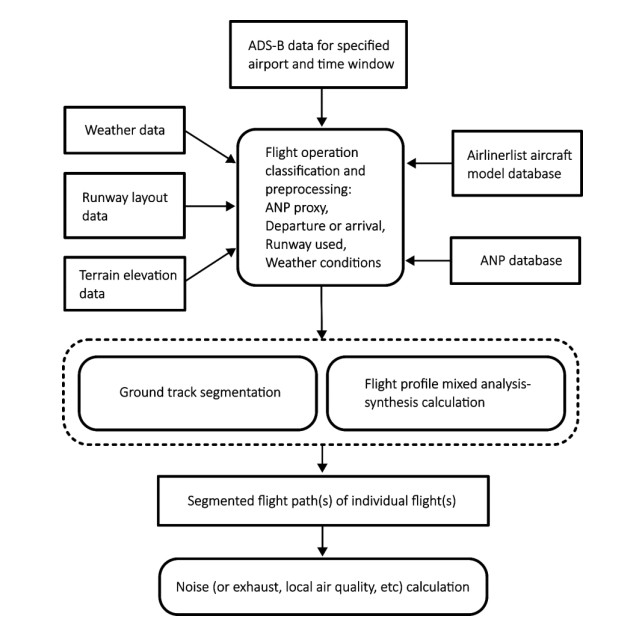
The high density of commercial aviation operations in Europe makes significant contributions to the emission of noise, greenhouse gases, and air pollutants. A key source of information which can be used in efforts to quantify these contributions is the OpenSky Network (OSN), which publishes automatic dependent surveillance - broadcast (ADS-B) data at time resolutions of up to one data point per second. This data can be used to reconstruct ground tracks and flight profiles, which can, in turn, be used to estimate local noise exposure, exhaust emissions, and local air quality. The use of such data in the reconstruction of departure flight paths is limited, however, by the lack of thrust settings and take-off weights. For this reason, a mixed analysis-synthesis approach was developed, in previous research, to reconstruct flight profiles by optimizing published departure procedures parameterized in terms of aircraft thrust settings and take-off weight, and departure procedure parameters. The approach can be used to reconstruct large numbers of flight profiles, throughout significant time windows, from open-source ADS-B data. Errors in the estimations of the parameters can lead to errors in the flight profile calculation which will propagate through to follow-on noise, fuel flow, and emissions calculations. In this paper, a global variance-based sensitivity analysis is presented, which evaluated the sensitivity of departure flight profile altitude to mixed analysis-synthesis flight profile parameters. The purpose was to improve understanding of the dominant sources of error and uncertainty in the flight profile reconstruction, and the influence of aspects of departure flight operations on resulting flight profiles. Analyses were presented for three different airports, Amsterdam Schiphol (EHAM), Dublin (EIDW) and Stockholm (ESSA) airports, considering departures of aircraft corresponding to the 737–800 and A320-211 aircraft classes.
Citation: James H. Page, Lorenzo Dorbolò, Marco Pretto, Alessandro Zanon, Pietro Giannattasio, Michele De Gennaro. Sensitivity analysis of mixed analysis-synthesis flight profile reconstruction[J]. Metascience in Aerospace, 2024, 1(4): 401-415. doi: 10.3934/mina.2024019
[1] | Grégoire Dannet, Olivier Boucher, Nicolas Bellouin . Features and evolution of civil aviation CO2 emissions based on ADS-B data for the period between 2019–2024. Metascience in Aerospace, 2024, 1(4): 346-370. doi: 10.3934/mina.2024016 |
[2] | Weizhen Tang, Jie Dai, Zhousheng Huang . Comprehensive assessment of air pollutant and noise emissions at airports across different altitudes. Metascience in Aerospace, 2024, 1(3): 292-308. doi: 10.3934/mina.2024013 |
[3] | O.N. Korsun, Om Moung Htang . The practical rules for aircraft parameters identification based on flight test data. Metascience in Aerospace, 2024, 1(1): 53-65. doi: 10.3934/mina.2024003 |
[4] | Wenchao Cai, Yadong Zhou . Preventing aircraft from wildlife strikes using trajectory planning based on the enhanced artificial potential field approach. Metascience in Aerospace, 2024, 1(2): 219-245. doi: 10.3934/mina.2024010 |
[5] | Jubilee Prasad Rao, Sai Naveen Chimata . Machine learning-based surrogates for eVTOL performance prediction and design optimization. Metascience in Aerospace, 2024, 1(3): 246-267. doi: 10.3934/mina.2024011 |
[6] | Xu Han, Bin Yu, Hong Liu . Mixing mechanisms in the view of mixing indicators: from passive-scalar mixing to variable-density mixing. Metascience in Aerospace, 2024, 1(1): 1-37. doi: 10.3934/mina.2024001 |
[7] | Igor V. Bezmenov . Fast algorithm for cleaning highly noisy measurement data from outliers, based on the search for the optimal solution with the minimum number of rejected measurement data. Metascience in Aerospace, 2024, 1(1): 110-129. doi: 10.3934/mina.2024005 |
[8] | Dulce M Graciano, Fernando Z Sierra-Espinosa, Juan C García . Numerical simulation of vortex-induced vibration in a bladeless turbine: Effects of separation distance between tandem harvesters. Metascience in Aerospace, 2024, 1(3): 309-328. doi: 10.3934/mina.2024014 |
[9] | Shorob Alam Bhuiyan, Ikram Hossain, Redwan Hossain, Md. Sakib Ibn Mobarak Abir, Dewan Hasan Ahmed . Effect of a bioinspired upstream extended surface profile on flow characteristics and a drag coefficient of a circular cylinder. Metascience in Aerospace, 2024, 1(2): 130-158. doi: 10.3934/mina.2024006 |
[10] | Pasynok Sergey . Cumulative STF coefficients evaluation and validation. Metascience in Aerospace, 2024, 1(4): 371-378. doi: 10.3934/mina.2024017 |
The high density of commercial aviation operations in Europe makes significant contributions to the emission of noise, greenhouse gases, and air pollutants. A key source of information which can be used in efforts to quantify these contributions is the OpenSky Network (OSN), which publishes automatic dependent surveillance - broadcast (ADS-B) data at time resolutions of up to one data point per second. This data can be used to reconstruct ground tracks and flight profiles, which can, in turn, be used to estimate local noise exposure, exhaust emissions, and local air quality. The use of such data in the reconstruction of departure flight paths is limited, however, by the lack of thrust settings and take-off weights. For this reason, a mixed analysis-synthesis approach was developed, in previous research, to reconstruct flight profiles by optimizing published departure procedures parameterized in terms of aircraft thrust settings and take-off weight, and departure procedure parameters. The approach can be used to reconstruct large numbers of flight profiles, throughout significant time windows, from open-source ADS-B data. Errors in the estimations of the parameters can lead to errors in the flight profile calculation which will propagate through to follow-on noise, fuel flow, and emissions calculations. In this paper, a global variance-based sensitivity analysis is presented, which evaluated the sensitivity of departure flight profile altitude to mixed analysis-synthesis flight profile parameters. The purpose was to improve understanding of the dominant sources of error and uncertainty in the flight profile reconstruction, and the influence of aspects of departure flight operations on resulting flight profiles. Analyses were presented for three different airports, Amsterdam Schiphol (EHAM), Dublin (EIDW) and Stockholm (ESSA) airports, considering departures of aircraft corresponding to the 737–800 and A320-211 aircraft classes.
Abbreviations: ADS-B: Automatic Dependent Surveillance – Broadcast; ANP: Aircraft Noise and Performance; CAA: Civil Aviation Authority; ECAC: European Civil Aviation Conference; EHAM: Amsterdam Schiphol airport; EIDW: Dublin airport; ESSA: Stockholm airport; EUROCONTROL: European Organization for the Safety of Air Navigation; FAA: Federal Aviation Authority; FDR: Flight Data Recorder; ICAO: International Civil Aviation Organization; METAR: Meteorological Aerodrome Report; (M)TOW: (Maximum) Take-Off Weight; OSN: (The) OpenSky Network
European commercial air traffic is forecast to reach pre-COVID-19 levels by 2025 and achieve an annual growth of 1.5% until 2029 [1]. With these expected volumes and growth comes associated emission of noise [2], greenhouse gases and air pollutants [3]. The aerospace, aviation and research sectors devote much time and effort to reducing emissions at aircraft technical and air traffic management levels. To understand the real-world impact of such efforts, noise exposure, exhaust emission, and local air quality modeling tools are under continual development and improvement.
A key aspect, well documented in the context of noise exposure modeling, which has been the subject of a number of publications in recent years, is flight path calculation [4,5,6,7]. The flight path of an aircraft is represented by 1) the ground track, which is the projection of the three-dimensional flight path onto the ground, typically expressed in terms of latitude and longitude, and 2) the flight profile, which is the altitude and speed of the aircraft along the ground track [4,5]. The 4th Edition of the European Civil Aviation Conference (ECAC) Doc 29 report on the standard method of calculating noise contours around civil airports provides extensive, detailed guidance on standardized and best-practice methods for noise exposure modeling [4,5]. The documented approach is to construct real, calculated, or default flight paths using data from airports, flight data recorder (FDR), radar, simulation, customized or default procedures, or other sources [4,5]. Ground tracks should consist of straight and circular arc sections and flight profiles of straight sections [4,5]. Noise exposure can then be calculated using data from aircraft databases and aircraft sound emission and propagation modeling techniques [4,5]. This enables calculation of past, hypothetical, and future projected noise exposure levels [4,5]. Noise exposure calculation tools have been developed, based on the ECAC Doc 29 methods and the aircraft noise and performance (ANP) database [8], which provides information on departure procedural step profiles such as 'DEFAULT' and 'ICAO A' and noise power distance data for numerous aircraft. Examples of such tools are the EUROCONTROL IMPACT platform [9], the Federal Aviation Administration (FAA) Aviation Environmental Design Tool (AEDT) [10], and the Civil Aviation Authority (CAA) aircraft noise contour (ANCON) tool [11].
Until recently, obtaining high quality air traffic data was challenging [7]. However, the advent of automatic dependent surveillance – broadcast (ADS-B) in Europe [12] and subsequent advances in the gathering and publishing of ADS-B data has enabled methodology to be developed to reconstruct the ground tracks and flight profiles of large numbers of flights throughout significant time windows, with considerable accuracy, for subsequent noise exposure calculations [6,7,13]. Gagliardi et al. [14] used ADS-B data to analyze the take-off ground run distances of 164 departures from Pisa airport. De Gennaro et al. [13] demonstrated the use of ADS-B data from websites such as Flightradar24 [15], FlightAware [16], and Plane Finder [17], in conjunction with the ANP database [8] and the aircraft model database Airfleets [18] to reconstruct a large number of flights, 34,818, having considered 2,300 airports in EUROCONTROL territory, for noise contour calculation. Pretto et al. used ADS-B data to reconstruct and calculate the near airport noise contours for 10,752 flights [19] and then later used similar methods to forecast future scenarios for airport noise [20]. All three works used published procedural steps to estimate the flight profile [13,19,20]. While researchers have been exploring the use of ABS-B data for reconstruction and analysis of large numbers of flights, methods have been under development and made available, with a prominent example being the development of the Python library 'traffic' [21,22], which provide bespoke implementations of ADS-B data acquisition, preprocessing, analysis, and visualization methods.
While the modeling of noise and other emissions can be carried out on hypothetical flight paths, future scenarios, and statistical dispersions [23], in this paper, the topic is real-world modeling, i.e., reconstruction of single flights, potentially in large numbers. In two recent papers, Pretto et al. developed a mixed analysis-synthesis approach to flight path modeling, whereby published ANP procedures are parameterized and optimized to fit more closely the ADS-B data [6,7]. The method makes use of the ADS-B data provided by the OpenSky Network (OSN) [24] at time resolutions of up to one data point per second. The flight profile reconstruction is still hampered by the joint lack of thrust setting and weight data, which likely leads to errors in the flight profiles that will propagate through to follow-on local noise and fuel flow calculations. For departures, the mixed analysis-synthesis method aims to address this by estimating the thrust levels and the aircraft take-off weight, in addition to three flight profile parameters, which aim to allow realistic flexibility for real-world variation in departure operations [7]. ECAC-based arrival modeling is much more constrained by ANP, which assumes only a DEFAULT procedure flown at fixed landing weight, and the mixed approach can currently account only for kinematic variations. Arrival and departure modeling can, therefore, be considered distinct problems. Accordingly, the departure flight profile is the sole subject of the following analysis.
In this paper, the results of a global variance-based sensitivity analysis are presented, focusing on the sensitivity of departure flight profile altitude to mixed analysis-synthesis aircraft and departure procedure parameters, with the aim of better understanding the dominant sources of error and uncertainty in altitude reconstruction, and which aspects of departure flight operations are most influential on this key aspect of the flight profile. While the departure flight profiles also exhibit other dependencies, for example, on atmospheric and wind conditions, the aim here is to focus on the parameters which are at present estimated via optimization. Analyses are carried out using open-source ADS-B data covering a time window of a week, for three different airports, Amsterdam Schiphol (EHAM), Dublin (EIDW), and Stockholm (ESSA) airports, two aircraft classes, represented by the 737–800 and A320-211 ANP proxy models, and two different departure procedures, DEFAULT and ICAO A. The mixed analysis-synthesis flight profile reconstruction method and global variance-based analysis methodology employed are recalled in some detail in Section 2 'Methodology'. Flight profile reconstructions spanning a full week of departure operations for each airport-proxy-profile combination, corresponding parameter ranges, and corresponding calculated first order Sobol sensitivity indices are presented in Section 3 'Results and analysis'. Finally, discussion of the conclusions drawn from the sensitivity indices are provided in Section 4 'Conclusions'.
The departure flight profile reconstruction method has already been described in detail in references [6],[25], and [7]. The method can be described in terms of two stages: preprocessing and processing. In the first of the three articles, a substantial preprocessing stage is described. In the second article, however, the use of higher time resolution OSN ADS-B data is reported to allow simplification of the preprocessing. The third article focuses on modifications to the flight profile reconstruction method which is based on optimization. The overall procedure is illustrated as a flow diagram in Figure 1.
The preprocessing stage begins with high time resolution ADS-B and Mode S data provided by OSN, containing information including: ICAO24 code, timestamp, latitude, longitude, groundspeed, track, vertical speed, call sign, altitude, geometric altitude, heading, and indicated air speed (IAS) for each individual flight operation. Runway layout and terrain data are used to augment the dataset with the runway used and runway gradient, by matching the ADS-B data with runway layout and terrain data. The ICAO24 code is matched to an aircraft registration using aircraft data provided by OSN. Registration is matched to airframe-engine type using Airlinerlist [26]. Airframe-engine type is matched to an ANP aircraft proxy model using the ANP database [27]. Lastly, meteorological aerodrome reports (METAR) are used with interpolation to calculate atmospheric and wind conditions for the flight. In addition to this, the positional data is smoothed to remove anomalies and reduce noise, and any duplicate flights are removed.
In the processing stage, the ground track is segmented into straight and circular arc sections, maintaining heading and positional continuity, and the straight segment-form flight profiles are calculated using the mixed analysis-synthesis approach [7]. Details on the ground track segmentation are provided in reference [25] and are omitted here as the focus is on flight profile calculation. The mixed analysis-synthesis approach applied to departure operations consists of two main steps: first, several optimization variables (shown in Table 1) are introduced to increase the flexibility of ANP procedural steps so that the resulting synthetic flight profile can better follow the ADS-B data [7]. Second, an objective function, based on the root mean square errors of the altitude and velocity between the analytic, straight segment profile and the ADS-B data is introduced [7]. Lastly, a basin hopping gradient-based optimization routine is used with the aim of minimizing the difference between the analytic and ADS-B flight profiles via objective function minimization [7]. An example of the application of the flight profile reconstruction algorithm to a departure operation is presented in Figure 2, which shows, on the left, the altitude progressions before and after optimization against the guiding ADS-B data and, for context on the right, the reconstructed ground track using only straight segments and circular arcs. The set of parameters, used in conjunction with the atmospheric condition and aircraft proxy data to determine the lengths and gradients of flight profile sections, is shown in Table 1.
Parameter | Symbol | Purpose |
Procedure type | Ptype | Procedural flexibility |
Take-off thrust reduction | KT | Variable take-off thrust |
Climb thrust reduction | KC | Variable climb thrust |
Weight fraction | KMTOW | Variable weight |
Initial climb height | ΔhL | Flexible initial climb section |
Mid-climb height | ΔhM | Flexible mid-climb section(s) |
Energy share fraction | fe | Flexible acceleration step(s) |
The procedure has been shown in [7] to segment flight profiles in a way suitable for aircraft performance reconstruction and airport noise estimation with reasonable matching to ADS-B data in well over 90% of the flight operations, with some limitations only in cases where the ANP flight procedures are not up to date with modern airport traffic management practices.
The methodology employed is the well-established method of global variance-based sensitivity analysis developed by Sobol [28,29,30]. The method is recalled here for completeness. Letting the flight profile altitude or ground speed be denoted by Y, let Y=f(X) denote the model where Y is a function of X=(X1,X2,X3,X4,X5,X6), which are the parameters in Table 1 excluding procedure type. Assume X is a vector of random variables with known distributions. The law of total variance states:
Var(Y)=E[(Y−E[Y])2]=E[Var(Y∣X)]+Var[E(Y∣X)], |
where E[Var(Y∣X)] represents the variance within subgroups defined by different levels of X, and Var[E(Y∣X)] represents the variance due to differences in the expected values of Y across different levels of X. For a model with independent inputs, the variance of the output can be decomposed additively:
Var(Y)=6∑i=1Var(YXi)+∑i≠jCov(YXi,YXj)+⋯+Cov(YX1,…,YX6), |
where YXi is the model output due to the input Xi, and the covariances capture interactions between different inputs. Sobol sensitivity indices extend variance decomposition to measure the contributions of individual inputs and their interactions. First-order Sobol indices Si, given by
Si=Var[E[Y∣Xi]]Var(Y), |
are the proportion of variance in Y directly attributable to the input Xi, while accounting for interactions with other inputs. Total Sobol indices STi, given by
STi=1−Var[E[Y∣X−i]]Var(Y), |
express the total effect of Xi, including interactions with all other input variables X−i.
Sobol indices can be estimated via Monte Carlo simulations and polynomial chaos expansions, or via sampling of the parameter space. For this initial investigation, all mixed analysis-synthesis departure flight profiles were calculated for a week's worth of OSN ADS-B data for each airport-proxy combination on the runway with the greatest number of relevant flights. The ranges of the flight profile parameters were then extracted from the data. Although the time window of a week provided for considerable numbers of departures, no calculations of the probability distributions were carried out. This was reserved for when larger datasets are considered. Therefore, Saltelli sampling [29,30] of the unit hypercube on the uniform distribution, was used to generate parameter samples for variance decomposition. The first and total order Sobol indices were then calculated. Sample sizes of 64,000 were created to give error margins which were small with respect to the variations between the indices. Due to the similarity of the first and total order indices, only the first order indices are presented.
The data obtained for the analysis is summarized here. ADS-B and Mode-S data for aircraft movements at EHAM, EIDW, and ESSA from 2nd March to 8th March inclusive, was downloaded from OSN. The data associated with each movement includes one entry for each transmission, delivered at a time resolution of up to one per second, with each containing the following fields: timestamp, ICAO24 code, latitude, longitude, groundspeed, track, vertical speed, call sign, altitude, geometric altitude, heading, IAS, Mach number, selected mode control panel (MCP), roll angle, rate of change of track, true airspeed (TAS), and flight ID. Airport atmospheric temperature and pressure as well as wind direction and wind speed data were extracted from METARs, obtained using Traffic. For each flight the data was linearly interpolated between the two METARs for the relevant airport just before and just after the first and last ADS-B timestamps associated with the movement. Airport runway data was provided by a database obtained from OurAirports [31] and taxiway layout data from OpenStreetMap [32]. Aircraft registration data linked to ICAO24 data was provided by the aircraft database downloaded from OSN. Airframe and engine data linked to aircraft registrations was provided by Airlinerlist [26], while the ANP proxy model data was provided by the ANP database [27].
Flight profiles from 2nd March to 8th March, inclusive, were reconstructed for each of EHAM, EIDW, and ESSA airports, using the mixed analysis-synthesis approach briefly described in Section 2.1. The ranges of each of the six parameters were extracted from the data for the aircraft represented by the proxies A320-200 and 737–800 at each airport separately. The ranges were used to bound the sampling for global sensitivity analyses for each aircraft proxy – airport combination.
Figure 3 to Figure 5 show the range of calculated flight profiles of 737–800 proxy represented aircraft departing from EHAM runway 36L, EIDW runway 28L and ESSA runway 01L, respectively. The results reveal significant ranges of altitudes achieved by the aircraft over the week at ground track distances of 10,000' (1.6 nm), 20,000' (3.3 nm), 40,000' (6.6 nm), 60,000' (9.9 nm), and 80,000' (13.2 nm). Both the DEFAULT and ICAO A departure profiles are shown. The mixed analysis-synthesis parameter ranges for the departure events at the same locations for the 737–800 proxy-represented aircraft using the DEFAULT and ICAO A profiles in the timeframe are shown in Table 2 and Table 3, respectively.
KT | KMTOW | ΔhL | fe | ΔhM | KC | |||||||
Min | Max | Min | Max | Min | Max | Min | Max | Min | Max | Min | Max | |
EHAM | 0.75 | 1.0 | 128472 | 174200 | −200 | 750 | 0.7 | 1.4 | 0 | 3000 | 0.8 | 1.0 |
EIDW | 0.75 | 1.0 | 124403 | 169750 | −200 | 750 | 0.7 | 1.4 | -500 | 5000 | 0.8 | 1.0 |
ESSA | 0.75 | 1.0 | 112823 | 174200 | −200 | 750 | 0.7 | 1.4 | -500 | 5000 | 0.8 | 1.0 |
KT | KMTOW | ΔhL | fe | ΔhM | KC | |||||||
Min | Max | Min | Max | Min | Max | Min | Max | Min | Max | Min | Max | |
EHAM | 0.75 | 1.0 | 133241 | 174200 | −2000 | 121 | 0.55 | 1.4 | 0 | 3000 | 0.8 | 1.0 |
EIDW | 0.75 | 1.0 | 124325 | 158842 | −2000 | 143 | 0.55 | 1.4 | −500 | 5000 | 0.8 | 1.0 |
ESSA | 0.75 | 1.0 | 114355 | 174012 | −2000 | 750 | 0.55 | 1.4 | −500 | 5000 | 0.8 | 1.0 |
Figure 6 to Figure 7 show the first order Sobol indices for each of the parameters at 1.6 nm, 3.3 nm, 6.6 nm, 9.9 nm, and 13.2 nm for the 737–800 and A320-211 proxy represented aircraft departing EHAM, EIDW, and ESSA using the DEFAULT flight profile. The overall patterns are similar between the two aircraft and airports. The results for the 737–800 in Figure 6 show that KMTOW is consistently the greatest contributor to altitude variance across the three airports, throughout the range of surface distances. However, there are some notable contributions from other parameters which vary with the surface distance. At 1.6 nm, the take-off thrust reduction parameter, KT, variance makes a sizeable contribution to the altitude variance. This is unsurprising as the remaining parameters have little connection to the flight profile mathematically at this distance. At 3.3 nm, KT makes a similar contribution. Here the ΔhL and fe parameters make a small noticeable contribution and their greatest contribution for this proxy and profile. At 6.6 nm, KT maintains minor influence and ΔhM emerges as a moderately contributing parameter. Here KMTOW is at its minimum due to the contributions of the aforementioned parameters. At 9.9 nm, KT and ΔhM maintain minor shares of the variance contribution and KC gains a small contribution. At 13.2 nm, the KT and ΔhM shares of the contribution are moderately smaller and the KC contribution has increased. The fe and ΔhL contributions remain small throughout. The results for the A320-211 in Figure 7 show some differences to those of the 737–800. Most notably, the fe and ΔhL contributions are of comparable size to that of KT and correspond to a noticeably reduced contribution from KMTOW particularly at EIDW. At 9.9 nm and 13.2 nm, KT and ΔhM each make much less of a contribution share.
Figure 8 and Figure 9 show the first order Sobol indices for each of the parameters at 1.6 nm, 3.3 nm, 6.6 nm, 9.9 nm, and 13.2 nm for the 737–800 and A320-211 proxy represented aircraft departing EHAM, EIDW, and ESSA using the ICAO A flight profile. Similarly to the DEFAULT profile results, the 737–800 indices in Figure 8 show that KMTOW is consistently high throughout. However, the contribution share of KT tails off at 3.3 nm and above, while ΔhL has a significant share of the variance contribution at 3.3 nm, particularly for EIDW and EHAM airports. At 6.6 nm and above, KC makes a noticeable contribution, increasing with surface distance and becoming comparable to that of KMTOW at 13.2 nm. The A320-211 proxy results in Figure 9 show a similar pattern to those of the 737–800 for this profile. However, at 3.3 nm, the share of ΔhL is noticeably greater, at the expense of and reaching approximately the same magnitude as KMTOW for the three airports. At 6.6 nm, the contribution of ΔhL persists but is reduced, while that of KMTOW is again increased. The extent of this is only slight for EIDW, but greater for ESSA and greater still for EHAM. At 9.9 and 13.2 nm, the pattern is similar to that of the 737–800, with the major contribution coming from KMTOW and a minor contribution from KC as with all the previous results.
In this paper, a global, variance-based sensitivity analysis of the mixed analysis-synthesis approach for flight profile reconstruction and segmentation [7] has been introduced and presented. The adopted flight profile reconstruction method has been recalled in Section 2.1 and the methodology for sensitivity analysis has been laid out in Section 2.2. The results have been described in detail in Section 3 and are discussed here.
The analysis focused on assessing the contributions of the six mixed analysis-synthesis [7] parameters KT, KMTOW, fe, ΔhL, ΔhL, and KC to altitude variance at specified surface distances along the ground track. Other factors, namely profile, runway, and weather specifics, were kept constant for each sensitivity calculation. The main observations across the considered airports, proxies and profiles can be summarized as follows: KMTOW variance is, by some margin, consistently the greatest contributor to altitude total variance throughout the considered ground track surface distance range; and KT and KC variances make similar and next greatest contributions to total variance, with KT more influential at lower and KC at greater distances. Out of the total six, these three parameters represent take-off weight, take-off thrust reduction and climb thrust reduction respectively, which represent the aircraft performance. Thus, it can be concluded that, in this study on mixed analysis-synthesis departure flight profile reconstruction, aircraft performance is collectively the most important contributor to altitude total variance over the ground track range considered. The remaining three parameters fe, ΔhL, and ΔhM are those of the flight profile geometry. While collectively making a less significant contribution to altitude total variance, the flight profile parameters fe and ΔhL do make notable contributions at certain ground track distance subranges. At the intermediate surface distances (3.3–6.6 nm), there are particularly notable contributions with the specifics varying with flight profile and proxy. The parameter ΔhL contributes to altitude total variance at 3.3 nm with the ICAO A profile of comparable magnitude to that of KMTOW at the same point, to that of KC at any other point in this analysis, and, in excess of that of KT, anywhere in this analysis.
The implication of these observations is that calculated aircraft performance has greater potential to be a source of inaccuracy in departure flight profile calculation than flight profile geometry with the current mixed analysis-synthesis approach. Greater accuracy and robustness of the approach is, therefore, suggested to be sought by finding ways to access data on aircraft performance factors and improve methods of their deduction from available flight data. The parameterization of the flight profiles is, however, also a consideration, albeit of secondary priority. Follow-up research will address the questions of specific parameter probability distribution, local sensitivities of specific flight profiles and how flight profile sensitivity translates into calculated noise and local pollutant emission sensitivity. Beyond that, further analysis may be carried out including more aspects of flight profile calculation, such as weather conditions. Similar analyses may also be applied to arrivals, but it is possible that advancements in the ECAC- and ANP-based profile modeling are necessary beforehand to lower the level of constraint in the computations and unlock additional degrees of freedom.
The authors are grateful to the European Commission for their support of this research, carried out as part of the NEEDED project, which was funded by the European Climate, Infrastructure and Environment Executive Agency (Grant Agreement no. 101095754). This publication solely reflects the authors' view and neither the EU, nor the Funding Agency can be held responsible for the information it contains.
The authors declare they have not used Artificial Intelligence (AI) tools in the creation of this article.
The authors declare no conflict of interest.
[1] | EUROCONTROL (2023) EUROCONTROL seven year forecast 2023-2029. Available from: https://www.eurocontrol.int/sites/default/files/2023-10/eurocontrol-seven-year-forecast-2023-2029-region-definition.pdf. |
[2] | ICAO (2023) Global trends in aircraft noise. |
[3] | European Commission (2024) Reducing emissions from aviation. Available: https://climate.ec.europa.eu/eu-action/transport/reducing-emissions-aviation_en. |
[4] | ECAC.CEAC (2025) Report on Standard Method of Computing Noise Contours around Civil Airports Volume 1: Applications Guide. |
[5] | ECAC.CEAC (2016) Report on Standard Method of Computing Noise Contours around Civil Airports Volume 2: Technical Guide. |
[6] |
Pretto M, Giannattasio P, De Gennaro M (2022) Mixed analysis-synthesis approach for estimating airport noise from civil air traffic. Transport Res D-Tr E 106: 103248. https://doi.org/10.1016/j.trd.2022.103248 doi: 10.1016/j.trd.2022.103248
![]() |
[7] |
Pretto M, Dorbolò L, Giannattasio P, et al. (2024) Aircraft trajectory reconstruction and airport noise prediction from high-resolution flight tracking data. Transport Res D-Tr E 135: 104397. https://doi.org/10.1016/j.trd.2024.104397 doi: 10.1016/j.trd.2024.104397
![]() |
[8] | EUROCONTROL (2023) The aircraft noise and performance database (ANP) database: an international data resource for aircraft noise modellers. |
[9] | EUROCONTROL (2024) IMPACT: integrated aircraft noise and emissions modelling platform. |
[10] | Federal Aviation Administration (FAA) Aviation Environmental Design Tool (AEDT). Available from: https://aedt.faa.gov/. |
[11] | Ollerhead JB (1992) The CAA Aircraft Noise Contour Model: ANCON Version 1, Cheltenham, UK: Civil Aviation Authority. |
[12] | Rekkas C, Rees M (2008) Towards ADS-B implementation in Europe. in 2008 Tyrrhenian International Workshop on Digital Communications - Enhanced Surveillance of Aircraft and Vehicles, Capri, Italy. https://doi.org/10.1109/TIWDC.2008.4649019 |
[13] | De Gennaro M, Zanon A, Kuehnelt H, et al. (2018) Big data for low-carbon transport: an overview of applications for designing the future of road and aerial transport, in 7th Transport Research Arena, Vienna. |
[14] | Gagliardi P, Fredianelli L, Simonetti D, et al. (2017) ADS-B System as a Useful Tool for Testing and Redrawing Noise Management Strategies at Pisa Airport. Acta Acust United Acust 103: 543–551. |
[15] | Flightradar24. Available from: https://www.flightradar24. |
[16] | FlightAware. Available from: https://www.flightaware.com. |
[17] | Plane Finder. Available from: https://planefinder.net/. |
[18] | Airfleets.net. Available from: https://www.airfleets.net. |
[19] |
Pretto M, Giannattasio P, De Gennaro M, et al. (2019) Web data for computing real-world noise from civil aviation. Transport Res D-Tr E 69: 224–249. https://doi.org/10.1016/j.trd.2019.01.022 doi: 10.1016/j.trd.2019.01.022
![]() |
[20] |
Pretto M, Giannattasio P, De Gennaro M, et al (2020) Forecasts of future scenarios for airport noise based on collection and processing of web data. Eur Transp Res Rev 12: 4. https://doi.org/10.1186/s12544-019-0389-x doi: 10.1186/s12544-019-0389-x
![]() |
[21] | Olive X (2019) Traffic: air traffic data processing with Python. |
[22] |
Olive X (2019) Traffic, a toolbox for processing and analysing air traffic data. J Open Source Softw 4: 1518, 1–3. https://doi.org/10.21105/joss.01518 doi: 10.21105/joss.01518
![]() |
[23] |
Sun J, Ellerbroek J, Hoekstra JM (2019) WRAP: An open-source kinematic aircraft performance model. Transport Res C-Emer 98: 118–138. https://doi.org/10.1016/j.trc.2018.11.009 doi: 10.1016/j.trc.2018.11.009
![]() |
[24] | Schä fer M, Strohmeier M, Lenders V, et al. (2014) Bringing up OpenSky: A large-scale ADS-B sensor network for research, in IPSN-14 Proceedings of the 13th International Symposium on Information Processing in Sensor Networks, Berlin, Germany, 83–94. https://doi.org/10.1109/IPSN.2014.6846743 |
[25] |
Pretto M, Dorbolò L, Giannattasio P (2023) (Poster) Exploiting high-resolution ADS-B data for flight operation reconstruction towards environmental impact assessment. J Open Aviat Sci 1. https://doi.org/10.59490/joas.2023.7208 doi: 10.59490/joas.2023.7208
![]() |
[26] | Airlinerlist. Available from: https://www.planelist.net/. |
[27] | EASA – ANP (2023) Aircraft Noise and Performance (ANP) Data. |
[28] |
Sobol IM (2001) Global sensitivity indices for nonlinear mathematical models and their Monte Carlo estimates. Math Comput Simulat 55: 271–280. https://doi.org/10.1016/S0378-4754(00)00270-6 doi: 10.1016/S0378-4754(00)00270-6
![]() |
[29] |
Saltelli A (2002) Making best use of model evaluations to compute sensitivity indices. Comput Phys Commun 145: 280–297. https://doi.org/10.1016/S0010-4655(02)00280-1 doi: 10.1016/S0010-4655(02)00280-1
![]() |
[30] | Campolongo F, Saltelli A, Cariboni J (2011) From screening to quantitative sensitivity analysis. A unified approach. Comput Phys Commun 182: 978–988. |
[31] | OurAirports. Available from: https://ourairports.com/. |
[32] | OpenStreetMap. Available from: https://www.openstreetmap.org. |
Parameter | Symbol | Purpose |
Procedure type | Ptype | Procedural flexibility |
Take-off thrust reduction | KT | Variable take-off thrust |
Climb thrust reduction | KC | Variable climb thrust |
Weight fraction | KMTOW | Variable weight |
Initial climb height | ΔhL | Flexible initial climb section |
Mid-climb height | ΔhM | Flexible mid-climb section(s) |
Energy share fraction | fe | Flexible acceleration step(s) |
KT | KMTOW | ΔhL | fe | ΔhM | KC | |||||||
Min | Max | Min | Max | Min | Max | Min | Max | Min | Max | Min | Max | |
EHAM | 0.75 | 1.0 | 128472 | 174200 | −200 | 750 | 0.7 | 1.4 | 0 | 3000 | 0.8 | 1.0 |
EIDW | 0.75 | 1.0 | 124403 | 169750 | −200 | 750 | 0.7 | 1.4 | -500 | 5000 | 0.8 | 1.0 |
ESSA | 0.75 | 1.0 | 112823 | 174200 | −200 | 750 | 0.7 | 1.4 | -500 | 5000 | 0.8 | 1.0 |
KT | KMTOW | ΔhL | fe | ΔhM | KC | |||||||
Min | Max | Min | Max | Min | Max | Min | Max | Min | Max | Min | Max | |
EHAM | 0.75 | 1.0 | 133241 | 174200 | −2000 | 121 | 0.55 | 1.4 | 0 | 3000 | 0.8 | 1.0 |
EIDW | 0.75 | 1.0 | 124325 | 158842 | −2000 | 143 | 0.55 | 1.4 | −500 | 5000 | 0.8 | 1.0 |
ESSA | 0.75 | 1.0 | 114355 | 174012 | −2000 | 750 | 0.55 | 1.4 | −500 | 5000 | 0.8 | 1.0 |
Parameter | Symbol | Purpose |
Procedure type | Ptype | Procedural flexibility |
Take-off thrust reduction | KT | Variable take-off thrust |
Climb thrust reduction | KC | Variable climb thrust |
Weight fraction | KMTOW | Variable weight |
Initial climb height | ΔhL | Flexible initial climb section |
Mid-climb height | ΔhM | Flexible mid-climb section(s) |
Energy share fraction | fe | Flexible acceleration step(s) |
KT | KMTOW | ΔhL | fe | ΔhM | KC | |||||||
Min | Max | Min | Max | Min | Max | Min | Max | Min | Max | Min | Max | |
EHAM | 0.75 | 1.0 | 128472 | 174200 | −200 | 750 | 0.7 | 1.4 | 0 | 3000 | 0.8 | 1.0 |
EIDW | 0.75 | 1.0 | 124403 | 169750 | −200 | 750 | 0.7 | 1.4 | -500 | 5000 | 0.8 | 1.0 |
ESSA | 0.75 | 1.0 | 112823 | 174200 | −200 | 750 | 0.7 | 1.4 | -500 | 5000 | 0.8 | 1.0 |
KT | KMTOW | ΔhL | fe | ΔhM | KC | |||||||
Min | Max | Min | Max | Min | Max | Min | Max | Min | Max | Min | Max | |
EHAM | 0.75 | 1.0 | 133241 | 174200 | −2000 | 121 | 0.55 | 1.4 | 0 | 3000 | 0.8 | 1.0 |
EIDW | 0.75 | 1.0 | 124325 | 158842 | −2000 | 143 | 0.55 | 1.4 | −500 | 5000 | 0.8 | 1.0 |
ESSA | 0.75 | 1.0 | 114355 | 174012 | −2000 | 750 | 0.55 | 1.4 | −500 | 5000 | 0.8 | 1.0 |