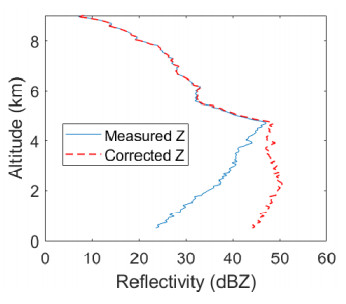
Citation: Stephen L. Durden. Spatial variability of airborne radar reflectivity and velocity measurements of tropical rain with application to spaceborne radar[J]. AIMS Electronics and Electrical Engineering, 2019, 3(2): 164-180. doi: 10.3934/ElectrEng.2019.2.164
[1] | Reza K. Amineh . Developments in three-dimensional near-field imaging with FMCW radar: A comparative study. AIMS Electronics and Electrical Engineering, 2020, 4(4): 359-368. doi: 10.3934/ElectrEng.2020.4.359 |
[2] | Ashraf A. Ahmad, Ameer Mohammed, Mohammed Ajiya, Zainab Yunusa, Habibu Rabiu . Estimation of time-parameters of Barker binary phase coded radar signal using instantaneous power based methods. AIMS Electronics and Electrical Engineering, 2020, 4(4): 347-358. doi: 10.3934/ElectrEng.2020.4.347 |
[3] | Deval V. Jansari, Reza K. Amineh . A two-element antenna array for compact portable MIMO-UWB communication systems. AIMS Electronics and Electrical Engineering, 2019, 3(3): 224-232. doi: 10.3934/ElectrEng.2019.3.224 |
[4] | Edwin Collado, Euribiel Valdés, Antony García, Yessica Sáez . Design and implementation of a low-cost IoT-based agroclimatic monitoring system for greenhouses. AIMS Electronics and Electrical Engineering, 2021, 5(4): 251-283. doi: 10.3934/electreng.2021014 |
[5] | Abhijeet Kumar, Arpit Kumar . Contactless temperature and distance measuring device: A low-cost, novel infrared ―based "Badge"-shaped structural model for measuring physical distance and body temperature. AIMS Electronics and Electrical Engineering, 2022, 6(1): 43-60. doi: 10.3934/electreng.2022004 |
[6] | Shaswat Chirantan, Bibhuti Bhusan Pati . Torque and d-q axis current dynamics of an inverter fed induction motor drive that leverages computational intelligent techniques. AIMS Electronics and Electrical Engineering, 2024, 8(1): 28-52. doi: 10.3934/electreng.2024002 |
[7] | Mostafa A.R. Eltokhy, Mohamed Abdel-Hady, Ayman Haggag, Hisham A. Hamad, Tarek Hosny, Ahmed A. F. Youssef, Ali M. El-Rifaie . An indoor IoT-based LiFi system using LEDs for sensor data transfer. AIMS Electronics and Electrical Engineering, 2025, 9(2): 118-138. doi: 10.3934/electreng.2025007 |
[8] | Tobias Müller . Challenges in representing information with augmented reality to support manual procedural tasks. AIMS Electronics and Electrical Engineering, 2019, 3(1): 71-97. doi: 10.3934/ElectrEng.2019.1.71 |
[9] | Yaoyao Gong, Zengtai Gong . Measures of separation for interval-valued intuitionistic fuzzy sets and their applications. AIMS Electronics and Electrical Engineering, 2025, 9(2): 139-164. doi: 10.3934/electreng.2025008 |
[10] | Minglong Zhang, Iek Cheong Lam, Arun Kumar, Kin Kee Chow, Peter Han Joo Chong . Optical environmental sensing in wireless smart meter network. AIMS Electronics and Electrical Engineering, 2018, 2(3): 103-116. doi: 10.3934/ElectrEng.2018.3.103 |
Radar meteorology is, in part, an application of electrical engineering to the measurement needs of atmospheric science [1,2,3]. Since 1997 at least four radars dedicated to atmospheric measurements have been launched into space [4,5,6,7]. One of the challenges in accurate estimation of rainfall from space is achieving adequate spatial resolution with reasonable-sized antennas. The radar equation describing the power received Pr for transmit power Pt is [8]:
Pr=Pt∫Vλ2G2(4π)3R4ηe−2γdV | (1) |
where λ is the radar wavelength, G is the antenna power pattern, R is the distance from the radar to the precipitation, η is the backscatter cross section per unit volume of precipitation, and γ is the attenuation between the radar and the resolution volume V being measured. Normally, both η and γ are assumed to be nearly constant over V, allowing them to be brought outside the integral in (1). If the spatial resolution is too coarse (i.e., the antenna pattern G is too broad), variability of precipitation within V (inside the integral) can significantly impact the rainfall rate estimated from the instrument, through variations in η and especially γ, due to the exponential in (1) [9,10,11]. A similar integral, but using the precipitation emission and the one-way antenna pattern, applies to microwave radiometers, creating a similar error source [12]. Durden & Tanelli [13] use airborne radar data to simulate the averaging that occurs in the 5-km footprint of the Global Precipitation Measurement (GPM) Dual-Frequency Precipitation Radar (DPR) [5]. They find that spatial variability can cause the estimated path-integrated attenuation to be biased low, as previously illustrated in Nakamura [9].
Data used in previous studies of the spatial properties of rain have come from both in situ and remote sensing. In situ measurements have used networks of rain gauges [14] or, more recently, networks of disdrometers (which measure the full drop size distribution), to examine spatial properties not only of rainfall rate but various microphysical parameters [15,16,17]. Tokay et al. [18], for example, are motivated by the problem of non-uniform beam-filling and use disdrometers to investigate variability of rainfall and other parameters over a scale of a few km. Ground-based radar measurements of precipitation spatial properties include [19,20,21,22,23]. Bringi et al. [24] use polarimetric radar data collocated with 2D video disdrometer data to derive horizontal and vertical correlations in two rain events. Zebo et al. [25] report correlation length from radar observations and from model simulations.
Besides rain rate and microphysical parameters, another parameter associated with precipitation is vertical velocity. Its measurement by spaceborne radar is also of interest [1], [26,27,28] but has yet to be accomplished; however, the EarthCare mission plans to measure Doppler velocity at W-band [29]. Simulations have shown that Doppler velocity measurements are affected by non-uniformity within the radar beam [30], so characterization of the spatial variability of vertical motion is also desirable. Previously, LeMone & Zipser [31] used radar data to infer vertical motion in convective systems, including the size of the updrafts. Additional radar studies of vertical motion in tropical oceanic convection include [32,33,34]. Anderson et al. [35] present in situ measurements of updrafts, including updraft horizontal scale, as measured by instruments on aircraft penetrations of convective storms. Heymsfield et al. [36] provide size and intensity of convective cores as derived from X-band airborne radar. Besides air motion, the measured vertical Doppler velocity is affected by the terminal velocity of rain drops, which is, in turn, a weak function of the reflectivity [34,37]. Hence, the variability of reflectivity can be used to estimate the spatial variability of terminal velocity.
In this work we investigate the spatial variability of radar reflectivity and vertical velocity using data acquired by airborne radar at Ku-band. These data were acquired in the tropics or summer-time subtropics over ocean. Apart from ground-based radar in a few island locations, the vast majority of radar observations of tropical oceanic convection have come from GPM [5] and its predecessor, the Tropical Rainfall Measuring Mission (TRMM) [4], with approximately 5-km horizontal resolution. The data used here are taken at nadir-incidence, giving curtains, or two-dimensional slices, of reflectivity along the aircraft flight track. In Sections 2 and 3 we describe the data and show the variability of reflectivity and vertical velocity. In Section 4 we then discuss the effects of non-uniform beam-filling (NUBF) on spaceborne radar measurements of rainfall and velocity, relating the variability measures reported in Section 3 to expected radar measurement errors.
The data used in this study were acquired from the Airborne Rain Mapping Radar (ARMAR) and its successor, the Airborne Precipitation Radar, 2nd Generation (APR2) [38,39]. These radars have good spatial resolution, about 55 m vertical and 800 m horizontal (at the surface, better above the surface); this horizontal resolution is generally similar to that of the ground-based radar data used in many previous studies (e.g., 1 km in [21,23]). ARMAR and APR2 have acquired data in more than a dozen field campaigns since 1993. The data analyzed here are from nine of those campaigns focused on tropical convection. While some of these data have been used for atmospheric studies and examination of non-uniform beam-filling effects [10,13,30], the analyses of the full data set presented here are new.
APR2 has both Ku-band and Ka-band capabilities, but only the Ku-band data are used here to be consistent with the Ku-band ARMAR data. Furthermore, Ku-band is much less affected by attenuation, which changes the spatial structure of the measured reflectivity. The attenuation present at Ku-band is corrected, as discussed below. Both ARMAR and APR2 instrument designs include calibration loops that couple a small amount of transmit power into the receiver for tracking transmit power and gain variations. The reflectivity products from both instruments use the calibration loop data to remove effects of such variations. The overall, absolute calibration of the datasets is adjusted so that ocean backscatter matches historic ocean scatterometer measurements [38,39]. Hence, the relative calibration differences between the two instruments and between the various field experiments should be less than 1–2 dB. Furthermore, absolute calibration should not impact analysis of spatial variability. Relative calibration over the distances being examined could impact the results but should be very small (well below the absolute calibration uncertainty).
For the nine field campaigns used here, the total data collection time is many hundreds of hours. However, these data include very light rain and clear areas. We manually examined the data and located aircraft passes through convective systems. These data were further down-selected to keep only those profiles with positive SNR and valid velocity estimates at all range bins from surface through about 5 km altitude. This reduced the total data to about 50,000 radar reflectivity profiles. All data were acquired with the NASA DC8 aircraft, with typical ground speed of 200 m/s, or 0.36 km per profile, resulting in roughly 18,000 km of data.
To remove the effects of attenuation from our analysis, we applied a simplified version of the Surface Reference Technique (SRT), used routinely in operational processing of TRMM and GPM data (see [40]). The SRT estimates the two-way path integrated attenuation (PIA) as the difference in surface return under rain and the expected surface return under non-raining conditions. The PIA is then used to adjust the attenuation-reflectivity (k-Z) relationship and provide a boundary condition for retrieving the unattenuated reflectivity, as described by Durden & Haddad [41]. Figure 1 shows an example of an observation of the vertical reflectivity profile in convective rain before and after attenuation correction. The SRT-measured PIA is 20 dB in this case; the near-surface reflectivity is thus increased by 20 dB in the attenuation correction process. The measured reflectivity above the surface is increased by lesser amounts, with the increase going to near zero above about 5 km altitude, where propagation in ice causes very small attenuation.
The terminal velocity of rain is estimated from the reflectivity using a relation of the form V = aZb, as used by Black et al. [34] in their study of vertical air motion in hurricanes. In rain, a = 2.6 and b = 0.107. Hence, as noted earlier, the terminal velocity is a weak function of Z, ranging from about −4.2 m/s at 25 dBZ to −8.8 m/s at 55 dBZ. The velocity sign convention is negative for downward motion. The measured Doppler velocity is corrected for aircraft attitude by removing the measured surface Doppler from all bins. This assumes that Doppler from surface motion is dominated by aircraft motion, which is generally the case, especially for the nadir pointing of the data used here. The air vertical velocity is then estimated from the corrected Doppler by subtracting the terminal velocity. We classify each profile as stratiform or convective [42], with the latter being areas of stronger vertical motion and usually larger reflectivity. The stratiform areas tend to be larger areas between convective cells with generally weak downward motion and smaller and more uniform reflectivity. We use a method that is a simplified version of the vertical profile algorithm used for TRMM and GPM [43]. Stratiform rain is primarily identified by presence of an upper level maximum reflectivity due to melting ice particles (the radar bright band). As discussed in [43] this detection is accomplished by applying a second-order finite difference to each profile after attenuation correction. The large curvature at the bright band peak is found by comparing the output of the second-order difference with a threshold. Our algorithm also checks that the altitude of the bright band is near that expected in the tropics, that the profile does not have too large of a slope, and that the reflectivity below the bright band is not too large. In our simplified approach, convective rain is all rain that does not meet the criteria for stratiform.
For analysis of spatial variability, we focus on the region below the bright band, where the precipitation should be liquid. Hence, effects of melting ice and other ice processes on spatial correlation is not considered here. To investigate horizontal variability, we use the reflectivity and velocity vertically averaged over each profile between altitudes of 3 and 4 km. This altitude is high enough that the effects of attenuation are reduced compared to bins very near the surface, but low enough to be below the melting level in these data (typically 4.5 km). We also examine the PIA for each profile. Because the SRT PIA is less reliable in light rain [40], we also estimate the PIA by converting the profile-averaged reflectivity to PIA using reflectivity-rainfall (Z-R) and attenuation-rainfall (k-R) relations [44]. Outliers in the reflectivity, velocity, and PIA data are removed in processing by applying median filtering [45]. To process and analyze the data, we use the R language (e.g., [46]) with several of its packages to implement median filtering and rolling statistics within a specified horizontal window that moves through the data.
Several measures of variability have been used in previous studies. The standard deviation of the observable (Z or V or PIA) within a specified horizontal window is an estimate of how much variation could be expected within a radar footprint corresponding to the window size, although caution must be exercised since the real footprints are two-dimensional. Another measure of variation is the gradient of the observable within the analysis window. For this we first smooth the data by fitting a third order polynomial and then computing the root-mean-square (RMS) slope. This reduces the impact of measurement noise on the results. The RMS rather than mean slope is used since one could have large variability and still have a mean slope of zero. For these metrics we use the radar reflectivity in dBZ, rather than the linear domain, since radar meteorologists are accustomed to working in these units. Yet another measure of spatial change is the correlation between spatially separated points. For networks of in situ instruments or ground-based radar, one gets multiple observations of each variable (e.g., same spatial location at multiple times). A standard measure in this case could be the Pearson or Spearman correlation coefficient between each variable [47], taking the average over the multiple observations. Unlike ground-based data, however, we do not have multiple measurements at the same location. Rather, with the airborne data we get one snapshot of the rain and so take an approach similar to that of Zawadzki [20] and Lee et al. [23], which calculates the autocorrelation function of the data. In this case the data are a single realization of a non-stationary time or spatial series, so we view the series as a deterministic function and compute R(x)=∫g(α)g(α+x)dα, where g(x) is the quantity of interest (Z or V or PIA) at a location x. This is just the communication systems definition of autocorrelation stated in [48]. For a length of data within the current horizontal window, we take the Fast Fourier Transform (FFT), form the magnitude squared, and take the inverse FFT to get R(x). Once we have R(x), we calculate the correlation length as the equivalent width of R(x) [49]; we calculate the integral of R(x) from 0 to the maximum value of x, with its peak normalized to unity. While one could fit an exponential to the calculated R(x), the method of using the area under R(x) for x > 0 is more robust to noise and variations in the shape of R(x). The autocorrelation is often defined with the mean removed from g(x) prior to computing the integral for R(x); however, the mean is not removed in [20] and [23] when analyzing reflectivity. Following these two references, the mean is kept in the correlation length calculations in this work. Tokay et al. [18] found different correlation lengths for different quantities associated with rain, e.g., rainfall rate, reflectivity in dBZ. This study uses reflectivity in the linear domain. The sensitivity of the results presented here to this and other choices is discussed further in the next section.
In this section we examine two different cases of data acquired over mesoscale convective systems (one in the Pacific Ocean in 1993, the other in the Atlantic Ocean in 2017). Figure 2, upper left panel, is a reflectivity image with a convective cell centered near along-track distance 30 km. A bright band (horizontal line of enhanced reflectivity) can be seen to the right of the convective cell; its altitude relative to sea level is about 4.5 km, and it extends horizontally from 50 km along-track to the right edge of the image. No such bright band occurs above the convective cell. The convective/stratiform classification algorithm (upper middle panel of Figure 2) classifies the convective cell and surrounding area as convective. Much of the area to the left of the cell is also classed as convective, but mixed with stratiform. The remaining area, to the right of the convective cell (starting at 40 km), is mostly classified as stratiform. The upper right panel of Figure 2 shows the profile-averaged reflectivity versus the along-track distance, clearly showing the convective cell at distance of 30 km. The reflectivity approaches 50 dBZ in the convective cell, while the reflectivity in the stratiform area at right is 5–10 dB lower. The PIA reaches 27 dB within the convective cell but is typically just below 10 dB within most of the stratiform region. Both the PIA from the SRT and the PIA derived from the path-averaged reflectivity Z are shown and track each other quite well.
The lower left panel in Figure 2 shows the standard deviation of the reflectivity; it reaches almost 5 dB in the transition between the convective and stratiform areas and drops to 1 dB or less in the stratiform area to the right. This panel also shows the standard deviation estimated from both versions of the PIA; these two curves are very similar. The lower middle panel shows the RMS gradients of reflectivity and PIA. The gradients are larger in the convective area, with maximum PIA gradient exceeding 3.5 dB per km. If this were a linear trend across a footprint, this would be more than 15 dB change in PIA over a 5-km spaceborne radar footprint. Such large changes are consistent with the upper right panel in Figure 2. The lower right panel in Figure 2 is the estimated correlation lengths for reflectivity, PIA, and air velocity. The calculated lengths for Z and PIA are smallest near the convective cell and larger away from the cell, although the reduction in correlation length for Z is much more than that for PIA.
The vertical (air motion) velocity correlation length in Figure 2 varies between maxima of about 12 km near 10, 23, and 65 km along-track and minima of less than 4 km near 40 km and surrounding 100 km along-track distance. The minima for both Z and velocity occur away from the center of the convection, closer to the transitions between convective and stratiform rain. The corresponding air velocity is shown in the left panel of Figure 3. Small velocities such as these are fairly typical in oceanic convection [31,33]. The terminal velocity calculated from the reflectivity is also shown. The middle panel in Figure 3 shows the standard deviation of the velocities, and the right panel shows the RMS horizontal gradient of the vertical velocities. Both plots show reduced spatial variability in the stratiform area in the right half of the data, except for a peak near 110 km, where the bright band fades away in the reflectivity image in Figure 2. The air velocity gradient in the convective area could correspond to several m/s across a 5-km spaceborne radar footprint. The vertical air velocity is correlated with the reflectivity, with the correlation coefficient fluctuating between −0.5 and +0.5.
Figure 4 shows the reflectivity and PIA, the horizontal gradient of the profile-averaged reflectivity, and correlation lengths for a second case, acquired in the 2017 field campaign. These data also have both stratiform and convective areas. However, in this case, the two types of precipitation alternate within the data take, giving a more complicated situation than in the previous case. Convective areas are near along-track distances 20, 28, and 37 km, although the last is significantly weaker than the first two. The reflectivity standard deviation (not shown) and gradient reach maximum values of 5 dB and 3.5 dB/km. Although not shown in Figure 4, velocity statistics were also calculated and are similar to the 1993 case. The air velocity reaches a maximum of nearly 5 m/s; its standard deviation and gradient reach values of 2.3 m/s and 2.4 m/s/km. The terminal velocity standard deviation and gradient for this case are lower than for the previous case, having maxima of 0.9 m/s and 0.7 m/s/km. The PIA in this case (also not shown) tracks the reflectivity, similar to the case in Figure 2 and reaches a maximum of 24 dB. The maxima just noted are typically near along-track distance 28 km. The right panel in Figure 4 shows the correlation lengths of Z, PIA, and air velocity. The correlation lengths in the convective area (15–40 km along-track distance) are generally smaller than for the more stratiform area at right. As with the first case, we find that the reflectivity is correlated with the vertical air velocity, with correlation coefficient reaching +0.7 over much of the data, dropping to −0.5 at the far right.
The sensitivity of our results to the various methods and assumptions is explored by repeating the analyses of these two cases and the full data set with various modifications. The attenuation correction is evaluated by changing the attenuation corrected profiles to simulate large PIA errors of ±6 dB. We expect actual errors to be significantly smaller than this, based on experience with the SRT since the 1990s. The simulated error in PIA causes the reflectivity at the surface in all the profiles to change by the change in the PIA. The most noticeable effect occurs in the precipitation classification when increasing PIA by 6 dB. For the case in Figure 2, many areas with bright band are classified as convective because the reflectivity profile in the PIA-corrected data strongly increases toward the surface, giving an unphysical profile shape. However, the resulting reflectivity and PIA spatial variability measures are changed by amounts small enough that the conclusions of this study are not affected. The velocity measurements are not affected by PIA errors. Hence, the results of this study should be reasonably robust to typical errors in the SRT PIA.
As noted earlier, this study calculates the correlation length without removing means from the data, as done in [20] and [23]. We find that a large mean relative to the variations within a window increases the estimated correlation length, if the mean is retained. If means are removed, resulting correlation lengths would be smaller than shown for the two cases and in results for the full data set in Section 3.2. The variable with the largest sensitivity to the presence or absence of the mean may be the correlation length for the terminal velocity. Because terminal velocity has a relatively small variation about a mean velocity of −6 m/s or so, the correlation lengths when keeping the mean can be quite long, well over 20 km. If the mean is removed, correlation lengths drop to only a few km. The correlation length for reflectivity is also influenced by whether the reflectivity is analyzed in dBZ or linear units. Generally, the reflectivity in dBZ has smaller variation and longer correlation length than the same reflectivity expressed in linear units. The latter are used here. To test the effect of median filtering, the analysis was run with it removed. The main effect on the cases presented above is noticeably more noise in the plots of the RMS gradient, although the basic shapes of the plots are similar (not shown). For the full data set in the next section, the histograms of the RMS gradient of reflectivity and PIA extend to somewhat larger values. Otherwise, results are similar to when median filtering is applied.
Running the analyses with different window lengths shows that the results are indeed influenced by the window length choice. For the standard deviations and gradients, the window length is 5 km, corresponding to the TRMM and GPM radar footprints. Varying the window length simulates varying the footprint size and is discussed in detail in Section 4. Correlation length, on the other hand, is viewed as being intrinsic to the rain, so minimizing the calculation's dependence on window size is preferred. While the window length limits the maximum calculated correlation length, using a window that is too long can result in overestimating the correlation length in some small and highly variable areas. Hence, we generally use a 21-km window for correlation length, which limits the maximum correlation length to around 18 km. Results with other window sizes are mentioned in the next section. The sensitivities of the correlation length calculations noted here should be considered when comparing the correlation lengths found here with other published results.
In this section we examine the statistics describing spatial variability over the full data set, separating the results into convective and stratiform types. The various statistics (e.g., standard deviation, gradient) are presented in overlapping histograms with red for convective areas and blue for stratiform areas. The histograms are normalized to represent probability density for ease of comparison. Over the full data set we find a maximum reflectivity of 56 dBZ, a maximum updraft velocity of 24 m/s, and maximum terminal velocity of −11 m/s, occurring in convective areas. The maximum PIA is 44 dB, also in convection.
Figure 5 shows histograms of the reflectivity and vertical velocities, while Figure 6 shows similar histograms of PIA statistics. For both reflectivity and PIA, the largest standard deviation and gradient and the smallest correlation length occur in convection. Correlation lengths less than about 0.7 km cannot be resolved due to the effect of the aircraft radar footprint size within the profiles. The mean reflectivity correlation length (both precipitation types) is 8.9 km; reflectivity correlation lengths of less than 5 km, for example, make up 19% of the cases in Figure 5. Recalculating the correlation length using a 10-km window, instead of 21 km, yields a mean length of 5 km, and lengths less than 5 km are much more common (46% of the data). Lengths less than 2 km, however, comprise only 5% of the data, so lengths of several km are more typical, even with the shorter analysis window. Increasing the window length to 31 km shifts the resulting correlation lengths in the opposite direction; the mean is increased to 11.4 km, while lengths less than 5 km are reduced to 14% of all the lengths. These window-varying experiments indicate that the reflectivity data have a mean correlation length of near 9 km with an uncertainty of a few km. Even with this uncertainty, it is clear that correlation lengths of less than 5 km can occur. The correlation lengths for PIA tend to be longer, with mean of 11 km and 7% of the data less than 5 km (for the standard 21-km processing window).
The reflectivity and PIA correlation lengths in Figures 5 and 6 are similar to other published radar correlation lengths. For example Bringi et al. [24] in mid-latitude rain found a rainfall rate horizontal correlation length of 3.4 km in their convective case and 10.2 km in stratiform rain, within the range of values in Figure 5, lower left. Lebo et al. [25] report a correlation length of 5 km for tropical convective rain, likely similar to the rain in the non-stratiform data studied here. Correlation lengths of 40 km and larger are shown by [23] for mid-latitude stratiform precipitation in Canada. Our 21-km windowing does not allow for such long lengths to be estimated, but as mentioned above, we do get larger values if the window length is increased. However, a 40-km correlation length is unlikely in the data studied here. The results in [23] were derived after applying time averaging to the spatial autocorrelation and possibly to the input radar field, which may increase correlation length [16]. Our measurements have no time-averaging, so shorter lengths than [23] might be expected. Mid-latitude winter precipitation also likely differs from that in the tropics.
The correlation lengths in Figures 5 and 6 are also similar to those from the disdrometer network measurements in [16], which reported a correlation length of 1.9 km for convective rain and 4.9 km for transitional cases for their shortest averaging time of 1 min. In our simple classification algorithm, transitional rain would be classed as convection. This is rain outside the main convective updrafts but still with moderate upward air motion, large reflectivity, and no radar bright band. While 1.9 km is uncommon in our data, 4.9 km is near the convective peak in Figure 5, lower left. The correlation length of terminal velocity in Figure 5 for both convective and stratiform rain is clearly much larger than the 20-km window, since most of the observations fall into bins just below 20 km (confirmed by using longer windows up to 80 km). The large correlation length is likely a result of the weak dependence of terminal velocity on reflectivity (to the 0.107 power); the spatial variation of the terminal velocity is thus relatively small. As noted previously, removing the mean would significantly reduce the correlation lengths for terminal velocity.
The behavior of the air velocity correlation length differs from reflectivity; both types of rain have similar histograms, with longer lengths being slightly more common in stratiform rain. The vertical air velocity is found to be correlated with reflectivity. This relationship is to be expected from physical considerations; correlation between vertical motion and precipitation at larger scales is noted in [50]. Previous studies of the spatial structure of vertical motion have mostly looked at size and intensity of convective cores and not correlation length. The size of updraft or downdrafts reported in LeMone & Zipser [31] are typically small, most less than 2–3 km and nearly all less than 5 km near the altitude of our data. The velocity magnitudes are mostly below 10 m/s. Similar statistics are found in [32] for measurements in hurricanes and [33] for measurements in oceanic convective systems. One study that does compute spatial autocorrelation of vertical velocity is that of Black et al. [34]; this study shows the autocorrelation for vertical Doppler data in hurricane eyewalls and rain bands. The latter areas should be more comparable with the data examined here. Their Figure 5 shows that the correlation coefficient for velocity drops to 0.25 within a few km. Hence, the horizontal correlation lengths implied by their results are in rough agreement with the red histogram at bottom right in Figure 5.
The airborne precipitation radar data analyzed here have shown that radar observables can vary by relatively large amounts over a few km, which is within the 5 km footprint of the current generation of spaceborne precipitation radars. Based on previous studies, this can create biases in measured quantities. Durden et al. [10] found that NUBF can cause errors in both the measured reflectivity and the measured PIA using the SRT. The errors in PIA are often much larger than for reflectivity and are almost always an underestimate of the true PIA. The example of a half-filled beam in Nakamura [9] helps to explain this. If one-half the beam is filled with heavy rain, then the PIA to the surface in rain can be very large; our airborne data had a maximum PIA of 44 dB. On the other hand, there is no attenuation in the clear area half of the beam. As mentioned previously, the SRT derives PIA by comparing the surface backscatter within the raining footprint with the surface backscatter for a similar surface but in non-raining conditions. For this example, the surface backscatter would be reduced by only 3 dB, since half the surface has no attenuation. Hence, the SRT would estimate a PIA of only 3 dB, grossly underestimating the large PIA in the rain area. While such an idealized scenario is probably very rare, our results do show that variability can occur on the scale of the 5 km footprint used for TRMM and GPM. In fact, the dual-frequency measurement capability of GPM has allowed the NUBF effects on PIA to be directly observed [51]. PIA underestimation at Ka-band, likely due to NUBF, can exceed 10 dB in some cases [13], [51], with smaller but non-negligible errors at Ku-band.
To see how the variability metrics studied in the previous section relate to the SRT-estimated PIA, we perform calculations similar to those in [10] and [13]. Within the moving window used for the other statistics, we also calculate the average of the PIA in both linear and logarithmic domains. The average of the logarithm of attenuation is analogous to averaging rainfall rate, since the path-averaged rain rate is nearly proportional to the PIA in dB [44]. The average of the linear path attenuation (varying between 0 and 1 and also called the transmissivity) is analogous to the SRT measurement. The difference is the bias. In the half-filled case above, the average of e.g. 60 dB in the rain and 0 outside the rain would yield a PIA of 30 dB. On the other hand, the linear transmissivity corresponding to 0 dB is 1, while that corresponding to 60 dB is 1.0e-6. The average of these two values is about 0.5, or 3 dB attenuation. We choose window sizes between 1 and 10 km to see the effect of reducing the radar footprint size. The results are shown in Table 1. Because the maximum of a data set can be easily skewed by outliers, we use the 95th percentile as a relatively robust measure of near worst case. As can be seen from columns two and three in the table, reducing the footprint (window) size reduces the standard deviation of the PIA within the window and reduces the underestimation bias in the SRT PIA in cases of severe NUBF. Indeed, the correlation coefficient between the PIA standard deviation and PIA bias is 0.9; it is 0.8 for RMS gradient and PIA bias. While the PIA bias is directly due to the variation of the PIA across the footprint, the correlation length is related to the likely variability. We find a statistically significant correlation coefficient of −0.2 between PIA bias and correlation length; the PIA bias tends to decrease as the correlation length increases.
Footprint Size (km) | PIA StandardDev (dB) | PIA Bias (dB) | Z Std Dev (dB) | Velocity Error (m/s) |
9.7 | 5.1 | 1.9 | 7.8 | 40 |
6.8 | 4.5 | 1.6 | 7.2 | 27 |
5.4 | 4.0 | 1.4 | 6.8 | 20 |
2.5 | 2.9 | 0.7 | 5.3 | 8 |
1.1 | 1.8 | 0.2 | 3.7 | 2 |
While the acceptable level of PIA error for a spaceborne radar depends on the planned mission science and applications, a reduction in resolution from 5 km to even 3 km would significantly reduce the worst case NUBF-related errors. At Ku-band this would require a large antenna, with a size approaching 4 m. Better resolution can also be achieved by using a 2-m antenna but with a higher frequency. The W-band (94 GHz) radar on CloudSat [6], for example, achieves an instantaneous footprint of less than 1.4 km. However, attenuation at W-band often obscures the lower portion of strong convection (with added complication of multiple scattering, as discussed by Battaglia et al. [52]). At lower frequencies, where very large antennas may be impractical, radar might be able to benefit from deconvolution techniques, such as used by the microwave radiometer community [53].
For Doppler measurement from space, there are several factors to be considered. Use of a single antenna requires the antenna to be relatively large to reduce the Doppler spectral width and increase the correlation between successive pulses, even with no NUBF. The reason is that echoes from forward and aft of nadir are Doppler shifted by the corresponding component of the spacecraft motion. A narrow Doppler spectrum requires the antenna gain to fall off rapidly away from nadir, and such narrow patterns generally require antennas large in the along-track dimension. If the antenna is small and the spectrum wide, low correlation between pulses results in a noisy Doppler estimate [1], although this can be partially compensated by use of a higher PRF with polarization diversity [54].
NUBF further complicates Doppler measurements of rain from space. Our findings indicate that vertical air velocity itself can vary significantly over small scales in some cases. While the desire to resolve velocity changes may argue for somewhat smaller footprints than current radars, the effect of linear averaging over these variations is simply a loss of resolution of horizontal structure, without the biases that affect rain retrieval [55]. However, the main effect of NUBF on velocity measurement would occur even with a fully uniform air velocity in the beam; it is the result of weighting the return by non-uniform reflectivity [30]. This effect was also considered along with velocity gradients in [55] for ground-based Doppler radars. The weighting due to reflectivity variations can effectively move the location of the main part of the echo off-nadir so that the motion of the spacecraft is combined with the Doppler velocity of the precipitation. This can create biases of several m/s even for a 5-m Ku-band antenna [30], reduced by improved processing [56]. The results in these two studies are based on a few cases from ARMAR.
Columns 4 and 5 of Table 1 show the 95th percentile reflectivity standard deviation and velocity error as a function of footprint size. The reflectivity standard deviation is calculated as before and shown for reference, since it is may be more indicative of the NUBF affecting Doppler velocity than the PIA standard deviation in column 2. For Doppler calculation a GPM-like platform is assumed, near 400 km altitude and moving at 7 km/s. The components of the platform velocity are calculated for each point in the footprint; the rainfall velocities are assumed to be zero for this calculation. Hence, the simulated Doppler for the footprint is the normalized sum of the individual velocity components weighted by a Gaussian-shaped two-way antenna pattern (symmetric about nadir) and by the reflectivity within the footprint. If the reflectivity were uniform, the measured Doppler velocity for this situation would be zero. However, the variability of the reflectivity within a footprint, i.e. NUBF, causes this sum to deviate from zero, and the magnitude of this deviation is the velocity error used for Table 1. As done with the PIA bias, we compute the correlation coefficient between the reflectivity statistics and velocity error. These correlation coefficients are: 0.5 for reflectivity standard deviation, 0.3 for RMS gradient, and −0.4 for reflectivity correlation length, showing that all three variability metrics are correlated with the magnitude of the velocity error.
The 95th percentile errors in Table 1 can be very large for the largest footprint sizes, but the median errors for smaller footprints are more reasonable. For 5.4 km the median error is 5 m/s, reduced to 1.9 m/s for a 2.5 km footprint. Since no attempt is made here to correct the measured Doppler for NUBF, some improvement could be expected using the processing described in [56,57]. Hence, a horizontal resolution of 2.5 km could be a reasonable choice for a mission in which some Doppler information is desired. However, for precise Doppler, a resolution near 1 km is preferred (median error magnitude 0.5 m/s). An alternative could be the use of a multi-antenna system for Doppler measurement, which eliminates the need for setting antenna size based on spectral broadening due to platform motion, as proposed in [27]. Such a system could, in principle, acquire Doppler with relatively small antennas (e.g., 2 m). Because this approach effectively cancels platform motion, the effect of NUBF due to reflectivity weighting as analyzed in [30] and [56] would be small. However, resolving spatially varying vertical air velocity could still require larger antennas or higher frequencies, as discussed for reflectivity and PIA.
We have examined spatial variability statistics in an extensive set of radar data in oceanic tropical convective systems. Statistics calculated for these data are standard deviations, spatial gradients and correlation lengths. The standard deviation and spatial gradient statistics indicate the possibility of large variability within current spaceborne precipitation radar footprints. We find correlation lengths from 1 km up to more than 15 km in a few cases. Previous studies and simple one-dimensional calculations here show that the level of variability found here can result in errors in path attenuation correction (and, hence, rainfall) and in velocity errors for spaceborne weather radars. Sensors that can achieve horizontal resolutions of 2–3 km are desirable to reduce non-uniform beam-filling errors, with 1 km being preferred for precise Doppler measurements. Resolution enhancement via deconvolution and use of multi-antenna systems are also noted as possible ways of reducing NUBF effects in future spaceborne precipitation radars.
The research described in this paper was carried out at the Jet Propulsion Laboratory, California Institute of Technology, under contract with the National Aeronautics and Space Administration. Funding was provided by the NASA Precipitation Measurement Missions Program. The data used here resulted from the efforts of the ARMAR and APR2 teams that maintained and operated the radars. The author benefited from discussions with Drs. S. Tanelli and O. Sy of JPL, including the suggestion of applying deconvolution techniques to radar data.
The author declares no conflicts of interest in this paper.
[1] | Meneghini R, Kozu T (1990) Spaceborne Weather Radar. Norwood, MA, USA: Artech House. |
[2] | Bringi VN, Chandrasekar V (2005) Polarimetric Doppler Weather Radar: Principles and Applications. Cambridge, UK: Cambridge University Press. |
[3] | Doviak RJ, Zrnic DS (1993) Doppler Radar and Weather Observations. New York, NY, USA: Academic Press. |
[4] |
Kozu T, Kawanishi T, Kuroiwa H, et al. (2001) Development of precipitation radar onboard the Tropical Rainfall Measuring Mission (TRMM) satellite. IEEE T Geosci Remote 39: 102–116. doi: 10.1109/36.898669
![]() |
[5] |
Hou AY, Kakar RK, Neeck S, et al. (2014) The Global Precipitation Measurement Mission. B Am Meteorol Soc 95: 701–722. doi: 10.1175/BAMS-D-13-00164.1
![]() |
[6] |
Tanelli S, Durden SL, Im E, et al. (2008) CloudSat's Cloud Profiling Radar after two years in orbit: performance, external calibration, and processing. IEEE T Geosci Remote 46: 3560–3573. doi: 10.1109/TGRS.2008.2002030
![]() |
[7] | Peral E, Statham S, Im E, et al. (2018) The Radar-in-a-Cubesat (RAINCUBE) and measurement results. In: IGARSS 2018-2018 IEEE International Geoscience Remote Sensing Symposium, pp. 6297–6300. |
[8] | Ishamaru A (1978) Wave Propagation and Scattering in Random Media. New York, NY, USA: Academic Press. |
[9] |
Nakamura K (1991) Biases of rain retrieval algorithms for spaceborne radar caused by nonuniformity of rain. J Atmos Ocean Tech 8: 363–373. doi: 10.1175/1520-0426(1991)008<0363:BORRAF>2.0.CO;2
![]() |
[10] |
Durden SL, Haddad ZS, Kitiyakara A, et al. (1998) Effects of non-uniform beam-filling on rainfall retrieval for the TRMM Precipitation Radar. J Atmos Ocean Tech 15: 635–646. doi: 10.1175/1520-0426(1998)015<0635:EONBFO>2.0.CO;2
![]() |
[11] |
Zhang L, Lu D, Duan S, et al. (2004) Small-Scale rain nonuniformity and its effect on evaluation of nonuniform beam-filling error for spaceborne radar rain measurement. J Atmos Ocean Tech 21: 1190–1197. doi: 10.1175/1520-0426(2004)021<1190:SRNAIE>2.0.CO;2
![]() |
[12] |
Ha E, North GR (1995) Model studies of the beam-filling error for rain-rate retrieval with microwave radiometers. J Atmos Ocean Tech 12: 268–281. doi: 10.1175/1520-0426(1995)012<0268:MSOTBF>2.0.CO;2
![]() |
[13] |
Durden SL, Tanelli S (2008) Predicted effects of nonuniform beam filling on GPM radar data. IEEE Geosci Remote S 5: 308–310. doi: 10.1109/LGRS.2008.916068
![]() |
[14] |
Huff FA, Shipp WL (1969) Spatial correlations of storm, monthly, and seasonal precipitation. J Appl Meteorol 8: 542–550. doi: 10.1175/1520-0450(1969)008<0542:SCOSMA>2.0.CO;2
![]() |
[15] | Tapiador FJ, Checa R, de Castro M (2010) An experiment to measure the spatial variability of rain drop size distribution using sixteen laser disdrometers. Geophys Res Lett 37: L16803. |
[16] |
Jaffrain J, Berne A (2012) Quantification of the small-scale spatial structure of the raindrop size distribution from a network of disdrometers. J Appl Meteorol Clim 51: 941–953. doi: 10.1175/JAMC-D-11-0136.1
![]() |
[17] |
Jameson, AR (2016) Quantifying drop size distribution variability over areas: Some implications for ground validation experiments. J Hydrometeorol 17: 2689–2698. doi: 10.1175/JHM-D-16-0094.1
![]() |
[18] |
Tokay A, D'Adderio LP, Procu F, et al. (2017) A field study of footprint-scale variability of raindrop size distribution. J Hydrometeorol 18: 3165–3179. doi: 10.1175/JHM-D-17-0003.1
![]() |
[19] |
Kessler E (1966) Computer program for calculating average lengths of weather radar echoes and pattern bandedness. J Atmos Sci 23: 569–574. doi: 10.1175/1520-0469(1966)023<0569:CPFCAL>2.0.CO;2
![]() |
[20] |
Zawadzki II (1973) Statistical properties of precipitation patterns. J Appl Meteorol 12: 459–472. doi: 10.1175/1520-0450(1973)012<0459:SPOPP>2.0.CO;2
![]() |
[21] |
Crane RK (1990) Space-time structure of rain fields. J Geophys Res 95: 2011–2020. doi: 10.1029/JD095iD03p02011
![]() |
[22] |
Kozu T, Iguchi T (1999) Nonuniform beamfilling correction for spaceborne radar rainfall measurement: Implications from TOGA COARE radar data analysis. J Atmos Ocean Tech 16: 1722–1735. doi: 10.1175/1520-0426(1999)016<1722:NBCFSR>2.0.CO;2
![]() |
[23] |
Lee CK, Lee GW, Zawadzki I, et al. (2009) A preliminary analysis of spatial variability of raindrop size distributions during stratiform rain events. J Appl Meteorol Clim 48: 270–283. doi: 10.1175/2008JAMC1877.1
![]() |
[24] |
Bringi VN, Tolstoy L, Thurai M, et al. (2015) Estimation of spatial correlation of drop size distribution parameters and rain rate using NASA's S-band polarimetric radar and 2D video disdrometer network: Two case studies from MC3E. J Hydrometeorol 16: 1207–1221. doi: 10.1175/JHM-D-14-0204.1
![]() |
[25] |
Lebo ZJ, Williams CR, Feingold G, et al. (2015) Parameterization of the spatial variability of rain for large-scale models and remote sensing. J Appl Meteorol Clim 54: 2027–2046. doi: 10.1175/JAMC-D-15-0066.1
![]() |
[26] |
Amayenc P, Testud J, Marzoug M (1993) Proposal for a spaceborne dual beam rain radar with Doppler capability. J Atmos Ocean Tech 10: 262–276. doi: 10.1175/1520-0426(1993)010<0262:PFASDB>2.0.CO;2
![]() |
[27] |
Durden SL, Siqueira P, Tanelli S (2007) On the use of multiantenna radars for spaceborne Doppler precipitation measurements. IEEE Geosci Remote S 4: 181–183. doi: 10.1109/LGRS.2006.887136
![]() |
[28] |
Durden SL, Tanelli S, Epp LW, et al. (2016) System design and subsystem technology for a future spaceborne cloud radar. IEEE Geosci Remote S 13: 560–564. doi: 10.1109/LGRS.2016.2525718
![]() |
[29] |
Kollias P, Tanelli S, Battaglia A, et al. (2014) Evaluation of EarthCARE Cloud Profiling Radar Doppler velocity measurements in particle sedimentation regimes. J Atmos Ocean Tech 31: 366–386. doi: 10.1175/JTECH-D-11-00202.1
![]() |
[30] |
Tanelli S, Im E, Durden SL, et al. (2002) The effects of nonuniform beam filling on vertical rainfall velocity measurements with a spaceborne Doppler radar. J Atmos Ocean Tech 19: 1019–1034. doi: 10.1175/1520-0426(2002)019<1019:TEONBF>2.0.CO;2
![]() |
[31] | LeMone MA, Zipser EJ (1980) Cumulonimbus vertical velocity events in GATE. Part I: Diameter, intensity, and mass flux. J Atmos Sci 37: 2444–2457. |
[32] |
Jorgensen DP, Zipser EJ, LeMone MA (1985) Vertical motions in hurricanes. J Atmos Sci 42: 839–856. doi: 10.1175/1520-0469(1985)042<0839:VMIIH>2.0.CO;2
![]() |
[33] |
Jorgensen DP, LeMone MA (1989) Vertical velocity characteristics of oceanic convection. J Atmos Sci 46: 621–640. doi: 10.1175/1520-0469(1989)046<0621:VVCOOC>2.0.CO;2
![]() |
[34] |
Black ML, Burpee RW, Marks FD (1996) Vertical motion characteristics of tropical cyclones determined with airborne Doppler radial velocities. J Atmos Sci 53: 1887–1909. doi: 10.1175/1520-0469(1996)053<1887:VMCOTC>2.0.CO;2
![]() |
[35] |
Anderson NF, Grainger CA, Stith JL (2005) Characteristics of Strong Updrafts in Precipitation Systems over the Central Tropical Pacific Ocean and in the Amazon. J Appl Meteorol 44: 731–738. doi: 10.1175/JAM2231.1
![]() |
[36] |
Heymsfield GM, Tian L, Heymsfield AJ, et al. (2010) Characteristics of deep tropical and subtropical convection from nadir-viewing high-altitude airborne Doppler radar. J Atmos Sci 67: 285–308. doi: 10.1175/2009JAS3132.1
![]() |
[37] | Atlas D, Srivastava RC. Sekhon S (1973) Doppler radar characteristics of precipitation at Vertical Incidence, Rev Geophys 11: 1–35. |
[38] |
Durden SL, Im E, Li FK, et al. (1994) ARMAR: An airborne rain mapping radar. J Atmos Ocean Tech 11: 727–737. doi: 10.1175/1520-0426(1994)011<0727:AAARMR>2.0.CO;2
![]() |
[39] |
Tanelli S, Durden SL, Im E (2006) Simultaneous measurements of Ku- and Ka-band sea surface cross-sections by an airborne radar. IEEE Geosci Remote S 3: 359–363. doi: 10.1109/LGRS.2006.872929
![]() |
[40] |
Meneghini R, Kim H, Liao L, et al. (2015) An initial assessment of the Surface Reference Technique applied to data from the Dual-Frequency Precipitation Radar (DPR) on the GPM satellite. J Atmos Ocean Tech 32: 2281–2296. doi: 10.1175/JTECH-D-15-0044.1
![]() |
[41] |
Durden SL, Haddad ZS (1998) Comparison of radar rainfall retrieval algorithms in convective rain during TOGA COARE. J Atmos Ocean Tech 15: 1091–1096. doi: 10.1175/1520-0426(1998)015<1091:CORRRA>2.0.CO;2
![]() |
[42] | Houze RA (2004) Mesoscale convective systems. Rev Geophys 42: 237–286. |
[43] | Awaka J, Iguchi T, Kumagai H, et al. (1997) Rain type classification algorithm for TRMM Precipitation Radar. In: IGARSS'97. 1997 IEEE International Geoscience and Remote Sensing Symposium Proceedings. Remote Sensing-A Scientific Vision for Sustainable Development, pp. 1633–1635. |
[44] |
Haddad ZS, Short DA, Durden SL, et al. (1997) A new parametrization of the rain drop size distribution. IEEE T Geosci Remote 35: 532–539. doi: 10.1109/36.581961
![]() |
[45] | Davies ER (2005) Machine Vision: Theory, Algorithms and Practicalities, Third Edition. Amsterdam, Netherlands: Elsevier. |
[46] | Elsner JB, Jagger TH (2013) Hurricane Climatology: A Modern Statistical Guide Using R. Oxford, UK: Oxford University Press. |
[47] | Wilcox RR (2010) Fundamentals of Modern Statistical Methods, 2nd Edition. New York, NY, USA: Springer. |
[48] | Haykin S (1978) Communication Systems. New York, NY, USA: Wiley. |
[49] | Bracewell RN (1978) The Fourier Transform and Its Applications, 2nd Ed. McGraw-Hill. |
[50] |
Sørland SL, Sorteberg A (2015) The dynamic and thermodynamic structure of monsoon low-pressure systems during extreme rainfall events. Tellus A: Dynamic Meteorology and Oceanography 67: 27039. doi: 10.3402/tellusa.v67.27039
![]() |
[51] |
Durden SL (2018) Relating GPM radar reflectivity profile characteristics to path-integrated attenuation. IEEE T Geosci Remote 56: 4065–4074. doi: 10.1109/TGRS.2018.2821601
![]() |
[52] |
Battaglia A, Tanelli S, Kobayashi S, et al. (2010) Multiple-scattering in radar systems: A review. J Quant Spectroscopy Radiative Trans 111: 917–947. doi: 10.1016/j.jqsrt.2009.11.024
![]() |
[53] |
Long DG, Brodzik MJ (2016) Optimum image formation for spaceborne microwave radiometer products. IEEE T Geosci Remote 54: 2763–2779. doi: 10.1109/TGRS.2015.2505677
![]() |
[54] | Wolde M, Battaglia A, Nguyen C, et al. (2019) Implementation of polarization diversity pulse-pair technique using airborne W-band radar. Atmos MeasTech 12: 253–269. |
[55] |
Atlas D, Srivastava RC, Sloss PW (1969) Wind shear and reflectivity gradient effects on Doppler radar spectra: II. J Appl Meteorol 8: 384–388. doi: 10.1175/1520-0450(1969)008<0384:WSARGE>2.0.CO;2
![]() |
[56] |
Tanelli S, Im E, Durden SL, et al. (2004) Rainfall Doppler velocity measurements from spaceborne radar: Overcoming NUBF effects. J Atmos Ocean Tech 21: 27–44. doi: 10.1175/1520-0426(2004)021<0027:RDVMFS>2.0.CO;2
![]() |
[57] |
Durden SL, Fischman MA, Johnson RA, et al. (2007) An FPGA-based Doppler processor for a spaceborne precipitation radar. J Atmos Ocean Tech 24: 1811–1815. doi: 10.1175/JTECH2086.1
![]() |
1. | Stephen L. Durden, Estimating Mean Surface Backscatter from GPM Surface Backscatter Observations, 2021, 2, 2673-4117, 492, 10.3390/eng2040031 |
Footprint Size (km) | PIA StandardDev (dB) | PIA Bias (dB) | Z Std Dev (dB) | Velocity Error (m/s) |
9.7 | 5.1 | 1.9 | 7.8 | 40 |
6.8 | 4.5 | 1.6 | 7.2 | 27 |
5.4 | 4.0 | 1.4 | 6.8 | 20 |
2.5 | 2.9 | 0.7 | 5.3 | 8 |
1.1 | 1.8 | 0.2 | 3.7 | 2 |