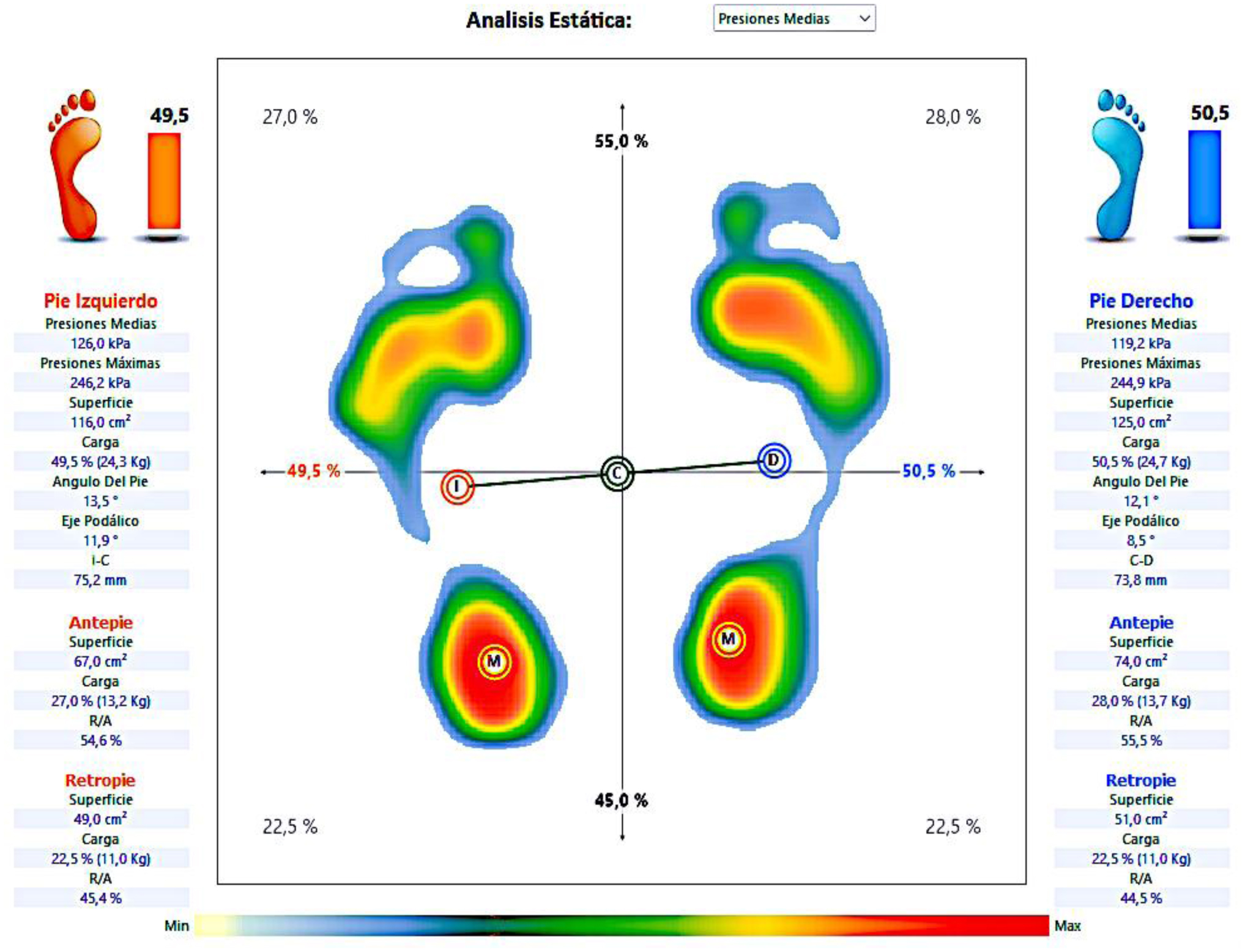
Research lacks an integrated approach that incorporates body composition, postural asymmetries, plantar pressure distribution, and sex comparisons to comprehensively understand the complex relationship between these variables and pain levels.
The study employed an observational cross-sectional design. The study sample comprised 52 participants of both sexes. The average age of participants was 57.35 years for males and 64.69 years for females. Pain levels were assessed using the numeric pain rating scale. Group comparisons (t-test) and machine learning algorithms were employed for analysis.
The results indicated sex differences in height, weight, lean mass percentage, basal metabolism, shoe size, left foot area, podal axis, and distance between foot and body center of pressure (COPs). Significant differences between sexes were also observed in shoulder angles (p = 0.002). Machine learning analysis revealed that neck left deviation and left knee angle were predictive of participants' pain levels.
In conclusion, this study highlights differences in baropodometry and anthropometrics between sexes, with neck deviation and left knee angle identified as predictors of pain levels.
Citation: Svitlana Dikhtyarenko, Samuel Encarnação, Dulce Esteves, Pedro Forte. The role of postural and plantar pressure asymmetries predicting pain in aging adults[J]. AIMS Biophysics, 2025, 12(2): 144-163. doi: 10.3934/biophy.2025009
[1] | Abhishek Mallick, Atanu Mondal, Somnath Bhattacharjee, Arijit Roy . Application of nature inspired optimization algorithms in bioimpedance spectroscopy: simulation and experiment. AIMS Biophysics, 2023, 10(2): 132-172. doi: 10.3934/biophy.2023010 |
[2] | Arijit Roy, Abhishek Mallick, Surajit Das, Abhijit Aich . An experimental method of bioimpedance measurement and analysis for discriminating tissues of fruit or vegetable. AIMS Biophysics, 2020, 7(1): 41-53. doi: 10.3934/biophy.2020004 |
[3] | Dina Falah Noori Al-Sabak, Leila Sadeghi, Gholamreza Dehghan . Predicting the correlation between neurological abnormalities and thyroid dysfunction using artificial neural networks. AIMS Biophysics, 2024, 11(4): 403-426. doi: 10.3934/biophy.2024022 |
[4] | Arijit Roy, Somnath Bhattacharjee, Soumyajit Podder, Advaita Ghosh . Measurement of bioimpedance and application of Cole model to study the effect of moisturizing cream on human skin. AIMS Biophysics, 2020, 7(4): 362-379. doi: 10.3934/biophy.2020025 |
[5] | Svetlana Kashina, Andrea Monserrat del Rayo Cervantes-Guerrero, Francisco Miguel Vargas-Luna, Gonzalo Paez, Jose Marco Balleza-Ordaz . Tissue-specific bioimpedance changes induced by graphene oxide ex vivo: a step toward contrast media development. AIMS Biophysics, 2025, 12(1): 54-68. doi: 10.3934/biophy.2025005 |
[6] | Mahamad Irfanulla Khan, Laxmikanth SM, Tarika Gopal, Praveen Kumar Neela . Artificial intelligence and 3D printing technology in orthodontics: future and scope. AIMS Biophysics, 2022, 9(3): 182-197. doi: 10.3934/biophy.2022016 |
[7] | Khadija Maqbool, Hira Mehboob, Abdul Majeed Siddiqui . Impact of viscosity on creeping viscous fluid flow through a permeable slit: a study for the artificial kidneys. AIMS Biophysics, 2022, 9(4): 308-329. doi: 10.3934/biophy.2022026 |
[8] | James C.L. Chow . Applications of artificial intelligence, mathematical modeling and simulation in medical biophysics. AIMS Biophysics, 2021, 8(1): 121-123. doi: 10.3934/biophy.2021009 |
[9] | Swarnava Biswas, Debajit Sen, Dinesh Bhatia, Pranjal Phukan, Moumita Mukherjee . Chest X-Ray image and pathological data based artificial intelligence enabled dual diagnostic method for multi-stage classification of COVID-19 patients. AIMS Biophysics, 2021, 8(4): 346-371. doi: 10.3934/biophy.2021028 |
[10] | Ulrich Lüttge . Physics and the molecular revolution in plant biology: union needed for managing the future. AIMS Biophysics, 2016, 3(4): 501-521. doi: 10.3934/biophy.2016.4.501 |
Research lacks an integrated approach that incorporates body composition, postural asymmetries, plantar pressure distribution, and sex comparisons to comprehensively understand the complex relationship between these variables and pain levels.
The study employed an observational cross-sectional design. The study sample comprised 52 participants of both sexes. The average age of participants was 57.35 years for males and 64.69 years for females. Pain levels were assessed using the numeric pain rating scale. Group comparisons (t-test) and machine learning algorithms were employed for analysis.
The results indicated sex differences in height, weight, lean mass percentage, basal metabolism, shoe size, left foot area, podal axis, and distance between foot and body center of pressure (COPs). Significant differences between sexes were also observed in shoulder angles (p = 0.002). Machine learning analysis revealed that neck left deviation and left knee angle were predictive of participants' pain levels.
In conclusion, this study highlights differences in baropodometry and anthropometrics between sexes, with neck deviation and left knee angle identified as predictors of pain levels.
Traditionally, postural assessment follows a certain standardization, namely the observation of the body in a static position in the three planes of space [frontal (anterior/posterior), sagittal, and transverse] using the gravitational line as an imaginary axis of symmetry between body segments and the body in relation to space [1]. Connecting reference points on the body allows angular measurements to be taken, especially in relation to spinal curves in the sagittal plane and asymmetries in the frontal plane, allowing quantitative postural assessment [2]. Numerous variables obtained lead to the emergence of various management practices related to classifications of good posture. This management represents a gap in clinical practice, which has encouraged the development of technological resources and the use of instruments designed to measure, evaluate, and quantify postural alterations [3]. Postural assessment by digitizing the individual's image (digital photography) is an easy, low-cost, and non-invasive method. The concepts of photogrammetry have become fundamental premises in the development and validation of procedures for assessing postural deformities [4].
In order to improve the quantification of data obtained from photogrammetry and carry out more accurate assessments, the baropodometer was developed, characterized by having numerous pressure sensors to assess the interaction of the foot with the support surface. [5] showed that there is a correlation between the distribution of forces in the plantar region and angles of postural deviations in the lumbosacral and cervo-thoracic regions. It is therefore possible to state that there is a correlation between plantar pressure distribution and a person's posture, considering not only the characteristics of the somatosensory system but also biometric data and body composition data. Upon that, body composition measures play an important role in postural profiling. Typically, body composition is easy to assess by bioimpedance analysis (BIA) due to its non-invasive assessment of body composition, including body water, muscular and fat mass, visceral fat, and metabolic rate [6]. BIA provides a comprehensive estimation of fat mass, fat-free mass, and body fluids, offering valuable insights into disease prognosis [6]. Moreover, BIA has been shown to yield comparable results to dual-energy X-ray absorptiometry, making it a reliable method for body composition assessment [6]. The evolution of BIA research has highlighted its diverse applications, ranging from the estimation of physiological function to the assessment of body composition, emphasizing its significance in clinical research [6].
According to [7], the distribution of plantar pressure can reveal important information about the structures that make up the feet, as well as identify changes that may alter the performance of the feet in both healthy individuals and people with pathologies. Increased plantar pressure can be a predisposing factor to the appearance of various diseases or serious dysfunctions, increasing discomfort and bodily pain [8]. Abnormal postures may also have the opposite effect of causing pain and increasing the risk of sports injuries. For example, flat feet and high arches can alter the limb line and increase risks [9]. Baropodometry has been widely used in assessing treatment results, whether conservative or surgical, in various conditions [10] such as musculoskeletal pain, dyslexia, fibromyalgia, and multiple sclerosis, as well as in other clinical settings [11] and gait analysis [12]. However, caution is advised in interpreting baropodometry findings in clinical practice and scientific research [13]. The reliability of baropodometry in evaluating plantar load distribution has been demonstrated, making it a valuable instrument in determining plantar pressure, postural control, and plantar pressure distribution in various conditions [14].
The most widely used method for studying postural problems is photogrammetry, which allows various data to be obtained from the photographs taken [15]. A detailed analysis of body posture makes it possible to obtain a vast number of variables such as assessing body alignment, head posture, orthostatic posture, and asymmetries [16],[17]. Most time, dealing with large amounts of data may be a challenge for researchers to summarize and create practical applications [18]. The complexity of the data obtained has contributed to the collaboration of artificial intelligence to verify significant variables, creating machine learning (ML) algorithms. According to [19], ML techniques have become powerful tools for finding patterns in the data obtained, reducing the volume of data.
This paper will be organized into a literature review on body composition, plantar pressure distribution, center of mass, center of gravity, and the relationship with pain level, followed by methods, results, discussion, and conclusion sections.
BIA is based on the principle that body tissues have different resistances (impedances) to the passage of an electric current. By measuring the resistances that the body exerts on the current, BIA estimates the body composition of fat, lean tissue, and water components, thus providing a percentage of body fat [20]. High levels of fat are strongly related to metabolic disorders, cardiovascular diseases, and postural imbalances, further highlighting the importance of the role of body composition analysis (BIA) in postural and nutritional assessment [21].
The baropodometry assessment involved the use of the Kinefis Podia baropodometer, equipped with four mats, an HD Logitech camera, and a Hama tripod, with technical specifications including a frequency of 800 Hz, maximum pressure of 1500 N/cm², 1600 sensor count, XY resolution of 2.5 dpi, Z resolution of 8 bits, and calibration validity [22]. The protocol included static and dynamic measurements, with the first stage capturing images in a static position [23]. This test makes it possible to quantitatively map the pressures on each segment of the plantar surface. For the static test, participants were asked to stand in the center of the platform for 5 s, with their arms on either side of their body in a natural position, looking straight ahead (Figure 1). For the dynamic test, participants were asked to walk barefoot on the platform at their normal pace, following the 3-step protocol, which requires landing on the platform on the third step of the gait. The duration of the assessment ranged from 8 to 15 min, and data were analyzed using Motux Studio software, version 1.9.69.0 [24].
In mechanical terms, a human body is in equilibrium when the sum of internal and external forces and the moments of these forces acting on it result in zero [25]. Thus, the human body is never subject to the condition of perfect equilibrium, oscillating constantly even when in a static position. In order to accurately assess postural control, it is necessary to quantify this body oscillation using variables such as the center of pressure (COP) and the center of mass (COM) [26]. The assessment of static balance using COP data and related variables (I–C: distance between left foot COP and body COP; C–D: distance between right foot COP and body COP) has been carried out for various purposes, from checking the effect of training to injury prevention and recovery [27]. The COP position appears to be an interesting biomechanical quantity in the analysis of movement. This quantity can be directly used to evaluate balance or reactivity in sports performance [27].
Participants were evaluated using the Numeric Pain Rating Scale (NPRS), which was presented orally and with a physical instrument. In a self-assessment action regarding pain, they reported the level of pain or discomfort experienced in their day-to-day activities.
Preventing body pain is an efficient way to avoid expenses with medicine treatment, unload healthcare systems, and maintain quality of life via avoiding chronic pain installation [28]. In this context, machine learning (ML) algorithms can be useful for providing insights into diverse types of data, specifically in health sciences where accurate prediction can anticipate negative outcomes and thus address preventive interventions [29]–[32]. The Numeric Pain Rating Scale (NPRS) is a widely used tool for assessing pain intensity in various clinical settings [33]–[36] (Figure 2). The NPRS has been shown to have excellent psychometric properties, making it a reliable and valid measure of pain intensity [37]. It has also been found to be sensitive to changes in pain intensity over time, demonstrating its responsiveness in capturing fluctuations in pain levels [38]. Additionally, the NPRS is easy to administer and has high compliance rates, making it a practical choice for assessing pain in diverse patient populations [39]. The NPRS has been compared to other pain-rating scales, such as the Visual Analogue Scale (VAS) and Verbal Rating Scale (VRS) and has been found to perform favorably in scaling equivalence and administration [40]. This scale ranges from 0 to 10 and allows for a quick and straightforward interpretation of pain intensity, enabling efficient communication between patients and healthcare providers [41].
The aim of this study was to identify significant variables contributing to the association between postural problems, plantar pressure distribution, and levels of bodily pain in middle-aged and elderly adults, utilizing an innovative ML approach. We hypothesize that plantar pressure characteristics and postural asymmetries differ by gender, are associated with body composition, and can predict pain levels. To our knowledge, this study is unique in incorporating variables that enable the identification of body asymmetries during postural analysis, based on plantar pressure distribution and body composition.
The study employed an observational cross-sectional design. A convenience sample was recruited to analyze differences between men and women in anthropometrics, body composition, plantar pressure distribution, and pain levels. Without intervention, researchers observed and recorded data at a single time point, allowing comparisons between sexes and exploring correlations among the measured variables. This type of design allowed a snapshot view of the differences and associations present within the sample. The sampling method followed convenience sampling, and the research was conducted between April and October 2023.
The population of the present study consisted of 52 (n = 52) participants of both sexes. The majority of study participants were female, comprising 67.3% (n = 35), while 32.7% of the sample were male (n = 17). The mean age of participants was 57.35 (male) and 64.69 years (female).
Due to the complexity of the study, all participants underwent three assessment protocols: physical, postural, and baropodometric. The physical assessment protocol measured height, weight, amount of fat mass, amount of lean mass, amount of body water, basal metabolic rate, and shoe size. Data was collected using the LAICA PS5006 bioimpedance scale and height was measured using a portable stadiometer. To complete the postural assessment protocol, four images were taken of each participant (frontal plane, posterior plane, and two sagittal planes). All images were analyzed using the APECS Pro Plus platform (version 8.4.11). Only static images were analyzed in the baropodometry protocol. Shoe size was recorded as self-reported values.
To participate in this study, the following criteria were defined: participants had to be at least eighteen years old and physically fit. Exclusion criteria included severe orthopedics problems (prosthesis placement, recent orthopedics surgeries), neurological issues (diseases requiring daily analgesic intake), cardiopulmonary diseases (pacemaker, use of oxygen cylinders), and pregnant women. All participants voluntarily took part, signing the informed consent form. The study was carried out in accordance with the Helsinki principles. The project was submitted to the Ethics Committee for Research with Human Subjects at the University of Beira Interior (Covilhã) and was approved under Opinion No. CE-UBI-Pj-2023-030; the study was retrospectively registered in the WHO International Clinical Trials Registry Platform under ID: NCT06647355 on October 15, 2024.
Descriptive statistics included mean, standard deviation, and minimum and maximum values. Exploratory analysis was conducted using the Kolmogorov–Smirnov and Levene tests to assess the normality and homogeneity of distributions, respectively. The statistical significance of the comparison between groups (sex) was assessed by a t-test. Effect sizes were interpreted as follows: Cohen's d < 0.2: small effect sizes; Cohen's d ≈ 0.2–0.5: moderate effect sizes; Cohen's d ≈ 0.5–0.8: medium effect sizes; and Cohen's d > 0.8: large effect sizes (Cohen, 2013). Pearson's correlation tests (rp) were used to check associations between variables (JASP, 2018). A representative correlation heatmap was created with software. All analyses were conducted using JASP v. 0.18.1 (University of Amsterdam, Amsterdam, Netherlands) (JASP, 2018). The significance of the analysis was defined as 5% for every test [42].
To find the adequate features to be inputted within the machine learning models, an exploratory analysis was performed through a correlation analysis due to prior knowledge within the literature about the linear correlation between body postures and pain rates [43]. Independent variables presenting correlations ≥ 0.30 (moderate size) with the patient's pain scores were selected, respecting Cohen's effect size cutoffs [42]. After this feature selection process, the dataset's dimensionality was reduced to prevent noise influence on ML algorithms' predictive performance [43].
A machine learning approach was applied to predict the patient's body pain scores by using Python™, a computational programming language [44]. After exploratory analysis (displayed in Table 1), we identified the most correlated variables with the patient's pain scores. The featuring selection procedure selected the variables most relevant in predicting patients' pain levels, namely BMI (kg/m2), lean mass (%), right foot (RF), C–D [distance between right foot COP and body COP (mm)], A9 [angle of lateral deviation of the left knee (°)], neck deviation for the left side (°), and RPE (rate of perceived effort exertion in a single training session). Figure 3 shows the correlation matrix between all independent variables and target-dependent variables.
Significant correlations were found (Table 1) between pain scores and various factors, such as BMI and RPE, highlighting the impact of body mass and perceived exertion on pain. Lean mass and RF_C–D distance were negatively correlated, reflecting their protective roles in reducing strain and improving alignment. A9 and neck deviation showed positive correlations, linking biomechanical misalignments to increased discomfort. These results emphasize the multifactorial nature of pain, influenced by both mechanical inefficiencies and subjective effort.
Variable | Correlation with pain scores |
Height (cm) | −0.20 |
BMI (kg/m²) | 0.30 |
Fat mass (%) | 0.23 |
Lean mass (%) | −0.30 |
LF_Podal axis (°) | 0.28 |
LF_I–C (mm) | −0.27 |
RF_C-D (mm) | −0.30 |
A9 (°) | 0.34 |
Neck deviation left side (°) | 0.41 |
RPE | 0.46 |
A9: left knee angle; LF: left foot; RF: right foot; I–C: distance between left foot COP and body COP; C–D: Distance between right foot COP and body COP; RPE: rate of perceived effort exertion.
Figure 3 shows a correlation matrix that underscores the multifactorial nature of pain, influenced by biomechanical inefficiencies, postural deviations, and subjective exertion levels. Significant correlations were particularly noted with RPE, neck deviation, and A9 alignment. The analysis highlights key correlations with pain levels, with both positive and negative relationships. RPE showed a strong positive correlation, indicating that individuals experiencing higher exertion tend to report greater pain. Similarly, neck deviation (left side) and A9 (left knee lateral deviation angle) were positively correlated, linking postural imbalances and biomechanical misalignments to increased discomfort. On the other hand, lean mass and RF_C–D exhibited strong negative correlations, suggesting their protective roles in reducing strain and improving alignment. Moderate positive correlations were observed with BMI, indicating that higher body mass may increase mechanical stress and exacerbate pain, and LF_Podal Axis, pointing to the influence of foot alignment on discomfort levels.
In the next step, we tested two configurations for algorithm training, splitting 20% of the data for training and 80% for testing [32]. Since this research was multidimensional in nature, i.e. a lot of data was processed and analyzed, we came to the conclusion that the most correlated characteristics (these are: BMI, lean mass, distance between the COP of the right foot and the COP of the body, angle of lateral deviation of the left knee and deviation of the neck to the left side) were normalized within a range of −1 to 1 to avoid overfitting and possibly a regression calculation in a multidimensional characteristics space [45]. To evaluate model performance, the mean squared error (MSE), root mean square error (RMSE), average, and standard deviation values of the predicted pain scores for each algorithm were also outputted considering the input features of X and Y arrays, thus making the estimation based on real and predicting features [46],[47]. This setup was applied to the seven following algorithms: extreme gradient boosting (EGB) [48], Bayesian regression [49], linear regression [50], ridge regression [51], decision tree regression [52], random forest regression [53], and support vector regression [53]. Performing different machine learning regressive models allows the comparison of their performance and the evaluation of dataset stability alongside different machine learning equations [54]. Finally, a K-fold cross-validation was applied to verify the stability of the prior ML algorithm. Cross-validation considered five folds and the same metrics of MSE and RMSE from the previous analysis [55].
Table 2 presents the mean and standard deviation for anthropometrics, body composition, pain level, baropodometric, and postural asymmetries for the total sample, male, and female participants.
Variables | Total (N = 52) Mean (± Sd) |
Females (N = 35) Mean (± Sd) |
Males (N = 17) Mean (± Sd) |
|
Anthropometrics and body composition | Age (years) | 62.29 ± 18.093 | 64.69 ± 18.706 | 57.35 ± 16.167 |
Height (cm) | 165.31 ± 10.151 | 160.46 ±7.294 | 175.29 ± 7.614 | |
Weight (kg) | 70.46 ± 13.222 | 65.64 ± 10.213 | 80.40 ± 13.406 | |
BMI (kg/m²) | 25.6483 ± 3.69897 | 25.4620 ± 3.87153 | 26.0318 ± 3.39550 | |
Fat mass (%) | 28.248 ± 6.7271 | 28.546 ± 6.3491 | 27.635 ± 7.6154 | |
H2O (%) | 50.88 ± 4.649 | 50.20 ± 4.526 | 52.28 ± 4.718 | |
Lean mass (%) | 29.298 ± 4.1095 | 28.289 ± 3.8629 | 31.376 ± 3.9116 | |
Basal metabolism (kcal) | 1341.21 ± 227.902 | 1235.89 ± 126.222 | 1558.06 ± 239.735 | |
Shoe number | 39.02 ± 2.834 | 37.44 ± 1.523 | 42.26 ± 2.009 | |
Pain level | Pain level | 6.50 ± 2.356 | 6.66 ± 2.086 | 6.18 ± 2.877 |
Baropodometry | LF_Lateral load (%) | 47.502 ± 5.2400 | 48.243 ± 5.9058 | 45.976 ± 3.1192 |
LF_Maximal pressure (kPa) | 234.500 ± 20.1625 | 232.243 ± 22.5655 | 239.147 ± 13.4036 | |
LF_Area (cm²) | 124.15 ± 24.902 | 114.89 ± 18.130 | 143.24 ± 26.520 | |
LF_Podal axis (°) | 8.692 ± 9.2125 | 6.669 ± 5.7419 | 12.859 ± 13.1551 | |
LF_I-C (mm) | 105.64 ± 23.604 | 97.68 ± 21.360 | 122.04 ± 19.547 | |
RF_lateral lead (%) | 52.498 ± 5.2400 | 51.757 ± 5.9058 | 54.024 ± 3.1192 | |
RF_Maximal pressure (kPa) | 238.465 ± 11.2321 | 237.397 ± 12.3577 | 240.665 ± 8.3658 | |
RF_Area (cm²) | 130.92 ± 28.776 | 119.77 ± 21.855 | 153.88 ± 28.149 | |
RF_Podal axis (°) | 10.442 ± 4.7455 | 9.800 ± 4.5166 | 11.765 ± 5.0665 | |
RF_C-D (mm) | 94.473 ± 16.5183 | 90.189 ± 16.3103 | 103.294 ± 13.4757 | |
Postural asymmetries in the anterior view of the frontal plane | A1 (°) | 0.62 ± .796 | 0.57 ± .850 | 0.71 ± .686 |
A2 (°) | 1.90 ± 2.452 | 2.26 ± 2.737 | 1.18 ± 1.551 | |
A3 (°) | 1.54 ± 1.686 | 1.60 ± 1.818 | 1.41 ± 1.417 | |
A4 (°) | 1.42 ± 1.944 | 1.54 ± 2.241 | 1.18 ± 1.131 | |
A5 (°) | 0.81 ± 1.344 | 0.91 ± 1.541 | 0.59 ± .795 | |
A6 (°) | 0.79 ± 1.273 | 0.94 ± 1.474 | 0.47 ± .624 | |
A7 (°) | 0.81 ± 1.155 | 0.91 ± 1.245 | 0.59 ± .939 | |
A8 (°) | 2.88 ± 2.691 | 2.43 ± 2.404 | 3.82 ± 3.067 | |
A9 (°) | 3.58 ± 3.102 | 3.31 ± 3.085 | 4.12 ± 3.160 | |
A10 (°) | 13.94 ± 7.770 | 12.89 ± 7.673 | 16.12 ± 7.737 | |
A11 (°) | 9.60 ± 7.807 | 9.03 ± 8.234 | 10.76 ± 6.933 | |
Postural asymmetries in the anterior view of the frontal plane | P1 (°) | 0.40 ± .569 | 0.46 ± .611 | 0.29 ± .470 |
P2 (°) | 1.50 ± 1.732 | 1.57 ± 1.945 | 1.35 ± 1.222 | |
P3 (°) | 1.77 ± 1.676 | 1.91 ± 1.805 | 1.47 ± 1.375 | |
P4 (°) | 1.04 ± 1.298 | 1.17 ± 1.317 | 0.76 ± 1.251 | |
P5 (°) | 0.96 ± 1.414 | 1.11 ± 1.623 | 0.65 ± .786 | |
P6 (°) | 0.98 ± 1.196 | 0.83 ± 1.150 | 1.29 ± 1.263 | |
P7 (°) | 1.17 ± 1.354 | 1.14 ± 1.004 | 1.24 ± 1.921 | |
P8 (°) | 4.17 ± 5.346 | 4.43 ± 5.853 | 3.65 ± 4.227 | |
Postural asymmetries in the right lateral of the sagittal plane | R1 (°) | 1.92 ± 1.426 | 1.89 ± 1.430 | 2.00 ± 1.458 |
R2 (°) | 33.83 ± 7.641 | 34.03 ± 8.414 | 33.41 ± 5.948 | |
R3 (°) | 14.94 ± 13.557 | 11.09 ± 7.789 | 22.88 ± 18.904 | |
R4 (°) | 8.42 ± 8.252 | 9.03 ± 8.719 | 7.18 ± 7.282 | |
R5 (°) | 4.73 ± 2.650 | 5.09 ± 3.033 | 4.00 ± 1.414 | |
R6 (°) | 4.42 ± 3.133 | 4.03 ± 2.995 | 5.24 ± 3.345 | |
R7 (°) | 32.85 ± 7.734 | 32.40 ± 7.624 | 33.76 ± 8.113 | |
Postural asymmetries in the left lateral of the sagittal plane | L1 (°) | 2.40 ± 1.636 | 2.11 ± 1.530 | 3.00 ± 1.732 |
L2 (°) | 35.96 ± 7.554 | 35.69 ± 7.892 | 36.53 ± 7.001 | |
L3 (°) | 8.04 ± 6.750 | 6.71 ± 6.926 | 10.76 ± 5.618 | |
L4 (°) | 10.40 ± 6.832 | 11.29 ± 7.303 | 8.59 ± 5.501 | |
L5 (°) | 4.71 ± 2.913 | 4.29 ± 3.073 | 5.59 ± 2.399 | |
L6 (°) | 3.73 ± 3.182 | 3.14 ± 2.724 | 4.94 ± 3.766 | |
L7 (°) | 29.50 ± 7.964 | 28.37 ± 8.229 | 31.82 ± 7.055 |
A1: body alignment; A2: head tilt; A3: shoulder alignment; A4: armpit alignment; A5: rib cage tilt; A6: deepest point on trunk; A7: pelvic tilt; A8: right knee angle; A9: left knee angle; A10: right foot rotation; A11: left foot rotation; P1: body alignment; P2: head tilt; P3: shoulder alignment; P4: armpit alignment; P5: deepest point on trunk; P6: pelvic tilt; P7: knees; P8: feet; R1: body alignment; R2: head displacement; R3: shoulder angle; R4: pelvic tilt; R5: knee; R6: tibia; R7: foot angle; L1: body alignment; L2: head displacement; L3: shoulder angle; L4: pelvic tilt; L5: knee; L6: tibia; D7: foot angle; LF: left foot; RF: right foot; I–C: distance between left foot COP and body COP; C–D: distance between right foot COP and body COP.
Comparisons between both sexes for anthropometrics, body composition, pain level, and baropodometric variables revealed significant differences, with males presenting higher scores for height, weight, lean mass percentage, basal metabolism, shoe number, left foot area, podal axis, distance between left foot COP and body COP, right foot area, and distance between right foot COP and body COP (Table 3).
Variables | F | p | Variables | F | P |
Age (years) | 1.913 | 0.173 | LF_Lateral load (%) | 2.190 | 0.145 |
Height (cm) | 46.028 | < 0.001 | LF_Maximal pressure (kPa) | 1.351 | 0.251 |
Weight (kg) | 19.416 | < 0.001 | LF_Area (cm²) | 20.501 | < 0.001 |
BMI (kg/m²) | 0.268 | 0.607 | LF_Podal axis (°) | 5.636 | 0.021 |
Fat mass (%) | 0.206 | 0.652 | LF_I–C (mm) | 15.701 | < 0.001 |
H2O (%) | 2.356 | 0.131 | RF_lateral lead (%) | 2.190 | 0.145 |
Lean mass (%) | 7.253 | 0.010 | RF_Maximal pressure (kPa) | 0.968 | 0.330 |
Basal metabolism (kcal) | 40.638 | < 0.001 | RF_Area (cm²) | 23.020 | < 0.001 |
Shoe number | 92.731 | < 0.001 | RF_Podal axis (°) | 2.000 | 0.164 |
Pain level | 0.471 | 0.495 | RF_C–D (mm) | 8.223 | 0.006 |
LF: left foot; RF: right foot; I–C: distance between left foot COP and body COP; C–D: distance between right foot COP and body COP.
The comparisons between sexes regarding postural asymmetries revealed no significant differences. Table 4 shows group comparisons.
Variable | F | p | Variable | F | p | Variable | F | P |
A1 (°) | 0.322 | 0.573 | P1 (°) | 0.938 | 0.338 | R4 (°) | 0.572 | 0.453 |
A2 (°) | 2.279 | 0.137 | P2 (°) | 0.179 | 0.674 | R5 (°) | 1.956 | 0.168 |
A3 (°) | 0.140 | 0.710 | P3 (°) | 0.799 | 0.376 | R6 (°) | 1.721 | 0.196 |
A4 (°) | 0.402 | 0.529 | P4 (°) | 1.126 | 0.294 | R7 (°) | 0.352 | 0.556 |
A5 (°) | 0.669 | 0.417 | P5 (°) | 1.256 | 0.268 | L1 (°) | 3.519 | 0.067 |
A6 (°) | 1.593 | 0.213 | P6 (°) | 1.759 | 0.191 | L2 (°) | 0.140 | 0.710 |
A7 (°) | 0.910 | 0.345 | P7 (°) | 0.052 | 0.820 | L3 (°) | 4.394 | 0.041 |
A8 (°) | 3.208 | 0.079 | P8 (°) | 0.241 | 0.626 | L4 (°) | 1.812 | 0.184 |
A9 (°) | 0.764 | 0.386 | R1 (°) | 0.072 | 0.789 | L5 (°) | 2.349 | 0.132 |
A10 (°) | 2.019 | 0.162 | R2 (°) | 0.073 | 0.788 | L6 (°) | 3.861 | 0.055 |
A11 (°) | 0.561 | 0.457 | R3 (°) | 10.233 | 0.002 | L7 (°) | 2.200 | 0.144 |
A1: body alignment; A2: head tilt; A3: shoulder alignment; A4: armpit alignment; A5: rib cage tilt; A6: deepest point on trunk; A7: pelvic tilt; A8: right knee angle; A9: left knee angle; A10: right foot rotation; A11: left foot rotation; P1: body alignment; P2: head tilt; P3: shoulder alignment; P4: armpit alignment; P5: deepest point on trunk; P6: pelvic tilt; P7: knees; P8: feet; R1: body alignment; R2: head displacement; R3: shoulder angle; R4: pelvic tilt; R5: knee; R6: tibia; R7: foot angle; L1: body alignment; L2: head displacement; L3: shoulder angle; L4: pelvic tilt; L5: knee; L6: tibia; D7: foot angle.
After applying all algorithms, we found the leading models for each predictive variable. The support vector machine was the better algorithm in predicting a score of 7 points in the NPRS scale pain perception (MSE = 4.0.9, RMSE = 2.02, predicted value = 7 points in NPRS) based on the best features retrieved. Therefore, the best model presented good metrics after passing the 5-fold cross-validation method (MSE = 3–7, RMSE = 2–3, predictions = 5–7 points in NPRS) (displayed in Table 5).
ML model | MSE | RMSE | Pred. (x. ± sd) |
GXBoost | 11.16 | 3.34 | 5 ± 2.43 |
Bayesian regression | 5.09 | 2.25 | 6 ± 1.28 |
Linear regression | 6.22 | 2.49 | 6 ± 1.79 |
Ridge regression | 6.09 | 2.46 | 6 ± 1.75 |
Decision tree regression | 5.45 | 2.34 | 6 ± 1.86 |
Support vector regression | 4.09 | 2.02 | 7 ± 0.98 |
Random forest regression | 7.25 | 2.69 | 6 ± 1.49 |
MSE: mean square error; RMSE: root mean square error;
This work aimed to identify significant variables that contribute to the association between postural problems, plantar pressure distribution, and levels of bodily pain in middle-aged and older adults. It was hypothesized that plantar pressure characteristics differ by sex, related to body composition and pain level. The results discussed next confirmed this hypothesis.
The present study revealed significant differences between males and females in foot-related and body composition variables. The variables that confirmed this were height, weight, left foot area, podal axis, left foot I–C, lean mass percentage, basal metabolism, shoe number, right foot area, and C–D; males presented higher values in all these variables. These results emphasize the unique physiological and biomechanical profiles of each sex [56]. These differences also applied to water percentage, foot dimensions, BMI, lean mass, and basal metabolism. The literature reports that these variables may influence plantar pressure distribution and foot function [57],[58]. Findings also highlighted moderate disparities in water content and foot dimensions between sexes, potentially contributing to variations in foot biomechanics and plantar pressure distribution [59]. Understanding these differences may be a starting point for developing tailored interventions that account for the unique physiological and biomechanical profiles of males and females [56],[57]. Further research is warranted to comprehensively understand the implications of these differences on foot biomechanics and plantar pressure distribution [28],[59].
Despite ongoing research and the development of new interventions, musculoskeletal pain in the spine remains a clinical challenge due to its multifactorial condition and high incidence [60]. Given the biomechanical complexity involved in maintaining body balance and postural alignment, it should be noted that various factors can intervene negatively, requiring compensatory body and motor adjustments. Alterations in the conduction, integration, or processing of sensory information caused by postural changes can lead to less efficient body balance [61].
In the present study, pain level scores were obtained by self-reports as a perceptive variable. It is possible to measure pain by objective measures without relying on perception through behavioral observation scales, such as the Critical-Care Pain Observation Tool (CPOT) and the Behavioral Pain Scale (BPS), which assess pain via observable behaviors like facial expressions and body movements [62]. Physiological markers, including heart rate, blood pressure, and cortisol levels, provide indirect indicators of pain [63],[64]. Advanced technologies like functional magnetic resonance imaging (FMRI), electroencephalography (EEG), and near-infrared spectroscopy (NIRS) quantify neural activity associated with pain [65],[66]. These methods are especially useful for non-verbal patients or those unable to self-report, as they allow for the assessment of pain through objective measures that do not rely on subjective reporting [67]–[69]. However, for the present study, the Numeric Pain Rating Scale (NPRS) was a reliable and valid measure instrument with psychometric properties [37],[64]–[70].
Sex differences were noted across various variables; anthropometrics, body composition, pain levels, and baropodometric variables were examined, revealing significant distinctions. Notably, disparities were observed in anthropometrics such as height, weight, lean mass percentage, basal metabolism, and shoe size [71]. In the present study, the left foot area, pedal axis, and distances between foot and body centers of pressure (COPs) revealed significant differences between sexes. These differences have also been noted and explained by the literature [72].
The machine learning prediction revealed predictive capability in the combination of BMI with postural features, explaining that subjects' pain levels were positively correlated with BMI, left knee angle related to the valgus knee, neck deviation degree, and RPE, and inversely correlated with lean mass and distance of the right foot COP in relation to body COP in a single training session. The same methodology has been used in previous studies. One study described running injury and pain prediction using machine learning [73]; another study revealed that women typically experience more pain due to biological markers [74]. No study was found employing machine learning techniques to explain the associations between postural asymmetries and pain levels. However, it is possible to find several studies reporting the relationships between pain levels and postural asymmetries. One study reported that the higher the level of postural imbalance, the higher the pain levels [70]. [75] revealed that postural correction therapeutics may help to reduce pain levels. Finally, and in agreement with the results of the present study, neck asymmetries are also related to increased pain levels [76]. Bodily pain can increase the relative effort required to train because it directly interferes with the perception of effort, physical performance, and motivation. This relationship is influenced by various factors: physiological, psychological, and neurological. Chronic pain is often associated with a chronic inflammatory state, which can affect muscle performance, reduced range of motion, and increased fatigue. Pain can alter posture and movement patterns, which can lead to less efficient muscle recruitment and a greater risk of injury [61].
Although the results found have scientific value, it is necessary to consider some limitations. This study was carried out with a convenience sample; also, it is an observational study, and there is a need for caution when interpreting the results. Additionally, the sample does not represent a specific population while being mostly made up of the female, which may have influenced the results, given that there are anthropometric differences between the genders. Finally, the sample size and characteristics do not allow more precise techniques such as deep learning. Based on the results of this study, we identify the need for future studies to develop and implement training programs to improve physical fitness and body composition. It is hoped that such training programs can influence the distribution of plantar pressure and postural asymmetries, improving body composition in the long term (increasing muscle mass and strength and reducing body fat mass). The integration of baropodometry with body posture asymmetries, RPE in exercise training sessions, and pain levels has practical implications for the quality of life, making it possible to identify more key variables in explaining the causes of pain. Finally, having a higher number of participants will allow running more advanced statistical procedures (i.e., deep learning).
This study allowed us to conclude that baropodometric variables differ between sexes; also, pain level is explained by body composition and posture. Males presented higher values of left foot area and podal axis, left foot I–C, lean mass percentage, basal metabolism, shoe number, right foot area, right foot C–D, and shoulder angle. Additionally, we found that higher BMI correlates with pain level, reduced lean mass, distanced right foot COP in relation to body COP, left knee angle exposing a valgus knee, neck deviation degree, and higher RPE.
This innovative study could play a crucial role in improving people's quality of life, as it makes it possible to identify significant variables in a postural analysis. Baropodometry can be integrated with other technologies, such as 3D motion analysis and imaging tests, offering a comprehensive view of the body's biomechanics. This multidisciplinary approach allows for more effective treatments and a more accurate understanding of patients' needs. Moreover, the application of models like convolutional neural networks can retrieve rich information about pain-related features in different populations.
The authors declare they have not used artificial intelligence (AI) tools in the creation of this article.
[1] | Hrysomallis C, Goodman C (2001) A review of resistance exercise and posture realignment. J Strength Cond Res 15: 385-390. |
[2] | Bell AC, Richards J, Zakrzewski-Fruer JK, et al. (2022) Sedentary behaviour—A target for the prevention and management of cardiovascular disease. Int J Env Res Pub He 20: 532. https://doi.org/10.3390/ijerph20010532 |
[3] | Bertolazzi A, Quaglia V, Bongelli R (2024) Barriers and facilitators to health technology adoption by older adults with chronic diseases: an integrative systematic review. BMC Public Health 24: 506. https://doi.org/10.1186/s12889-024-18036-5 |
[4] | Santos CI, Cunha AB, Braga VP, et al. (2009) Ocorrência de desvios posturais em escolares do ensino público fundamental de Jaguariúna, São Paulo. Revista Paulista de Pediatria 27: 74-80. https://doi.org/10.1590/S0103-05822009000100012 |
[5] | Wojtkow M, Szkoda-Poliszuk K, Szotek S (2018) Influence of body posture on foot load distribution in young school-age children. Acta Bioeng Biomech 20: 101-107. https://doi.org/10.5277/ABB-01079-2018-01 |
[6] | Kuriyan R (2018) Body composition techniques. Indian J Med Res 148: 648-658. https://doi.org/10.4103/ijmr.ijmr\_1777\_18 |
[7] | Azevedo N, Ribeiro JC, Machado L (2022) Balance and posture in children and adolescents: a cross-sectional study. Sensors 22: 4973. https://doi.org/10.3390/s22134973 |
[8] |
Menz HB, Dufour AB, Riskowski JL, et al. (2013) Foot posture, foot function and low back pain: The Framingham Foot Study. Rheumatology (Oxford, England) 52: 2275-2282. https://doi.org/10.1093/rheumatology/ket298 ![]() |
[9] |
Eriksson O, Jauhiainen A, Maad Sasane S, et al. (2019) Uncertainty quantification, propagation and characterization by Bayesian analysis combined with global sensitivity analysis applied to dynamical intracellular pathway models. Bioinformatics 35: 284-292. https://doi.org/10.1093/bioinformatics/bty607 ![]() |
[10] | Gutiérrez-Vilahú L, Guerra-Balic M (2021) Footprint measurement methods for the assessment and classification of foot types in subjects with Down syndrome: a systematic review. J Orthop Surg Res 16: 537. https://doi.org/10.1186/S13018-021-02667-0/TABLES/2 |
[11] |
Wong WY, Wong MS, Lo KH (2007) Clinical applications of sensors for human posture and movement analysis: a review. Prosthet Orthot Int 31: 62-75. https://doi.org/10.1080/03093640600983949 ![]() |
[12] |
Cramer H, Mehling WE, Saha FJ, et al. (2018) Postural awareness and its relation to pain: Validation of an innovative instrument measuring awareness of body posture in patients with chronic pain. BMC Musculoskel Dis 19: 1-10. https://doi.org/10.1186/S12891-018-2031-9/TABLES/7 ![]() |
[13] |
Alves R, Borel WP, Rossi BP, et al. (2018) Test-retest reliability of baropodometry in young asyntomatic individuals during semi static and dynamic analysis. Fisioterapia em Movimento 31: e003114. https://doi.org/10.1590/1980-5918.031.AO14 ![]() |
[14] |
Baumfeld D, Baumfeld T, da Rocha RL, et al. (2017) Reliability of baropodometry on the evaluation of plantar load distribution: a transversal study. BioMed Res Int 2017: 5925137. https://doi.org/10.1155/2017/5925137 ![]() |
[15] |
Fortin C, Ehrmann Feldman D, et al. (2011) Clinical methods for quantifying body segment posture: a literature review. Disabil Rehabil 33: 367-383. https://doi.org/10.3109/09638288.2010.492066 ![]() |
[16] |
Gouveia JP, Forte P, Ribeiro J, et al. (2021) Study of the association between postural misalignments in school students. Symmetry 13: 1959. https://doi.org/10.3390/sym13101959 ![]() |
[17] |
Singla D, Veqar Z (2017) Association between forward head, rounded shoulders, and increased thoracic kyphosis: a review of the literature. J Chiropr Med 16: 220-229. https://doi.org/10.1016/j.jcm.2017.03.004 ![]() |
[18] |
Luo J, Wang Z, Xu L, et al. (2019) Flexible and durable wood-based triboelectric nanogenerators for self-powered sensing in athletic big data analytics. Nat Commun 10: 5147. https://doi.org/10.1038/s41467-019-13166-6 ![]() |
[19] |
Biamonte J, Wittek P, Pancotti N, et al. (2017) Quantum machine learning. Nature 549: 195-202. https://doi.org/10.1038/nature23474 ![]() |
[20] |
Silva AM, Siqueira GR, Silva GA (2013) Repercussões do uso do calçado de salto alto na postura corporal de adolescentes. Revista Paulista de Pediatria 31: 265-271. https://doi.org/10.1590/S0103-05822013000200020 ![]() |
[21] |
Eickemberg M, Oliveira CC, Roriz AK, et al. (2011) Bioimpedância elétrica e sua aplicação em avaliação nutricional. Rev Nutr 24: 883-893. https://doi.org/10.1590/S1415-52732011000600009 ![]() |
[22] |
Palmieri RM, Ingersoll CD, Stone MB, et al. (2002) Center-of-pressure parameters used in the assessment of postural control. J Sport Rehabil 11: 51-66. https://doi.org/10.1123/JSR.11.1.51 ![]() |
[23] |
Castelo LD, Saad M, Tamaoki MJ, et al. (2022) Correlation between baropodometric parameters and functional evaluation in patients with surgically treated congenital idiopathic clubfoot. J Pediatr Orthop Part B 31: 391-396. https://doi.org/10.1097/BPB.0000000000000937 ![]() |
[24] | Choi S, Ashdown SP 3D body scan analysis of dimensional change in lower body measurements for active body positions (2010)81: 81-93. https://doi.org/10.1177/0040517510377822 |
[25] |
Duarte M, Freitas SM (2010) Revision of posturography based on force plate for balance evaluation. Braz J Phys Ther 14: 183-192. https://doi.org/10.1590/S1413-35552010000300003 ![]() |
[26] | Mochizuki L, Amadio AC (2003) Aspectos biomecânicos da postura ereta: a relação entre o centro de massa e o centro de pressão. Rev Port Cien Desp 3: 77-83. |
[27] |
Bonfim TR, Grossi DB, Paccola CA, et al. (2009) Efeito de informação sensorial adicional na propriocepção e equilíbrio de indivíduos com lesão do LCA. Acta Ortop Bras 17: 291-296. https://doi.org/10.1590/S1413-78522009000500008 ![]() |
[28] |
Mutalimov RK, Kravtsova KV, Bairamkulova AM, et al. (2021) Diseases of the musculoskeletal system and rheumatic diseases: prevention and rehabilitation in modern conditions. J Pharm Res Int 33: 419-424. https://doi.org/10.9734/jpri/2021/v33i46B32956 ![]() |
[29] |
Al Kuwaiti A, Nazer K, Al-Reedy A, et al. (2023) A review of the role of artificial intelligence in healthcare. J Pers Med 13: 951. https://doi.org/10.3390/jpm13060951 ![]() |
[30] | Naveed MA (2023) Transforming healthcare through artificial intelligence and machine learning. Pak J Health Sci 4: 1. https://doi.org/10.54393/pjhs.v4i05.844 |
[31] |
Nichols E, Steinmetz JD, Vollset SE, et al. (2022) Estimation of the global prevalence of dementia in 2019 and forecasted prevalence in 2050: an analysis for the Global Burden of Disease Study 2019. Lancet Public Health 7: e105-e125. https://doi.org/10.1016/S2468-2667(21)00249-8 ![]() |
[32] |
Unpingco J (2016) Python for Probability, Statistics, and Machine Learning.Springer. ![]() |
[33] |
Brunelli C, Zecca E, Martini C, et al. (2010) Comparison of numerical and verbal rating scales to measure pain exacerbations in patients with chronic cancer pain. Health Qual Life Out 8: 1-8. https://doi.org/10.1186/1477-7525-8-42/TABLES/4 ![]() |
[34] |
Li L, Liu X, Herr K (2007) Postoperative pain intensity assessment: a comparison of four scales in Chinese adults. Pain Med 8: 223-234. https://doi.org/10.1111/J.1526-4637.2007.00296.X/2/PME_296_F4.JPEG ![]() |
[35] |
Michener LA, Snyder AR, Leggin BG (2011) Responsiveness of the numeric pain rating scale in patients with shoulder pain and the effect of surgical status. J Sport Rehabil 20: 115-128. https://doi.org/10.1123/JSR.20.1.115 ![]() |
[36] |
Moisset X, Attal N, de Andrade DC (2022) An emoji-based visual analog scale compared with a numeric rating scale for pain assessment. JAMA 328: 1980-1980. https://doi.org/10.1001/JAMA.2022.16940 ![]() |
[37] |
Miró J, Castarlenas E, Huguet A (2009) Evidence for the use of a numerical rating scale to assess the intensity of pediatric pain. Eur J Pain 13: 1089-1095. https://doi.org/10.1016/J.EJPAIN.2009.07.002 ![]() |
[38] |
Pagé MG, Katz J, Stinson J, et al. (2012) Validation of the numerical rating scale for pain intensity and unpleasantness in pediatric acute postoperative pain: sensitivity to change over time. J Pain 13: 359-369. https://doi.org/10.1016/j.jpain.2011.12.010 ![]() |
[39] |
Kim JM, Kim MW, Do HJ (2016) Influence of hyperlipidemia on the treatment of supraspinatus tendinopathy with or without tear. Ann Rehabil Med 40: 463-469. https://doi.org/10.5535/ARM.2016.40.3.463 ![]() |
[40] |
Hjermstad MJ, Fayers PM, Haugen DF, et al. (2011) Studies comparing numerical rating scales, verbal rating scales, and visual analogue scales for assessment of pain intensity in adults: a systematic literature review. J Pain Symptom Manag 41: 1073-1093. https://doi.org/10.1016/j.jpainsymman.2010.08.016 ![]() |
[41] |
Pieh C, Neumeier S, Loew T, et al. (2014) Effectiveness of a multimodal treatment program for somatoform pain disorder. Pain Pract 14: E146-E151. https://doi.org/10.1111/PAPR.12144 ![]() |
[42] |
Cohen J (2013) Statistical Power Analysis for the Behavioral Sciences.Routledge. https://doi.org/10.4324/9780203771587 ![]() |
[43] |
Cai J, Luo J, Wang S, et al. (2018) Feature selection in machine learning: a new perspective. Neurocomputing 300: 70-79. https://doi.org/10.1016/j.neucom.2017.11.077 ![]() |
[44] | Python, março 8, Welcome to Python.org, Python, 2023. Available from: https://www.python.org |
[45] |
Singh D, Singh B (2020) Investigating the impact of data normalization on classification performance. Appl Soft Comput 97: 105524. https://doi.org/10.1016/j.asoc.2019.105524 ![]() |
[46] |
Hicks SA, Strümke I, Thambawita V, et al. (2022) On evaluation metrics for medical applications of artificial intelligence. Sci Rep-UK 12: 5979. https://doi.org/10.1038/s41598-022-09954-8 ![]() |
[47] |
Chai T, Draxler RR (2014) Root mean square error (RMSE) or mean absolute error (MAE)?—Arguments against avoiding RMSE in the literature. Geosci Model Dev 7: 1247-1250. https://doi.org/10.5194/gmd-7-1247-2014 ![]() |
[48] | Xgboost: XGBoost Python Package (Versão 2.1.4) [Python; OS Independent], 2024. Available from: https://pypi.org/project/xgboost/ |
[49] | BayesianRidge, Scikit-Learn, 2007. Available from: https://scikit-learn.org/stable/modules/generated/sklearn.linear_model.BayesianRidge.html |
[50] | LinearRegression, Scikit-Learn, 2007. Available from: https://scikit-learn.org/stable/modules/generated/sklearn.linear_model.LinearRegression.html |
[51] | Scikit-learn, RandomForestRegressor, Scikit-Learn, 2007. Available from: https://scikit-learn.org/stable/modules/generated/sklearn.ensemble.RandomForestRegressor.html |
[52] | DecisionTreeRegressor, Scikit-Learn, 2007. Available from: https://scikit-learn.org/stable/modules/generated/sklearn.tree.DecisionTreeRegressor.html |
[53] | SVR, Scikit-Learn, 2007. Available from: https://scikit-learn.org/stable/modules/generated/sklearn.svm.SVR.html |
[54] |
Chicco D, Warrens MJ, Jurman G (2021) The coefficient of determination R-squared is more informative than SMAPE, MAE, MAPE, MSE and RMSE in regression analysis evaluation. PeerJ Comput Sci 7: e623. https://doi.org/10.7717/peerj-cs.623 ![]() |
[55] |
Bradshaw TJ, Huemann Z, Hu J, et al. (2023) A guide to cross-validation for artificial intelligence in medical imaging. Radiol Artif Intell 5: 220232. https://doi.org/10.1148/ryai.220232 ![]() |
[56] |
Hooker SA, Oswald LB, Reid KJ, et al. (2020) Do physical activity, caloric intake, and sleep vary together day to day? Exploration of intraindividual variability in 3 key health behaviors. J Phys Act Health 17: 45-51. https://doi.org/10.1123/jpah.2019-0207 ![]() |
[57] |
Caselli A, Pham H, Giurini JM, et al. (2002) The forefoot-to-rearfoot plantar pressure ratio is increased in severe diabetic neuropathy and can predict foot ulceration. Diabetes Care 25: 1066-1071. https://doi.org/10.2337/diacare.25.6.1066 ![]() |
[58] |
Henriksson H, Henriksson P, Tynelius P, et al. (2020) Cardiorespiratory fitness, muscular strength, and obesity in adolescence and later chronic disability due to cardiovascular disease: a cohort study of 1 million men. Eur Heart J 41: 1503-1510. https://doi.org/10.1093/eurheartj/ehz774 ![]() |
[59] |
Cheung JT, Zhang M, Leung AK, et al. (2005) Three-dimensional finite element analysis of the foot during standing—A material sensitivity study. J Biomech 38: 1045-1054. https://doi.org/10.1016/j.jbiomech.2004.05.035 ![]() |
[60] |
Koes BW, Van Tulder M, Lin CW, et al. (2010) An updated overview of clinical guidelines for the management of non-specific low back pain in primary care. Eur Spine J 19: 2075-2094. https://doi.org/10.1007/s00586-010-1502-y ![]() |
[61] |
Field S, Treleaven J, Jull G (2008) Standing balance: a comparison between idiopathic and whiplash-induced neck pain. Manual Ther 13: 183-191. https://doi.org/10.1016/j.math.2006.12.005 ![]() |
[62] | Storsveen M (2013) Observational pain scales in critically Ill adults. Crit Care Nurse 33: 68-78. https://doi.org/10.4037/ccn2013804 |
[63] |
Roué JM, Rioualen S, Gendras J, et al. (2018) Multi-modal pain assessment: are near-infrared spectroscopy, skin conductance, salivary cortisol, physiologic parameters, and Neonatal Facial Coding System interrelated during venepuncture in healthy, term neonates?. J Pain Res 11: 2257-2267. https://doi.org/10.2147/JPR.S165810 ![]() |
[64] |
Yücel MA, Aasted CM, Petkov MP, et al. (2015) Specificity of hemodynamic brain responses to painful stimuli: a functional near-infrared spectroscopy study. Sci Rep 5: 9469. https://doi.org/10.1038/srep09469 ![]() |
[65] | Tu Y, Tan A, Bai Y, et al. (2016) Decoding subjective intensity of nociceptive pain from pre-stimulus and post-stimulus brain activities. Front Comput Neurosc 10: 32. https://doi.org/10.3389/fncom.2016.00032 |
[66] |
Bak N, Rostrup E, Larsson HB, et al. (2013) Concurrent functional magnetic resonance imaging and electroencephalography assessment of sensory gating in schizophrenia. Hum Brain Mapp 35: 3578-3587. https://doi.org/10.1002/hbm.22422 ![]() |
[67] |
Ocay DD, Teel EF, Luo OD, et al. (2022) Electroencephalographic characteristics of children and adolescents with chronic musculoskeletal pain. Pain Rep 7: e1054. https://doi.org/10.1097/PR9.0000000000001054 ![]() |
[68] |
Gibson RM, Fernández-Espejo D, Gonzalez-Lara LE, et al. (2014) Multiple tasks and neuroimaging modalities increase the likelihood of detecting covert awareness in patients with disorders of consciousness. Front Hum Neurosci 8: 950. https://doi.org/10.3389/fnhum.2014.00950 ![]() |
[69] |
Vijayakumar V, Case M, Shirinpour S, et al. (2017) Quantifying and characterizing tonic thermal pain across subjects from EEG data using random forest models. IEEE T Bio-Med Eng 64: 2988-2996. https://doi.org/10.1109/TBME.2017.2756870 ![]() |
[70] |
Ruhe A, Fejer R, Walker B (2011) Center of pressure excursion as a measure of balance performance in patients with non-specific low back pain compared to healthy controls: a systematic review of the literature. Eur Spine J 20: 358-368. https://doi.org/10.1007/s00586-010-1543-2 ![]() |
[71] |
Kirchengast S (2010) Gender differences in body composition from childhood to old age: an evolutionary point of view. J Life Sc 2: 1-10. https://doi.org/10.1080/09751270.2010.11885146 ![]() |
[72] |
Capodaglio P, Cimolin V, Tacchini E, et al. (2012) Balance control and balance recovery in obesity. Curr Obes Rep 1: 166-173. https://doi.org/10.1007/s13679-012-0018-7 ![]() |
[73] | Lövdal SS, Den Hartigh RJ, Azzopardi G (2021) Injury prediction in competitive runners with machine learning. Int J Sport Physiol 16: 1522-1531. https://doi.org/10.1123/ijspp.2020-0518 |
[74] |
Fillingim RB, King CD, Ribeiro-Dasilva MC (2009) Sex, gender, and pain: a review of recent clinical and experimental findings. J Pain 10: 447-485. https://doi.org/10.1016/j.jpain.2008.12.001 ![]() |
[75] |
Balthillaya GM, Parsekar SS, Gangavelli R, et al. (2022) Effectiveness of posture-correction interventions for mechanical neck pain and posture among people with forward head posture: protocol for a systematic review. BMJ Open 12: e054691. https://doi.org/10.1136/bmjopen-2021-054691 ![]() |
[76] |
Mahmoud NF, Hassan KA, Abdelmajeed SF, et al. (2019) The relationship between forward head posture and neck pain: a systematic review and meta-analysis. Curr Rev Musculoske 12: 562-577. https://doi.org/10.1007/s12178-019-09594-y ![]() |
Variable | Correlation with pain scores |
Height (cm) | −0.20 |
BMI (kg/m²) | 0.30 |
Fat mass (%) | 0.23 |
Lean mass (%) | −0.30 |
LF_Podal axis (°) | 0.28 |
LF_I–C (mm) | −0.27 |
RF_C-D (mm) | −0.30 |
A9 (°) | 0.34 |
Neck deviation left side (°) | 0.41 |
RPE | 0.46 |
A9: left knee angle; LF: left foot; RF: right foot; I–C: distance between left foot COP and body COP; C–D: Distance between right foot COP and body COP; RPE: rate of perceived effort exertion.
Variables | Total (N = 52) Mean (± Sd) |
Females (N = 35) Mean (± Sd) |
Males (N = 17) Mean (± Sd) |
|
Anthropometrics and body composition | Age (years) | 62.29 ± 18.093 | 64.69 ± 18.706 | 57.35 ± 16.167 |
Height (cm) | 165.31 ± 10.151 | 160.46 ±7.294 | 175.29 ± 7.614 | |
Weight (kg) | 70.46 ± 13.222 | 65.64 ± 10.213 | 80.40 ± 13.406 | |
BMI (kg/m²) | 25.6483 ± 3.69897 | 25.4620 ± 3.87153 | 26.0318 ± 3.39550 | |
Fat mass (%) | 28.248 ± 6.7271 | 28.546 ± 6.3491 | 27.635 ± 7.6154 | |
H2O (%) | 50.88 ± 4.649 | 50.20 ± 4.526 | 52.28 ± 4.718 | |
Lean mass (%) | 29.298 ± 4.1095 | 28.289 ± 3.8629 | 31.376 ± 3.9116 | |
Basal metabolism (kcal) | 1341.21 ± 227.902 | 1235.89 ± 126.222 | 1558.06 ± 239.735 | |
Shoe number | 39.02 ± 2.834 | 37.44 ± 1.523 | 42.26 ± 2.009 | |
Pain level | Pain level | 6.50 ± 2.356 | 6.66 ± 2.086 | 6.18 ± 2.877 |
Baropodometry | LF_Lateral load (%) | 47.502 ± 5.2400 | 48.243 ± 5.9058 | 45.976 ± 3.1192 |
LF_Maximal pressure (kPa) | 234.500 ± 20.1625 | 232.243 ± 22.5655 | 239.147 ± 13.4036 | |
LF_Area (cm²) | 124.15 ± 24.902 | 114.89 ± 18.130 | 143.24 ± 26.520 | |
LF_Podal axis (°) | 8.692 ± 9.2125 | 6.669 ± 5.7419 | 12.859 ± 13.1551 | |
LF_I-C (mm) | 105.64 ± 23.604 | 97.68 ± 21.360 | 122.04 ± 19.547 | |
RF_lateral lead (%) | 52.498 ± 5.2400 | 51.757 ± 5.9058 | 54.024 ± 3.1192 | |
RF_Maximal pressure (kPa) | 238.465 ± 11.2321 | 237.397 ± 12.3577 | 240.665 ± 8.3658 | |
RF_Area (cm²) | 130.92 ± 28.776 | 119.77 ± 21.855 | 153.88 ± 28.149 | |
RF_Podal axis (°) | 10.442 ± 4.7455 | 9.800 ± 4.5166 | 11.765 ± 5.0665 | |
RF_C-D (mm) | 94.473 ± 16.5183 | 90.189 ± 16.3103 | 103.294 ± 13.4757 | |
Postural asymmetries in the anterior view of the frontal plane | A1 (°) | 0.62 ± .796 | 0.57 ± .850 | 0.71 ± .686 |
A2 (°) | 1.90 ± 2.452 | 2.26 ± 2.737 | 1.18 ± 1.551 | |
A3 (°) | 1.54 ± 1.686 | 1.60 ± 1.818 | 1.41 ± 1.417 | |
A4 (°) | 1.42 ± 1.944 | 1.54 ± 2.241 | 1.18 ± 1.131 | |
A5 (°) | 0.81 ± 1.344 | 0.91 ± 1.541 | 0.59 ± .795 | |
A6 (°) | 0.79 ± 1.273 | 0.94 ± 1.474 | 0.47 ± .624 | |
A7 (°) | 0.81 ± 1.155 | 0.91 ± 1.245 | 0.59 ± .939 | |
A8 (°) | 2.88 ± 2.691 | 2.43 ± 2.404 | 3.82 ± 3.067 | |
A9 (°) | 3.58 ± 3.102 | 3.31 ± 3.085 | 4.12 ± 3.160 | |
A10 (°) | 13.94 ± 7.770 | 12.89 ± 7.673 | 16.12 ± 7.737 | |
A11 (°) | 9.60 ± 7.807 | 9.03 ± 8.234 | 10.76 ± 6.933 | |
Postural asymmetries in the anterior view of the frontal plane | P1 (°) | 0.40 ± .569 | 0.46 ± .611 | 0.29 ± .470 |
P2 (°) | 1.50 ± 1.732 | 1.57 ± 1.945 | 1.35 ± 1.222 | |
P3 (°) | 1.77 ± 1.676 | 1.91 ± 1.805 | 1.47 ± 1.375 | |
P4 (°) | 1.04 ± 1.298 | 1.17 ± 1.317 | 0.76 ± 1.251 | |
P5 (°) | 0.96 ± 1.414 | 1.11 ± 1.623 | 0.65 ± .786 | |
P6 (°) | 0.98 ± 1.196 | 0.83 ± 1.150 | 1.29 ± 1.263 | |
P7 (°) | 1.17 ± 1.354 | 1.14 ± 1.004 | 1.24 ± 1.921 | |
P8 (°) | 4.17 ± 5.346 | 4.43 ± 5.853 | 3.65 ± 4.227 | |
Postural asymmetries in the right lateral of the sagittal plane | R1 (°) | 1.92 ± 1.426 | 1.89 ± 1.430 | 2.00 ± 1.458 |
R2 (°) | 33.83 ± 7.641 | 34.03 ± 8.414 | 33.41 ± 5.948 | |
R3 (°) | 14.94 ± 13.557 | 11.09 ± 7.789 | 22.88 ± 18.904 | |
R4 (°) | 8.42 ± 8.252 | 9.03 ± 8.719 | 7.18 ± 7.282 | |
R5 (°) | 4.73 ± 2.650 | 5.09 ± 3.033 | 4.00 ± 1.414 | |
R6 (°) | 4.42 ± 3.133 | 4.03 ± 2.995 | 5.24 ± 3.345 | |
R7 (°) | 32.85 ± 7.734 | 32.40 ± 7.624 | 33.76 ± 8.113 | |
Postural asymmetries in the left lateral of the sagittal plane | L1 (°) | 2.40 ± 1.636 | 2.11 ± 1.530 | 3.00 ± 1.732 |
L2 (°) | 35.96 ± 7.554 | 35.69 ± 7.892 | 36.53 ± 7.001 | |
L3 (°) | 8.04 ± 6.750 | 6.71 ± 6.926 | 10.76 ± 5.618 | |
L4 (°) | 10.40 ± 6.832 | 11.29 ± 7.303 | 8.59 ± 5.501 | |
L5 (°) | 4.71 ± 2.913 | 4.29 ± 3.073 | 5.59 ± 2.399 | |
L6 (°) | 3.73 ± 3.182 | 3.14 ± 2.724 | 4.94 ± 3.766 | |
L7 (°) | 29.50 ± 7.964 | 28.37 ± 8.229 | 31.82 ± 7.055 |
A1: body alignment; A2: head tilt; A3: shoulder alignment; A4: armpit alignment; A5: rib cage tilt; A6: deepest point on trunk; A7: pelvic tilt; A8: right knee angle; A9: left knee angle; A10: right foot rotation; A11: left foot rotation; P1: body alignment; P2: head tilt; P3: shoulder alignment; P4: armpit alignment; P5: deepest point on trunk; P6: pelvic tilt; P7: knees; P8: feet; R1: body alignment; R2: head displacement; R3: shoulder angle; R4: pelvic tilt; R5: knee; R6: tibia; R7: foot angle; L1: body alignment; L2: head displacement; L3: shoulder angle; L4: pelvic tilt; L5: knee; L6: tibia; D7: foot angle; LF: left foot; RF: right foot; I–C: distance between left foot COP and body COP; C–D: distance between right foot COP and body COP.
Variables | F | p | Variables | F | P |
Age (years) | 1.913 | 0.173 | LF_Lateral load (%) | 2.190 | 0.145 |
Height (cm) | 46.028 | < 0.001 | LF_Maximal pressure (kPa) | 1.351 | 0.251 |
Weight (kg) | 19.416 | < 0.001 | LF_Area (cm²) | 20.501 | < 0.001 |
BMI (kg/m²) | 0.268 | 0.607 | LF_Podal axis (°) | 5.636 | 0.021 |
Fat mass (%) | 0.206 | 0.652 | LF_I–C (mm) | 15.701 | < 0.001 |
H2O (%) | 2.356 | 0.131 | RF_lateral lead (%) | 2.190 | 0.145 |
Lean mass (%) | 7.253 | 0.010 | RF_Maximal pressure (kPa) | 0.968 | 0.330 |
Basal metabolism (kcal) | 40.638 | < 0.001 | RF_Area (cm²) | 23.020 | < 0.001 |
Shoe number | 92.731 | < 0.001 | RF_Podal axis (°) | 2.000 | 0.164 |
Pain level | 0.471 | 0.495 | RF_C–D (mm) | 8.223 | 0.006 |
LF: left foot; RF: right foot; I–C: distance between left foot COP and body COP; C–D: distance between right foot COP and body COP.
Variable | F | p | Variable | F | p | Variable | F | P |
A1 (°) | 0.322 | 0.573 | P1 (°) | 0.938 | 0.338 | R4 (°) | 0.572 | 0.453 |
A2 (°) | 2.279 | 0.137 | P2 (°) | 0.179 | 0.674 | R5 (°) | 1.956 | 0.168 |
A3 (°) | 0.140 | 0.710 | P3 (°) | 0.799 | 0.376 | R6 (°) | 1.721 | 0.196 |
A4 (°) | 0.402 | 0.529 | P4 (°) | 1.126 | 0.294 | R7 (°) | 0.352 | 0.556 |
A5 (°) | 0.669 | 0.417 | P5 (°) | 1.256 | 0.268 | L1 (°) | 3.519 | 0.067 |
A6 (°) | 1.593 | 0.213 | P6 (°) | 1.759 | 0.191 | L2 (°) | 0.140 | 0.710 |
A7 (°) | 0.910 | 0.345 | P7 (°) | 0.052 | 0.820 | L3 (°) | 4.394 | 0.041 |
A8 (°) | 3.208 | 0.079 | P8 (°) | 0.241 | 0.626 | L4 (°) | 1.812 | 0.184 |
A9 (°) | 0.764 | 0.386 | R1 (°) | 0.072 | 0.789 | L5 (°) | 2.349 | 0.132 |
A10 (°) | 2.019 | 0.162 | R2 (°) | 0.073 | 0.788 | L6 (°) | 3.861 | 0.055 |
A11 (°) | 0.561 | 0.457 | R3 (°) | 10.233 | 0.002 | L7 (°) | 2.200 | 0.144 |
A1: body alignment; A2: head tilt; A3: shoulder alignment; A4: armpit alignment; A5: rib cage tilt; A6: deepest point on trunk; A7: pelvic tilt; A8: right knee angle; A9: left knee angle; A10: right foot rotation; A11: left foot rotation; P1: body alignment; P2: head tilt; P3: shoulder alignment; P4: armpit alignment; P5: deepest point on trunk; P6: pelvic tilt; P7: knees; P8: feet; R1: body alignment; R2: head displacement; R3: shoulder angle; R4: pelvic tilt; R5: knee; R6: tibia; R7: foot angle; L1: body alignment; L2: head displacement; L3: shoulder angle; L4: pelvic tilt; L5: knee; L6: tibia; D7: foot angle.
ML model | MSE | RMSE | Pred. (x. ± sd) |
GXBoost | 11.16 | 3.34 | 5 ± 2.43 |
Bayesian regression | 5.09 | 2.25 | 6 ± 1.28 |
Linear regression | 6.22 | 2.49 | 6 ± 1.79 |
Ridge regression | 6.09 | 2.46 | 6 ± 1.75 |
Decision tree regression | 5.45 | 2.34 | 6 ± 1.86 |
Support vector regression | 4.09 | 2.02 | 7 ± 0.98 |
Random forest regression | 7.25 | 2.69 | 6 ± 1.49 |
MSE: mean square error; RMSE: root mean square error;
Variable | Correlation with pain scores |
Height (cm) | −0.20 |
BMI (kg/m²) | 0.30 |
Fat mass (%) | 0.23 |
Lean mass (%) | −0.30 |
LF_Podal axis (°) | 0.28 |
LF_I–C (mm) | −0.27 |
RF_C-D (mm) | −0.30 |
A9 (°) | 0.34 |
Neck deviation left side (°) | 0.41 |
RPE | 0.46 |
Variables | Total (N = 52) Mean (± Sd) |
Females (N = 35) Mean (± Sd) |
Males (N = 17) Mean (± Sd) |
|
Anthropometrics and body composition | Age (years) | 62.29 ± 18.093 | 64.69 ± 18.706 | 57.35 ± 16.167 |
Height (cm) | 165.31 ± 10.151 | 160.46 ±7.294 | 175.29 ± 7.614 | |
Weight (kg) | 70.46 ± 13.222 | 65.64 ± 10.213 | 80.40 ± 13.406 | |
BMI (kg/m²) | 25.6483 ± 3.69897 | 25.4620 ± 3.87153 | 26.0318 ± 3.39550 | |
Fat mass (%) | 28.248 ± 6.7271 | 28.546 ± 6.3491 | 27.635 ± 7.6154 | |
H2O (%) | 50.88 ± 4.649 | 50.20 ± 4.526 | 52.28 ± 4.718 | |
Lean mass (%) | 29.298 ± 4.1095 | 28.289 ± 3.8629 | 31.376 ± 3.9116 | |
Basal metabolism (kcal) | 1341.21 ± 227.902 | 1235.89 ± 126.222 | 1558.06 ± 239.735 | |
Shoe number | 39.02 ± 2.834 | 37.44 ± 1.523 | 42.26 ± 2.009 | |
Pain level | Pain level | 6.50 ± 2.356 | 6.66 ± 2.086 | 6.18 ± 2.877 |
Baropodometry | LF_Lateral load (%) | 47.502 ± 5.2400 | 48.243 ± 5.9058 | 45.976 ± 3.1192 |
LF_Maximal pressure (kPa) | 234.500 ± 20.1625 | 232.243 ± 22.5655 | 239.147 ± 13.4036 | |
LF_Area (cm²) | 124.15 ± 24.902 | 114.89 ± 18.130 | 143.24 ± 26.520 | |
LF_Podal axis (°) | 8.692 ± 9.2125 | 6.669 ± 5.7419 | 12.859 ± 13.1551 | |
LF_I-C (mm) | 105.64 ± 23.604 | 97.68 ± 21.360 | 122.04 ± 19.547 | |
RF_lateral lead (%) | 52.498 ± 5.2400 | 51.757 ± 5.9058 | 54.024 ± 3.1192 | |
RF_Maximal pressure (kPa) | 238.465 ± 11.2321 | 237.397 ± 12.3577 | 240.665 ± 8.3658 | |
RF_Area (cm²) | 130.92 ± 28.776 | 119.77 ± 21.855 | 153.88 ± 28.149 | |
RF_Podal axis (°) | 10.442 ± 4.7455 | 9.800 ± 4.5166 | 11.765 ± 5.0665 | |
RF_C-D (mm) | 94.473 ± 16.5183 | 90.189 ± 16.3103 | 103.294 ± 13.4757 | |
Postural asymmetries in the anterior view of the frontal plane | A1 (°) | 0.62 ± .796 | 0.57 ± .850 | 0.71 ± .686 |
A2 (°) | 1.90 ± 2.452 | 2.26 ± 2.737 | 1.18 ± 1.551 | |
A3 (°) | 1.54 ± 1.686 | 1.60 ± 1.818 | 1.41 ± 1.417 | |
A4 (°) | 1.42 ± 1.944 | 1.54 ± 2.241 | 1.18 ± 1.131 | |
A5 (°) | 0.81 ± 1.344 | 0.91 ± 1.541 | 0.59 ± .795 | |
A6 (°) | 0.79 ± 1.273 | 0.94 ± 1.474 | 0.47 ± .624 | |
A7 (°) | 0.81 ± 1.155 | 0.91 ± 1.245 | 0.59 ± .939 | |
A8 (°) | 2.88 ± 2.691 | 2.43 ± 2.404 | 3.82 ± 3.067 | |
A9 (°) | 3.58 ± 3.102 | 3.31 ± 3.085 | 4.12 ± 3.160 | |
A10 (°) | 13.94 ± 7.770 | 12.89 ± 7.673 | 16.12 ± 7.737 | |
A11 (°) | 9.60 ± 7.807 | 9.03 ± 8.234 | 10.76 ± 6.933 | |
Postural asymmetries in the anterior view of the frontal plane | P1 (°) | 0.40 ± .569 | 0.46 ± .611 | 0.29 ± .470 |
P2 (°) | 1.50 ± 1.732 | 1.57 ± 1.945 | 1.35 ± 1.222 | |
P3 (°) | 1.77 ± 1.676 | 1.91 ± 1.805 | 1.47 ± 1.375 | |
P4 (°) | 1.04 ± 1.298 | 1.17 ± 1.317 | 0.76 ± 1.251 | |
P5 (°) | 0.96 ± 1.414 | 1.11 ± 1.623 | 0.65 ± .786 | |
P6 (°) | 0.98 ± 1.196 | 0.83 ± 1.150 | 1.29 ± 1.263 | |
P7 (°) | 1.17 ± 1.354 | 1.14 ± 1.004 | 1.24 ± 1.921 | |
P8 (°) | 4.17 ± 5.346 | 4.43 ± 5.853 | 3.65 ± 4.227 | |
Postural asymmetries in the right lateral of the sagittal plane | R1 (°) | 1.92 ± 1.426 | 1.89 ± 1.430 | 2.00 ± 1.458 |
R2 (°) | 33.83 ± 7.641 | 34.03 ± 8.414 | 33.41 ± 5.948 | |
R3 (°) | 14.94 ± 13.557 | 11.09 ± 7.789 | 22.88 ± 18.904 | |
R4 (°) | 8.42 ± 8.252 | 9.03 ± 8.719 | 7.18 ± 7.282 | |
R5 (°) | 4.73 ± 2.650 | 5.09 ± 3.033 | 4.00 ± 1.414 | |
R6 (°) | 4.42 ± 3.133 | 4.03 ± 2.995 | 5.24 ± 3.345 | |
R7 (°) | 32.85 ± 7.734 | 32.40 ± 7.624 | 33.76 ± 8.113 | |
Postural asymmetries in the left lateral of the sagittal plane | L1 (°) | 2.40 ± 1.636 | 2.11 ± 1.530 | 3.00 ± 1.732 |
L2 (°) | 35.96 ± 7.554 | 35.69 ± 7.892 | 36.53 ± 7.001 | |
L3 (°) | 8.04 ± 6.750 | 6.71 ± 6.926 | 10.76 ± 5.618 | |
L4 (°) | 10.40 ± 6.832 | 11.29 ± 7.303 | 8.59 ± 5.501 | |
L5 (°) | 4.71 ± 2.913 | 4.29 ± 3.073 | 5.59 ± 2.399 | |
L6 (°) | 3.73 ± 3.182 | 3.14 ± 2.724 | 4.94 ± 3.766 | |
L7 (°) | 29.50 ± 7.964 | 28.37 ± 8.229 | 31.82 ± 7.055 |
Variables | F | p | Variables | F | P |
Age (years) | 1.913 | 0.173 | LF_Lateral load (%) | 2.190 | 0.145 |
Height (cm) | 46.028 | < 0.001 | LF_Maximal pressure (kPa) | 1.351 | 0.251 |
Weight (kg) | 19.416 | < 0.001 | LF_Area (cm²) | 20.501 | < 0.001 |
BMI (kg/m²) | 0.268 | 0.607 | LF_Podal axis (°) | 5.636 | 0.021 |
Fat mass (%) | 0.206 | 0.652 | LF_I–C (mm) | 15.701 | < 0.001 |
H2O (%) | 2.356 | 0.131 | RF_lateral lead (%) | 2.190 | 0.145 |
Lean mass (%) | 7.253 | 0.010 | RF_Maximal pressure (kPa) | 0.968 | 0.330 |
Basal metabolism (kcal) | 40.638 | < 0.001 | RF_Area (cm²) | 23.020 | < 0.001 |
Shoe number | 92.731 | < 0.001 | RF_Podal axis (°) | 2.000 | 0.164 |
Pain level | 0.471 | 0.495 | RF_C–D (mm) | 8.223 | 0.006 |
Variable | F | p | Variable | F | p | Variable | F | P |
A1 (°) | 0.322 | 0.573 | P1 (°) | 0.938 | 0.338 | R4 (°) | 0.572 | 0.453 |
A2 (°) | 2.279 | 0.137 | P2 (°) | 0.179 | 0.674 | R5 (°) | 1.956 | 0.168 |
A3 (°) | 0.140 | 0.710 | P3 (°) | 0.799 | 0.376 | R6 (°) | 1.721 | 0.196 |
A4 (°) | 0.402 | 0.529 | P4 (°) | 1.126 | 0.294 | R7 (°) | 0.352 | 0.556 |
A5 (°) | 0.669 | 0.417 | P5 (°) | 1.256 | 0.268 | L1 (°) | 3.519 | 0.067 |
A6 (°) | 1.593 | 0.213 | P6 (°) | 1.759 | 0.191 | L2 (°) | 0.140 | 0.710 |
A7 (°) | 0.910 | 0.345 | P7 (°) | 0.052 | 0.820 | L3 (°) | 4.394 | 0.041 |
A8 (°) | 3.208 | 0.079 | P8 (°) | 0.241 | 0.626 | L4 (°) | 1.812 | 0.184 |
A9 (°) | 0.764 | 0.386 | R1 (°) | 0.072 | 0.789 | L5 (°) | 2.349 | 0.132 |
A10 (°) | 2.019 | 0.162 | R2 (°) | 0.073 | 0.788 | L6 (°) | 3.861 | 0.055 |
A11 (°) | 0.561 | 0.457 | R3 (°) | 10.233 | 0.002 | L7 (°) | 2.200 | 0.144 |
ML model | MSE | RMSE | Pred. (x. ± sd) |
GXBoost | 11.16 | 3.34 | 5 ± 2.43 |
Bayesian regression | 5.09 | 2.25 | 6 ± 1.28 |
Linear regression | 6.22 | 2.49 | 6 ± 1.79 |
Ridge regression | 6.09 | 2.46 | 6 ± 1.75 |
Decision tree regression | 5.45 | 2.34 | 6 ± 1.86 |
Support vector regression | 4.09 | 2.02 | 7 ± 0.98 |
Random forest regression | 7.25 | 2.69 | 6 ± 1.49 |