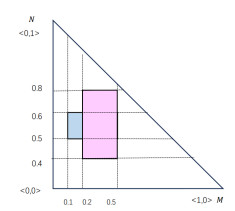
The interval-valued intuitionistic fuzzy set (IVIFS), which is an extension of the intuitionistic fuzzy set (IFS), characterizes the membership and non-membership degree with the number of intervals. This paper begins with an introduce to order relation, which is embedding. Based on the embedding, we have proposed a separation measure for intervals, and used the coimplication function and interval width to construct it. Then, considering epistemic interpretation of IVIFSs, we generalized the measure of the interval values to IVIFS, and obtained the IVI-separation measure, which can compare the accuracy of two elements on IVIFSs. At the same time, a special construction method was provided based on aggregate functions. Finally, we studied the separation measure of intersection, union, and complement operations on IVIFSs.
Citation: Yaoyao Gong, Zengtai Gong. Measures of separation for interval-valued intuitionistic fuzzy sets and their applications[J]. AIMS Electronics and Electrical Engineering, 2025, 9(2): 139-164. doi: 10.3934/electreng.2025008
[1] | Romain Breuneval, Guy Clerc, Babak Nahid-Mobarakeh, Badr Mansouri . Classification with automatic detection of unknown classes based on SVM and fuzzy MBF: Application to motor diagnosis. AIMS Electronics and Electrical Engineering, 2018, 2(3): 59-84. doi: 10.3934/ElectrEng.2018.3.59 |
[2] | J. Rajeshwari, M. Sughasiny . Dermatology disease prediction based on firefly optimization of ANFIS classifier. AIMS Electronics and Electrical Engineering, 2022, 6(1): 61-80. doi: 10.3934/electreng.2022005 |
[3] | Ryan Michaud, Romain Breuneval, Emmanuel Boutleux, Julien Huillery, Guy Clerc, Badr Mansouri . Application of blind source separation to the health monitoring of electrical and mechanical faults in a linear actuator. AIMS Electronics and Electrical Engineering, 2019, 3(4): 328-346. doi: 10.3934/ElectrEng.2019.4.328 |
[4] | Abhijeet Kumar, Arpit Kumar . Contactless temperature and distance measuring device: A low-cost, novel infrared ―based "Badge"-shaped structural model for measuring physical distance and body temperature. AIMS Electronics and Electrical Engineering, 2022, 6(1): 43-60. doi: 10.3934/electreng.2022004 |
[5] | Nikos Petrellis . Skin disorder diagnosis with ambiguity reduction assisted by lesion color adaptation. AIMS Electronics and Electrical Engineering, 2019, 3(3): 290-308. doi: 10.3934/ElectrEng.2019.3.290 |
[6] | Anjan Ku. Sahoo, Ranjan Ku. Jena . Improved DTC strategy with fuzzy logic controller for induction motor driven electric vehicle. AIMS Electronics and Electrical Engineering, 2022, 6(3): 296-316. doi: 10.3934/electreng.2022018 |
[7] | Ashraf A. Ahmad, Ameer Mohammed, Mohammed Ajiya, Zainab Yunusa, Habibu Rabiu . Estimation of time-parameters of Barker binary phase coded radar signal using instantaneous power based methods. AIMS Electronics and Electrical Engineering, 2020, 4(4): 347-358. doi: 10.3934/ElectrEng.2020.4.347 |
[8] | Jun Yoneyama . H∞ disturbance attenuation of nonlinear networked control systems via Takagi-Sugeno fuzzy model. AIMS Electronics and Electrical Engineering, 2019, 3(3): 257-273. doi: 10.3934/ElectrEng.2019.3.257 |
[9] | Reiner North . Sensitivity validation of a fuzzy system for asset allocation. AIMS Electronics and Electrical Engineering, 2020, 4(2): 169-187. doi: 10.3934/ElectrEng.2020.2.169 |
[10] | Patrick Seeling . Augmented Reality Device Operator Cognitive Strain Determination and Prediction. AIMS Electronics and Electrical Engineering, 2017, 1(1): 100-110. doi: 10.3934/ElectrEng.2017.1.100 |
The interval-valued intuitionistic fuzzy set (IVIFS), which is an extension of the intuitionistic fuzzy set (IFS), characterizes the membership and non-membership degree with the number of intervals. This paper begins with an introduce to order relation, which is embedding. Based on the embedding, we have proposed a separation measure for intervals, and used the coimplication function and interval width to construct it. Then, considering epistemic interpretation of IVIFSs, we generalized the measure of the interval values to IVIFS, and obtained the IVI-separation measure, which can compare the accuracy of two elements on IVIFSs. At the same time, a special construction method was provided based on aggregate functions. Finally, we studied the separation measure of intersection, union, and complement operations on IVIFSs.
Zadeh [1] published the initial seminal paper on fuzzy sets in 1965. Traditional binary logic is broken by fuzzy sets, where the membership function determining whether an object belongs to this set can be analyzed in $ [0, 1] $. Its development makes it possible for the computer to imitate the human brain to recognize the complex system and improve the level of automation. On the other hand, its shortcomings have increasingly come to light as fuzzy information processing technology has matured. Therefore, there are other methods in which fuzzy set theory was expanded. The intuitionistic fuzzy set (IFS) proposed by Atanassov [2] is the most active and fruitful development theory among many others. The interval-valued intuitionistic fuzzy set [3] was formed by combining it with interval-valued fuzzy set (IVFS) [4] proposed by Zadeh. The Bulgarian scholar Atanassov was the first to propose it, transforming the membership and non-membership degrees from a single number to a subinterval on $ [0, 1] $. Therefore, it is more flexible and practical in dealing with vagueness and uncertainty. For example, Manda et al. [5] recently looked at a hot issue---supervisor selection for doctoral students. They applied the hybrid multi-criteria decision-making method to the IVIF environment, providing a scientific model for students to choose the right tutor.
Through the viewpoints and analysis of set value proposed by Couso et al. [6], we can take into account epistemic interpretation for an IVIFS, which can assume that there exists a real-valued degree of membership and non-membership within the membership interval of possible membership and the non-membership interval of possible non-membership, respectively.
For the study of IVIFSs, after entering the 21st century, in addition to continuing to study from the perspective of mathematics, there are gradually related applied studies. Hong [7] and Xu et al. [8] studied its correlation coefficient, and Mondal [9] discussed its classification of topological structure and topological spaces. Besides, the relationship between two elements on an IVIFS is also a vital subject. Information measure is a useful tool for processing them, including the distance, similarity, inclusion, entropy, and so on. The distances of Burillo and Bustince were extended to the IVIFS by Park [10], and a distance on the IVIFS using the Hausdorff and Euclidean metrics was presented. Thereafter, Gao and Sun et al. [11], Tiwari and Gupta [12,13], and Rashid et al. [14] constructed distance measures from different aspects. Liu and Jiang [15] defined a distance measure of the interval-valued intuitionistic fuzzy set based on the distance of the number of intervals, which is used to evaluate a house and make purchasing decisions. Malik and Gupta [16] studied the division and subtraction operations of interval-valued intuitionistic fuzzy sets using the Hamming distance, and used it to make optimal choices for capital investment, reducing the risks for investment companies. Li and Suo [17] proposed a new knowledge measure function based on the distance function of IVIFSs, which was used to select the appropriate air-conditioning system for a library.
In the same year of establishing fuzzy sets, a tool for characterizing the extent of fuzziness of fuzzy sets, called entropy, was initially introduced by Zadeh [19]. Later, Liu [20] and Zhang [21] proposed a series of axiomatic requirements for the entropy measure of an IVIFS, extending the axioms of the definitions of Szmidt and Kacprzyk. Hereafter, Zhao defined the entropy of the IVIFS axiomatically in literature[22]. Jin and Pei et al. [23] proposed interval-valued intuitionistic fuzzy continuous weighted entropy using the continuous ordered weighted average operator, and applied it to multi-criteria group decision-making to evaluate emergency management and reduce risks. Nazari and Mosapour [24] proposed the concept of entropy of a dynamical system on an interval-valued intuitionistic fuzzy set based on discriminative entropy, which can be used to measure fuzzy experimental information. In order to better deal with decision-making problems in the real world, Ohlan [25] introduced a new exponential entropy application to multi-criteria decision-making in the context of interval-valued intuitionistic fuzzy sets. They used this method to select the best investment plan for a bank and get the highest profit.
For the purpose of describing the extent to which one fuzzy set is included in another, Sinha [26] created the inclusion measure. Extending upon Zeng's idea of the inclusion measure of IVFSs in [27], Zhang et al. [28,29] introduced the inclusion axiom among IVIFSs. Zhang and Yang et al. [30] proposed the hybrid monotonic (HM) inclusion measure of IFSs, which ranked seven ecoregions in Hubei Province. The HM inclusion measure on IVIFSs is given for the selection of corporate partners. Furthermore, similarity measures also play an important role in the theory of IVIFSs. Wei et al. [31] derived the similarity measure of IVIFSs from the entropy measurement. In 2009, Xu and Yager [32] studied the preference relationship and similarity in the context of interval-valued intuitionistic ambiguity. In order to improve the counter-intuitive case that occurs in the classical similarity measure, Xu and Xia [33] considered hesitation in the similarity measure. A novel cosine similarity for interval-valued intuitionistic fuzzy sets was created by Singh [34] in 2012 utilizing the modulus formula. The similarity of IVIFSs taking hesitation into account was introduced and applied to expert system issues by Wu et al. [35] in 2014. In order to solve the problem of interval-valued intuitionistic multi-criteria decision-making in fuzzy environments, Luo and Liang [36] created a novel similarity measure in 2018. The non-hesitant score function's interval value served as the foundation for Jeevaraj [37] in 2020. When it comes to solving pattern reorganization difficulties, intuition obscures the similarities between numbers. Alolaiyan and Razq et al. [38] improved the similarity measure on the interval-valued intuitionistic fuzzy set. It is used to evaluate the quality of software and develop the best production strategy for each product.
In 2020, Bustince et al. studied a distance measure for IVFSs, which was the first time that the width of the membership interval was considered in the calculation of the distance measure [39]. On this basis, Li [18] suggested a technique that makes the uncertainty of input and output tightly associated by building distance measures of IVIFSs based on interval widths. In several practical issue environments, the relation between two IVIFSs is significant. For example, the exclusion of some causes problems in medical diagnosis, pattern recognition, and so on. Thus, it makes sense to explore how to build a relationship between them.
In short, with the increasing demand for processing fuzzy information in real life, the research on IVIFSs is deepening. Fuzzy entropy is the study of a single set, and the construction of similarity and inclusion is based on uncertainty evaluation, order relations, and aggregation operations. IVIFSs need to be compared differently in many modeling frameworks, such as company decision analysis, medical diagnosis, and image processing. Therefore, the question of establishing the relationship between two IVIFSs is a topic of practical significance. The accuracy of membership and non-affiliation is very important. Inspired by this, in order to compare one IVIFS more accurately than another, we introduce a separation measure. This paper's primary contributions are as follows:
● The interval separation measure is proposed to measure the degree of separation between two intervals. The axiomatic definition and the construction method based on the entailment function and the interval width are given.
● In the context of IVIFSs, IVI-separation is proposed as a new measure, which can be used to compare the accuracy of two elements. A special construction method based on the aggregate function is given.
● On the basis of the interval-valued t-norm and interval-valued t-conorm, the intersection and union operations on IVIFSs are defined. Other properties and IVI-separation measures are proposed.
The rest of the paper is organized as follows: In Section $ 2 $, we briefly list a few of the fundamental principles of IVIFSs needed in this paper. In Section $ 3 $, we define the separation measure of intervals and discuss some of its theorems, along with two different construction techniques. In Section $ 4 $, we explore the definition of the separation degree for IVIFSs. In Section $ 5 $, the separation degree of the main operations on IVIFSs are introduced. Section $ 6 $ offers some examples, Section $ 7 $ is the discussion, and Section $ 8 $ is the conclusion.
In this section, we will briefly recall several basic notions of interval-valued intuitionistic fuzzy sets (IVIFSs). Specifically, two important partial orders on IVIFSs will be given. Let $ [I] $ denote the set of closed subintervals of the unit interval $ [0, 1] $.
Definition 2.1. Let $ \alpha = [\alpha^-, \alpha^+] $, $ \beta = [\beta^-, \beta^+] $ be any two intervals in $ [I] $. Then two partial orders are defined below:
(1) $ \alpha \subseteq \beta \; \Longleftrightarrow \; \beta^-\leq\alpha^-\leq\alpha^+\leq\beta^+ $,
(2) $ \alpha \leq_L \beta \; \Longleftrightarrow \; \alpha^-\leq\beta^- $ and $ \alpha^+\leq\beta^+ $.
The above orders are named contained and lattice orders, respectively. Considering the above two relations, we can define the partial-order relation of IVIFSs.
Definition 2.2. [3] Let $ X $ denote the finite set of points. An interval-valued intuitionistic fuzzy set $ A $ over $ X $ is defined as
$ A = \{\langle x,M_{A}(x),N_{A}(x)\rangle \mid x\in X\}, $ |
where $ M_{A}(x), N_A(x) $ is called the interval membership degree and the interval non-membership degree of an element $ x_i $ to $ A $, respectively, i.e.,
$ M_{A}(x) : X \rightarrow [I], $ |
$ N_{A}(x) : X \rightarrow [I], $ |
and for every $ x \in X $,
$ 0 \leq \sup M_{A} + \sup N_{A} \leq 1. $ |
The collection of all interval intuitionistic fuzzy sets on $ X $ is denoted as IVIFS($ X $). For convenience, the lower and upper bound of $ M_A(x) $ and $ N_A(x) $ are denoted by $ M_A(x)^- $, $ M_A(x)^+ $, $ N_A(x)^- $, and $ N_A(x)^+ $, respectively. Thus,
$ M_{A}(x) = [M_A(x)^-,M_A(x)^+], $ |
$ N_{A}(x) = [N_A(x)^-,N_A(x)^+]. $ |
Remark 2.3. Obviously, IVIFSs can be converted to IFSs and IVFSs under certain conditions:
(1) IVIFS = IFS $ \Longleftrightarrow \; M_A(x)^- = M_A(x)^+ $ and $ N_A(x)^- = N_A(x)^+ $,
(2) IVIFS = IVFS $ \Longleftrightarrow \; N_{A}(x) = \emptyset $,
for any $ x\in X $.
Definition 2.4. Let $ A, B $ be two IVIFSs, and we define
(1) $ A \sqsubseteq B \; \Longleftrightarrow \; M_A(x) \subseteq M_B(x) $ and $ N_B(x) \subseteq N_A(x) $,
(2) $ A \subseteq B \; \Longleftrightarrow \; M_A(x) \leq_L M_B(x) $ and $ N_B(x) \leq_L N_A(x) $,
for any $ x\in X $.
The above orders are named embedded and inclusion, respectively.
Example 2.5. Let $ A $, $ B $, $ C\in $ IVIFS($ X $).
$ A = \{\langle[0.2,0.5],[0.4,0.8]\rangle\}, $ |
$ B = \{\langle[0.1,0.5],[0.5,0.6]\rangle\}, $ |
$ C = \{\langle[0.3,0.6],[0.2,0.7]\rangle\}. $ |
Note that $ A \sqsubseteq B $ as $ [0.2, 0.5] \subseteq [0.1, 0.5] $, $ [0.5, 0.6]\subseteq[0.4, 0.8] $. While $ A \nsubseteq B $, because it does not satisfy $ M_A \leq_L M_B $ and $ N_B \leq_L N_A $. On the other hand, we can obverse $ A \subseteq C $ when we have $ [0.2, 0.5] \leq_L [0.3, 0.6] $ and $ [0.2, 0.7]\leq_L [0.4, 0.8] $. In addition, $ B $ does not have any partial-order relationship with $ C $.
The following figures show the order relation of $ A, B $, and $ C $ above.
In this paper, there are two types of relations: inclusion and embedding of in interval-valued intuitionistic fuzzy set. The example above makes it clear that they are totally distinct from one another. Inclusion degree describes the extent to which one set is contained by another set. It is a quantitative description of the inclusion relationship, which includes the uncertainty of the relationship. Embedding compares the accuracy of information on the IVIFS.
In this section, we discuss axioms of the separation of intervals. Information measures have been studied in the literature. For example, to measure the extent to which one interval is included in another, they utilized inclusion measures. Here, we attempt to propose an interval separation theorem and calculation method to measure the separation degree of intervals. The first is the case of the minimal degree of separation, which occurs when the first interval is completely contained by the second interval, and their separation degree is zero. On the contrary, if two intervals have no common element, then the separation measure is maximum, which is one. Next, along with the increase of the second variable, we know that the separation is decreasing. It states that if interval $ \beta $ is a subinterval of $ \gamma $, for $ \alpha $ in $ \gamma $, the separation is less than or equal to the separation of $ \alpha $ in $ \beta $ eternally. Finally, if intervals have an inclusion relation, i.e., in the interval $ \alpha\subseteq\beta\subseteq\gamma $, the interval $ \gamma $ is always more separated from $ \alpha $ than $ \beta $ is from $ \alpha $.
The above axioms express the essence of the separation of intervals, that is, when there are fewer common elements between two intervals, their separation degree is higher. Thus, we have the definition of separation as follows.
Definition 3.1. Let $ S $: $ [I]^2 \rightarrow [0, 1] $ be a function, and if the following holds:
$ (1) \; S(\alpha, \beta) = 0 \Longleftrightarrow \alpha \subseteq \beta $,
$ (2) $ if $ \alpha \neq \emptyset $ and $ \alpha \cap \beta = \emptyset $, then $ S(\alpha, \beta) = S(\beta, \alpha) = 1 $,
$ (3) $ if $ \beta \subseteq\gamma $, then $ S(\alpha, \beta) \geq S(\alpha, \gamma) $,
$ (4) $ if $ \alpha\subseteq\beta $ and $ \beta\subseteq\gamma $, then $ S(\gamma, \alpha) \geq S(\beta, \alpha) $ and $ S(\gamma, \alpha) \geq S(\gamma, \beta) $,
then $ S $ is a separation measure of intervals.
The following are a few properites of the interval separation on $ [I] $.
Proposition 3.2. For every $ \alpha, \beta, \gamma \in [I] $, then
(1) $ S(\alpha, \alpha \cup \beta) = S(\beta, \alpha \cup \beta) = S(\alpha \cap \beta, \alpha) = S(\alpha \cap \beta, \beta) = 0 $,
(2) $ S(\alpha, \beta \cap \gamma) \geq \max\{S(\alpha, \beta), S(\alpha, \gamma)\} $.
Proof. The proof is easy to understand.
In order to quantify the separation of intervals, next, we study the different construction methods. In this paper, by using the interval width and coimplication function, we attempt to provide the construction of separation measures.
Definition 3.3. [40,41] The function $ I_c $: $ [0, 1]^2 \rightarrow [0, 1] $ is called a coimplication if it satisfies the following conditions:
$ (1) $ if $ x \leq z $, then $ I_c(x, y) \geq I_c(z, y) $, for any $ y\in[0, 1] $,
$ (2) $ if $ y \leq t $, then $ I_c(x, y) \leq I_c(x, t) $, for any $ x\in[0, 1] $,
$ (3) \; I_c(0, 0) = I_c(1, 1) = 0 $,
$ (4) \; I_c(0, 1) = 1 $,
$ (5) \; I_c(x, y) = 0 \Longleftrightarrow x \geq y $.
Proposition 3.4. Let $ I_c $ be a coimplication function satisfying Definition 3.3 and $ S_{Ic} $: $ [I]^2 \rightarrow [0, 1] $ is a separation measure of intervals, then
$ (1) $ if $ \alpha\cap \beta = \emptyset $, then $ S_{Ic}(\alpha, \beta) = 1 $,
$ (2) $ if $ \alpha\cap \beta\neq \emptyset $, then $ S_{Ic}(\alpha, \beta) = \max(I_c(\alpha^-, \beta^-), I_c(\beta^+, \alpha^+)). $
Proof. It is evident that the value of $ S_{Ic} $ is between $ 0 $ and $ 1 $. Therefore, let us prove that $ S_{Ic} $ satisfies four conditions of Definition 3.1.
$ (1) $ Given $ S_{Ic}(\alpha, \beta) = 0 $, then $ I_c(\alpha^-, \beta^-) = 0 $ and $ I_c(\beta^+, \alpha^+) = 0 $ by the ordering of $ I_c $. Thus, we have that $ \beta^- \leq \alpha^- $ and $ \alpha^+\leq\beta^+ $ by Definition 3.3 (5), that is, $ \alpha\subseteq\beta $.
$ (2) $ Given $ \alpha\cap\beta = \emptyset $, then through the definition of separation, we know that $ S_{Ic}(\alpha, \beta) = 1 $. In the contrary direction, $ S_{Ic}(\beta, \alpha) = 1 $ can be held.
$ (3) $ Given $ \beta \subseteq \gamma $, we have $ \alpha\cap\beta = \emptyset $ when $ \alpha\cap\gamma = \emptyset $, and then $ \alpha\cap\beta = \emptyset $. Thus, $ S_{Ic}(\alpha, \gamma) = S_{Ic}(\alpha, \beta) = 1 $. Given $ \beta \subseteq \gamma $ but $ \alpha\cap\gamma \neq\emptyset $, then we divide it into a different case. The first case is $ \alpha \cap \beta = \emptyset $, and we obtain that $ S_{Ic}(\alpha, \beta) = 1\geq S_{Ic}(\alpha, \gamma) $. Moreover, if $ \alpha \cap \beta\neq\emptyset $, we can know that $ I_c(\alpha^-, \beta^-)\geq I_c(\alpha^-, \gamma^-) $, $ I_c(\beta^+, \alpha^+)\geq I_c(\gamma^+, \alpha^+) $ because $ \gamma^-\leq\beta^- $, $ \beta^+\leq\gamma^+ $, and then $ \max(I_c(\alpha^-, \beta^-), I_c(\alpha^+, \beta^+)) \geq \max(I_c(\alpha^-, \gamma^-), I_c(\alpha^+, \gamma^+)) $. Thus, $ S_{Ic}(\alpha, \beta)\geq S_{Ic}(\alpha, \gamma) $.
$ (4) $ Given $ \alpha \subseteq \beta $ and $ \beta\subseteq \gamma $, then $ \gamma^+\geq\beta^+\geq\alpha^+\geq \alpha^-\geq\beta^-\geq\gamma^- $. So we have $ I_c(\gamma^-, \alpha^-)\geq I_c(\beta^-, \alpha^-) $, $ I_c(\alpha^+, \gamma^+)\geq I_c(\alpha^+, \beta^+) $ by Definition 3.3 (1)–(2). Thus, $ S_{Ic}(\gamma, \alpha)\geq S_{Ic}(\beta, \alpha) $.
A series of coimplication functions fulfilling properties $ (1) $–$ (5) $ are given as follows [40].
Proposition 3.5. Let $ g $: $ [0, 1]^2 \rightarrow [0, 1] $ be a mapping. If $ y \leq x $, then
$ (1) \; g(x, y) \geq g(z, y) $ if $ x \leq z $,
$ (2) \; g(x, y) \leq g(x, t) $ if $ y \leq t $,
$ (3) \; g(0, 1) = 1 $,
for any $ z, t\in[0, 1] $.
For simplicity, we assume $ \Box = \{(x, y)\in [0, 1]^2|x < y\} $.
Thus, the function $ I_{c_g} $ is a coimplication as follows,
$ \begin{equation*} {I_{c_g}(x,y)} = \begin{cases} 0,& \mathit{\text{ if }}\ x\geq y,\\ g(x,y),& \mathit{\text{ if }}\ x < y. \end{cases} \end{equation*} $ |
Proof. Since $ g(x, y) $ satisfies Definition 3.3 $ (1) $ and $ (2) $, it is immediate to verify that $ I_{c_g} $ fulfills conditions $ (1) $, $ (2) $, and ordering $ (5) $. For function $ I_{c_g} $, by the definition we have that $ I_{c_g}(0, 0) = I_{c_g}(1, 1) = 0, \; I_{c_g}(0, 1) = g(0, 1) = 1 $. So $ I_{c_g} $ is a coimplication.
For a variety of fuzzy coimplications, we take into account the partial order that the order of the functions that created them induces from the unit interval [0, 1], in accordance with the terminology that was previously introduced.
Corollary 3.6. Let $ g_1, g_2 $ be coimplication functions. If $ g_1\leq g_2 $, then $ S_{I_{c_{g_1}}}\leq S_{I_{c_{g_2}}} $.
Proof. For every $ (x, y)\in \Box $, since $ g_1\leq g_2 $, then $ I_{c_{g_1}}\leq I_{c_{g_2}} $. From $ \alpha\cap\beta = \emptyset $, we can obtain that $ S_{I_{c_{g_1}}}(\alpha, \beta) = S_{I_{c_{g_2}}}(\alpha, \beta) = 1 $ by the definition of separation. Otherwise, since $ I_{c_{g_1}}(\alpha^-, \beta^-)\leq I_{c_{g_2}}(\alpha^-, \beta^-) $ and $ I_{c_{g_1}}(\beta^+, \alpha^+) \leq I_{c_{g_2}}(\beta^+, \alpha^+) $, then $ S_{I_{c_{g_1}}}\leq S_{I_{c_{g_2}}} $.
The coimplication functions which fulfill the order (5) and their associated separation measures can be obtained in accordance with Proposition 3.5. Table 1 presents and discusses the corresponding separations for the coimplication functions that were presented in reference[40].
Lukasiewicz | |
$ g_{LK}(x, y)=y-x $ | $ S_{LK}=\left\{\begin{array}{cl}1 & \ \text{if} \ \alpha\cap\beta=\emptyset \\ \max(\beta^–\alpha^-, \alpha^+-\beta^+, 0) & \ \text{otherwise} \end{array}\right. $ |
Kleene-Dienes | |
$ g_{KD}(x, y)=\min(1-x, y) $ | $ S_{KD}=\left\{\begin{array}{cl}0 & \ \text{if}\ \alpha\subseteq\beta \\ 1 & \ \text{if}\ \alpha\cap\beta=\emptyset \\ \min(1-\beta^+, \alpha^+) & \ \text{if}\ \beta^- < \alpha^- \leq\beta^+ < \alpha^+ \\ \min(1-\alpha^-, \beta^-) & \ \text{if}\ \alpha^- < \beta^-\leq\alpha^+ < \beta^+ \\ \max\{\min(1-\alpha^-, \beta^-), \min(1-\beta^+, \alpha^+)\} & \ \text{if}\ \beta\subset\alpha \end{array}\right. $ |
Gödel | |
$ g_{GD}(x, y)=y $ | $ S_{GD}=\left\{\begin{array}{cl}0 & \ \text{if}\ \alpha\subseteq\beta \\ 1 & \ \text{if}\ \alpha\cap\beta=\emptyset \\ \beta^- & \ \text{if}\ \alpha^-\leq\beta^-\leq\alpha^+\leq\beta^+ \\ \alpha^+ & \ \text{otherwise} \end{array}\right. $ |
Goguen | |
$ g_{GG}(x, y)=\cfrac{y-x}{1-x} $ | $ S_{GG}=\left\{\begin{array}{cl}0 & \ \text{if}\ \alpha\subseteq\beta \\ 1 & \ \text{if}\ \alpha\cap\beta=\emptyset \\ \cfrac{\beta^–\alpha^-}{1-\alpha^-} & \ \text{if}\ \alpha\cap\beta\neq\emptyset\wedge\beta^+=1 \\ \max\{\cfrac{\beta^–\alpha^-}{1-\alpha^-}, \cfrac{\alpha^+-\beta^+}{ 1-\beta^+} \} & \ \text{otherwise}\ \end{array}\right. $ |
As a consequence of Corollary 3.6, we can establish an order among the different separations described earlier.
Proposition 3.7. Let $ S_{LK} $, $ S_{KD} $, $ S_{GD} $, $ S_{GG} $ be different separation measures. Then $ S_{LK} \leq S_{KD} \leq S_{GD} $, and $ S_{LK} \leq S_{GG} \leq S_{GD} $.
Proof. The order relation between the coimplication functions constituting the separation measures is as follows. Since any $ (x, y)\in \Box $, then $ y < x $. So we get
$ g_{LK}(x,y) = y-x \leq \min(1-x,y) = g_{KD}, $ |
$ g_{KD}(x,y) = \min(1-x,y)\leq y = g_{GD}, $ |
and
$ g_{LK}(x,y) = y-x \leq (y-x)/(1-x) = g_{GG}. $ |
As $ (x, y)\in\Box $, then $ 1-x \geq 0 $ and $ y-1\leq 0 $. Hence, $ (y-x)/(1-x)-y = x(y-1)/(1-x)\leq0 $, that is, $ (y-x)/(1-x)\leq y $, i.e.,
$ g_{GG}(x,y)\leq g_{GD}(x,y). $ |
Then, $ g_{LK} \leq g_{KD} \leq g_{GD} $ and $ g_{LK} \leq g_{KD} \leq g_{GD} $. From this we can obtain the order relation between the above separation measures.
Note that since $ g_{KD} $ and $ g_{GG} $ are not in order, there is no order between the separation measures $ S_{KD} $ and $ S_{GG} $ formed by them. For example,
$ S_{GG}([0, 0.9], [0, 0.5]) = \max(0, 0.4/0.5) = 4/5 = 0.8, $
$ S_{KD}([0, 0.9], [0, 0.5]) = \max(\min(1, 0), \min(0.5, 0.9)) = \max(0, 0.5) = 0.5, $
but
$ S_{GG}([0, 0.6], [0, 0.5]) = \max(0, 0.1/0.5) = 1/5 = 0.2, $
$ S_{KD}([0, 0.6], [0, 0.5]) = \max(\min(1, 0), \min(0.5, 0.6)) = \max(0, 0.5) = 0.5. $
To illustrate the validation relationship more intuitively, we give the following example.
Example 3.8. To confirm the order of several distinct separations derived by the coimplication function, let us provide distinct intervals $ \alpha $ and $ \beta $. Suppose $ \beta = [0.2, 0.6] $, $ \beta = [0.4, 0.8] $. For $ \alpha_x = [x, x+0.1] $ for $ x\in[0, 0.9] $, a series of separation degrees between interval $ \alpha $ and $ \beta $ can be obtained through the calculation formula in Table 1, as shown in Figure 3. Next, we explore various intervals $ \alpha_y = [y, y+0.5] $ for $ y\in[0, 0.5] $, given the same $ \beta $. The result is shown in Figure 4.
From the figures above, it can be observed that different values of separation measures are obtained due to the different values of $ \alpha $ and $ \beta $. However the order relationship between $ S_{LK} $, $ S_{KD} $, $ S_{GD} $, and $ S_{GG} $ is fixed. Especially when $ \alpha\sqsubseteq\beta $ and $ \alpha\cap\beta = \emptyset $, the value is the same in all cases.
Interval width refers to the length or range of an interval, denoted by $ \omega $. It is defined as the difference between the left and right endpoints of the interval. If an interval is just a point, then its width is zero. In addition, we logically assume that the width of the empty set also is zero.
Definition 3.9. Let $ \Box = \{(x, y, z) \in [0, 1]^3\; |\; x \leq y, x \leq z\} $. The mapping $ \varphi $: $ \Box \rightarrow [0, 1] $ satisfies:
$ (1) \; \varphi(0, y, z) = 1 $,
$ (2) $ if $ x \neq 0 $, then $ \varphi(x, y, z) = 0 $ iff $ x = y $,
$ (3) \; \varphi(\cdot, y, \cdot) $ is decreasing,
$ (4) \; \varphi(x, \cdot, z) $ is increasing.
Proposition 3.10. Let $ S_{\varphi} $: $ [I]^2 \rightarrow [0, 1] $ be a separation measure of intervals. Then
$ (1) $ if $ \omega(\alpha)\neq 0 $, then $ S_{\varphi}(\alpha, \beta) = \varphi(\omega(\alpha \cap \beta), \omega(\alpha), \omega(\beta)), $ $ (2) $ if $ \omega(\alpha) = 0 $, then
$ (i) $ if $ \alpha \in \beta $, then $ S_{\varphi}(\alpha, \beta) = 0 $,
$ (ii) $ if $ \alpha \not\in \beta $, then $ S_{\varphi}(\alpha, \beta) = 1 $.
Proof. Let $ \alpha, \beta, \gamma\in [I] $. First, it is evident that the value of $ S_{\varphi} $ is between $ 0 $ and $ 1 $. Next, we need to prove that $ S_{\varphi} $ is a separation of the interval.
$ (1) $ At first, assume $ \omega(\alpha) = 0 $. According to the properties above we have $ \alpha \in \beta $ when $ S_{\varphi}(\alpha, \beta) = 0 $, and then $ \alpha \subseteq \beta $ by common sense. If $ \omega(\alpha) \neq 0 $, $ S_{\varphi}(\alpha, \beta) $ = $ \varphi(\omega(\alpha \cap \beta), \omega(\alpha), \omega(\beta)) = 0 $. According to Definition 3.9 (2), we can obtain that $ \omega(\alpha) = \omega(\alpha \cap \beta) $. It can only be $ \alpha \cap \beta = \alpha $ by the properties of intersection. It is said that $ \alpha \subseteq \beta $.
$ (2) $ Depending on the width of $ \alpha $, we can prove it with a different condition. Due to $ \alpha \neq \emptyset $ and $ \alpha \cap \beta = \emptyset $, first, if $ \omega(\alpha) = 0 $, $ S_{\varphi}(\alpha, \beta) = 1 $ if and only if $ \alpha \not\in \beta $, then it equals that $ \alpha \cap \beta = \emptyset $. Otherwise, we have that $ S_{\varphi}(\alpha, \beta) = \varphi(0, \omega(\alpha), \omega(\beta)) = 1 $ when $ \omega(\alpha)\neq 0 $ through the first condition of the map $ \varphi $. Besides, since $ \beta \cap \alpha = \alpha \cap \beta = \emptyset $, then $ S_{\varphi}(\alpha, \beta) = 1 $.
$ (3) $ Given $ \beta\subseteq\gamma $, first, if $ \omega(\alpha) = 0 $ and $ S_{\varphi }(\alpha, \beta) = 1 $, then $ \alpha\not\in\beta $. In the case where $ S_{\varphi}(\alpha, \beta) = 0 $, then $ \alpha \in \beta $. Therefore, $ \alpha \in \gamma $, and it is said that $ S_{\varphi}(\alpha, \gamma) = 0 $. Second, if $ \omega(\alpha) \neq 0 $, by $ \beta\subseteq\gamma $ we have that $ \omega(\beta) \leq \omega(\gamma) $, and by the properties of intersection we also have $ \omega(\alpha \cap \beta)\leq \omega(\alpha \cap \gamma) $. Thus, $ S_\varphi(\alpha, \beta)\geq S_{\varphi}(\alpha, \gamma) $ since $ \varphi $ is decreasing with respect to the first and third variable.
$ (4) $ If $ \alpha\subseteq\beta\subseteq\gamma $, first, if $ \omega(\gamma) = 0 $, then $ \omega(\alpha) = \omega(\beta) = \omega(\gamma) = 0 $ and $ \alpha = \beta = \gamma $. Thus, we can obtain that $ S_{\varphi }(\gamma, \alpha) = S_{\varphi}(\beta, \alpha) = 0 $. Second, given $ \omega(\gamma) \neq 0 $, we have $ \omega(\alpha) = \omega(\beta) = 0 $ when $ \omega(\beta) = 0 $, and then $ \alpha = \beta $. Thus, $ S_{\varphi }(\beta, \alpha) = 0\leq S_{\varphi}(\gamma, \alpha) $. In addition, in the second case where $ \omega(\beta) \neq 0 $, then $ S_{\varphi }(\beta, \alpha) = \varphi(\omega(\alpha), \omega(\beta), \omega(\alpha)) $ as well as $ S_{\varphi }(\gamma, \alpha) = \varphi(\omega(\alpha), \omega(\gamma), \omega(\alpha)) $. As $ \beta\subseteq \gamma $, we have that $ \omega(\beta)\leq \omega(\gamma) $. So $ S_{\varphi }(\gamma, \alpha) \geq S_{\varphi }(\beta, \alpha) $.
The following are mainly some corollaries about Proposition 3.10.
Corollary 3.11. The function $ S_\varphi^*(x) $: $ [I]^2 \rightarrow [0, 1] $ is a separation measure of the interval. Then
$ (1) $ if $ \omega(\alpha)\neq 0 $, then $ S_{\varphi}(\alpha, \beta) = 1-\cfrac{\omega(\alpha \cap \beta)}{\omega(\alpha)}, $
$ (2) $ if $ \omega(\alpha) = 0 $, then
$ (i) $ if $ \alpha \in \beta $, then $ S_{\varphi}(\alpha, \beta) = 0 $,
$ (ii) $ if $ \alpha \not\in \beta $, then $ S_{\varphi}(\alpha, \beta) = 1 $.
Proof. We can know that $ S_\varphi^*(x) = S_\varphi $ when the function $ \varphi $ is defined by
$ (1) $ if $ x = 0 $, then $ \varphi(x, y, z) = 1 $,
$ (2) $ if $ x\neq 0 $, then $ \varphi(x, y, z) = 1-\cfrac{x}{y} $.
It is clear that $ \varphi $ is a function that Corollary 3.11, since
$ (1) \; \varphi(0, y, z) = 1 $,
$ (2) $ if $ x \neq 0 $ and $ x = y $, then $ \varphi(x, y, z) = 0 $,
$ (3) $ if $ x\neq0 $, then $ 1-\cfrac{x}{y} $ is decreasing with respect to $ x $,
$ (4) $ if $ x\neq0 $, then $ 1-\cfrac{x}{y} $ is increasing with respect to $ y $.
Example 3.12. Let us investigate the degree of separation between the interval $ [0.3, 0.6] $ and the interval $ [0.2, 0.8] $, $ [0.4, 0.9] $, $ [0.8, 0.9] $.
$ S_\varphi^*([0.3,0.6],[0.2,0.8]) = 1-\cfrac{0.3}{0.3} = 0, $ |
$ S_\varphi^*([0.3,0.6],[0.4,0.9]) = 1-\cfrac{0.2}{0.3} = \frac{1}{3}, $ |
$ S_\varphi^*([0.3,0.6],[0.8,0.9]) = 1-\cfrac{0}{0.3} = 1. $ |
Observe the example above, and it is easy to know that $ [0.3, 0.6] $ and $ [0.2, 0.8] $ have at least 0 separation measures, which means they do not separate. Since $ [0.3, 0.6]\cap[0.8, 0.9] = \emptyset $, they are completely separated. We need note that $ S_\varphi^*([0.3, 0.6], [0.8, 0.9]) = S_\varphi^*([0.8, 0.9], [0.3, 0.6]) = 1 $. These two examples illustrate Definition 3.1 (1) and (2). $ [0.3, 0.6] $ is separated from $ [0.4, 0.9] $ to a certain extent.
Moreover, note that $ S_\varphi^*([0.8, 0.9], [0.3, 0.6]) = 1 $ implements Definition 3.1 (2) in the other direction. If $ \alpha $ and $ \beta $ have only one element in common, then $ \alpha \cap \beta \neq 0 $, but there is still $ S_\varphi^*(\alpha, \beta) = 1 $. For example, $ S_\varphi^*([0.4, 0.6], [0.6, 0.8]) = 1 $, $ S_\varphi^*([0.6, 0.8], [0.4, 0.6]) = 1 $.
Remark 3.13. About the inference above, it is evident that the function $ \varphi $ provided is independent of variable $ z $ and solely dependent on variables $ x $ and $ y $. But in the other circumstances, the variable $ z $ can have an impact on the outcome. Such as, we can replace $ 1-\dfrac{x}{y} $ with $ 1-\dfrac{xz}{y} $ in the preceding corollary.
In the next proposition, we will give a more general function of the separation of intervals from the construction proposed in Proposition 3.3.
Proposition 3.14. Let the function $ S_\kappa(x) $: $ [I]^2 \rightarrow [0, 1] $ be a separation measure of intervals. Then
(1) if $ \omega(\alpha)\neq 0 $, then $ S_{\kappa}(\alpha, \beta) = \kappa, $
(2) if $ \omega(\alpha) = 0 $, then
$ (i) $ if $ \alpha \in \beta $, then $ S_{\kappa}(\alpha, \beta) = 0 $,
$ (ii) $ if $ \alpha \not\in \beta $, then $ S_{\kappa}(\alpha, \beta) = 1, $
for $ \kappa \in (0, 1] $.
Proof. Its corresponding function $ \varphi $ is as follows:
$ (1) $ if $ x = 0 $, then $ \varphi(x, y, z) = 1 $,
$ (2) $ if $ x\neq 0 $ and $ x = y $, then $ \varphi(x, y, z) = 0 $,
$ (3) $ if $ x\neq 0 $ and $ x\neq y $, then $ \varphi(x, y, z) = \kappa $.
The function $ S_\kappa $ in this instance fails to satisfy Definition 3.1 (1) because $ \kappa $ is not equal to $ 0 $. Yet, we speculate that it could have been $ 1 $. Thus, we can derive an upper bound for a series of interval separation measures.
Proposition 3.15. For all $ S $, there is always $ S_1 \geq S $, i.e., the mapping $ \varphi $ is the maximum separation measure when $ \kappa = 1 $.
Proof. This proof is obvious when $ S_1(\alpha, \beta) = 1 $. Alternatively, $ S_1(\alpha, \beta) = 1 $ if $ \alpha\cap\beta = \emptyset $. Nonetheless, since $ S $ is a separation function, we can infer from Definition 3.1 (1) that $ S(\alpha, \beta) = 1 $. Thus, $ S(\alpha, \beta) $ = $ S_1(\alpha, \beta) $ in this case.
We explore an interval separation measure in the third section. Since the membership and non-membership of each element are intervals in IVIFSs, we study a measure where an IVIFS is separated from another to compare their accuracy based on the section before it.
Definition 4.1. If the function $ \delta $: IVIFS($ X $) $ \times $ IVIFS($ X $) $ \rightarrow [0, 1] $ is named as a separation of IVIFSs, IVI-separation for short, then it satisfies
$ (1) \; 0 \leq \delta(A, B) \leq 1 $,
$ (2) \; \delta(A, B) = 0 \Longleftrightarrow A \sqsubseteq B $,
$ (3) $ if $ A(x)\cap B(x) = \emptyset $ for all $ x\in X $, then $ \delta(A, B) = \delta(B, A) = 1 $,
$ (4) $ if $ \delta(A, B) = 0 $ and $ \delta(B, C) = 0 $, then $ \delta(C, A) \geq \delta(C, B) $ and $ \delta(C, A) \geq \delta(B, A) $.
Proposition 4.2. Let $ A(x) = \langle M_A(x), N_A(x)\rangle $ and $ B(x) = \langle M_B(x), N_B(x)\rangle $ be any two elements of $ A $ and $ B $ on IVIFSs. Then the function $ \delta $: IVIFS($ X $) $ \times $ IVIFS($ X $)$ \rightarrow $ [0, 1] is defined as
$ \delta(A(x),B(x)) = (1-\lambda)S(M_A(x),M_B(x))+ \lambda S(N_B(x),N_A(x)), $ |
for any $ x\in X. $
Proof. It can easily be testified to satisfy the above definition.
Remark 4.3. (1) If $ \lambda < \frac{1}{2} $, then the weight of the membership degree is greater,
(2) if $ \lambda > \frac{1}{2} $, then the weight of the non-membership degree is greater,
(3) if $ \lambda = \frac{1}{2} $, then the weights of both are the same.
Definition 4.4. [42,43] The function $ \mathcal{A} $: $ [0, 1]^{n} \rightarrow [0, 1] $ is called an aggregation function if it satisfies the following conditions:
(1) $ \mathcal{A} $ is non-decreasing in each argument,
(2) $ \mathcal{A}(0, ..., 0) = 0 $ and $ \mathcal{A}(1, ..., 1) = 1 $.
An aggregation function $ \mathcal{A} $ is idempotent if $ \mathcal{A}(x, ..., x) = x $, for any $ x\in [0, 1] $.
Through Definition 4.1 (1), we can see that the separation measure equals 0 only when the first set fully embeds in the second set. So we need the aggregation function $ \mathcal{A} $ to satisfy the condition $ \mathcal{A}(x_1, x_2, ..., x_n) = 0 $ iff $ x_i = 0, i = 1, 2, ..., n. $ For our convenience, let us denote this condition as a zero-boundary condition. For example, in common aggregation functions, the above requirements are met with the geometric mean, arithmetic mean, and any t-conorm that they generate.
Proposition 4.5. Let $ \delta $: IVIFS($ X $) $ \times $ IVIFS($ X $) $ \rightarrow [0, 1] $ be an IVI-separation measure. Then it is given by
$ \delta_\mathcal{A}(A,B) = \mathcal{A} \delta(A(x),B(x)), $ |
where $ \delta $: IVIFS($ X $) $ \times $ IVIFS($ X $) $ \rightarrow [0, 1] $ is an interval-valued intuitionistic fuzzy separation measure and $ \mathcal{A} $: $ [0, 1]^{n}\rightarrow [0, 1] $ satisfies a zero-boundary condition.
Proof. $ (1) $ It is obvious that $ \delta_\mathcal{A} \in[0, 1] $.
$ (2) $ By definition, $ \delta_\mathcal{A}(A, B) = 0 $ is equivalent to $ \mathcal{A} \delta(A(x), B(x)) = 0 $. Since for any $ x\in X $, $ \mathcal{A} $ satisfies a zero-boundary condition, then $ \delta(A(x), B(x)) = 0 $. Therefore, we can get $ A\sqsubseteq B $ because $ A(x)\sqsubseteq B(x) $.
$ (3) $ If $ A(x)\cap B(x) = \emptyset $, then $ \delta(A(x), B(x)) = 1 $ for any $ x \in X $. Hence, for any $ x\in X $, $ \mathcal{A}\delta(A(x), B(x)) = 1 $ by the aggregation function of the boundary condition, i.e., $ \delta_\mathcal{A}(A, B) = 1 $.
$ (4) $ Given $ \delta_\mathcal{A}(A, B) = 0 $ and $ \delta_\mathcal{A}(B, C) = 0 $, then we get $ A\sqsubseteq B\sqsubseteq C $. Thus, we have that $ M_A \subseteq M_B \subseteq M_C $ and $ N_C \subseteq N_B \subseteq N_A $. According to Definition 3.1, we can obtain $ S(M_C(x), M_A(x))\geq S(M_B(x), M_A(x)) $ and $ S(N_A(x), N_C(x)) \geq S(N_A(x), N_B(x)) $, so $ \delta(C(x), A(x))\geq \delta(B(x), A(x)) $ and $ \mathcal{A} \delta(C(x), A(x)) \geq \mathcal{A} \delta(B(x), A(x)) $. Then $ \delta_\mathcal{A}(C, A) \geq \delta_\mathcal{A}(B, A) $ by the monotonicity of the aggregation function.
Remark 4.6. In Section 3, different construction methods of the separation measure for intervals are described, based on which IVI-separation can be constructed.
In Proposition 3.7, $ S_1 $ is the maximum separation measure. So we can have that the upper bound of a series of IVI-separation measures on IVIFS($ X $) is obtained based on $ S_1 $. Consider this specific measure and aggregation function $ \mathcal{A}^{1}(x_1, ..., x_n) = 1 $ where at least one $ x_i\neq 0 $. The following proposition is obtained.
Proposition 4.7. Let $ \delta_{{\mathcal{A}^{1}}}^{S_1}: [I]\times [I] \rightarrow [I] $ be the largest IVI-separation. Then it is given by
$ \begin{equation*} {\delta_{{\mathcal{A}^{1}}}^{S_1}(A,B)} = \begin{cases} 0,& \mathit{\text{ if }}\ A\sqsubseteq B,\\ 1,& \mathit{\text{otherwise}}. \end{cases} \end{equation*} $ |
Proposition 4.8. Let $ \delta $ be any IVI-separation measure, and then $ \delta_{{\mathcal{A}^{1}}}^{S_1}\geq \delta $.
Proof. First, $ \delta_{{\mathcal{A}^{1}}}^{S_1}(A, B) = 0 $ if and only if $ A\sqsubseteq B $, and we also have that $ \delta(A, B) = 0 $ iff $ A\sqsubseteq B $ since $ \delta $ is an IVI-separation. So, $ \delta_{{\mathcal{A}^{1}}}^{S_1} = \delta $. Moreover, if $ A\not\sqsubseteq B $, then it is obvious that $ \delta_{{\mathcal{A}^{1}}}^{S_1} \geq \delta $ as $ \delta_{{\mathcal{A}^{1}}}^{S_1} = 1 $.
Let us consider some common aggregate functions and consider the IVI-separation built from the minimum, maximum, arithmetic mean, geometric mean, and square mean, which will be denoted by $ \delta_{MIN}^S $, $ \delta_{MAX}^S $, $ \delta_{AM}^S $, $ \delta_{GM}^S $, $ \delta_{RMS}^S $, respectively.
Proposition 4.9. $ \delta_{MIN}^S\leq \delta_{GM}^S \leq \delta_{AM}^S\leq\delta_{RMS}^S\leq\delta_{MAX}^S $.
Proof. First, it is straightforward that $ \delta_{MIN}^S $ is the smallest and $ \delta_{MAX}^S $ is the largest. For the remaining, since
$ \sqrt[n]{x_1\cdot x_2\cdot\ldots\cdot x_n^2}\leq\sqrt{\dfrac{x_1^2+x_2^2+\ldots+x_n^2}{n}}\leq\dfrac{x_1+x_2+\ldots+x_n}{n} $, then $ \delta_{GM}^S \leq \delta_{AM}^S\leq\delta_{RMS}^S $.
The intersection, union, and complement of IVIFS($ X $) are explored in this section. They are crucial to define the IVI-separation measure. As the membership value and non-membership value are an interval on $ [I] $, then, in the first part, we import the t-norms and t-conorms of the interval and some related concepts. Subsequently, we attempt to apply them to provide the intersection, union, and complement of interval-valued intuitionistic fuzzy sets. Bedregal et al.[44] presented an expansion of the concept of the t-norm on $ [I] $.
Definition 5.1. [44] Let the mapping $ \mathcal{T} $: $ [I]^2 \rightarrow [I] $ be the interval-valued t-norm, in short IV t-norm, if the following conditions hold:
$ (1) \; \mathcal{T}(\alpha, \beta) = \mathcal{T}(\beta, \alpha), $
$ (2) \; \mathcal{T}(\alpha, \mathcal{T}(\beta, \gamma)) = \mathcal{T}(\mathcal{T}(\alpha, \beta), \gamma), $
$ (3) $ if $ \alpha_1 \leq \alpha_2 $ and $ \beta_1 \leq \beta_2 $, then $ \mathcal{T}(\alpha_1, \beta_1) \leq \mathcal{T}(\alpha_2, \beta_2), $
$ (4) $ if $ \alpha_1 \subseteq \alpha_2 $ and $ \beta_1 \subseteq \beta_2 $, then $ \mathcal{T}(\alpha_1, \beta_1) \subseteq \mathcal{T}(\alpha_2, \beta_2), $
$ (5) \; \mathcal{T}([1, 1], \alpha) = \alpha $,
for any $ \alpha, \beta, \gamma, \alpha_1, \alpha_2, \beta_1, \beta_2 \in [I] $.
Definition 5.2. [44] If the function $ \mathcal{S} $: $ [I]^2 \rightarrow [I] $ satisfies Definition 5.1 (1), (2), (3), (4), and the following condition:
$ (5) \; \mathcal{S}([0, 0], \alpha) = \alpha $, for any $ \alpha \in [I] $,
then it is named the interval-valued t-conorm, in short IV t-conorm.
Consider the $ T $-intersection and $ S $-union proposed by Bouchet et al. on IVFS($ X $). In particular, this concept of an intersection preserves the idea of an intersection of crisp sets, so it belongs to either of the sets. Likewise, their union contains either of them.
Next, we will define the intersection on IVIFS by the IV t-norm and IV t-conorm.
Definition 5.3. Let $ A, B\in $ IVIFS($ X $). Then the $ \mathcal{T}\mathcal{S} $-intersection of the interval-valued intuitionistic fuzzy set is defined as
$ A \cap_{\mathcal{T}\mathcal{S}} B(x) = \{\mathcal{T}(M_A(x),M_B(x)),\mathcal{S}(N_A(x),N_B(x))\}. $ |
Note that this intersection ought to be included within both intersected sets since this proposal should maintain the initial meaning of it for crisp sets.
Proposition 5.4. Let $ A $, $ B $ be any IVIFS($ X $). Then we get $ A \cap_{TS} B \subseteq A $ and $ A \cap_{TS} B \subseteq B $.
Proof. This is straightforward and is therefore omitted.
Bedgregal [45] intruduced an IV representable t-norm as follows:
$ \mathcal{T}(\alpha,\beta) = [t_1(\alpha^-,\beta^-),t_2(\alpha^+,\beta^+)], $ |
where $ t_1, t_2 $: $ [0, 1]\times[0, 1]\rightarrow [0, 1] $ with $ t_1\leq t_2 $. Take into account a few of the common $ t $-norms. Here, let us take $ t_1 $ and $ t_2 $ to be the minimum, which represents the biggest $ t $-norm.
$ \mathcal{T}_M(\alpha,\beta) = [\min(\alpha^-,\beta^-),\min(\alpha^+,\beta^+)]. $ |
Thus, for membership of an INIFS,
$ M_A \cap_{\mathcal{T}_M} M_B(x) = [\min(M_A(x)^-,M_B(x)^-),\min(M_A(x)^+,M_B(x)^+)]. $ |
A representable IV t-conorm is given similarly. It is said that for any two t-conorms $ s_1, s_2 $ in [0, 1] with $ s_1\leq s_2 $, where $ s_1, s_2 $ both choose the maximum, then
$ \mathcal{S}_M(\alpha,\beta) = [s_1(\alpha^-,\beta^-),s_2(\alpha^+,\beta^+)]. $ |
The maximum is the lowest t-conorm.
Analogously, we can obtain that
$ N_A \cup_{\mathcal{S}_M} N_B(x) = [\max(N_A^-,N_B^-),\max(N_A^+,N_B^+)]. $ |
Thus, a representable intersection of the IVIFS is given by
$ \begin{equation*} \begin{aligned} A\cap_{\mathcal{T}\mathcal{S}} B(x)& = \langle M_A \cap_{\mathcal{T}_M} M_B(x),N_A \cup_{\mathcal{S}_M} N_B(x)\rangle\\ & = \langle[\min(M_A^-,M_B^-),\min(M_A^+,M_B^+)],[\max(N_A^-,N_B^-),\max(N_A^+,N_B^+)]\rangle, \end{aligned} \end{equation*} $ |
for all $ x\in X $.
For the rest of this work, $ \cap $ will be used instead of $ \cap_{\mathcal{T}\mathcal{S}} $ for convenience.
Proposition 5.5. Let $ A, B, C\in $ IVIFS($ X $). If $ A\subseteq B $ and $ A\subseteq C $, then $ A\subseteq B\cap C $.
Proof. For any $ x\in X $, if $ A\subseteq B $, then we can deduce that $ M_A(x)\leq_L M_B(x) $ and $ N_A(x)\leq_L N_B(x) $ by the order of the IVIFS. Likewise, we can infer that $ M_A(x)\leq_L M_C(x) $ and $ N_A(x)\leq_L N_C(x) $. Thus, we get
$ M_A(x) = \mathcal{T}(M_A(x),M_A(x))\leq_L \mathcal{T}(M_B(x),M_C(x)) = M_B \cap_{\mathcal{T}_M} M_C(x), $ |
and
$ N_B \cup_{\mathcal{S}_M} N_C(x) = \mathcal{S}(N_B(x),N_C(x)) \leq_L \mathcal{S}(N_A(x),N_A(x)) = N_A(x), $ |
by the proposition of $ \mathcal{T} $ and $ \mathcal{S} $. So we have $ A\subseteq B\cap C $.
Note that the intersection proposed in Definition 5.5 means that it is contained in any set, but not embedded in any set. Once again, it illustrates the essential difference behind inclusion and embedding.
Example 5.6. Given that $ A_1, A_2, A_3 $ are three elements in IVIFS($ X $), for any $ x\in X $, then
$ X $ | $ x $ | $ y $ |
$ A $ | $ \langle[0.20, 0.80], [0.50, 0.60]\rangle $ | $ \langle[0.30, 0.40], [0.70, 0.80]\rangle $ |
$ B $ | $ \langle[0.30, 0.70], [0.40, 0.50]\rangle $ | $ \langle[0.10, 0.50], [0.30, 0.50]\rangle $ |
$ C $ | $ \langle[0.40, 0.50], [0.30, 0.50]\rangle $ | $ \langle[0.20, 0.40], [0.40, 0.60]\rangle $ |
$ A\cup B $ | $ \langle[0.30, 0.80], [0.40, 0.50]\rangle $ | $ \langle[0.30, 0.50], [0.30, 0.50]\rangle $ |
$ B\cup C $ | $ \langle[0.40, 0.70], [0.30, 0.50]\rangle $ | $ \langle[0.20, 0.50], [0.30, 0.50]\rangle $ |
From the table above, it is easily observed that $ A\cap B \not\sqsubseteq A $, $ A\cap B \not\sqsubseteq B $. Together, $ B\cap C \sqsubseteq B $ and $ B\cap C \sqsubseteq C $ also cannot be obtained. Thus, it does not satisfy the general properties of the embedding in the intersections in IVIFS$ (X) $.
To this, consider the real membership value and real non-membership value of fuzzy sets, and we can define the intersection as follows:
$ A\sqcap B(x) = \{\langle M_A(x) \cap M_B(x),N_A(x) \cup N_B(x)\rangle\}, $ |
for any $ x\in X $.
It is necessary that $ A\sqcap B \in $ IVIFS($ X $) when $ M_A(x) \cap M_B(x)\neq 0 $ and $ N_A(x) \cup N_B(x)\neq 0 $. Note that the "greatest" set embedding in $ A $ and $ B $ is $ A\sqcap B $.
In order to explain $ A\sqcap B $ more intuitively, let us give the following figure.
In this case, the intersection satisfies the general property that $ A\sqcap B \sqsubseteq A $ and $ A\sqcap B \sqsubseteq B $.
Proposition 5.7. Let $ A, B, C\in $ IVIFS($ X $). If $ \delta(A, C) = \delta(A, B) = 0 $, then $ \delta(A, B \sqcap C) = 0 $.
Proof. If $ \delta(A, C) = 0 $, then $ A\sqsubset C $. So $ M_A\subseteq M_C $ and $ N_A \supseteq N_C $ can be obtained by Definition 2.3. Similarly, if $ \delta(A, B) = 0 $, then there are $ M_A \subseteq M_B $ and $ N_A \supseteq N_B $. By the propositions of the intersection and union of crisp sets, we have that $ M_A(x) \subseteq M_B(x) \cap M_C(x) $ and $ N_B(x) \cap N_C(x) \subseteq N_A(x) $. So we have $ A \sqsubseteq B \sqcap C $, i.e., $ \delta(A, B\sqcap C) = 0 $.
Proposition 5.8. Let $ \delta $ be an IVI-separation. Then we have that
$ (1) $ if $ A(x) \cap B(x) \neq \emptyset $ for all $ x\in X $, then $ \delta(A\sqcap B, A) = 0 $ and $ \delta(A\sqcap B, B) = 0 $,
$ (2) $ if $ A \sqsubseteq B $, then $ \delta(A, A\cap B) = 0 $ and $ \delta(A\cap B, B) = 0 $,
$ (3) $ if $ A\sqsubseteq B $, then $ \delta(C, A)\geq \delta(C, A\cap B)\geq \delta(C, B) $,
for any $ A, B, C \in $ IVIFS($ X $).
Proof. (1) For all $ x\in X $, if $ A(x) \cap B(x) \neq \emptyset $, by the definition of separation and intersection, we can infer that $ A\sqcap B\sqsubseteq A $ as well as $ A\sqcap B\sqsubseteq B $. Therefore, $ \delta(A\sqcap B, A) = 0 $ and $ \delta(A\sqcap B, B) = 0 $ can be obtained.
(2) If $ A\sqsubseteq B, \forall x \in X $, then $ M_A(x)\subseteq M_B(x) $ and $ N_B(x)\subseteq N_A(x) $. Thus, we can obtain that
$ \min(M_A(x)^-,M_B(x)^-)\leq M_A(x)^- \leq M_A(x)^+ = \min(M_A(x)^+,M_B(x)^+), $ |
$ M_B(x)^+ = \min(M_A(x)^-,M_B(x)^-)\leq \min(M_A(x)^+,M_B(x)^+)\leq M_B(x)^+, $ |
and
$ N_A(x)^-\leq\max(N_B(x)^-,N_A(x)^-)\leq \max(N_B(x)^+,N_A(x)^+) = N_A(x)^+, $ |
$ \max(N_B(x)^-,N_A(x)^-) = N_B(x)^- \leq N_B(x)^+\leq\max(N_B(x)^+,N_A(x)^+). $ |
It is said that $ A\sqsubseteq A\cap B \sqsubseteq B $. So the conclusion is confirmed.
(3) We know from the previous proof that $ A\sqsubseteq A\cap B $ as well as $ A\cap B \sqsubseteq B $. Combined with Definition 4.2, we can directly get the consequence.
Next, let us do a study on union sets. Similarly, we use the t-norm and t-conorm to give the union of two elements in IVIFS($ X $).
Definition 5.9. Let $ A, B\in $ IVIFS($ X $). Then the $ \mathcal{S}\mathcal{T} $-union of the interval-valued intuitionistic fuzzy set is defined as
$ A \cup_{\mathcal{S}\mathcal{T}} B(x) = \{\mathcal{S}(M_A(x),M_B(x)),\mathcal{T}(N_A(x),N_B(x))\}. $ |
Proposition 5.10. Assume $ A, B \in $ IVIFS($ X $). Then $ A \subseteq A\cup B $ and $ B \subseteq A\cup B $.
Proof. The proof is direct.
Proposition 5.11. Let $ A, B, C $ be any three IVIVSs. If $ A\subseteq C $ and $ B\subseteq C $, then $ A\cup B\subseteq C $.
Proof. Since $ A\subseteq C $ and $ B\subseteq C $, then $ M_A(x)\leq_L M_C(x) $, $ N_C(x)\leq_L N_A(x) $ and $ M_B(x)\leq_L M_C(x) $, $ N_C(x)\leq_L N_B(x) $. By Definition 5.1 and Definition 5.2, we can obtain that $ \mathcal{S}(M_A, M_B)\leq \mathcal{S}(M_C, M_C) $ and $ \mathcal{T}(M_A, M_B)\geq \mathcal{T}(M_C, M_C) $. So $ A\cup B\subseteq C $.
Next, we define a representable union of the interval-valued intuitionistic fuzzy set when we make the t-norm equal to the minimum and select the t-conorm for the maximum, i.e.,
$ \begin{equation*} \begin{aligned} A\cup_{\mathcal{S}\mathcal{T}} B(x)& = \langle M_A \cup_{\mathcal{T}_M} M_B(x),N_A \cap_{\mathcal{S}_M} N_B(x)\rangle\\ & = \langle[\max(M_A^-,M_B^-),\max(M_A^+,M_B^+)],[\min(N_A^-,N_B^-),\min(N_A^+,N_B^+)]\rangle, \end{aligned} \end{equation*} $ |
for any $ x\in X $.
For the rest of this work, $ \cup $ will be used instead of $ \cup_{\mathcal{S}\mathcal{T}} $ for convenience.
Note, if we used the data in Example 5.6, we will find that the union in IVIFS($ X $) also does not have the general properties of the union. Thus, for any $ x\in X $,
$ A\sqcup B(x) = \{\langle M_A(x) \cup M_B(x), N_A(x) \cap N_B(x)\rangle\}. $ |
So, if $ A(x) \cap B(x) \neq \emptyset $, then we have that $ A\sqsubseteq A\sqcup B $ as $ M_A(x)\subseteq M_A(x) \cup M_B(x) $ and $ N_A(x) \cap N_B(x) \subseteq M_A(x) $. In a similar way, $ B\sqsubseteq A\sqcup B $.
Proposition 5.12. Let $ \delta $ be an IVI-separation. Then we can obtain that
$ (1) $ if $ A(x) \cap B(x) \neq \emptyset $ for all $ x\in X $, then $ \delta(A, A\sqcup B) = 0 $ and $ \delta(B, A\sqcup B) = 0 $,
$ (2) $ if $ A \sqsubseteq B $, then $ \delta(A, A\cup B) = 0 $ and $ \delta(A\cup B, B) = 0 $,
$ (3) $ if $ A\sqsubseteq B $, then $ \delta(C, A)\geq \delta(C, A\cup B)\geq\delta(C, B) $,
for any $ A, B, C \in $ IVIFS($ X $).
Proof. The proof is similar to Proposition 5.8.
Definition 5.13. [45] Let $ \mathcal{N} $: IVIFS($ X $) $ \rightarrow $ IVIFS($ X $) be named as an interval-valued intuitionistic fuzzy negation, if it satisfies the following conditions:
(1) $ \mathcal{N}(\langle[0, 0], [1, 1]\rangle) = \langle[1, 1], [0, 0]\rangle $ and $ \mathcal{N}(\langle[1, 1], [0, 0]\rangle) = \langle[0, 0], [1, 1]\rangle $,
(2) $ \mathcal{N} $ is decreasing,
(3) $ \mathcal{N}(\mathcal{N}(A)) = A $ for any $ A\in $ IVIFS($ X $) is called a strong interval-valued intuitionistic fuzzy negation.
Using IVIF negation, the complement in IVIFS($ X $) can be defined as follows. Let $ A\in $ IVIFS($ X $), and then
$ A^c(x) = \mathcal{N}(A(x)) = \{\langle N_A(x),M_A(x)\rangle\} $ |
for any $ x\in X $.
Proposition 5.14. Let $ \delta $ be an IVI-separation. For every $ A = \{\langle M_A(x), N_A(x)\rangle | x\in X\}\in $ IVIFS($ X $), we can obtain that
(1) if $ M_A(x) = N_A(x) $ for any $ x\in X $, then $ \delta(A, A^c) = \delta(A^c, A) = 0 $,
(2) if $ M_A(x) \cap N_A(x) = \emptyset $ for any $ x\in X $, then $ \delta(A, A^c) = 1 $.
Proof. (1) From the definition of the complement and $ M_A(x) = N_A(x) $, we get $ A = A^c $. Thus, $ \delta(A, A^c) = 0 $ and $ \delta(A^c, A) = 0 $ by Definition 4.1 (2).
(2) For any $ x\in X $, if $ M_A(x) \cap N_A(x) = \emptyset $, then $ A\cap A^c = \emptyset $. Therefore, we can obtain that $ \delta(A, A^c) = 1 $.
Example 6.1. Assume that an aluminum mining company has four different types of ores, each of which has an interval-valued intuitionistic fuzzy set $ M_i(i = 1, 2, 3, 4) $ in the feature space which includes the pertinent information (see Table 2). The firm has now produced an ore $ N $, the category of which is unknown. To solve the category problem of the unknown ore $ N $, we apply the separation measure method of the interval-valued intuitionistic fuzzy set suggested in this work (given that all attribute weights are equal).
Mineral soil type | Feature 1 | Feature 2 |
$ M_1 $ | $ \langle[0.60, 0.80], [0.10, 0.20]\rangle $ | $ \langle[0.60, 0.70], [0.20, 0.30]\rangle $ |
$ M_2 $ | $ \langle[0.20, 0.60], [0.20, 0.40]\rangle $ | $ \langle[0.20, 0.60], [0.30, 0.50]\rangle $ |
$ M_3 $ | $ \langle[0.20, 0.40], [0.20, 0.50]\rangle $ | $ \langle[0.20, 0.80], [0.30, 0.60]\rangle $ |
$ M_4 $ | $ \langle[0.40, 0.60], [0.30, 0.40]\rangle $ | $ \langle[0.10, 0.80], [0.40, 0.70]\rangle $ |
$ B $ | $ \langle[0.50, 0.80], [0.10, 0.30]\rangle $ | $ \langle[0.50, 0.70], [0.20, 0.40]\rangle $ |
Feature 3 | Feature 4 | |
$ M_1 $ | $ \langle[0.30, 0.70], [0.00, 0.20]\rangle $ | $ \langle[0.30, 0.80], [0.00, 0.20]\rangle $ |
$ M_2 $ | $ \langle[0.10, 0.70], [0.10, 0.40]\rangle $ | $ \langle[0.40, 0.60], [0.20, 0.50]\rangle $ |
$ M_3 $ | $ \langle[0.20, 0.30], [0.30, 0.50]\rangle $ | $ \langle[0.10, 0.50], [0.30, 0.70]\rangle $ |
$ M_4 $ | $ \langle[0.40, 0.60], [0.20, 0.30]\rangle $ | $ \langle[0.30, 0.60], [0.20, 0.30]\rangle $ |
$ B $ | $ \langle[0.30, 0.60], [0.00, 0.20]\rangle $ | $ \langle[0.20, 0.80], [0.10, 0.40]\rangle $ |
According to the formula of the separation degree of interval-valued intuitionistic fuzzy sets proposed in this paper, the following table can be obtained after calculation. Here we take $ \lambda = \frac{1}{2} $.
$ (1) $ The results are obtained by the function $ S_\varphi^* $.
Feature 1 | Feature 2 | Feature 3 | Feature 4 | |
$ \delta(N, M_1) $ | 0.16 | 0.25 | 0.00 | 0.33 |
$ \delta(N, M_2) $ | 0.58 | 0.50 | 0.33 | 0.50 |
$ \delta(N, M_3) $ | 0.83 | 0.33 | 1.00 | 0.62 |
$ \delta(N, M_4) $ | 0.83 | 0.50 | 0.67 | 0.25 |
Here we use the arithmetic mean as the aggregate function to obtain the following results.
$ \delta(N, M_1) $ | $ \delta(N, M_2) $ | $ \delta(N, M_3) $ | $ \delta(N, M_4) $ |
0.18 | 0.48 | 0.69 | 0.56 |
According to the tables above, we have that $ \delta(N, M_1) < \delta(M, M_2) < \delta(N, M_4) < \delta(N, M_3) $.
$ (2) $ The results are obtained by the function $ S_{LK} $.
Feature 1 | Feature 2 | Feature 3 | Feature 4 | |
$ \delta(N, M_1) $ | 0.05 | 0.05 | 0.00 | 0.10 |
$ \delta(N, M_2) $ | 0.15 | 0.10 | 0.10 | 0.15 |
$ \delta(N, M_3) $ | 0.60 | 0.10 | 0.65 | 0.30 |
$ \delta(N, M_4) $ | 0.15 | 0.15 | 0.10 | 0.10 |
Here we use the arithmetic mean as the aggregate function to obtain the following results.
$ \delta(N, M_1) $ | $ \delta(N, M_2) $ | $ \delta(N, M_3) $ | $ \delta(N, M_4) $ |
0.05 | 0.13 | 0.41 | 0.13 |
$ (3) $ The results are obtained by the function $ S_{KD} $.
Feature 1 | Feature 2 | Feature 3 | Feature 4 | |
$ \delta(N, M_1) $ | 0.25 | 0.25 | 0.00 | 0.20 |
$ \delta(N, M_2) $ | 0.40 | 0.45 | 0.20 | 0.45 |
$ \delta(N, M_3) $ | 0.75 | 0.13 | 0.80 | 0.55 |
$ \delta(N, M_4) $ | 0.40 | 0.30 | 0.35 | 0.20 |
Here we use the arithmetic mean as the aggregate function to obtain the following results.
$ \delta(N, M_1) $ | $ \delta(N, M_2) $ | $ \delta(N, M_3) $ | $ \delta(N, M_4) $ |
0.18 | 0.38 | 0.69 | 0.31 |
$ (4) $ The results are obtained by the function $ S_{GD} $.
Feature 1 | Feature 2 | Feature 3 | Feature 4 | |
$ \delta(N, M_1) $ | 0.30 | 0.35 | 0.00 | 0.20 |
$ \delta(N, M_2) $ | 0.60 | 0.60 | 0.20 | 0.65 |
$ \delta(N, M_3) $ | 0.75 | 0.30 | 0.80 | 0.75 |
$ \delta(N, M_4) $ | 0.50 | 0.35 | 0.35 | 0.40 |
Here we use the arithmetic mean as the aggregate function to obtain the following results.
$ \delta(N, M_1) $ | $ \delta(N, M_2) $ | $ \delta(N, M_3) $ | $ \delta(N, M_4) $ |
0.21 | 0.51 | 0.65 | 0.40 |
$ (5) $ The results are obtained by the function $ S_{GG} $.
Feature 1 | Feature 2 | Feature 3 | Feature 4 | |
$ \delta(N, M_1) $ | 0.10 | 0.10 | 0.00 | 0.11 |
$ \delta(N, M_2) $ | 0.32 | 0.21 | 0.13 | 0.34 |
$ \delta(N, M_3) $ | 0.65 | 0.17 | 0.72 | 0.55 |
$ \delta(N, M_4) $ | 0.32 | 0.25 | 0.13 | 0.25 |
Here we use the arithmetic mean as the aggregate function to obtain the following results.
$ \delta(N, M_1) $ | $ \delta(N, M_2) $ | $ \delta(N, M_3) $ | $ \delta(N, M_4) $ |
0.08 | 0.25 | 0.52 | 0.24 |
According to the tables above, we have that $ \delta(N, M_1) < \delta(M, M_4)\leq\delta(N, M_2) < \delta(N, M_3) $. Thus, the unknown ore $ N $ belongs to the known ore $ M_1 $. At the same time, we verify Proposition 3.7 again.
Remark 6.2. There is a special case in the data where two intervals intersect by only one point. In this case, when using the interval width construction method, the separation measure will be 1. However, the result of using the constructor of the entailment function is not necessarily one. As a result, the results will vary. We need to choose a more appropriate construction method based on the data.
Example 6.3. Information system security is abstracted as the perfect solution, and hosts are abstracted as IVIFSs based on their real-world circumstances. The host's risk assessment is determined by computing the mainframe's bias toward the optimal solution. Three mainframes ($ A_1 $, $ A_2 $, and $ A_3 $) make up a company's information system. Identity authentication, access control, and security audit are the three aspects of security to take into consideration. Considering that membership is the level of membership security from the standpoint of security analysis, $ B $ is the best option.
Mainframe | Identity authentication | Access control | Security audit |
$ A_1 $ | $ \langle[0.70, 0.90], [0.20, 0.40]\rangle $ | $ \langle[0.70, 0.90], [0.20, 0.40]\rangle $ | $ \langle[0.85, 0.95], [0.40, 0.60]\rangle $ |
$ A_2 $ | $ \langle[0.80, 0.95], [0.20, 0.30]\rangle $ | $ \langle[0.80, 1.00], [0.00, 0.10]\rangle $ | $ \langle[0.90, 0.95], [0.25, 0.45]\rangle $ |
$ A_3 $ | $ \langle[0.75, 1.00], [0.10, 0.30]\rangle $ | $ \langle[0.60, 0.80], [0.10, 0.40]\rangle $ | $ \langle[0.70, 1.00], [0.15, 0.40]\rangle $ |
In order of importance, the weights of the above attributes are recorded as IVIFS $ \{\langle[0.80, 0.85], [0.05, 0.1]\rangle, \langle [0.90, 0.91], [0.01, 0.02]\rangle, \langle[0.89, 0.92], [0.01, 0.05]\rangle\} $. The weighted matrix of interval-valued intuitionistic fuzzy set is as follows:
Mainframe | Identity authentication | Access control | Security audit |
$ A_1 $ | $ \langle[0.56, 0.77], [0.24, 0.46]\rangle $ | $ \langle[0.63, 0.82], [0.21, 0.41]\rangle $ | $ \langle[0.76, 0.87], [0.41, 0.62]\rangle $ |
$ A_2 $ | $ \langle[0.64, 0.81], [0.24, 0.37]\rangle $ | $ \langle[0.72, 0.91], [0.01, 0.12]\rangle $ | $ \langle[0.80, 1.87], [0.26, 0.48]\rangle $ |
$ A_3 $ | $ \langle[0.60, 0.85], [0.15, 0.37]\rangle $ | $ \langle[0.54, 0.73], [0.12, 0.41]\rangle $ | $ \langle[0.62, 0.92], [0.16, 0.43]\rangle $ |
We abstract the ideal solution into an IVIFS $ B = \{\langle[0.70, 1.00], [0.00, 0.30]\rangle\} $. Let $ \lambda = \frac{1}{2} $, and then the calculation relations are shown in the table below.
Identity authentication | Access control | Security audit | |
$ \delta(B, A_1) $ | 0.75 | 0.58 | 0.82 |
$ \delta(B, A_2) $ | 0.59 | 0.19 | 0.88 |
$ \delta(B, A_3) $ | 0.41 | 0.64 | 0.63 |
Here we use the arithmetic mean as the aggregate function to obtain the following results.
$ \delta(B, A_1) $ | $ \delta(B, A_2) $ | $ \delta(B, A_3) $ |
0.72 | 0.55 | 0.56 |
Through the table above, we can conclude that $ A_2 $ has the highest security, followed by mainframe $ A_3 $, and mainframe $ A_1 $ is the lowest among the three.
In the above two examples, the IVI-separation measure was used to obtain valid conclusions. This measure takes into account the width of the interval, so that there is a close relationship between the input and output uncertainties. The IVI-separation, presented in this article, is an efficient method for comparing the accuracy of two fuzzy sets on an IVIFS. It can be applied to image processing, which can represent image pixel features as interval intuitionistic fuzzy sets, and realize image classification and recognition by comparing the accuracy between the blurred features of different image pixels. In medical diagnosis, the characteristics of the disease and the actual characteristics of the patient are abstracted into the intuitionistic fuzzy set of interval values, and the type of disease of the patient can be judged by calculating the IVI-separation measure. However, since IVI-separation is defined on unit intervals, other intervals on $ R $ cannot be addressed. Thus, IVI-separation is not effective in comparing sets in some cases.
The interval-valued intuitionistic fuzzy set can better describe the data of complex practical problems. When the relevant information of the actual problem is expressed in data, it is not possible to obtain accurate values due to various factors. Therefore, the membership function of the interval value is easier to determine than the membership of the fuzzy set, and it is more realistic. In this paper, we take interval-valued intuitionistic fuzzy sets as a main research object to explore a new measure between them. This paper begins with an introduction to the order relation of IVIFSs, which is embedding. The embedding relationship can be understood as the classic inclusion relationship of the interval. Highlighted and illustrated is the distinction between the notions of embedding and inclusion on IVIFSs. The concept of the separation measure on $ [0, 1] $ as well as the axiomatic definition used to describe it are proposed. Based on the coimplication function and interval width, we propose different construction methods to quantify the separation measure. In the context of IVIFSs, the concept of the separation function is proposed by using the embedding relation, and the theorem satisfying the definition is given for quantifying the separation measure. Additionally, we use the aggregate function to obtain a special separation that can measure a finite set of arbitrary points. Finally, the separation measures of intersection, union, and complement are studied by using the interval t-norm and the interval t-conorm on IVIFSs. In the future, we intend to expand the meaning of $ \delta $ to include IVI-separation, which is measured in terms of intervals instead of the value in [0, 1], and use them to address actual issues. We also plan to study these measures by replacing the number of intervals with fuzzy numbers.
Yaoyao Gong: Conceptualization, writing–original draft, resources, writing–review and editing; Zengtai Gong: Investigation, methodology, supervision.
The authors declare they have not used Artificial Intelligence (AI) tools in the creation of this article.
The authors would like to thank the anonymous referees and the editor. The study is supported by the National Natural Science Foundation of China (Grant No. 12471444).
The authors declare that they have no conflict of interest.
[1] |
Zadeh LA (1965) Fuzzy sets. Inf Control 8: 338-353. https://doi.org/10.1016/S0019-9958(65)90241-X doi: 10.1016/S0019-9958(65)90241-X
![]() |
[2] |
Atanassov KT (1986) Intuitionistic fuzzy sets. Fuzzy Sets Syst 20: 87-96. https://doi.org/10.1016/S0165-0114(86)80034-3 doi: 10.1016/S0165-0114(86)80034-3
![]() |
[3] |
Atanassov KT (1989) Interval-valued intuitionistic fuzzy sets. Fuzzy Sets Syst 31: 343-349. https://doi.org/10.1016/0165-0114(89)90205-4 doi: 10.1016/0165-0114(89)90205-4
![]() |
[4] |
Zadeh LA (1975) The concept of a linguistic variable and its application to approximate reasoning. Inf Sci 8: 199-249. https://doi.org/10.1016/0020-0255(75)90036-5 doi: 10.1016/0020-0255(75)90036-5
![]() |
[5] |
Mandala S, Gazib KH, Salahshourc S, Mondalb SP, Bhattacharyaf P, Sahaf AK (2024) Application of interval valued intuitionistic fuzzy uncertain MCDM methodology for Ph.D supervisor selection problem. Results in Control and Optimization 15: 100411. https://doi.org/10.1016/j.rico.2024.100411 doi: 10.1016/j.rico.2024.100411
![]() |
[6] |
Couso I, Dubois D (2014) Statistical reasoning with set-valued information: ontic vs. epistemic views. Int J Approx Reason 55: 1502-1518. https://doi.org/10.1016/j.ijar.2013.07.002 doi: 10.1016/j.ijar.2013.07.002
![]() |
[7] |
Hong DH (1998) A note on correlation of interval-valued intuitionistic fuzzy sets. Fuzzy Sets Syst 95: 113-117. https://doi.org/10.1016/S0165-0114(96)00311-9 doi: 10.1016/S0165-0114(96)00311-9
![]() |
[8] |
Xu ZS, Chen J (2008) An overview of distance similarity measures of intuitionistic fuzzy sets. Int J Uncertain Fuzz 16: 529-555. https://doi.org/10.1142/S0218488508005406 doi: 10.1142/S0218488508005406
![]() |
[9] |
Mondal TK, Samanta SK (2001) Topology of interval-valued intuitionistic fuzzy sets. Fuzzy Sets Syst 119: 483-494. https://doi.org/10.1016/S0165-0114(98)00436-9 doi: 10.1016/S0165-0114(98)00436-9
![]() |
[10] |
Park JH, Lim KM, Park JS (2008) Distances between interval-valued intuitionistic fuzzy sets. J Phys Conf Ser 96: 1-8. https://doi.org/10.1088/1742-6596/96/1/012089 doi: 10.1088/1742-6596/96/1/012089
![]() |
[11] |
Gao MM, Sun T, Zhu JJ (2016) Interval-valued intuitionistic fuzzy multiple attribute decision-making method based on revised fuzzy entropy and new scoring function. Control Decis 31: 1757-1764. https://doi.org/10.13195/j.kzyjc.2015.1042 doi: 10.13195/j.kzyjc.2015.1042
![]() |
[12] |
Tiwari P, Gupta P (2018) Entropy, distance and similarity measures under interval-valued intuitionistic fuzzy environment. Informatica 42: 617-627. https://doi.org/10.31449/inf.v42i4.1303 doi: 10.31449/inf.v42i4.1303
![]() |
[13] |
Tiwari P, Gupta P (2022) Novel distance, similarity and entropy measures for interval valued intuitionistic fuzzy soft set. J Intell Fuzzy Syst 43: 3067-3086. https://doi.org/10.3233/jifs-212647 doi: 10.3233/jifs-212647
![]() |
[14] |
Rashid T, Faizi S, Zafar S (2018) Distance based entropy measure of interval-valued intuitionistic fuzzy sets and its application in multicriteria decision making. Adv Fuzzy Syst 2018: 3637897. https://doi.org/10.1155/2018/3637897 doi: 10.1155/2018/3637897
![]() |
[15] |
Liu Y, Jiang W (2020) A new distance measure of interval-valued intuitionistic fuzzy sets and its application in decision making. Soft Comput 24: 6987-7003. https://doi.org/10.1007/s00500-019-04332-5 doi: 10.1007/s00500-019-04332-5
![]() |
[16] |
Malik M, Gupta SK (2024) On basic arithmetic operations for interval-valued intuitionistic fuzzy sets using the Hamming distance with their application in decision making. Expert Syst Appl 239: 0957-4174. https://doi.org/10.1016/j.eswa.2023.122429 doi: 10.1016/j.eswa.2023.122429
![]() |
[17] |
Suo CF, Li XC, Li YM (2023) Distance-Based Knowledge Measure and Entropy for Interval-Valued Intuitionistic Fuzzy Sets. Mathematics 11: 2227-7390. https://doi.org/10.3390/math11163468 doi: 10.3390/math11163468
![]() |
[18] |
Li X, Suo CF, Li YM (2021) Width-based distance measures on interval valued intuitionistic fuzzy sets. J Intell Fuzzy Syst 40: 1-13. https://doi.org/10.3233/jifs-200889 doi: 10.3233/jifs-200889
![]() |
[19] |
Zadeh LA (1968) Probability measures of fuzzy events. J Math Anal Appl 23: 421-427. https://doi.org/10.1016/0022-247X(68)90078-4 doi: 10.1016/0022-247X(68)90078-4
![]() |
[20] |
Liu XD, Zheng SH, Xiong FL (2005) Entropy and subsethood for general interval-valued intuitionistic fuzzy sets. Lecture Notes Artif Intell 3613: 42-52. https://doi.org/10.1007/11539506-6 doi: 10.1007/11539506-6
![]() |
[21] | Zhang YJ, Ma PJ, Su XH, Zhang CP (2011) Entropy on interval-valued intuitionistic fuzzy sets and its application in multi-attribute decision making. Proceedings of the 14th International Conference on Information Fusion, 1-7. |
[22] |
Zhao N, Xu Z (2016) Entropy measures for interval-valued intuitionistic fuzzy information from a comparative perspective and their application to decision making. Informatica 27: 203-229. https://doi.org/10.15388/Informatica.2016.82 doi: 10.15388/Informatica.2016.82
![]() |
[23] |
Jin FF, Pei LD, Chen HY, Zhou LG (2014) Interval-valued intuitionistic fuzzy continuous weighted entropy and its application to multi-criteria fuzzy group decision making. Knowl-Based Syst 59: 132-141. https://doi.org/10.1016/j.knosys.2014.01.014 doi: 10.1016/j.knosys.2014.01.014
![]() |
[24] |
Nazari Z, Mosapour B, Zangiabadi E, Ebrahimzadeh A (2022) Entropy of dynamical systems on interval-valued intuitionistic fuzzy set. New Math Nat Comput 19: 1-16. https://doi.org/10.1142/S1793005723500217 doi: 10.1142/S1793005723500217
![]() |
[25] |
Ohlan A (2022) Novel entropy anddistance measuresforinterval-valued intuitionistic fuzzy sets with application in multi-criteria groupdecision-making. Int J Gen Syst 51: 413-440. https://doi.org/10.1080/03081079.2022.2036138 doi: 10.1080/03081079.2022.2036138
![]() |
[26] |
Sinha D, Dougherty ER (1993) Fuzzification of set inclusion: theory and applications. Fuzzy Sets Syst 55: 15-42. https://doi.org/10.1016/0165-0114(93)90299-W doi: 10.1016/0165-0114(93)90299-W
![]() |
[27] |
Zeng WY, Guo P (2008) Normalized distance, similarity measure, inclusion measure and entropy of interval-valued fuzzy sets and their relationship. Inf Sci 178: 1334-1342. https://doi.org/10.1016/j.ins.2007.10.007 doi: 10.1016/j.ins.2007.10.007
![]() |
[28] |
Zhang QS, Jiang SY, Jia B.G, Luo S.H (2010) Some information measures for interval-valued intuitionistic fuzzy sets. Inf Sci 180: 5130-5145. https://doi.org/10.1016/j.ins.2010.08.038 doi: 10.1016/j.ins.2010.08.038
![]() |
[29] |
Zhang QS, Xing HY, Liu FC, Ye J, Tang P (2014) Some new entropy measures for interval-valued intuitionistic fuzzy sets based on distances and their relationships with similarity and inclusion measures. Inf Sci 283: 55-69. https://doi.org/10.1016/j.ins.2014.06.012 doi: 10.1016/j.ins.2014.06.012
![]() |
[30] |
Zhang HY, Yang SY, Yue ZW (2016) On inclusion measures of intuitionistic and interval-valued intuitionistic fuzzy values and their applications to group decision making. Int J Mach Learn Cybern 7: 833-843. https://doi.org/10.1007/s13042-015-0410-1 doi: 10.1007/s13042-015-0410-1
![]() |
[31] |
Wei CP, Wang P, Zhang YZ (2011) Entropy, similarity measure of interval-valued intuitionistic fuzzy sets and their applications. Inf Sci 181: 4273-4286. https://doi.org/10.1016/j.ins.2011.06.001 doi: 10.1016/j.ins.2011.06.001
![]() |
[32] |
Xu ZS, Yager RR (2009) Intuitionistic and Interval-Valued Intutionistic Fuzzy Preference Relations and Their Measures of Similarity for the Evaluation of Agreement within a Group. Fuzzy Optim Decis Ma 8: 123-139. https://doi.org/10.1007/s10700-009-9056-3 doi: 10.1007/s10700-009-9056-3
![]() |
[33] |
Xia MM, Xu ZS (2010) Some new similarity measures for intuitionistic fuzzy values and their application in group decision making. J Syst Sci Syst Eng 19: 430-452. https://doi.org/ 10.1007/s11518-010-5151-9 doi: 10.1007/s11518-010-5151-9
![]() |
[34] |
Singh P (2012) A new method on measure of similarity between interval-valued intuitionistic fuzzy sets for pattern recognition. J Appl Comput Math 1: 1-5. https://doi.org/10.4172/2168-9679.1000101 doi: 10.4172/2168-9679.1000101
![]() |
[35] |
Wu C, Luo P, Li YL, Ren XK (2014) A new similarity measure of interval-valued intuitionistic fuzzy sets considering its hesitancy degree and applications in expert systems. Math Probl Eng 2014: 359214. https://doi.org/10.1155/2014/359214 doi: 10.1155/2014/359214
![]() |
[36] |
Luo MX, Liang JJ (2018) A novel similarity measure for interval-valued intuitionistic fuzzy sets and its applications. Symmetry 10: 441. https://doi.org/10.3390/sym10100441 doi: 10.3390/sym10100441
![]() |
[37] |
Jeevaraj S (2020) Similarity measure on interval valued intuitionistic fuzzy numbers based on non-hesitance score and its application to pattern recognition. Comput Appl Math 39: 212. https://doi.org/10.1007/s40314-020-01250-3 doi: 10.1007/s40314-020-01250-3
![]() |
[38] |
Alolaiyan H, Razaq A, Ashfaq H, Alghazzaw D, Shuaib U, Lu JB (2024) Improving Similarity Measures for Modeling Real-World Issues With Interval-Valued Intuitionistic Fuzzy Sets. IEEE Access 12: 10482-10496. https://doi.org/10.1109/ACCESS.2024.3351205 doi: 10.1109/ACCESS.2024.3351205
![]() |
[39] |
Takác Z, Bustince H, Pintor JM, Marco-Detchart C, Couso I (2019) Width-based interval-valued distances and fuzzy entropies. IEEE Access 7: 14044-14057. https://doi.org/10.1109/ACCESS.2019.2893800 doi: 10.1109/ACCESS.2019.2893800
![]() |
[40] | Fodor J, Roubens M (1994) Fuzzy preference modelling and multicriteria decision support, Springer, Netherlands. https://doi.org/10.1007/978-94-017-1648-2 |
[41] | Baczyński M, Jayaram B (2008) Fuzzy implications, Springer-Verlag, Berlin, Heidelberg. https://doi.org/10.1007/978-3-540-69082-5 |
[42] | Beliakov G, Pradera A, Calvo T (2007) Aggregation functions: a guide for practitioners, Springer, Berlin. https://doi.org/10.1007/978-3-540-73721-6 |
[43] | Mayor G, Trillas E (1986) On the representation of some aggregation functions. Proceedings of IEEE International Symposium on MultipleValued Logic, 111-114. |
[44] |
Bedregal BC, Takahashi A (2006) The best interval representations of t-norms and automorphism. Fuzzy Sets Syst 157: 3220-3230. https://doi.org/10.1016/j.fss.2006.06.013 doi: 10.1016/j.fss.2006.06.013
![]() |
[45] |
Reiser RHS, Bedregal B (2017) Correlation in interval-valued Atanassov's intuitionistic fuzzy sets conjugate and negation operators. Int J Uncertain Fuzziness Knowl Based Syst 25: 787-819. https://doi.org/10.1142/S0218488517500349 doi: 10.1142/S0218488517500349
![]() |
Lukasiewicz | |
$ g_{LK}(x, y)=y-x $ | $ S_{LK}=\left\{\begin{array}{cl}1 & \ \text{if} \ \alpha\cap\beta=\emptyset \\ \max(\beta^–\alpha^-, \alpha^+-\beta^+, 0) & \ \text{otherwise} \end{array}\right. $ |
Kleene-Dienes | |
$ g_{KD}(x, y)=\min(1-x, y) $ | $ S_{KD}=\left\{\begin{array}{cl}0 & \ \text{if}\ \alpha\subseteq\beta \\ 1 & \ \text{if}\ \alpha\cap\beta=\emptyset \\ \min(1-\beta^+, \alpha^+) & \ \text{if}\ \beta^- < \alpha^- \leq\beta^+ < \alpha^+ \\ \min(1-\alpha^-, \beta^-) & \ \text{if}\ \alpha^- < \beta^-\leq\alpha^+ < \beta^+ \\ \max\{\min(1-\alpha^-, \beta^-), \min(1-\beta^+, \alpha^+)\} & \ \text{if}\ \beta\subset\alpha \end{array}\right. $ |
Gödel | |
$ g_{GD}(x, y)=y $ | $ S_{GD}=\left\{\begin{array}{cl}0 & \ \text{if}\ \alpha\subseteq\beta \\ 1 & \ \text{if}\ \alpha\cap\beta=\emptyset \\ \beta^- & \ \text{if}\ \alpha^-\leq\beta^-\leq\alpha^+\leq\beta^+ \\ \alpha^+ & \ \text{otherwise} \end{array}\right. $ |
Goguen | |
$ g_{GG}(x, y)=\cfrac{y-x}{1-x} $ | $ S_{GG}=\left\{\begin{array}{cl}0 & \ \text{if}\ \alpha\subseteq\beta \\ 1 & \ \text{if}\ \alpha\cap\beta=\emptyset \\ \cfrac{\beta^–\alpha^-}{1-\alpha^-} & \ \text{if}\ \alpha\cap\beta\neq\emptyset\wedge\beta^+=1 \\ \max\{\cfrac{\beta^–\alpha^-}{1-\alpha^-}, \cfrac{\alpha^+-\beta^+}{ 1-\beta^+} \} & \ \text{otherwise}\ \end{array}\right. $ |
Mineral soil type | Feature 1 | Feature 2 |
$ M_1 $ | $ \langle[0.60, 0.80], [0.10, 0.20]\rangle $ | $ \langle[0.60, 0.70], [0.20, 0.30]\rangle $ |
$ M_2 $ | $ \langle[0.20, 0.60], [0.20, 0.40]\rangle $ | $ \langle[0.20, 0.60], [0.30, 0.50]\rangle $ |
$ M_3 $ | $ \langle[0.20, 0.40], [0.20, 0.50]\rangle $ | $ \langle[0.20, 0.80], [0.30, 0.60]\rangle $ |
$ M_4 $ | $ \langle[0.40, 0.60], [0.30, 0.40]\rangle $ | $ \langle[0.10, 0.80], [0.40, 0.70]\rangle $ |
$ B $ | $ \langle[0.50, 0.80], [0.10, 0.30]\rangle $ | $ \langle[0.50, 0.70], [0.20, 0.40]\rangle $ |
Feature 3 | Feature 4 | |
$ M_1 $ | $ \langle[0.30, 0.70], [0.00, 0.20]\rangle $ | $ \langle[0.30, 0.80], [0.00, 0.20]\rangle $ |
$ M_2 $ | $ \langle[0.10, 0.70], [0.10, 0.40]\rangle $ | $ \langle[0.40, 0.60], [0.20, 0.50]\rangle $ |
$ M_3 $ | $ \langle[0.20, 0.30], [0.30, 0.50]\rangle $ | $ \langle[0.10, 0.50], [0.30, 0.70]\rangle $ |
$ M_4 $ | $ \langle[0.40, 0.60], [0.20, 0.30]\rangle $ | $ \langle[0.30, 0.60], [0.20, 0.30]\rangle $ |
$ B $ | $ \langle[0.30, 0.60], [0.00, 0.20]\rangle $ | $ \langle[0.20, 0.80], [0.10, 0.40]\rangle $ |
Feature 1 | Feature 2 | Feature 3 | Feature 4 | |
$ \delta(N, M_1) $ | 0.16 | 0.25 | 0.00 | 0.33 |
$ \delta(N, M_2) $ | 0.58 | 0.50 | 0.33 | 0.50 |
$ \delta(N, M_3) $ | 0.83 | 0.33 | 1.00 | 0.62 |
$ \delta(N, M_4) $ | 0.83 | 0.50 | 0.67 | 0.25 |
$ \delta(N, M_1) $ | $ \delta(N, M_2) $ | $ \delta(N, M_3) $ | $ \delta(N, M_4) $ |
0.18 | 0.48 | 0.69 | 0.56 |
Feature 1 | Feature 2 | Feature 3 | Feature 4 | |
$ \delta(N, M_1) $ | 0.05 | 0.05 | 0.00 | 0.10 |
$ \delta(N, M_2) $ | 0.15 | 0.10 | 0.10 | 0.15 |
$ \delta(N, M_3) $ | 0.60 | 0.10 | 0.65 | 0.30 |
$ \delta(N, M_4) $ | 0.15 | 0.15 | 0.10 | 0.10 |
$ \delta(N, M_1) $ | $ \delta(N, M_2) $ | $ \delta(N, M_3) $ | $ \delta(N, M_4) $ |
0.05 | 0.13 | 0.41 | 0.13 |
Feature 1 | Feature 2 | Feature 3 | Feature 4 | |
$ \delta(N, M_1) $ | 0.25 | 0.25 | 0.00 | 0.20 |
$ \delta(N, M_2) $ | 0.40 | 0.45 | 0.20 | 0.45 |
$ \delta(N, M_3) $ | 0.75 | 0.13 | 0.80 | 0.55 |
$ \delta(N, M_4) $ | 0.40 | 0.30 | 0.35 | 0.20 |
$ \delta(N, M_1) $ | $ \delta(N, M_2) $ | $ \delta(N, M_3) $ | $ \delta(N, M_4) $ |
0.18 | 0.38 | 0.69 | 0.31 |
Feature 1 | Feature 2 | Feature 3 | Feature 4 | |
$ \delta(N, M_1) $ | 0.30 | 0.35 | 0.00 | 0.20 |
$ \delta(N, M_2) $ | 0.60 | 0.60 | 0.20 | 0.65 |
$ \delta(N, M_3) $ | 0.75 | 0.30 | 0.80 | 0.75 |
$ \delta(N, M_4) $ | 0.50 | 0.35 | 0.35 | 0.40 |
$ \delta(N, M_1) $ | $ \delta(N, M_2) $ | $ \delta(N, M_3) $ | $ \delta(N, M_4) $ |
0.21 | 0.51 | 0.65 | 0.40 |
Feature 1 | Feature 2 | Feature 3 | Feature 4 | |
$ \delta(N, M_1) $ | 0.10 | 0.10 | 0.00 | 0.11 |
$ \delta(N, M_2) $ | 0.32 | 0.21 | 0.13 | 0.34 |
$ \delta(N, M_3) $ | 0.65 | 0.17 | 0.72 | 0.55 |
$ \delta(N, M_4) $ | 0.32 | 0.25 | 0.13 | 0.25 |
$ \delta(N, M_1) $ | $ \delta(N, M_2) $ | $ \delta(N, M_3) $ | $ \delta(N, M_4) $ |
0.08 | 0.25 | 0.52 | 0.24 |
Mainframe | Identity authentication | Access control | Security audit |
$ A_1 $ | $ \langle[0.70, 0.90], [0.20, 0.40]\rangle $ | $ \langle[0.70, 0.90], [0.20, 0.40]\rangle $ | $ \langle[0.85, 0.95], [0.40, 0.60]\rangle $ |
$ A_2 $ | $ \langle[0.80, 0.95], [0.20, 0.30]\rangle $ | $ \langle[0.80, 1.00], [0.00, 0.10]\rangle $ | $ \langle[0.90, 0.95], [0.25, 0.45]\rangle $ |
$ A_3 $ | $ \langle[0.75, 1.00], [0.10, 0.30]\rangle $ | $ \langle[0.60, 0.80], [0.10, 0.40]\rangle $ | $ \langle[0.70, 1.00], [0.15, 0.40]\rangle $ |
Mainframe | Identity authentication | Access control | Security audit |
$ A_1 $ | $ \langle[0.56, 0.77], [0.24, 0.46]\rangle $ | $ \langle[0.63, 0.82], [0.21, 0.41]\rangle $ | $ \langle[0.76, 0.87], [0.41, 0.62]\rangle $ |
$ A_2 $ | $ \langle[0.64, 0.81], [0.24, 0.37]\rangle $ | $ \langle[0.72, 0.91], [0.01, 0.12]\rangle $ | $ \langle[0.80, 1.87], [0.26, 0.48]\rangle $ |
$ A_3 $ | $ \langle[0.60, 0.85], [0.15, 0.37]\rangle $ | $ \langle[0.54, 0.73], [0.12, 0.41]\rangle $ | $ \langle[0.62, 0.92], [0.16, 0.43]\rangle $ |
Identity authentication | Access control | Security audit | |
$ \delta(B, A_1) $ | 0.75 | 0.58 | 0.82 |
$ \delta(B, A_2) $ | 0.59 | 0.19 | 0.88 |
$ \delta(B, A_3) $ | 0.41 | 0.64 | 0.63 |
$ \delta(B, A_1) $ | $ \delta(B, A_2) $ | $ \delta(B, A_3) $ |
0.72 | 0.55 | 0.56 |
Lukasiewicz | |
$ g_{LK}(x, y)=y-x $ | $ S_{LK}=\left\{\begin{array}{cl}1 & \ \text{if} \ \alpha\cap\beta=\emptyset \\ \max(\beta^–\alpha^-, \alpha^+-\beta^+, 0) & \ \text{otherwise} \end{array}\right. $ |
Kleene-Dienes | |
$ g_{KD}(x, y)=\min(1-x, y) $ | $ S_{KD}=\left\{\begin{array}{cl}0 & \ \text{if}\ \alpha\subseteq\beta \\ 1 & \ \text{if}\ \alpha\cap\beta=\emptyset \\ \min(1-\beta^+, \alpha^+) & \ \text{if}\ \beta^- < \alpha^- \leq\beta^+ < \alpha^+ \\ \min(1-\alpha^-, \beta^-) & \ \text{if}\ \alpha^- < \beta^-\leq\alpha^+ < \beta^+ \\ \max\{\min(1-\alpha^-, \beta^-), \min(1-\beta^+, \alpha^+)\} & \ \text{if}\ \beta\subset\alpha \end{array}\right. $ |
Gödel | |
$ g_{GD}(x, y)=y $ | $ S_{GD}=\left\{\begin{array}{cl}0 & \ \text{if}\ \alpha\subseteq\beta \\ 1 & \ \text{if}\ \alpha\cap\beta=\emptyset \\ \beta^- & \ \text{if}\ \alpha^-\leq\beta^-\leq\alpha^+\leq\beta^+ \\ \alpha^+ & \ \text{otherwise} \end{array}\right. $ |
Goguen | |
$ g_{GG}(x, y)=\cfrac{y-x}{1-x} $ | $ S_{GG}=\left\{\begin{array}{cl}0 & \ \text{if}\ \alpha\subseteq\beta \\ 1 & \ \text{if}\ \alpha\cap\beta=\emptyset \\ \cfrac{\beta^–\alpha^-}{1-\alpha^-} & \ \text{if}\ \alpha\cap\beta\neq\emptyset\wedge\beta^+=1 \\ \max\{\cfrac{\beta^–\alpha^-}{1-\alpha^-}, \cfrac{\alpha^+-\beta^+}{ 1-\beta^+} \} & \ \text{otherwise}\ \end{array}\right. $ |
Mineral soil type | Feature 1 | Feature 2 |
$ M_1 $ | $ \langle[0.60, 0.80], [0.10, 0.20]\rangle $ | $ \langle[0.60, 0.70], [0.20, 0.30]\rangle $ |
$ M_2 $ | $ \langle[0.20, 0.60], [0.20, 0.40]\rangle $ | $ \langle[0.20, 0.60], [0.30, 0.50]\rangle $ |
$ M_3 $ | $ \langle[0.20, 0.40], [0.20, 0.50]\rangle $ | $ \langle[0.20, 0.80], [0.30, 0.60]\rangle $ |
$ M_4 $ | $ \langle[0.40, 0.60], [0.30, 0.40]\rangle $ | $ \langle[0.10, 0.80], [0.40, 0.70]\rangle $ |
$ B $ | $ \langle[0.50, 0.80], [0.10, 0.30]\rangle $ | $ \langle[0.50, 0.70], [0.20, 0.40]\rangle $ |
Feature 3 | Feature 4 | |
$ M_1 $ | $ \langle[0.30, 0.70], [0.00, 0.20]\rangle $ | $ \langle[0.30, 0.80], [0.00, 0.20]\rangle $ |
$ M_2 $ | $ \langle[0.10, 0.70], [0.10, 0.40]\rangle $ | $ \langle[0.40, 0.60], [0.20, 0.50]\rangle $ |
$ M_3 $ | $ \langle[0.20, 0.30], [0.30, 0.50]\rangle $ | $ \langle[0.10, 0.50], [0.30, 0.70]\rangle $ |
$ M_4 $ | $ \langle[0.40, 0.60], [0.20, 0.30]\rangle $ | $ \langle[0.30, 0.60], [0.20, 0.30]\rangle $ |
$ B $ | $ \langle[0.30, 0.60], [0.00, 0.20]\rangle $ | $ \langle[0.20, 0.80], [0.10, 0.40]\rangle $ |
Feature 1 | Feature 2 | Feature 3 | Feature 4 | |
$ \delta(N, M_1) $ | 0.16 | 0.25 | 0.00 | 0.33 |
$ \delta(N, M_2) $ | 0.58 | 0.50 | 0.33 | 0.50 |
$ \delta(N, M_3) $ | 0.83 | 0.33 | 1.00 | 0.62 |
$ \delta(N, M_4) $ | 0.83 | 0.50 | 0.67 | 0.25 |
$ \delta(N, M_1) $ | $ \delta(N, M_2) $ | $ \delta(N, M_3) $ | $ \delta(N, M_4) $ |
0.18 | 0.48 | 0.69 | 0.56 |
Feature 1 | Feature 2 | Feature 3 | Feature 4 | |
$ \delta(N, M_1) $ | 0.05 | 0.05 | 0.00 | 0.10 |
$ \delta(N, M_2) $ | 0.15 | 0.10 | 0.10 | 0.15 |
$ \delta(N, M_3) $ | 0.60 | 0.10 | 0.65 | 0.30 |
$ \delta(N, M_4) $ | 0.15 | 0.15 | 0.10 | 0.10 |
$ \delta(N, M_1) $ | $ \delta(N, M_2) $ | $ \delta(N, M_3) $ | $ \delta(N, M_4) $ |
0.05 | 0.13 | 0.41 | 0.13 |
Feature 1 | Feature 2 | Feature 3 | Feature 4 | |
$ \delta(N, M_1) $ | 0.25 | 0.25 | 0.00 | 0.20 |
$ \delta(N, M_2) $ | 0.40 | 0.45 | 0.20 | 0.45 |
$ \delta(N, M_3) $ | 0.75 | 0.13 | 0.80 | 0.55 |
$ \delta(N, M_4) $ | 0.40 | 0.30 | 0.35 | 0.20 |
$ \delta(N, M_1) $ | $ \delta(N, M_2) $ | $ \delta(N, M_3) $ | $ \delta(N, M_4) $ |
0.18 | 0.38 | 0.69 | 0.31 |
Feature 1 | Feature 2 | Feature 3 | Feature 4 | |
$ \delta(N, M_1) $ | 0.30 | 0.35 | 0.00 | 0.20 |
$ \delta(N, M_2) $ | 0.60 | 0.60 | 0.20 | 0.65 |
$ \delta(N, M_3) $ | 0.75 | 0.30 | 0.80 | 0.75 |
$ \delta(N, M_4) $ | 0.50 | 0.35 | 0.35 | 0.40 |
$ \delta(N, M_1) $ | $ \delta(N, M_2) $ | $ \delta(N, M_3) $ | $ \delta(N, M_4) $ |
0.21 | 0.51 | 0.65 | 0.40 |
Feature 1 | Feature 2 | Feature 3 | Feature 4 | |
$ \delta(N, M_1) $ | 0.10 | 0.10 | 0.00 | 0.11 |
$ \delta(N, M_2) $ | 0.32 | 0.21 | 0.13 | 0.34 |
$ \delta(N, M_3) $ | 0.65 | 0.17 | 0.72 | 0.55 |
$ \delta(N, M_4) $ | 0.32 | 0.25 | 0.13 | 0.25 |
$ \delta(N, M_1) $ | $ \delta(N, M_2) $ | $ \delta(N, M_3) $ | $ \delta(N, M_4) $ |
0.08 | 0.25 | 0.52 | 0.24 |
Mainframe | Identity authentication | Access control | Security audit |
$ A_1 $ | $ \langle[0.70, 0.90], [0.20, 0.40]\rangle $ | $ \langle[0.70, 0.90], [0.20, 0.40]\rangle $ | $ \langle[0.85, 0.95], [0.40, 0.60]\rangle $ |
$ A_2 $ | $ \langle[0.80, 0.95], [0.20, 0.30]\rangle $ | $ \langle[0.80, 1.00], [0.00, 0.10]\rangle $ | $ \langle[0.90, 0.95], [0.25, 0.45]\rangle $ |
$ A_3 $ | $ \langle[0.75, 1.00], [0.10, 0.30]\rangle $ | $ \langle[0.60, 0.80], [0.10, 0.40]\rangle $ | $ \langle[0.70, 1.00], [0.15, 0.40]\rangle $ |
Mainframe | Identity authentication | Access control | Security audit |
$ A_1 $ | $ \langle[0.56, 0.77], [0.24, 0.46]\rangle $ | $ \langle[0.63, 0.82], [0.21, 0.41]\rangle $ | $ \langle[0.76, 0.87], [0.41, 0.62]\rangle $ |
$ A_2 $ | $ \langle[0.64, 0.81], [0.24, 0.37]\rangle $ | $ \langle[0.72, 0.91], [0.01, 0.12]\rangle $ | $ \langle[0.80, 1.87], [0.26, 0.48]\rangle $ |
$ A_3 $ | $ \langle[0.60, 0.85], [0.15, 0.37]\rangle $ | $ \langle[0.54, 0.73], [0.12, 0.41]\rangle $ | $ \langle[0.62, 0.92], [0.16, 0.43]\rangle $ |
Identity authentication | Access control | Security audit | |
$ \delta(B, A_1) $ | 0.75 | 0.58 | 0.82 |
$ \delta(B, A_2) $ | 0.59 | 0.19 | 0.88 |
$ \delta(B, A_3) $ | 0.41 | 0.64 | 0.63 |
$ \delta(B, A_1) $ | $ \delta(B, A_2) $ | $ \delta(B, A_3) $ |
0.72 | 0.55 | 0.56 |