et al. (2020) show that a disadvantaged socioeconomic position is associated with disease and mortality, inclusive in the context of the current pandemic. Patel et al. (2020) confirm that a low socioeconomic status causes a rise in the cumulative number of new confirmed COVID-19 cases. The authors claim that a reduced immunity caused by factors such as the lack of medical services at a more advanced stage of illness, poverty, overcrowded accommodation, unstable income and precarious labor conditions make individuals more vulnerable and susceptible to the COVID-19 disease. Weill et al. (2020) confirm that low-income communities show less social distancing than high-income areas. Jalan and Sen (2020) conclude that the enhancement of public trust was a relevant factor to contain COVID-19 cases in the initial stage of spread. The reduction of migration (Zhan et al., 2020; Zhang et al., 2020; Fang et al., 2020), employment opportunities characterized by the possibility of developing home working (Barmby and Larguem, 2009; Pichler and Ziebarth, 2017), breaks of interpersonal contact from holiday school closings (Adda, 2016), reactive school closures (Litvinova et al., 2020), strategic targeting of travelers from locations with a high incidence rate (Milusheva, 2017) and public transportation strikes (Godzinski and Suarez-Castillo, 2019) can be effective on avoiding disease transmissions. Varkey et al. (2020) empirically examine socioeconomic determinants of COVID-19 for 42 Asian countries by specifying a cross-sectional ordinary least squares (OLS) regression model with heteroscedasticity corrected through the application of robust standard errors to show a positive and significant association of net migration and GDP per capita with the total number and new daily cases of COVID-19. The social capital, which is measured by voters' turnout ratio to indicate the willingness to participate in elections, is statistically significant and negative for new COVID-19 cases per one million inhabitants. The authors also show a positive and significant relation between poverty and COVID-19 cases. Interestingly, the previous year's health expenditure and diabetic prevalence rate are not statistically significant in their analysis. By focusing on countries from the Sub-Saharan Africa, Okoi and Bwawa (2020) compile evidence that health disparities limit the capacity to address COVID-19. Consequently, the following research hypothesis is formulated:
H3: Public governance intrinsic to a given spatial unit reduces health-related uncertainty.
Third, a few studies also demonstrate that the spread of viral infections depends on the economic conjuncture. In the case of the influenza virus, Adda (2016) not only demonstrates that its spread is faster during economic booms, but also confirms that the growth of trade can significantly increase the risk of contagion. Maurer (2009) and White (2019) confirm that vaccination is effective on reducing the spread of the influenza virus, while similar conclusion is found in Slusky and Zeckhauser (2018) by considering sunlight exposure as the explanatory variable. Markowitz et al. (2019) reveals that employment growth rates are positively related to the incidence of the influenza virus over the active population. As such, the following research hypothesis is established:
H4: Economic factors intrinsic to a given spatial unit reduce health-related uncertainty.
An interesting but not explored topic in this study due to the lack of data is the role of ESG criteria at the firm level. This is not a new idea, but there is often a vague idea about what each E, S and G factor means in practice. The introduction of the Sustainable Finance Disclosure Regulation (SFDR), which came into force on March 2021, is part of a wider movement towards sustainable finance. Collectively, these activities demonstrate that responsible investing has become a mainstream expectation rather than a mere niche concern. The SFDR compels market participants to justify their decisions with respect to sustainable investments, so that companies must be able to demonstrate how they monitor and measure ESG indicators before making the claim of being actively engaged in sustainable practices. Although most companies are already familiar with environmental and governance factors, many still need to internalize the social factor in their corporate values. When the social context is handled poorly, it can affect a company's value and increase the investment risk. Therefore, firms must demonstrate their readiness to make relevant disclosures, measure their social performance and report it clearly. To do this successfully:
● Companies must define a list of social criteria applied to their business organization;
● Companies must implement procedures and improvements to support the social framework; and
● Companies must establish indicators for monitoring the information to be disclosed.
Making progress on social factors requires a common understanding about their significance. Some themes result from the United Nations Principles for Responsible Investment (UN PRI):
● Health and safety, where some firms already have policies in their corporate agenda, but only now investors are factoring them into the risk assessment. The major shift of paradigm relies on the concern with employees' wellbeing by providing them access to services such as lifestyle advice, mental health and work autonomy. This not only reveals that companies care about their workers, but also reflects the theory that improved wellbeing increases productivity.
● Labor market issues, modern slavery and human rights, where firms' onus consists of demonstrating that they can adequately manage the risk of modern slavery in a context characterized by unprecedented globalization and remote work. Failures on this domain are no longer socially acceptable, otherwise companies are likely to face a reputational damage.
● Data privacy and security, which reflects the need to prioritize the safety of customers, suppliers, employees and stakeholders. Given the focus of investors on the likelihood of occurring data breaches that can affect consumer trust, companies need to quantify and manage data privacy and security risks.
● Customer orientation, which suggests that investors now look at customer-oriented metrics (e.g., quality of service) to assess firms' performance and risks.
● Diversity and equality, where companies should demonstrate how they perform in practice rather than merely explaining their policies. Diversity is not always easy to achieve, despite business organizations often make impressive mission statements. Also, the gender gap is a key indicator of within-firm equality, so that investors may avoid companies that are adverse to change it.
Assuming that health-related uncertainty is the main causal variable responsible for leveraging the EU green bond market EC (2022), the goal of this study is to identify which – environmental, social, governance and economic – factors have influential power on the spatial and time propagation of health-related uncertainty.* Hence, this study provides an answer to the following research question:
*In order to properly understand why the link made between health-related uncertainty and the EU green bond market is everything less artificial or, equivalently, not as vague as it may seem at first glance, consider the motivating example of a married woman who asks her husband for a divorce. Also, consider that it is publicly known that this request is derived from the fact that the woman cheated on her husband. In this case, the betrayed husband is not interested in identifying determinants of the divorce (i.e. the ultimate end of the problem) but rather the set of causes justifying the betrayal committed by his wife (i.e. the source of the problem). Similarly, the objective of this study is not to identify determinants of the exponential increase in green bond issuance in Europe (i.e. the ultimate end of the problem) as it is already documented in multiple EU official reports that this boom was caused by the COVID-19 period and the need to rebuild this continent in the post-pandemic period. Instead, analogously to the example of the woman who betrays her husband, this study is concerned with identifying causes behind the proliferation of health-related uncertainty (i.e. the source of the problem). In this sense, following the terminology employed by Kreps and Wilson (1982), any claim that the interconnection between health-related uncertainty and the EU green bond market is vague constitutes a non-credible threat. This implies that the determination of statistically significant regressors affecting the topic under scrutiny (i.e. measurement of health-related uncertainty) is mandatory to explain the target (i.e. EU green bond issuance) and the European green market in general. We thank to the anonymous reviewers for alerting us to the need of better explaining that the boom in EU green bond issues should be properly contextualized by means of identifying causes that affect the source of the problem (i.e. the treatment variable that corresponds to health-related uncertainty).
1. Can ESG criteria effectively dissuade health-related uncertainty at the societal level?
If that is the case, then the boom observed in the EU green bond market can be indirectly explained by the ability of ESG criteria on influencing health-related uncertainty and restoring investors' confidence. If that is not the case, then targeted actions must be disclosed to ensure the future persistence of a significant causal effect of ESG criteria on reducing health-related uncertainty given that, ceteris paribus, this would ensure an even greater growth of the green bond market in Europe.
From a methodological point of view, this study applies a dynamic spatial econometrics regression model to assess the influence of environmental, social, governance and economic factors on health-related uncertainty. Although this concept has a subjective nature, it can be proxied by objective metrics and composite indices (Hamilton et al., 2020). In particular, this study takes advantage of available data on the number of new confirmed cases of COVID-19 to measure health-related uncertainty at the municipal level, so that a higher (lower) number of confirmed cases is a proxy for a greater (smaller) degree of uncertainty, respectively. In order to reinforce the need to evaluate impacts in the context of health-related uncertainty, the time span covers the period when uncertainty reached its highest peak (i.e. the first wave of COVID-19) and the geographical scope is mainland Portugal. Currently, this country leads in hosting companies that achieved Unicorn status, which suggests that it is able to capture a substantial part of the funding coming from social bond issuance in Europe.
Main normative results of this study indicate that:
● Environmental criteria proxied by air pollution metrics have no significant impact on reducing health-related uncertainty;
● Public governence criteria proxied by the transparency of economic activities and the reduction of bureaucracy in international trade have no significant impact on reducing health-related uncertainty;
● The economic factor related to the variation in tourism inflows also does not have explanatory power on the spatial and temporal propagation of health-related uncertainty; and
● Only the criterion focused on the degree of social proximity exhibits a significant effect on explaining the dynamics of health-related uncertainty. This component of the social dimension has a significant, direct, positive and permanent impact on the spread of health-related uncertainty. Benchmark results indicate that a 1% increase in internal consumption through a face-to-face interaction between buyer and seller rises the number of COVID-19 cases by 0.47% (0.72%) in the short (long) run, respectively.
Although these normative results are exclusively related to a single European country, Portugal has become a breeding ground for startups and new ideas, being currently one of the world leaders with the highest number of businesses that reached Unicorn status. Intuitively, this means that it is a country that captures a substantial part of the funding coming from social bond issuance and the private sector in Europe. Therefore, these results can explain, at least partially, why the EU is taking the lead in green bond issuance for financing recovery and resilience projects: the old continent is not yet prepared to deal with health-related uncertainty at the societal level. Since the social dimension has a significant, positive and direct effect on health-related uncertainty, financing projects concerned with this specific domain can be an optimal strategy to ensure social welfare gains in the future. Moreover, as discussed in Section 4, the lack of significance observed in environmental and governance criteria suggests the need to enhance the set of policy measures centered on these two dimensions to foster an even greater growth of the EU green bond market. Finally, knowing that the benchmark exercise consists of a dynamics spatial econometric analysis, technical results can be summarized as follows:
● First order queen contiguity (QC) is the optimal spatial weights matrix, which implies that the geographical propagation of health-related uncertainty is a local phenomenon;
● Both time and spatial dependence effects are statistically significant at the 1% level;
● Long-term effects are stronger than short-term effects, which means that reducing health-related uncertainty depends on discovering a safe and effective vaccine or, equivalently, achieving a full state of certainty;
● Benchmark results are qualitatively validated through several extensions described in Subsection 2.2.3.
The study is organized as follows. Methods are presented in Section 2. Section 3 clarifies benchmark results and robustness checks. A discussion is exposed in Section 4, while Section 5 concludes. The Supplementary Appendix provides details not exposed in the main text for the sake of brevity.
According to Santana et al. (2020), first cases diagnosed with COVID-19 in Portugal were reported on March 2, 2020 and the first official death caused by the new coronavirus was registered on March 16, 2020. Meanwhile, the World Health Organization (WHO) declared the outbreak of COVID-19 as a pandemic on March 11, 2020. In light of the Portuguese Law, a set of diversified measures can be adopted to mitigate adverse effects of extraordinary events such as pandemics, which are contextualized into five possible states of governmental actuation: alert, contingency, calamity, emergency and siege.† In a rapid intervention without historical precedent in Portugal, the first period of State of Emergency was declared on March 19, which was renewed on April 3 and April 18. In the period of Easter (i.e. 9–13 April, 2020), there was the first imposition of limited circulation between municipalities. The same action was applied to the period 1–3 May for the second time due to the national holiday related to the Worker's Day on May 1, 2020. The transition to a State of Calamity occurred on May 3, which was followed by three phases of confinement relaxation. On July 1, 2020 the State of Alert was declared to the majority of Portuguese municipalities, the State of Contingency was applied to the Metropolitan Area of Lisbon (MAL), but the State of Calamity remained for 19 parishes of five municipalities of the MAL. On August 1, 2020 the State of Alert remained for most regions of the country and the State of Contingency was declared for all municipalities belonging to the MAL.
†State of Alert (a.k.a., early warning response system) prevails under the threat, event, condition or public health agent whose presence may cause either direct or indirect damage to an exposed population in such a way that it can promote a public health crisis (ECDPC, 2018). State of Contingency corresponds to different means and biological safety measures used in the maintenance and handling of potentially infectious microorganisms that aim to protect workers, facilities, the environment and the community (PMH, 2014). State of Calamity is characterized by reducing one alert level in relation to the State of Emergency given that it corresponds to the immediately below intervention degree to ensure the maintenance of public order. As highlighted in the International Health Regulation (IHR), State of Emergency corresponds to an extraordinary occurrence where one concludes that: (Ⅰ) a risk to public health exists in other States due to the possibility of international spread; (Ⅱ) a timely and coordinated international response is mandatory (PMFA, 2008). Decreed by the President of the Portuguese Republic, it defines a framework of restrictions that, right after the state of siege, can lead to the partial suspension of the exercise of rights, freedoms and guarantees with the strengthening of the powers of the civil administrative authorities and the support to them by part of the Armed Forces (APR 1986, p. 2781). In particular, Article 9 of Law 44/86 regulates various levels of State of Emergency, from which one can infer that it also accommodates the State of Calamity given that this corresponds to the immediately below intervention degree to ensure the maintenance of public order, thereby implying that the scope of APR (1986) is broad enough to give a sufficiently high capacity of intervention to Portuguese governmental authorities whenever deemed justified.
In what follows, the exploratory spatial data analysis (ESDA) is organized in two parts. First, one reveals mortality data throughout the Portuguese national territory until the beginning of October 2020 for any given cause of death. This information is obtained from the civil registry data set provided by the Portuguese Integrated Civil Registration and Identification System (PT ICRIS) (i.e. death certificates). Second, one explores the differentiation between incidences and the evolution in accumulated number of confirmed COVID-19 cases by focusing on municipal level data periodically released by the Portuguese Directorate-General for Health (PT DGH).
Between March 1, 2020 and October 7, 2020, the preliminary number of deaths was 7 123 (9 597) higher compared to the homologous period in 2019 (2015), respectively. As clarified in Figure 2, the positive variation is reflected in different indices, where 2010 is assumed as the base-year. While the number of deaths decreased by 0.64% from 2010 to 2015, this statistic increased 3.63% from 2010 to 2019 and 15.37% in the last decade.
A more refined outlook confirms that the positive variation in the total number of deaths between 2019 and 2020 during the period between March 2020 and September 2020 is predominantly caused by the increase of mortality in people aged 75 and above (+ 5 944) in 179 (out of 278) municipalities of mainland Portugal, which is a geographical space that represents approximately 70% of the resident population. Figure 3 clarifies that the eleventh week in 2020 defines an inflection point due to two main reasons. First, one observes a clear reversal of the trend regarding the number of deaths aged equal or over 75 years because all the values recorded in 2020 turn out to permanently surpass those recorded in 2019. Second, precisely in that same time period, there is a clear reversal of the trend related to the mortality weight of elderly population aged equal or over 75 years on the total number of deaths given that registered values in 2020 overcome those recorded in the previous year for most of the remaining weeks of the year. Furthermore, one can observe that this outcome is resilient to gender differences despite women have a significantly higher mortality rate in the population aged 75 years or older. Although men live on average less years than women, this result suggests that both groups are similarly affected by the new coronavirus in Portugal, which contradicts evidence that SARS-CoV-2 is significantly more lethal in men.
Evolution of new confirmed cases of COVID-19 during the health-related uncertainty period. On the evolution of location coefficients representing new confirmed COVID-19 cases between March 2020 and September 2020, simple computations allow to identify the following outcomes: April 19 (41.9), May 17 (47.1), June 14 (65.8), July 12 (51.7), August 9 (46.4), September 6 (37.2) and September 20 (34.5). These values suggest a positive trend until June 14, 2020 followed by a progressive reduction that reached the highest level of territorial dispersion by the end of September 2020, where the largest curve of territorial concentration is found. A positive trend of new confirmed cases of COVID-19 emerged particularly after the beginning of September 2020, with accumulated values of the last 7 days above 2 500 new cases since September 6, 2020. In relative terms, 2.4 new confirmed cases of COVID-19 per 10 000 inhabitants were registered on average by the end of this day. The number of new confirmed cases per 10 000 inhabitants was higher than the average value in 53 Portuguese municipalities, with 13 municipalities of the Metropolitan Area of Porto (MAP) and 11 municipalities of the MAL standing out. In the 7-days window ended on September 6, MAL represented 40% of the new cases in Portugal, which achieved 4.5 new cases per 10 000 inhabitants on September 20, 2020. This ratio was higher than the average value in 55 Portuguese municipalities, especially in municipalities of the MAL: Amadora (9.4), Sintra (9.0), Lisbon (8.8), Odivelas (8.1), Cascais (7.3), Loures (7.2) and Oeiras (6.9). Over the last two weeks of September 2020, it was observed an increase in the accumulated number of new confirmed cases in municipalities belonging to the MAP, which became closer to the average value of new confirmed cases per 10 000 inhabitants. Both metropolitan areas represented more than half (56%) of the total new confirmed cases identified in Portugal. Indeed, by the end of September, there were 58.8 cases of COVID-19 per 10 000 inhabitants. Reading the relation between confirmed cases and new accumulated cases in the last 7-days window per 10 000 inhabitants allows to confirm that 9 municipalities of the MAL sustained values above the national average in both indicators and concentrated around one third of total new cases in Portugal and more than 80% of new confirmed COVID-19 cases within the MAL. Overall, ESDA results suggest that the first wave of COVID-19 in Portugal corresponds to the time window between March 2020 and September 2020.
Numbers by the end of September 2020. Figure 4 shows the territorial dispersion of COVID-19 cases accumulated by the end of September 2020, considering a distribution by municipalities.
The natural breaks graph indicates that 3 municipalities of the MAL have the highest accumulated number of new confirmed COVID-19 cases: Lisbon (6517), Sintra (5441) and Loures (3092). At the second critical level of natural breaks, 6 municipalities stand out: Amadora (3038), Vila Nova de Gaia (2275), ‡ Odivelas (2209), Cascais (2036), Porto (1802), Oeiras (1725) and Vila Franca de Xira (1630). It should be noted that Guarda is the inland municipality with the highest accumulated number of new confirmed COVID-19 cases: 1302. One should also emphasize that 161 (out of 278) municipalities in mainland Portugal or, similarly, 57.9% of the sample have less than 84 accumulated cases of COVID-19 by the end of September 2020. A refinement of the analysis shows that 46.0% (17.6%) of municipalities in mainland Portugal have less than 50 (10 or less) accumulated cases of COVID-19 by the end of September 2020, respectively. In turn, the excess risk graph reveals that high (low) risk regions are predominantly located in coastal areas (inland and cross-border regions) of the country, respectively.
‡This is the municipality of MAP with the highest number of accumulated cases by the end of September 2020.
Figure 5 confirms that the territorial dispersion in the accumulated number of new confirmed COVID-19 cases by the end of September 2020 is robust to the application of different methods. Consequently, one can conclude from the ESDA results that the first wave of COVID-19 in Portugal is characterized by a strong territorial heterogeneity. However, the concentration of COVID-19 cases is restricted to a small number of coastal municipalities characterized by a high population density, which can put pressure on the public health service available in these spatial units.
Indicators of spatial association. Relying on the accumulated number of new confirmed COVID-19 cases provides a limited perspective about the complexity of space associations, which means that both global and local indicators of spatial association should not be disregarded. As such, a first order QC spatial weights matrix and 9 999 permutations are considered to obtain spatial clustering associations and their pseudo p-values in the GeoDa software (Anselin et al., 2010). The Global Moran I's statistic is estimated to formally test for global geographic connectivity among Portuguese municipalities (Moran, 1950). The resulting pseudo p-values strongly reject the null hypothesis of spatial randomness. Figure 6 not only shows the Global Moran's I statistics associated with the monthly variation in the accumulated number of new confirmed COVID-19 cases, but also reveals the Global Moran's I statistic related to the accumulated number of new confirmed COVID-19 cases by the end of September 2020. The final value of 0.615 is statistically significant at the 0.001 level, which suggests the persistence of positive spatial autocorrelation. Therefore, one concludes that Portuguese municipalities with either high or low accumulated number of new confirmed COVID-19 cases are surrounded by municipalities dealing with a similar epidemiological situation.
Local Indicators of Spatial Association (LISA) are observed in Figure 7, which presents a choropleth map describing the space association between Portuguese municipalities and the statistical significance of Local Moran's I statistics for a default significance set at the 0.05 critical pseudo p-value level. Overall, four conclusions can be highlighted. First, there is a predominance of HH or hotspots (i.e. municipalities with a high number of infected individuals are surrounded by neighbors holding the same situation) and LL or cold spots (i.e. municipalities with a low number of infected individuals are surrounded by neighbors holding similar environment) given that there are only 6 statistically significant hybrid spots at the 0.05 critical pseudo p-value level, where 4 correspond to LH regions (i.e. municipalities with a low number of infected individuals are surrounded by municipalities with a high number of infected individuals) and 2 correspond to HL regions (i.e. municipalities with a high number of infected individuals are surrounded by municipalities with a low number of infected individuals).
Second, the number of hotspots is lower than the number of cold spots, which implies that it is less likely to observe municipalities with a high number of infected individuals being surrounded by municipalities holding the same characteristic compared to the opposite paradigm. Third, municipalities with a higher degree of statistical significance (i.e. communities with observed pseudo p-value below 0.001) are predominantly hotspots of the MAL. This means that, despite being a lower number compared to cold spots, hotspots are more likely to persist in the near future. Finally, within the set of municipalities holding statistical significance below the critical pseudo p-value of 0.05, one can observe a larger territorial dispersion of cold spots compared to hotspots. This implies that there is a stronger territorial concentration of hotspots and the spatial propagation of COVID-19 exhibits a local nature, which is particularly strong in both metropolitan areas of the country.
Data were retrieved from three sources: Portuguese National Statistics Institute (PT INE), Portuguese Air Quality Database (PT QualAR) and Portuguese Social Security (PT SS). Table 1 presents the variables of interest and respective descriptive statistics. The analysis deals with a panel data set that covers 278 municipalities of mainland Portugal over a period of 7 months from March 2020 to September 2020. Considering that February 29, 2020 corresponds to the moment t=0 and knowing that each additional time period or, similarly, each discrete time unit augmentation (+1) corresponds to one month, the variation in the accumulated number of new confirmed COVID-19 cases between period t and period t+1 in municipality i is considered the dependent variable (VD2dInfCov), which can be potentially affected by six covariates. In the environmental dimension, we consider:
Dimension | Acronym | Min | Max | Mean | Std Dev | Source (Definition) # |
Dependent variable | VD2dInfCov | 0 | 1 | 0.1380 | 0.2624 | PT DGH (1.) |
Environmental | VI3Mob | 0 | 1 | 0.0588 | 0.1567 | PT QualAR (2.) |
Social | VI6EldIPSS | 0 | 1 | 0.4209 | 0.3190 | PT SS (3.) |
VI5IntConsump | 0 | 1 | 0.2809 | 0.3158 | PT INE (4.) | |
Governance | VI4ForeignOpen | 0 | 1 | 0.1703 | 0.2353 | PT INE (5.) |
VI1BusinInten | 0 | 1 | 0.3516 | 0.2199 | PT INE (6.) | |
Economic | VI2ATourismFlow | 0 | 1 | 0.4970 | 0.1329 | PT INE (7.) |
Notes: superscript '#' stands for the need of reading the Supplementary Appendix for additional details. |
● An air pollution index, which was built with the objective of capturing the concentration of different air pollutants at the contemporaneous period t+1 in municipality i (VI3Mob) given that, from a theoretical point of view, residents of municipalities with higher air pollution are more likely to be susceptible to airborne infection due to their exposure to pollutants. For instance, Persico and Johnson (2020) find a strong and positive relationship between air pollution and COVID-19 mortality and case rates in the USA, particularly in counties with a higher population of black, lower income and unemployed individuals.
To obtain a perception about the degree of social isolation across Portuguese municipalities, the social dimension is represented by:
● Age normalized by private health supporting conditions (VI6EldIPSS), which is measured by the number of pensioners divided by the number of social solidarity private institutions (SSPIs) at the contemporaneous period t+1 in municipality i. Using this ratio can be justified by a twofold argument. On the one hand, the inclusion of the numerator stems from the fact that, despite SARS-CoV-2 infections are proved to be more serious for older individuals who are more likely to avoid them if they can do so, younger individuals are more likely to avoid public health interventions (PHIs) and contribute to the spread of COVID-19 cases. Ceteris paribus, this implies that the risk of propagation decreases when the numerator increases. On the other hand, the denominator reflects a constant term over the period of analysis, which not only allows to normalize the numerator but also controls for potential negative effects resulting from the degradation of healthcare conditions in private institutions with a social character, thereby meaning that residents of municipalities with a higher health risk index are more likely to be susceptible to the COVID-19 disease. This argumentation suggests that a reduction in the number of pensioners by SSPI is expected to have a negative impact on the dependent variable.
● Internal consumption and degree of social proximity through a commercial face-to-face interaction at period t in municipality i (VI5IntConsump), which is measured by the amount of withdrawals in automated teller machines (ATMs) located within the domestic market. Municipalities with more cash trades are expected to have a higher number of COVID-19 cases.
In terms of public governance, we adopt:
● Degree of coverage between period t−1 and period t in municipality i (VI4ForeignOpen) is used to capture the degree of openness of the domestic economy to foreign markets since it corresponds to the percentage of the value of the import of goods covered by the value of the export of goods, which corresponds to the statistical value of exports divided by the statistical value of imports.
● Intensity of the transparency in economic activities at period t in municipality i (VI1BusinInten), which is measured by the number of created legal collective organizations and similar entities (i.e. the birth of firms) deduced by the number of dissolved legal collective organizations and similar entities (i.e. the death of firms). From a theoretical point of view, the inclusion of this explanatory variable is justified by the fact that municipalities with a lower net creation of companies are more likely to sustain less active cases of COVID-19.
In the economic spectrum, we consider:
● Variation in tourism inflows between period t−1 and period t in municipality i (VI2ATourism-Flow), which is measured by a proxy that corresponds to the value of domestic purchases through automatic payment terminals made by individuals whose source of provenance (i.e. origin) is a foreign country. From a theoretical point of view, municipalities with a higher variation in tourism inflows are more likely to have a higher percentage of foreign visitors, which can facilitate the spread of the new coronavirus. Furthermore, these municipalities tend to be highly intensive in activities belonging to the tertiary sector, whose labor force is less likely to work from home.
A final mention should be given to the fact that covariates with a social character are contemporaneous, but covariates belonging to the economic spectrum are lagged by one period in time compared to the dependent variable. This option is justified not only to dissuade concerns related to endogeneity, but also because the SARS-CoV-2 virus is characterized by an incubation period that can reach up to 30 days and the isolation period required for mild or moderate cases during the first wave of COVID-19 in Portugal was 14 days. Moreover, we also applied several algorithms to perform feature selection.§ These allow to confirm that the set of inputs described in Table 1 have explanatory power on the dependent variable, which legitimizes our ex-ante choices from a statistical point of view.
§In particular, Marine Predators Algorithm, Generalized Normal Distribution Optimization, Slime Mold Algorithm, Tree Seed Algorithm, Gray Wolf Optimizer, Symbiotic Organisms Search, Flower Pollination Algorithm, Fruit Fly Optimization Algorithm, Bat Algorithm, Firefly Algorithm, Cuckoo Search Algorithm, Gravitational Search Algorithm, Artificial Bee Colony, Ant Colony Optimization, Particle Swarm Optimization and Simulated Annealing lead to the same set of input variables.
After the raw data collection, we apply feature engineering techniques. First, removal of outliers is considered given that a small number of highly dispersed observations can substantially influence the ability to obtain unbiased estimations and, consequently, explanatory variables with this type of bias may not sustain a statistically significant impact on the dependent variable despite of potentially being influential to its dynamics. Second, despite the detection of outliers being useful to mitigate the white noise, it may not constitute a sufficient condition to infer proper estimations. Indeed, the opposite case may occur in the sense that one may have to deal with highly concentrated data in a very small portion of the input range. Explanatory variables subject to this bias are unlikely to have a statistically significant effect on dependent variable. However, Deboeck (1994) proposes a solution to mitigate this source of concern, which consists of applying a bilateral truncation to the variables of interest that fall into this situation. Left and right truncations are defined by limits ¯x±σ. Third, we follow the recommendation proposed by Hancock et al. (1988), which highlights for the need to standardize data in cases where inputs and output have different units of measure, as verified in our data (e.g., VI4ForeignOpen is measured in percentage, while VI5IntConsump is measured in €). We finalize the data pre-processement stage by making a normalization to ensure that estimated coefficients are interpreted as elasticities as suggested by Hamilton et al. (2007). This is particularly relevant because the analysis deals with aggregated monthly data. In Portugal, the daily series of new confirmed cases of COVID-19 shows a time structure characterized by the recording of a greater number of daily cases each week, in relative terms, on weekdays from Tuesday to Friday compared to the remaining days. This cyclical standard determines the complementary use of a moving average with 7-days window or, similarly, the accumulated values of 7 consecutive days to assess the trend behavior of the daily series of COVID-19. Once these values are aggregated by month, it is convenient to interpret the effect of covariates on the dependent variable as elasticities because it may exist an abrupt variation in the number of cases between two or more consecutive weeks, which is a source of concern that can be mitigated by considering coefficients measured in relative terms. All steps of the data treatment were systematically applied to the dependent variable and respective covariates, with the exception of VI3Mob since this corresponds to an index, being thus already within the interval [0,1].
To illustrate the relation between ESG criteria at the municipal level in Portugal, Table 2 shows the correlation matrix and variance inflation factor (VIF) values. In general, the correlation of each independent variable relative to the dependent variable is stronger than the correlation of each independent variable in relation to other covariates, the mean VIF value is below 2 and individual VIF values are not high (i.e. they are all below 5), which suggests that the selection of covariates has the potential to explain the dependent variable without raising multicollinearity concerns.
VD2dInfCov | VI3Mob | VI6EldIPSS | VI5IntConsump | VI4ForeignOpen | VI1BusinInten | VI2ATourismFlow | VIF | |
VD2dInfCov | 1 | |||||||
VI3Mob | 0.6577*** | 1 | 1.05 | |||||
VI6EldIPSS | −0.0264 | −0.1577*** | 1 | 1.03 | ||||
VI5IntConsump | −0.1266*** | −0.1935*** | −0.0056 | 1 | 1.06 | |||
VI4ForeignOpen | 0.0018 | 0.0262 | −0.0279 | −0.1551*** | 1 | 1.14 | ||
VI1BusinInten | 0.5776*** | 0.8307*** | −0.1652*** | −0.1481*** | 0.0602 | 1 | 2.65 | |
VI2ATourismFlow | 0.1057*** | 0.2540*** | −0.0513** | −0.0355 | −0.0013*** | 0.2527*** | 1 | 2.53 |
Notes: Symbol *** (**) [*] for 1% (5%) [10%] of significance level, respectively. Mean VIF of 1.57 is below the critical threshold 5, thus, confirming the absence of multicollinearity. |
This study applies a methodology to identify determinants behind the time and spatial propagation of COVID-19 cases during the pandemic's first wave in mainland Portugal. The framework provides empirical estimates of E, S and G criteria on health-related uncertainty, which can help understanding whether the sign and size of parameter estimates are aligned with the economic theory or not. These relationships, which have to be empirically measured using relevant data points to scan the sign and magnitude of effects, include:
● Marginal propensity to dissuade health-related uncertainty;
● Elasticity of health-related uncertainty with respect to E, S and G criteria;
● Own-municipality and cross-municipality impacts of different explanatory variables;
● Returns to scale affecting health-related uncertainty;
● Short run (i.e. the period of time during which it is not possible for stakeholders to adjust completely to changes in explanatory variables of health-related uncertainty) and long run (i.e. the period of time during which it is possible for stakeholders to adjust to changes in explanatory variables of health-related uncertainty) marginal effects, which can be average marginal effects, marginal effects at the mean or average effects at specific representative values; and
● Short-term and long-term equilibrium and/or disequilibrium relationships between stationary explanatory variables and the target.
In most empirical studies, both correlation and regression techniques are used to estimate economic relationships. The use of correlation to examine the degree of relationship between target and independent variables has certain limitations, in particular the fact that a classification of variables as being either dependent or independent is something considered irrelevant because correlation does not mean causation. The estimation of relationships by resorting to spatial panel data models not only overcomes this source of concern, but also allows to estimate these relationships by considering a fully endogenous procedure that allows to associate different spatial units which, differently from the classical – linear or nonlinear – regression panel data model, may not be all interconnected with each other. In the field of spatial econometrics, several estimation procedures have been developed to model spatial dependence and examine spatial relationships among neighboring units. Anselin (1988) provides a comprehensive discussion on the use of various estimators (least squares, maximum likelihood, instrumental variable and method of moments) to account for spatial correlation issues in the context of a linear regression model. Kelejian and Prucha(1998, 1999) examine Generalized Spatial Two Stage Least Squares (GS2SLS) and Generalised Method of Moments (GMM) estimators, while Lee (2004) derives properties of the maximum likelihood estimator and its robust variance-covariance matrix. LeSage and Pace (2009) introduce the Spatial Autoregressive (SAR) model and Spatial Bayesian inference. With that being said, two different methods are considered in this study to find the adequate model specification and the optimal spatial weights matrix: the econometric approach suggested in Elhorst (2017) and the Bayesian inference proposed in LeSage (2014).
Econometric procedure. Following Elhorst (2017), the econometric analysis considers five steps. First, Lagrange Multiplier (LM) spatial lag tests and LM spatial autocorrelation error term tests should be performed to investigate the null hypothesis that spatial instead of non-spatial panel data models are more adequate for the problem under scrutiny. Table 3 exposes the results of formal statistical tests applied to the non-spatial pooled OLS (POLS) model, which indicate that spatial models are appropriate to analyze the research question under scrutiny due to evidence in favor of a positive and statistically significant spatial autocorrelation.
Statistical test | Value |
BP LM test for random effects | 215.8200*** [1] |
LM spatial lag (Anselin) | 467.7729*** [1] |
LM spatial error (Burridge) | 297.0096*** [1] |
Robust LM spatial lag | 799.4608*** [1] |
Robust LM spatial error | 628.6976*** [1] |
Global Moran | 0.2499*** (17.3760) |
Global Geary | 0.7710*** (−12.8250) |
Global Getis-Ord | −0.2499*** (−17.3760) |
Moran error test | 17.2858*** (1199.6900) |
LM SAC (LMErr+LMLag_R) | 1096.4704*** [2] |
LM SAC (LMLag+LMErr_R) | 1096.4704***[2] |
Notes: z-statistics in parentheses and degrees of freedom in brackets. Symbol *** (**) [*] represents 1% (5%) [10%] of significance level, respectively. Results reveal significant spatial autocorrelation, which validates the transition towards spatial panel data models. BP LM stands for Breusch–Pagan Lagrangian multiplier. Since p<0.05, the null hypothesis of homoscedasticity is rejected and, thus, a random effects model is preferred to the POLS model. Therefore, heteroscedasticity needs to be controlled for by resorting to a dynamic panel data estimation. LM stands for Lagrangian multiplier. Since p<0.05, the null hypothesis regarding absence of spatial autocorrelation is rejected, so that a spatial model is preferred to the POLS model. |
Second, one should identify if there is joint significance of spatial specific effects and time-period specific effects by means of performing likelihood ratio (LR) tests. Given the cross-sectional variability of attributes, the inclusion of both spatial and time-period fixed effects is a priori recommended to ensure that the unobserved heterogeneity between municipalities and years is effectively captured. Although the inclusion of two-way fixed effects can rely on theoretical arguments, the proposal in Elhorst (2017) is strictly based on formal statistical tests.¶ Results in Table 4 confirm the need to incorporate two-way fixed effects when the analysis is extended to spatial panel data models.‖
¶On the one hand, a time-period fixed effects specification in a setting composed by 278 municipalities and 7 months helps in identifying the real impact of covariates on the dependent variable because considering changes in explanatory variables over time within a given municipality may be a useful strategy to identify statistically significant effects. Time-period fixed effects are justified when background covariates are time-specific (e.g., expansion periods may be strongly affected by tourism inflows, while the opposite is verified in recession periods). As such, not controlling for spatial-invariant or, similarly, time-variant effects can increase the likelihood of obtaining biased estimates. On the other hand, a spatial fixed effects specification also helps in identifying the real impact of covariates on the dependent variable. After covariates are assigned to a given municipality, changes over municipalities within a given year is an adequate strategy because one expects that these exhibit some degree of persistence. Indeed, spatial fixed effects should be considered when background covariates are space-specific, difficult to measure or hard to obtain (e.g., data on coastal municipalities is often more precise than data on interior municipalities). Consequently, not controlling for spatial-variant or, similarly, time-invariant effects can increase the likelihood of sustaining biased estimates. Nevertheless, recent contributions in the literature provide reasons against the support of imposing fixed effects. According to Bell and Jones (2015), adding fixed effects is a way to increase the noise due to the additional structure imposed. Consequently, this action should be considered if the researcher provides valid reasons for imposing it (e.g., formal statistical tests).
‖On the one hand, the LR test result indicates that the null hypothesis of insignificant spatial fixed effects must be rejected (1247.40,277 df, p<0.05). Similarly, he hypothesis that time-period fixed effects are jointly insignificant must be rejected (1870.44, 6 df, p<0.05). The Hausman test (45.86, p<0.05) also confirms that two-way fixed effects are preferred to random effects.
Value | Spatial fixed effects | Time-period fixed effects | Spatial and time-period fixed effects |
F-statistic | F(283, 1656) = 18.7300*** | ||
Log Likelihood (null) | 22.4350 | −128.8935 | 1094.7750 |
Log Likelihood (model) | 171.1414 | 482.6482 | 1106.3600 |
AIC | −328.2829 | −951.3164 | −2198.7200 |
BIC | −289.2681 | −912.3017 | −2159.7060 |
Likelihood ratio test | 1247.4000*** [277] | 1870.4400*** [6] | |
Hausman test | 45.8600***[6] | ||
Notes: Degrees of freedom are within brackets. Symbol *** (**) [*] represents 1% (5%) [10%] of significance level, respectively. Since the F-statistic is significant sincep<0.05 for municipalities and time dummies, then the null hypothesis of homogeneity (pooling) is rejected at the 5% level. Thus, the LSDV (FE) model is most applicable since the data are favorably to the two-way fixed effects estimation. The inclusion of two-way fixed effects is also justified by the statistical significance observed in both likelihood ratio tests. Finally, the Hausman test indicatesp<0.05, which implies that the null hypothesis that the random effects model is preferred to the fixed effects model is rejected. |
Third, after restricting the optimal choice to the universe of spatial panel data models, Wald tests are executed to find the appropriate specification. Table 5 reveals that Dynamic Spatial Durbin Model (DSDM) is the optimal option among several static alternatives, which include the SAR model, Spatial Autocorrelation (SAC) model, Spatial Error Model (SEM) and Spatial Durbin Model (SDM), and associated dynamic variants: Dynamic Spatial Autoregressive (DSAR) model, Dynamic Spatial Error Model (DSEM) and Dynamic Spatial Durbin Error Model (DSDEM).
Comparison | χ2 | p-value | AIC | BIC |
(1) SAR versus SDM | 14.5900 [6] | 0.0237 | ||
(2) SEM versus SDM | 13.5400 [6] | 0.0353 | ||
(3) SAR | −2811.1730 | −2766.5850 | ||
(4) SEM | −2817.9920 | −2773.4040 | ||
(5) SAC | −2903.1390 | −2852.9770 | ||
(6) SDM versus DSDM | 85.6200 [2] | 0.0000 | ||
(7) SAR versus DSAR | 94.1900 [2] | 0.0000 | ||
(8) DSAR versus DSDM | 39.3400 [6] | 0.0000 | ||
(9) SAR versus DSDM | 250.5100 [8] | 0.0000 | ||
(10) SAC versus DSDM | 292.0000 [14] | 0.0000 | ||
(11) SEM versus DSDM | 245.2100 [8] | 0.0000 | ||
Notes: Degrees of freedom are within brackets. Values obtained while holding the first order queen contiguity matrix fixed Abbreviation SAR stands for spatial autoregressive model, SDM stands for spatial Durbin model, SEM stands for spatial error, SAC stands for spatial autocorrelation model, DSDM stands for dynamic spatial Durbin model and DSAR stands for dynamic spatial autoregressive model. The interpretation of outcomes is given as follows. Under (1), one cannot reject the null hypothesis that SAR is preferred to SDM for a critical significance level of 5%. Under (2), one cannot rejected the null hypothesis that SEM is preferred to SDM for a critical significance level of 5%. Therefore, at this phase, the static SDM is excluded from being the appropriate option. To select between SAR, SEM and SAC models, AIC and BIC values can be checked in (3) – (5). One can infer that SAC is the optimal static model. Taking thereafter into account dynamic models, (6) shows that one can reject the null hypothesis that SDM is preferred to the DSDM for a critical significance level of 5%. Based on (7), similar conclusion is applied to the rejection of SAR relatively to DSAR for a critical significance level of 5%. According to (8), one can reject the null hypothesis that DSAR is preferred to DSDM for a critical significance level of 5%. Therefore, the main conclusion for dynamic panel data models is that DSDM is the optimal choice. Finally, (10) reflects the need to compare the SAC model against the DSDM from which one rejects the null hypothesis that SAC is preferred to DSDM. Two caveats are worth mentioning. First, spatial models should not be compared by transitivity (i.e. based on a circular argument) but rather directly on a two-by-two basis. Second, (9) and (11) are redundant due to (3)–(5). |
Additionally, one should test whether stability and identification conditions are not violated. Results indicate that both conditions are unambiguously satisfied given that:
● F-test results indicate that the model is stable (i.e. it does not suffer from spatial co-integration) because ρ+χ+φ=0.7544<1; and
● Rank conditions derived in Lee and Yu (2016) for parameters of the DSDM to be identified are met since −χρ=0.1685≠φ=−0.0785 and, thus, the model is not space-time separable.
The last step consists of testing several spatial weights matrices, while keeping the optimal spatial model fixed. The optimal option consists of choosing the spatial weights matrix that minimizes the information loss by resorting to the observation of Akaike's Information Criterion (AIC) and Bayesian Information Criterion (BIC) values. Table 6 confirms that the optimal choice is the first order QC spatial weights matrix since it exhibits the lowest AIC and BIC value, which suggests that explanatory variables of municipality i are expected to influence a restrictive number of neighboring peers, namely the ones contiguous by a first order magnitude. This spatial weights matrix implies that municipalities are considered first order neighbors if they share a common border rather than a mere vertex. Since proximity plays a key role on the space and time propagation of COVID-19 across Portuguese municipalities, one can conclude that health-related uncertainty has a local nature.
Criterion | First order QC | Second order QC | hird order QC | First order RC | Second order RC | Third order RC |
AIC | −2587.8640 | −2522.5570 | −2468.8490 | −2582.2790 | − 2517.7480 | −4187.071 |
BIC | −2501.1540 | −2435.8470 | −2382.1390 | −2495.5690 | −2431.0380 | −4101.999 |
Notes: All values are obtained while holding the DSDM fixed. |
Bayesian inference. LeSage (2014) claims that Bayesian estimation methods can be used to solve model comparison problems and the lack of a theoretical ground on the choice of spatial weights matrices.
Four types of row-normalized geographical distance matrices are considered for the purpose of comparison: k−nearest neighbors matrices, inverse distance matrices with cutoffs and n−order QC and rook contiguity (RC) matrices. Furthermore, Bayesian inference is applied to test between the adoption of DSDM, DSAR, DSDEM and DSEM dynamic models or, equivalently, in favor of global-local, only global, only local or null spillover effects. Columns (1)–(4) in Table 7 report Posterior Model Probabilities (PMPs) for different model specifications, while keeping the spatial weights matrix constant. Results show that DSDM is the best model, which means that the monthly variation of COVID-19 cases is better described by the simultaneous absorption of exogenous and endogenous interaction effects and by the simultaneous presence of global and local spillovers, which reinforces that both contexts are relevant to evaluate the dependent variable at the municipal level in Portugal. Columns (5)–(8) in Table 7 report PMPs for different spatial weights matrices, while keeping the model's specification constant. For the DSDM, the spatial weights matrix displaying the highest probability is the first order queen contiguity, which implies a similarity between the outcome that stems from the procedure proposed by Elhorst (2017) and the one resulting from the proposal in LeSage (2014). Lastly, Figure 8 confirms that only a few spatial units are considered neighbors from each other, which confirms that the spatial propagation of COVID-19 cases is a local phenomenon.
PMP | ||||||||
Spatial model | Spatial weights matrix | Spatial weights matrix | Spatial model | |||||||
Matrix form | DSDM | DSAR | DSDEM | DSEM | DSDM | DSAR | DSDEM | DSEM |
Knn=4 | 1.000 | 0.000 | 0.000 | 0.000 | 0.000 | 0.000 | 0.000 | 0.000 |
Knn=5 | 1.000 | 0.000 | 0.000 | 0.000 | 0.000 | 0.000 | 0.000 | 0.000 |
Knn=6 | 1.000 | 0.000 | 0.000 | 0.000 | 0.000 | 0.000 | 0.000 | 0.000 |
Knn=7 | 1.000 | 0.000 | 0.000 | 0.000 | 0.000 | 0.000 | 0.000 | 0.000 |
Knn=10 | 1.000 | 0.000 | 0.000 | 0.000 | 0.000 | 0.000 | 0.000 | 0.000 |
Knn=15 | 1.000 | 0.000 | 0.000 | 0.000 | 0.000 | 0.000 | 0.000 | 0.000 |
Knn=20 | 1.000 | 0.000 | 0.000 | 0.000 | 0.000 | 0.000 | 0.000 | 0.000 |
Cut off = 15 km | 1.000 | 0.000 | 0.000 | 0.000 | 0.000 | 0.000 | 0.000 | 0.000 |
Cut off = 30 km | 1.000 | 0.000 | 0.000 | 0.000 | 0.000 | 0.000 | 0.000 | 0.000 |
Cut off = 50 km | 1.000 | 0.000 | 0.000 | 0.000 | 0.000 | 0.000 | 0.000 | 0.000 |
Cut off = 100 km | 1.000 | 0.000 | 0.000 | 0.000 | 0.000 | 0.000 | 0.000 | 0.000 |
First order QC | 1.000 | 0.000 | 0.000 | 0.000 | 1.000 | 1.000 | 1.000 | 1.000 |
Second order QC | 1.000 | 0.000 | 0.000 | 0.000 | 0.000 | 0.000 | 0.000 | 0.000 |
Third order QC | 1.000 | 0.000 | 0.000 | 0.000 | 0.000 | 0.000 | 0.000 | 0.000 |
First order RC | 1.000 | 0.000 | 0.000 | 0.000 | 0.000 | 0.000 | 0.000 | 0.000 |
Second order RC | 1.000 | 0.000 | 0.000 | 0.000 | 0.000 | 0.000 | 0.000 | 0.000 |
Third order RC | 1.000 | 0.000 | 0.000 | 0.000 | 0.000 | 0.000 | 0.000 | 0.000 |
Notes: Simulations from Matlab routines provided by LeSage (2014). Knn denotes k-nearest neighbor matrix, cut-off stands for inverse distance matrix temp1/dtempij, QC denotes queen contiguity matrix and RC denotes rook contiguity matrix. Parameterc is set to zero.T is a very large number, which results in a diffuse forβ, θ, χ andφ. Diffuse priors forσ are obtained by settingd=0 andv=0. Finally, a0=1.01. Results indicate that DSDM and first order queen contiguity matrix are the optimal choices. |
Extensions. To dissuade the omitted variable bias problem, a first robustness check consists of a two-step procedure. The first part of the exercise finds the optimal supervised ML model to apply a regularization technique to the set of initial covariates in order to identify the ones with statistically significant impact on the dependent variable. Thereafter, coefficients are re-estimated by considering the optimal spatial model previously identified in the benchmark exercise. The technical refinement was complemented by the inclusion of input-oriented stochastic frontier analysis (SFA) based on the ground that a policymaker pretends to minimize the monthly variation in the accumulated number of new confirmed COVID 19 cases. Various estimators were tested besides quasi-maximum likelihood (QML) to account for spatial correlation. Moreover, we consider alternative dependent variables (i.e. a health-related uncertainty index and the accumulated number of COVID-19 cases by the end of September 2020 divided by the population density of 2019). Additionally, outcomes of municipalities belonging to the MAP and MAL − where the risk of contagion or, similarly, where the degree of health-related uncertainty is higher − were confronted against outcomes of the remaining Portuguese municipalities. Lastly, we empirically confirm that regressors are not endogenous, so that using instruments is unnecessary.
As clarified in Section 3, DSDM is the optimal spatial regression model to analyze the data. The respective specification is formally defined as follows:
yi,t=χyi,t−1+ρWyi,t+φWyi,t−1+βxi,t+θWxi,t+μi+ξi,tτi,t+εi,t | (1) |
where yi,t denotes the N×1 vector that consists of a single observation of the dependent variable at time t (t=1,...,T) for each municipality i (i=1,...,N), yi,t−1 corresponds to the N×1 vector that represents the lagged dependent variable and the N×K matrix of exogenous and non-spatially weighted explanatory variables denoted by xi,t is associated with the K×1 vector of β coefficients, where K is the total number of covariates. Moreover εi,t=(ε1t,...,εNt)′ is a vector of disturbance terms, where εt are independently and identically distributed error terms for all i with zero mean and variance σ2. The vector μi=(μ1,...,μN)′ represents spatial specific effects for each time t, while the term ξi,t multiplied by τi,t represents time-period specific effects for each municipality i. Finally, it is clear that χ, ρ and φ correspond to scalar parameters, whereas the K×1 vector of θ parameters measures the strength of the spatial dependence between municipalities. Since spatial dependencies can hold in multiple channels, the DSDM incorporates endogenous interaction effects (Wyi,t) and exogenous interaction effects (Wxi,t) by including a spatially lagged dependent variable and spatially lagged independent variables in the respective specification. Moreover, within the set of municipalities considered neighbors from each other by the optimal spatial weights matrix, the spatial regression model can be characterized only by global spillovers (ρ≠0), only by local spillovers (ρ=0 and θ≠0) or it can sustain both types of spillovers (ρ≠0 and θ≠0). Global (local) spillovers are more likely when the optimal spatial weights matrix is sparse (dense) given that only a limited number of elements is (all non-diagonal elements are) different from zero, respectively. In order to systematize the empirical analysis, Figure 9 shows the conceptual framework − including research hypotheses − and the methodological pipeline of this study.
Coefficients of spatial, time and spatial-time diffusion effects are presented in Table 8. The dynamic response parameter χ is statistically significant, which implies that the time lagged dependent variable has influential power on the dependent variable. The positive sign means that, for each Portuguese municipality, the monthly variation in the accumulated number of new confirmed COVID-19 cases observed in the past has a positive effect on the target's current value.
DSDM (spatial and time fixed effects) | Short run | Lon run | |||||||
Dimension | Variable | β vector | θ vector | Direct | Indirect | Total | Direct | Indirect | Total |
Dependence | Time-lagged χ | 0.3459∗∗∗(0.0397) | |||||||
Spatial and time-lagged φ | −0.0785(0.0525) | ||||||||
Environmental | VI3Mob | −0.0385(0.0648) | 0.0722(0.1167) | −0.0296(0.0672) | 0.1029(0.2095) | 0.0733(0.2313) | −0.0408(0.1079) | 0.2055(0.4536) | 0.1647(0.5021) |
Social | VI6EldIPSS | −1.2504(5.6748) | −7.2833(9.0573) | −2.4050(5.2987) | −15.0124(13.3379) | −17.4174(12.4784) | −4.5189(8.0918) | −32.5838(27.9652) | −37.1057(27.7303) |
VI5IntConsump | 0.4683∗∗∗(0.1479) | −0.3088∗∗(0.1520) | 0.4662∗∗∗(0.1437) | −0.1495(0.2519) | 0.3166(0.2872) | 0.7244∗∗∗(0.2266) | −0.0483(0.5437) | 0.6761((0.6310) | |
Governance | VI4ForeignOpen | 0.0180∗(0.0104) | 0.0140(0.0304) | 0.0208∗(0.0111) | 0.0433(0.0537) | 0.0641(0.0585) | 0.0351∗(0.0188) | 0.1054(0.1223) | 0.1405(0.1333) |
VI1BusinInten | 0.0363(0.0480) | −0.0107(0.0673) | 0.0384(0.0464) | 0.0140(0.1093) | 0.0524(0.1278) | 0.0612(0.0744) | 0.0545(0.2437) | 0.1157(0.2816) | |
Economic | VI2ATourismFlow | −0.0289(0.0560) | 0.0720(0.0741) | −0.0235(0.0521) | 0.1064(0.0998) | 0.0829(0.0874) | −0.0316(0.0795) | 0.2086(0.2022) | 0.1770(0.1942) |
Mean of FE | 3.3062 | ||||||||
Spatial ρ | 0.4870*** (0.046) | ||||||||
σ2ε | 0.0135*** (0.0013) | ||||||||
R2 | 0.0109 | ||||||||
Log Pseudo-likelihood | 1244.3117 | ||||||||
AIC | −2587.8640 | ||||||||
BIC | −2501.1540 | ||||||||
Obs. | 1668 | ||||||||
Stability (Identification) [Endogeneity] | Satisfied (Satisfied) [Satisfied] | ||||||||
Notes: t-statistics in parentheses and degrees of freedom in brackets. Symbol *** (**) [*] represents 1% (5%) [10%] of significance level, respectively. By definition, dynamic spatial panel data models only consider fixed effects. The regression includes robust standard errors estimations and robust variance estimations, which accurately take into account the cluster-correlated data by adjusting for within-cluster correlation. |
The spatial dependence parameter ρ is significant and exhibits a positive sign, which implies that it corroborates the presence of global spillovers within the set of municipalities considered neighbors from each other by the optimal spatial weights matrix. This result suggests evidence in favor of the spatial propagation of COVID-19 cases across Portuguese municipalities. The combination of both results indicates that municipalities characterized by a low (high) monthly variation in the accumulated number of new confirmed COVID-19 cases are likely to persist with low (high) episodes of new infections caused by SARS-CoV-2, respectively. Hence, spatial and time dependence effects are statistically significant phenomena, which have a positive influence on health-related uncertainty. Different conclusion is applied to the representative parameter φ of spatial-time diffusion dependence, which reflects that the propagation of COVID-19 cases is unlikely to be transmitted to neighboring municipalities over time. Among several reasons, the lack of significance on the spatial-time dependence outcome can be explained by the interruption of daily work trips when the number of COVID-19 cases escalated exponentially due to the imposition of prophylactic isolation to block transmission chains. From the set of exogenous and non-spatially weighted explanatory variables associated with the β vector, only VI4ForeignOpen and VI5IntConsump are statistically significant, respectively at the critical p-value level of 0.10 and 0.01. The vector of θ coefficients confirms that a statistically significant local spillover is found for VI5IntConsump, whose interpretation should be preferably done in terms of marginal effects and based on their short- and long-term decomposition (Firmino et al., 2017).
Short- and long-term marginal effects are reported in Table 8. From the set of regressors, results reveal that only VI4ForeignOpen and VI5IntConsump are significant covariates. This is only verified in the direct channel, but both in the short and long run equilibria. Although these covariates have a positive impact on the dependent variable, results do not appear to be overly expressive for the case of VI4ForeignOpen given that an increase of 1 p.p. in the coverage rate of exports by imports leads to a rise of 0.02 (0.04)% in the monthly variation of COVID-19 cases in the short (long) run, respectively. However, for the case of VI5IntConsump, results indicate that an increase of 1% in the internal consumption (i.e. social proximity caused by the use of cash in commercial relationships) leads to a rise of 0.47 (0.72)% in the monthly variation of new confirmed COVID-19 cases in the short (long) run, respectively. It is crucial to remember at this phase that this regressor captures the amount of withdrawals in ATMs, which accommodates multiple social events such as shopping and face-to-face contact through the frequency of bars and restaurants, weddings, and so on. Although sustaining an inelastic relationship with the dependent variable, it should be noted that the short-term value is, by itself, alarming and it is leveraged almost 1.5 times once considering long run effects. Therefore, this result seems to confirm that VI5IntConsump is the main driver of health-related uncertainty by sustaining a significant, direct, positive and permanent effect on the spatial and time spread of new confirmed COVID-19 cases in Portugal.
Consequently, one can conclude that only H2 is confirmed for a significance level of 1%. Results also reveal that the magnitude of direct effects is lower than the magnitude of indirect effects in all the covariates with the exception of VI1BusinInten. Although this outcome a priori reflects complementarity effects between different Portuguese municipalities, it is not necessarily relevant due to the fact that the indirect channel does not exhibit statistical significance. The lack of significant indirect effects suggests the presence of a reasonable doubt on the efficacy of municipal lockdowns to dissuade the spatial and time propagation of COVID-19. Finally, results indicate that long run effects are stronger than short run effects.
Two-step machine learning approach. The main conclusion from applying machine learning is that the qualitative findings of the benchmark exercise are reinforced. As clarified in Table A1 of the Supplementary Appendix, the first-step of the procedure indicates that RIDGE is the optimal machine learning regression model to explain the dependent variable, which implies that the regularization technique does not eliminate any explanatory variable from the initial set of covariates selected for the benchmark exercise. Consequently, results of the second-step procedure are necessarily coincident with those obtained in the benchmark exercise, thereby allowing us to confirm that this study does not suffer from the omitted variable bias problem.
Stochastic frontier analysis. Knowing that coefficients of input-oriented SFA models should be interpreted as direct or own-municipality effects, results presented in Table A2 of the Supplementary Appendix reinforce the positive and significant influence of internal consumption on the dependent variable. Coefficients of the true fixed effects (Tfe) SFA and true random effects (Tre) SFA models respectively exhibit values 0.8140 and 0.8350 for observed p-values below 0.01. Perhaps, the most relevant message resulting from this extension is to alert for the significant, positive and direct impact of covariate VI6EldIPSS on the dependent variable. This outcome is identified in most SFA models, which suggests that municipalities with a higher percentage of pensioners by SSPI are predicted to have more COVID-19 cases, ceteris paribus. One should also highlight the negative effect of tourism influx observed in some SFA models, despite the respective magnitude is not significantly different from zero. Similar argument is applied to VI1BusinInten and VI4ForeignOpen under the Tfe SFA model, but with the difference that the dependent variable is subject to the opposite sign effect. In the column (1) of Table A2 in Supplementary Appendix, the impact of air pollution index also significantly increases the confirmed case count. Overall, one concludes that SFA models provide support to the benchmark findings given that, on top of the significant spatial and time dependence effects, results show that health-related uncertainty is positively influenced by domestic consumption patterns and social relationships.
Alternative estimators. Table A3 in the Supplementary Appendix shows direct or own-municipality effects on the dependent variable under alternative options to the QML estimator considered in the benchmark exercise, namely GMM and GS2SLS. With the exception of VI3Mob and VI6EldIPSS, coefficients obtained with the GMM estimator have the same sign as those obtained with the QML estimator. It is also important to note that the impact of covariate VI6EldIPSS is close to zero. Hence, GMM estimator is relevant to highlight that mobility and intensity of economic activities (influx of tourists) can positively (negatively) and significantly influence the dependent variable, respectively. Recall that, in the benchmark exercise, covariates that capture the economic dimension are lagged by one period in time to dissuade endogeneity concerns. Since the dependent variable is also lagged by one period in time with the GS2SLS estimator, one may have to deal with endogeneity since the lagged dependent variable can now be a function of explanatory variables with economic dimension that were initially used in the benchmark exercise. Consequently, we decompose results associated with this estimator in two parts: first, we present the estimated coefficients without including lags in explanatory variables and, thenceforth, we present the estimated coefficients after including a one-period lag in covariates holding economic dimension and, thus, that are likely to have influential power on the lagged dependent variable, namely VI5IntConsump, VI1BusinInten and VI2ATourismFlow. GS2SLS outcomes presented in columns (2)–(5) of Table A3 in the Supplementary Appendix reinforce the statistical significance of the representative covariate of internal consumption and reveal that covariates lagged in time by one period have a positive and significant effect on the dependent variable.
Endogeneity assessment. Endogeneity is a problem frequently observed in social sciences, which occurs when either one or several independent variables (i.e. the deterministic component of the regression model) are correlated with the disturbance term (i.e. the non-deterministic component of the regression model). Conceptually, endogeneity holds when the classical hypothesis that represents the exogeneity of regressors – i.e. E(u|x)=0 – is violated. Sources of endogeneity include:
● Omitted variables (i.e. unobserved independent variables that are necessarily reflected in the non-deterministic component of the linear regression model, which forces the disturbance term being correlated with independent variables); and
● Measurement errors.
Standard solutions for endogeneity require to:
● Find and incorporate either unobserved explanatory variables or proxies in the original model;
● Use fixed effects estimator with panel data in order to eliminate individual (i.e. specific) effects;
● Apply the 2SLS/IV method, which replaces the endogenous variable with a predicted value that only contains exogenous information.
At this point, it is relevant to briefly explain the 2SLS/IV method. For the sake of brevity and simplicity, consider the simple linear regression model (SLRM), which formally corresponds to y=β0+β1x+u. It is widely known that, if x is exogenous, then β1 will be unbiased and consistent with the application of OLS estimation that minimizes the sum of squared residuals (SSR). However, if x is endogenous such that cov(x,u)≠0, both properties are only sustained with the application of 2SLS/IV estimation. Under this method:**
**If x is exogenous, it can serve as own instrument z=x. Under this circumstance, IV and OLS estimates are identical: βIV1=βOLS1.
● The endogenous variable x is correlated with the disturbance term u;
● An instrument or IV corresponds to a variable that is used to correct for the endogeneity problem;
● The instrument z is related to the endogenous variable x but does not belong to the original model where y is dependent variable, which implies that it is uncorrelated with the disturbance term.
From a theoretical point of view, instrument z must satisfy three properties to be considered good:
1. It cannot appear in the original regression model y=β0+β1x+u;
2. It must be correlated with the endogenous variable x – i.e. cov(z,x)≠0 – such that x=δ0+δ1z+v, where δ1≠0;
3. It must be uncorrelated with the disturbance term u – i.e. cov(z,u)=0 – given that
cov(z,u)=cov(z,y−β0−β1x)=cov(z,y)−β1cov(z,x)=0. |
From a methodological point of view, the 2SLS/IV method consists of two steps:††
††If there is one endogenous variable and one instrument, then 2SLS estimates (replacing x with ˆx based on z) will be the same as IV estimates, cov(z,y)/cov(z,x). The 2SLS estimation can also be used if there is more than one endogenous variable and at least as many instruments.
● In a first stage, x=δ0+δ1z+v is a regression model of the endogenous variable x, whose deterministic component corresponds to the instrument z. This estimation allows to obtain the predicted value ˆx=ˆδ0+ˆδ1z, which only contains exogenous information;
● In a second stage, y=β0+β1ˆx+u is estimated. The coefficient ˆδ1 estimated with 2SLS/IV is unbiased because ˆx is exogenous and uncorrelated with the disturbance term u.
From a statistical point of view, two main differences can be observed once confronting the 2SLS/IV method with the standard OLS approach:
● The variance of the estimated coefficient β1 using the 2SLS/IV estimation is higher than the variance of this coefficient using the OLS estimation because, by definition, the coefficient of determination R2x,z is lower than 1; moreover, a weaker relation between x and z leads to lower R2x,z and higher variance of 2SLS/IV coefficients, which implies a reduced statistical significance;
● A very different value taken by the estimated coefficient β1 may be a sign of endogeneity.
Before proceeding with the empirical assessement of endogeneity, some andragogy is mandatory to ensure that readers understand a key detail: differently from classical econometrics, the effect of a regressor in a given spatial unit may not be propagated into all spatial units that compose the sample in the context of a spatial econometrics model such as the one used in this study. Indeed, under this circumstance, the possibility of observing endogeneity affecting some explanatory variable is highly dissuaded because the optimal spatial weights matrix may interconnect a limited number of municipalities with each other in equilibrium. As a result, any claim that a given regressor is correlated with the non-deterministic component of the regression model is likely to be false. In spite of this relevant point, we moved forward and applied the following procedure:
1. We start by choosing instruments;
2. We execute endogeneity tests to confirm whether each explanatory variable is exogenous or not. This task is developed:
(a) Considering the POLS model, as if we were investigating endogeneity before moving into the choice of spatial models, thus improving the methodology proposed by Elhorst (2017);
(b) Considering the optimal DSDM, as if we were investigating endogeneity as a robustness check to the benchmark analysis.
3. If statistical tests detect the presence of endogeneity, then we test the orthogonality of instruments;
4. If statistical tests detect the presence of endogeneity, then we confirm the optimal number of instruments to be added by testing whether the model should be just-identified or over-identified;
5. If statistical tests detect the presence of endogeneity, then we also test the quality of instruments. The current state-of-the-art rule claims that the global significance of the first stage of the 2SLS/IV procedure – where we estimate the endogenous variable as a function of an independent term plus the set of instruments (a.k.a. external instruments) and original exogenous variables (a.k.a. internal instruments) – should contemplate F>104.7 or, alternatively, maintaining 10 as a threshold requires replacing the critical value 1.96 with 3.43 (Lee et al., 2022). Since this statistical rule is not valid for spatial econometrics models, future work should find optimal values of the F-statistics for different types of spatial econometrics models; and
6. If all the previous steps are satisfied, then we apply the 2SLS/IV method to estimate coefficients and interpret them.
Regarding the first point – choice of instruments – Coles et al. (2008) and McKnight and Weir (2009) suggest using lagged control variables as external instruments. Knowing that this option has been adopted in several past studies, including Choi et al. (2013) and Muttakin et al. (2015), we also follow this recommendation:
● When considering each explanatory variable separately, we use the air quality/mobility regressor lagged in time by two periods (i.e. VI3Mobt−2) as the external instrument in most cases. Exceptions include:
– Adoption of tourism flow regressor lagged in time by 7 periods (i.e. VI2ATourismFlowt−7) as the external instrument when detecting for the presence of endogeneity in VI2ATourismFlow;
– Use of air quality/mobility regressor lagged in time by three periods (i.e. VI3Mobt−3) as the external instrument when detecting for the presence of endogeneity in VI4ForeignOpen; and
– Application of IPSS regressor lagged in time by two periods (i.e. VI6EldIPSSt−2) when detecting for the presence of endogeneity in VI6EldIPSS.
● When all the explanatory variables are considered together, we use the air quality/mobility regressor lagged in time by two, three, four, five, six and seven periods (i.e. VI3Mobt−2, ..., VI3Mobt−7) as external instruments.
Regarding the second point – testing endogeneity – there are several ways to identify whether a regression model is characterized by exogeneity. Regardless of considering ex-ante endogeneity detection – in the POLS model – or ex-post endogeneity detection – in the DSDM – we follow the conceptual idea seminally provided in Davidson and MacKinnon (1993), which assume that a given regressor to be considered endogenous must simultaneously have:
● A deterministic component; and
● A non-deterministic component.
Then, taking as a given the following nomenclature:
● y1 is the dependent variable;
● y2 is the endogenous variable; and
● z1 and z2 are exogenous variables or, similarly, internal instruments
the original structural equation POLS model can be generically described as follows:
y1=β0+β1y2+β2z1+β3z2+u, |
and it corresponds to the first step of the procedure to detect endogeneity. Bearing in mind that the goal is to test the endogeneity of y2, let us assume that two external instruments z3 and z4 are found for y2 (i.e. the case of overidentifying restrictions). In a second step, we can then estimate the reduced form equation:
y2=δ0+δ1z1+δ2z2+δ3z3+δ4z4+v2 |
to obtain the residuals ˆv2, which contain the endogenous information. Also note that, similarly to the first step of the 2SLS/IV method, predicted values ˆy2 only contain the exogenous information. Hence, the endogenous variable y2 is segmented into exogenous part ˆy2 and endogenous part ˆv2. Formally:
y2=ˆy2+ˆv2 |
Thenceforth, the third step consists of estimating the unrestricted structural equation model, whose specification includes the endogenous component ˆv2. It is formally written as follows:
y1=β0+β1y2+β2z1+β3z2+γˆv2+u |
in order to test the null hypothesis H0: γ=0 (i.e. the absence of endogenous information ˆv2 and, consequently, the exogeneity of y2) against the alternative hypothesis H1: γ≠0 (i.e. the presence of endogenous information ˆv2 and, consequently, the endogeneity of y2).
If the observed p-value is below 5% (i.e. the critical p-value assumed to establish a decision), we reject the null hypothesis. Under this circumstance, ˆv2 is significant such that y2 is endogenous and the use of either one or several instruments is mandatory. If the observed p-value is above 5%, we do not reject the null hypothesis. Under this circumstance, ˆv2 is not significant such that y2 is exogenous and the use of instruments becomes unnecessary.
Mutatis mutandis, the same reasoning can be applied to the DSDM, although with the caveat that the model specification needs to be changed in order to accommodate the spatial specificities presented in Eq. (1). A final remark is also made to highlight that, during this procedure, we correct for the violation of homoscedasticity.
Tables B1–B8 in the Supplementary Appendix show the results when endogeneity is tested in the POLS model. These allow to confirm that all the explanatory variables are exogenous because each coefficient representative of the residuals is not significant at the 5% level of significance. Tables B9–B32 in the Supplementary Appendix present the results when endogeneity is tested in the DSDM. These allow to conclude that all the explanatory variables are exogenous in the indirect channel. In the direct channel, only VI1BusinInten and VI2ATourismFlow may suffer from endogeneity. However, results are in favor of not rejecting the null hypothesis at the aggregated/total effects level, so that all the explanatory variables are considered exogenous. Consequently, the analysis of steps 3−6 described above becomes unnecessary and the benchmark analysis remains qualitatively valid.
Table A4 in the Supplementary Appendix shows estimated coefficients after considering that the dependent variable is a composite index of health-related uncertainty computed through a principal component analysis (PCA).‡‡ Similar to the benchmark exercise, internal consumption has a significant, positive, direct and permanent effect on the dependent variable. However, differently from the benchmark exercise, the number of pensioners by SSPI has a significant, negative, indirect and permanent impact on the dependent variable. Both cases exhibit stronger long run effects compared to short run counterparts. In turn, when considering that the dependent variable corresponds to the cumulative number of COVID-19 cases divided by the population density observed in 2019, results are summarized as follows. The internal consumption has a significant, negative, indirect and short-term impact on the dependent variable, which implies that it no longer exhibits statistical significance in the direct channel. This result suggests that, under the presence of agglomeration effects and, thus, under the threat of a higher number of COVID-19 cases within a given local administrative unit (LAU), it is more likely to be observed complementarity (i.e. learning) effects between interconnected municipalities (i.e. local communities considered neighbors from each other by the optimal spatial weights matrix). Moreover, one can observe that the direct channel turns out to be characterized by a significant, positive and short-term effect of VI6EldIPSS on the dependent variable. One should also highlight that, differently from the benchmark exercise, all the dependence parameters are statistically significant, which suggests that spatial-time dependence effects should not be neglected. In the context of this extension, the change of sign in φ from the first to the second case is explained by the small size of each Portuguese municipality and the subsequent presence of agglomeration effects when the population density is taken into account for the target's definition.
‡‡For this computation, we consider a total number of 25 input variables. For the sake of brevity, details regarding PCA can be provided upon request to the author.
Table A5 in Supplementary Appendix allows to confront dependence and marginal effects that prevail only in municipalities belonging to the metropolitan areas with those observed in the remaining municipalities of mainland Portugal. This geographic segmentation is useful because it allows to distinguish the magnitude and statistical significance of dependence and marginal effects in spatial units with a high risk of contagion (i.e. municipalities of the MAP and MAL) compared to those observed in regions with a lower risk of contagion (i.e. municipalities that do not belong to the MAP and MAL). Regardless of the geographical segmentation, results indicate that both time and spatial dependence effects are statistically significant. Time dependence effects exhibit almost the same magnitude, despite being slightly higher in inland municipalities. Differently, spatial dependence effects are much stronger in municipalities belonging to both metropolitan areas, which suggests the need to apply differentiated measures to reduce the contagion risk in these spatial units.
The statistical significance of covariates in municipalities belonging to MAP and MAL is predominantly observed in the short run, which suggests that the future evolution of COVID-19 cases in this group of municipalities is more unpredictable. In both metropolitan areas, results show that internal consumption has a significant, positive and direct effect on the monthly variation of the cumulative number of new confirmed COVID-19 cases. However, differently from the benchmark exercise, the statistical significance is only observed in the short run. Notwithstanding, the most important aspect indicates that the degree of openness to foreign markets increases the number of COVID-19 cases in the direct channel. Furthermore, the relation is elastic and this value duplicates in the long run. On the one hand, this result reveals that municipalities belonging to MAP and MAL constitute entry gates for the new coronavirus due to their international links. On the other hand, this result must be taken with the utmost seriousness and, in similar vein to the outcome related to spatial dependences, it urges for a paradigm shift in both metropolitan areas. Furthermore, the representative covariate of trade openness to foreign markets also exhibits a positive and significant indirect effect only in the short-term, which suggests that this explanatory variable justifies the spread of COVID-19 within the country's metropolitan areas.
Regarding municipalities outside MAP and MAL, one should highlight the statistical significance of the negative effect of VI3Mob and VI6EldIPSS on the dependent variable in the indirect channel, both in the short and long run equilibria. These effects, which are complementary since they reflect the learning capacity of neighboring municipalities, show the need for low density territories (LDTs) to transit towards the promotion of transparency in public policies of local administration and strengthen intermunicipal institutional cooperation mechanisms in a context of co-opetition. We highlight the estimated coefficient of VI6EldIPSS, according to which an increase of 1% in the number of pensioners per SSPI or, economically speaking, an improvement in the healthcare provided to the elderly population in a given inland municipality allows to reduce by a magnitude of around 33% the monthly variation in the cumulative number of new confirmed cases of COVID-19 in neighboring peers.
Insofar, EC (2022) identified three barriers for a sustainable development of the EU green bond market:
1. Absence of agreement on a common definition of green projects and green bonds;
2. Complex review procedures for green bonds; and
3. Lack of projects and assets for investment.
At the internal level, EC (2022) reveals problems for investors (e.g., difficulties to identify high-quality green bonds) and issuers (e.g., additional costs of issuing green bonds due to market fragmentation, uncertainty around green assets and reputational risks). At the external level, EC (2022) clarifies possible issues concerning heterogeneity, the lack of transparency and possible conflicts of interest. These sources of concern can lead to serious consequences such as:
1. Potential market disruption and reputational damage from greenwashing incidents;
2. Lack of supply of green bonds; and
3. Redundant effects due to the lack of investments being assigned to projects with a substantial impact on society.
In terms of benchmarking practices, two market standards have emerged:
● Green Bond Principles (GBPs) of the International Capital Market Association (ICMA);
● Climate Bond Standard (CBS) of the Climate Bonds Initiative (CBI).
The former has less strict requirements than the later and, thus, it dominates the market. Initially established in 2014 by a consortium of investment banks, GBPs have been monitored by the ICMA, and its practical guidelines were updated in June 2021. Although Next Generation EU green bonds are aligned with GBPs, the EC legislative proposal to regulate the EU green bond market is absent of a clear definition of green economic activities and only recommends a third-party external review. These two elements constitute key differences compared to the GBS emanated from the CBI, which has stricter requirements and, thus, fewer users. In particular, the CBI includes a taxonomy with screening criteria to define green economic activities and requires green bonds to be certified by approved external reviewers. In 2020, about a quarter of green bonds worldwide were issued under the CBI standard. In 2015, the People's Bank of China (PBoC) issued the first set of guidelines defining criteria for green bond projects and a taxonomy in the form of a Green Bond Endorsed Project Catalogue. In April 2021, the PBoC issued a new catalogue of projects considered eligible for green bond issuance, which came into force on 1 July 2021.
The 2022 EC legislative proposal − EU GBS − aims to establish an official EU voluntary standard for green bonds aligned with the EU taxonomy for sustainable finance based on a registration system and supervisory framework for external reviewers of EU green bonds. EC (2022) clarifies that this regulatory framework pretends to introduce uniform requirements for both EU and non-EU issuers (e.g., corporate issuers, sovereign issuers, financial institutions) that pretend to call European green bond (EuGB) to their bonds. The main requirement consists of being aligned with the EU taxonomy given that issuers should allocate 100% of funding to economic activities contemplated in the EU taxonomy before reaching the bond's maturity. This new standard is expected to finance long-term projects − up to 10 years − and help issuers on their green transition. EU GBS also allows for partial grandfathering where, in case of a change in the EU taxonomy technical screening criteria (TSC) after the bond issuance, issuers can make use of preexisting criteria for 5 additional years. In terms of transparency, issuers can initially report their commitment with the EU voluntary standard and only then express the aggregate environmental impact of their projects. Additionally, these disclosures are expected to suffer post-issuance assessments conducted by external reviewers, which must ensure compliance with:
● EU GBS regulation; and
● EU taxonomy.
The European Securities and Markets Authority (ESMA)'s supervisory role consists of analyzing complaints, impose fines and, if necessary, withdraw registration. The 2022 EC legislative proposal also gives some degree of flexibility for sovereign issuers, namely:
● Public subsidy programs and tax-relief programs are exempted from project-by-project assessment and the external reviewer only needs to assess the alignment of a program's terms with the EU taxonomy; and
● Sovereign issuers can use state auditors as external reviewers, which are exempted from the registration's system.
Recent months have served as a reminder that:
● Work and home lives are interconnected; and
● Health-related uncertainty should be in the forefront of public and private sector priorities.
Meanwhile, the coronavirus pandemic placed health disparities and increased social stratification. As the market and regulators begin to coalesce on the demand for sustainable strategies and investments across ESG criteria, the healthcare sector has a key opportunity to unlock value. Results of the present research, in particular the fact that only social factors have influential power on the set of dependent variables considered to capture health-related uncertainty, are important to expose a potential source of market failure: the inability of E, S and G criteria to reduce societal health-related uncertainty and, because of it, their inadequacy to leverage the green bond market. This then suggests the need of being not only concerned with ESG enforcement at the firm level, but also requires to ensure that this trend occurs at the community level or, equivalently, in a broader and integrated context where the so-called Quintuple Helix (i.e. universities, government, civil society and the environment in addition to industry) should have a key and active role in the EU green bond market's growth. This can be achieved by balancing firm-specific financing strategies and processes with community-based metrics in order to meaningfully decrease health-related uncertainty and improve performance outcomes at the regional level.
Intuitively, this argumentation suggests that the level of regional development observable in Europe must influence the growth of the EU green bond market. Otherwise, any attempt of obtaining funding through green bond issuance faces the risk of not having a distinctive character compared to traditional financial operations that took place in the past. It is then essential to understand that ESG criteria should contain a broader scope than being limited to business organizations. However, insofar, the 2022 EC legislative proposal is absolutely silent on the need for an alignment between the EU green bond market's growth and ESG criteria's compliance at the local community level, as the scope of EU GBS is restricted to firm-level measures.
For a mission-driven industry like healthcare, with many institutions organized as not-for-profit entities, the social element of ESG criteria has always been revelant. Currently, bond holders and stakeholders interconnect social determinants and corporate operations to understand how these metrics affect business outcomes. While the regulatory framework on for-profit institutions has been mostly concerned with environmental goals, it is now expected to incorporate alternative domains holding a local character (e.g., access to healthcare, justice, food equity, affordable housing). As stakeholders examine this multilevel relation, efforts should persist to review basic factors (e.g. patient access, quality and distribution of care services). In addition to financial reporting requirements mandated for not-for-profit entities, stakeholders have been focused on social issues through charity care policies. Since the main result of this study reveals that the social dimension has a significant, direct and permanent effect on health-related uncertainty, it is mandatory to consider a holistic and integrated view where the assessment of ESG practices should accommodate the territorial dynamics around the company. This step is crucial to understand the full impact of a firm's operational activities on local communities.
From a theoretical point of view, Agliardi and Agliardi (2019) develop a mathematical model that serves as a reference to explain the formation and dynamics of green bond prices and the so-called greenium. Their equilibrium results suggest that the improvement in credit quality reduces the cost of capital for green bond issuers, and that governmental tax-based incentives jointly with the increase in investors' green awareness have a significant role in leveraging the green bonds market. Additionally, Aleksandrova-Zlatanska and Kalcheva (2019) examine alternatives for financing municipal investments related to climate change, the so-called subnational pooled financing mechanisms, which have already gained some popularity in Western Europe but are not yet properly understood in Eastern Europe. The authors claim that such a public policy action can boost the EU green bond market.
In turn, the empirical literature tends to be focused on confronting returns of green bonds with counterfactuals (i.e. non-green bonds). In their analysis of attractive risk–return profiles compared to conventional (i.e. non-green) bonds, Hachenberg and Schiereck (2018) confirm that rating classes AA–BBB of green bonds as well as the full sample trade marginally tighter for the respective period compared to non-green bonds of the same issuers. Using a propensity score matching approach, Gianfrate and Peri (2019) analyze 121 European green bonds issued between 2013 and 2017 to find that green bonds are more financially convenient than non-green ones. Moreover, they find that the advantage is larger for corporate issuers, and it persists in the secondary market. Laborda and Sánchez-Guerra (2021) examine the increasing importance of green, social and sustainable bonds in the EU financial market to confirm that the announcement of a green bond has a positive market reaction by increasing the return on shares of green bond issuing.
Fatica et al. (2021) identify a premium for green bonds issued by supranational institutions and corporate firms, but no yield differences in case of issuances by financial institutions. They also confirm that banks issuing green bonds reduce lending towards carbon-intensive sectors, but limited to the loan amounts granted in the role of lead bank in the deal. As a result, the authors claim that this mixed evidence explains the absence of a green premium for financial issuers. Leitao et al. (2021) assess the effect of green bonds, conventional bonds and energy commodities on the behavior of the cap-and-trade European Union carbon market using a Markov-switching econometric model. They empirically confirm the presence of non-linearities in the dynamic behaviour of S&P Green and Sol Green (i.e. green bonds) in regimes of high and low volatility. Differently, S&P Agg and energy commodities (i.e. conventional bonds) tend to exhibit a decreasing value particularly in regimes of high volatility. Dan and Tiron-Tudor (2021) explore the impact of environmental, social, governance and macroeconomic indicators on the level of EU green bond issues in the period 2014–2019 and identify that rating, ESG index, fiscal balance, inflation rate and population have a significant impact and lead to a higher volume of green bond issuance. Rannou et al. (2021) evaluate the interconnection between the European carbon and green bond markets from the lens of the European energy firms' trading activity between 2013 and 2020 by considering two segments of the EU carbon market: short-term hedging (i.e. with speculative purposes) and long-term hedging (i.e. without speculative purposes). They show that energy firms have used the green bond market as a complement (substitute) to the carbon futures market in order to leverage their speculative (long-term hedging) activities before (after) 2018, respectively. Accordingly, this reflects a change in the behavior of European energy firms, which tend to leave the carbon market to become engaged in the transition to clean energy production systems.
Jakubik and Uguz (2021) assess whether green bond policies introduced by European insurance companies have a positive impact on their equity prices using a sample of listed (re)insurers in Europe that covers monthly data for the 2012–2019 period. Interestingly, there is no empirical confirmation of a positive effect of green policies, despite market investors positively price the issuance of green bonds and green funds. Taghizadeh-Hesary et al. (2021) confirm that the green bond market in Asia tends to exhibit higher returns, but also higher risk and heterogeneity. Since the Asian green bond market is dominated by the banking sector and knowing that bonds issued by banks tend to show below-average returns, the authors claim the need of measures to increase the rate of bond returns issued by the banking sector through the adoption of tax spillovers. Using a sample of 217 corporate public companies, Flammer (2021) shows that green bonds are effective in improving companies' environmental footprint.
Han and Li (2022) explore the role of green bonds in asset allocation using a dynamic mean-CVaR approach by confronting the performance of portfolios including green bonds with that of portfolios including conventional bonds in the USA and European markets. They show that portfolios with green bonds outperform portfolios with conventional bonds in terms of risk-adjusted returns in the majority of cases in both markets and justify that the benefit of green bonds stems from the increase in the returns and the decrease in the volatility for most cases. Cicchiello et al. (2022) inspect determinants affecting firms' choice between green or conventional bond issuance during 2015–2020 by resorting to a discrete choice analysis. Their results indicate that different issuer and corporate governance characteristics (i.e. current ratio, long debt, independent director) significantly affect the decision between issuing either green or conventional bonds. They also confirm that the combined effect of board gender diversity and debt maturity structure is positively related to green bond issuance. Jankovic et al. (2022) analyze green bond transparency by applying a panel data analysis to EU government green bonds. Their results confirm the significant effect of transparency on green bond yields in Europe.
Bearing in mind that the sovereign green bond market has been growing rapidly worldwide, Dell'Atti et al. (2022) analyze the empirical response of the stock and credit default swap (CDS) market to green bond issuance by 10 EU countries during the period 2016–2021. By considering that the issuance of a green bond is regarded by investors as reflecting the value-enhancing and risk-reducing behavior of EU countries, they confirm that the issuance of sovereign green bond provides a strong signal of the country's commitment to a low-carbon economy by means of increasing its social and reputational benefits, particularly during the pandemic crisis. Consequently, despite holding a different focus, this study shall be considered the one whose findings are closest to ours. According to Dell'Atti et al. (2022), the reaction of stock and CDS market is driven by several factors such as bond and country-specific characteristics, and their main conclusion is that the sovereign issuance of green bonds acts as a mitigation mechanism for a country's systemic risk.
Recently, Gilchrist et al. (2021) provide a systematic literature survey with a focus on the determinants and the potential benefits of corporate engagement in environmentally responsible practices in the context of green bonds and green loans. They show that past studies have discovered that environmentally responsible practices not only enhance shareholder value, but also the value accrued to nonfinancial stakeholders. In another literature review, Bhutta et al. (2022) emphasize that favorable regulatory environment and improvement in disclosure quality are essential factors for the growth of green bonds. From the above literature, we identified at least four debilities that the present study helped to overcome:
1. The excessive narrow vision on identifying drivers behind green bond issuance – differently from the existing literature, this study takes as a given the main message of recent EU official reports that COVID-19 is the causal effect of green bond issuance in Europe, so that the research goal is to assess determinants of COVID-19 propagation over time and space (i.e. the source behind the exponential growth observed in the EU green bond market);
2. The non-proportional focus on the role of the firm – differently from the existing literature, this study is focused on municipalities, thus pooling different firms according to their geographical location and empirically testing the theoretical idea clarified in Aleksandrova-Zlatanska and Kalcheva (2019);
3. The rampant use of standard econometric approaches – differently from the existing literature, this study uses spatial econometrics and machine learning models to estimate drivers of health-related uncertainty; and
4. The absence of concern with endogeneity – differently from the existing literature, this study provides a deep analysis of endogeneity, including in the context of spatial econometrics, which constitutes a technical requirement that this strand of literature is currently missing.
Despite the progress to enhance data availability, efforts are needed to strengthen ESG practices. Knowing that financial markets are progressing in heterogeneous speeds and directions, at least four steps are useful to promote a global consistency, avoid market fragmentation and ensure that the green bond market can efficiently support long-term value and sustainable growth:
1. Terrain-driven ESG criteria to improve stakeholder engagement in the EU green bond market;
2. Consistency, comparability and quality of metrics, both at the company and regional levels;
3. Level-playing field through the proper report, label and communication of ESG criteria;
4. Transparency on methodologies of established ESG ratings and indices for large and small issuers;
5. Within the landscape of green bonds, giving primacy to the social bond issuance.
An implicit argument is that enhancing ESG practices requires a greater involvement of investors and stakeholders, which has accentuated the persistence of metric inconsistencies and comparison risks. Additional efforts are needed to ensure that ESG practices do not give rise to market fragmentation. In order to strenghen the integrity and trust in the EU green bond market, there is scope for improving awareness and discuss solutions to promote consistency and transparency, while ensuring their alignment with benchmarking practices. Given the abundance of ESG information being disclosed through a variety of public and private entities, ESG rating agencies can play a relevant role to improve comparability through structured assessments based on rigorous methodologies. Since ESG practices over financial materiality are still at the early stage of development, ESG rating agencies are important players to extract value from ESG disclosure. However, their evaluations can give rise to several challenges, namely:
● Outputs across different ESG rating agencies can have a low degree of correlation;
● Sharp differences with respect to subcategories, metrics, weighting schemes and subjective judgment contribute to undermine comparability; and
● Rebalancing methodologies can obfuscate the comparison of ratings across industries.
Consequently, ESG rating agencies have room for improvement in the following managerial areas:
● Enhance the quality of information by explaining frameworks, subcategories and metrics;
● Clarify weighting schemes applied to metrics − including subjective judgment − to obtain final scores and disclose the relationship between ESG criteria and financial materiality, while promoting andragogy on their methodologies;
● Explain which elements distinguish ESG portfolio investment results from those yielding with a traditional portfolio.
This study demonstrates that health-related uncertainty has a spatial dimension that needs to be managed through a mix of complementary responses. More vulnerable regions and populations (e.g., urban areas) are expected to be harder affected than others. As recognized by the empirical analysis, differentiating socioeconomic factors is a key action that policymakers should not jeopardize (OECD, 2020). Many large cities have strong international linkages, which may define entry points of viral infections. Yet, rural areas can experience this event as well, in particular those characterized by a high percentage of elderly population living in SSPIs. As Iacobucci (2020) advances, health-related uncertainty can be quickly verified in large urban areas due to proximity effects if protective measures are not promptly implemented. Moreover, health-related uncertainty depends on the health system's capacity and pre-existing conditions of individuals (e.g., arterial hypertension, type I and II diabetes), which tend to be correlated with income and education. A potential risk in local governance policies is that these may be strictly focused on short-term priorities. Long run effects should be considered in the immediate response to health-related uncertainty to ensure that the demographic, social and economic resilience of each LAU is not compromised. Most local authorities were underprepared for the coronavirus pandemic due to several reasons, namely:
● Underestimation of risks;
● Absence of management plans for pandemic crisis, lack of essential equipment (e.g., ventilators);
● Reduced public investment in the endowment of capacity and quality improvement of healthcare;
● Structural debilities in the provision of public goods and services (e.g., water distribution and sanitation, waste collection, cleaning of streets, hygiene of public spaces and public transport, maintenance of public safety, delivery of administrative services with fast time-to-market responses).
Notwithstanding, the coronavirus pandemic led local authorities to take initiatives in areas not belonging to their primary scope, either mandated by the central government (e.g., responsibility on the coordination of basic education plans) or voluntarily (e.g., allocation of public spaces for drive-testing) (OECD, 2020). Hence, policy recommendations transversely applied to all the municipalities include:
1. Reinforce vertical coordination links and top-down policies.
2. Improve horizontal co-opetition and intermunicipal communication.
The empirical analysis also confirms that a one-size-fits-all approach may not be optimal due to the heterogeneous behavior of the first wave of COVID-19 in Portugal. The need to reduce health-related uncertainty is an opportunity to implement efficient and differentiated strategies, where stricter ones are expected to be imposed in the most affected regions (Capello and Faggian, 2020). Main targeted actions to reduce health-related uncertainty in LDTs include:
1. Manage exit barriers through massive testing and social distancing (WHO, 2020).
2. Boost the smart city's idiosyncrasy by strengthening a new era of digital governance (Vanolo, 2014; Fritsch and Kublina, 2018).
In turn, main actions focused on core regions include:
1. Tracing outbreaks, transmission chains and symptomatology at the local level (Camagni, 2017).
2. Assessing the impact on local public finance, fostering more flexible administrative procedures and discussing the need for regionalization and decentralization of competences from the central government (Boschma, 2005).
In light of the fast growth of assets under management that can be eligible as ESG practices, regulatory authorities have begun to assess forms of sustainable finance, with increasing focus on taxonomies and oriented marketing to investors. Results of this study reinforce that policymakers need to reinforce EGS practices in several ways, namely:
● Establish a transversal taxonomy to clarify and simplify the meaning of green finance;
● Benchmark the disclosure of ESG criteria and green products in corporate and financial services;
● Coordinate EGS rating agencies to make ESG practices more transparency, consistent and resilient to to the free will of subjective opinions.
Other sources of concern for further consideration include:
● Current opacity of subjective elements in ESG scoring;
● Improvement of the alignment between materiality and performance;
● Overcome existing market bias;
● Transparency of ESG products, which should be aligned with sustainability and social returns;
● Increase public engagement; and
● Internalize the geographical context where firms are inserted.
Despite the effort to provide a valuable contribution, this study is not exempted from limitations. Due to the scarcity of available data, it is difficult to develop an empirical study where the dependent variable materializes objective metrics of the EU green bond market. Demographic characteristics were also disregarded as they are reported annually, but the period of analysis is only 7 months. Another limitation is the sample size, which is justified by the need to perform a statistical inference based on real data covering the time period corresponding to the highest degree of health-related uncertainty. Alternative empirical approaches (e.g., spatial Tobit regression, spatial binary classification analysis) can be considered in subsequent works. Future research can also use deep learning artificial neural networks to perform a policy evaluation of different inputs on the target and investigate the impact of health-related uncertainty on different economic sectors.
Given the recent growth in the green bond market and knowing that this boom is explained by the coronavirus pandemic that has affected the world since December 2019, this study identifies which E, S or G criterion has influential power on health-related uncertainty at the societal level. To achieve this purpose, a dynamic spatial econometrics regression analysis is applied to 278 Portuguese municipalities covering the time period between March 2020 and September 2020. Although the number of new confirmed COVID-19 cases is used as a proxy of health-related uncertainty in the benchmark exercise, robustness checks consider a health-related uncertainty index and a relative measure of COVID-19 as dependent variables, whose results are qualitatively similar to those yielding in the initial analysis.
Overall, results indicate that environmental, governance and economic factors do not have explanatory power on health-related uncertainty. Instead, only a covariate that represents the degree of social proximity exhibits a significant impact on health-related uncertainty. More specifically, the social dimension has a direct, positive and permanent effect on the spread of health-related uncertainty. Moreover, their relation is inelastic and progressive given that a 1% rise in internal consumption by means of a face-to-face interaction between buyer and seller increases the number of new confirmed COVID-19 cases by 0.47% (0.72%) in the short (long) run, respectively.
Although the results are related to a single European country, Portugal has become a breeding ground for startups and new ideas, being currently a leading country in the retention of businesses that reached Unicorn status. Intuitively, this means that it is a country able to absorb a substantial part of the funds coming from social bond issuance in Europe. Therefore, results of this study can explain, at least partially, why the EU is taking the lead in the global green bond market. While the old continent may not be yet prepared to deal with health-related uncertainty, the social dimension of the EU green bond market has a significant, positive and direct effect on health-related uncertainty. Consequently, financing projects concerned with this specific domain is an optimal strategy to overcome other epidemiological events and ensure social welfare gains in the future. Differently, the lack of statistical significance identified in environmental and governance criteria suggests the need to enhance policy measures centered on these two dimensions in order to foster an even greater growth of the EU green bond market. As such, the study is finalized by presenting several theoretical, managerial, public policy and regulatory recommendations aimed at improving market failures related to these two dimensions of the EU green bond market.
V. M. Ribeiro acknowledges financial support from the ongoing project DSAIPA-CS-0086-2020 led by Fernando Lobo Pereira, who died suddenly in June 2022. This study is a tribute to the memory of this brilliant researcher. His absence of social prejudices, great intellect, dedication, guidance and ability to motivate will never be forgotten. See you one day, dear Professor, colleague and friend! In the opposite prism, this study is also dedicated to my son Gabriel Resende Ribeiro, who was born in February 2022. I hope you have the chance to live in a better world, in a greener world, in a world where the social dimension is not marginalized. V. M. Ribeiro also appreciates comments and suggestions from three anonymous reviewers, Editor-in-Chief, Editor-in-Charge, Assistant Editor and English Editor, which substantially improved the quality of the final version of this manuscript.
V. M. Ribeiro declares that there are no conflicts of interest in this study.
Supplementary content including code is available at the GitLab's personal page of the author (https://gitlab.com/vmsrib).
Notes on Table 1
1. VD2dInfCov captures the variation in the accumulated number of new confirmed COVID-19 cases between period t and period t+1 in municipality i. Data related to the number of deaths used in the ESDA comes directly from the mortality surveillance platform SICO-eVM, which is available https://evm.min-saude.pt/. Mortality data are extremely important to understand the impact of COVID-19 pandemic in Portugal. Data on mortality in Portugal are available at SICO-eVM, but without an associated data structure and dictionary. The dependent variable of this study is computed from a public data set available https://github.com/dssg-pt/covid19pt-data/blob/master/data_concelhos.csv. This online repository has two main sources: COVID-19 dashboard provided by the Portuguese DGH − that is no longer available online but whose location was hosted https://covid19.min-saude.pt/ponto-de-situacao-atual-em-portugal/ − and ESRI Portugal database available https://esriportugal.maps.arcgis.com/home/item.html?id=803d4c90bbb04c03999e65e5ce411cf8#data. It is important mentioning that some data contain errors (e.g., accumulated number of new confirmed COVID-19 cases in period t+1 lower than that observed in period t in some municipalities), which was adequately corrected by the author for the purpose of performing the present analysis. It is considered that t=1 corresponds to accumulated values by 31−03−2020 deduced by the accumulated values by 28−02−2020 (which were zero), t=2 corresponds to accumulated values by 30−04−2020 deduced by the accumulated values by 31−03−2020, t=3 corresponds to accumulated values by 31−05−2020 deduced by the accumulated values by 30−04−2020, t=4 corresponds to accumulated values by 30−06−2020 deduced by the accumulated values by 31−05−2020, t=5 corresponds to accumulated values by 27−07−2020 deduced by the accumulated values by 30−06−2020, t=6 corresponds to accumulated values by31−08−2020 deduced by the accumulated values by 27−07−2020, t=7 corresponds to accumulated values by 28−09−2020 deduced by accumulated values by 31−08−2020.
2. VI6EldIPSS captures aging normalized by private health supporting conditions, which is measured by the number of pensioners divided by the number of SSPIs at the contemporaneous period t+1 in municipality i.
3. VI3Mob represents an air pollution index at the contemporaneous period t+1 in municipality i. Data were available https://qualar1.apambiente.pt/qualar/index.php?page=2, but QualAR is constantly changing the online host location. We consider that t=1 corresponds to the air pollution index value on 31−03−2020, t=2 corresponds to the air pollution index value on 30−04−2020, t=3 corresponds to the air pollution index value on 31−05−2020, t=4 corresponds to the air pollution index value on 30−06−2020, t=5 corresponds to the air pollution index value on 27−07−2020, t=6 corresponds to the air pollution index value on 31−08−2020, t=7 corresponds the air pollution index value on 28−09−2020. The total number of stations does not cover the 278 municipalities, which means that this input is partially filled with positive values. For the purpose of performing the spatial econometric panel data analysis, Stata commands require to substitute missing values by zeros to ensure that the panel data set is strongly balanced in order to estimate coefficients. Air pollutants used for the construction of the air pollution index include O3 (maximum hourly value in μg/m3), NO2 (maximum hourly value in μg/m3), CO (max octo hour in mg/m3), SO2 (maximum hourly value in μg/m3), PM10 (daily mean in μg/m3), PM2.5 (daily mean in μg/m3) and C6H6 (in μg/m3).
4. VI5IntConsump captures the internal consumption and subsequent perception of the degree of 'buyer-seller' social interactions at period t in municipality i, which is measured by the amount of national withdrawals in ATMs. Thus, one lag period is imposed relative to the time structure that prevails in the dependent variable. Monthly data on the number of retirement pensions and, thus, the number of pensioners are available http://www.seg-social.pt/estatisticas. Data on the number of active SSPIs or, similarly, IPSS (in Portuguese) in 2020 can be accessed http://www.seg-social.pt/documents/10152/13140219/Listagem_ipss/8371faa4-dea5-4c03-a47f-3446f1f4c6c3.
5. VI4ForeignOpen captures the degree of coverage between period t−1 and period t in municipality i, it corresponds to the percentage of the value of the import of goods that is covered by the value of the export of goods and it is given by the formula 100 × (statistical value of exports/statistical value of imports). Therefore, one lag period is imposed relative to the time structure that prevails in the dependent variable. The respective data are available www.ine.pt. INE directly collects this information, namely its department of statistics on International Trade.
6. VI1BusinInten captures the intensity of economic activities at period t in municipality i, which is measured by difference between the number of created legal collective organizations and similar entities (i.e. the birth of firms) and the number of dissolved legal collective organizations and similar entities (i.e. the death of firms). Therefore, a one-lag period is imposed relative to the time structure that prevails in the dependent variable. The respective data are available www.ine.pt. A 'legal collective organization' is an entity constituted by a group of individuals or by a heritage complex with a view to pursuing a determined common interest and to which the legal order attributes the status of a legal person (i.e. legal personality). They may be of public or private law. A 'similar entity' means that, for the purpose of registration in the Central File of Collective Persons of the General Directorate of Justice Policy, constitution and dissolution of legal persons and similar entities, all non-personalized services, entities or bodies whose registration is of interest, namely for the purpose of planning and management, are considered. These should have an economic activity in mind, which is a result of the combination of productive factors (e.g., labor, raw materials, equipment) with a view to the production of goods and services. Regardless of the productive factors that build the good or service produced, all the activities assume, in general terms, the entry of products (i.e. goods or services), an intermediate process in which added value is incorporated and the exit of products.
7. VI2ATourismFlow captures the variation in tourism inflows between period t−1 and period t in municipality i, which is measured by the value of domestic purchases through automatic payment terminals made by individuals whose source of provenance (i.e. origin) is a foreign country. Therefore, a one-period lag is imposed relative to the time structure that prevails in the dependent variable. The respective data are available www.ine.pt. In turn, INE obtain this information from the department of statistics from credit institutions and financial companies of Sociedade Interbancária de Serviços (SIBS), which is online available https://www.sibs.com.
[1] |
Adda J (2016) Economic activity and the spread of viral diseases: Evidence from high frequency data. Quart J Econ 131: 891–941. https://doi.org/10.1093/qje/qjw005 doi: 10.1093/qje/qjw005
![]() |
[2] |
Agliardi E, Agliardi R (2019) Financing environmentally-sustainable projects with green bonds. Environ Develop Econ 24: 608–623. https://doi.org/10.1017/S1355770X19000020 doi: 10.1017/S1355770X19000020
![]() |
[3] |
Aleksandrova-Zlatanska S, Kalcheva DZ (2019) Alternatives for financing of municipal investments — green bonds. Rev Econ Bus Stud 12: 59–78. https://doi.org/10.1515/rebs-2019-0082 doi: 10.1515/rebs-2019-0082
![]() |
[4] | Anselin L (1988) Spatial econometrics: Methods and models. Kluwer Academic: Boston, MA. ISBN: 90-247-3735-4 |
[5] | Anselin L, Syabri I, Kho Y (2010) GeoDa: an introduction to spatial data analysis, Hand Appl Spat Anal, Springer: 73–89. https://doi.org/10.1007/978-3-642-03647-7_5 |
[6] | APR (1986) Artigo 9 da Lei no. 44/86 da Série I do Diário da República no. 225/1986 de 1986-09-30, 2779-2783. Available from: https://dre.pt/application/conteudo/221696. |
[7] | Barnes SR, Beland LP, Huh J, et al (2020) The Effect of COVID-19 Lockdown on Mobility and Traffic Accidents: Evidence from Louisiana. GLO Discussion Paper. Available from: https://econpapers.repec.org/paper/zbwglodps/616.htm. |
[8] |
Barmby T, Larguem M (2009) Coughs and sneezes spread diseases: An empirical study of absenteeism and infectious illness. J Health Econ 28: 1012–1017.https://doi.org/10.1016/j.jhealeco.2009.06.006 doi: 10.1016/j.jhealeco.2009.06.006
![]() |
[9] |
Battese GE, Coelli TJ (1992) Frontier production functions, technical efficiency and panel data: with application to paddy farmers in India. J Prod Analy 3: 153–169. https://doi.org/10.1007/BF00158774 doi: 10.1007/BF00158774
![]() |
[10] |
Battese GE, Coelli TJ (1995) A model for technical inefficiency effects in a stochastic frontier production function for panel data. Empirical Econ 20: 325–332. https://doi.org/10.1007/BF01205442 doi: 10.1007/BF01205442
![]() |
[11] |
Bell A, Jones K (2015) Explaining fixed effects: Random effects modeling of time-series cross-sectional and panel data. Pol Sci Res Meth 3: 133–153. https://doi.org/10.1017/psrm.2014.7 doi: 10.1017/psrm.2014.7
![]() |
[12] | Bilgin NM (2020) Tracking COVID-19 Spread in Italy with Mobility Data. SSRN 3585921. Available from: https://econpapers.repec.org/paper/kocwpaper/2012.htm |
[13] |
Boshcma R (2005) Proximity and innovation: a critical assessment. Reg Stud 39: 61–74. https://doi.org/10.1080/0034340052000320887 doi: 10.1080/0034340052000320887
![]() |
[14] |
Bhutta US, Tariq A, Farrukh M, et al (2022) Green bonds for sustainable development: Review of literature on development and impact of green bonds. Tech For Soc Change 175: 121378. https://doi.org/10.1016/j.techfore.2021.121378 doi: 10.1016/j.techfore.2021.121378
![]() |
[15] |
Camagni R (2017) Regional competitiveness: towards a concept of territorial capital. Sem Stud Reg Urb Econ 1: 115–131. https://doi.org/10.1007/978-3-319-57807-1_6 doi: 10.1007/978-3-319-57807-1_6
![]() |
[16] |
Capello R, Faggian A (2005) Collective learning and relational capital in local innovation processes. Reg Stud 39: 75–87. https://doi.org/10.1080/0034340052000320851 doi: 10.1080/0034340052000320851
![]() |
[17] | Caselli M, Fracasso A, Scicchitano S (2020) From the lockdown to the new normal: An analysis of the limitations to individual mobility in Italy following the Covid-19 crisis. GLO Discussion Paper. Available from: https://www.econstor.eu/handle/10419/225064 |
[18] | CBI (2022) H1 Market Report: Green and other labelled bond volumes reach $417.8bn in first half of 2022. Available from: https://www.climatebonds.net/resources/press-releases/2022/08/h1-market-report-green-and-other-labelled-bond-volumes-reach-4178bn |
[19] |
Choi BB, Lee D, Park Y (2013) Corporate social responsibility, corporate governance and earnings quality: Evidence from Korea. Corp Gov: Intern Rev 21: 447-–467. https://doi.org/10.1111/corg.12033 doi: 10.1111/corg.12033
![]() |
[20] |
Cicchiello AF, Cotugno M, Monferrà S, et al (2022) Which are the factors influencing green bonds issuance? Evidence from the European bonds market. Fin Res Let 50: 103190. https://doi.org/10.1016/j.frl.2022.103190 doi: 10.1016/j.frl.2022.103190
![]() |
[21] |
Coles JL, Daniel ND, Naveen L (2008) Boards: Does one size fit all? J Fin Econ 87: 329–356. https://doi.org/10.1016/j.jfineco.2006.08.008 doi: 10.1016/j.jfineco.2006.08.008
![]() |
[22] |
Cornwell C, Schmidt P, Sickles RC (1990) Production frontiers with cross-sectional and time-series variation in efficiency levels. J Econometrics 46: 185–200. https://doi.org/10.1016/0304-4076(90)90054-W doi: 10.1016/0304-4076(90)90054-W
![]() |
[23] |
Crowley F, Doran J (2020) Covid-19, Occupational Social Distancing and Remote Working Potential: An Occupation, Sector and Regional Perspective. Reg Sci Pol Pract: 1211–1234. https://doi.org/10.1111/rsp3.12347 doi: 10.1111/rsp3.12347
![]() |
[24] |
Dan A, Tiron-Tudor A (2021) The determinants of green bond issuance in the European Union. J Risk Fin Manag 14: 446. https://doi.org/10.3390/jrfm14090446 doi: 10.3390/jrfm14090446
![]() |
[25] | Davidson R, MacKinnon JG (1993) Estimation and inference in econometrics 63. New York: Oxford University Press. https://doi.org/10.1017/S0266466600009452 |
[26] | Deboeck GJ (1994) Trading on the edge: neural, genetic, and fuzzy systems for chaotic financial markets. London: John Wiley and Sons. ISBN: 0-471-31100-6 |
[27] | Dell'Atti S, Tommaso C, Pacelli V (2022) Sovereign green bond and country value and risk: Evidence from European Union countries. J Intern Fin Manag Account: In press. https://doi.org/10.1111/jifm.12155 |
[28] | EC (2022) European green bonds A standard for Europe, open to the world. Available from: https://www.europarl.europa.eu/RegData/etudes/BRIE/2022/698870/EPRS_BRI(2022)698870_EN.pdf |
[29] | ECDPC (2018) European Center for Disease Prevention and Control Technical document - 2018 HEPSA (Health emergency preparedness self-assessment tool user guide). Stockholm: ECDC. Available from: https://www.ecdc.europa.eu/en/publications-data/hepsa-health-emergency-preparedness-self-assessment-tool-user-guide |
[30] | Elhorst JP (2017) Spatial Panel Data Analysis. Ency GIS 2: 2050–2058. Available fom: https://spatial-panels.com/wp-content/uploads/2017/07/Elhorst-Spatial-Panel-Data-Analysis-Encyclopedia-GIS-2nd-ed_Working-Paper-Version.pdf |
[31] | Engle S, Stromme J, Zhou A (2020) Staying at home: mobility effects of covid-19. Mimeo. Available from: https://papers.ssrn.com/sol3/papers.cfm?abstract_id=3565703 |
[32] |
Fang H, Wang L, Yang Y (2020) Human mobility restrictions and the spread of the novel coronavirus (2019-ncov) in China. J Public Econ 191: 104272. https://doi.org/10.1016/j.jpubeco.2020.104272 doi: 10.1016/j.jpubeco.2020.104272
![]() |
[33] |
Fatica S, Panzica R, Rancan M (2021) The pricing of green bonds: are financial institutions special?. J Fin Stab 54: 100873. https://doi.org/10.1016/j.jfs.2021.100873 doi: 10.1016/j.jfs.2021.100873
![]() |
[34] | Favero CA, Ichino A, Rustichini A (2020) Restarting the economy while saving lives under Covid-19. CEPR Discussion Paper No. DP14664. Available from: https://econpapers.repec.org/paper/cprceprdp/14664.htm |
[35] |
Firmino D, Elhorst JP, Neto RMS (2017) Urban and rural population growth in a spatial panel of municipalities. Reg Stud 51: 894–908. https://doi.org/10.1080/00343404.2016.1144922 doi: 10.1080/00343404.2016.1144922
![]() |
[36] |
Flammer C (2021) Corporate green bonds. J Fin Econ 142: 499–516. https://doi.org/10.1016/j.jfineco.2021.01.010 doi: 10.1016/j.jfineco.2021.01.010
![]() |
[37] |
Fritsch M, Kublina S (2018) Related variety, unrelated variety and regional growth: the role of absorptive capacity and entrepreneurship. Reg Stud 52: 1360–1371. https://doi.org/10.1080/00343404.2017.1388914 doi: 10.1080/00343404.2017.1388914
![]() |
[38] |
Gianfrate G, Peri M (2019) The green advantage: Exploring the convenience of issuing green bonds. J Clean Prod 219: 127–135. https://doi.org/10.1016/j.jclepro.2019.02.022 doi: 10.1016/j.jclepro.2019.02.022
![]() |
[39] | Glaeser EL, Gorback CS, Redding SJ (2020) How much does covid-19 increase with mobility? evidence from new york and four other us cities. National Bureau of Economic Research. Available from: https://www.nber.org/system/files/working_papers/w27519/w27519.pdf |
[40] | Godzinski A, Suarez-Castillo M (2019) Short-term health effects of public transport disruptions: air pollution and viral spread channels. Mimeo. Available from: https://econpapers.repec.org/paper/nsedoctra/g2019-03.htm |
[41] |
Greene W (2005) Fixed and random effects in stochastic frontier models. J Prod Analy 23: 7–32. doi: https://doi.org/10.1007/s11123-004-8545-1 doi: 10.1007/s11123-004-8545-1
![]() |
[42] |
Gilchrist D, Yu J, Zhong R (2021) The limits of green finance: A survey of literature in the context of green bonds and green loans. Sustainability 13: 478. https://doi.org/10.3390/su13020478 doi: 10.3390/su13020478
![]() |
[43] |
Hamilton JD, Waggoner DF, Zha T (2007) Normalization in econometrics. Econometric Rev 26: 221–252. https://doi.org/10.1080/07474930701220329 doi: 10.1080/07474930701220329
![]() |
[44] |
Hamilton JG, Genoff MC, Han PK (2020) Health‐Related Uncertainty. Wiley Ency Health Psych 305–313. https://doi.org/10.1002/9781119057840.ch80 doi: 10.1002/9781119057840.ch80
![]() |
[45] |
Han Y, Li J (2022) Should investors include green bonds in their portfolios? Evidence for the USA and Europe. Intern Rev Fin Analy 80: 101998. https://doi.org/10.1016/j.irfa.2021.101998 doi: 10.1016/j.irfa.2021.101998
![]() |
[46] |
Han PKJ, Klein WMP, Arora NK (2011) Varieties of uncertainty in health care: A conceptual taxonomy. Med Decis Making 31: 828–838. https://doi.org/10.1177/0272989X103939 doi: 10.1177/0272989X103939
![]() |
[47] |
Hancock AA, Bush EN, Stanisic D, et al (1988) Data normalization before statistical analysis: keeping the horse before the cart. Trend Pharma Sci 9: 29–32. https://doi.org/10.1016/0165-6147(88)90239-8 doi: 10.1016/0165-6147(88)90239-8
![]() |
[48] |
Hachenberg B, Schiereck D (2018) Are green bonds priced differently from conventional bonds?. J Asset Manag 19: 371–383. https://doi.org/10.1057/s41260-018-0088-5 doi: 10.1057/s41260-018-0088-5
![]() |
[49] |
Hsiang S, Allen D, Annan-Phan S, et al (2020) The effect of large-scale anti-contagion policies on the COVID-19 pandemic. Nature 584: 262–267. https://doi.org/10.1038/s41586-020-2404-8 doi: 10.1038/s41586-020-2404-8
![]() |
[50] |
Iacobucci G (2020) Covid-19: Deprived areas have the highest death rates in England and Wales. British Med J 369: 1. https://doi.org/10.1136/bmj.m1810 doi: 10.1136/bmj.m1810
![]() |
[51] |
Laborda J, Sánchez-Guerra A (2021) Green bond finance in Europe and the stock market reaction. Estud Economía Aplicada 39: 5. https://doi.org/10.25115/eea.v39i3.4125 doi: 10.25115/eea.v39i3.4125
![]() |
[52] | Lee YH, Schmidt P (1993). A production frontier model with flexible temporal variation in technical efficiency. The measurement of productive efficiency: Techniques and applications. 237–255. ISBN: 0-19-507218-9 |
[53] |
Lee LF (2004) Asymptotic distributions of quasi-maximum likelihood estimators for spatial autoregressive models. Econometrica 72: 1899–1925. https://doi.org/10.1111/j.1468-0262.2004.00558.x doi: 10.1111/j.1468-0262.2004.00558.x
![]() |
[54] |
Lee LF, Yu J (2016). Identification of spatial Durbin panel models. J Appl Econometrics 31: 133–162. https://doi.org/10.1002/jae.2450 doi: 10.1002/jae.2450
![]() |
[55] |
Lee DL, McCrary J, Moreira MJ, et al (2020) Valid t-ratio Inference for Ⅳ. Amer Econ Rev 112: 3260–3290. https://doi.org/10.1257/aer.20211063 doi: 10.1257/aer.20211063
![]() |
[56] |
Leitao J, Ferreira J, Santibanez‐Gonzalez E (2021) Green bonds, sustainable development and environmental policy in the European Union carbon market. Bus Strat Environ 30: 2077–2090. https://doi.org/10.1002/bse.2733 doi: 10.1002/bse.2733
![]() |
[57] | LeSage JP, Pace RK (2009) Introduction to Spatial Econometrics. Boca Raton, FL: CRC Press. https://doi.org/10.1201/9781420064254 |
[58] |
LeSage JP (2014) Spatial econometric panel data model specification: A Bayesian approach. Spat Stat 9: 122–145. https://doi.org/10.1016/j.spasta.2014.02.002 doi: 10.1016/j.spasta.2014.02.002
![]() |
[59] |
Litvinova M, Liu QH, Kulikov ES, et al (2019) Reactive school closure weakens the network of social interactions and reduces the spread of influenza. Proc Nat Acad Scie 116: 13174–13181. https://doi.org/10.1073/pnas.182129811 doi: 10.1073/pnas.182129811
![]() |
[60] |
Jalan J, Sen A (2020) Containing a pandemic with public actions and public trust: the Kerala story. Indian Econ Rev 1: 1–20. https://doi.org/10.1007/s41775-020-00087-1 doi: 10.1007/s41775-020-00087-1
![]() |
[61] |
Jakubik P, Uguz S (2021) Impact of green bond policies on insurers: evidence from the European equity market. J Econ Fin 45: 381–393. https://doi.org/10.1007/s12197-020-09534-4 doi: 10.1007/s12197-020-09534-4
![]() |
[62] |
Jankovic I, Vasic V, Kovacevic V (2022) Does transparency matter? Evidence from panel analysis of the EU government green bonds. Energy Econ 1: 106325. https://doi.org/10.1016/j.eneco.2022.106325 doi: 10.1016/j.eneco.2022.106325
![]() |
[63] |
Kelejian HH, Prucha IR (1998) A generalized spatial two-stage least squares procedure for estimating a spatial autoregressive model with autoregressive disturbances. J Real Est Fin Econ 17: 99–121. https://doi.org/10.1023/A:1007707430416 doi: 10.1023/A:1007707430416
![]() |
[64] |
Kelejian HH, Prucham IR (1999) A generalized moments estimator for the autoregressive parameter in a spatial model. Intern Econ Rev 40: 509–533. https://doi.org/10.1111/1468-2354.00027 doi: 10.1111/1468-2354.00027
![]() |
[65] | Khalatbari-Soltani S, Cumming RG, Delpierre C, et al (2020) Importance of collecting data on socioeconomic determinants from the early stage of the COVID-19 outbreak onwards. J Epidem Commun Health 1: 1–10. Available from: https://jech.bmj.com/content/74/8/620.info |
[66] |
Kreps DM, Wilson R (1982) Sequential equilibria. Econometrica 863–894. https://doi.org/10.2307/1912767 doi: 10.2307/1912767
![]() |
[67] |
Markowitz S, Nesson E, Robinson J (2019) The effects of employment on influenza rates. Econ Hum Biol 34: 286–295. https://doi.org/10.1016/j.ehb.2019.04.004 doi: 10.1016/j.ehb.2019.04.004
![]() |
[68] |
Maurer J (2009) Who has a clue to preventing the flu? Unravelling supply and demand effects on the take-up of influenza vaccinations. J Health Econ 28: 704–717. https://doi.org/10.1016/j.jhealeco.2009.01.005 doi: 10.1016/j.jhealeco.2009.01.005
![]() |
[69] |
McKnight PJ, Weir C (2009) Agency costs, corporate governance mechanisms and ownership structure in large UK publicly quoted companies: A panel data analysis. Quart Rev Econ Fin 49: 139–158. https://doi.org/10.1016/j.qref.2007.09.008 doi: 10.1016/j.qref.2007.09.008
![]() |
[70] | MFF (2020) Questions and answers about the effects of the coronavirus. Available from: https://vm.fi/kysymyksia-ja-vastauksia-koronaviruksen-vaikutuksista |
[71] |
Milani F (2020) COVID-19 Outbreak, Social Response, and Early Economic Effects: A Global VAR Analysis of Cross-Country Interdependencies. J Pop Econ 34: 223–252. https://doi.org/10.1007/s00148-020-00792-4 doi: 10.1007/s00148-020-00792-4
![]() |
[72] | Milusheva S (2017) Less bite for your buck: Using cell phone data to target disease prevention. Mimeo. Available from: https://www.semanticscholar.org/paper/Less-Bite-for-Your-Buck-3A-Using-Cell-Phone-Data-to-Milusheva/2ba1aa5c668f50990d269f48cbc9acf5b007e592 |
[73] |
Moran P (1950) Notes on continuous stochastic phenomena. Biometrika 37: 17–23. https://doi.org/10.2307/2332142 doi: 10.2307/2332142
![]() |
[74] |
Muttakin MB, Khan A, Azim MI (2015) Corporate social responsibility disclosures and earnings quality. Manag Audit J 30: 277–298. https://doi.org/10.1108/MAJ-02-2014-0997 doi: 10.1108/MAJ-02-2014-0997
![]() |
[75] | OECD (2020) The Territorial Impact of COVID-19: Managing the Crisis across Levels of Government. OECD Paris. Available from: https://www.oecd.org/coronavirus/policy-responses/the-territorial-impact-of-COVID-19-managing-the-crisis-across-levels-of-government-d3e314e1/ |
[76] |
Okoi O, Bwawa T (2020) How health inequality affect responses to the COVID-19 pandemic in Sub-Saharan Africa. World Devel 135: 105067. https://doi.org/10.1016/j.worlddev.2020.105067 doi: 10.1016/j.worlddev.2020.105067
![]() |
[77] |
Patel JA, Nielsen FBH, Badiani AA, et al (2020) Poverty, inequality and COVID-19: the forgotten vulnerable. Pub Health 183: 110. https://doi.org/10.1016/j.puhe.2020.05.006 doi: 10.1016/j.puhe.2020.05.006
![]() |
[78] |
Pepe E, Bajardi P, Gauvin L, et al (2020) COVID-19 outbreak response: a first assessment of mobility changes in Italy following national lockdown. Sci Data 7: 230. https://doi.org/10.1038/s41597-020-00575-2 doi: 10.1038/s41597-020-00575-2
![]() |
[79] | Persico C, Johnson KR (2020) Deregulation in a Time of Pandemic: Does Pollution Increase Coronavirus Cases or Deaths? Available from: https://ideas.repec.org/p/iza/izadps/dp13231.html |
[80] |
Pichler S, Ziebarth NR (2017) The pros and cons of sick pay schemes: Testing for contagious presenteeism and noncontagious absenteeism behavior. J Publ Econ 156: 14–33. https://doi.org/10.1016/j.jpubeco.2017.07.003 doi: 10.1016/j.jpubeco.2017.07.003
![]() |
[81] | PMFA (2008). Ministério dos Negócios Estrangeiros. Aviso n.º 12/2008, de 23 de janeiro, do Ministério dos Negócios Estrangeiros. Regulamento Sanitário Internacional. Available from: https://files.dre.pt/1s/2008/11/22600/0813508177.pdf |
[82] | PMH (2014) Ministério da Saúde. Programa Nacional de erradicação da Poliomielite: Plano de acção após erradicação. Norma nº017/2014 de 27/11/2014 - Direção-Geral da Saúde. Available from: http://www.aenfermagemeasleis.pt/2014/11/27/norma-dgs-programa-nacional-de-erradicacao-da-poliomielite-plano-de-acao-pos-eliminacao/ |
[83] |
Qiu Y, Chen X, Shi W (2020) Impacts of social and economic factors on the transmission of coronavirus disease 2019 (COVID-19) in China. J Pop Econ 1: 1–27. https://doi.org/10.1007/s00148-020-00778-2 doi: 10.1007/s00148-020-00778-2
![]() |
[84] |
Rannou Y, Boutabba MA, Barneto P (2021) Are Green Bond and Carbon Markets in Europe complements or substitutes? Insights from the activity of power firms. Energy Econ 104: 105651. https://doi.org/10.1016/j.eneco.2021.105651 doi: 10.1016/j.eneco.2021.105651
![]() |
[85] |
Rossman H, Keshet A, Shilo S, et al (2020) A framework for identifying regional outbreak and spread of COVID-19 from one-minute population-wide surveys, Nature Med 26: 634–638. https://doi.org/10.1038/s41591-020-0857-9 doi: 10.1038/s41591-020-0857-9
![]() |
[86] |
Santana R, Sousa JS, Soares P, et al (2020) The demand for hospital emergency services: trends during the first month of COVID-19 response, Port J Publ Health 38: 30–36. https://doi.org/10.1159/000507764 doi: 10.1159/000507764
![]() |
[87] |
Slusky D, Zeckhauser RJ (2018) Sunlight and protection against influenza. Econ Hum Biol 40: 100942. https://doi.org/10.1016/j.ehb.2020.100942 doi: 10.1016/j.ehb.2020.100942
![]() |
[88] |
Taghizadeh-Hesary F, Yoshino N, Phoumin H (2021). Analyzing the characteristics of green bond markets to facilitate green finance in the post-COVID-19 world. Sustainability 13: 5719. https://doi.org/10.3390/su13105719 doi: 10.3390/su13105719
![]() |
[89] |
Tian H, Liu Y, Li Y, et al (2020) An investigation of transmission control measures during the first 50 days of the COVID-19 epidemic in China. Sci 368: 638–642. https://doi.org/10.1126/science.abb6105 doi: 10.1126/science.abb6105
![]() |
[90] |
Vanolo A (2014) Smartmentality: The smart city as disciplinary strategy. Urb Stud 51: 883–898. https://doi.org/10.1177/00420980134944 doi: 10.1177/00420980134944
![]() |
[91] |
Varkey RS, Joy J, Sarmah G, et al (2020). Socioeconomic determinants of COVID-19 in Asian countries: An empirical analysis J Publ Affairs: e2532. https://doi.org/10.1002/pa.2532 doi: 10.1002/pa.2532
![]() |
[92] |
Weill JA, Stigler M, Deschenes O, et al (2020) Social distancing responses to COVID-19 emergency declarations strongly differentiated by income. Proc Nat Acad Sci 117: 19658–19660. https://doi.org/10.1073/pnas.2009412117 doi: 10.1073/pnas.2009412117
![]() |
[93] |
White C (2019) Measuring social and externality benefits of influenza vaccination. J Hum Resourc: 1118–9893R2. https://doi.org/10.3368/jhr.56.3.1118-9893R2 doi: 10.3368/jhr.56.3.1118-9893R2
![]() |
[94] | WHO (2020) 2019 Novel Coronavirus (2019 nCoV): STRATEGIC PREPAREDNESS AND RESPONSE PLAN. Available from: https://www.who.int/docs/default-source/coronaviruse/srp-04022020.pdf |
[95] | WHO (2020) Strategy Update. April 14, 2020. WHO Report. Available from: https://www.who.int/publications-detail-redirect/covid-19-strategy-update—14-april-2020 |
[96] |
Yilmazkuday H (2020) Stay-at-Home Works to Fight Against COVID-19: International Evidence from Google Mobility Data. J Hum Behav Soc Environ 31: 210–220. https://doi.org/10.1080/10911359.2020.1845903 doi: 10.1080/10911359.2020.1845903
![]() |
[97] |
Zhan C, Tse C, Fu X, et al (2020) Modelling and prediction of the 2019 Coronavirus Disease spreading in China incorporating human migration data. PLoS One 15: e0241171. https://doi.org/10.1371/journal.pone.0241171 doi: 10.1371/journal.pone.0241171
![]() |
[98] |
Zhang C, Chen C, Shen W, et al (2020) Impact of population movement on the spread of 2019-nCoV in China. Emerg Microb Infect 9: 988–990. https://doi.org/10.1080/22221751.2020.1760143 doi: 10.1080/22221751.2020.1760143
![]() |
![]() |
![]() |
![]() |
![]() |
1. | Ahmed Diab, Aref M. Eissa, ESG Performance, Auditor Choice, and Audit Opinion: Evidence from an Emerging Market, 2023, 16, 2071-1050, 124, 10.3390/su16010124 | |
2. | Bo Zhang, Rongbao Huang, Yiluan Liu, Liwei Wang, Yunpeng Chen, Effects of atmospheric particulate pollution on lung function of athletes, 2024, 252, 00139351, 118763, 10.1016/j.envres.2024.118763 | |
3. | Juzhang Feng, Sha Tang, Junhao Zhong, Can corporate environmental, social, and governance performance influence foreign institutional investors to hold shares? Evidence from China, 2024, 33, 0964-4733, 4310, 10.1002/bse.3708 | |
4. | Sergio Soza-Amigo, Jean Pierre Doussoulin, Regional Economic Development, Climate Change, and Work Force in a Gender Perspective in Chile: Insights from the Input–Output Matrix, 2024, 16, 2071-1050, 8692, 10.3390/su16198692 | |
5. | Xiangsong Ye, Longju Zhou, Zhiyong Wang, Spatial convergence characteristics of low carbon economy and economic growth quality: based on Guangdong urban data, 2024, 9, 2473-6988, 11660, 10.3934/math.2024572 |
Dimension | Acronym | Min | Max | Mean | Std Dev | Source (Definition) # |
Dependent variable | VD2dInfCov | 0 | 1 | 0.1380 | 0.2624 | PT DGH (1.) |
Environmental | VI3Mob | 0 | 1 | 0.0588 | 0.1567 | PT QualAR (2.) |
Social | VI6EldIPSS | 0 | 1 | 0.4209 | 0.3190 | PT SS (3.) |
VI5IntConsump | 0 | 1 | 0.2809 | 0.3158 | PT INE (4.) | |
Governance | VI4ForeignOpen | 0 | 1 | 0.1703 | 0.2353 | PT INE (5.) |
VI1BusinInten | 0 | 1 | 0.3516 | 0.2199 | PT INE (6.) | |
Economic | VI2ATourismFlow | 0 | 1 | 0.4970 | 0.1329 | PT INE (7.) |
Notes: superscript '#' stands for the need of reading the Supplementary Appendix for additional details. |
VD2dInfCov | VI3Mob | VI6EldIPSS | VI5IntConsump | VI4ForeignOpen | VI1BusinInten | VI2ATourismFlow | VIF | |
VD2dInfCov | 1 | |||||||
VI3Mob | 0.6577*** | 1 | 1.05 | |||||
VI6EldIPSS | −0.0264 | −0.1577*** | 1 | 1.03 | ||||
VI5IntConsump | −0.1266*** | −0.1935*** | −0.0056 | 1 | 1.06 | |||
VI4ForeignOpen | 0.0018 | 0.0262 | −0.0279 | −0.1551*** | 1 | 1.14 | ||
VI1BusinInten | 0.5776*** | 0.8307*** | −0.1652*** | −0.1481*** | 0.0602 | 1 | 2.65 | |
VI2ATourismFlow | 0.1057*** | 0.2540*** | −0.0513** | −0.0355 | −0.0013*** | 0.2527*** | 1 | 2.53 |
Notes: Symbol *** (**) [*] for 1% (5%) [10%] of significance level, respectively. Mean VIF of 1.57 is below the critical threshold 5, thus, confirming the absence of multicollinearity. |
Statistical test | Value |
BP LM test for random effects | 215.8200*** [1] |
LM spatial lag (Anselin) | 467.7729*** [1] |
LM spatial error (Burridge) | 297.0096*** [1] |
Robust LM spatial lag | 799.4608*** [1] |
Robust LM spatial error | 628.6976*** [1] |
Global Moran | 0.2499*** (17.3760) |
Global Geary | 0.7710*** (−12.8250) |
Global Getis-Ord | −0.2499*** (−17.3760) |
Moran error test | 17.2858*** (1199.6900) |
LM SAC (LMErr+LMLag_R) | 1096.4704*** [2] |
LM SAC (LMLag+LMErr_R) | 1096.4704***[2] |
Notes: z-statistics in parentheses and degrees of freedom in brackets. Symbol *** (**) [*] represents 1% (5%) [10%] of significance level, respectively. Results reveal significant spatial autocorrelation, which validates the transition towards spatial panel data models. BP LM stands for Breusch–Pagan Lagrangian multiplier. Since p<0.05, the null hypothesis of homoscedasticity is rejected and, thus, a random effects model is preferred to the POLS model. Therefore, heteroscedasticity needs to be controlled for by resorting to a dynamic panel data estimation. LM stands for Lagrangian multiplier. Since p<0.05, the null hypothesis regarding absence of spatial autocorrelation is rejected, so that a spatial model is preferred to the POLS model. |
Value | Spatial fixed effects | Time-period fixed effects | Spatial and time-period fixed effects |
F-statistic | F(283, 1656) = 18.7300*** | ||
Log Likelihood (null) | 22.4350 | −128.8935 | 1094.7750 |
Log Likelihood (model) | 171.1414 | 482.6482 | 1106.3600 |
AIC | −328.2829 | −951.3164 | −2198.7200 |
BIC | −289.2681 | −912.3017 | −2159.7060 |
Likelihood ratio test | 1247.4000*** [277] | 1870.4400*** [6] | |
Hausman test | 45.8600***[6] | ||
Notes: Degrees of freedom are within brackets. Symbol *** (**) [*] represents 1% (5%) [10%] of significance level, respectively. Since the F-statistic is significant sincep<0.05 for municipalities and time dummies, then the null hypothesis of homogeneity (pooling) is rejected at the 5% level. Thus, the LSDV (FE) model is most applicable since the data are favorably to the two-way fixed effects estimation. The inclusion of two-way fixed effects is also justified by the statistical significance observed in both likelihood ratio tests. Finally, the Hausman test indicatesp<0.05, which implies that the null hypothesis that the random effects model is preferred to the fixed effects model is rejected. |
Comparison | χ2 | p-value | AIC | BIC |
(1) SAR versus SDM | 14.5900 [6] | 0.0237 | ||
(2) SEM versus SDM | 13.5400 [6] | 0.0353 | ||
(3) SAR | −2811.1730 | −2766.5850 | ||
(4) SEM | −2817.9920 | −2773.4040 | ||
(5) SAC | −2903.1390 | −2852.9770 | ||
(6) SDM versus DSDM | 85.6200 [2] | 0.0000 | ||
(7) SAR versus DSAR | 94.1900 [2] | 0.0000 | ||
(8) DSAR versus DSDM | 39.3400 [6] | 0.0000 | ||
(9) SAR versus DSDM | 250.5100 [8] | 0.0000 | ||
(10) SAC versus DSDM | 292.0000 [14] | 0.0000 | ||
(11) SEM versus DSDM | 245.2100 [8] | 0.0000 | ||
Notes: Degrees of freedom are within brackets. Values obtained while holding the first order queen contiguity matrix fixed Abbreviation SAR stands for spatial autoregressive model, SDM stands for spatial Durbin model, SEM stands for spatial error, SAC stands for spatial autocorrelation model, DSDM stands for dynamic spatial Durbin model and DSAR stands for dynamic spatial autoregressive model. The interpretation of outcomes is given as follows. Under (1), one cannot reject the null hypothesis that SAR is preferred to SDM for a critical significance level of 5%. Under (2), one cannot rejected the null hypothesis that SEM is preferred to SDM for a critical significance level of 5%. Therefore, at this phase, the static SDM is excluded from being the appropriate option. To select between SAR, SEM and SAC models, AIC and BIC values can be checked in (3) – (5). One can infer that SAC is the optimal static model. Taking thereafter into account dynamic models, (6) shows that one can reject the null hypothesis that SDM is preferred to the DSDM for a critical significance level of 5%. Based on (7), similar conclusion is applied to the rejection of SAR relatively to DSAR for a critical significance level of 5%. According to (8), one can reject the null hypothesis that DSAR is preferred to DSDM for a critical significance level of 5%. Therefore, the main conclusion for dynamic panel data models is that DSDM is the optimal choice. Finally, (10) reflects the need to compare the SAC model against the DSDM from which one rejects the null hypothesis that SAC is preferred to DSDM. Two caveats are worth mentioning. First, spatial models should not be compared by transitivity (i.e. based on a circular argument) but rather directly on a two-by-two basis. Second, (9) and (11) are redundant due to (3)–(5). |
Criterion | First order QC | Second order QC | hird order QC | First order RC | Second order RC | Third order RC |
AIC | −2587.8640 | −2522.5570 | −2468.8490 | −2582.2790 | − 2517.7480 | −4187.071 |
BIC | −2501.1540 | −2435.8470 | −2382.1390 | −2495.5690 | −2431.0380 | −4101.999 |
Notes: All values are obtained while holding the DSDM fixed. |
PMP | ||||||||
Spatial model | Spatial weights matrix | Spatial weights matrix | Spatial model | |||||||
Matrix form | DSDM | DSAR | DSDEM | DSEM | DSDM | DSAR | DSDEM | DSEM |
Knn=4 | 1.000 | 0.000 | 0.000 | 0.000 | 0.000 | 0.000 | 0.000 | 0.000 |
Knn=5 | 1.000 | 0.000 | 0.000 | 0.000 | 0.000 | 0.000 | 0.000 | 0.000 |
Knn=6 | 1.000 | 0.000 | 0.000 | 0.000 | 0.000 | 0.000 | 0.000 | 0.000 |
Knn=7 | 1.000 | 0.000 | 0.000 | 0.000 | 0.000 | 0.000 | 0.000 | 0.000 |
Knn=10 | 1.000 | 0.000 | 0.000 | 0.000 | 0.000 | 0.000 | 0.000 | 0.000 |
Knn=15 | 1.000 | 0.000 | 0.000 | 0.000 | 0.000 | 0.000 | 0.000 | 0.000 |
Knn=20 | 1.000 | 0.000 | 0.000 | 0.000 | 0.000 | 0.000 | 0.000 | 0.000 |
Cut off = 15 km | 1.000 | 0.000 | 0.000 | 0.000 | 0.000 | 0.000 | 0.000 | 0.000 |
Cut off = 30 km | 1.000 | 0.000 | 0.000 | 0.000 | 0.000 | 0.000 | 0.000 | 0.000 |
Cut off = 50 km | 1.000 | 0.000 | 0.000 | 0.000 | 0.000 | 0.000 | 0.000 | 0.000 |
Cut off = 100 km | 1.000 | 0.000 | 0.000 | 0.000 | 0.000 | 0.000 | 0.000 | 0.000 |
First order QC | 1.000 | 0.000 | 0.000 | 0.000 | 1.000 | 1.000 | 1.000 | 1.000 |
Second order QC | 1.000 | 0.000 | 0.000 | 0.000 | 0.000 | 0.000 | 0.000 | 0.000 |
Third order QC | 1.000 | 0.000 | 0.000 | 0.000 | 0.000 | 0.000 | 0.000 | 0.000 |
First order RC | 1.000 | 0.000 | 0.000 | 0.000 | 0.000 | 0.000 | 0.000 | 0.000 |
Second order RC | 1.000 | 0.000 | 0.000 | 0.000 | 0.000 | 0.000 | 0.000 | 0.000 |
Third order RC | 1.000 | 0.000 | 0.000 | 0.000 | 0.000 | 0.000 | 0.000 | 0.000 |
Notes: Simulations from Matlab routines provided by LeSage (2014). Knn denotes k-nearest neighbor matrix, cut-off stands for inverse distance matrix temp1/dtempij, QC denotes queen contiguity matrix and RC denotes rook contiguity matrix. Parameterc is set to zero.T is a very large number, which results in a diffuse forβ, θ, χ andφ. Diffuse priors forσ are obtained by settingd=0 andv=0. Finally, a0=1.01. Results indicate that DSDM and first order queen contiguity matrix are the optimal choices. |
DSDM (spatial and time fixed effects) | Short run | Lon run | |||||||
Dimension | Variable | β vector | θ vector | Direct | Indirect | Total | Direct | Indirect | Total |
Dependence | Time-lagged χ | 0.3459∗∗∗(0.0397) | |||||||
Spatial and time-lagged φ | −0.0785(0.0525) | ||||||||
Environmental | VI3Mob | −0.0385(0.0648) | 0.0722(0.1167) | −0.0296(0.0672) | 0.1029(0.2095) | 0.0733(0.2313) | −0.0408(0.1079) | 0.2055(0.4536) | 0.1647(0.5021) |
Social | VI6EldIPSS | −1.2504(5.6748) | −7.2833(9.0573) | −2.4050(5.2987) | −15.0124(13.3379) | −17.4174(12.4784) | −4.5189(8.0918) | −32.5838(27.9652) | −37.1057(27.7303) |
VI5IntConsump | 0.4683∗∗∗(0.1479) | −0.3088∗∗(0.1520) | 0.4662∗∗∗(0.1437) | −0.1495(0.2519) | 0.3166(0.2872) | 0.7244∗∗∗(0.2266) | −0.0483(0.5437) | 0.6761((0.6310) | |
Governance | VI4ForeignOpen | 0.0180∗(0.0104) | 0.0140(0.0304) | 0.0208∗(0.0111) | 0.0433(0.0537) | 0.0641(0.0585) | 0.0351∗(0.0188) | 0.1054(0.1223) | 0.1405(0.1333) |
VI1BusinInten | 0.0363(0.0480) | −0.0107(0.0673) | 0.0384(0.0464) | 0.0140(0.1093) | 0.0524(0.1278) | 0.0612(0.0744) | 0.0545(0.2437) | 0.1157(0.2816) | |
Economic | VI2ATourismFlow | −0.0289(0.0560) | 0.0720(0.0741) | −0.0235(0.0521) | 0.1064(0.0998) | 0.0829(0.0874) | −0.0316(0.0795) | 0.2086(0.2022) | 0.1770(0.1942) |
Mean of FE | 3.3062 | ||||||||
Spatial ρ | 0.4870*** (0.046) | ||||||||
σ2ε | 0.0135*** (0.0013) | ||||||||
R2 | 0.0109 | ||||||||
Log Pseudo-likelihood | 1244.3117 | ||||||||
AIC | −2587.8640 | ||||||||
BIC | −2501.1540 | ||||||||
Obs. | 1668 | ||||||||
Stability (Identification) [Endogeneity] | Satisfied (Satisfied) [Satisfied] | ||||||||
Notes: t-statistics in parentheses and degrees of freedom in brackets. Symbol *** (**) [*] represents 1% (5%) [10%] of significance level, respectively. By definition, dynamic spatial panel data models only consider fixed effects. The regression includes robust standard errors estimations and robust variance estimations, which accurately take into account the cluster-correlated data by adjusting for within-cluster correlation. |
Dimension | Acronym | Min | Max | Mean | Std Dev | Source (Definition) # |
Dependent variable | VD2dInfCov | 0 | 1 | 0.1380 | 0.2624 | PT DGH (1.) |
Environmental | VI3Mob | 0 | 1 | 0.0588 | 0.1567 | PT QualAR (2.) |
Social | VI6EldIPSS | 0 | 1 | 0.4209 | 0.3190 | PT SS (3.) |
VI5IntConsump | 0 | 1 | 0.2809 | 0.3158 | PT INE (4.) | |
Governance | VI4ForeignOpen | 0 | 1 | 0.1703 | 0.2353 | PT INE (5.) |
VI1BusinInten | 0 | 1 | 0.3516 | 0.2199 | PT INE (6.) | |
Economic | VI2ATourismFlow | 0 | 1 | 0.4970 | 0.1329 | PT INE (7.) |
Notes: superscript '#' stands for the need of reading the Supplementary Appendix for additional details. |
VD2dInfCov | VI3Mob | VI6EldIPSS | VI5IntConsump | VI4ForeignOpen | VI1BusinInten | VI2ATourismFlow | VIF | |
VD2dInfCov | 1 | |||||||
VI3Mob | 0.6577*** | 1 | 1.05 | |||||
VI6EldIPSS | −0.0264 | −0.1577*** | 1 | 1.03 | ||||
VI5IntConsump | −0.1266*** | −0.1935*** | −0.0056 | 1 | 1.06 | |||
VI4ForeignOpen | 0.0018 | 0.0262 | −0.0279 | −0.1551*** | 1 | 1.14 | ||
VI1BusinInten | 0.5776*** | 0.8307*** | −0.1652*** | −0.1481*** | 0.0602 | 1 | 2.65 | |
VI2ATourismFlow | 0.1057*** | 0.2540*** | −0.0513** | −0.0355 | −0.0013*** | 0.2527*** | 1 | 2.53 |
Notes: Symbol *** (**) [*] for 1% (5%) [10%] of significance level, respectively. Mean VIF of 1.57 is below the critical threshold 5, thus, confirming the absence of multicollinearity. |
Statistical test | Value |
BP LM test for random effects | 215.8200*** [1] |
LM spatial lag (Anselin) | 467.7729*** [1] |
LM spatial error (Burridge) | 297.0096*** [1] |
Robust LM spatial lag | 799.4608*** [1] |
Robust LM spatial error | 628.6976*** [1] |
Global Moran | 0.2499*** (17.3760) |
Global Geary | 0.7710*** (−12.8250) |
Global Getis-Ord | −0.2499*** (−17.3760) |
Moran error test | 17.2858*** (1199.6900) |
LM SAC (LMErr+LMLag_R) | 1096.4704*** [2] |
LM SAC (LMLag+LMErr_R) | 1096.4704***[2] |
Notes: z-statistics in parentheses and degrees of freedom in brackets. Symbol *** (**) [*] represents 1% (5%) [10%] of significance level, respectively. Results reveal significant spatial autocorrelation, which validates the transition towards spatial panel data models. BP LM stands for Breusch–Pagan Lagrangian multiplier. Since p<0.05, the null hypothesis of homoscedasticity is rejected and, thus, a random effects model is preferred to the POLS model. Therefore, heteroscedasticity needs to be controlled for by resorting to a dynamic panel data estimation. LM stands for Lagrangian multiplier. Since p<0.05, the null hypothesis regarding absence of spatial autocorrelation is rejected, so that a spatial model is preferred to the POLS model. |
Value | Spatial fixed effects | Time-period fixed effects | Spatial and time-period fixed effects |
F-statistic | F(283, 1656) = 18.7300*** | ||
Log Likelihood (null) | 22.4350 | −128.8935 | 1094.7750 |
Log Likelihood (model) | 171.1414 | 482.6482 | 1106.3600 |
AIC | −328.2829 | −951.3164 | −2198.7200 |
BIC | −289.2681 | −912.3017 | −2159.7060 |
Likelihood ratio test | 1247.4000*** [277] | 1870.4400*** [6] | |
Hausman test | 45.8600***[6] | ||
Notes: Degrees of freedom are within brackets. Symbol *** (**) [*] represents 1% (5%) [10%] of significance level, respectively. Since the F-statistic is significant sincep<0.05 for municipalities and time dummies, then the null hypothesis of homogeneity (pooling) is rejected at the 5% level. Thus, the LSDV (FE) model is most applicable since the data are favorably to the two-way fixed effects estimation. The inclusion of two-way fixed effects is also justified by the statistical significance observed in both likelihood ratio tests. Finally, the Hausman test indicatesp<0.05, which implies that the null hypothesis that the random effects model is preferred to the fixed effects model is rejected. |
Comparison | χ2 | p-value | AIC | BIC |
(1) SAR versus SDM | 14.5900 [6] | 0.0237 | ||
(2) SEM versus SDM | 13.5400 [6] | 0.0353 | ||
(3) SAR | −2811.1730 | −2766.5850 | ||
(4) SEM | −2817.9920 | −2773.4040 | ||
(5) SAC | −2903.1390 | −2852.9770 | ||
(6) SDM versus DSDM | 85.6200 [2] | 0.0000 | ||
(7) SAR versus DSAR | 94.1900 [2] | 0.0000 | ||
(8) DSAR versus DSDM | 39.3400 [6] | 0.0000 | ||
(9) SAR versus DSDM | 250.5100 [8] | 0.0000 | ||
(10) SAC versus DSDM | 292.0000 [14] | 0.0000 | ||
(11) SEM versus DSDM | 245.2100 [8] | 0.0000 | ||
Notes: Degrees of freedom are within brackets. Values obtained while holding the first order queen contiguity matrix fixed Abbreviation SAR stands for spatial autoregressive model, SDM stands for spatial Durbin model, SEM stands for spatial error, SAC stands for spatial autocorrelation model, DSDM stands for dynamic spatial Durbin model and DSAR stands for dynamic spatial autoregressive model. The interpretation of outcomes is given as follows. Under (1), one cannot reject the null hypothesis that SAR is preferred to SDM for a critical significance level of 5%. Under (2), one cannot rejected the null hypothesis that SEM is preferred to SDM for a critical significance level of 5%. Therefore, at this phase, the static SDM is excluded from being the appropriate option. To select between SAR, SEM and SAC models, AIC and BIC values can be checked in (3) – (5). One can infer that SAC is the optimal static model. Taking thereafter into account dynamic models, (6) shows that one can reject the null hypothesis that SDM is preferred to the DSDM for a critical significance level of 5%. Based on (7), similar conclusion is applied to the rejection of SAR relatively to DSAR for a critical significance level of 5%. According to (8), one can reject the null hypothesis that DSAR is preferred to DSDM for a critical significance level of 5%. Therefore, the main conclusion for dynamic panel data models is that DSDM is the optimal choice. Finally, (10) reflects the need to compare the SAC model against the DSDM from which one rejects the null hypothesis that SAC is preferred to DSDM. Two caveats are worth mentioning. First, spatial models should not be compared by transitivity (i.e. based on a circular argument) but rather directly on a two-by-two basis. Second, (9) and (11) are redundant due to (3)–(5). |
Criterion | First order QC | Second order QC | hird order QC | First order RC | Second order RC | Third order RC |
AIC | −2587.8640 | −2522.5570 | −2468.8490 | −2582.2790 | − 2517.7480 | −4187.071 |
BIC | −2501.1540 | −2435.8470 | −2382.1390 | −2495.5690 | −2431.0380 | −4101.999 |
Notes: All values are obtained while holding the DSDM fixed. |
PMP | ||||||||
Spatial model | Spatial weights matrix | Spatial weights matrix | Spatial model | |||||||
Matrix form | DSDM | DSAR | DSDEM | DSEM | DSDM | DSAR | DSDEM | DSEM |
Knn=4 | 1.000 | 0.000 | 0.000 | 0.000 | 0.000 | 0.000 | 0.000 | 0.000 |
Knn=5 | 1.000 | 0.000 | 0.000 | 0.000 | 0.000 | 0.000 | 0.000 | 0.000 |
Knn=6 | 1.000 | 0.000 | 0.000 | 0.000 | 0.000 | 0.000 | 0.000 | 0.000 |
Knn=7 | 1.000 | 0.000 | 0.000 | 0.000 | 0.000 | 0.000 | 0.000 | 0.000 |
Knn=10 | 1.000 | 0.000 | 0.000 | 0.000 | 0.000 | 0.000 | 0.000 | 0.000 |
Knn=15 | 1.000 | 0.000 | 0.000 | 0.000 | 0.000 | 0.000 | 0.000 | 0.000 |
Knn=20 | 1.000 | 0.000 | 0.000 | 0.000 | 0.000 | 0.000 | 0.000 | 0.000 |
Cut off = 15 km | 1.000 | 0.000 | 0.000 | 0.000 | 0.000 | 0.000 | 0.000 | 0.000 |
Cut off = 30 km | 1.000 | 0.000 | 0.000 | 0.000 | 0.000 | 0.000 | 0.000 | 0.000 |
Cut off = 50 km | 1.000 | 0.000 | 0.000 | 0.000 | 0.000 | 0.000 | 0.000 | 0.000 |
Cut off = 100 km | 1.000 | 0.000 | 0.000 | 0.000 | 0.000 | 0.000 | 0.000 | 0.000 |
First order QC | 1.000 | 0.000 | 0.000 | 0.000 | 1.000 | 1.000 | 1.000 | 1.000 |
Second order QC | 1.000 | 0.000 | 0.000 | 0.000 | 0.000 | 0.000 | 0.000 | 0.000 |
Third order QC | 1.000 | 0.000 | 0.000 | 0.000 | 0.000 | 0.000 | 0.000 | 0.000 |
First order RC | 1.000 | 0.000 | 0.000 | 0.000 | 0.000 | 0.000 | 0.000 | 0.000 |
Second order RC | 1.000 | 0.000 | 0.000 | 0.000 | 0.000 | 0.000 | 0.000 | 0.000 |
Third order RC | 1.000 | 0.000 | 0.000 | 0.000 | 0.000 | 0.000 | 0.000 | 0.000 |
Notes: Simulations from Matlab routines provided by LeSage (2014). Knn denotes k-nearest neighbor matrix, cut-off stands for inverse distance matrix temp1/dtempij, QC denotes queen contiguity matrix and RC denotes rook contiguity matrix. Parameterc is set to zero.T is a very large number, which results in a diffuse forβ, θ, χ andφ. Diffuse priors forσ are obtained by settingd=0 andv=0. Finally, a0=1.01. Results indicate that DSDM and first order queen contiguity matrix are the optimal choices. |
DSDM (spatial and time fixed effects) | Short run | Lon run | |||||||
Dimension | Variable | β vector | θ vector | Direct | Indirect | Total | Direct | Indirect | Total |
Dependence | Time-lagged χ | 0.3459∗∗∗(0.0397) | |||||||
Spatial and time-lagged φ | −0.0785(0.0525) | ||||||||
Environmental | VI3Mob | −0.0385(0.0648) | 0.0722(0.1167) | −0.0296(0.0672) | 0.1029(0.2095) | 0.0733(0.2313) | −0.0408(0.1079) | 0.2055(0.4536) | 0.1647(0.5021) |
Social | VI6EldIPSS | −1.2504(5.6748) | −7.2833(9.0573) | −2.4050(5.2987) | −15.0124(13.3379) | −17.4174(12.4784) | −4.5189(8.0918) | −32.5838(27.9652) | −37.1057(27.7303) |
VI5IntConsump | 0.4683∗∗∗(0.1479) | −0.3088∗∗(0.1520) | 0.4662∗∗∗(0.1437) | −0.1495(0.2519) | 0.3166(0.2872) | 0.7244∗∗∗(0.2266) | −0.0483(0.5437) | 0.6761((0.6310) | |
Governance | VI4ForeignOpen | 0.0180∗(0.0104) | 0.0140(0.0304) | 0.0208∗(0.0111) | 0.0433(0.0537) | 0.0641(0.0585) | 0.0351∗(0.0188) | 0.1054(0.1223) | 0.1405(0.1333) |
VI1BusinInten | 0.0363(0.0480) | −0.0107(0.0673) | 0.0384(0.0464) | 0.0140(0.1093) | 0.0524(0.1278) | 0.0612(0.0744) | 0.0545(0.2437) | 0.1157(0.2816) | |
Economic | VI2ATourismFlow | −0.0289(0.0560) | 0.0720(0.0741) | −0.0235(0.0521) | 0.1064(0.0998) | 0.0829(0.0874) | −0.0316(0.0795) | 0.2086(0.2022) | 0.1770(0.1942) |
Mean of FE | 3.3062 | ||||||||
Spatial ρ | 0.4870*** (0.046) | ||||||||
σ2ε | 0.0135*** (0.0013) | ||||||||
R2 | 0.0109 | ||||||||
Log Pseudo-likelihood | 1244.3117 | ||||||||
AIC | −2587.8640 | ||||||||
BIC | −2501.1540 | ||||||||
Obs. | 1668 | ||||||||
Stability (Identification) [Endogeneity] | Satisfied (Satisfied) [Satisfied] | ||||||||
Notes: t-statistics in parentheses and degrees of freedom in brackets. Symbol *** (**) [*] represents 1% (5%) [10%] of significance level, respectively. By definition, dynamic spatial panel data models only consider fixed effects. The regression includes robust standard errors estimations and robust variance estimations, which accurately take into account the cluster-correlated data by adjusting for within-cluster correlation. |